- 1State Key Laboratory of Swine and Poultry Breeding Industry, College of Animal Science and Technology, Sichuan Agricultural University, Chengdu, China
- 2Chongqing Academy of Animal Sciences, Chongqing, China
- 3National Center of Technology Innovation for Pigs, Chongqing, China
- 4Ministry of Agriculture Key Laboratory of Pig Sciences, Chongqing Key Laboratory of Pig Sciences, Chongqing, China
- 5College of Engineering, Peking University, Beijing, China
Pigs are widely used as animal models in various studies related to humans. The interaction between the gut microbiota and the host has significant effects on the host’s health and disease status. However, although there have been many studies investigating the pig gut microbiota, the findings have been inconsistent due to variations in rearing conditions. Interactions between the gut microbiota and host have not been fully explored in pigs. Specific pathogen-free (SPF) pigs are ideal non-primate large animals to study the interactions between the gut microbiota and the host. In this study, we performed high-throughput sequencing analysis of the gut microbiota and the gut tissue transcriptome of six SPF pigs to provide a systematic understanding of the composition, function, and spatial distribution of gut microbiota in SPF pigs. We identified significant differences in microbial diversity and functionality among different gastrointestinal tract sites. Metagenomics data analysis revealed significant differences in alpha diversity and beta diversity of microbiota in different gastrointestinal sites of SPF pigs. Additionally, transcriptomic data indicated significant differences in gene expression as well as KEGG and GO functional enrichment between the small intestine and large intestine. Furthermore, by combining microbial metagenomics and host transcriptomics analyses, specific correlations were found between gut microbiota and host genes. These included a negative correlation between the TCN1 gene and Prevotella dentalis, possibly related to bacterial metabolic pathways involving vitamin B12, and a positive correlation between the BDH1 gene and Roseburia hominis, possibly because both are involved in fatty acid metabolism. These findings lay the groundwork for further exploration of the co-evolution between the microbiota and the host, specifically in relation to nutrition, metabolism, and immunity. In conclusion, we have elucidated the diversity of the gut microbiota in SPF pigs and conducted a detailed investigation into the interactions between the gut microbiota and host gene expression. These results contribute to our understanding of the intricate dynamics between the gut microbiota and the host, offering important references for advancements in life science research, bioproduct production, and sustainable development in animal husbandry.
Introduction
Animal models play a significant role in studying human development and disease, and in the search for effective therapies and vaccines. Compared with rodent models, pigs are closer to humans in anatomical size and structure, and in immunological, genomic, and physiological processes (Lunney, 2007; Meurens et al., 2012; Swindle et al., 2012; Gerdts et al., 2015; Gutierrez et al., 2015; Klymiuk et al., 2016; Pabst, 2020), making them advantageous for translational and clinical research applications. Additionally, pigs have several advantages over primate models and other animal models, including shorter generational intervals, larger litter sizes, and ease of genome editing. Therefore, pigs hold tremendous potential for the generation of biomedical models to study human developmental processes (Swindle et al., 1996; Ferenc et al., 2014; Jiang et al., 2019), congenital diseases (Hu et al., 2016; Perleberg et al., 2018), and pathogen response mechanisms (Amaral et al., 2020; Ramos et al., 2020).
Recent studies using model pigs have generally used animals raised under conventional (farm) conditions (Qiao et al., 2021; Song et al., 2022; Narduzzi et al., 2023; Rupp et al., 2023). However, research on the gut microbiota of farm-raised pigs can be influenced by various factors, such as pathogen contamination, dietary differences, environmental factors, and the use of drugs and antibiotics. Specific pathogen-free (SPF) pigs are raised in an environment free from pathogenic microorganisms, resulting in a relatively stable and clean gut microbial community. Compared with germ-free and gnotobiotic pigs, SPF pigs have lower housing facility requirements but these are still higher than those used for conventional rearing (Safron and Gonder, 1997). The gut microbiota plays a critical role in host immune training (Donald and Finlay, 2023; Koren et al., 2023), food digestion (Caminero et al., 2019; García-Pérez et al., 2024), regulation of intestinal endocrine function and neural signal transmission (Mazzoli and Pessione, 2016; Zhao et al., 2020), drug action and metabolism (Wilson and Nicholson, 2017; Kolli and Roy, 2023), toxin clearance (Wang et al., 2019), and production of various compounds that can influence the host (Kudjordjie et al., 2019; Tennoune et al., 2022). Pigs share many similarities with humans in terms of gut microbiota composition and function (Roura et al., 2016), making SPF pigs an ideal non-primate large animal model for studying the interaction between gut microbiota and the host (Zhou et al., 2021). Although research into the gut microbiota of pigs has progressed rapidly in recent years, resulting in the construction and improvement of pig gut microbiota catalogs (Xiao et al., 2016; Chen et al., 2021a), there are no unified standard procedures and these studies have used different rearing conditions and pig breeds, which has led to inconsistent results (Yang et al., 2016; Holman et al., 2017; Wang et al., 2019; Chen et al., 2021b). Additionally, the interaction between the gut microbiota and host gene expression has not been fully considered. Therefore, there is an urgent need to establish a standardized gut microbiota profile of SPF pigs as an important reference for the livestock industry, life science research, and bioproduct production.
Here, we collected the gastrointestinal tract (GIT) contents and intestinal tissues of SPF Bama females in which the gut microbiota had reached a stable and plateau phase (Frese et al., 2015; Dong et al., 2023), and performed high-throughput sequencing analysis. We comprehensively revealed the diversity and functional differences of the gut microbiota in different GIT sites of SPF pigs. Furthermore, we investigated potential functional interactions between the gut microbiota and the host. This provides a foundation for further research into the co-evolutionary mechanisms between microbiota and the host. In summary, through in-depth investigation of changes in gut microbiota composition, function, and host gene expression, a better understanding of gut microbiota ecology in SPF pigs can be determined and the interaction between the gut microbiota and the host can be explored to provide a scientific basis for improving pig health and productivity.
Materials and methods
Ethics approval
This study was approved by the Experimental Animal Ethics Committee, Chongqing Academy of Animal Science, under reference number XKY-No. 20210606.
Sample collection
To investigate differences in the gut microbiota of SPF pigs and their interaction with the host, we selected Bama females provided by Chongqing Academy of Animal Science (Chongqing, China) that were disease-free, unrelated, in good physical condition, and normal in appearance. These females were pregnant and tested negative for the placental transmission diseases, African swine fever, porcine pseudorabies, porcine parvovirus disease, and porcine reproductive and respiratory syndrome, and for other serum-specific pathogens, such as African swine fever, foot-and-mouth disease, Japanese encephalitis, porcine circovirus, swine influenza, and porcine brucellosis.
Three days before the expected delivery date, piglets were delivered through aseptic cesarean section. Six female piglets were randomly selected as subjects for this study (Figure 1). These piglets were reared following the SPF pig model construction and rearing standards (Zhang et al., 2023) on the standardized aseptic pig breeding platform at the Institute of Biotechnology, Chongqing Academy of Animal Science. In summary, SPF piglets were fed with sterilized milk powder (0–21 days) and pelleted feed (21–42 days) diluted 1:4 with sterile water and sterilized using Co60 γ radiation. During the feeding process, milk powder, feed, and water were introduced into the isolator through a transfer tube and disinfected with 1% peracetic acid to prevent microbial contamination.
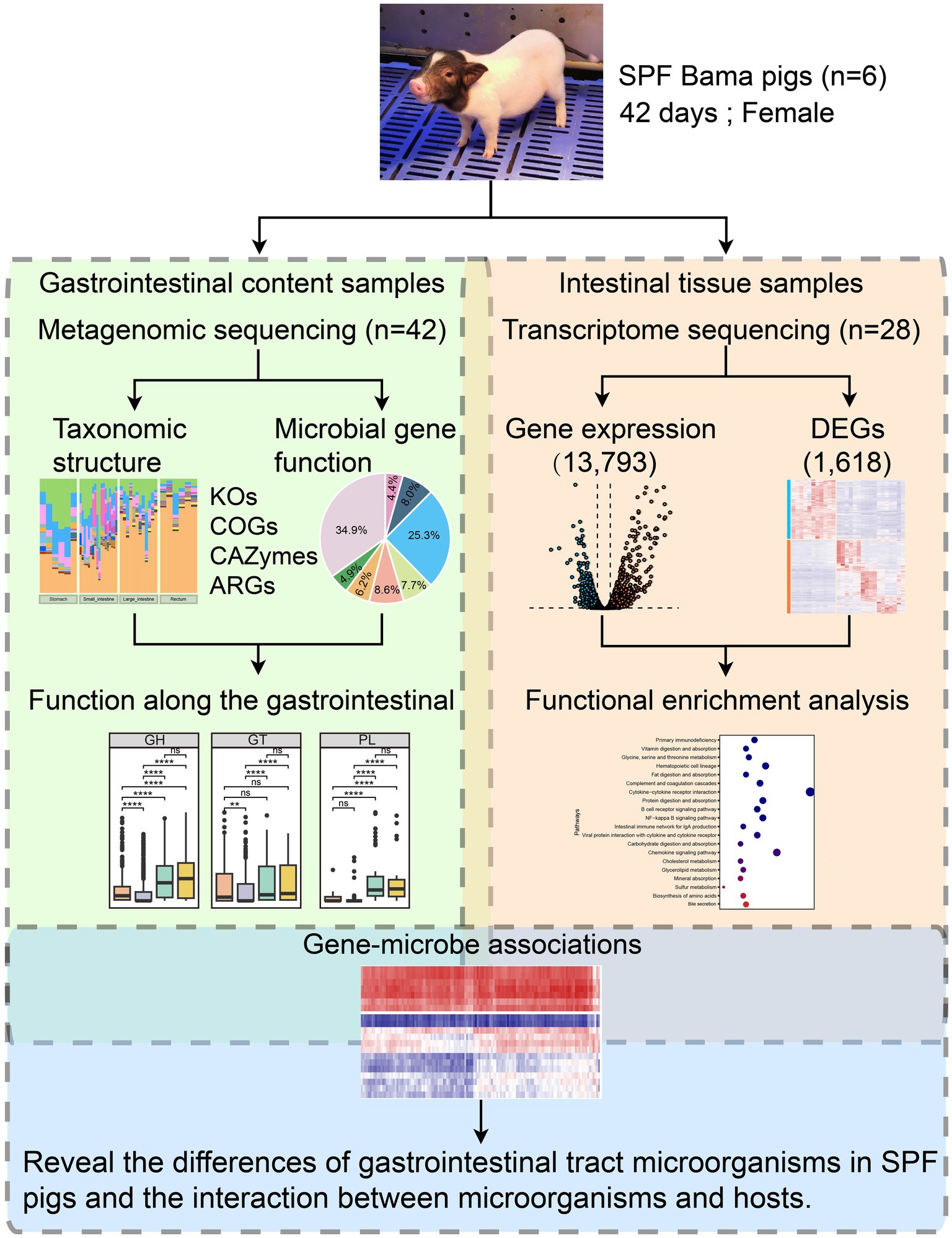
Figure 1. Overview of the integrated multi-omics workflow combining metagenomics and transcriptomics. We selected six 42-day-old SPF Bama females as the study subjects and extracted gastrointestinal content samples (n = 42) and intestinal tissue samples (n = 28) for metagenomic and transcriptomic sequencing. The metagenomic data underwent microbial taxonomic structure analysis (including diversity and taxonomic composition) and microbial gene functional analysis (including KEGG Orthology and Cluster of Orthologous Groups analyses, and identification of CAZymes and antimicrobial resistance genes) to study the functional profiles along the GIT. The transcriptome data underwent gene expression analysis (with 13,793 genes expressed in intestinal tissues) and DEG analysis (with 1,618 genes identified as DEGs). Functional enrichment analysis was performed on the DEGs. Finally, correlation analysis was conducted among the 20 most abundant species in the SPF pig gut and the 130 most highly up-and down-regulated DEGs in the small intestine (after converting human genes to pig homologs) to understand differences in the gut microbiota and the microbial-host gene interactions in SPF pigs.
After feeding for 42 days, in order to comprehensively study the gut microbial characteristics of SPF pigs and their interactions with the host, we collected a total of 42 GIT content samples and 28 intestinal tissue samples from 6 SPF Bama females at the Chongqing Academy of Animal Sciences. Among them, the GIT content samples included samples from four different sites, including 6 stomach samples, 18 small intestine samples (duodenum, jejunum, and ileum), 12 large intestine samples (cecum and colon), and 6 rectum samples. The intestinal tissue samples consisted of 17 small intestine samples (duodenum, jejunum, and ileum) and 11 large intestine samples (cecum and colon) (Supplementary Tables S1, S2). GIT contents and intestinal tissue samples from the SPF pigs were collected and stored at −80°C prior to sequencing.
Community analysis of the GIT microbiome
Total DNA was extracted from GIT content samples using a QIAamp DNA Stool Mini Kit (Qiagen, Germany), following the manufacturer’s instructions. Each sample yielded 0.2 μg of total DNA, which was used as input material for DNA library preparation. Briefly, genomic DNA samples were fragmented by sonication to an average size of 350 bp. The DNA fragments then underwent end-polishing, A-tailing, and ligation with full-length adapters for Illumina sequencing, followed by PCR amplification. The PCR products were purified using the AMPure XP system from Beverly. Next, we assessed the quality of the libraries using the Agilent 5400 system and quantified by QPCR (1.5 nM). Based on the effective library concentration and the required amount of data, qualified libraries were pooled and sent to Novogene Bioinformatics Technology Co., Ltd. (Beijing, China) for metagenomic sequencing using the Illumina NovaSeq 6000 platform with a paired-end-150 bp strategy, aiming to generate at least 10 G of raw data per sample. Forty-two samples were sequenced, resulting in approximately 448.08 G of raw data. Basic quality processing was performed on the raw data using Fastp (Chen et al., 2018) (v0.23.1) to obtain clean data. The data processing steps were as follows: if either of the paired reads contained adapter contamination, the reads were discarded; if either of the paired reads had more than 10% of bases with uncertainty, the reads were discarded; if the proportion of low-quality bases (Phred quality < 5) in either of the paired reads exceeded 50%, the reads were discarded. To remove reads that might originate from the host or food, we downloaded the reference genome sequence of Sus scrofa (Sus_scrofa.Sscrofa11.1.dna.toplevel.fa) from Ensembl and aligned the sequencing data to the host genome using BWA-MEM (Li, 2013) (v0.7.17). Subsequently, we used SAMtools (Li et al., 2009) (v1.17) to remove host reads. After this step, we obtained approximately 271.94 G of filtered data and 1.866 billion high-quality paired-end filtered reads for subsequent analysis.
The high-quality reads for each sample were assembled using MEGAHIT (Li et al., 2015) (v1.2.9) with the parameter “-min-contig-len 500,” resulting in 446,890 contigs. The assembly quality was assessed using QUAST (Gurevich et al., 2013) (v5.2.0). Next, open reading frames were predicted using metaProdigal (Hyatt et al., 2010) (v2.6.3) with the parameter “-p meta,” resulting in 1,213,609 open reading frames from which 620,325 complete genes were extracted (51.1%, partial = 00; Supplementary Figure S1). Subsequently, CD-HIT (Huang et al., 2010) (v4.8.1) was employed to remove redundancy among the predicted genes and proteins. Clustering was performed using 95% sequence identity and 90% sequence coverage, resulting in the formation of the most similar clusters. The nucleotide sequences were then translated into their corresponding protein sequences. The abundance of non-redundant genes was calculated as transcripts per million (TPM) fragments using Salmon (Patro et al., 2017) (v1.10.1).
To obtain the taxonomic composition of the gut microbiota for each sample, we used Kraken 2 (Lu et al., 2022) (v2.1.3) with the default parameters to align the translated protein sequences against its database (k2_pluspf_20231009). We calculated alpha diversity based on the Shannon index using the vegen package in R (v4.3.1), computed beta diversity distances using Bray–Curtis dissimilarity, and performed species rarefaction analysis using USEARCH (Edgar, 2010) (v10.0) (Figure 1). Differences in the GIT microbiota between SPF pigs were assessed using the Wilcoxon test. Spearman rank correlation was used to calculate the correlation coefficients of the 20 most abundant microorganisms at the species level in the GIT. Only correlations with Spearman correlation coefficient (r) ≥ 0.3 or ≤ −0.3 and p < 0.05 were retained and visualized using R (v4.3.1). Linear discriminant analysis implemented in the LEfSe (Segata et al., 2011) tool was used to identify different taxonomic groups between sample groups. We identified discriminative groups with a linear discriminant analysis score > 3 and assessed the statistical significance of abundance differences between GIT microbiota groups in SPF pigs using the Wilcoxon test.
Functional analysis of the GIT microbiome
We used GhostKOALA (Kanehisa et al., 2016) to map non-redundant protein sequences to the KEGG database for KEGG Orthology annotation. For protein Cluster of Orthologous Groups annotation, we aligned non-redundant protein sequences to the eggNOG database (v5.0.2) using the default parameters of eggNOG-mapper (Cantalapiedra et al., 2021) (v2.1.12). Additionally, we used the BLASTp option of DIAMOND (Buchfink et al., 2015) (v2.0.15) with the parameter “-e 1e-102” to align non-redundant protein sequences to the latest CAZy database (CAZyDB.07262023) for the detection of carbohydrate-active enzymes (CAZymes). To detect antimicrobial resistance genes in each microorganism, we used the RGI (Alcock et al., 2023) (v5.2.0) tool with the option “DIAMOND --include_loose” to align non-redundant protein sequences to the latest CARD database (v3.2.6) (Figure 1).
Transcriptome analysis of intestinal tissues
We extracted total RNA from intestinal samples using the Trizol method. After qualifying the RNA samples, eukaryotic mRNAs were enriched using Oligo(dT) magnetic beads. For prokaryotes, a mRNA purification kit to remove rRNA and enrich mRNA was used. Subsequently, we added a cleavage buffer to break the mRNA into short fragments, which was then used as a template to synthesize single-stranded cDNA using random hexamers. Next, a buffer, dNTPs, DNA polymerase I, and RNase H were added to synthesize double-stranded cDNA, which was then purified using AMPure XP beads. The cDNA then underwent end repair, A-tailing, and sequencing adapter ligation, followed by fragment size selection using AMPure XP beads. Finally, PCR amplification was performed, and the PCR products were purified using AMPure XP beads to obtain the final library. After construction, we quantified the library using Qubit 2.0 and diluted it accordingly. Subsequently, we used the Agilent 2100 system to assess the insert size of the library, ensuring that the insert fragments met the expected size. Next, we used q-PCR to accurately quantify the effective concentration of the library, ensuring library quality. After passing the quality check, the library was sequenced using DNBSEQ-T7.
The data obtained from sequencing is referred to as raw reads. Prior to reference genome alignment, we obtained clean reads by filtering out reads containing adapters (adapter contamination), reads containing unknown bases (N bases), and reads with low sequencing quality. Based on the pig reference genome and annotation files downloaded from Ensembl, we performed alignment of the clean reads using STAR (Dobin et al., 2012) (v2.7.10b), assembly using Cufflinks (Trapnell et al., 2010) (v2.2.1) and TACO (Niknafs et al., 2017) (v0.7.3), and calculation of the protein-coding potential of the assembled transcripts using CPC (Kang et al., 2017) (v2.0). Ultimately, we obtained reference genome files for mRNAs and lncRNAs, which were used for subsequent analysis. We quantified the data using Kallisto (Bray et al., 2016) (v0.49.0) to obtain the counts and TPM values for mRNAs and lncRNAs, which were used for downstream analysis. After calculating expression levels, we grouped the samples based on treatments and conditions. First, we selected expressed genes by choosing genes that were expressed (TPM > 0) in all samples. Subsequently, differential gene expression analysis was conducted using the edgeR package (Chen et al., 2016) in R (v4.3.1). We defined differentially expressed genes (DEGs) as genes with |Log2(FoldChange)| > 1 and FDR.P (false discovery rate adjusted p-value) < 0.05. Kyoto Encyclopedia of Genes and Genomes (KEGG) (Kanehisa and Goto, 2000) and Gene Ontology (GO) (Gene Ontology Consortium, 2007) enrichment analyses were performed using the clusterProfiler package (Wu et al., 2021) (Figure 1). The gene sets used were primarily of mouse or human origin; therefore, we retained only the genes that exhibited one-to-one orthology with pigs.
Comprehensive analysis of the interaction between the host transcriptome and the gut microbiota
To investigate potential functional interactions between DEGs in the host gut and the gut microbiota (top 20 species), we performed Spearman rank correlation analysis. We defined DEGs as genes with |Log2(FoldChange)| > 1 and FDR.P < 0.05. Specifically, we selected the 130 most up-and down-regulated DEGs based on |Log2(FoldChange)| (after converting human genes to pig homologs). Spearman rank correlation, p-values and significant associations (FDR.P < 0.05) between genes were calculated using the corr.test() function from the psych package in R.
Results
Taxonomic characteristics of microbiota at different GIT sites
We used the species annotation software, Kraken2, to classify the non-redundant protein sequences from the GIT of SPF pigs. A total of 269,251 non-redundant proteins (43.4% of total proteins) were annotated into four domains (Bacteria 89.4%, Eukaryota 8.2%, Viruses 1.3%, and Archaea 0.1%; Figure 2A). Sixty-one phyla, 113 classes, 226 orders, 470 families, 1,646 genera, and 5,421 species were annotated (Supplementary Table S3). We then performed rarefaction analysis on the 5,421 annotated species. Moreover, with increasing sequencing depth, the species rarefaction curves obtained from different GIT sites reached a saturation stage (Supplementary Figure S2A).
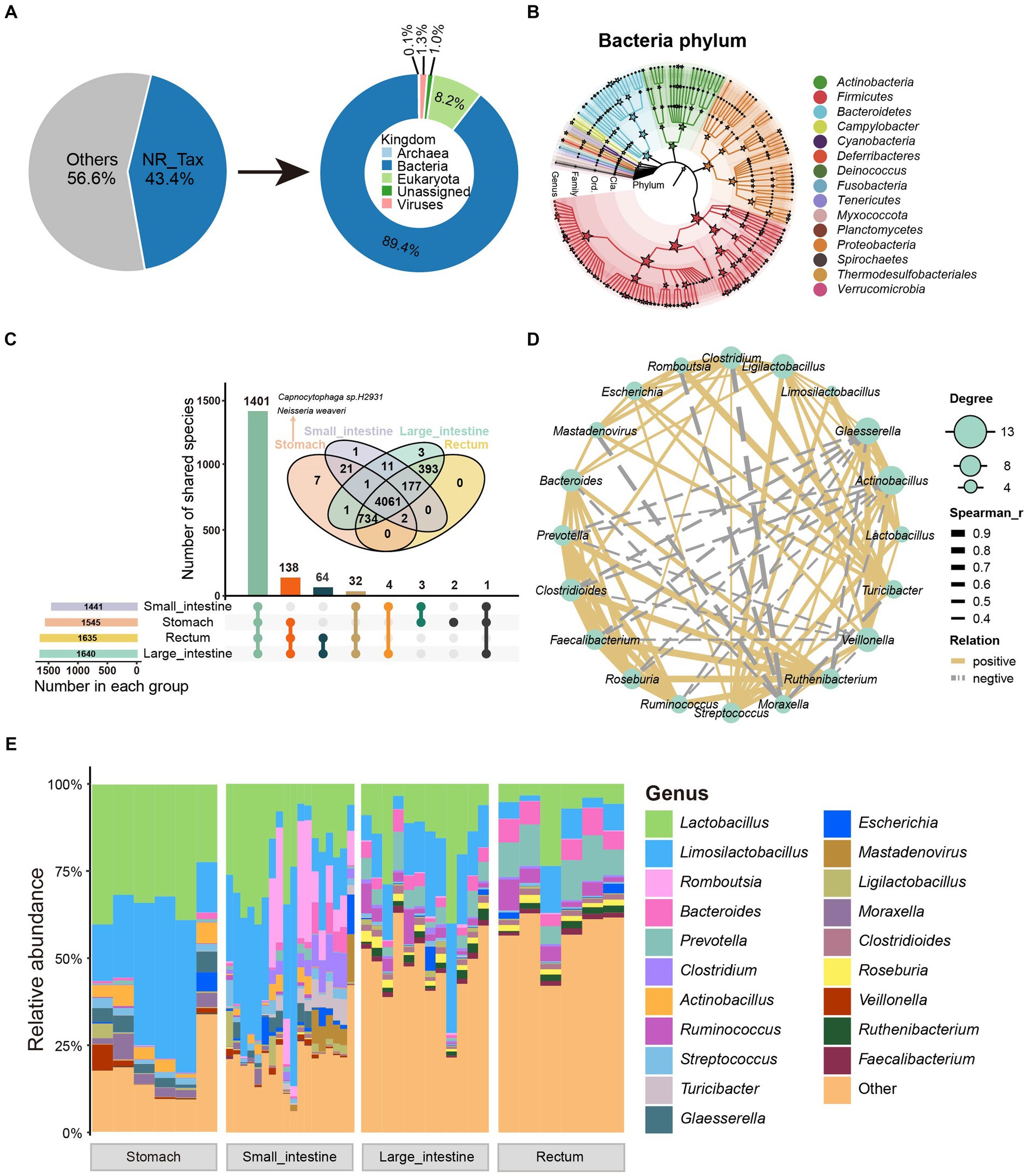
Figure 2. Taxonomic characteristics and co-occurrence network of GIT microbiota in SPF pigs. (A) Taxonomic annotation of microbial proteins in SPF pigs. The pie chart shows the proportion of annotated proteins (left) and the overall composition of the microbiota at the kingdom level (right). (B) The phylogenetic relationships and taxonomic classification of 146 bacterial genera (having >150 mapped reads) in the SPF pig GIT were constructed using GraPhlAn. Stars on internal branches and leaf branches represent branches at each taxonomic level; coloring is based on phylum level branches. (C) Shared and unique relationships of GIT microbiota in SPF pigs at different taxonomic levels. Features are considered to be present in a group only if the total abundance of samples in that group is greater than 0. The UpSet plot shows shared and unique microbiota at the genus level in the GIT of SPF pigs, while the Venn diagram above shows shared and unique microbiota at the species level. (D) Co-occurrence network analysis of microbial genera based on the 20 most abundant genera in the GIT of SPF pigs. Solid lines indicate Spearman rank correlation coefficient > 0.30; dashed lines indicate Spearman rank correlation coefficient < −0.30. Node size is proportional to the degree (the number of connections a node has with other nodes). (E) Genus-level composition of microbiota at different sites along the GIT of SPF pigs. X-axis: different sites (stomach, small intestine, large intestine, and rectum), Y-axis: relative abundance of microbiota.
We then performed statistical analysis on the dominant phyla and genera in the GIT. The dominant phyla were Firmicutes, Proteobacteria, and Bacteroidetes, while the dominant genera were Lactobacillus, Limosilactobacillus, and Romboutsia (Supplementary Figure S2B). Additionally, to demonstrate better, we constructed an evolutionary tree using the selected bacteria after filtering and annotated them to 15 phyla and 146 genera. The four most abundant bacterial phyla (dominant phyla) were Firmicutes, Proteobacteria, Actinobacteria, and Bacteroidetes (Figure 2B), which is consistent with studies conducted on humans and other animals (Xiao et al., 2017; Long et al., 2022; Maiuolo et al., 2022; Yu et al., 2023). To further investigate changes in the relative abundance of microbiota along the GIT, we analyzed the genus-level microbial composition, as shown in Figure 2E. We found that Lactobacillus, Limosilactobacillus, Romboutsia, and Bacteroides were the predominant bacteria in the GIT. These bacteria are considered probiotics and play a significant role in maintaining GIT health of animals (Abuqwider et al., 2022). Both Lactobacillus and Limosilactobacillus exhibited a gradual decrease in abundance moving anally along the GIT (Figure 2E).
Using 0 as the threshold for presence (a feature is considered present in a group only if the total abundance across all samples in that group is greater than the threshold), we found that 1,441, 1,545, 1,635, and 1,640 genera were present in samples from the four GIT sites (stomach, small intestine, large intestine, rectum) (Figure 2C). Additionally, we observed that 1,401 genera (85% of all annotated genera) were present in all four GIT sites, 138 genera (8%) were present in samples from three sites, and only a small fraction of genera showed site-specificity (two in the stomach; Figure 2C). According to these criteria, we did not find any site-specific genera in other sites, possibly because of the similar composition of microbial species along the SPF pig GIT. At the species level, seven showed gastric specificity. Among them, the most abundant were Capnocytophaga sp. H2931 [SUM Abundance (all samples) = 543.11] from the Capnocytophaga genus [a Gram-negative bacterial genus typically found in the oral-pharyngeal cavity of mammals and associated with the pathogenesis of periodontal disease and infection following animal bites (Jolivet-Gougeon et al., 2007)], and Neisseria weaveri [SUM Abundance (all samples) = 395.36] from the Neisseria genus [a Gram-negative bacterial genus colonizing the mucosal surfaces of various animals (Panagea et al., 2002)] (Figure 2C). The reason for their high abundance might result from their advantageous use of nutrients compared with other gastric-specific microorganisms. Alternatively, they may have a close interaction with receptors on the gastric mucosa that interferes with the colonization of other microbes.
We analyzed microbial diversity further and found significant differences in alpha diversity among all GIT sites based on the Shannon index measurement (pairwise Wilcoxon rank-sum test, p < 0.05; Supplementary Figure S3A). The rectum exhibited the highest diversity, followed by the large intestine and small intestine, while the stomach showed the lowest diversity (Supplementary Figure S3A). However, considering different sources, the duodenum displayed the lowest diversity (Supplementary Figure S3B). To further determine the overall microbial characteristics of the four GIT sites, beta diversity comparisons were performed using paired non-parametric multivariate analysis of variance.
Based on principal coordinate analysis (PCoA) of Bray-Curtis distances between samples, the difference between the four GIT sites was extremely significant (R2 = 0.4441, p = 0.001, paired non-parametric multivariate analysis of variance; Supplementary Figure S3C). Interestingly, samples from the cecum, colon, and rectum formed a highly clustered group (Supplementary Figure S3D), indicating strikingly similar microbial community structures in these three sources. This is consistent with their alpha diversity, as there was little difference in alpha diversity among these three sources (Supplementary Figure S3B).
To investigate the interactions among the GIT microbiota of SPF pigs, we selected core genera (average relative abundance >0.5%) and calculated the Spearman rank correlation coefficients, as shown in Figure 2D. Lactobacillus and Limosilactobacillus (the two most abundant genera) exhibited a strong positive correlation (Spearman’s rank correlation coefficient = 0.95, p = 0), indicating a potential mutualistic relationship between them. Similarly, there was a strong positive correlation among the three genera with the highest degree of centrality (Actinobacillus, Glaesserella, and Veillonella) (Figure 2D). In contrast, Moraxella showed a strong negative correlation with Clostridium, Romboutsia, and Mastadenovirus, indicating a potential competitive inhibition effect among them. Additionally, certain beneficial gut bacteria, such as Prevotella, Clostridioides, Faecalibacterium, Roseburia, Ruminococcus, and Ruthenibacterium, exhibited positive correlations with each other, forming a relatively independent and stable cluster (Figure 2D). Overall, the richness and diversity of the SPF pig GIT microbiota gradually increased from the stomach to the rectum. Moreover, these microorganisms formed a stable symbiotic network within the GIT.
In the ileum, we identified the presence of Porcine adenovirus A, which belongs to the phylum Preplasmiviricota (Supplementary Figure S3E). Infection with this virus is usually subclinical in pigs, and when clinical symptoms do occur, they manifest as mild and transient GIT symptoms, such as diarrhea, anorexia, and dehydration, and are most commonly observed in piglets (Liu et al., 2020; Gainor et al., 2022).
Different bacteria along the GIT correspond to GIT functions
The overall microbial structures in the stomach, small intestine, large intestine, and rectum differ from one another. The phyla, Firmicutes and Bacteroidetes, were the two most abundant phyla, accounting for 95% (stomach), 86% (small intestine), 71% (large intestine), and 55% (rectum) of the relative bacterial abundance in the four GIT sites (Supplementary Table S4). Interestingly, the Actinobacteria and Bacteroidetes phyla showed a increasing trend in abundance moving anally along the GIT, while the Firmicutes and Proteobacteria phyla exhibited the opposite pattern (Figures 3A,C). Based on this pattern, the ratio of Firmicutes to Bacteroidetes (F/B) was lowest in the rectum and highest in the stomach. The F/B ratio is associated with energy harvesting (obesity) (Ley et al., 2006; Turnbaugh et al., 2006); therefore, its trend aligns with the physiological changes along the GIT, from food digestion (in the stomach) to energy harvesting (in the intestine).
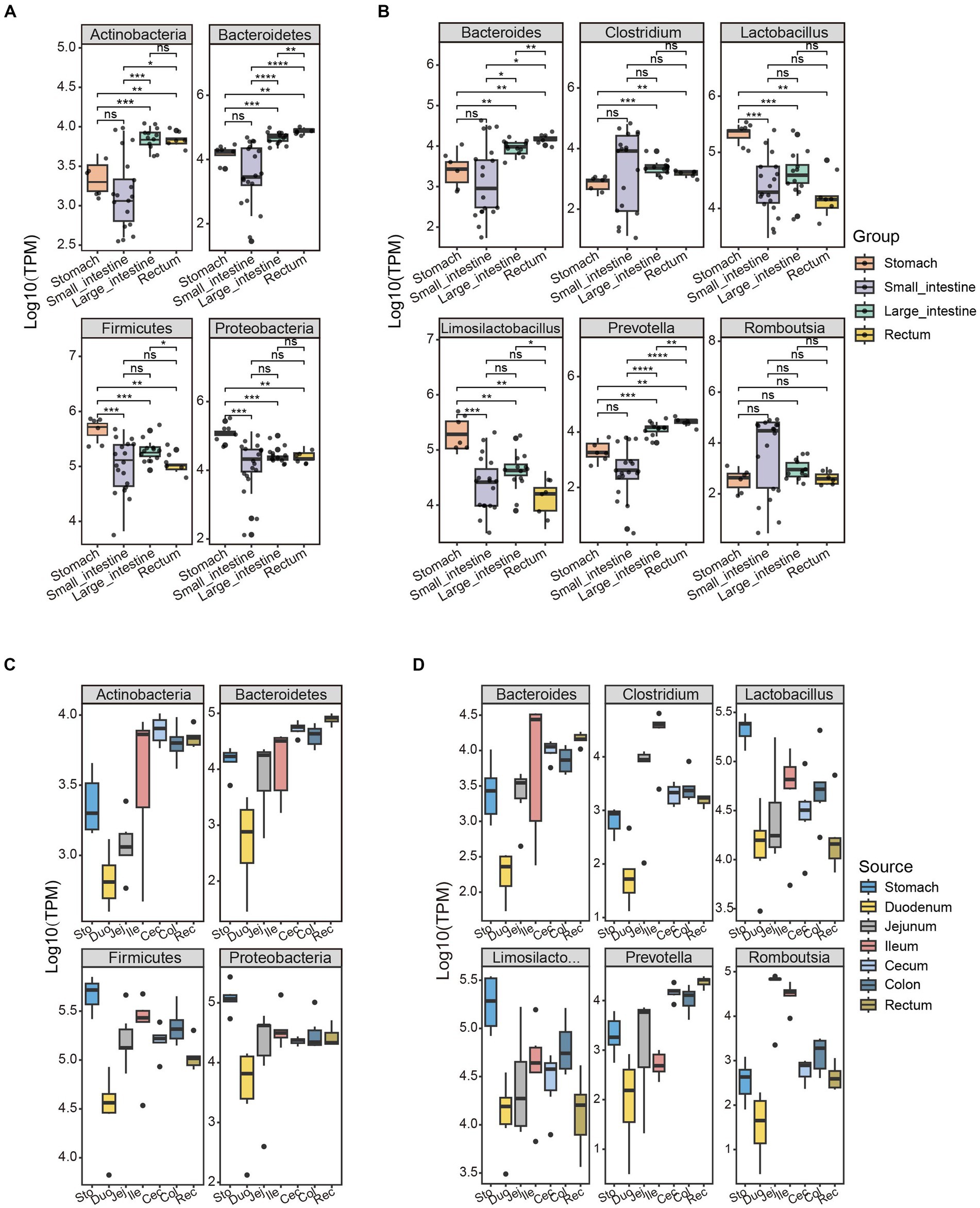
Figure 3. Unique patterns of bacterial abundance in the GIT of SPF pigs are associated with physiological functions. The abundance distribution patterns of the four bacterial phyla with the highest abundance in the GIT of SPF pigs were selected along the (A) Group and (C) Source. The abundance distribution patterns of the six bacterial genera with the highest abundance in the GIT of SPF pigs were selected along the (B) Group and (D) Source. Y-axis: TPM values transformed by logarithm base 10. The different colors of the boxes represent different parts of the GIT: stomach (orange, n = 6), small intestine (purple, n = 18), large intestine (green, n = 12), and rectum (yellow, n = 6). Pairwise Wilcoxon rank-sum tests were used to compare the groups. The boxplots display the median, 25th and 75th percentiles; solid lines represent the minimum and maximum values, and points falling outside the whiskers of the boxplot represent outliers. Significance levels: nsp ≥ 0.05, *p < 0.05, **p < 0.01, ***p < 0.001, ****p < 0.0001.
The abundance of the phylum Bacteroidetes did not differ significantly between the stomach and small intestine (pairwise Wilcoxon rank-sum test, p > 0.05), but did show significant differences in abundance among other GIT sites (Figures 3A,C). This may be because of the presence of gastric acid, bile, and the lower pH in the stomach and small intestine affecting the survival and proliferation of the Bacteroidetes (Ding and Shah, 2007). Several functions have been reported for Bacteroidetes in the GIT. These microorganisms are involved in the fermentation of carbohydrates, converting complex carbohydrates into usable products (Hu et al., 2020; Fan and Pedersen, 2021). Additionally, they are capable of using nitrogenous substances and participating in the biotransformation of bile acids and other sterols in metabolic processes (Guzior and Quinn, 2021).
Firmicutes was the most abundant phylum in the stomach because of the high abundance of the genera Lactobacillus and Limosilactobacillus (Figure 3B). Microorganisms belonging to these genera are able to survive and establish themselves in the highly acidic environment of the stomach (Su et al., 2011). Lactobacillus is a common beneficial bacterium and is often used as a probiotic in yogurt and other fermented dairy products (Oleksy and Klewicka, 2018). These microorganisms can produce acetate (a beneficial short-chain fatty acid), lactate, and antimicrobial substances, which help to inhibit pathogens (Tomova et al., 2019). A higher ratio of Firmicutes to Bacteroidetes in the gut of obese subjects has been observed, which may be associated with more efficient absorption of calories from food by Firmicutes, leading to obesity (Moreira Júnior et al., 2021) One reason for the increase in abundance of the phylum Bacteroidetes in the GIT is the higher abundance of the genus Prevotella. Prevotella is a major genus within the Bacteroidetes, with a high abundance in the rectum and significantly lower abundance in other sites (Figures 3B,D). Prevotella is associated with the degradation of non-fibrous plant tissue and can produce short-chain fatty acids through the fermentation of cellulose and other non-digestible carbohydrates (Rubino et al., 2017). Additionally, Prevotella plays an important role in influencing host immune regulation (Iljazovic et al., 2021).
There was a significant difference in the abundance of the genus Clostridium within the phylum Firmicutes between the stomach and the large intestine/rectum (pairwise Wilcoxon rank-sum test, p < 0.05; Figure 3B). This may be because of notable differences in environmental conditions between the stomach and the large intestine/rectum (Sengupta et al., 2014; Chu and Traverso, 2022) The stomach is an acidic environment with a high concentration of gastric acid, which has inhibitory effects on many bacteria. Certain strains within the genus Clostridium may have weaker resistance to the acidic environment, resulting in lower numbers in the stomach. In contrast, the environment of the large intestine and rectum is neutral to slightly alkaline, providing more suitable growth conditions for Clostridium strains, allowing them to thrive and reproduce. However, there was no significant difference in the abundance of the genus Romboutsia across different sites of the GIT (pairwise Wilcoxon rank-sum test, p > 0.05; Figure 3B). This may be because strains within the genus Romboutsia have relatively stronger adaptability to gastric acid and intestinal environments, allowing them to survive and reproduce in different locations. The varying abundance of these bacterial genera reflect the interactive trends between the microbiota and the different GIT sites that collectively promote digestion and absorption functions along the GIT.
Additionally, we employed a linear discriminant analysis effect size (LEfSe) and linear discriminant analysis scores >3 to identify 52 taxonomic groups with significant differences in abundance at the genus level (Supplementary Figure S4). Among these taxonomic groups, the genera Lactobacillus, Romboutsia, Roseburia, and Prevotella were significantly more abundant in the stomach, small intestine, large intestine, and rectum compared with other taxonomic groups (Supplementary Figure S4). Other taxonomic groups with significant differences in abundance may also contribute to the physiological functions of the corresponding GIT sites. Therefore, further research is needed to explore their specific roles.
Functional characteristics of microbiota in different sites of the GIT
As shown in Figure 4A, nearly half of the proteins (274,379, 44.3% of the total) were annotated to KEGG Orthology pathways. KEGG pathway mapping indicated the most abundant pathways in the GIT of SPF pigs to be metabolic pathways (25.3%). Additionally, a significant proportion of the proteins (34.9%) could be assigned to BRITE hierarchical classifications (Figure 4A). According to KEGG functional analysis (Supplementary Figure S5), the microbial community in the small intestine exhibited richness in nucleotide metabolism, xenobiotic biodegradation, and metabolism, as well as digestive system. The microbial community in the large intestine was enriched in functions related to amino acid metabolism, biosynthesis of other secondary metabolites, and energy metabolism. Interestingly, similar results were obtained based on eggNOG analysis (Supplementary Figures S6A,E). These findings are consistent with the large intestine being a site of abundant microbial fermentation and the production of various metabolites, such as amino acids and short-chain fatty acids (Williams et al., 2001; Dai et al., 2011; Vital et al., 2014; Oliphant and Allen-Vercoe, 2019), which are crucial for host health. Therefore, we believe that the differences in composition and functionality of the microbial communities in the small and large intestines are related to their positions and nutritional conditions in the digestive system.
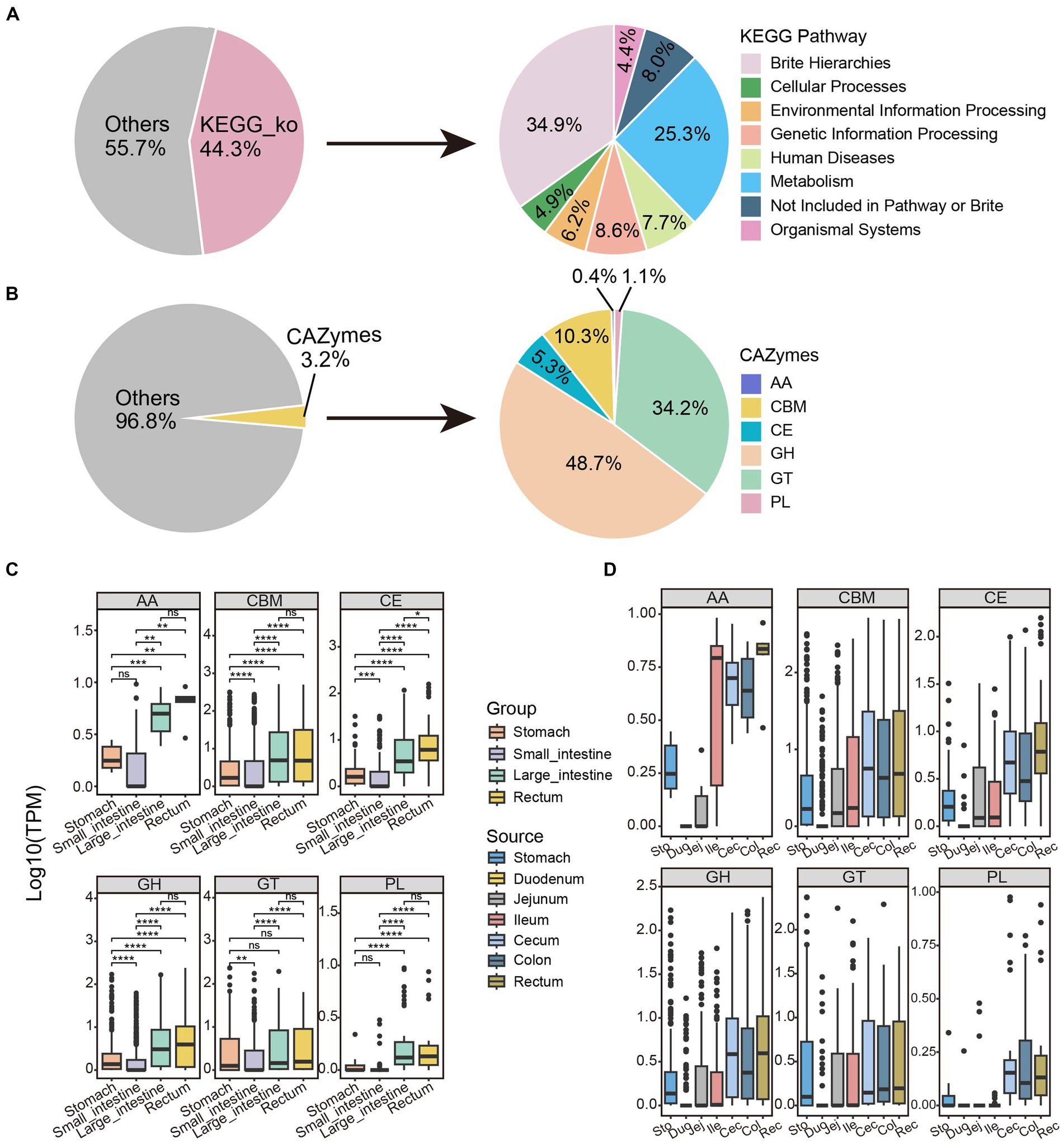
Figure 4. Functional annotation and distribution of non-redundant proteins along the GIT in SPF pigs. Functional annotation of microbial protein sequences in SPF pigs was performed using (A) GhostKOALA and (B) dbCAN2. The pie charts display the proportions of proteins annotated by these two methods (left) and the overall categories (right). The box plots illustrate the abundance distribution patterns of the six major families of CAZymes along the (C) Group and (D) Source. AA, auxiliary activities; CBM, carbohydrate-binding modules; CE, carbohydrate esterases; GH, glycoside hydrolases; GT, glycosyltransferases; PL, polysaccharide lyases. Y-axis: TPM values transformed by logarithm base 10. Boxes of different colors represent different segments of the GIT: stomach (orange, n = 6), small intestine (purple, n = 18), large intestine (green, n = 12), and rectum (yellow, n = 6). Pairwise Wilcoxon rank-sum tests were used to compare the groups. The box plots display the median, 25th, and 75th percentiles, while the solid lines represent the minimum and maximum values. Points outside the whiskers of the box plots indicate outliers. Significance levels are denoted as follows: nsp ≥ 0.05, *p < 0.05, **p < 0.01, ***p < 0.001, and ****p < 0.0001.
According to the CAZy database, only a small fraction of proteins (19,857, 3.2% of the total) were annotated as CAZymes (Figure 4B). Similar to studies in ruminant animals (Tong et al., 2022; Cao et al., 2023), the majority of these enzymes were annotated as glycoside hydrolases (9,664, 48.7% of 19,857), followed by glycosyltransferases (6,788, 34.2%) and carbohydrate-binding modules (2,052, 10.3%). The other three classes of enzymes, including carbohydrate esterases (1,048, 5.3%), polysaccharide lyases (221, 1.1%), and auxiliary activity (74, 0.4%), were represented by much smaller numbers of proteins.
We calculated the abundance of all proteins in each sample and compared their distribution. As shown in Figure 4C, the abundance of six CAZy families was relatively similar in the large intestine and rectum, but was significantly different from the other two sites (pairwise Wilcoxon rank-sum test, p < 0.05). Interestingly, the abundance of all CAZy protein families showed a trend of initially decreasing and then increasing moving anally along the GIT, with the lowest abundance in the small intestine and the highest in the rectum (Figure 4C). This might be because the small intestine is primarily responsible for nutrient absorption (Chin et al., 2017), not carbohydrate breakdown, resulting in a lower demand for CAZymes. In contrast, the microbial diversity was significantly increased in the rectum (Supplementary Figure S3A), where abundant CAZymes are involved in the degradation and fermentation of complex carbohydrates. Therefore, this trend reflects the characteristics and changing demands of different GIT sites in carbohydrate digestion and metabolism.
There were no significant differences in abundance of polysaccharide lyases and auxiliary activity enzymes between the stomach and small intestine, while differences in carbohydrate-binding modules, carbohydrate esterases, glycoside hydrolases, and glycosyltransferases were highly significant (pairwise Wilcoxon rank-sum test, p < 0.05; Figure 4C). This may be because polysaccharide lyases and auxiliary activity enzymes are primarily involved in the degradation of polysaccharides, while carbohydrate-binding modules, carbohydrate esterases, glycoside hydrolases, and glycosyltransferase enzymes have different functions, such as polysaccharide structure recognition, binding, and modification (Ndeh et al., 2017; Ye et al., 2020). Therefore, the degradation and digestion processes of polysaccharides were relatively similar in the stomach and small intestine. Interestingly, unlike other families, the abundance differences of glycosyltransferases in the stomach, large intestine, and rectum were not significant (Figure 4C; pairwise Wilcoxon rank-sum test, p > 0.05). This can be attributed to similar expression patterns and functions of glycosyltransferases in these tissues and indicates that they might be expressed at similar levels in the stomach, large intestine, and rectum, resulting in non-significant differences in their abundances. The duodenum had the lowest abundance among the six families (Figure 4D); therefore, we identified it as an outlier, possibly because of its lowest alpha diversity (Supplementary Figure S3B).
We investigated the distribution of CAZyme subfamilies in four GIT sites. The most abundant subfamily in the GIT was carbohydrate-binding module (CBM)48 (Supplementary Figure S6D), which possesses the ability to recognize and bind polysaccharide substrates, particularly xylan substances (Zhang et al., 2022). Interestingly, CBM91 exhibited lower abundance in the small intestine and stomach, but higher abundance in the large intestine and rectum (Supplementary Figure S6D). CBM91 is capable of binding xylan substances (such as birch and oat bran) (Ito et al., 2022), and the large intestine and rectum are the sites of fiber digestion (Moran and Bedford, 2022). The abundance of CBM50 gradually decreased along the GIT (Supplementary Figure S6D); CBM50 is involved in the degradation of chitin or peptidoglycans (Gruber et al., 2011). CBM6 exhibited higher abundance in the large intestine and rectum. CBM34, which binds granular starch (Kuchtová and Janeček, 2016), showed higher abundance in the stomach and small intestine, but the lowest abundance in the rectum (Supplementary Figure S6D). These differences may be closely associated with the host’s diet.
We also performed protein sequence alignment with the Comprehensive Antibiotic Resistance Database (CARD) and found a total of 43,892 protein sequences that matched antibiotic resistance genes (accounting for 7.1% of the total; Supplementary Figure S6B). The most abundant protein in the GIT was macB (Supplementary Figure S6C), which forms an antibiotic efflux complex with macA and TolC. The resistance mechanism of this complex is achieved through antibiotic efflux, with the best antibody match being against Neisseria gonorrhoeae (Xu et al., 2009). It is worth noting that the abundance of vanU gene in vanG cluster and evgS were higher in the large intestine and rectum (Supplementary Figure S6C) and lower in the stomach and small intestine. Their resistance mechanisms involve antibiotic target alteration and antibiotic efflux, with the best matches to antibodies against Streptococcus agalactiae and Escherichia coli (Nishino and Yamaguchi, 2002; Boyd et al., 2006). The highest abundance of RanA was found in the stomach and small intestine, while it was lower in the large intestine and rectum (Supplementary Figure S6C). RanA, together with RanB, confers resistance to aminoglycoside antibiotics through antibiotic efflux, with the best to the antibody match being against Riemerella anatipestifer (Li et al., 2020). Therefore, we believe there are different antimicrobial resistance genes present in different sites of the SPF pig GIT that have varying resistance mechanisms and best antibody matches. The identification of abundance differences of these antimicrobial resistance genes in the GIT provides background information to inform antibiotic use in pig farming. In summary, these findings reveal the microbial composition and functional differences within the four GIT sites of SPF pigs, along with characteristics associated with digestion and metabolism.
Transcriptome differences between the small intestine and large intestine
We determined transcriptome differences between the small intestine (n = 17) and large intestine (n = 11) by sequencing the transcriptomes of 28 tissue samples. In conjunction with the metagenomic data described above, we explored the interaction between SPF pig intestinal microbiota and the host.
By comparing gene expression profiles from different sites of the host GIT, we identified 1,618 DEGs between the small and large intestines (|Log2(FoldChange)| > 1 and FDR.P < 0.05). Among these genes, 912 were up-regulated and 706 were down-regulated in the small intestine (Figures 5A,B).
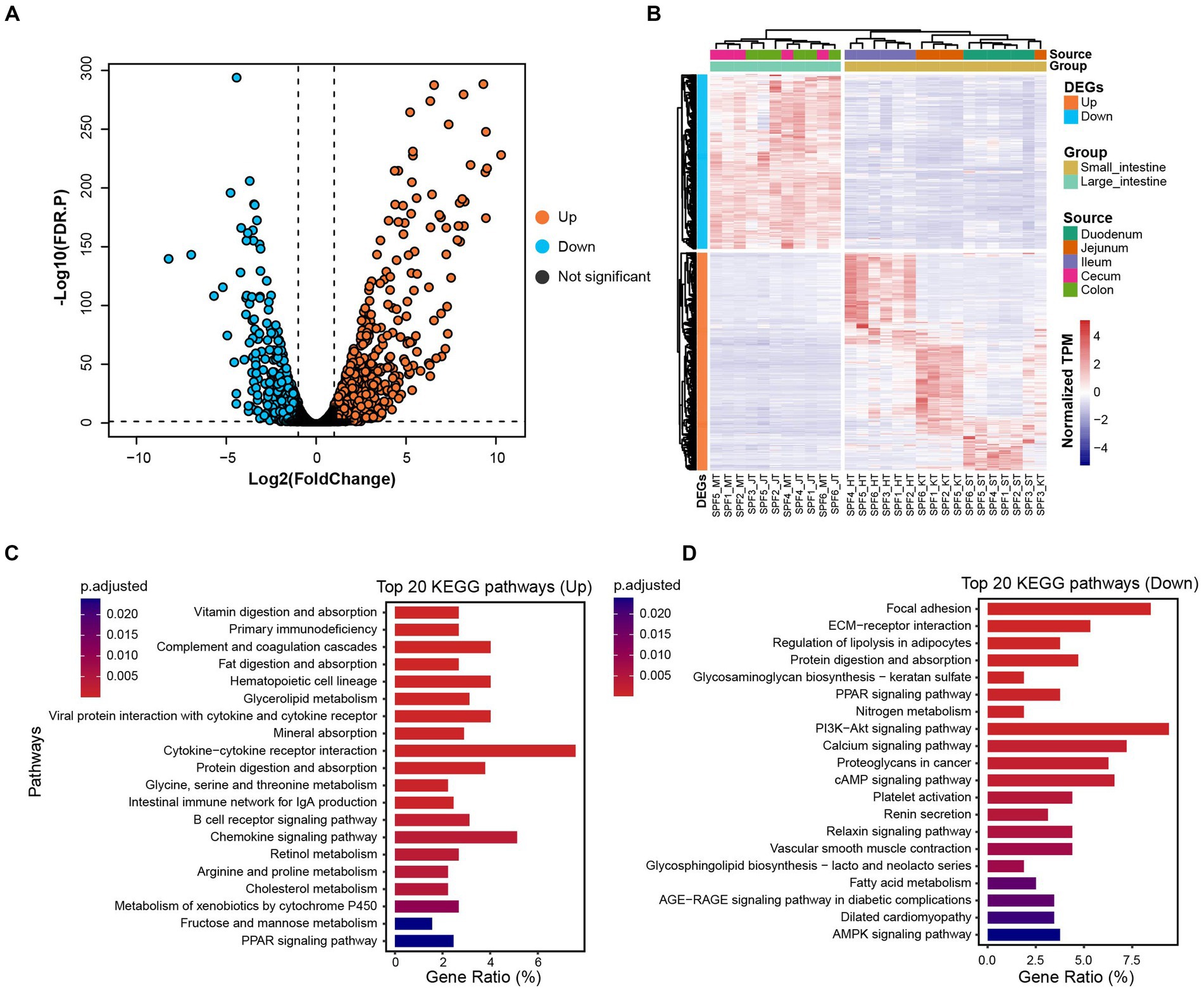
Figure 5. Distribution of DEGs and their associated functional enrichment in small and large intestines of SPF pigs. (A) Volcano plot of DEGs between the small intestine (n = 17) and large intestine (n = 11). DEGs with |Log2(FoldChange)| > 1 and FDR. p < 0.05 are colored orange (up-regulated in the small intestine) and blue (down-regulated in the small intestine). (B) Composition of DEGs between the small intestine (yellow) and large intestine (green). (C) Top 20 KEGG enrichment pathways for up-regulated genes in the small intestine (adjusted p < 0.05). (D) Top 20 KEGG enrichment pathways for down-regulated genes in the small intestine (adjusted p < 0.05). X-axis: gene ratio (number of genes enriched in the pathway/total number of genes enriched in all pathways).
We observed significant enrichment of up-regulated genes in multiple KEGG pathways in the small intestine (Figure 5C). Specifically, these up-regulated genes were significantly enriched in digestion and absorption-related pathways, including vitamin digestion and absorption, fat digestion and absorption, mineral absorption, and protein digestion and absorption (adjusted p < 0.05). Furthermore, immune-related pathways, such as primary immunodeficiency, complement and coagulation cascades, hematopoietic cell lineage, and viral protein interaction with cytokine and cytokine receptor, also showed significant enrichment (adjusted p < 0.05). We also observed significant enrichment in metabolism-related pathways, including glycerolipid metabolism, glycine, serine, and threonine metabolism, retinol metabolism, and arginine and proline metabolism (adjusted p < 0.05). Therefore, we conclude that the main functions of the SPF pig small intestine involve digestion and absorption, metabolism, and immune responses.
For the down-regulated genes in the small intestine (Figure 5D), we found significant enrichment in signaling transduction-related pathways, including focal adhesion, ECM-receptor interaction, and the PPAR signaling pathway (adjusted p < 0.05). Additionally, significant enrichment was observed in pathways related to nutrient metabolism, such as regulation of lipolysis in adipocytes, protein digestion and absorption, glycosaminoglycan biosynthesis—keratan sulfate, and nitrogen metabolism (adjusted p < 0.05). Therefore, we conclude that the main functions of the SPF pig large intestine involve nutrient metabolism and disease-related processes.
Further analysis of the GO enrichment results for Biological Process (BP) terms (Supplementary Figure S7A) revealed that the up-regulated genes in the small intestine were primarily enriched in immune system-related terms, such as mononuclear cell proliferation, leukocyte proliferation, lymphocyte proliferation, and lymphocyte differentiation (adjusted p < 0.05). Additionally, significant enrichment was observed in terms related to metabolic processes, such as alcohol metabolic process, and organic anion transport (adjusted p < 0.05). This indicates that the small intestine plays an important role in substance transport, homeostatic regulation, immune modulation, and cell activation.
The enriched down-regulated genes in the small intestine (Supplementary Figure S7B), were mainly enriched in terms related to extracellular matrix organization and development, such as extracellular matrix organization, extracellular structural organization, external encapsulating structure organization, wound healing, and cell-substrate adhesion (adjusted p < 0.05). Terms related to metabolic regulation, such as fatty acid metabolic process, and response to steroid hormone (adjusted p < 0.05), were also enriched. This indicates that the large intestine primarily functions in extracellular matrix organization and development, as well as nutrient metabolism.
In summary, there are differences in KEGG and GO enrichment between the small and large intestines, reflecting their specific biological functions and physiological processes. The small intestine is mainly involved in processes related to digestion and absorption, metabolism, and immune processes, while the large intestine is more involved in processes related to nutrient metabolism. These differences are consistent with our previous findings on the functional differences between the microbiota of the small and large intestines (Supplementary Figure S5). Overall, these findings indicate a co-evolutionary trend between the gut microbiota of SPF pigs and their hosts. These results provide important clues and foundations for further research on the characteristics and functions of SPF pig intestinal tissues, and contribute to a better understanding of the biological functions of the intestine and its role in host health.
Coevolution of host DEGs and gut microbiota
To investigate correlations between host genes and the gut microbiota and potential roles that any associations may have in intestinal function, we conducted a correlation analysis between 260 DEGs and the 20 most abundant microbial taxa in the SPF pig gut. We identified 1729 specific and strong microbiota-host associations in the small intestine and large intestine (Spearman rank correlation ≥ 0.5 or ≤ −0.5, FDR.P ≤ 0.01). Interestingly, the microbial taxa associated with up-regulated and down-regulated DEGs in the small intestine were mostly distinct (Figure 6A). Specifically, we identified 11 microbial taxa (Limosilactobacillus reuteri, Lactobacillus amylovorus, Bacteroides fragilis, Lactobacillus mucosae, Roseburia hominis, Prevotella ruminicola, Prevotella dentalis, Clostridioides difficile, Ruthenibacterium lactatiformans, Ruminococcus bicirculans, and Desulfovibrio piger) that showed positive correlations with most down-regulated genes but negative correlations with up-regulated genes (Figure 6A). In contrast, we found that nine microbial taxa (Glaesserella parasuis, Actinobacillus indolicus, Romboutsia ilealis, Turicibacter sanguinis, Lactobacillus crispatus, Porcine mastadenovirus A, Escherichia coli, Lactobacillus johnsonii, and Ligilactobacillus salivarius) exhibited positive correlations with the majority of up-regulated genes but negative correlations with down-regulated genes (Figure 6A).
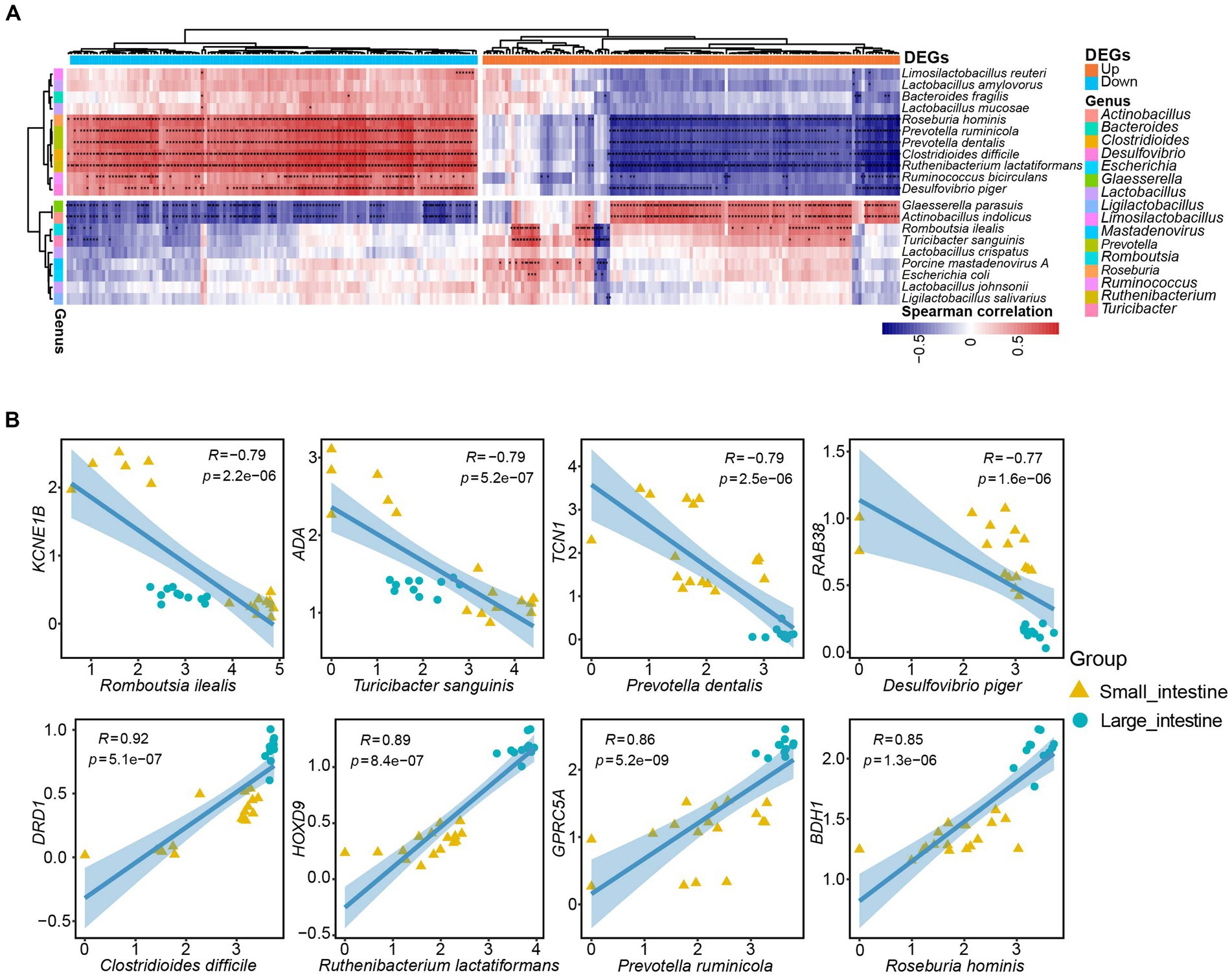
Figure 6. Specific strong associations between SPF pig gut microbiota and host genes. (A) Correlation analysis was performed between the top 20 ranked species-level microbiota in the SPF pig gut and the top 130 differentially expressed genes (DEGs) in the small intestine with |Log2(FoldChange)| (a total of 260 DEGs after converting human genes to pig homologs). The horizontal bars in orange and blue represent up-regulated and down-regulated genes in the small intestine, respectively, while the 16 vertical bars of different colors represent genera corresponding to the species-level microbiota. Only Spearman rank correlations with p-value < 0.01 were considered as strong associations and are marked with “*” in the heatmap (*p < 0.01, p > 0.001, and **p < 0.001). The red and blue cells in the heatmap indicate positive and negative correlations between genes and microbiota, respectively. (B) Scatter plots depict the grouping patterns of the eight pairs of gene-microbiota correlations of interest in the small intestine (yellow, n = 17) and large intestine (green, n = 11) samples. X and Y axes: TPM values transformed by logarithm base 10. Spearman rank correlation (R) and significance (p) are displayed at the top of each graph.
We then selected eight microbial taxa of interest to describe the pairwise correlations between host gene expression and microbial taxonomic groups (Figure 6B). The TCN1 gene encodes vitamin B12-binding protein, which binds to and protects vitamin B12 from the acidic environment of the stomach (Johnston et al., 1992). There was a strong negative correlation between Prevotella dentalis and TCN1 gene expression (R = −0.79, p = 2.5e-06; Figure 6B). This negative correlation may be attributed to Prevotella dentalis being a Gram-negative bacteria with metabolic pathways related to vitamin B12 (Franco-Lopez et al., 2020). The transport protein encoded by the TCN1 gene is responsible for transporting vitamin B12 into cells and participating in the metabolic processes in which it is involved. If Prevotella dentalis is involved in the intracellular metabolism of vitamin B12, a negative correlation with TCN1 expression may exist. Similarly, the ADA gene encodes adenosine deaminase, an enzyme involved in adenosine metabolism. Adenosine is an important cell signaling molecule that plays a significant role in regulating intestinal immune function and inflammatory responses. The function of adenosine deaminase is to convert adenosine into inosine, thereby regulating adenosine levels (Eltzschig et al., 2006). Although the exact function and impact of Turicibacter sanguinis in the intestines are still under investigation, some studies suggest its association with gut health and metabolism (Hoffman and Margolis, 2020). The involvement of the ADA enzyme in regulating adenosine levels and the important role of adenosine in immune regulation and inflammatory responses mean that Turicibacter sanguinis may indirectly affect ADA gene expression or enzyme activity by influencing immune cells or inflammatory signaling pathways. This could result in the negative correlation between ADA gene expression and Turicibacter sanguinis (R = −0.79, p = 5.2e-07; Figure 6B).
In addition, the 3-hydroxybutyrate dehydrogenase encoded by BDH1 participates in reactions of the fatty acid metabolism pathway. It is responsible for converting 3-hydroxybutyrate into acetoacetate, which is an important step in fatty acid oxidation metabolism. This process contributes to the generation of energy supply and the maintenance of normal intestinal cell function (Marks et al., 1992; Otsuka et al., 2020). Meanwhile, Roseburia hominis, a beneficial bacteria in the gut microbiota, is primarily involved in the degradation of cellulose and other complex carbohydrates. Bacteria belonging to the Roseburia genus play important roles in the human intestinal tract, particularly in the production of short-chain fatty acids, such as propionate, butyrate, and valerate (Tamanai-Shacoori et al., 2017). Therefore, there was a positive correlation between the BDH1 gene and Roseburia hominis (R = 0.85, p = 1.3e-06; Figure 6B). Finally, the DRD1 gene encodes the dopamine D1 receptor, which plays a crucial role in the nervous system. The dopamine D1 receptor is a key component in the transmission of dopamine neurotransmitter signals and is involved in regulating numerous functions, such as motor control, emotion, cognition, and reward (Dearry et al., 1990). Clostridioides difficile is a pathogenic bacteria that can cause intestinal infections and inflammation. It is typically transmitted through the ingestion of spores or bacteria, and produces toxins that result in symptoms such as diarrhea, abdominal pain, and fever (Sandhu and Mcbride, 2018). There was a positive correlation between Clostridioides difficile infection and the DRD1 gene (R = 0.92, p = 5.1e-07; Figure 6B). A possible reason for this is that Clostridioides difficile infection alters the gut microbiota environment, including the composition and functionality of the microbial community. Such environmental changes may impact the activity of the dopamine signaling pathway and expression of the DRD1 gene.
Discussion
In this study, we employed a metagenomic and transcriptomic approach to investigate the gut microbiota community in SPF pigs after stable colonization and to reveal its interaction with the host. By comparing the microbial composition in different GIT sites of SPF pigs, we identified differences in the microbial communities and explored their potential impacts on the host and the mechanisms that underlie these impacts. To the best of our knowledge, this is the first comprehensive study using metagenomics and transcriptomics to investigate structural and functional differences in the gut microbiota among different GIT sites (stomach, small intestine, large intestine, and rectum) of SPF pigs, while also associating the microbiota with host DEGs to explore their interactions.
We employed metagenomics approaches to obtain comprehensive genomic information of the gut microbiota in SPF pigs. This enabled us to gain a more comprehensive understanding of the genetic potential and metabolic functions of the microbiota community and potential mechanisms of its interaction with the host. We observed significant differences in microbial composition among GIT sites, which were closely related to their functions. For example, we found distinct abundance patterns of the two predominant phyla, Firmicutes and Bacteroidetes, along the GIT: Firmicutes abundance decreased in the rectum direction along the GIT (Figure 3A), while Bacteroidetes exhibited the opposite pattern (Figure 3A). The high gastric acid and bile salt concentrations in the stomach and upper intestine provide conditions favorable for the survival and proliferation of Firmicutes, leading to its increased abundance, while the lower oxygen levels and presence of abundant fermentation substrates in the middle and lower intestines create a suitable environment for the growth of Bacteroidetes, potentially resulting in its higher abundance. This contrasting pattern may reflect the adaptability and functional specialization of different microbial communities to different intestinal segments, thereby maintaining the stability and functional balance of the entire GIT. We also discovered the presence of abundant cellulose-degrading microbial communities, such as Prevotella, in the large intestine and rectum of SPF pigs, highlighting their important role in the degradation of cellulose and other complex carbohydrates, this suggests that the genus Prevotella also includes starch-degrading bacteria. In contrast, the small intestine harbored other microbial groups involved in protein and fat degradation, nutrient absorption, and other essential metabolic processes. These results indicate functional differences in microbial composition among different GIT sites.
After annotation, we identified Porcine mastadenovirus A virus in the ileum. We propose three hypotheses to explain its presence. Firstly, the unique anatomical and physiological characteristics of the ileum provide an ideal environment for fecal retention and pathogen proliferation. Secondly, Porcine mastadenovirus A may be transmitted through the fecal-oral route. Considering the virus’s mode of transmission, we speculate that the ileum is the site where the virus remains for the longest duration, facilitating its replication and spread. Lastly, the ileal microenvironment is conducive to viral growth. Compared with other intestinal sites, the ileum exhibits a higher pH and electrolyte concentrations that are favorable for the replication of Porcine mastadenovirus A.
We annotated the bacterial protein-coding genes and compared them with the KEGG, eggNOG, CAZy, and CARD databases to assess the functionality of the GIT sites and their associated microbial ecology. Surprisingly, we found the functional profiles of the gut microbiota to be similar using KEGG and eggNOG. The gut microbiota in the small intestine of SPF pigs were enriched for nucleotide metabolism, xenobiotic biodegradation and metabolism and digestive system, while the microbiota in the large intestine were enriched for amino acid metabolism, secondary metabolite biosynthesis, and energy metabolism (Supplementary Figures S5, S6A,E). Furthermore, we observed similar differences in the functional enrichment of host DEGs (Figures 5C,D; Supplementary Figure S7). This finding indicates a co-evolutionary trend between the gut microbiota and the host. In addition, we observed significant differences in six CAZyme families across different sites of the GIT. Moreover, different antimicrobial resistance genes within the GIT exhibited variations in resistance mechanisms and best match antibodies, indicating their distinct roles in different GIT sites.
By combining metagenomics with transcriptomics, we revealed interactions between the intestinal microbiota and their impacts on host gene expression. By measuring the mRNA levels in host cells, we observed differences in gene expression between the small and large intestines (Figure 5B). Notably, the distribution of gene expression within the small intestine was not uniform; there were significant differences between the ileum and the other two segments (Figure 5B). Functional enrichment analysis revealed immune-related pathways and terms in the small intestinal tissue, as indicated by both KEGG and GO enrichment analyses (Figure 5C; Supplementary Figure S7A). This suggests that the ileum plays a crucial role as an immune barrier. The ileal mucosa contains numerous lymphoid tissues and immune cells, including Peyer’s patches, lymphoid follicles, and intestinal epithelial cells (Mörbe et al., 2021). Peyer’s patches are lymphoid follicles on the ileal mucosa consisting of aggregated lymphoid cells. These follicles contain a large number of lymphocytes, including B cells and T cells, which are key components of the immune response. Upon exposure to pathogens, these lymphocytes can recognize and initiate appropriate immune reactions, including antibody production and activation of cellular immunity (Carrasco Garcia et al., 2020). Furthermore, the ileum contains a substantial population of intestinal epithelial cells that contribute to immune responses. This immune response is essential for maintaining intestinal health and the normal functioning of the overall immune system (Rath and Haller, 2022). Finally, by analyzing the association between microbiota and host genes, we discovered that the presence of microbiota can regulate host immune responses, metabolic pathways, and other crucial biological processes. This indicates that changes in the microbial community can have a significant impact on the host’s physiological status.
we identified some gut bacteria that are closely associated with host gene expression, and these findings have important implications for future applications. For example, we observed a negative correlation between the expression of Prevotella dentalis and the gene TCN1, which is related to vitamin B12 metabolism. This finding may contribute to the assessment of vitamin B12 absorption and utilization in the gut. The expression of Turicibacter sanguinis was negatively correlated with the immune-regulatory gene ADA, suggesting that microorganisms may indirectly regulate ADA gene expression by influencing immune cells or inflammatory pathways. The expression of Roseburia hominis was positively correlated with the gene BDH1, providing new clues for studying intestinal energy metabolism. Additionally, we found a positive correlation between Clostridioides difficile infection and the expression of the neuro-related gene DRD1. This finding may reveal the impact of gut microbiota on the nervous system, providing a novel perspective for understanding the relationship between gut microbiota and neurological disorders.
This study has some limitations. Firstly, we only investigated a limited number of SPF pig samples. Expanding the sample size would contribute to a more comprehensive understanding of the diversity and functionality of the gut microbiota in SPF pigs. Secondly, while we focused on the composition of the microbial community and changes in host gene expression, further research is needed to understand the specific mechanisms of interaction between the microbiota and the host.
Conclusion
In this study, we investigated the gut microbiota of SPF Bama females and their interactions with the host. We found significant differences in microbial diversity and functions across different sites of the gastrointestinal tract (GIT). The large intestine and rectum exhibited higher microbial abundances and functions related to polysaccharide fermentation. Additionally, we correlated host gene expression with microbial communities, revealing potential functional interactions between the microbiota and the host, which would be an expected outcome of co-evolution. Our findings highlight the influence of the microbiota on gut function, including immune responses, metabolism, and neural signaling. Overall, this study provides valuable insights into the gut microbiota-host interactions in SPF pigs, contributing to our understanding of pig physiology and husbandry.
In conclusion, our study sheds light on the gut microbiota composition and its interaction with the host in SPF pigs. These findings have implications for animal husbandry, life science research, and bioproduct production.
Data availability statement
The datasets presented in this study can be found in the Genome Sequence Archive (GSA), accession numbers CRA014067 and CRA014068.
Ethics statement
The animal studies were approved by the Experimental Animal Ethics Committee, Chongqing Academy of Animal Science. The studies were conducted in accordance with the local legislation and institutional requirements. Written informed consent was obtained from the owners for the participation of their animals in this study.
Author contributions
MW: Formal analysis, Methodology, Software, Visualization, Writing – original draft, Conceptualization, Data curation, Investigation, Project administration, Resources, Validation. SC: Data curation, Formal analysis, Methodology, Software, Visualization, Validation, Writing – original draft. YZ: Formal analysis, Investigation, Validation, Writing – original draft. YL: Investigation, Methodology, Validation, Writing – original draft. CT: Data curation, Formal analysis, Software, Writing – original draft. JZ: Conceptualization, Funding acquisition, Software, Validation, Writing – original draft. JS: Formal analysis, Investigation, Methodology, Resources, Writing – original draft. XiL: Project administration, Software, Supervision, Visualization, Writing – original draft. YD: Investigation, Software, Writing – original draft. LL: Conceptualization, Project administration, Supervision, Writing – original draft. KL: Investigation, Methodology, Validation, Writing – original draft. YN: Investigation, Methodology, Writing – original draft. XuL: Investigation, Supervision, Validation, Writing – review & editing. ML: Supervision, Writing – review & editing, Funding acquisition. LG: Writing – review & editing, Supervision. JM: Investigation, Methodology, Validation, Writing – review & editing.
Funding
The author(s) declare that financial support was received for the research, authorship, and/or publication of this article. This study has received funding from the National Natural Science Foundation of China (32072687, 32302712), the National Center of Technology Innovation for Pigs (NCTIP-XD/B13), the Science Foundation of Sichuan Province (2022YFQ0022), Sichuan International Science and Technology Innovation Cooperation/Hong Kong, Macao, and Taiwan Science and Technology Innovation Cooperation Project (2021YFH0033), Agricultural germplasm resources survey, collection, protection, and identification service project of the Ministry of Agriculture and Rural Affairs (A120202); and the Major Science and Technology Projects of Tibet Autonomous Region (XZ202101ZD0005N).
Acknowledgments
We thank Jeremy Allen, PhD, from Liwen Bianji (Edanz) (www.liwenbianji.cn) for editing a draft of this manuscript.
Conflict of interest
The authors declare that the research was conducted in the absence of any commercial or financial relationships that could be construed as a potential conflict of interest.
Publisher's note
All claims expressed in this article are solely those of the authors and do not necessarily represent those of their affiliated organizations, or those of the publisher, the editors and the reviewers. Any product that may be evaluated in this article, or claim that may be made by its manufacturer, is not guaranteed or endorsed by the publisher.
Supplementary material
The Supplementary material for this article can be found online at: https://www.frontiersin.org/articles/10.3389/fmicb.2024.1402807/full#supplementary-material
References
Abuqwider, J., Altamimi, M., and Mauriello, G. (2022). Limosilactobacillus reuteri in health and disease. Microorganisms 10:522. doi: 10.3390/microorganisms10030522
Alcock, B. P., Huynh, W., Chalil, R., Smith, K. W., Raphenya, A. R., Wlodarski, M. A., et al. (2023). CARD 2023: expanded curation, support for machine learning, and resistome prediction at the comprehensive antibiotic resistance database. Nucleic Acids Res. 51, D690–d699. doi: 10.1093/nar/gkac920
Amaral, A. F., Rahman, K. S., Kick, A. R., Cortes, L. M., Robertson, J., Kaltenboeck, B., et al. (2020). Mucosal vaccination with UV-inactivated Chlamydia suis in pre-exposed outbred pigs decreases pathogen load and induces CD4 T-cell maturation into IFN-γ(+) effector memory cells. Vaccines (Basel) 8:353. doi: 10.3390/vaccines8030353
Boyd, D. A., Du, T., Hizon, R., Kaplen, B., Murphy, T., Tyler, S., et al. (2006). VanG-type vancomycin-resistant Enterococcus faecalis strains isolated in Canada. Antimicrob. Agents Chemother. 50, 2217–2221. doi: 10.1128/AAC.01541-05
Bray, N. L., Pimentel, H., Melsted, P., and Pachter, L. (2016). Near-optimal probabilistic RNA-seq quantification. Nat. Biotechnol. 34, 525–527. doi: 10.1038/nbt.3519
Buchfink, B., Xie, C., and Huson, D. H. (2015). Fast and sensitive protein alignment using DIAMOND. Nat. Methods 12, 59–60. doi: 10.1038/nmeth.3176
Caminero, A., Meisel, M., Jabri, B., and Verdu, E. F. (2019). Mechanisms by which gut microorganisms influence food sensitivities. Nat. Rev. Gastroenterol. Hepatol. 16, 7–18. doi: 10.1038/s41575-018-0064-z
Cantalapiedra, C. P., Hernández-Plaza, A., Letunic, I., Bork, P., and Huerta-Cepas, J. (2021). eggNOG-mapper v2: functional annotation, orthology assignments, and domain prediction at the metagenomic scale. Mol. Biol. Evol. 38, 5825–5829. doi: 10.1093/molbev/msab293
Cao, Y., Feng, T., Wu, Y., Xu, Y., du, L., Wang, T., et al. (2023). The multi-kingdom microbiome of the goat gastrointestinal tract. Microbiome 11:219. doi: 10.1186/s40168-023-01651-6
Carrasco Garcia, A., Rao, A., Kokkinou, E., Haapaniemi, S., Lindforss, U., Winberg, M. E., et al. (2020). P073 compartmentalised human gut immunity: studies of innate and adaptive lymphocyte distribution in the epithelium, lamina propria and GALT of healthy gut mucosa by flow cytometry. J. Crohn's Colitis 14:S172. doi: 10.1093/ecco-jcc/jjz203.202
Chen, C., Fang, S., Wei, H., He, M., Fu, H., Xiong, X., et al. (2021b). Prevotella copri increases fat accumulation in pigs fed with formula diets. Microbiome 9:175. doi: 10.1186/s40168-021-01110-0
Chen, Y., Lun, A., and Smyth, G. (2016). From reads to genes to pathways: differential expression analysis of RNA-Seq experiments using Rsubread and the edgeR quasi-likelihood pipeline. F1000Research 5:1438. doi: 10.12688/f1000research.8987.2
Chen, S., Zhou, Y., Chen, Y., and Gu, J. (2018). Fastp: an ultra-fast all-in-one FASTQ preprocessor. Bioinformatics 34, i884–i890. doi: 10.1093/bioinformatics/bty560
Chen, C., Zhou, Y., Fu, H., Xiong, X., Fang, S., Jiang, H., et al. (2021a). Expanded catalog of microbial genes and metagenome-assembled genomes from the pig gut microbiome. Nat. Commun. 12:1106. doi: 10.1038/s41467-021-21295-0
Chin, A. M., Hill, D. R., Aurora, M., and Spence, J. R. (2017). Morphogenesis and maturation of the embryonic and postnatal intestine. Semin. Cell Dev. Biol. 66, 81–93. doi: 10.1016/j.semcdb.2017.01.011
Chu, J. N., and Traverso, G. (2022). Foundations of gastrointestinal-based drug delivery and future developments. Nat. Rev. Gastroenterol. Hepatol. 19, 219–238. doi: 10.1038/s41575-021-00539-w
Dai, Z. L., Wu, G., and Zhu, W. Y. (2011). Amino acid metabolism in intestinal bacteria: links between gut ecology and host health. Front. Biosci. (Landmark Ed.) 16, 1768–1786. doi: 10.2741/3820
Dearry, A., Gingrich, J. A., Falardeau, P., Fremeau, R. T. Jr., Bates, M. D., and Caron, M. G. (1990). Molecular cloning and expression of the gene for a human D1 dopamine receptor. Nature 347, 72–76. doi: 10.1038/347072a0
Ding, W. K., and Shah, N. P. (2007). Acid, bile, and heat tolerance of free and microencapsulated probiotic bacteria. J. Food Sci. 72, M446–M450. doi: 10.1111/j.1750-3841.2007.00565.x
Dobin, A., Davis, C. A., Schlesinger, F., Drenkow, J., Zaleski, C., Jha, S., et al. (2012). STAR: ultrafast universal RNA-seq aligner. Bioinformatics 29, 15–21. doi: 10.1093/bioinformatics/bts635
Donald, K., and Finlay, B. B. (2023). Early-life interactions between the microbiota and immune system: impact on immune system development and atopic disease. Nat. Rev. Immunol. 23, 735–748. doi: 10.1038/s41577-023-00874-w
Dong, W., Ricker, N., Holman, D. B., Johnson, T. A., van de Vliet, M., and Liu, H. Y. (2023). Meta-analysis reveals the predictable dynamic development of the gut microbiota in commercial pigs. Microbiol. Spectr. 11:e0172223. doi: 10.1128/spectrum.01722-23
Edgar, R. C. (2010). Search and clustering orders of magnitude faster than BLAST. Bioinformatics 26, 2460–2461. doi: 10.1093/bioinformatics/btq461
Eltzschig, H. K., Faigle, M., Knapp, S., Karhausen, J., Ibla, J., Rosenberger, P., et al. (2006). Endothelial catabolism of extracellular adenosine during hypoxia: the role of surface adenosine deaminase and CD26. Blood 108, 1602–1610. doi: 10.1182/blood-2006-02-001016
Fan, Y., and Pedersen, O. (2021). Gut microbiota in human metabolic health and disease. Nat. Rev. Microbiol. 19, 55–71. doi: 10.1038/s41579-020-0433-9
Ferenc, K., Pietrzak, P., Godlewski, M. M., Piwowarski, J., Kilianczyk, R., Guilloteau, P., et al. (2014). Intrauterine growth retarded piglet as a model for humans – studies on the perinatal development of the gut structure and function. Reprod. Biol. 14, 51–60. doi: 10.1016/j.repbio.2014.01.005
Franco-Lopez, J., Duplessis, M., Bui, A., Reymond, C., Poisson, W., Blais, L., et al. (2020). Correlations between the composition of the bovine microbiota and vitamin B(12) abundance. mSystems 5:e00107-20. doi: 10.1128/mSystems.00107-20
Frese, S. A., Parker, K., Calvert, C. C., and Mills, D. A. (2015). Diet shapes the gut microbiome of pigs during nursing and weaning. Microbiome 3:28. doi: 10.1186/s40168-015-0091-8
Gainor, K., Fortuna, Y. C., Alakkaparambil, A. S., González, W., Malik, Y. S., and Ghosh, S. (2022). High rates of detection and molecular characterization of porcine adenovirus serotype 5 (porcine mastadenovirus C) from diarrheic pigs. Pathogens 11:1210. doi: 10.3390/pathogens11101210
García-Pérez, P., Tomas, M., Rivera-Pérez, A., Patrone, V., Giuberti, G., Cervini, M., et al. (2024). Pectin conformation influences the bioaccessibility of cherry laurel polyphenols and gut microbiota distribution following in vitro gastrointestinal digestion and fermentation. Food Chem. 430:137054. doi: 10.1016/j.foodchem.2023.137054
Gene Ontology Consortium (2007). The gene ontology project in 2008. Nucleic Acids Res. 36, D440–D444. doi: 10.1093/nar/gkm883
Gerdts, V., Wilson, H. L., Meurens, F., van Drunen Littel - van den Hurk, S., Wilson, D., Walker, S., et al. (2015). Large animal models for vaccine development and testing. ILAR J. 56, 53–62. doi: 10.1093/ilar/ilv009
Gruber, S., Vaaje-Kolstad, G., Matarese, F., López-Mondéjar, R., Kubicek, C. P., and Seidl-Seiboth, V. (2011). Analysis of subgroup C of fungal chitinases containing chitin-binding and LysM modules in the mycoparasite Trichoderma atroviride. Glycobiology 21, 122–133. doi: 10.1093/glycob/cwq142
Gurevich, A., Saveliev, V., Vyahhi, N., and Tesler, G. (2013). QUAST: quality assessment tool for genome assemblies. Bioinformatics 29, 1072–1075. doi: 10.1093/bioinformatics/btt086
Gutierrez, K., Dicks, N., Glanzner, W. G., Agellon, L. B., and Bordignon, V. (2015). Efficacy of the porcine species in biomedical research. Front. Genet. 6:293. doi: 10.3389/fgene.2015.00293
Guzior, D. V., and Quinn, R. A. (2021). Review: microbial transformations of human bile acids. Microbiome 9:140. doi: 10.1186/s40168-021-01101-1
Hoffman, J. M., and Margolis, K. G. (2020). Building community in the gut: a role for mucosal serotonin. Nat. Rev. Gastroenterol. Hepatol. 17, 6–8. doi: 10.1038/s41575-019-0227-6
Holman, D. B., Brunelle, B. W., Trachsel, J., and Allen, H. K. (2017). Meta-analysis to define a Core microbiota in the swine gut. mSystems 2:e00004-17. doi: 10.1128/mSystems.00004-17
Hu, J., Cao, Y., Xie, Y., Wang, H., Fan, Z., Wang, J., et al. (2016). Periodontal regeneration in swine after cell injection and cell sheet transplantation of human dental pulp stem cells following good manufacturing practice. Stem Cell Res Ther 7:130. doi: 10.1186/s13287-016-0362-8
Hu, Y., He, Y., Gao, S., Liao, Z., Lai, T., Zhou, H., et al. (2020). The effect of a diet based on rice straw co-fermented with probiotics and enzymes versus a fresh corn Stover-based diet on the rumen bacterial community and metabolites of beef cattle. Sci. Rep. 10:10721. doi: 10.1038/s41598-020-67716-w
Huang, Y., Niu, B., Gao, Y., Fu, L., and Li, W. (2010). CD-HIT suite: a web server for clustering and comparing biological sequences. Bioinformatics 26, 680–682. doi: 10.1093/bioinformatics/btq003
Hyatt, D., Chen, G. L., LoCascio, P. F., Land, M. L., Larimer, F. W., and Hauser, L. J. (2010). Prodigal: prokaryotic gene recognition and translation initiation site identification. BMC Bioinformatics 11:119. doi: 10.1186/1471-2105-11-119
Iljazovic, A., Roy, U., Gálvez, E. J. C., Lesker, T. R., Zhao, B., Gronow, A., et al. (2021). Perturbation of the gut microbiome by Prevotella spp. enhances host susceptibility to mucosal inflammation. Mucosal Immunol. 14, 113–124. doi: 10.1038/s41385-020-0296-4
Ito, D., Nakano, E., Karita, S., Umekawa, M., Ratanakhanokchai, K., and Tachaapaikoon, C. (2022). Characterization of a GH family 43 β-Xylosidase having a novel carbohydrate-binding module from <i>Paenibacillus xylaniclasticus</i> strain TW1. J. Appl. Glycosci. 69, 65–71. doi: 10.5458/jag.jag.JAG-2022_0001
Jiang, Y., Jiang, Y., Zhang, H., Mei, M., Song, H., Ma, X., et al. (2019). A mutation in MAP2 is associated with prenatal hair follicle density. FASEB J. 33, 14479–14490. doi: 10.1096/fj.201901187R
Johnston, J., Yang-Feng, T., and Berliner, N. (1992). Genomic structure and mapping of the chromosomal gene for transcobalamin I (TCN1): comparison to human intrinsic factor. Genomics 12, 459–464. doi: 10.1016/0888-7543(92)90435-U
Jolivet-Gougeon, A., Sixou, J.-L., Tamanai-Shacoori, Z., and Bonnaure-Mallet, M. (2007). Antimicrobial treatment of Capnocytophaga infections. Int. J. Antimicrob. Agents 29, 367–373. doi: 10.1016/j.ijantimicag.2006.10.005
Kanehisa, M., and Goto, S. (2000). KEGG: Kyoto encyclopedia of genes and genomes. Nucleic Acids Res. 28, 27–30. doi: 10.1093/nar/28.1.27
Kanehisa, M., Sato, Y., and Morishima, K. (2016). BlastKOALA and GhostKOALA: KEGG tools for functional characterization of genome and metagenome sequences. J. Mol. Biol. 428, 726–731. doi: 10.1016/j.jmb.2015.11.006
Kang, Y.-J., Yang, D.-C., Kong, L., Hou, M., Meng, Y. Q., Wei, L., et al. (2017). CPC2: a fast and accurate coding potential calculator based on sequence intrinsic features. Nucleic Acids Res. 45, W12–W16. doi: 10.1093/nar/gkx428
Klymiuk, N., Seeliger, F., Bohlooly, Y. M., Blutke, A., Rudmann, D. G., Wolf, E., et al. (2016). Tailored pig models for preclinical efficacy and safety testing of targeted therapies. Toxicol. Pathol. 44, 346–357. doi: 10.1177/0192623315609688
Kolli, U., and Roy, S. (2023). The role of the gut microbiome and microbial metabolism in mediating opioid-induced changes in the epigenome. Front. Microbiol. 14:1233194. doi: 10.3389/fmicb.2023.1233194
Koren, O., Konnikova, L., Brodin, P., Mysorekar, I. U., and Collado, M. C. (2023). The maternal gut microbiome in pregnancy: implications for the developing immune system. Nature reviews. Gastroenterol. Hepatol. 21, 35–45. doi: 10.1038/s41575-023-00864-2
Kuchtová, A., and Janeček, Š. (2016). Domain evolution in enzymes of the neopullulanase subfamily. Microbiology (Reading) 162, 2099–2115. doi: 10.1099/mic.0.000390
Kudjordjie, E. N., Sapkota, R., Steffensen, S. K., Fomsgaard, I. S., and Nicolaisen, M. (2019). Maize synthesized benzoxazinoids affect the host associated microbiome. Microbiome 7:59. doi: 10.1186/s40168-019-0677-7
Ley, R. E., Turnbaugh, P. J., Klein, S., and Gordon, J. I. (2006). Microbial ecology: human gut microbes associated with obesity. Nature 444, 1022–1023. doi: 10.1038/4441022a
Li, H. (2013). Aligning sequence reads, clone sequences and assembly contigs with BWA-MEM. arxiv:1303.3997. doi: 10.48550/arXiv.1303.3997
Li, S., Chen, Q., Gong, X., Liu, Y., and Zheng, F. (2020). RanB, a putative ABC-type multidrug efflux transporter contributes to aminoglycosides resistance and organic solvents tolerance in Riemerella anatipestifer. Vet. Microbiol. 243:108641. doi: 10.1016/j.vetmic.2020.108641
Li, H., Handsaker, B., Wysoker, A., Fennell, T., Ruan, J., Homer, N., et al. (2009). The sequence alignment/map format and SAMtools. Bioinformatics 25, 2078–2079. doi: 10.1093/bioinformatics/btp352
Li, D., Liu, C. M., Luo, R., Sadakane, K., and Lam, T. W. (2015). MEGAHIT: an ultra-fast single-node solution for large and complex metagenomics assembly via succinct de Bruijn graph. Bioinformatics 31, 1674–1676. doi: 10.1093/bioinformatics/btv033
Liu, S.-J., Wang, Q., Li, T.-T., Zhang, S. H., Li, J. Y., Wu, L. J., et al. (2020). Characterization of the first genome of porcine mastadenovirus B (HNU1 strain) and implications on its lymphoid and special origin. Virol. Sin. 35, 528–537. doi: 10.1007/s12250-020-00210-9
Long, C.-X., Wu, J.-Q., Tan, Z.-J., and Wang, S. P. (2022). Different intestinal microbiota with growth stages of three-breed hybrid pig. Biomed. Res. Int. 2022, 1–9. doi: 10.1155/2022/5603451
Lu, J., Rincon, N., Wood, D. E., Breitwieser, F. P., Pockrandt, C., Langmead, B., et al. (2022). Metagenome analysis using the kraken software suite. Nat. Protoc. 17, 2815–2839. doi: 10.1038/s41596-022-00738-y
Lunney, J. K. (2007). Advances in swine biomedical model genomics. Int. J. Biol. Sci. 3, 179–184. doi: 10.7150/ijbs.3.179
Maiuolo, J., Musolino, V., Gliozzi, M., Carresi, C., Scarano, F., Nucera, S., et al. (2022). Involvement of the intestinal microbiota in the appearance of multiple sclerosis: Aloe vera and Citrus bergamia as potential candidates for intestinal health. Nutrients 14:2711. doi: 10.3390/nu14132711
Marks, A. R., Mcintyre, J. O., Duncan, T. M., Erdjument-Bromage, H., Tempst, P., and Fleischer, S. (1992). Molecular cloning and characterization of (R)-3-hydroxybutyrate dehydrogenase from human heart. J. Biol. Chem. 267, 15459–15463. doi: 10.1016/S0021-9258(19)49556-2
Mazzoli, R., and Pessione, E. (2016). The neuro-endocrinological role of microbial glutamate and GABA signaling. Front. Microbiol. 7:1934. doi: 10.3389/fmicb.2016.01934
Meurens, F., Summerfield, A., Nauwynck, H., Saif, L., and Gerdts, V. (2012). The pig: a model for human infectious diseases. Trends Microbiol. 20, 50–57. doi: 10.1016/j.tim.2011.11.002
Moran, E. T., and Bedford, M. R. (2022). Large intestinal dynamics differ between fowl and swine: anatomical modifications, microbial collaboration, and digestive advantages from fibrolytic enzymes. Anim. Nutr. 11, 160–170. doi: 10.1016/j.aninu.2022.07.004
Mörbe, U. M., Jørgensen, P. B., Fenton, T. M., von Burg, N., Riis, L. B., Spencer, J., et al. (2021). Human gut-associated lymphoid tissues (GALT); diversity, structure, and function. Mucosal Immunol. 14, 793–802. doi: 10.1038/s41385-021-00389-4
Moreira Júnior, R. E., De Carvalho, L. M., Dos Reis, D. C., Cassali, G. D., Faria, A. M. C., Maioli, T. U., et al. (2021). Diet-induced obesity leads to alterations in behavior and gut microbiota composition in mice. J. Nutr. Biochem. 92:108622. doi: 10.1016/j.jnutbio.2021.108622
Narduzzi, L., Hernández-Mesa, M., Vincent, P., Guitton, Y., García-Campaña, A. M., le Bizec, B., et al. (2023). Deeper insights into the effects of low dietary levels of polychlorinated biphenyls on pig metabolism using gas chromatography-high resolution mass spectrometry metabolomics. Chemosphere 341:140048. doi: 10.1016/j.chemosphere.2023.140048
Ndeh, D., Rogowski, A., Cartmell, A., Luis, A. S., Baslé, A., Gray, J., et al. (2017). Complex pectin metabolism by gut bacteria reveals novel catalytic functions. Nature 544, 65–70. doi: 10.1038/nature21725
Niknafs, Y. S., Pandian, B., Iyer, H. K., Chinnaiyan, A. M., and Iyer, M. K. (2017). TACO produces robust multisample transcriptome assemblies from RNA-seq. Nat. Methods 14, 68–70. doi: 10.1038/nmeth.4078
Nishino, K., and Yamaguchi, A. (2002). EvgA of the two-component signal transduction system modulates production of the yhiUV multidrug transporter in Escherichia coli. J. Bacteriol. 184, 2319–2323. doi: 10.1128/JB.184.8.2319-2323.2002
Oleksy, M., and Klewicka, E. (2018). Exopolysaccharides produced by Lactobacillus sp.: biosynthesis and applications. Crit. Rev. Food Sci. Nutr. 58, 450–462. doi: 10.1080/10408398.2016.1187112
Oliphant, K., and Allen-Vercoe, E. (2019). Macronutrient metabolism by the human gut microbiome: major fermentation by-products and their impact on host health. Microbiome 7:91. doi: 10.1186/s40168-019-0704-8
Otsuka, H., Kimura, T., Ago, Y., Nakama, M., Aoyama, Y., Abdelkreem, E., et al. (2020). Deficiency of 3-hydroxybutyrate dehydrogenase (BDH1) in mice causes low ketone body levels and fatty liver during fasting. J. Inherit. Metab. Dis. 43, 960–968. doi: 10.1002/jimd.12243
Pabst, R. (2020). The pig as a model for immunology research. Cell Tissue Res. 380, 287–304. doi: 10.1007/s00441-020-03206-9
Panagea, S., Bijoux, R., Corkill, J. E., al Rashidi, F., and Hart, C. A. (2002). A case of lower respiratory tract infection caused by Neisseria weaveri and review of the literature. J. Infect. 44, 96–98. doi: 10.1053/jinf.2001.0965
Patro, R., Duggal, G., Love, M. I., Irizarry, R. A., and Kingsford, C. (2017). Salmon provides fast and bias-aware quantification of transcript expression. Nat. Methods 14, 417–419. doi: 10.1038/nmeth.4197
Perleberg, C., Kind, A., and Schnieke, A. (2018). Genetically engineered pigs as models for human disease. Dis. Model. Mech. 11:dmm030783. doi: 10.1242/dmm.030783
Qiao, N., Yang, Y., Liao, J., Zhang, H., Yang, F., Ma, F., et al. (2021). Metabolomics and transcriptomics indicated the molecular targets of copper to the pig kidney. Ecotoxicol. Environ. Saf. 218:112284. doi: 10.1016/j.ecoenv.2021.112284
Ramos, L., Lunney, J. K., and Gonzalez-Juarrero, M. (2020). Neonatal and infant immunity for tuberculosis vaccine development: importance of age-matched animal models. Dis. Model. Mech. 13:dmm045740. doi: 10.1242/dmm.045740
Rath, E., and Haller, D. (2022). Intestinal epithelial cell metabolism at the interface of microbial dysbiosis and tissue injury. Mucosal Immunol. 15, 595–604. doi: 10.1038/s41385-022-00514-x
Roura, E., Koopmans, S. J., Lallès, J. P., le Huerou-Luron, I., de Jager, N., Schuurman, T., et al. (2016). Critical review evaluating the pig as a model for human nutritional physiology. Nutr. Res. Rev. 29, 60–90. doi: 10.1017/S0954422416000020
Rubino, F., Carberry, C., Waters, M. S., Kenny, D., McCabe, M. S., and Creevey, C. J. (2017). Divergent functional isoforms drive niche specialisation for nutrient acquisition and use in rumen microbiome. ISME J. 11, 932–944. doi: 10.1038/ismej.2016.172
Rupp, J., Guckert, M., Berger, U., Drost, W., Mader, A., Nödler, K., et al. (2023). Comprehensive target analysis and TOP assay of per-and polyfluoroalkyl substances (PFAS) in wild boar livers indicate contamination hot-spots in the environment. Sci. Total Environ. 871:162028. doi: 10.1016/j.scitotenv.2023.162028
Safron, J., and Gonder, J. C. (1997). The SPF pig in research. ILAR J. 38, 28–31. doi: 10.1093/ilar.38.1.28
Sandhu, B. K., and Mcbride, S. M. (2018). Clostridioides difficile. Trends Microbiol. 26, 1049–1050. doi: 10.1016/j.tim.2018.09.004
Segata, N., Izard, J., Waldron, L., Gevers, D., Miropolsky, L., Garrett, W. S., et al. (2011). Metagenomic biomarker discovery and explanation. Genome Biol. 12:R60. doi: 10.1186/gb-2011-12-6-r60
Sengupta, C., Ray, S., and Chowdhury, R. (2014). Fine tuning of virulence regulatory pathways in enteric bacteria in response to varying bile and oxygen concentrations in the gastrointestinal tract. Gut Pathogens 6:38. doi: 10.1186/s13099-014-0038-9
Song, B., Zheng, C., Zheng, J., Zhang, S., Zhong, Y., Guo, Q., et al. (2022). Comparisons of carcass traits, meat quality, and serum metabolome between Shaziling and Yorkshire pigs. Anim. Nutr. 8, 125–134. doi: 10.1016/j.aninu.2021.06.011
Su, M. S., Schlicht, S., and Gänzle, M. G. (2011). Contribution of glutamate decarboxylase in Lactobacillus reuteri to acid resistance and persistence in sourdough fermentation. Microb. Cell Factories 10:S8. doi: 10.1186/1475-2859-10-S1-S8
Swindle, M. M., Makin, A., Herron, A. J., Clubb, F. J. Jr., and Frazier, K. S. (2012). Swine as models in biomedical research and toxicology testing. Vet. Pathol. 49, 344–356. doi: 10.1177/0300985811402846
Swindle, M. M., Wiest, D. B., Smith, A. C., Garner, S. S., Case, C. C., Thompson, R. P., et al. (1996). Fetal surgical protocols in Yucatan miniature swine. Lab. Anim. Sci. 46, 90–95
Tamanai-Shacoori, Z., Smida, I., Bousarghin, L., Loreal, O., Meuric, V., Fong, S. B., et al. (2017). Roseburia spp.: a marker of health? Future Microbiol. 12, 157–170. doi: 10.2217/fmb-2016-0130
Tennoune, N., Andriamihaja, M., and Blachier, F. (2022). Production of indole and indole-related compounds by the intestinal microbiota and consequences for the host: the good, the bad, and the ugly. Microorganisms 10:930. doi: 10.3390/microorganisms10050930
Tomova, A., Bukovsky, I., Rembert, E., Yonas, W., Alwarith, J., Barnard, N. D., et al. (2019). The effects of vegetarian and vegan diets on gut microbiota. Front. Nutr. :47:6. doi: 10.3389/fnut.2019.00047
Tong, F., Wang, T., Gao, N. L., Liu, Z., Cui, K., Duan, Y., et al. (2022). The microbiome of the buffalo digestive tract. Nat. Commun. 13:823. doi: 10.1038/s41467-022-28402-9
Trapnell, C., Williams, B. A., Pertea, G., Mortazavi, A., Kwan, G., van Baren, M. J., et al. (2010). Transcript assembly and quantification by RNA-Seq reveals unannotated transcripts and isoform switching during cell differentiation. Nat. Biotechnol. 28, 511–515. doi: 10.1038/nbt.1621
Turnbaugh, P. J., Ley, R. E., Mahowald, M. A., Magrini, V., Mardis, E. R., and Gordon, J. I. (2006). An obesity-associated gut microbiome with increased capacity for energy harvest. Nature 444, 1027–1031. doi: 10.1038/nature05414
Vital, M., Howe, A. C., and Tiedje, J. M. (2014). Revealing the bacterial butyrate synthesis pathways by analyzing (meta)genomic data. MBio 5:e00889. doi: 10.1128/mBio.00889-14
Wang, X., Tsai, T., Deng, F., Wei, X., Chai, J., Knapp, J., et al. (2019). Longitudinal investigation of the swine gut microbiome from birth to market reveals stage and growth performance associated bacteria. Microbiome 7:109. doi: 10.1186/s40168-019-0721-7
Wang, W., Zhai, S., Xia, Y., Wang, H., Ruan, D., Zhou, T., et al. (2019). Ochratoxin a induces liver inflammation: involvement of intestinal microbiota. Microbiome 7:151. doi: 10.1186/s40168-019-0761-z
Williams, B. A., Verstegen, M. W., and Tamminga, S. (2001). Fermentation in the large intestine of single-stomached animals and its relationship to animal health. Nutr. Res. Rev. 14, 207–228. doi: 10.1079/NRR200127
Wilson, I. D., and Nicholson, J. K. (2017). Gut microbiome interactions with drug metabolism, efficacy, and toxicity. Transl. Res. 179, 204–222. doi: 10.1016/j.trsl.2016.08.002
Wu, T., Hu, E., Xu, S., Chen, M., Guo, P., Dai, Z., et al. (2021). clusterProfiler 4.0: a universal enrichment tool for interpreting omics data. Innovation (Camb) 2:100141. doi: 10.1016/j.xinn.2021.100141
Xiao, L., Estellé, J., Kiilerich, P., Ramayo-Caldas, Y., Xia, Z., Feng, Q., et al. (2016). A reference gene catalogue of the pig gut microbiome. Nat. Microbiol. 1:16161. doi: 10.1038/nmicrobiol.2016.161
Xiao, Y., Xiang, Y., Zhou, W., Chen, J., Li, K., and Yang, H. (2017). Microbial community mapping in intestinal tract of broiler chicken. Poult. Sci. 96, 1387–1393. doi: 10.3382/ps/pew372
Xu, Y., Sim, S. H., Nam, K. H., Jin, X. L., Kim, H. M., Hwang, K. Y., et al. (2009). Crystal structure of the periplasmic region of MacB, a noncanonic ABC transporter. Biochemistry 48, 5218–5225. doi: 10.1021/bi900415t
Yang, H., Huang, X., Fang, S., Xin, W., Huang, L., and Chen, C. (2016). Uncovering the composition of microbial community structure and metagenomics among three gut locations in pigs with distinct fatness. Sci. Rep. 6:27427. doi: 10.1038/srep27427
Ye, M., Yu, J., Shi, X., Zhu, J., Gao, X., and Liu, W. (2020). Polysaccharides catabolism by the human gut bacterium-Bacteroides thetaiotaomicron: advances and perspectives. Crit. Rev. Food Sci. Nutr. 61, 3569–3588. doi: 10.1080/10408398.2020.1803198
Yu, C., Guo, Z., Lei, Z., Mao, X., Chen, S., and Wang, K. (2023). Comparison of fecal microbiota of SPF and non-SPF beagle dogs. Front. Vet. Sci. 10:1021371. doi: 10.3389/fvets.2023.1021371
Zhang, L., Li, N., Zhang, J., Zhao, L., Qiu, J., and Wei, C. (2022). The CBM48 domain-containing protein FLO6 regulates starch synthesis by interacting with SSIVb and GBSS in rice. Plant Mol. Biol. 108, 343–361. doi: 10.1007/s11103-021-01178-0
Zhang, J., Shen, Y., Yang, G., Sun, J., Tang, C., Liang, H., et al. (2023). Commensal microbiota modulates phenotypic characteristics and gene expression in piglet Peyer's patches. Front. Physiol. 14:1084332. doi: 10.3389/fphys.2023.1084332
Zhao, M., Ren, K., Xiong, X., Cheng, M., Zhang, Z., Huang, Z., et al. (2020). Protein O-GlcNAc modification links dietary and gut microbial cues to the differentiation of Enteroendocrine L cells. Cell Rep. 32:108013. doi: 10.1016/j.celrep.2020.108013
Keywords: specific pathogen-free pigs, metagenomics, transcriptomics, gut microbiota, host-microbial interactions
Citation: Wen M, Chen S, Zhang Y, Liu Y, Tang C, Zhang J, Sun J, Li X, Ding Y, Lu L, Long K, Nie Y, Li X, Li M, Ge L and Ma J (2024) Diversity and host interaction of the gut microbiota in specific pathogen-free pigs. Front. Microbiol. 15:1402807. doi: 10.3389/fmicb.2024.1402807
Edited by:
Benoit St-Pierre, South Dakota State University, United StatesReviewed by:
Yafei Duan, South China Sea Fisheries Research Institute, ChinaHengjia Ni, Chinese Academy of Sciences (CAS), China
Copyright © 2024 Wen, Chen, Zhang, Liu, Tang, Zhang, Sun, Li, Ding, Lu, Long, Nie, Li, Li, Ge and Ma. This is an open-access article distributed under the terms of the Creative Commons Attribution License (CC BY). The use, distribution or reproduction in other forums is permitted, provided the original author(s) and the copyright owner(s) are credited and that the original publication in this journal is cited, in accordance with accepted academic practice. No use, distribution or reproduction is permitted which does not comply with these terms.
*Correspondence: Liangpeng Ge, Z2VsaWFuZ3BlbmcxOTgyQDE2My5jb20=; Jideng Ma, amlkZW5nLm1hQHNpY2F1LmVkdS5jbg==
†These authors share first authorship