- 1Genomic and Applied Microbiology and Göttingen Genomics Laboratory, Institute of Microbiology and Genetics, Georg-August-University, Göttingen, Germany
- 2Leibniz Institute DSMZ-German Collection of Microorganisms and Cell Cultures, Braunschweig, Germany
- 3German Center for Infection Research (DZIF), Partner Site Braunschweig-Hannover, Braunschweig, Germany
- 4Institute of Microbiology, Technische Universität Braunschweig, Braunschweig, Germany
The pathogenic bacterium Clostridioides difficile is a worldwide health burden with increasing morbidity, mortality and antibiotic resistances. Therefore, extensive research efforts are made to unravel its virulence and dissemination. One crucial aspect for C. difficile is its mobilome, which for instance allows the spread of antibiotic resistance genes (ARG) or influence strain virulence. As a nosocomial pathogen, the majority of strains analyzed originated from clinical environments and infected individuals. Nevertheless, C. difficile can also be present in human intestines without disease development or occur in diverse environmental habitats such as puddle water and soil, from which several strains could already be isolated. We therefore performed comprehensive genome comparisons of closely related clinical and non-clinical strains to identify the effects of the clinical background. Analyses included the prediction of virulence factors, ARGs, mobile genetic elements (MGEs), and detailed examinations of the pan genome. Clinical-related trends were thereby observed. While no significant differences were identified in fundamental C. difficile virulence factors, the clinical strains carried more ARGs and MGEs, and possessed a larger accessory genome. Detailed inspection of accessory genes revealed higher abundance of genes with unknown function, transcription-associated, or recombination-related activity. Accessory genes of these functions were already highlighted in other studies in association with higher strain virulence. This specific trend might allow the strains to react more efficiently on changing environmental conditions in the human host such as emerging stress factors, and potentially increase strain survival, colonization, and strain virulence. These findings indicated an adaptation of the strains to the clinical environment. Further, implementation of the analysis results in pairwise genome comparisons revealed that the majority of these accessory genes were encoded on predicted MGEs, shedding further light on the mobile genome of C. difficile. We therefore encourage the inclusion of non-clinical strains in comparative analyses.
1 Introduction
The bacterium Clostridioides difficile is a globally widespread pathogen that constitutes a major cause of nosocomial and antibiotic-associated infections, with disease severity ranging from mild diarrhea to pseudomembranous colitis, eventually leading to death (Balsells et al., 2019). A C. difficile infection is mainly elicited after antibiotic treatment and increasing antibiotic resistances in this species impede successful treatment of an infection (Spigaglia, 2016). C. difficile is extensively studied, especially in the context of increasing multi-drug resistances, but also concerning its virulence heterogeneity. C. difficile strains can extremely vary in the induced symptoms, and even non-toxigenic strains without disease-causing toxins exist (Czepiel et al., 2019). Research on C. difficile virulence already pointed towards the importance of mobile genetic elements (MGE). About 11% of a C. difficile genome is composed of MGEs, including plasmids, bacteriophages, IS elements, and conjugative and mobilizable transposons (Sebaihia et al., 2006; Mullany et al., 2015). Plasmids can contribute to virulence by carrying toxin genes or promoting antibiotic resistances (Smits et al., 2022), and also bacteriophages can influence C. difficile virulence (Govind et al., 2009; Sekulovic et al., 2011; Goh et al., 2013; Riedel et al., 2017b; Mehner-Breitfeld et al., 2018). MGEs are especially crucial for horizontal gene transfer that allows fast adaptation to environmental conditions, e.g., spreading genes conferring antibiotic resistances between different strains or even species (de la Cruz and Davies, 2000). In addition, the pathogenicity locus of C. difficile, which encodes the C. difficile-typical toxin genes, exhibits a mobile character and can transfer to a previously non-toxigenic strain (Brouwer et al., 2013). Although the toxin genes represent the major virulence factors of C. difficile, their contribution to overall virulence is still under debate, and other aspects such as tolerance to secondary bile acids or specific accessory genes rather correlated with disease severity (Lewis et al., 2017).
As a prominent pathogen with increasing morbidity and mortality, most of the analyzed C. difficile strains originate from clinical specimen of infected individuals. However, C. difficile was also found in asymptomatic, healthy individuals, and is also a natural inhabitant of various animal species and environmental reservoirs (Ozaki et al., 2004; Janezic et al., 2016; Weese, 2020). Although several C. difficile strains were isolated from diverse environmental sources in recent years, genome-based comparisons always comprised strains associated with infection (“clinical”) or only worked on draft genomes and also rather focused on epidemiological studies (Knight et al., 2017; Xu et al., 2021; Zhou et al., 2021; Dong et al., 2023). Zhou et al. (2021) compared strains of environmental or clinical origin and could not observe a connection to the isolation source. However, their analyses did not comprise pan-genomic studies but focused on virulence factors and antibiotic resistance genes (ARG). Comprehensive pan-genomic analyses specifically comparing clinical and non-clinical strains have not been conducted, yet.
In this study, we performed genomic analyses between high quality genomes of C. difficile strains originating from environmental samples (non-clinical background) and from infected humans (clinical reference strains). Analyses were compared between clinical and non-clinical strain corresponding in their sequence type. We focused on MGEs and potentially linked genes encoding antibiotic resistances or virulence factors, and conducted a pan genome analysis. All these analyses were put into genomic context through direct genome comparisons of the corresponding clinical and non-clinical strains. We detected genomic differences that were linked to clinical background and might reflect increased physiological adaptation ability.
2 Methods
2.1 Strain isolation and cultivation
Non-clinical C. difficile strains were isolated from horse feces, biogas fermenter sludge and mud. The environmental samples were collected between November 2019 and July 2020 with sterile canonical falcon tubes and were stored at 4°C upon arrival in the laboratory. We opted for antibiotic-free isolation to overcome a potential isolation bias (Schüler et al., 2023). Different antibiotic-free and antibiotic-based isolation approaches were therefore employed. As a result, strains J2_1 and TS3_3 were isolated without antibiotics, whereas MA_1 and B1_2 originated from isolation approaches with moxalactam norfloxacin (CDMN, Oxoid Deutschland GmbH, Wesel, Germany). Details of isolation protocols are described in Supplementary Presentation 1. In general, environmental samples were dissolved in anoxic PBS (pH 7.4) [Phosphate-buffered saline (PBS), 2006] and pasteurized before inoculating the enrichment media. Grown enrichment cultures were plated on solid media with 1.5% agar and colonies examined for identity via 16S rRNA gene Sanger sequencing using colony PCR with Phusion High-Fidelity polymerase (Thermo Fisher Scientific, Waltham, MA, USA) and primers 08f (5′-AGAGTTTGATCCTGGC-3′) and 1504r (5′-TACCTTGTTACGACTT-3′), following the recommendations of the manufacturer. PCR products were purified with the QIAquick PCR Purification kit (Qiagen, Hilden, Germany) as recommended by the manufacturer and subjected to Sanger sequencing by Microsynth Seqlab GmbH (Göttingen, Germany).
Clinical reference strains of sequence types (ST)/ribotypes (RT) corresponding to the four non-clinical strains were kindly provided by the Institute of Medical Microbiology, Göttingen, Germany. Strains DSM 28196, DSM 29747, SC083-01-01, and SC084-01-01 had been isolated from infected humans as described in Riedel et al. (2017a).
Isolates were routinely cultivated at 37°C under anoxic conditions in supplemented Brain Heart Infusion Broth (BHIS; supplemented with 0.5% yeast extract, 0.05% L-cysteine, 0.0001% Na-resazurin, purged with nitrogen).
2.2 DNA extraction
Genomic DNA was extracted from overnight cultures using the MasterPure Gram Positive DNA Purification kit as recommended by the manufacturer (Epicentre, Madison, WI, United States). DNA quality was assessed on a NanoDrop ND-1000 (Peqlab Biotechnologie GmbH, Erlangen, Germany), and DNA concentration was measured using the Qubit 3.0 Fluorometer (Thermo Fisher Scientific) with the BR dsDNA assay kit.
2.3 Ribotyping of Clostridioides difficile isolates
Isolated strains were phylogenetically examined via ribotyping based on Bidet et al. (1999). Amplification of the 16S-23S rRNA intergenic spacer region was conducted with the Dreamtaq polymerase (Thermo Fisher Scientific) using reagents as recommended by the manufacturer with 0.2 mM of each primer and 50 ng template DNA per 50 μL PCR reaction. PCR cycling comprised initial denaturation at 95°C for 3 min, followed by 30 cycles of 95°C for 1 min, 56°C for 30 s, and 72°C for 1 min. Final elongation was performed at 72°C for 5 min. PCR products were separated on a 2% agarose gel ran at 5 V/cm with subsequent staining using ethidium bromide and visualization with the AlphaImager HP (Alpha Innotech Corp., San Leandro, USA) and AlphaView Software (v3.5.0). For RT assignment, observed band patterns were compared to already known RTs.
2.4 Genome sequencing, assembly, and annotation
For whole-genome sequencing of the non-clinical isolates, genomic DNA was subjected to short-read and long-read sequencing using Illumina and Oxford Nanopore technology, respectively. Illumina sequencing libraries were prepared with the Nextera XT DNA sample preparation kit and sequenced using a MiSeq instrument and reagent kit v3 (2 × 300 bp, 600 cycles) as recommended by the manufacturer (Illumina, San Diego, CA, USA). For Nanopore sequencing, genomic DNA without specific size selection was processed using the ligation sequencing kit 1D (SQK-LSK109) and the native barcode expansion kit (EXP-NBD104) according to the manufacturer’s specifications (Oxford Nanopore Technologies, Oxford, United Kingdom). Nanopore sequencing was performed with the MinION system using a SpotON flow cell Mk I (R9.4.0) for 72 h. All following software was used with default settings unless otherwise stated. The MinKNOW software (v19.12.5) with implemented Guppy (v3.2.10) was used in fast mode for demultiplexing and base calling. Nanopore reads were first trimmed using Porechop (v0.2.4)1 and filtered with Filtlong (v0.2.1),2 following assembly with Flye (v2.9.2) (Kolmogorov et al., 2019). Illumina reads were processed with fastp (v0.23.3) (Chen, 2023) and trimmed using Trimmomatic (v0.39) (Bolger et al., 2014). The long-read assembly was polished with the processed short reads using softwares BWA (v0.7.17, r1188) (Li and Durbin, 2010) and Polypolish (v0.5.0) (Wick and Holt, 2022). Circularization of the assemblies were verified with Bandage v0.8.1 (Wick et al., 2015) and assemblies rotated with Circlator (v1.5.5) (Hunt et al., 2015) to begin with the gene dnaA. The assembled genome sequences were annotated with Prokka (v1.14.5) (Seemann, 2014). Selenoproteins were curated manually.
Genome sequencing and assembly of the clinical reference strains was done by Leibniz Institute DSMZ-German Collection of Microorganisms and Cell Cultures, Braunschweig, Germany. High molecular weight DNA was prepared using the Qiagen Genomic Tip/100 G kit (Qiagen, Hilden, Germany). SMRTbell template libraries were prepared according to the instructions from Pacific Biosciences, Menlo Park, CA, United States, following the Procedure & Checklist - 20 kb Template Preparation Using BluePippin Size-Selection System. Briefly, for preparation of 15 kb libraries 5 μg genomic DNA were end-repaired and ligated overnight to hairpin adapters applying components from the DNA/Polymerase Binding Kit P6 from Pacific Biosciences, Menlo Park, CA, United States. Reactions were carried out according to the manufacturer’s instructions. BluePippin Size-Selection was performed according to the manufacturer’s instructions (Sage Science, Beverly, MA, United States). Conditions for annealing of sequencing primers and binding of polymerase to purified SMRTbell template were assessed with the Calculator in RS Remote, PacificBiosciences, Menlo Park, CA, United States. SMRT sequencing was carried out on the PacBio RSII (PacificBiosciences, Menlo Park, CA, United States) taking 240-min movies. Long read genome assembly was performed with the “RS_HGAP_Assembly.3 “protocol included in SMRTPortal (v2.3.0) using default parameters. Chromosomal contigs and plasmids were circularized, particularly artificial redundancies at the ends of the contigs were removed and adjusted to dnaA. Identification of redundancies and the replication genes has been done based on BLAST, circularization and rotation to the replication genes has been performed by genomecirculator.jar tool.3 Error-correction was performed by a mapping of Illumina short reads onto finished genome using BWA (v0.6.2) in paired-end (sampe) mode using default settings (Li and Durbin, 2010) with subsequent variant and consensus calling using VarScan (v2.3.6) (Koboldt et al., 2012).
2.5 Genomic analyses
In general, plots were created with RStudio (v2022.06.0) (RStudio Team, 2020) using the package ggplot2 (v3.4.2) (Wickham, 2016), and final modifications were done with Inkscape (v0.48).4
MLST assignment of the non-clinical strains was done using PubMLST (Jolley et al., 2018). Genome qualities were assessed with CheckM2 (v1.0.2) (Chklovski et al., 2023) before performing genome analyses.
The program antiSMASH (v7.0.0) (Blin et al., 2023) was used for predicting secondary metabolite biosynthetic gene clusters. Putative ARGs were identified with RGI (v6.0.2), CARD (v3.2.7) (Alcock et al., 2023), and AMRFinderPlus (v3.11.14) (Feldgarden et al., 2021) employing the NCBI Bacterial Antimicrobial Resistance Reference Gene Database (v2023-07-13.2).
Screening for virulence factors was performed by BLAST+ blastp analysis (v2.12.0) (Camacho et al., 2009) (options: -num_alignment 1; −outfmt “6 delim=, qaccver saccver pident length evalue qcovs qcovhsp bitscore”) using the C. difficile-associated protein sequences present in the full dataset (retrieved on 14.07.2023) from the virulence factor database (VFDB (Liu et al., 2022)) as query against the whole-genome protein sequences of the analyzed strains. The spo0A sporulation gene from C. difficile strain 630 was additionally included in the analysis (CP010905.2, CDIF630_01363). Protein sequences of each virulence factor between corresponding genomes were additionally compared with blastp (Camacho et al., 2009) (options like above) to check for sequence deviations.
Presence/absence of ARGs and protein sequence query coverage /percentage identity of virulence factors was visualized as heatmaps.
After initial assessment of toxin gene presence with the aforementioned VFDB analysis, the corresponding toxin-operons and adjacent genes were inspected for nucleotide sequence similarity and genomic location by sequence alignment with clinker (v1.32) (Gilchrist and Chooi, 2021), including reference sequences from C. difficile strain 630 (CP010905.2, CDIF630_00771–00782) and R20291 (CP029423.1, CDIF27147_02765–02770), respectively.
2.5.1 Analysis of MGEs
Genomes were analyzed with PlasmidFinder (v2.1) (Carattoli et al., 2014) for plasmid family identification. Insertion sequences (IS) were identified with ISEScan (v1.7.2.3) (Xie and Tang, 2017). Genomic islands (GI) were predicted with various tools, including PHASTEST in deep mode (on 25.6.23) (Wishart et al., 2023) for prophage prediction, IslandViewer 4 (accessed on 4.7.23) (Bertelli et al., 2017) and ICEscreen (v1.2.0) (Lao et al., 2022). The numbers of identified MGEs and their types were visualized as heatmaps.
2.5.2 Pan genome analysis
The core/pan genome including all genomes was calculated with Roary (v3.13.0) (Page et al., 2015), and a Venn diagram visualizing the results was created with Inkscape (v0.48)5 Venn diagrams showing the shared and unique genes for each pair of non-clinical and corresponding clinical strain as estimated by Roary were visualized with ggplot2. The unique genes were assigned to functional clusters of orthologous groups of proteins (COG) with eggNOG-mapper (v2.1.9) (Cantalapiedra et al., 2021). Relative abundance of unique genes of a specified COG was determined for each genome relative to its total number of coding sequences (CDS) and visualized as bar charts. Further, the differences between clinical and non-clinical strain of these relative COG-gene abundances were calculated by subtracting the relative values of the non-clinical from the clinical strain. These difference values were also plotted.
2.5.3 Pairwise genome alignment and comparison
For direct genome comparison, genomes of non-clinical and corresponding clinical reference strains were first aligned with Mauve (v20150226) (Darling et al., 2004) and inspected for significant sequence deviations detected as alignment gaps over multiple CDSs. These CDSs were inspected for their predicted function and compared to the previous pan genome analysis. Further, Prok(see specifically: CGView Builder v1.1.2 + Features v1.0.0) (Grant et al., 2023) was used for visualization of each genome complemented with its previously predicted ARGs, MGEs and unique genes. Additionally, pairwise genome alignments of clinical and non-clinical strains were performed with MUMmer (v3.23) (Kurtz et al., 2004) (options: -maxmatch; −l 100; −b), with each genome used as query. The resulting alignment positions at the reference sequence were also implemented in the genome visualization. Proksee depictions of corresponding genomes were combined and modified using Inkscape (v0.48) for direct genome comparison.
3 Results and discussion
3.1 General genome characteristics
The four non-clinical C. difficile strains belong to ST1/RT027 (C. difficile TS3_3), ST3/RT001/072 (C. difficile B1_2), ST8/RT002 (C. difficile J2_1), and ST11/RT078 (C. difficile MA_1). These STs/RTs are known for their high clinical relevance and/or prevalence and prominent representatives of the phylogenetic clades (Table 1) (Walker et al., 2013; Knight et al., 2021). Strains ST1/RT027 and ST11/RT078 are further prominent representatives of C. difficile strains that carry genes for the binary toxins additionally to the C. difficile-typical toxins (Martínez-Meléndez et al., 2022). Clinical strains DSM 28196 (ST1/RT027), SC084-01-01 (ST3/RT001/072), SC083-01-01 (ST8/RT002), and DSM 29747 (ST11/RT078) corresponding in ST/RT to the non-clinical strains were used in genome-based investigations covering analyses of MGEs as well as core and accessory genes. Throughout this work, the mentioning of corresponding strains refers to clinical and non-clinical strains of the same ST.
All eight genomes were initially evaluated for quality. The CheckM2 analysis thoroughly verified uniform genome completeness (99.86–99.99%) and purity (0.1–0.78% contamination).
General genomic features of the analyzed strains are listed in Table 1. In the following, the analyzed strains/genomes will be designated with their ST and clinical background (clinical = med, non-clinical = env) instead of their actual strain name (see Table 1). Most of the genomes comprised extrachromosomal elements (ECE). The ST3 genomes exhibited even two co-occurring ECEs. However, ECE carriage was not necessarily linked to the ST. Additionally, ECE size varied between the genomes of corresponding clinical and non-clinical strains, indicating their divergence. The clinical strains exhibited larger total genome size (including ECEs), and correspondingly more CDSs than non-clinical strains. No differences between clinical and non-clinical strains were recorded with respect to the number of rRNA and tRNA genes.
The screening for putative gene clusters encoding biosynthesis of secondary metabolites did not show differences between corresponding strains. All strains possessed regions predicted to encode cyclic-lactone-autoinducer, non-ribosomal peptide synthetase, or ranthipeptide. Since the capacity for secondary metabolite production did not differ between the strains, they were not considered in further analyses.
3.2 In silico examination of virulence factors for genomic assessment of virulence potential
Genomic examinations of the strains for the presence of fundamental virulence factors of C. difficile (listed in VFDB (Liu et al., 2022)) with BLAST+ blastp (Camacho et al., 2009) were performed to assess the virulence potential of the corresponding strains. Thereby, protein sequences of the virulence factors were compared to the reference sequence to define the query coverage (Figure 1A), and were further analyzed for sequence deviations between corresponding clinical and non-clinical strains (Figures 1A,B). Most of the characterized C. difficile virulence factors were present in the genomes of all strains. The main virulence factors in C. difficile pathogenicity, the disease-causing toxin genes tcdA and tcdB encoded by the pathogenicity locus (PaLoc), were identified in all strains (Figure 1A). Closer investigations of the PaLoc in all genomes including the regulatory genes tcdC and tcdR, along with tcdE (Mani and Dupuy, 2001; Matamouros et al., 2007; Govind and Dupuy, 2012) confirmed its consistent genomic location between the same genes (cdu1 and cdd1) like in the reference genome of C. difficile strain 630 (Monot et al., 2015) (Figures 2A,B). The recently described gene tcdL was also identified next to tcdE in all strains (Mehner-Breitfeld et al., 2018). DNA alignment of the PaLoc-operons demonstrated that genes were 100% identical between corresponding clinical and non-clinical strains and even between strains of different STs (Figure 2A), and also intergenic sequences of the PaLoc were verified to be identical among corresponding strains via BLAST analysis. All genes except tcdE shared at least 80% nucleotide sequence similarity to all other aligned genomes (Figure 2B). In addition to the PaLoc, another toxin-harboring locus (CdtLoc) is known in certain C. difficile strains of clades 2 (e.g., ST1/RT027), 3 and 5 (e.g., ST11/RT078) (Martínez-Meléndez et al., 2022), which harbors the binary toxin CDT encoded by the genes cdtA and cdtB (Gerding et al., 2014). The entire CDT genes were identified in both clinical and non-clinical strains of ST1 and ST11, while only 13% of the gene sequences were present in the other strains (Figure 1A). The CdtLoc was also found at a consistent genomic location between the same genes (CDIF27147_02765 and trpS) in accordance with the reference genome of C. difficile strain R20291 (Wang et al., 2022) (Figures 2C,D). Moreover, the regulatory gene cdtR was observed in all genomes. The cdtR gene sequences were identical in most of the genomes (Figure 2C). Strains without genes cdtA and cdtB harbored the same five small CDS instead (Figures 2C,D). In summary, toxin gene analyses confirmed uniform presence and location of toxin genes among corresponding clinical and non-clinical strains as well as identical toxin gene sequences. Sequence variants of toxin genes or the corresponding regulatory genes were demonstrated to influence strain virulence (Lanis et al., 2013; Dong et al., 2023). Based on our sequence comparisons, the corresponding clinical and non-clinical strains exhibit the same genomic virulence potential.
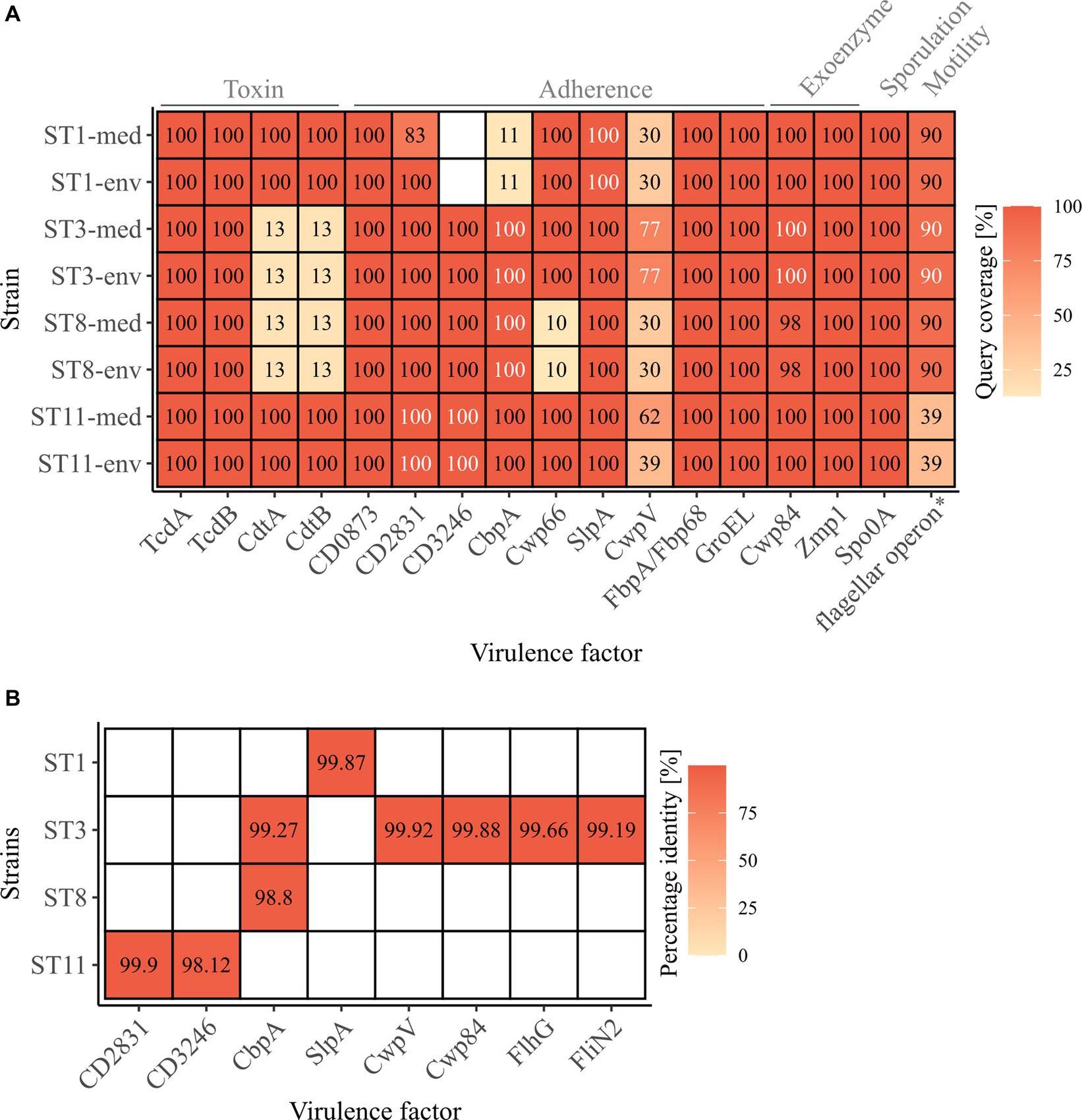
Figure 1. C. difficile-associated virulence factors in the analyzed strains. (A) Presence of the examined virulence factors is indicated as the protein sequence query coverage to the reference VFDB dataset, by color and stated coverage value. White coverage values highlight deviating sequences in proteins of the same query coverage between clinical and non-clinical strain. Virulence factors are labelled with their names as obtained from the VFDB dataset, and their related functions are stated on top. *flagellar operon comprising 41 CDSs obtained from the VFDB dataset, and its query coverage calculated as the relative number of present CDSs of the total 41. (B) Sequence similarity as percentage identity between clinical and non-clinical strains of the proteins highlighted in (A) as white.
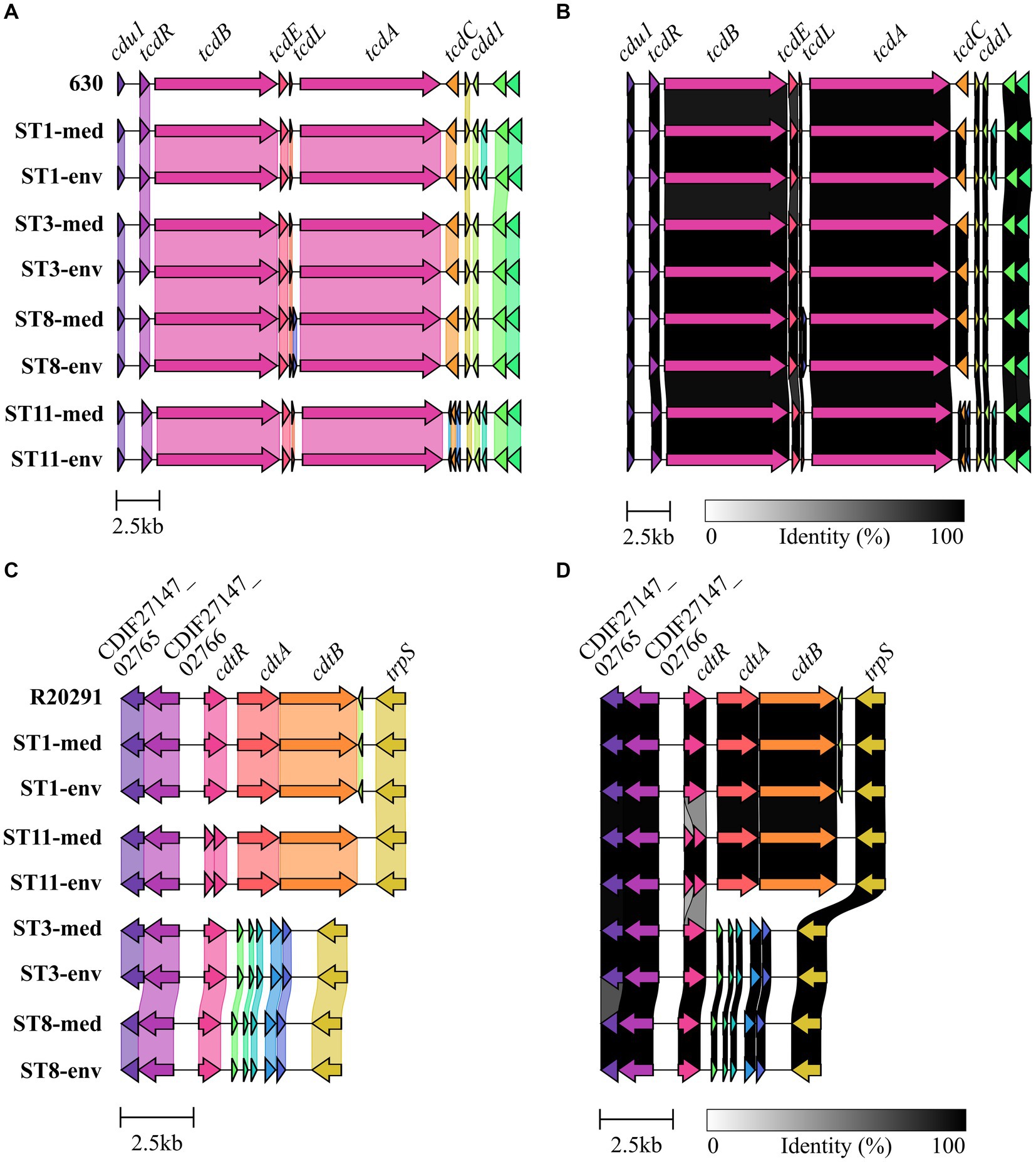
Figure 2. Gene cluster comparisons of toxin loci. Genes within and next to the toxin-encoding loci were compared on nucleotide sequence level between all analyzed strains for (A,B) the PaLoc with 630 as reference, and (C,D) the CdtLoc with R20291 as reference sequence. (A,C) depict 100% sequence identity, while (B,D) represent identities above 80%.
In addition to the toxin genes, other proteins are relevant for C. difficile pathogenicity, such as genes involved in cell adherence that are crucial for biofilm formation, which in turn affects resistance to harmful substances like antibiotics (Dapa and Unnikrishnan, 2013). Further important virulence factors are exoenzymes, sporulation and motility. All these virulence factors together determine the colonization efficiency (Awad et al., 2014). The majority of these virulence factors among the C. difficile-specific proteins were identified in the analyzed strains with 100% query coverage to the VFDB reference sequences, and only few proteins were only partially present (CbpA in ST1 strains, CwpV in all strains, Cwp66 in ST8 strains, flagellar operon in ST11 strains) or missing (CD3246 in ST1 strains) (Figure 1A). Thereby, the query coverage of the virulence factors with regard to the VFDB references was largely identical between the corresponding strains except for ST1-CD2831 and ST11-CwpV. In some cases, the protein sequences deviated between the corresponding strains by only 0.10 to 1.88% (Figures 1A,B), which often involved only one amino acid. The bigger sequence deviations were found in protein CbpA of ST3 and ST8 strains at different regions and in CD3246 of ST11 strains and consisted of a missing stretch of several amino acids (9–11 amino acids). These protein differences were detected in strains of both clinical/environmental background with regard to the VFDB reference. Protein CbpA is described with a modular architecture comprising different repeat types and repetitions (Tulli et al., 2013), which is also true for protein CD3246 (van Leeuwen et al., 2021). The effect of the described sequence variants in the analyzed adherence proteins on strain virulence so far remains unclear. Different studies addressed divergent protein sequences of different adherence virulence factors such as CwpV, CbpA, or Cwp66 (Reynolds et al., 2011; Tulli et al., 2013; Zhou et al., 2022). However, these studies focused on the modular architecture of the protein (CwpV) instead of single amino acid deviations or investigated the effect of complete gene deletion or disruption (CbpA and Cwp66), which significantly altered cell adhesion, but also stress tolerance and antibiotic resistance in the case of Cwp66. Thus, the observed differences in the protein sequences of adherence virulence factors did not indicate a significant influence on bacterial colonization and the accompanying virulence of the corresponding clinical and non-clinical strains. Further, no clinical-related pattern in the various differences was observed.
The flagellar operon was represented by 41 CDSs in the C. difficile-specific protein sequence VFDB dataset. Here, the amount of the flagellar CDSs with over 90% query coverage to their VFDB reference sequence was used instead of sequence coverage of the individual proteins. Clinical and non-clinical counterparts showed identical coverages of predominantly 90% of the flagellar CDSs, whereas strains of ST11 only possessed 39% (Figure 1A). This coincided with our observation under the electron microscope and indicated that these two strains lack a flagellum. Sequence comparisons by BLAST+ blastp analysis (Camacho et al., 2009) between corresponding genomes revealed complete congruence for almost all strains and CDSs. Solely ST3 strains deviated in two protein sequences from each other (FlhG, FliN2) by maximal 0.81% (Figures 1A,B).
Concluding on the analysis of virulence factors between clinical and non-clinical strains, only a few differences in the protein sequences were observed that did not exhibit a connection to the clinical background of the strains. Therefore, a similar virulence would be expected for all strains, independent of their environmental or clinical origin. This conclusion coincided with the observations in the study by Zhou and colleagues (Zhou et al., 2021), where differences in the virulence factors (presence and sequence identity) between clinical and environmental strains were linked to the genotype but not bacterial origin.
3.3 Core/Pan genome analysis
Overall core/pan genome analysis with all eight genomes resulted in 2,735 groups of core genes and varying numbers of unique genes between 12 and 351 (Figure 3A). A pan genome analysis of clinical strains grouped together and compared to the non-clinical strains demonstrated that the clinical strains possessed more accessory genes (634 vs. 400). However, none of these accessory genes were shared by all genomes of the four clinical strains. Similarly, the accessory genome of the non-clinical strains did not comprise genes present in all of the four genomes. Following, pairwise pan genome analyses of corresponding clinical and non-clinical strains verified the observation of more accessory genes in the genomes of the clinical strains. We calculated the relative proportions of unique genes per genome in relation to the total number of CDSs to take account of the different genome sizes (Figure 3B). Differences in accessory genome size between clinical and non-clinical strain ranged from 0.21% between ST11 strains to 4.36% between ST3 strains.
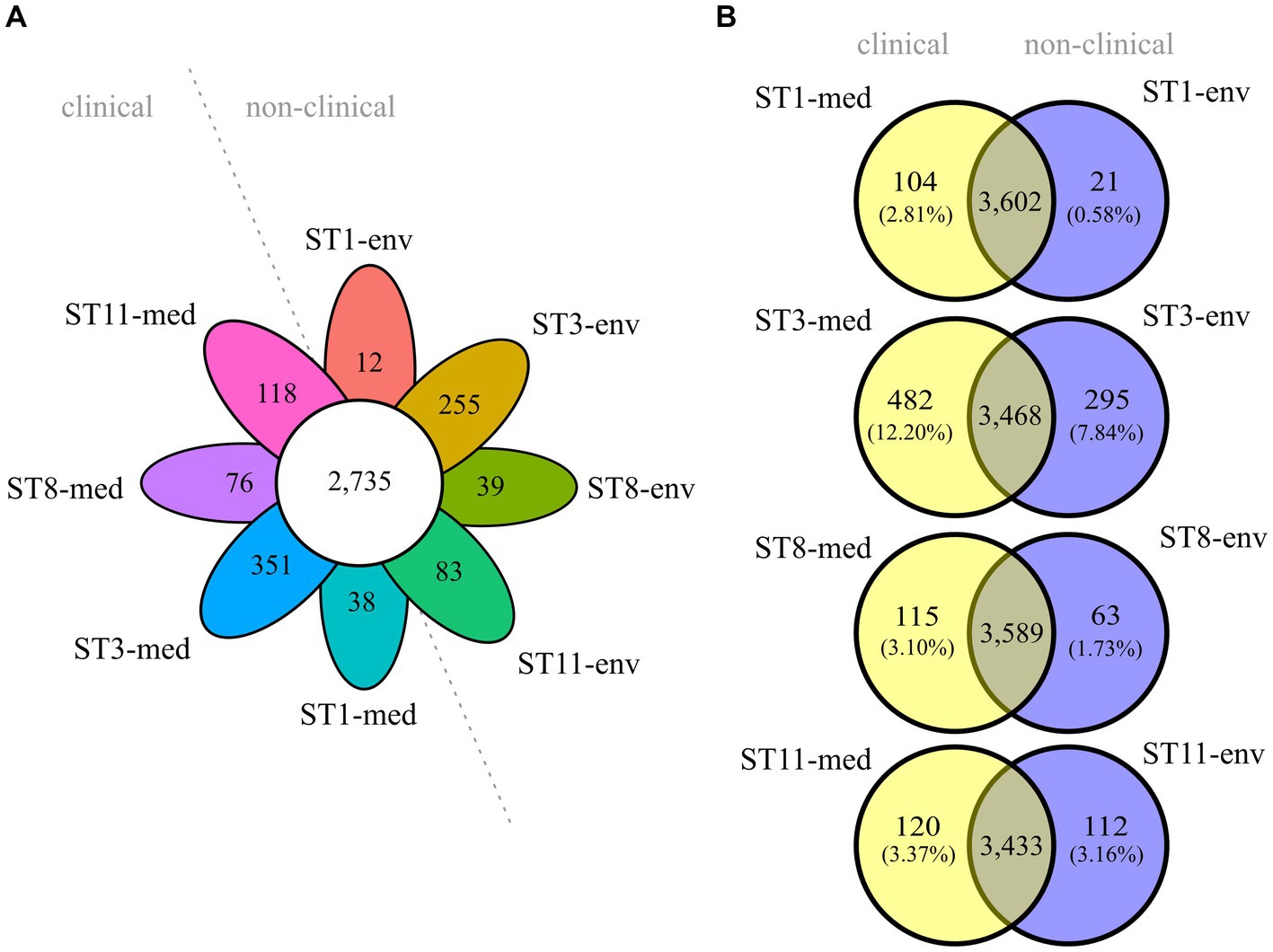
Figure 3. Core and accessory genome sizes of the analyzed C. difficile strains. Venn diagrams depicting the shared and unique genes among (A) all eight strains, or (B) pairwise between ST-corresponding clinical and non-clinical strain. The relative proportions of unique genes with regard to the total number of CDS per genome are indicated in parentheses below each absolute number of unique genes.
To elucidate genomic differences between clinical and non-clinical strains, unique genes were functionally classified into COGs with eggNOG-mapper (Cantalapiedra et al., 2021). Thereby, not all input genes could be classified, and several classified genes were not assigned to a specific COG. The number of un-classified or un-assigned genes varied between the genomes, but almost all these genes were annotated as hypothetical proteins. For better comparison, the relative abundance of assigned COGs in each accessory genome was calculated as described above (Figure 4A). This illustrated the previously determined differences in relative unique gene carriage within clinical and non-clinical strain pairs. Besides the noticeable numbers of unclassified and unassigned genes (hypothetical proteins), further bars representing the COG categories S (“Function unknown”), K (“Transcription”), L (“Replication, recombination and repair”) and also M (“Cell wall/membrane/envelope biogenesis”) were prominent to varying degrees in all strains, and seemed to be more abundant in the clinical strains. To further examine this, the differences in unique gene/COG proportions between corresponding clinical and non-clinical strains were determined (Figure 4B). This allowed identifying abundance trends of unique genes of specific COGs. The COG category S was the most abundant category and dominated in the clinical strains. Prokka-annotated functions (Seemann, 2014) of the genes assigned to COG category S were diverse and included for example phage-related proteins. Inspection of these genes for further potential virulence factors revealed the two genes encoding haemolysin XhlA and the virulence-associated protein E. Haemolysin XhlA and virulence-associated protein E are not associated with C. difficile virulence according to the data in VFDB (Liu et al., 2022), but are involved in the virulence of other bacteria such as Clostridium chauvoei (Thomas et al., 2021) and Streptococcus suis serotype 2 (Ji et al., 2016). Nevertheless, though C. difficile is not established as hemolytic pathogen, some evidence of hemolysis was recorded (Alkudmani, 2018). Influence of haemolysin XhlA and virulence-associated protein E on C. difficile virulence however has not been examined, yet, so that their virulence potential remains unknown. Both of these genes were present in ST3-env, while ST3-med and ST8-med possessed the haemolysin gene and ST11-med the virulence-associated protein E only. Thus, a direct relation to clinical background was not recorded for these specific genes.
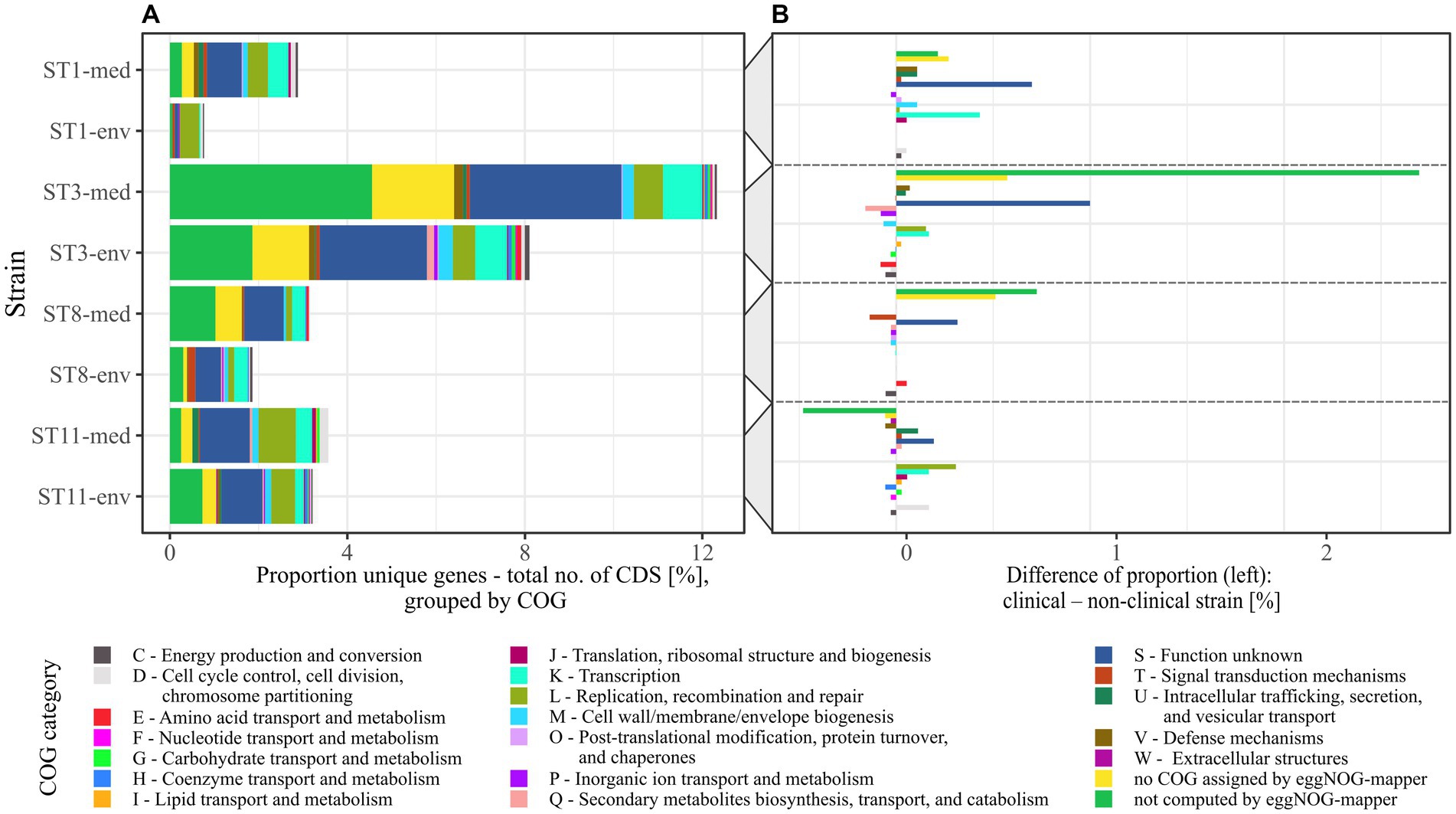
Figure 4. Relative abundance of COGs assigned to the unique genes from pairwise pan genome analyses. (A) The relative proportions of unique genes to total number of CDSs per genome in pairwise comparisons was transferred to their assigned COGs, which are designated with COG category name and function. (B) Individual COG proportions of unique genes of non-clinical strains were subtracted from the corresponding clinical strain to see if specific COGs are more frequent among accessory genes of a certain clinical background.
The next highest differences were visible for COG categories L and K in particular, both being more abundant in three of the four clinical strains (ST1-/ST3-/ST11-med). Therefore, the accessory genomes of the clinical strains encoded more genes of unknown function, recombination- or transcription-associated activity than the genomes of the non-clinical strains. Interestingly, Lewis et al. identified accessory genes of these functions to correlate with higher strain virulence (Lewis et al., 2017). For example, the genes rep and recF encoding DNA helicase and DNA recombinase, and the gene iap encoding the lysozyme-like family protein were found in ST1 strains of high virulence, whereas they were absent in low-virulence ST1 strains. This gene presence/absence pattern was likewise true for our ST1 strains with respect to clinical background instead of in vivo-measured disease severity, implying a higher virulence potential of the clinical strain based on the accessory genome. Another study also identified accessory transcriptional regulators in hypervirulent strains of RT027 in contrast to the less virulent predecessor strain and pointed to the significance of these genomic accessories on strain virulence (Stabler et al., 2009). The increased proportion of transcriptional accessory genes compared to total CDS content along with the increased genome size/CDS carriage (Table 1) in the clinical strains might reflect adaptation to higher environmental variability (Parter et al., 2007). Noteworthy, Sebaihia et al. already pointed to the high amount of transcriptional regulators in C. difficile strain 630 and associated them with its potential ability to adapt to a rapidly changing environment (Sebaihia et al., 2006). Likewise, pan genome investigations on RT014 C. difficile strains isolated from farm pigs and infected humans did not compare clinical and non-clinical strains but also observed that the majority of accessory genes belonged to the functions of genetic or environmental information processing (Knight et al., 2017). Consequently, the clinical strains exhibited the genomic potential for faster reaction to changing environmental conditions such as emerging stress factors, which in turn affects survival as well as colonization and disease manifestation.
Although COG category M seemed noticeably abundant in the individual proportions (Figure 4A), it did neither exhibit a specific trend nor remarkable abundance in the proportional differences (Figure 4B). Contrary, category U (“Intracellular trafficking, secretion, and vesicular transport”) showed a noticeable trend toward genomes of clinical strains. Examination of the U-unique genes revealed that they were effectively restricted to clinical strains of ST1, ST3, and ST11, and thereby encoded only proteins involved in conjugal transfer of DNA, such as type IV secretory system components and relaxases/mobilization nuclease domain proteins. This is interesting in the context of horizontal gene transfer for fast adaptation to changing environmental conditions such as the presence of antibiotics (de la Cruz and Davies, 2000). Moreover, Brouwer et al. demonstrated the conjugative transfer of the PaLoc from a toxigenic to a non-toxigenic C. difficile strains, which turned the non-toxigenic strain into a toxin-producing one (Brouwer et al., 2013). Bacterial conjugation is mediated by cell-to-cell contact, which is eminently present in bacterial biofilms. Biofilm production and conjugation activity are therefore intertwined. This was already demonstrated in other bacteria such as Escherichia coli (Ghigo, 2001) and Bacillus subtilis (Lécuyer et al., 2018), where biofilm formation was shown to significantly impact conjugation efficiency. Taking this and the occurrence of conjugal accessory genes in clinical strains into account, the above identified sequence deviations in some adherence virulence factors could also be related to conjugal activity in the context of biofilm formation. This potential association has not been addressed in C. difficile so far, but would be worth considering in investigations on its virulence.
The only tendency to the non-clinical strains showed unique genes of the COG category P (“Inorganic ion transport and metabolism”), which was represented by only one gene per each non-clinical accessory genome encoding a cobalt transport protein, an ABC transporter transmembrane region, or a sodium sulfate symporter.
Conclusively, the comprehensive pan genome analysis in clinical and non-clinical strain comparisons established associations between clinical background and higher abundance of hypothetical proteins or proteins of unknown functions, and of genes linked to increased potential of conjugal and transcriptional activity. This trend is possibly linked to higher virulence, and in general can contribute to rapid physiological and evolutionary adaptation, which implies elevated virulence.
3.4 Prediction of ARGs
Antibiotic resistances are another crucial factor in C. difficile virulence, as they often allow C. difficile colonization and infection manifestation (Spigaglia, 2016). Further, ARGs can be linked to MGEs and contribute to the accessory genome (Sebaihia et al., 2006), which might partially explain the previously determined higher number of unique genes in the genomes of the clinical strains (Figure 3). We inspected all eight genomes for putative ARGs (including AR-conferring mutations) (Figure 5). Corresponding strains exhibited similar ARG patterns. Few genes were only identified in one strain or in multiple strains of the same ST or clinical background, respectively. For example, genes contributing to resistance against streptothricins (sat4) or aminoglycosides (ant(6)-Ia, aph(3′)-IIIa) were only identified in one non-clinical strain (ST11-env), while genes conferring resistance against macrolides (erm(B)), rifampin (rpoBR505K) or tetracyclines (tet(40), tet(M)) were only detected in one clinical strain (ST11-med). Interestingly, tetracycline resistance has been linked to the emergence of ST11/RT078 strains as human pathogen (Dingle et al., 2019). However, the studies of Knetsch et al. (2014) and Zhou et al. (2021) identified the same ARGs in C. difficile strains of both environmental and clinical origin. Thus, a specific antibiotic resistance could not be linked to the clinical background. Regarding the ARG distribution with respect to the bacterial genotype, ST1 strains encoded most ARGs. Overall, clinical strains encoded one to four more ARGs than non-clinical strains.
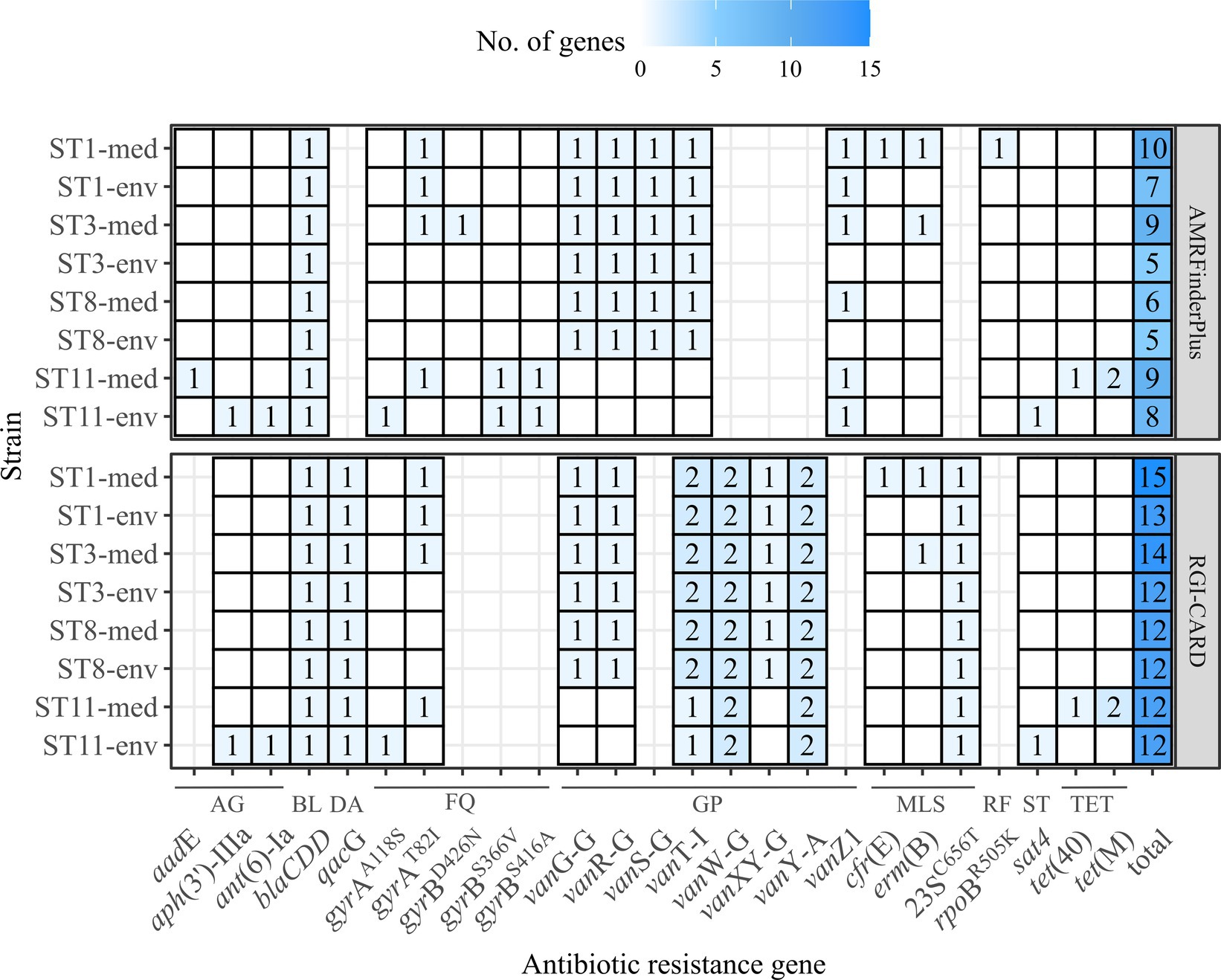
Figure 5. Predicted ARGs in the analyzed C. difficile strains. The number of identified ARGs and AR-conferring mutations as predicted with RGI-CARD (Alcock et al., 2023) and AMRFinderPlus (Feldgarden et al., 2021) were indicated by color and respective value, with white/no value meaning gene absence. Heatmap-tiles are missing for genes that were not part of the analysis tools. The total number of predicted ARGs for each program is additionally stated. ARGs are grouped according the associated antibiotic class: AG, aminoglycosides; BL, beta-lactams; DA, disinfecting agent and antiseptics; FQ, fluorquinolones; GP, glycopeptides; MLS, macrolides/lincosamides/streptogramins; RF, rifamycin; ST, streptothricins; TET, tetracyclines.
3.5 Prediction of MGEs
All analyzed C. difficile genomes were investigated for MGEs. A plasmid family was solely present in ST11-med, representing the type repUS43 twice. Consequently, none of the observed ECEs was classified as plasmid, indicating another extrachromosomal type such as cryptic plasmids or prophages (Amy et al., 2018; Ramírez-Vargas et al., 2018). Thus, the genomes of all strains were analyzed for putative prophage regions. Contrary to the plasmid analysis, all ECEs besides the 7.6 kb element of ST3-env represented putatively intact prophages spanning the entire ECEs. Up to four incomplete or intact prophage regions were predicted per genome, except for ST11-med, which carried only one putative, intact prophage (Figure 6). Thereby, corresponding clinical and non-clinical strains showed comparable prophage carriage. This is in line with the study by Blau and Gallert (2024), where prophage analysis in 166 environmental C. difficile strains suggested a correlation between prophage carriage and strain genotype (ST/RT).
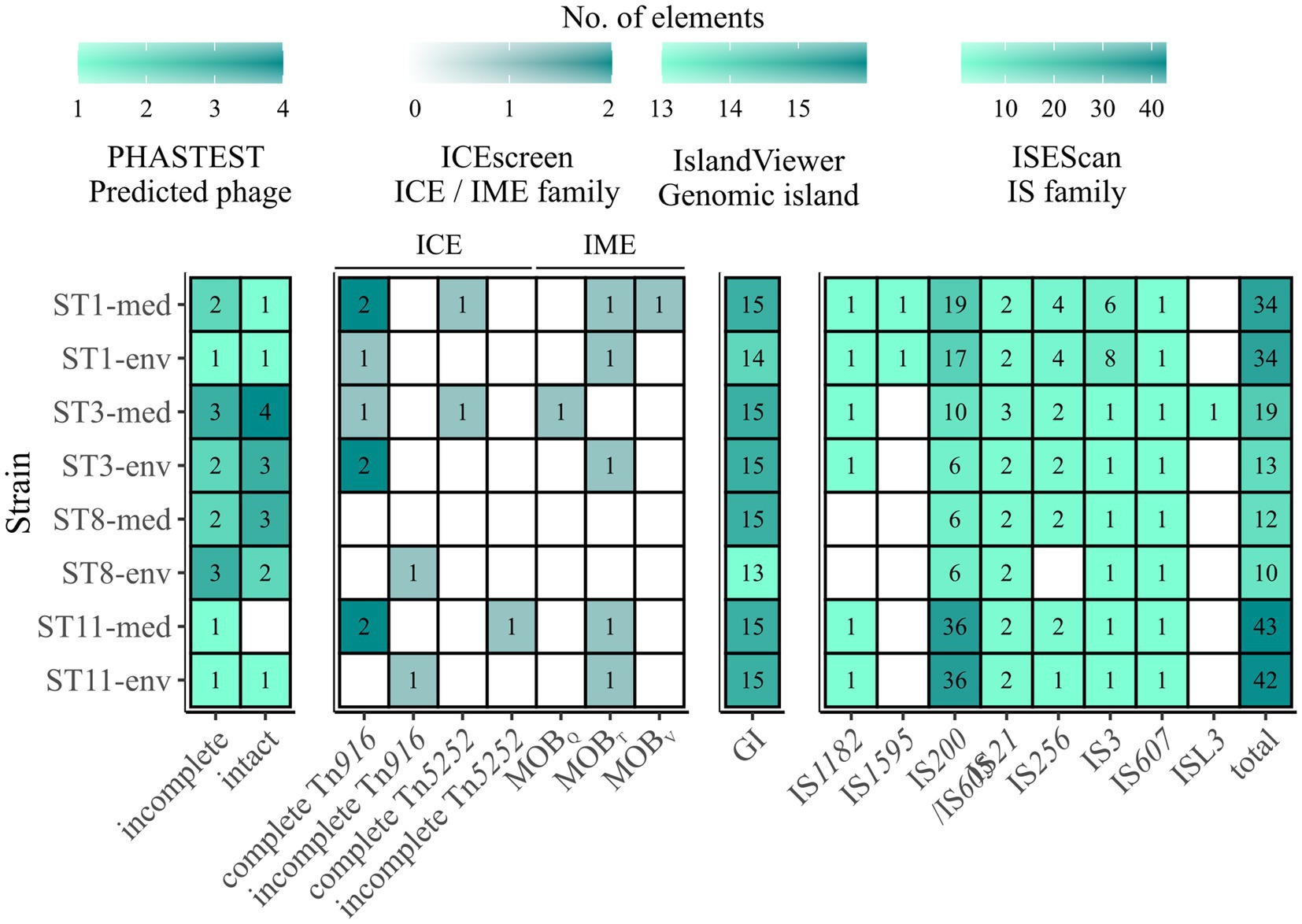
Figure 6. Predicted MGEs in the analyzed strains. The number of the analyzed MGEs prophages, integrative elements, GIs, and IS elements were indicated by color and the respective number, with white/no value meaning no prediction. Prophages are described as incomplete or intact as predicted by PHASTEST (Wishart et al., 2023). Integrative elements are categorized by the assigned superfamily and grouped into ICE and IME. Presence of IS elements is described in the context of the identified families, and additionally given as total number of IS elements.
Integrative elements were detected in all strains except ST8-med (Figure 6). Two different types of elements, integrative and conjugative elements (ICEs) and integrative mobilizable elements (IMEs) were identified in both clinical and non-clinical strains. The two superfamilies Tn916 and Tn5252 represented ICEs, both observed as complete or incomplete modules without the ability of integration (“conjugation module”). The complete Tn916 elements dominated, while incomplete Tn916 and in−/complete Tn5252 modules were likewise minor abundant. The ICE Tn916 was already described in C. difficile and associated with antibiotic resistances, predominantly with tet(M) followed by erm(B) (Spigaglia et al., 2005, 2007; Dong et al., 2014), although the latter one is rather linked to other MGEs (Kartalidis et al., 2021). Consequently, this ICE is of interest in the context of spreading antibiotic resistances. Tn5252 modules were restricted to clinical strains, while the incomplete Tn916 modules were only found in non-clinical strains. Moreover, clinical ST1 and ST11 strains possessed three times more ICE modules than their non-clinical counterpart. Altogether, the ICE predictions could explain the presence of accessory genes with conjugal function (COG U) in the clinical strains described above, as they possessed elements that were missing in the corresponding non-clinical strains. Looking on IMEs, none were identified in ST8-strains, while all other isolates possessed IME modules of the families MOBQ, MOBT, and MOBV. MOBT elements dominated with similar occurrence in clinical and non-clinical strains, whereas each of the other two IME modules occurred once, but only in clinical strains. Overall, IMEs were less common than ICEs. Similarly to ICEs, IMEs are potential carrier for ARGs such as tet(M) and, thus, are also involved in distribution of antibiotic resistances (López de Egea et al., 2023).
13 and predominantly 15 GIs were detected on each chromosome (Figure 6). These numbers exceeded the occurrences of the previously examined GI prophages and integrative elements and indicated the presence of other GI types. Depending on the ST, clinical strains possessed the same or a higher number of predicted GIs than the corresponding non-clinical strain. In addition to various GIs, we also examined IS elements and detected eight different IS families (Figure 6). The prevailing family was IS200/IS605 with an incidence of six to 36 elements in the genomes of both ST11 strains. Elements belonging to families IS21 and IS256 occurred twice per genome on average, while family IS3 was present several times ST1 strain genomes and once in all other genomes. These IS families were identified to be potentially linked to ARGs and, consequently, might further contribute to ARG spread among C. difficile strains (Razavi et al., 2020). All other IS families were identified once per genome or not at all. Elements of type IS1595 and ISL3 were only found in ST11 or ST3-med strain genomes, respectively. Taken together, corresponding clinical and non-clinical strains exhibited similar IS patterns. Thereby, the total number of IS elements ranged between ten and 43 per genome, with clinical strains mostly possessing more IS elements (between one and six more elements) than the corresponding non-clinical strain. Further, general IS abundance was correlated to ST strain genomes, with ST8-strain genomes possessing the lowest and ST11-strain genomes the highest number of IS elements. Thus, regarding all above described MGEs, an overall trend of higher MGE carriage in clinical than in non-clinical strains was recorded, while no specific MGE was connected to clinical background.
3.6 Pairwise genome comparisons with implementation of preceding analyses
The previous analyses of accessory genes, ARGs, and MGEs showed differences between clinical and non-clinical strains. All these results were combined with MUMmer alignments (Kurtz et al., 2004) and together put into genomic context in pairwise genome alignments (Figures 7, 8). This representation revealed connections between the various analyzed elements based on co-occurrence. First, the MUMmer alignments again demonstrated the higher abundance of unique genes in the clinical strains, as they exhibited more alignment gaps that corresponded to missing regions in the corresponding non-clinical strain. Consequently, the majority of the unique genes were found next to each other in clusters. Mapping of the predicted MGEs and ARGs illustrated that they resided at the same genomic positions in the corresponding strains. MGEs and ARGs that were only present in one of the two compared strains (mostly the clinical strain) often occurred together. The genome of strain ST1-med (Figure 7A) possessed the ARG erm(B) that resided within ICE Tn916. This conjunction was observed in ST3-med genome as well (Figure 7B) and supported the already mentioned connection between Tn916 elements and ARG erm(B) (Spigaglia et al., 2005, 2007). ARG tet(M) in the ST11-med genome exhibited the presumed connection with Tn916 (Figure 8B) (Dong et al., 2014). ST11-med further possessed ARGs tet(40) and aadE, which occurred within an incomplete Tn5252 element (Figure 8B). In contrast, the non-clinical ST11 strain carried three ARGs (ant(6)-Ia, sat4, aph(3′)-IIIa) close to each other and to an incomplete Tn916 element, thus located outside of this predicted ICE region (Figure 8B). However, the GI prediction identified a larger mobile region than determined for the incomplete Tn916 that included the three ARGs. The mobile region was confirmed by an alignment gap and a cluster of unique genes of similar size. The occurrence of the ARGs ant(6)-Ia, sat4, and aph(3′)-IIIa as a resistance cassette was already observed in genomes of ST13 and ST49 C. difficile strains (RT014, clade 1) from porcine origin (Knight et al., 2017) and in genomes of ST11 strains (RT126 and RT078) from environmental sources (Blau et al., 2023). Both studies did not address a connection between this resistance cassette and a MGE, although the latter one hypothesized the possibility of genetic transmission (Blau et al., 2023). Another ARG, qacG of the ST3-med genome (Figure 7B), was located within a predicted GI and next to a complete IME of family MOBQ. All further qagG genes and other ARGs were not colocalized with MGEs. However, some of these ARGs resided in close proximity to predicted MGEs, such as the remaining qacG genes (Figures 7A,B, 8A,B), cfr(E) in the ST1-med genomes (Figure 7A), or vanT-G in ST1 and ST3 strain genomes (Figures 7A,B). Connections between these colocalized ARGs and MGEs are speculative but might still be interesting for further investigations regarding dissemination of antibiotic resistances. For instance, ARG cfr(E) was also found within an undescribed MGE in genomes of RT027 C. difficile strains from Mexico (Stojković et al., 2019).
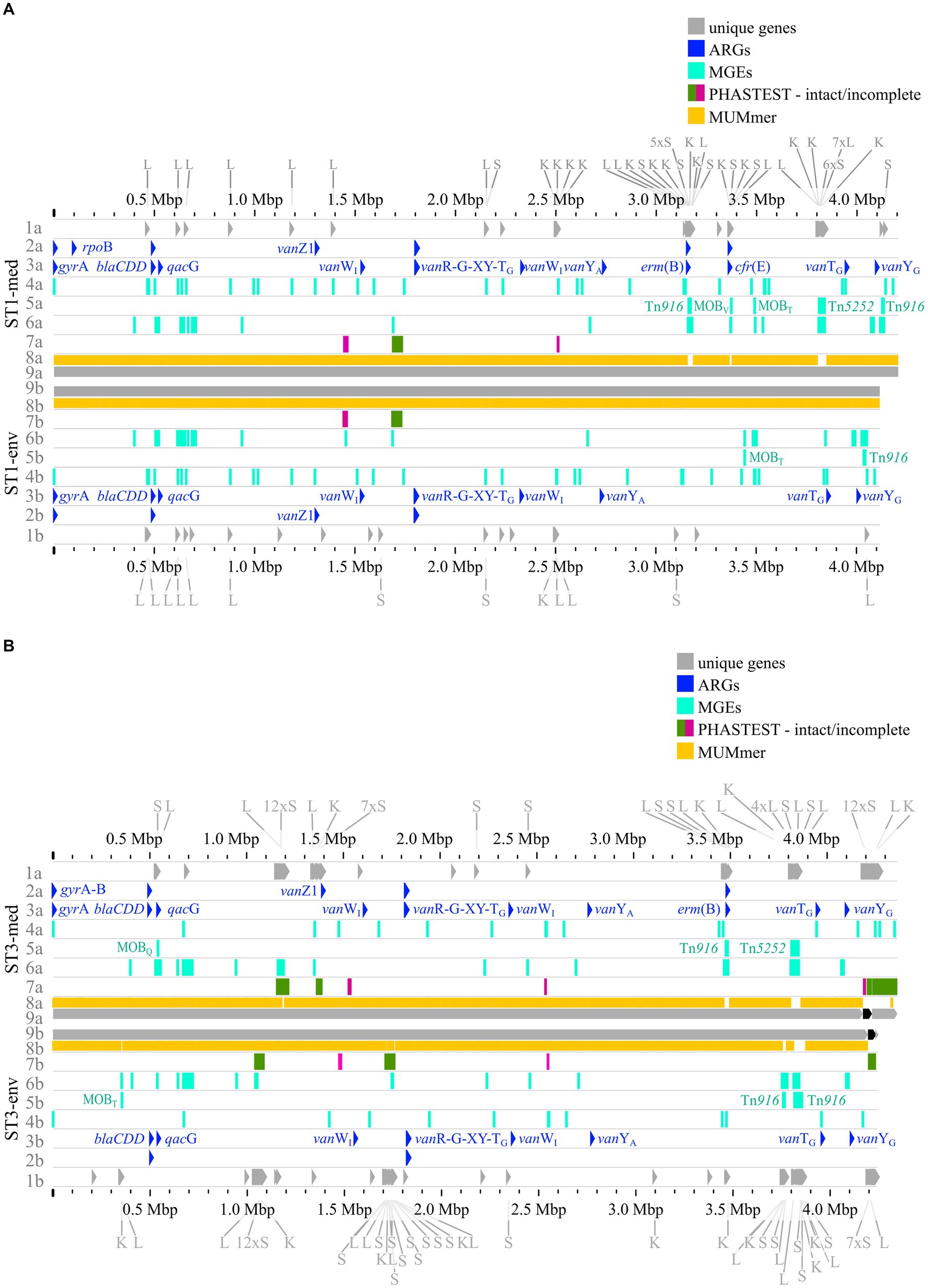
Figure 7. Pairwise genome comparisons complemented with the predicted ARGs, MGEs, and accessory genes. Genome comparisons of (A) ST1-strains and (B) ST3-strains are depicted with different tracks for each visualized feature in the clinical strain at the top (track letter a) and non-clinical strain at the bottom (track letter b). The tracks represent: 1 unique genes with genes assigned to COG S, K, L highlighted, and multiple genes of the same COG grouped together if necessary for better visibility, 2 AMRFinderPlus (Feldgarden et al., 2021) predicted ARGs, 3 RGI+CARD (Alcock et al., 2023) predicted ARGs, 4 predicted IS elements, 5 predicted integrative elements labelled with assigned superfamily, 6 predicted GIs, 7 prophage prediction with completeness color-coded according to PHASTEST (Wishart et al., 2023), 8 MUMmer alignment (Kurtz et al., 2004), 9 replicons.
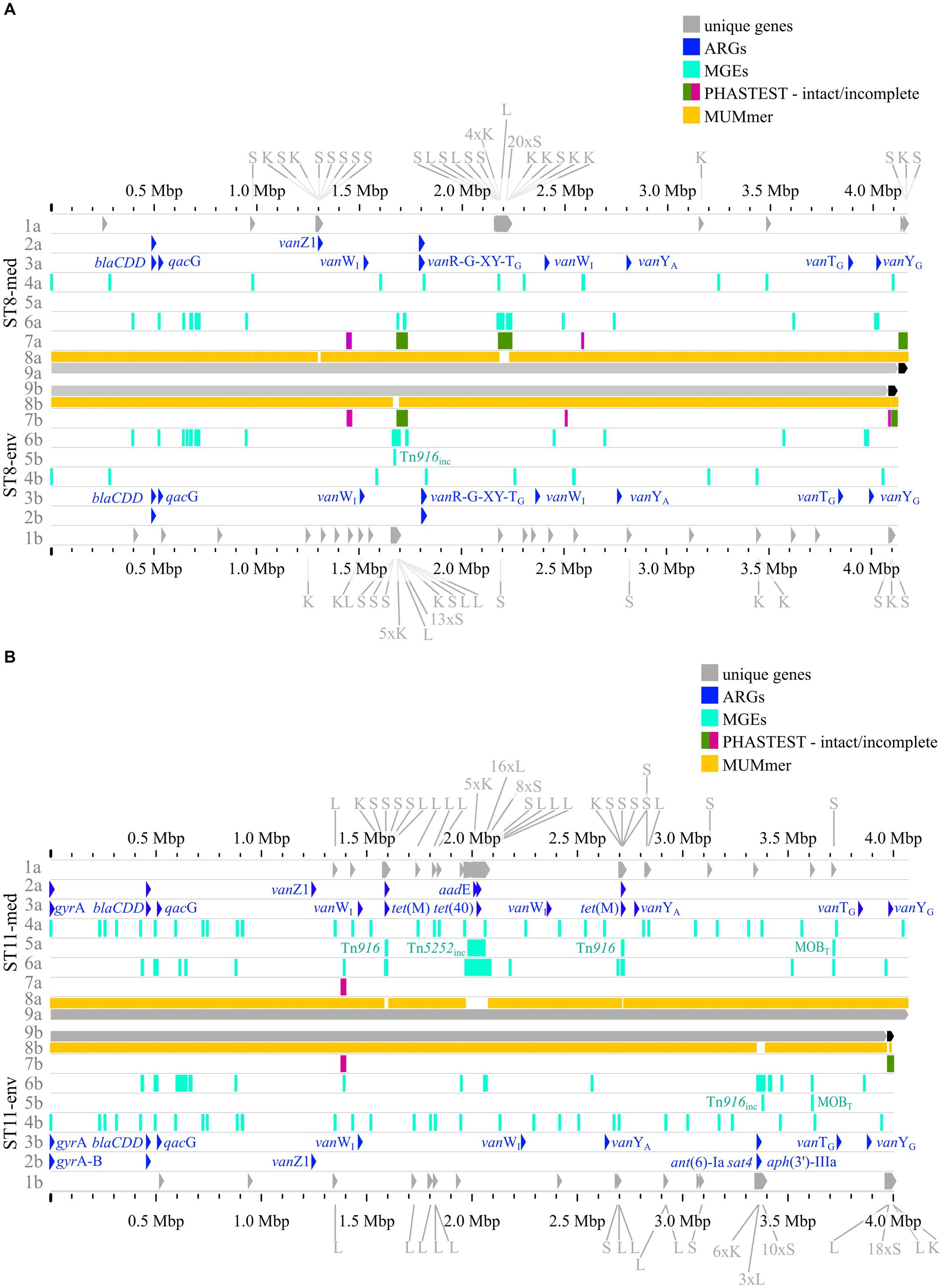
Figure 8. Pairwise genome comparisons complemented with the predicted ARGs, MGEs, and accessory genes. (A) ST8-strains, and (B) ST11-strains are depicted with different tracks for each visualized feature in the clinical strain at the top (track letter a) and non-clinical strain at the bottom (track letter b). The tracks represent: 1 unique genes with genes assigned to COG S, K, L highlighted, and multiple genes of the same COG grouped together if necessary for better visibility, 2 AMRFinderPlus (Feldgarden et al. 2021) predicted ARGs, 3 RGI+CARD (Alcock et al. 2023) predicted ARGs, 4 predicted IS elements, 5 predicted integrative elements labelled with assigned superfamily, 6 predicted GIs, 7 prophage prediction with completeness color-coded according to PHASTEST (Wishart et al. 2023), 8 MUMmer alignment (Kurtz et al. 2004), 9 replicons.
The predicted prophage regions, integrative elements, and GIs accounted for the majority of the genomic differences between corresponding strains in the form of alignment gaps (Figures 7A,B, 8A,B). Hargreaves et al. likewise already pointed out that major regions of genome divergence in C. difficile strains from estuarine samples belonged to the MGE-type transposons (Hargreaves et al., 2015). The unique genes in these regions largely belonged to COG categories S, K, and L (Figures 7A,B, 8A,B), which showed the association of these unique genes to MGEs. This is especially interesting for the COG category S of “Unknown function,” which implied their involvement in the function of the respective MGE or an encoded accessory function that is potentially relevant for strain virulence. Accessory genes of COG category S with virulence potential were already identified during the pan genome analysis, which encoded haemolysin XhlA and the virulence-associated protein E. Within this genome comparison analyses, these potential virulence factors were now associated to MGEs and, thus, might be transferrable between cells, which sheds another light on these potential virulence factors. Many of the unique genes that were only annotated as hypothetical proteins belonged to the MGE-associated clusters, which indicates involvement in MGE-related functions.
4 Conclusion
The comprehensive genome analyses and comparisons of corresponding clinical and non-clinical C. difficile strains revealed genomic patterns associated with clinical background. No distinct differences in virulence factors known to be crucial for C. difficile virulence, such as toxins and proteins involved in adherence, sporulation, exoenzymatic reaction and motility were detected. Thus, corresponding strains possessed the same fundamental virulence equipment, which suggested same virulence regardless of the clinical/non-clinical background. Pan genome analysis revealed that clinical strains possessed a larger accessory genome. Assignment of the unique genes to functional clusters demonstrated the trend in clinical strains with more unique genes previously annotated as hypothetical proteins or functionally assigned to COG categories S, K, L, and U. Such trend of those accessory genes/functions is linked to higher virulence and enables the strain to rapidly respond to changing environmental conditions such as emerging stress, which supports bacterial survival, colonization, and disease manifestation. Further analyses predicted various ARGs and MGEs. No particular ARG/MGE was specifically linked to clinical background, but the overall trend of more ARGs and MGEs in clinical strains was observed. Results from pan genome, ARG, and MGE analyses together in genome alignments revealed conjunctions between specific ARGs and MGEs. The genome comparisons further demonstrated that genomic differences between clinical and non-clinical strains mainly originated from MGEs. This also included the majority of unique genes with higher abundance in clinical strains that were assigned to COG categories with connection to increased virulence and faster physiological reaction capacity. Consequently, these trends suggested adaptations of the clinical strains by gene acquisition that might manifest in higher strain virulence. This should be further investigated to elucidate C. difficile virulence and progression, especially in the context of clinical and non-clinical strain comparison. Therefore, future investigations are advised to incorporate non-clinical strains in comparative analyses for a comprehensive understanding of C. difficile virulence development. These findings also highlight the importance of MGEs for C. difficile, since they seem to be involved not only in the dissemination of ARGs or virulence factors but also impact virulence in another way. We further advise to examine genomic analyses in whole-genome context to reveal conjunctions between the various elements.
Data availability statement
Genome data of the bacterial strains is deposited at NCBI (https://www.ncbi.nlm.nih.gov/) under following GenBank accession numbers: Clostridioide difficile strain TS3_3 (ST1-env) under CP134872, strain B1_2 (ST3-env) under CP132141-3, strain J2_1 (ST8-env) under CP134690-1, strain MA_1 (ST11-env) under CP132139-40, DSM28196 (ST1-med) under CP012320, SC084-01-01 (ST3-med) under CP132146-8, SC083-01-01 (ST8-med) under CP132144-5, and DSM29747 (ST11-med) under CP019864.
Author contributions
MS: Conceptualization, Data curation, Formal analysis, Methodology, Validation, Visualization, Writing – original draft, Writing – review & editing. TR: Validation, Writing – review & editing. JO: Validation, Writing – review & editing. RD: Investigation, Project administration, Validation, Writing – review & editing. AP: Supervision, Validation, Writing – review & editing.
Funding
This work was funded by the Federal State of Lower Saxony, Niedersächsisches Vorab CDiff and CDInfect projects (VWZN2889/3215/3266). The authors acknowledge support by the Open Access Publication Funds of the Göttingen University. This work was also partly supported by the Göttingen Graduate Center for Neurosciences, Biophysics, and Molecular Biosciences at the Georg-August-Universität Göttingen.
Acknowledgments
The authors thank Uwe Groß, Wolfgang Bohne, Julian Schwanbeck, and Ines Oehmig for providing the clinical reference strains. The authors thank also Cathrin Spröer (DSMZ) for support and Melanie Heinemann, Mechthild Bömeke as well as Nicole Heyer (DSMZ) for technical assistance.
Conflict of interest
The authors declare that the research was conducted in the absence of any commercial or financial relationships that could be construed as a potential conflict of interest.
Publisher's note
All claims expressed in this article are solely those of the authors and do not necessarily represent those of their affiliated organizations, or those of the publisher, the editors and the reviewers. Any product that may be evaluated in this article, or claim that may be made by its manufacturer, is not guaranteed or endorsed by the publisher.
Supplementary material
The Supplementary material for this article can be found online at: https://www.frontiersin.org/articles/10.3389/fmicb.2024.1404491/full#supplementary-material
Footnotes
1. ^https://github.com/rrwick/Porechop
2. ^https://github.com/rrwick/Filtlong
References
Alcock, B. P., Huynh, W., Chalil, R., Smith, K. W., Raphenya, A. R., Wlodarski, M. A., et al. (2023). CARD 2023: expanded curation, support for machine learning, and resistome prediction at the comprehensive antibiotic resistance database. Nucleic Acids Res. 51, D690–D699. doi: 10.1093/nar/gkac920
Alkudmani, Z. S. B. (2018). The identification and characterization of novel haemolysin genes from Clostridium difficile : University College London.
Amy, J., Bulach, D., Knight, D., Riley, T., Johanesen, P., and Lyras, D. (2018). Identification of large cryptic plasmids in Clostridioides (Clostridium) difficile. Plasmid 96-97, 25–38. doi: 10.1016/j.plasmid.2018.04.001
Awad, M. M., Johanesen, P. A., Carter, G. P., Rose, E., and Lyras, D. (2014). Clostridium difficile virulence factors: insights into an anaerobic spore-forming pathogen. Gut Microbes 5, 579–593. doi: 10.4161/19490976.2014.969632
Balsells, E., Shi, T., Leese, C., Lyell, I., Burrows, J., Wiuff, C., et al. (2019). Global burden of Clostridium difficile infections: a systematic review and meta-analysis. J. Glob. Health 9:010407. doi: 10.7189/jogh.09.010407
Bertelli, C., Laird, M. R., Williams, K. P., Lau, B. Y., Hoad, G., Winsor, G. L., et al. (2017). IslandViewer 4: expanded prediction of genomic islands for larger-scale datasets. Nucleic Acids Res. 45, W30–W35. doi: 10.1093/nar/gkx343
Bidet, P., Barbut, F., Lalande, V., Burghoffer, B., and Petit, J.-C. (1999). Development of a new PCR-ribotyping method for Clostridium difficile based on ribosomal RNA gene sequencing. FEMS Microbiol. Lett. 175, 261–266. doi: 10.1111/j.1574-6968.1999.tb13629.x
Blau, K., Berger, F. K., Mellmann, A., and Gallert, C. (2023). Clostridioides difficile from Fecally contaminated environmental sources: resistance and genetic relatedness from a molecular epidemiological perspective. Microorganisms 11:2497. doi: 10.3390/microorganisms11102497
Blau, K., and Gallert, C. (2024). Prophage carriage and genetic diversity within environmental isolates of Clostridioides difficile. Int. J. Mol. Sci. 25. doi: 10.3390/ijms25010002
Blin, K., Shaw, S., Augustijn, H. E., Reitz, Z. L., Biermann, F., Alanjary, M., et al. (2023). antiSMASH 7.0: new and improved predictions for detection, regulation, chemical structures and visualisation. Nucleic Acids Res. 51, W46–W50. doi: 10.1093/nar/gkad344
Bolger, A. M., Lohse, M., and Usadel, B. (2014). Trimmomatic: a flexible trimmer for Illumina sequence data. Bioinformatics 30, 2114–2120. doi: 10.1093/bioinformatics/btu170
Brouwer, M. S. M., Roberts, A. P., Hussain, H., Williams, R. J., Allan, E., et al. (2013). Horizontal gene transfer converts non-toxigenic Clostridium difficile strains into toxin producers. Nat. Commun. 4:2601. doi: 10.1038/ncomms3601
Camacho, C., Coulouris, G., Avagyan, V., Ma, N., Papadopoulos, J., Bealer, K., et al. (2009). BLAST+: architecture and applications. BMC Bioinform. 10, 1–9. doi: 10.1186/1471-2105-10-421
Cantalapiedra, C. P., Hernández-Plaza, A., Letunic, I., Bork, P., and Huerta-Cepas, J. (2021). eggNOG-mapper v2: functional annotation, Orthology assignments, and domain prediction at the metagenomic scale. Mol. Biol. Evol. 38, 5825–5829. doi: 10.1093/molbev/msab293
Carattoli, A., Zankari, E., García-Fernández, A., Voldby Larsen, M., Lund, O., et al. (2014). In Silico detection and typing of plasmids using PlasmidFinder and plasmid multilocus sequence typing. Antimicrob. Agents Chemother. 58, 3895–3903. doi: 10.1128/AAC.02412-14
Chen, S. (2023). Ultrafast one-pass FASTQ data preprocessing, quality control, and deduplication using fastp. iMeta 2:e107. doi: 10.1002/imt2.107
Chklovski, A., Parks, D. H., Woodcroft, B. J., and Tyson, G. W. (2023). CheckM2: a rapid, scalable and accurate tool for assessing microbial genome quality using machine learning. Nat. Methods 20, 1203–1212. doi: 10.1038/s41592-023-01940-w
Czepiel, J., Dróżdż, M., Pituch, H., Kuijper, E. J., Perucki, W., Mielimonka, A., et al. (2019). Clostridium difficile infection: review. Eur. J. Clin. Microbiol. Infect. Dis. 38, 1211–1221. doi: 10.1007/s10096-019-03539-6
Dapa, T., and Unnikrishnan, M. (2013). Biofilm formation by Clostridium difficile. Gut Microbes 4, 397–402. doi: 10.4161/gmic.25862
Darling, A. C. E., Mau, B., Blattner, F. R., and Perna, N. T. (2004). Mauve: multiple alignment of conserved genomic sequence with rearrangements. Genome Res. 14, 1394–1403. doi: 10.1101/gr.2289704
de la Cruz, F., and Davies, J. (2000). Horizontal gene transfer and the origin of species: lessons from bacteria. Trends Microbiol. 8, 128–133. doi: 10.1016/S0966-842X(00)01703-0
Dingle, K. E., Didelot, X., Quan, T. P., Eyre, D. W., Stoesser, N., Marwick, C. A., et al. (2019). A role for tetracycline selection in recent evolution of agriculture-associated Clostridium difficile PCR Ribotype 078. MBio 10, e02790–e02718. doi: 10.1128/mBio.02790-18
Dong, D., Chen, X., Jiang, C., Zhang, L., Cai, G., Han, L., et al. (2014). Genetic analysis of Tn916-like elements conferring tetracycline resistance in clinical isolates of Clostridium difficile. Int. J. Antimicrob. Agents 43, 73–77. doi: 10.1016/j.ijantimicag.2013.09.004
Dong, Q., Lin, H., Allen, M.-M., Garneau, J. R., Sia, J. K., Smith, R. C., et al. (2023). Virulence and genomic diversity among clinical isolates of ST1 (BI/NAP1/027) Clostridioides difficile. Cell Rep. 42:112861. doi: 10.1016/j.celrep.2023.112861
Feldgarden, M., Brover, V., Gonzalez-Escalona, N., Frye, J. G., Haendiges, J., et al. (2021). AMRFinderPlus and the reference gene catalog facilitate examination of the genomic links among antimicrobial resistance, stress response, and virulence. Sci. Rep. 11:12728. doi: 10.1038/s41598-021-91456-0
Gerding, D. N., Johnson, S., Rupnik, M., and Aktories, K. (2014). Clostridium difficile binary toxin CDT. Gut Microbes 5, 15–27. doi: 10.4161/gmic.26854
Ghigo, J.-M. (2001). Natural conjugative plasmids induce bacterial biofilm development. Nature 412, 442–445. doi: 10.1038/35086581
Gilchrist, C. L. M., and Chooi, Y.-H. (2021). Clinker & clustermap.Js: automatic generation of gene cluster comparison figures. Bioinformatics 37, 2473–2475. doi: 10.1093/bioinformatics/btab007
Goh, S., Hussain, H., Chang, B. J., Emmett, W., Riley, T. V., and Mullany, P. (2013). Phage ϕC2 mediates transduction of Tn 6215, encoding erythromycin resistance, between Clostridium difficile strains. MBio 4, 1–7. doi: 10.1128/mBio.00840-13
Govind, R., and Dupuy, B. (2012). Secretion of Clostridium difficile toxins a and B requires the Holin-like protein TcdE. PLoS Pathog. 8:e1002727. doi: 10.1371/journal.ppat.1002727
Govind, R., Vediyappan, G., Rolfe, R. D., Dupuy, B., and Fralick, J. A. (2009). Bacteriophage-mediated toxin gene regulation in Clostridium difficile. J. Virol. 83, 12037–12045. doi: 10.1128/JVI.01256-09
Grant, J. R., Enns, E., Marinier, E., Mandal, A., Herman, E. K., Chen, C., et al. (2023). Proksee: in-depth characterization and visualization of bacterial genomes. Nucleic Acids Res. 51, W484–W492. doi: 10.1093/nar/gkad326
Hargreaves, K. R., Otieno, J. R., Thanki, A., Blades, M. J., Millard, A. D., Browne, H. P., et al. (2015). As clear as mud? Determining the diversity and prevalence of prophages in the draft genomes of estuarine isolates of Clostridium difficile. Genome Biol. Evol. 7, 1842–1855. doi: 10.1093/gbe/evv094
Hunt, M., De Silva, N., Otto, T. D., Parkhill, J., Keane, J. A., and Harris, S. R. (2015). Circlator: automated circularization of genome assemblies using long sequencing reads. Genome Biol. 16:294. doi: 10.1186/s13059-015-0849-0
Janezic, S., Potocnik, M., Zidaric, V., and Rupnik, M. (2016). Highly divergent Clostridium difficile strains isolated from the environment. PLoS One 11:e0167101. doi: 10.1371/journal.pone.0167101
Ji, X., Sun, Y., Liu, J., Zhu, L., Guo, X., Lang, X., et al. (2016). A novel virulence-associated protein, vapE, in Streptococcus suis serotype 2. Mol. Med. Rep. 13, 2871–2877. doi: 10.3892/mmr.2016.4818
Jolley, K. A., Bray, J. E., and Maiden, M. C. J. (2018). Open-access bacterial population genomics: BIGSdb software, the PubMLST.org website and their applications. Wellcome Open Res. 3:124. doi: 10.12688/wellcomeopenres.14826.1
Kartalidis, P., Skoulakis, A., Tsilipounidaki, K., Florou, Z., Petinaki, E., and Fthenakis, G. C. (2021). Clostridioides difficile as a dynamic vehicle for the dissemination of antimicrobial-resistance determinants: review and in Silico analysis. Microorganisms 9:1383. doi: 10.3390/microorganisms9071383
Knetsch, C. W., Connor, T. R., Mutreja, A., van Dorp, S. M., Sanders, I. M., Browne, H. P., et al. (2014). Whole genome sequencing reveals potential spread of Clostridium difficile between humans and farm animals in the Netherlands, 2002 to 2011. Eur. Secur. 19:20954. doi: 10.2807/1560-7917.ES2014.19.45.20954
Knight, D. R., Imwattana, K., Kullin, B., Guerrero-Araya, E., Paredes-Sabja, D., Didelot, X., et al. (2021). Major genetic discontinuity and novel toxigenic species in Clostridioides difficile taxonomy. eLife 10:e64325. doi: 10.7554/eLife.64325
Knight, D. R., Squire, M. M., Collins, D. A., and Riley, T. V. (2017). Genome analysis of Clostridium difficile PCR Ribotype 014 lineage in Australian pigs and humans reveals a diverse genetic repertoire and signatures of long-range interspecies transmission. Front. Microbiol. 7:2138. doi: 10.3389/fmicb.2016.02138
Koboldt, D. C., Zhang, Q., Larson, D. E., Shen, D., McLellan, M. D., Lin, L., et al. (2012). VarScan 2: somatic mutation and copy number alteration discovery in cancer by exome sequencing. Genome Res. 22, 568–576. doi: 10.1101/gr.129684.111
Kolmogorov, M., Yuan, J., Lin, Y., and Pevzner, P. A. (2019). Assembly of long, error-prone reads using repeat graphs. Nat. Biotechnol. 37, 540–546. doi: 10.1038/s41587-019-0072-8
Kurtz, S., Phillippy, A., Delcher, A. L., Smoot, M., Shumway, M., Antonescu, C., et al. (2004). Versatile and open software for comparing large genomes. Genome Biol. 5:R12. doi: 10.1186/gb-2004-5-2-r12
Lanis, J. M., Heinlen, L. D., James, J. A., and Ballard, J. D. (2013). Clostridium difficile 027/BI/NAP1 encodes a Hypertoxic and antigenically variable form of TcdB. PLoS Pathog. 9:e1003523. doi: 10.1371/journal.ppat.1003523
Lao, J., Lacroix, T., Guédon, G., Coluzzi, C., Payot, S., Leblond-Bourget, N., et al. (2022). ICEscreen: a tool to detect Firmicute ICEs and IMEs, isolated or enclosed in composite structures. NAR Genom. Bioinform. 4:lqac079. doi: 10.1093/nargab/lqac079
Lécuyer, F., Bourassa, J.-S., Gélinas, M., Charron-Lamoureux, V., Burrus, V., and Beauregard, P. B. (2018). Biofilm formation drives transfer of the conjugative element ICE Bs1 in Bacillus subtilis. mSphere 3:e00473. doi: 10.1128/mSphere.00473-18
Lewis, B. B., Carter, R. A., Ling, L., Leiner, I., Taur, Y., Kamboj, M., et al. (2017). Pathogenicity locus, Core genome, and accessory gene contributions to Clostridium difficile virulence. MBio 8, e00885–e00817. doi: 10.1128/mBio.00885-17
Li, H., and Durbin, R. (2010). Fast and accurate long-read alignment with Burrows-wheeler transform. Bioinformatics 26, 589–595. doi: 10.1093/bioinformatics/btp698
Liu, B., Zheng, D., Zhou, S., Chen, L., and Yang, J. (2022). VFDB 2022: a general classification scheme for bacterial virulence factors. Nucleic Acids Res. 50, D912–D917. doi: 10.1093/nar/gkab1107
López de Egea, G., González-Díaz, A., Guédon, G., Lao, J., Berbel, D., Casabella, A., et al. (2023). A new integrative and Mobilizable element is a major contributor to tetracycline resistance in Streptococcus dysgalactiae subsp. equisimilis. Antibiotics 12:579. doi: 10.3390/antibiotics12030579
Mani, N., and Dupuy, B. (2001). Regulation of toxin synthesis in Clostridium difficile by an alternative RNA polymerase sigma factor. Proc. Natl. Acad. Sci. 98, 5844–5849. doi: 10.1073/pnas.101126598
Martínez-Meléndez, A., Cruz-López, F., Morfin-Otero, R., Maldonado-Garza, H. J., and Garza-González, E. (2022). An update on Clostridioides difficile binary toxin. Toxins (Basel). 14:305. doi: 10.3390/toxins14050305
Matamouros, S., England, P., and Dupuy, B. (2007). Clostridium difficile toxin expression is inhibited by the novel regulator TcdC. Mol. Microbiol. 64, 1274–1288. doi: 10.1111/j.1365-2958.2007.05739.x
Mehner-Breitfeld, D., Rathmann, C., Riedel, T., Just, I., Gerhard, R., Overmann, J., et al. (2018). Evidence for an adaptation of a phage-derived holin/endolysin system to toxin transport in Clostridioides difficile. Front. Microbiol. 9:2446. doi: 10.3389/fmicb.2018.02446
Monot, M., Eckert, C., Lemire, A., Hamiot, A., Dubois, T., and Tessier, C. (2015). Clostridium difficile: new insights into the evolution of the pathogenicity locus. Sci. Rep. 5:15023. doi: 10.1038/srep15023
Mullany, P., Allan, E., and Roberts, A. P. (2015). Mobile genetic elements in Clostridium difficile and their role in genome function. Res. Microbiol. 166, 361–367. doi: 10.1016/j.resmic.2014.12.005
Ozaki, E., Kato, H., Kita, H., Karasawa, T., Maegawa, T., Koino, Y., et al. (2004). Clostridium difficile colonization in healthy adults: transient colonization and correlation with enterococcal colonization. J. Med. Microbiol. 53, 167–172. doi: 10.1099/jmm.0.05376-0
Page, A. J., Cummins, C. A., Hunt, M., Wong, V. K., Reuter, S., Holden, M. T. G., et al. (2015). Roary: rapid large-scale prokaryote pan genome analysis. Bioinformatics 31, 3691–3693. doi: 10.1093/bioinformatics/btv421
Parter, M., Kashtan, N., and Alon, U. (2007). Environmental variability and modularity of bacterial metabolic networks. BMC Evol. Biol. 7:169. doi: 10.1186/1471-2148-7-169
Phosphate-buffered saline (PBS) (2006). Phosphate-buffered saline. Cold spring Harb. Protoc. :pdb.rec8247. doi: 10.1101/pdb.rec8247
Ramírez-Vargas, G., Goh, S., and Rodríguez, C. (2018). The novel phages phiCD5763 and phiCD2955 represent two groups of big Plasmidial Siphoviridae phages of Clostridium difficile. Front. Microbiol. 9:26. doi: 10.3389/fmicb.2018.00026
Razavi, M., Kristiansson, E., Flach, C.-F., and Larsson, D. G. J. (2020). The association between insertion sequences and antibiotic resistance genes. mSphere 5:20. doi: 10.1128/mSphere.00418-20
Reynolds, C. B., Emerson, J. E., de la Riva, L., Fagan, R. P., and Fairweather, N. F. (2011). The Clostridium difficile Cell Wall protein CwpV is antigenically variable between strains, but exhibits conserved aggregation-promoting function. PLoS Pathog. 7:e1002024. doi: 10.1371/journal.ppat.1002024
Riedel, T., Wetzel, D., Hofmann, J. D., Plorin, S. P. E. O., Dannheim, H., Berges, M., et al. (2017a). High metabolic versatility of different toxigenic and non-toxigenic Clostridioides difficile isolates. Int. J. Med. Microbiol. 307, 311–320. doi: 10.1016/j.ijmm.2017.05.007
Riedel, T., Wittmann, J., Bunk, B., Schober, I., Spröer, C., Gronow, S., et al. (2017b). A Clostridioides difficile bacteriophage genome encodes functional binary toxin-associated genes. J. Biotechnol. 250, 23–28. doi: 10.1016/j.jbiotec.2017.02.017
RStudio Team (2020). RStudio: integrated development environment for R. Available at: http://www.rstudio.com/ (Accessed May 11, 2024)
Schüler, M. A., Schneider, D., Poehlein, A., and Daniel, R. (2023). Direct and culture-independent detection of low-abundant Clostridioides difficile in environmental DNA via PCR. bioRxiv :392. doi: 10.1101/2023.07.24.550392
Sebaihia, M., Wren, B. W., Mullany, P., Fairweather, N. F., Minton, N., Stabler, R., et al. (2006). The multidrug-resistant human pathogen Clostridium difficile has a highly mobile, mosaic genome. Nat. Genet. 38, 779–786. doi: 10.1038/ng1830
Seemann, T. (2014). Prokka: rapid prokaryotic genome annotation. Bioinformatics 30, 2068–2069. doi: 10.1093/bioinformatics/btu153
Sekulovic, O., Meessen-Pinard, M., and Fortier, L.-C. (2011). Prophage-stimulated toxin production in Clostridium difficile NAP1/027 Lysogens. J. Bacteriol. 193, 2726–2734. doi: 10.1128/JB.00787-10
Smits, W. K., Roseboom, A. M., and Corver, J. (2022). Plasmids of Clostridioides difficile. Curr. Opin. Microbiol. 65, 87–94. doi: 10.1016/j.mib.2021.10.016
Spigaglia, P. (2016). Recent advances in the understanding of antibiotic resistance in Clostridium difficile infection. Ther. Adv. Infect. Dis. 3, 23–42. doi: 10.1177/2049936115622891
Spigaglia, P., Barbanti, F., and Mastrantonio, P. (2007). Detection of a genetic linkage between genes coding for resistance to tetracycline and erythromycin in Clostridium difficile. Microb. Drug Resist. 13, 90–95. doi: 10.1089/mdr.2007.723
Spigaglia, P., Carucci, V., Barbanti, F., and Mastrantonio, P. (2005). ErmB determinants and Tn 916 -like elements in clinical isolates of Clostridium difficile. Antimicrob. Agents Chemother. 49, 2550–2553. doi: 10.1128/AAC.49.6.2550-2553.2005
Stabler, R. A., He, M., Dawson, L., Martin, M., Valiente, E., Corton, C., et al. (2009). Comparative genome and phenotypic analysis of Clostridium difficile 027 strains provides insight into the evolution of a hypervirulent bacterium. Genome Biol. 10:R102. doi: 10.1186/gb-2009-10-9-r102
Stojković, V., Ulate, M. F., Hidalgo-Villeda, F., Aguilar, E., Monge-Cascante, C., Pizarro-Guajardo, M., et al. (2019). Cfr(B), cfr(C), and a new cfr-like gene, cfr(E), in Clostridium difficile strains recovered across Latin America. Antimicrob. Agents Chemother. 64, e01074–e01019. doi: 10.1128/AAC.01074-19
Thomas, P., Abdel-Glil, M. Y., Eichhorn, I., Semmler, T., Werckenthin, C., Baumbach, C., et al. (2021). Genome sequence analysis of Clostridium chauvoei strains of European origin and evaluation of typing options for outbreak investigations. Front. Microbiol. 12:732106. doi: 10.3389/fmicb.2021.732106
Tulli, L., Marchi, S., Petracca, R., Shaw, H. A., Fairweather, N. F., Scarselli, M., et al. (2013). CbpA: a novel surface exposed adhesin of Clostridium difficile targeting human collagen. Cell. Microbiol. 15, 1674–1687. doi: 10.1111/cmi.12139
van Leeuwen, H. C., Roelofs, D., Corver, J., and Hensbergen, P. (2021). Phylogenetic analysis of the bacterial pro-pro-endopeptidase domain reveals a diverse family including secreted and membrane anchored proteins. Curr. Res. Microb. Sci. 2:100024. doi: 10.1016/j.crmicr.2021.100024
Walker, A. S., Eyre, D. W., Wyllie, D. H., Dingle, K. E., Griffiths, D., Shine, B., et al. (2013). Relationship between bacterial strain type, host biomarkers, and mortality in Clostridium difficile infection. Clin. Infect. Dis. 56, 1589–1600. doi: 10.1093/cid/cit127
Wang, S., Heuler, J., Wickramage, I., and Sun, X. (2022). Genomic and phenotypic characterization of the nontoxigenic Clostridioides difficile strain CCUG37785 and demonstration of its therapeutic potential for the prevention of C. difficile infection. Microbiol. Spectr. 10:e0178821. doi: 10.1128/spectrum.01788-21
Weese, J. S. (2020). Clostridium (Clostridioides) difficile in animals. J. Vet. Diagnostic Investig. 32, 213–221. doi: 10.1177/1040638719899081
Wick, R. R., and Holt, K. E. (2022). Polypolish: short-read polishing of long-read bacterial genome assemblies. PLoS Comput. Biol. 18:e1009802. doi: 10.1371/journal.pcbi.1009802
Wick, R. R., Schultz, M. B., Zobel, J., and Holt, K. E. (2015). Bandage: interactive visualization of de novo genome assemblies. Bioinformatics 31, 3350–3352. doi: 10.1093/bioinformatics/btv383
Wishart, D. S., Han, S., Saha, S., Oler, E., Peters, H., Grant, J. R., et al. (2023). PHASTEST: faster than PHASTER, better than PHAST. Nucleic Acids Res. 51, W443–W450. doi: 10.1093/nar/gkad382
Xie, Z., and Tang, H. (2017). ISEScan: automated identification of insertion sequence elements in prokaryotic genomes. Bioinformatics 33, 3340–3347. doi: 10.1093/bioinformatics/btx433
Xu, X., Bian, Q., Luo, Y., Song, X., Lin, S., Chen, H., et al. (2021). Comparative whole genome sequence analysis and biological features of Clostridioides difficile sequence type 2‡. Front. Microbiol. 12:651520. doi: 10.3389/fmicb.2021.651520
Zhou, Q., Rao, F., Chen, Z., Cheng, Y., Zhang, Q., Zhang, J., et al. (2022). The cwp66 gene affects cell adhesion, stress tolerance, and antibiotic resistance in Clostridioides difficile. Microbiol. Spectr. 10:e0270421. doi: 10.1128/spectrum.02704-21
Keywords: Clostridioide difficile, virulence, mobile genetic element, clinical, non-clinical, genome comparison
Citation: Schüler MA, Riedel T, Overmann J, Daniel R and Poehlein A (2024) Comparative genome analyses of clinical and non-clinical Clostridioides difficile strains. Front. Microbiol. 15:1404491. doi: 10.3389/fmicb.2024.1404491
Edited by:
Lígia M. Saraiva, Universidade Nova de Lisboa, PortugalReviewed by:
Dhruv Desai, University of Pennsylvania, United StatesCopyright © 2024 Schüler, Riedel, Overmann, Daniel and Poehlein. This is an open-access article distributed under the terms of the Creative Commons Attribution License (CC BY). The use, distribution or reproduction in other forums is permitted, provided the original author(s) and the copyright owner(s) are credited and that the original publication in this journal is cited, in accordance with accepted academic practice. No use, distribution or reproduction is permitted which does not comply with these terms.
*Correspondence: Anja Poehlein, YXBvZWhsZTNAZ3dkZy5kZQ==
Camila Fernandes, National Health Institute Doutor Ricardo Jorge (INSA), Portugal