- 1Juniata College, Huntingdon, PA, United States
- 2Wright Labs LLC, Huntingdon, PA, United States
- 3Molecular Biology and Microbiology, Tufts University, Boston, MA, United States
- 4University of Virginia School of Medicine, Charlottesville, VA, United States
- 5Department of Surgery, Southern Illinois University School of Medicine, Springfield, IL, United States
Clostridioides difficile infection (CDI) is responsible for around 300,000 hospitalizations yearly in the United States, with the associated monetary cost being billions of dollars. Gut microbiome dysbiosis is known to be important to CDI. To the best of our knowledge, metatranscriptomics (MT) has only been used to characterize gut microbiome composition and function in one prior study involving CDI patients. Therefore, we utilized MT to investigate differences in active community diversity and composition between CDI+ (n = 20) and CDI− (n = 19) samples with respect to microbial taxa and expressed genes. No significant (Kruskal-Wallis, p > 0.05) differences were detected for richness or evenness based on CDI status. However, clustering based on CDI status was significant for both active microbial taxa and expressed genes datasets (PERMANOVA, p ≤ 0.05). Furthermore, differential feature analysis revealed greater expression of the opportunistic pathogens Enterocloster bolteae and Ruminococcus gnavus in CDI+ compared to CDI− samples. When only fungal sequences were considered, the family Saccharomycetaceae expressed more genes in CDI−, while 31 other fungal taxa were identified as significantly (Kruskal-Wallis p ≤ 0.05, log(LDA) ≥ 2) associated with CDI+. We also detected a variety of genes and pathways that differed significantly (Kruskal-Wallis p ≤ 0.05, log(LDA) ≥ 2) based on CDI status. Notably, differential genes associated with biofilm formation were expressed by C. difficile. This provides evidence of another possible contributor to C. difficile’s resistance to antibiotics and frequent recurrence in vivo. Furthermore, the greater number of CDI+ associated fungal taxa constitute additional evidence that the mycobiome is important to CDI pathogenesis. Future work will focus on establishing if C. difficile is actively producing biofilms during infection and if any specific fungal taxa are particularly influential in CDI.
1 Introduction
Hospital-acquired Clostridioides difficile infection (CDI) accounts for approximately 300,000 hospitalizations per year (Bobo et al., 2011) with mortality rates as high as 16.7% during outbreaks (Dubberke et al., 2008), and it has surpassed methicillin-resistant Staphylococcus aureus as the highest incidence hospital-acquired infection in the United States (Lessa et al., 2015), all resulting in billions of dollars in annual healthcare costs (Dubberke and Olsen, 2012). Antibiotics remain the most important risk factor for CDI due to their mechanism of action lacking species-level specificity, with their broad impact on gut ecology creating an intestinal dysbiosis in which C. difficile has a selective advantage for population growth (Aslam et al., 2005; Shivashankar et al., 2014). Antibiotics are also the most common intervention for CDI, with unacceptably high recurrence rates of 15%–30% after first treatment, representing another manifestation of their causal influence in creating gut microbial environments that promote this disease state (Choi et al., 2011). These observations underscore the importance of gut dysbiosis in the pathogenesis of CDI, which has implications both for disease prevention and disease treatment. A more precise understanding of the dysbiosis of CDI may, therefore, impact the prevention and treatment of this disease.
The majority of microbiome studies on CDI have used amplicons (16S rRNA and/or ITS) to characterize the dominant microbial communities in CDI (Antharam et al., 2013; Sangster et al., 2016; Lamendella et al., 2018; Zuo et al., 2018). However, the studies that relied solely on 16S rRNA data were unable to comment on the possible role of fungi in the pathogenesis of CDI. More recent investigations, including several by our team, suggest that fungi may have a role in CDI (Lamendella et al., 2018; Markey et al., 2018; Stewart et al., 2019). These data suggest that pre-colonization of mice with C. albicans lowers their susceptibility to CDI (Markey et al., 2018). Several human cohort studies also demonstrate the consistent presence of fungi, especially C. glabrata, in patients with CDI, while CDI− patients with diarrhea and comparable antibiotic exposures lack this fungal enrichment (Lamendella et al., 2018; Stewart et al., 2019). Furthermore, while predictive functional tools exist, namely PICRUSt2 (Douglas et al., 2020) and Tax4Fun2 (Wemheuer et al., 2020), 16S rRNA and ITS data do not allow for the direct detection of expressed genes, and neither of those tools provide any information on which of the predictive functions were likely being expressed.
Shotgun metagenomics can directly detect genes, but it does not distinguish between genes that were expressed vs. those that were only present. In contrast, metatranscriptomics (MT) both overcomes limitations associated with amplicon sequencing and allows us to focus on transcriptionally active microbes, as well as the genes they were expressing. Therefore, we once more utilized MT sequencing to characterize microbial communities within the context of CDI. In this study, we sought to examine potential differences in overall community diversity and identify differentially active microbes and differentially expressed functions based on CDI status. Additionally, building on previous work, we were able to link specific genes and pathways to fungal expression. Despite only having information on CDI status, we were able to detect multiple differences in active community composition and function, which seem to have remained consistent regardless of possible confounding variables.
2 Materials and methods
2.1 Sample collection
The Institutional Review Board at the University of Virginia approved this study (IRB-HSR# 21646) with waiver of consent, as samples were de-identified remnants. Diarrheal samples from CDI+ (n = 20) and CDI− (n = 20) patients collected by the University of Virginia Medical Center between September 2019 and August 2020. CDI was diagnosed based on the presence of a conserved sequence of the tcdB gene, as determined by Xpert® C. difficile assay (Cepheid, Sunnyvale, CA, United States). To be included in the study, patients had to be at least 18 years old. Samples were stored in a −80°C freezer after collection until further processing.
2.2 RNA extraction, library preparation, and sequencing
RNA was extracted from samples using the ZymoBIOMICS RNA Miniprep Kit (Zymo Research, Irvine, CA, United States) according to the manufacturer’s protocol with the following exceptions: 1 volume of lysis buffer was used, the optional DNase I treatment was completed, and extracts were eluted with 50 uL of DNase/RNase free water. After extraction, quantification was conducted using an Invitrogen Qubit 4 Fluorometer and Qubit RNA High Sensitivity Assay Kit (ThermoFisher Scientific, Waltham, MA, United States).
RNA was then processed to make MT libraries using the NEBNext Ultra II RNA Library Prep Kit for use with Illumina (New England Biolabs, Ipswich, MA, United States), which utilizes a random priming approach for reverse transcription. The protocol specifically for use with purified mRNA was followed. Depletion of rRNA was not performed.
Libraries were quality checked using an Agilent 2100 Bioanalyzer and DNA High Sensitivity kit. Results from these steps were used to pool samples in an equimolar ratio. The pool was then gel purified using a 2% agarose gel and the Qiagen QIAquick gel extraction kit (Qiagen, Germantown, MD, United States). Following purification, the pool was sequenced using an Illumina NextSeq 550 platform to produce 2 × 150 bp reads by Wright Labs LLC (Huntingdon, PA, United States).
2.3 Bioinformatics and statistical analyses
2.3.1 Quality checking and sequence annotation
Quality was checked in the raw sequence data using VSEARCH (Rognes et al., 2016). Following initial quality evaluation, fastp (Chen et al., 2018) was used to filter the data with a sliding window of 4 with a minimum average Phred Q score of 20 in which a window not meeting the average would result in the window as well as the remainder of the sequence being dropped. Sequences shorter than 90 bp after filtering were discarded.
Kraken2 (Wood et al., 2019) was subsequently used to taxonomically annotate the remaining sequences with a database, including its standard libraries, as well as fungi. Species-level annotations were then collated into a table for use with downstream analyses. Counts for the species Homo sapiens were excluded to avoid human contamination impacting results. Another table was created by subsetting the full species table to only include fungal species.
The Kraken2-annotated sequences, except for those identified as Homo sapiens, were paired with PEAR (Zhang et al., 2014) and dereplicated with VSEARCH. Emapper v2.0 (Huerta-Cepas et al., 2017) using the eggNOG 5.0 database (Huerta-Cepas et al., 2019) was run on the dereplicated sequences. Hits against KEGG Orthologs (KOs) were used to create a table of reads per kilobase (rpk) values for downstream analysis. During table creation, the counts were rpk normalized by dividing sequence occurrences by three times the respective length of the protein they were annotated as (to convert from amino acid length to nucleotide length) and multiplying the quotient by 1,000. The full KOs table was subsetted to create another table containing only annotations of fungal sequences.
Additionally, a fifth dataset containing predicted metabolites based on the paired filtered data was created for use with LEfSe analysis (Segata et al., 2011). The filtered paired sequences were used with HUMAnN3 (Beghini et al., 2021) to generate UniRef90 annotations as input for MelonnPan (Mallick et al., 2019) predicted metabolite analysis using their pre-trained model (Franzosa et al., 2019). The “Unmapped” row was removed from the table, and UniRef90 counts were converted to relative abundances prior to MelonnPan analysis.
In total, five datasets were generated and used for all subsequent analyses: total active species, fungal active species, total expressed genes, fungal expressed genes, and predicted metabolites. Of the five, total active species and total expressed genes were used with all subsequent analyses, while the other three were only used for differential feature analysis. One sample (73) was omitted from all analyses due to yielding fewer than 500,000 raw sequences.
2.3.2 Alpha diversity analysis
Alpha diversity was calculated by subsampling the active microbial taxa and expressed genes dataset tables at 10 different depths, ranging from 99,000 to 990,000 for the active microbial species dataset (Supplementary Figure S1) and 590 to 5,900 for the expressed genes dataset (Supplementary Figure S2). A single sample (68) was omitted from alpha diversity analyses with the microbial taxa dataset to facilitate a higher rarefaction depth as opposed to having to use a maximum depth of 117,600 instead to retain it. Twenty iterations were performed at each depth to obtain average alpha diversity values for the different metrics [Observed Features and Pielou’s Evenness (Pielou, 1966)]. Averages for the greatest depth were used to see if any alpha diversity metrics differed significantly based on CDI status (Kruskal-Wallis, p ≤ 0.05) with QIIME2 (Bolyen et al., 2019).
2.3.3 Beta diversity analysis
Beta diversity analyses were conducted after the tables had first undergone counts per million normalization to mitigate differences between samples based on sequencing depth. The Bray-Curtis distance metric (Sørensen, 1948) was used to create a distance matrix for both datasets. The resulting distance matrices were visualized as Principal Coordinates Analysis plots with 95% confidence intervals around the centroids using the ggordiplot package (Quensen, 2020) through R (R Core Team, 2023). Statistical differences between sample groupings based on CDI status were evaluated as well (PERMANOVA, p ≤ 0.05) through QIIME2.
2.3.4 Differential feature analysis
Differential feature analysis was performed using LEfSe (Segata et al., 2011) to identify features (genes, pathways, predicted metabolites, and taxa) that had significantly different abundances based on CDI status. For all datasets, the table was normalized with the counts per million (CPM) method. Only features identified as having significantly differential abundance (Kruskal-Wallis, p ≤ 0.05) with a log(LDA) score of at least 2.0 were considered to be enriched, with the exception of the predicted metabolites dataset in which features only had to differ significantly based on Kruskal-Wallis. Levene’s test was performed using the rstatix package (Kassambara, 2020) in R with CPM-normalized values for differential features identified within the active microbial species dataset, as several of the most differential taxa seemed to be highly variable.
SparseDossa2 (Ma et al., 2021) was used to simulate 1,000 tables with various minimum fold change differences for the active microbial taxa and expressed genes datasets in order to assess the corresponding change required to achieve 80% power for LEfSe to detect significant features. Each simulated table consisted of 39 samples, split into two groups of sizes 19 and 20, and was based on a SparseDossa2 model fitted for the respective data type.
For every simulation, the active microbial taxa simulated tables used 17 of the 346 species (5%) that were present in at least 30% of the samples with a minimum average relative abundance of 0.01% to model differential features and 76 of the 1,515 KOs (5%) meeting those requirements were used for each simulation based on the expressed genes dataset. Simulated samples had an average depth of 10,000,000 for both the active microbial taxa and expressed genes datasets. All simulated tables were CPM-normalized prior to being used as input for LEfSe and subject to the same criteria as the observed data to be considered differential. Differential features associated with the expected group were counted as true positives.
2.3.5 Differential contributors analysis
The stratified gene contribution table was subsetted to include only genes of interest. The rpk-normalized values were then converted to relative abundances such that for each sample, the values of the taxa contributing to the gene(s) of interest summed to 100. Wilcoxon rank sum tests were then used to assess the significance of differences in relative contribution based on CDI status.
2.3.6 Machine learning
Random forest models were created to assess how well the features identified as differential by LEfSe could be used to predict CDI status compared to models trained on the full datasets. The models were generated with Scikit-learn in Python based on 500 decision trees (Pedregosa et al., 2011). They were evaluated using k-fold cross-validation with five repetitions of 10 folds. The datasets used for model creation include the full species datasets and the species identified as differential by LEfSe. Those two datasets were used to generate additional models after excluding Clostridioides difficile. Models were also generated using the full genes dataset and the genes identified as differential. All datasets were subject to CPM normalization prior to model creation. Feature importance was evaluated using Gini importance, and the 10 most important features were plotted for all models.
2.3.7 CoNet analysis
Cooccurrence networks for the active microbial taxa datasets were generated with CoNet (Faust and Raes, 2016) in Cytoscape (Shannon et al., 2003), using automatic thresholds for Spearman correlation (Spearman Rank Correlation Coefficient, 2008), Bray-Curtis (Sørensen, 1948), and Kullback–Leibler (Kullback and Leibler, 1951), with an edge selection parameter of 100 and a minimum occurrence of 10 samples for a feature to be considered. The p-values from the individual methods were combined with the Brown method (Brown, 1975), and Benjamini-Hochberg (Benjamini and Hochberg, 1995) p-value correction was performed with a cutoff of 0.05 for significance. Networks were created for CDI+ Total Active Species and CDI− Total Active Species datasets and then juxtaposed using CytoGEDEVO (Malek et al., 2016).
3 Results
3.1 Description of sequencing results
In total, 40 samples were subjected to MT sequencing, yielding 759,555,378 raw sequences, and after quality filtration, 639,700,992 sequences remained. Of those samples, 39 (CDI + =20, CDI− = 19) had enough data for downstream analysis after quality filtering (range 942,194–82,265,454 sequences per sample, Figure 1).
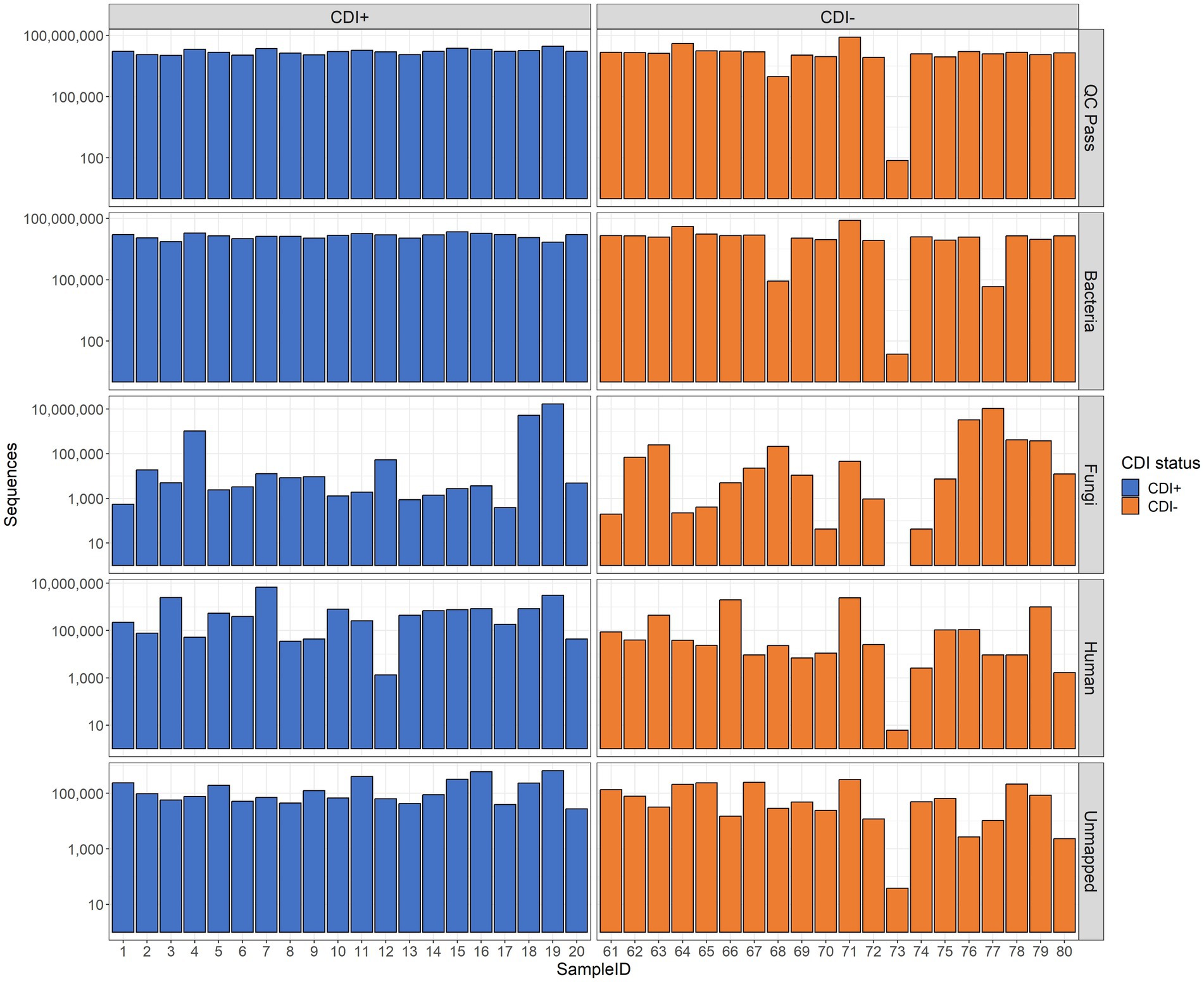
Figure 1. Bar plots of sequencing results. The number of sequences retained after quality control (QC Pass), annotated as Bacteria (Bacteria), annotated as Fungi (Fungi), annotated as Homo sapiens (Human), and unidentified by Kraken2 (Unmapped) are shown. Raw sequence counts are shown on the y-axis. The x-axis shows individual samples. Each patient was associated with a single sample.
3.2 Richness and evenness
Pielou’s evenness values within the expressed genes dataset tended to be higher in CDI− samples compared to the CDI+ cohort, though the difference was not significant (Table 1). Overall, alpha diversity was variable among samples but did not differ significantly between CDI+ and CDI− for the active microbial taxa or expressed genes datasets based on either the Pielou’s evenness or Observed Features metrics (Figure 2).
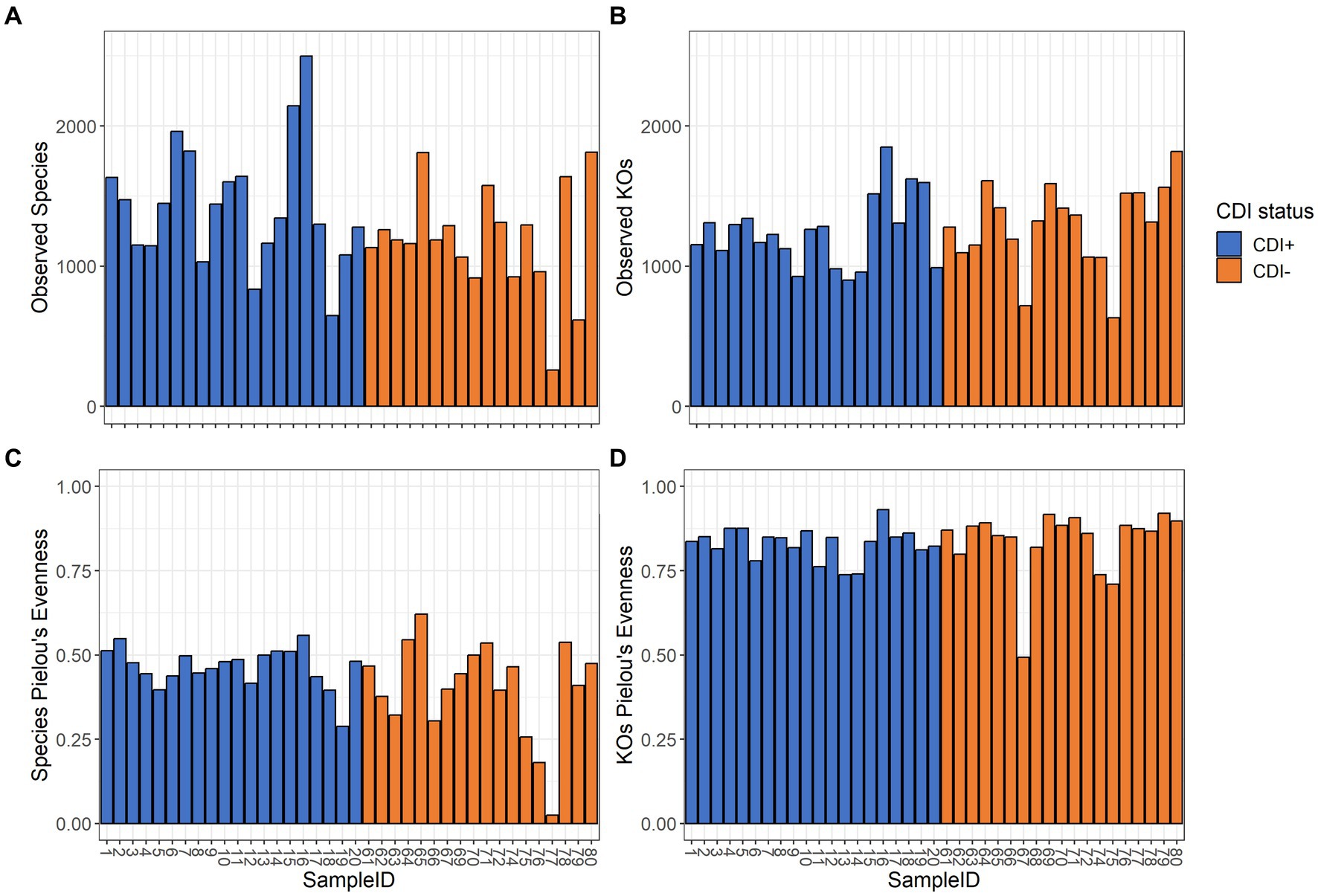
Figure 2. Alpha diversity values for the active composition and expressed genes datasets based on the Observed Features and Pielou’s Evenness metrics per sample. Samples are colored based on CDI status. Sample 68 was omitted from the active microbial species analyses to allow for a greater maximum rarefaction depth. (A) Observed Features within the active microbial species dataset. (B) Observed Features within the expressed genes dataset. (C) Pielou’s evenness within the active microbial species dataset. (D) Pielou’s evenness within the expressed genes dataset. Alpha diversity did not differ significantly (Kruskal-Wallis, p ≤ 0.05) based on CDI status within either dataset by either metric.
3.3 Differential active microbial communities
Significant clustering based on CDI status was observed for both active taxa and expressed gene datasets (PERMANOVA, p = 0.005 and p = 0.013, respectively; Figure 3). Subsequent LEfSe analysis identified the microbial taxa and KEGG Orthologs (KOs) driving differential clustering.
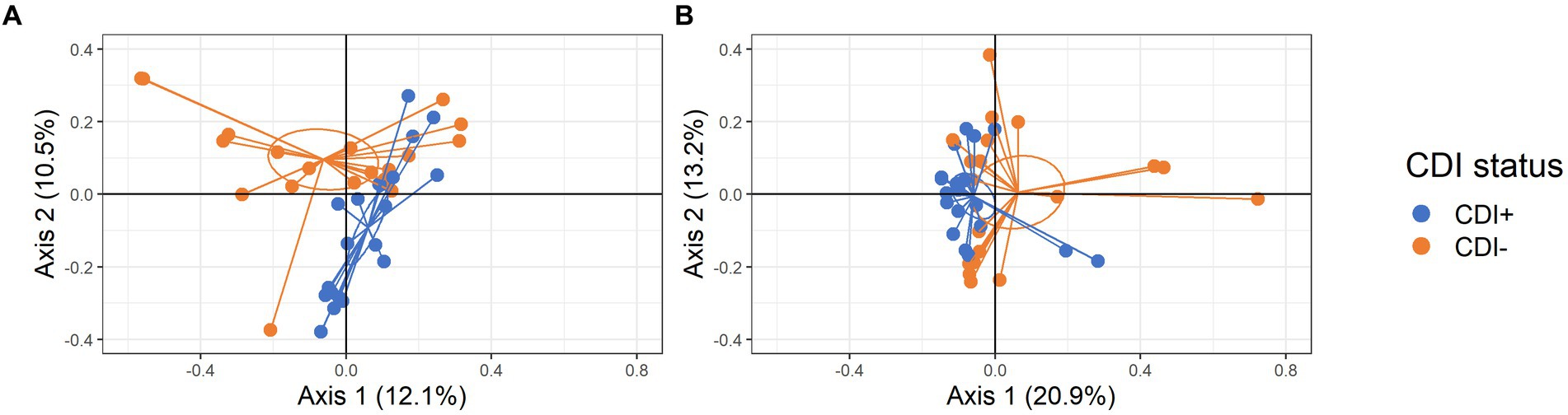
Figure 3. PCoA plots of samples colored by CDI status with centroids shown within 95% confidence interval ellipses based on Bray-Curtis distances calculated with active microbial species (A) and expressed genes (B).
The commensal bacteria Clostridium butyricum, Lacticaseibacillus rhamnosus, and Roseburia intestinalis were the most differentially active microbial species in CDI− (Figure 4). However, variability in the expression of all three taxa within CDI− was relatively high (Supplementary Table S1). In contrast, though its corresponding LDA score was lower, Lactiplantibacillus plantarum was more consistently active in the CDI− samples (Supplementary Table S1).
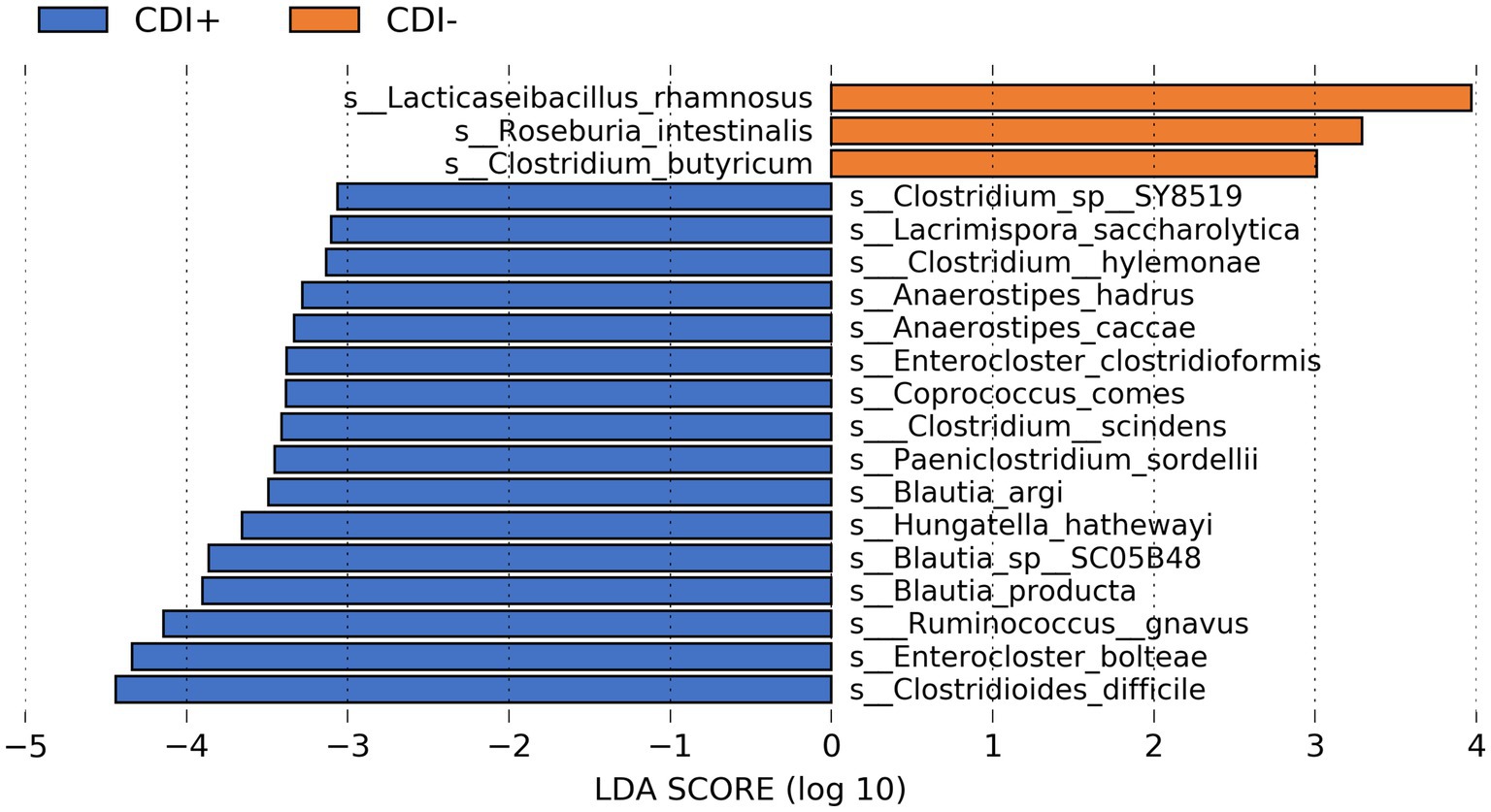
Figure 4. Bar plot of LEfSe results showing highly differential active species (LDA ≥ 3.0, Kruskal-Wallis p ≤ 0.05) based on CDI status. See Supplementary Table S1 for all active taxa identified as differential by LEfSe.
Multiple butyrate producers were identified as being more active in CDI+, including Anaerostipes hadrus (Allen-Vercoe et al., 2012), Coproccocus comes (Louis and Flint, 2009), and Roseburia hominis (Louis and Flint, 2009), and all three were found to express genes within the Butanoate Metabolism pathway (ko00650) in this study. However, the taxa Clostridioides difficile, Enterocloster bolteae, and Ruminococcus gnavus were the three most differentially active species in CDI+ (Kruskal-Wallis, p ≤ 0.05, log(LDA) ≥ 3.0), with C. difficile being the most differential (Supplementary Figure S3). Clostridium scindens was also identified as being more active in CDI+.
When our microbial taxa table was subsetted to include only fungal taxa, the family Saccharomycetaceae was more active in CDI− samples (Figure 5). In contrast, 31 fungal taxa were more active in CDI+ (Supplementary Table S2). Several differential genes (Supplementary Table S3) and pathways (Supplementary Table S4) were also identified within the fungi functional gene datasets. However, we recovered relatively low numbers of fungal sequences (Supplementary Figure S4).
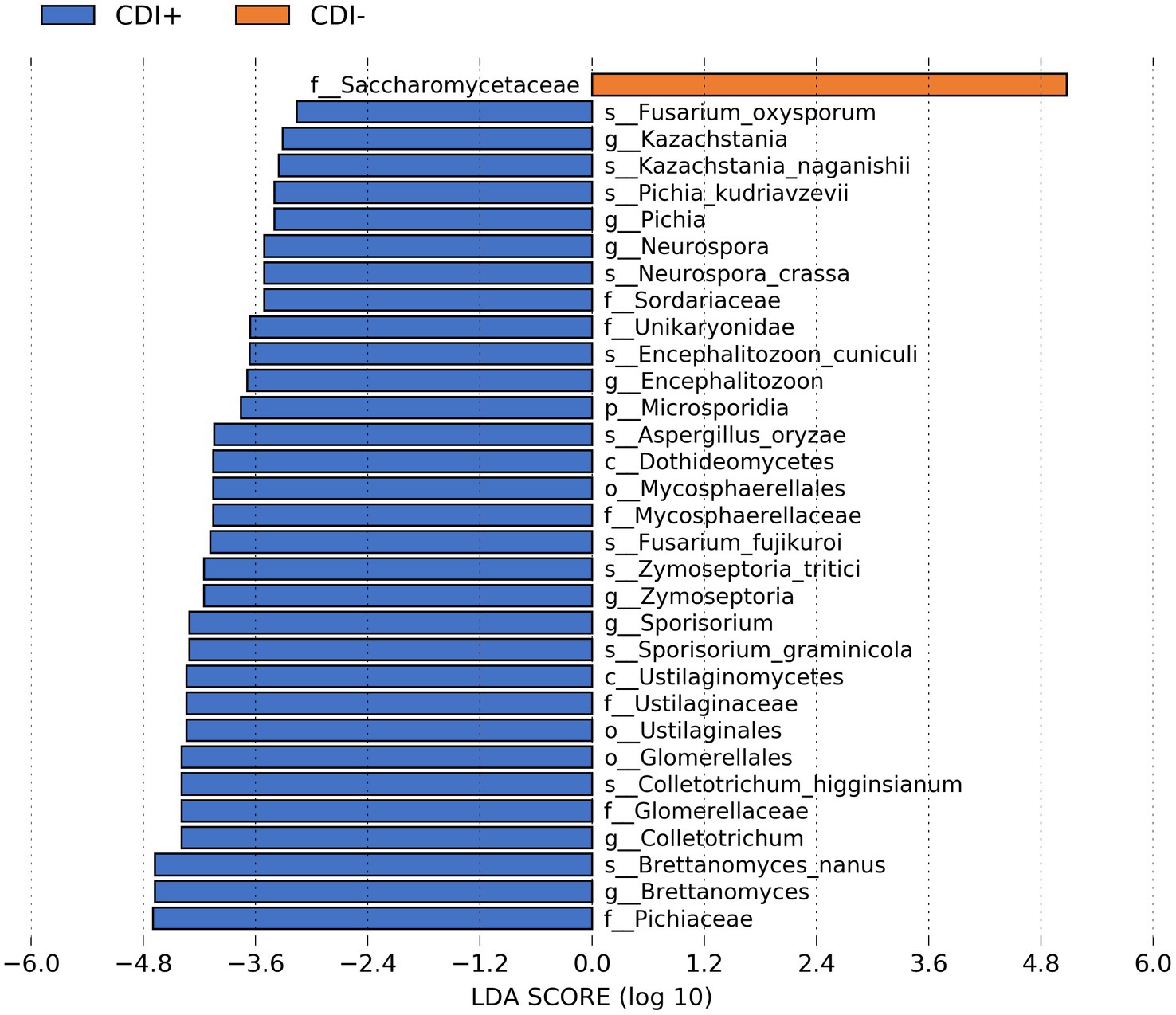
Figure 5. Bar plot of LEfSe results showing differential active fungal taxa (LDA ≥ 2.0, Kruskal-Wallis p ≤ 0.05) based on CDI status.
More differential genes were identified within the CDI+ cohort in the total expressed genes dataset (CDI+ genes = 99, CDI− genes = 31), including several that were especially of interest due to their involvement with biofilm formation or sporulation and their expression by C. difficile (Table 2). However, a greater number of pathways were expressed associated as differential in CDI− (Supplementary Table S5). Interestingly, 22 of the genes more expressed in CDI+ code for ribosomal subunits (Supplementary Table S6). The most significantly different contributors to those genes were taxa significantly more active in CDI+ (Supplementary Table S7).
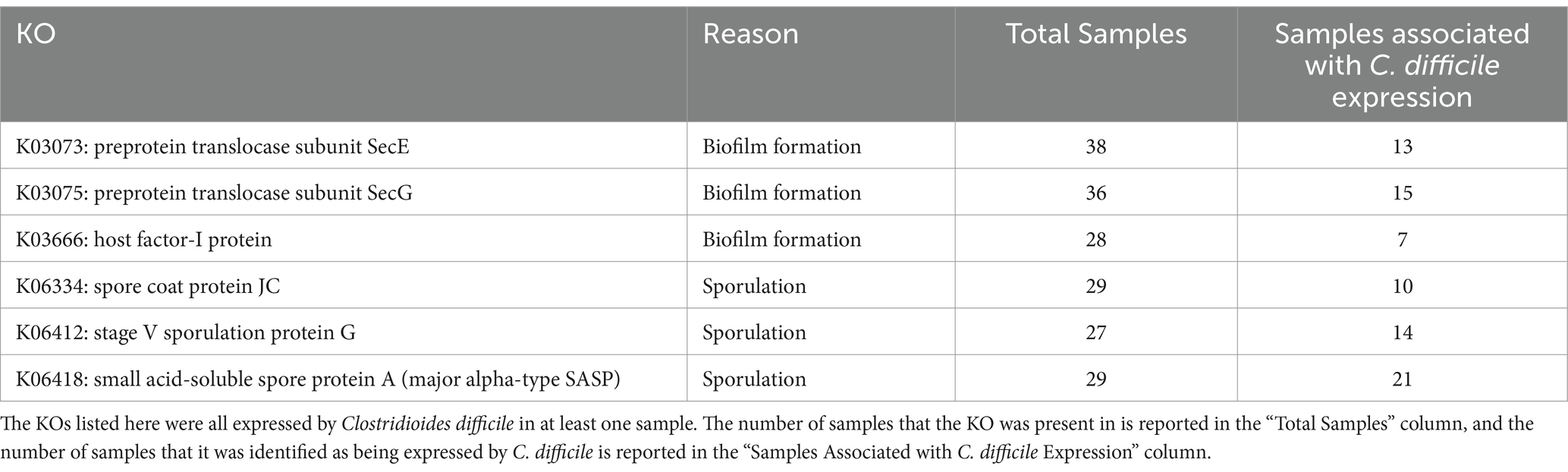
Table 2. Differential CDI+ KEGG Orthologs (KOs) of interest due to their potential to facilitate CDI recurrence.
Clostridioides difficile was one of the taxa found to be significantly more critical to the expression of differential rRNA genes in CDI+ (Supplementary Table S7). It was also found to be a significantly more important contributor to the expression of three differential KOs related to spore formation in CDI+ (Supplementary Table S8). Likewise, Enterocloster bolteae contributed to the differential expression of two of those KOs (Supplementary Table S8). Similarly, though it was not associated with differential expression of those three spore formation KOs, Ruminoccocus gnavus expressed genes relating to spore formation as well. Clostridioides difficile also constituted a greater proportion of the expression of genes relating to biofilm formation in CDI+, specifically K03073 (SecE), K03075 (SecG), and K03666 (hfq; Supplementary Tables S9, S10). Clostridioides difficile and R. gnavus were also significantly more important to the expression of genes within the differential Flagellar Assembly (ko02040) in CDI+ (Supplementary Table S11). Additionally, C. difficile was identified as expressing the tcdB gene in three CDI+ samples (13, 19, and 5), albeit very few sequences were associated with both (1, 2, and 5 sequences respectively).
Random forest modeling was used to help evaluate the consistency of features identified by LEfSe for differentiating samples based on CDI status, regardless of possible confounding factors. The performance of those models indicates that both microbial taxa and expressed genes identified by LEfSe differed consistently between CDI+ and CDI–. Both random forest models using differential features (genes or species) had average accuracies of 84.2% (Table 3). As expected, the differential species model excluding C. difficile performed worse, but it still had a 9% greater average accuracy than the random forest model that was generated using all species. Likewise, the genes model had a 7% increase in average accuracy between the dataset containing all genes and the dataset containing only genes identified as differentially expressed by LEfSe. Additionally, eight of the 10 best predictors for the full species dataset were also identified as being predictive of CDI status by LEfSe (Figure 6). The best predictors for the other models are shown in Supplementary Figures S5–S9.
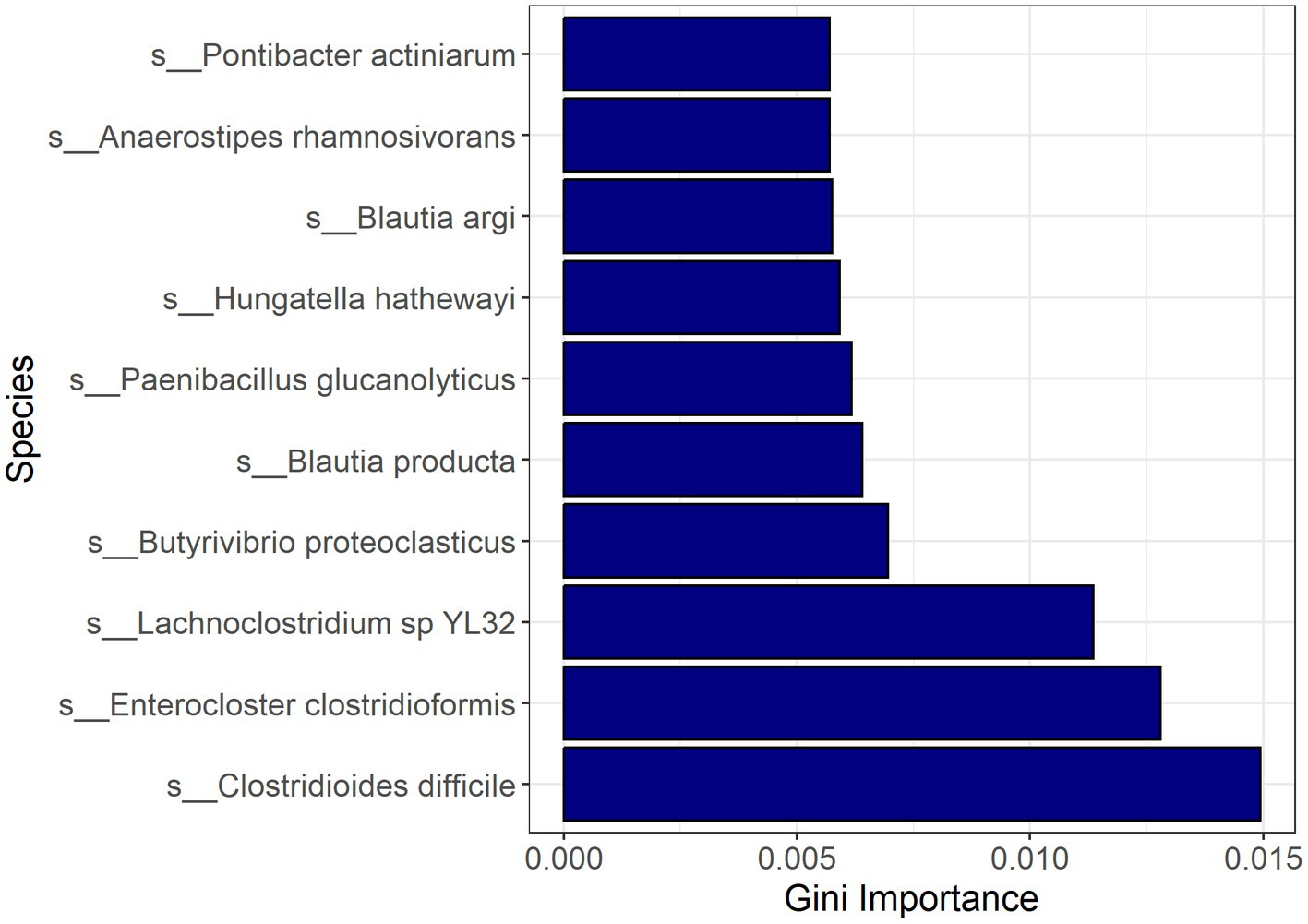
Figure 6. Bar plot of most important features for classification with random forest based on the full active microbial taxa dataset. Feature importance was measured by the decrease in impurity after splitting by the feature (Gini Importance). Pontibacter actiniarum and Butyrivibrio proteoclasticus were not identified as differential (LDA ≥ 2.0, Kruskal-Wallis p ≤ 0.05) by LEfSe.
3.4 Cross domain interactions
Co-occurrence network analysis helped us model potential transkingdom interactions between bacteria and fungi with respect to CDI status. The networks revealed negative relationships between fungi and bacterial taxa in both CDI− and CDI+ networks. In the CDI− network, 18 fungi were present, with Nakaseomyces glabratus (formerly Candida glabrata) having negative correlations with 57 taxa, 55 of which were bacteria (Figure 7). In contrast, N. glabratus was also entirely absent in the CDI+ network (Figure 7). Furthermore, the CDI+ network only had two fungi, Saccharomyces cerevisiae and Debaryomyces hansenii.
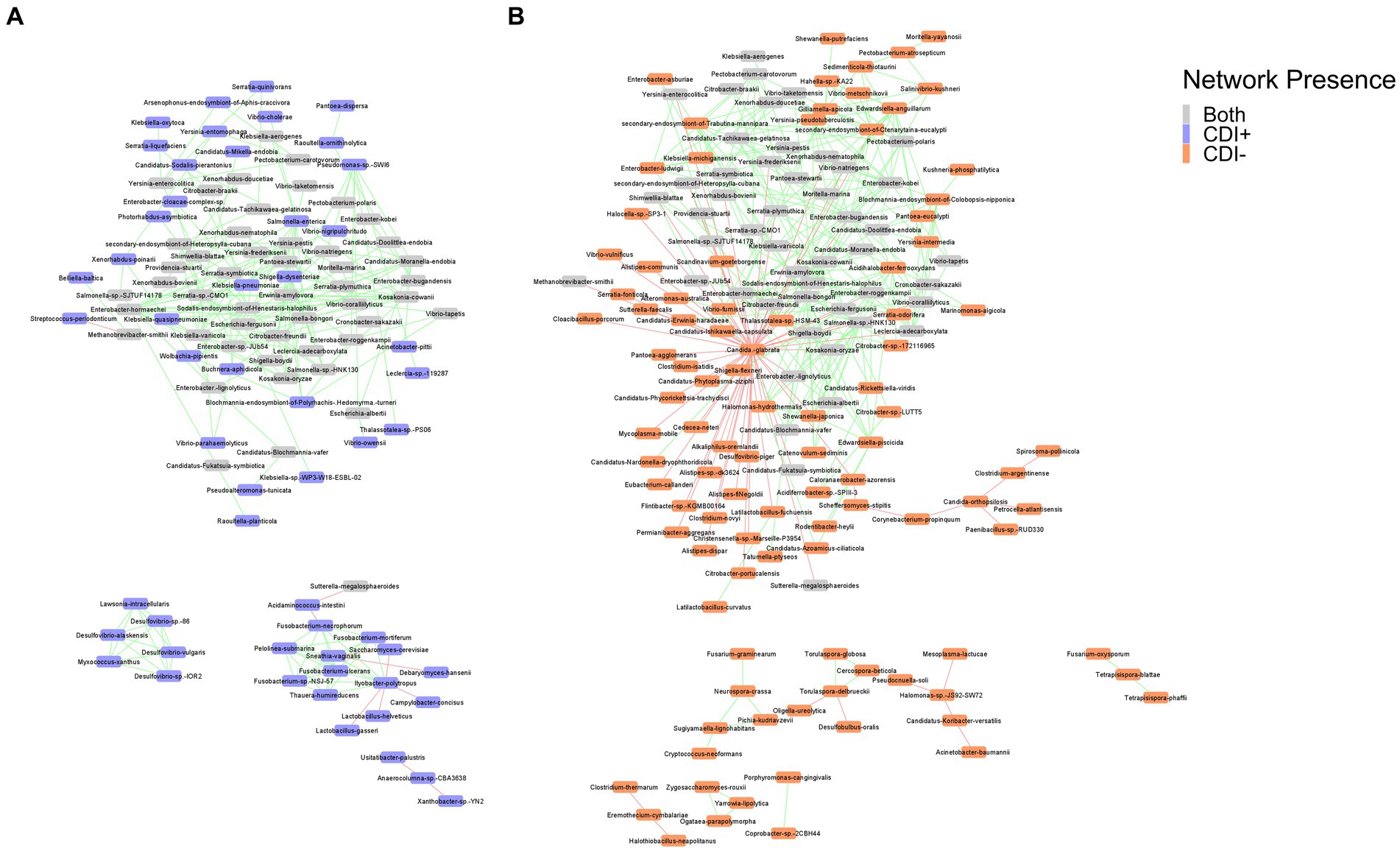
Figure 7. Co-occurrence networks for CDI+ (A) and CDI− (B). Nodes are labeled by species name and colored by network presence. Edges showing positive correlations between the connected nodes are shown in green, and edges showing negative correlations are shown in red. Pairs of nodes that were only connected to each other were omitted from the final networks.
3.5 Predictive metabolic modeling
Several predicted metabolites (n = 16) were found to be significantly more abundant (Kruskal-Wallis, p ≤ 0.05; Supplementary Table S12) in CDI+ patients, including caproic acid (Figure 8). In addition, a secondary bile acid, lithocholic acid, was also predicted to be more abundant in CDI+. Fewer predicted metabolites (n = 6) were associated with CDI–.
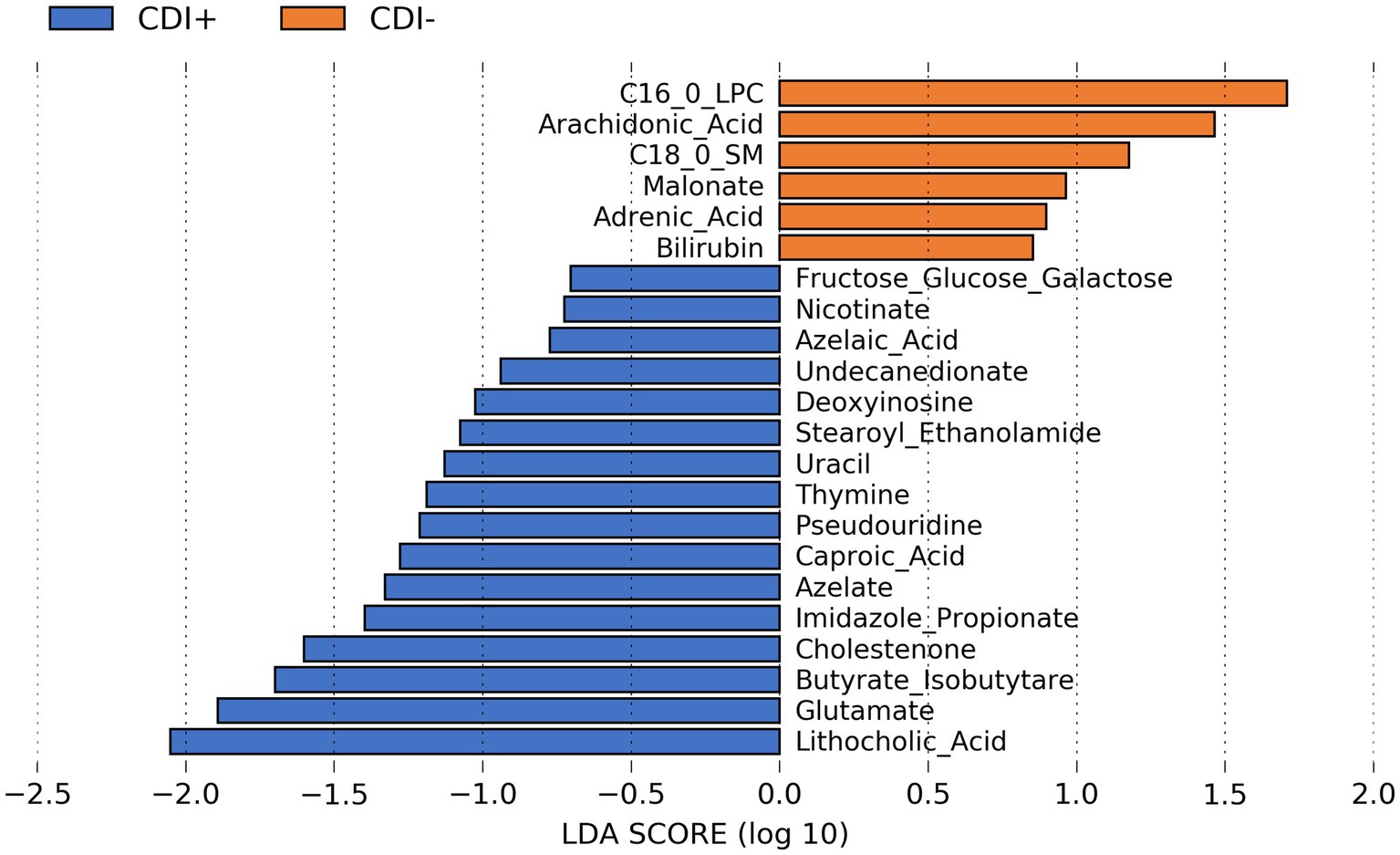
Figure 8. LEfSe bar plot showing differentially abundant predicted metabolites resulting from MelonnPan analysis (Kruskal-Wallis p ≤ 0.05) based on CDI status.
4 Discussion
4.1 Microbiome differences
Although previous studies have noted significant differences in alpha diversity, those studies utilized 16S rRNA and ITS data to characterize microbial communities (Antharam et al., 2013; Lamendella et al., 2018; Zuo et al., 2018). To the best of our knowledge, this study is the first to examine possible alpha diversity differences based on CDI status using MT data. However, these results are aligned with another study that compared CDI− diarrheal communities to CDI+ communities that also did not observe significant differences between those groups (Antharam et al., 2013).
Still, similar to other CDI microbiome studies (Lamendella et al., 2018; Zuo et al., 2018; Stewart et al., 2019) overall community composition and gene expression differed significantly based on CDI status. The recapitulation of this finding is notable because these results indicate that both the active community members and the functions they express differ with respect to CDI status.
The species most strongly associated with CDI− samples are beneficial to human health. Specifically, both Clostridium butyricum and Roseburia intestinalis are butyrate producers (Hayashi et al., 2021; Nie et al., 2021). Similarly, the use of Lacticaseibacillus rhamnosus as a probiotic has been associated with increased butyrate production (Berni Canani et al., 2016; Lin et al., 2020; Carucci et al., 2022), and Clostridium and Roseburia genera are contained in a newly FDA-approved fecal microbiota capsule (SER-109) for use against recurrent CDI (Khanna et al., 2016). In the context of CDI, butyrate has been found to reduce inflammation and improve intestinal barrier function following C. difficile colonization (Fachi et al., 2019). Roseburia intestinalis was most abundant in the healthy cohort in a study examining the gut microbiome of children based on CDI and hospitalization (IC vs. non-IC) status (Mohandas et al., 2020). Additionally, multiple studies have found C. butryicum promotes resistance to C. difficile infection (Hagihara et al., 2021; Hayashi et al., 2021), and it has been investigated as a possible treatment for CDI (Oka et al., 2018; Lee et al., 2022). Likewise, studies have found evidence administering Lactiplantibacillus plantarum as a probiotic may help prevent CDI (Klarin et al., 2008; Kujawa-Szewieczek et al., 2015; Dudzicz et al., 2018). Therefore, the higher activity of C. butryicum and L. plantarum in CDI− samples could have helped protect those patients against acquiring C. difficile.
Multiple butyrate producers were also identified as more active in CDI+. Although previous research has shown that butyrate was significantly reduced in CDI cohorts compared to healthy controls (Pensinger et al., 2023), another study did not find a significant difference in butyrate producers (based on 16S rRNA analysis) in their diarrheal controls compared to CDI, while their healthy cohort had significantly more butyrate producers compared to their CDI and diarrheal control groups (Antharam et al., 2013). Therefore, since both CDI+ and CDI− groups in this study were comprised of diarrheal samples, differential gene expression attributed to butyrate producers in both is consistent with previous findings.
Interestingly, Clostridium scindens was also identified as being more active in CDI+. Clostridium scindens had previously been associated with resistance to CDI as a result of producing secondary bile acids (Buffie et al., 2015; Greathouse et al., 2015), but resistance may be contingent on the resulting concentration of deoxycholate (Dubois et al., 2019). Still, C. scindens has also been found to inhibit C. difficile growth independent of bile acid production (Aguirre et al., 2021). Instead, the authors of that study suggested resistance could be due to competition for nutrients with C. difficile (Aguirre et al., 2021). Therefore, though unexpected, if nutrient conditions were conducive to C. difficile growth in our CDI+ patients, increased activity of C. scindens could have also been favored.
Besides Clostridioides difficile, the other species most strongly associated with CDI+ are also known pathogens. Enterocloster bolteae has been previously noted to be an opportunistic pathogen and are also correlated with the expression of inflammatory genes (Pandit et al., 2021). Similarly, Ruminococcus gnavus has been found to produce a glucorhamnan that causes inflammation (Henke et al., 2019). Additionally, Ruminoccocus gnavus was previously associated with CDI in another study that found several operational taxonomic units (OTUs) belonging to this species were more abundant in their CDI IBD group compared to the non-CDI IBD group and the healthy subjects (Sokol et al., 2017). Notably, Ruminococcus gnavus is a mucin degrader, and a previous study found C. difficile grown in the presence of MUC2 with mucin-degrading taxa also present had increased expression of genes associated with flagella (Engevik et al., 2021).
The larger number of fungal taxa associated with CDI+ aligns with previous studies that found fungal taxa were more abundant in CDI patients (Sangster et al., 2016; Lamendella et al., 2018; Stewart et al., 2019). Therefore, this study provides further evidence that the mycobiome may have an important impact on the pathogenesis of CDI. For instance, Encephalitozoon cuniculi, a species of fungi enriched in CDI+ patients, is a known opportunistic pathogen (Moretto et al., 2015). Conversely, the use of Saccharomyces boulardii, which is a part of the family Saccharomycetaceae (more active in CDI− patients), as a probiotic seems to reduce CDI-associated diarrhea (Goldenberg et al., 2017).
The relatively large number of differential KEGG Orthologs associated with ribosomal subunits was likely due to the taxa expressing them being more transcriptionally active in CDI+ samples because of other reasons, rather than a driving reason for increased activity themselves. Higher expression of genes coding for ribosomes has previously been correlated with an increase in activity for the microbes expressing them, though not consistently (Blazewicz et al., 2013). Still, the relatively greater contribution of CDI+ differential taxa to these genes provides another line of evidence that they were more active in CDI+.
Clostridioides difficile is known to form spores during infection (Paredes-Sabja et al., 2014), which fits with the observation of it as a significantly more important contributor to the expression of three differential KOs related to spore formation in CDI+ in this study. Likewise, Enterocloster bolteae can form spores (Magdy Wasfy et al., 2023) and contributed to the differential expression of two of those KOs (Supplementary Table S8). Similarly, though Ruminoccocus gnavus had historically been considered to be non-spore forming, more recent work has demonstrated that it can form spores under certain conditions (Crost et al., 2023). Therefore, it is possible that members of all three taxa could form spores in response to antibiotics, which may help explain their presence in CDI+ samples, as antibiotics are often used to treat CDI.
Both SecE and SecG have previously been proposed to be involved with C. difficile biofilm (Poquet et al., 2018). SecE and SecG have also been associated with biofilm formation in Staphylococcus aureus (Resch et al., 2005) and Bifidobacterium longum (Zhang et al., 2023) respectively. Therefore, although we did not recapture our previous finding of differential biofilm pathways (Stewart et al., 2019), the observed overall increased expression of hfq, SecE and SecG and their expression by C. difficile in this study, as well as other genes relating to biofilm formation, provides further in vivo evidence that biofilm formation is involved in the pathogenesis of CDI. However, hfq, SecE, and SecG are also components of other pathways, and of the 21 KOs expressed by C. difficile that are associated with at least one biofilm formation pathway, only two are associated solely with biofilm formation (Supplementary Table S10). Therefore, future work is needed to confirm in vivo biofilm formation. Still, biofilm formation, if occurring, could help protect C. difficile from antibiotics and consequently, contribute to CDI’s high reoccurrence rate.
Two of the four pathways more expressed in CDI+ [Ethylbenzene Degradation (ko00642) and Flagellar Assembly (ko02040)] were also identified as being differentially expressed in our prior study (Stewart et al., 2019). Notably, the production of flagella has been associated with pathogen colonization (Rossez et al., 2015), and C. difficile, E. bolteae, and Parabacteroides distasonis were significantly more important contributors to genes within that pathway in CDI+ (Supplementary Table S11). Like E. bolteae, P. distasonis is also an opportunistic pathogen (Ezeji et al., 2021). Therefore, the enrichment of the flagellar assembly pathway here was driven by the increased activity of potentially pathogenic bacteria.
Correlations between bacteria and fungi within CDI+ samples have been observed in other studies (Lamendella et al., 2018; Stewart et al., 2019). However, Lamendella et al., 2018 found no correlations between fungi and bacteria in CDI− (Lamendella et al., 2018), and Stewart et al., 2019 had only one unidentified fungi with a significant correlation to an unidentified Bacteroides OTU (Stewart et al., 2019). Networks in both were based on ITS data, so the use of MT data here instead is a likely contributor to the differences in the prevalence of fungi in the CDI− network. Still, despite evidence of fungi’s importance to CDI pathogenesis, co-occurrence analysis itself did not identify a significant correlation between fungal species and C. difficile in this study or in either of those previous studies (Lamendella et al., 2018; Stewart et al., 2019).
Caproic acid was previously detected in 41% of CDI+ fecal samples according to a gas chromatography study used to predict C. difficile (Levett, 1984). In contrast, lithocholic acid, also associated with CDI+ in this study, has previously been found to inhibit the growth of C. difficile (Kang et al., 2019). However, it is possible that lithocholic acid could have been more abundant in our CDI+ samples but still below a concentration that would have been lethal to C. difficile, and if so, then it is also possible that lithocholic acid could have induced biofilm formation, as was the case with deoxycholate in a previous study (Dubois et al., 2019). This possibility is supported by our observance of C. difficile expressing multiple genes related to biofilm formation.
Still, it should be noted that tools like MelonnPan are predictive metabolic modeling tools, and it is unknown whether the lithocholic acid metabolite was truly more abundant in our CDI+ samples. Consequently, directly measuring metabolites of interest like lithocholic acid and caproic acid, in conjunction with microbiome analysis, would be valuable to further understanding how the abundance of metabolites previously associated with CDI relates to microbiome composition and function.
4.2 Limitations
Unfortunately, no information about antibiotic usage or clinical history was reported for the individuals used in this study. Previous work has shown that antibiotic usage in conjunction with CDI has a large impact on the host’s overall microbial community (Lamendella et al., 2018). Specifically, in that study, the fidaxomicin and metronidazole groups had significant differences in alpha and beta diversity between CDI+ and CDI−, while the vancomycin group did not (Lamendella et al., 2018). Additionally, different taxa were detected as being significantly differential between CDI+/− depending on the antibiotic class (Lamendella et al., 2018). Still, another study did not find a significant difference in alpha diversity within their CDI+ and CDI− groups when split by antibiotics exposure (Antharam et al., 2013). However, it is possible that a differential usage of antibiotics could have impacted our results. Consequently, we limited much of our discussion to comparing the results obtained herein to previous CDI studies.
The failure to consistently capture sequences associated with both C. difficile and the gene used for diagnosis (tcdB) could also indicate our sequencing depth was not sufficient to fully capture all expressed genes in our samples. Additionally, due to the cost associated with MT, our sample size was smaller than some previous studies. Though, post hoc power analysis using SparseDossa2 showed we had reasonable (>80%) power to detect changes corresponding to at least 7.5 log2 fold change in the active species dataset and 4.5 log2 fold in the expressed genes dataset with LEfSe.
4.3 Conclusions and future directions
Despite antibiotic usage being a potentially large confounding variable, this work has contributed great value in characterizing active microbes and their associated functions in the context of CDI. To the best of our knowledge, MT analysis had only been applied to human samples associated with this disease state in a previous study by our group, in which it was used to identify differential genes and pathways (Stewart et al., 2019). Therefore, this study represents one of the first applications of MT to characterize differences in overall community diversity between CDI+ and CDI− cohorts. Although alpha diversity did not differ significantly, beta diversity for active species and expressed genes differentiated CDI+ and CDI− cohorts.
Subsequent differential feature analysis identified the specific genes and taxa driving those overall differences, revealing significant increases in the activity of several beneficial bacteria in CDI− and increased activity of taxa associated with inflammation in CDI+. Although the previous finding of differential biofilm formation pathways (Stewart et al., 2019) was not recaptured, several specific genes associated with biofilm formation were identified as differentially expressed in CDI+, and C. difficile was identified as a contributor to them, serving as additional evidence for the role of biofilm formation in CDI pathogenesis. Biofilm formation during infection would help explain CDI’s high recurrence rate, and if that is the case, treatments designed to target biofilms specifically could be effective for preventing reoccurrence.
Additionally, multiple fungal taxa were identified as being more transcriptionally active in CDI patients, which provides additional support for the potential importance of the mycobiome to CDI. Notably, E. cuniculi can cause inflammation, and increased inflammation due to it or other fungi could be one mechanism by which severe CDI could occur irrespective of bacterial toxin production. However, coverage for both E. cuniculi and fungi overall in this study tended to be low. Determining if specific fungal taxa are important for preventing or enabling CDI could facilitate the use of additional treatment methods. The low proportion of CDI+ samples that had tcdB identified within them suggests that greater sequencing coverage would also be valuable for characterizing the functional capabilities of these microbial communities. Therefore, future work should include deeper metatranscriptomics sequencing in order to increase coverage of the entire microbial community.
Increasing fungal sequences specifically, either through overall increased sequencing depth or targeting sequencing, would also aid better understanding if fungal activity contributes to the pathogenesis of CDI. Likewise, the development of methods to increase the proportion of fungal nucleic acids recovered would similarly help facilitate investigations of fungi’s role in CDI. Additionally, future iterations of the CDI status model could theoretically be trained to differentiate non-C. difficile diarrhea with colonization from clinically-relevant C. difficile infection, as a means to reduce unnecessary anti-C. difficile antibiotic use (Polage et al., 2015). Metabolomics data would also be invaluable for further exploring the role that previously identified metabolites of interest play in CDI.
Data availability statement
Raw sequencing data are available from NCBI’s Short Read Archive under BioProject ID PRJNA1035947.
Ethics statement
The studies involving humans were approved by Institutional Review Board at the University of Virginia. The studies were conducted in accordance with the local legislation and institutional requirements. The ethics committee/institutional review board waived the requirement of written informed consent for participation from the participants or the participants’ legal guardians/next of kin because samples were de-identified remnants.
Author contributions
JC: Formal analysis, Visualization, Writing – original draft, Writing – review & editing. JL: Investigation, Writing – review & editing. JW: Resources, Supervision, Writing – review & editing. PK: Formal analysis, Writing – review & editing. MK: Formal analysis, Writing – original draft, Writing – review & editing. CB: Formal analysis, Writing – original draft, Writing – review & editing. CK: Conceptualization, Writing – review & editing. GM: Conceptualization, Writing – review & editing. DS: Conceptualization, Writing – original draft, Writing – review & editing. RL: Conceptualization, Supervision, Writing – original draft, Writing – review & editing.
Funding
The author(s) declare that financial support was received for the research, authorship, and/or publication of this article. This project was funded by Juniata College’s George and Cynthia Valko Endowment.
Acknowledgments
The authors would like to thank Mary Young and William Petri for their assistance with sample collection. We would also like to thank Christine Walls and Vincent Buonaccorsi for their technical support at Juniata.
Conflict of interest
JC, JL, JW and RL was employed by Wright Labs LLC.
The remaining authors declare that the research was conducted in the absence of any commercial or financial relationships that could be construed as a potential conflict of interest.
Publisher’s note
All claims expressed in this article are solely those of the authors and do not necessarily represent those of their affiliated organizations, or those of the publisher, the editors and the reviewers. Any product that may be evaluated in this article, or claim that may be made by its manufacturer, is not guaranteed or endorsed by the publisher.
Supplementary material
The Supplementary material for this article can be found online at: https://www.frontiersin.org/articles/10.3389/fmicb.2024.1398018/full#supplementary-material
References
Aguirre, A. M., Yalcinkaya, N., Wu, Q., Swennes, A., Tessier, M. E., Roberts, P., et al. (2021). Bile acid-independent protection against Clostridioides difficile infection. PLoS Pathog. 17:e1010015. doi: 10.1371/journal.ppat.1010015
Allen-Vercoe, E., Daigneault, M., White, A., Panaccione, R., Duncan, S. H., Flint, H. J., et al. (2012). Anaerostipes hadrus comb. nov., a dominant species within the human colonic microbiota; reclassification of Eubacterium hadrum Moore et al. 1976. Anaerobe 18, 523–529. doi: 10.1016/j.anaerobe.2012.09.002
Antharam, V. C., Li, E. C., Ishmael, A., Sharma, A., Mai, V., Rand, K. H., et al. (2013). Intestinal dysbiosis and depletion of butyrogenic bacteria in Clostridium difficile infection and nosocomial diarrhea. J. Clin. Microbiol. 51, 2884–2892. doi: 10.1128/JCM.00845-13
Aslam, S., Hamill, R. J., and Musher, D. M. (2005). Treatment of Clostridium difficile-associated disease: old therapies and new strategies. Lancet Infect. Dis. 5, 549–557. doi: 10.1016/S1473-3099(05)70215-2
Beghini, F., McIver, L. J., Blanco-Míguez, A., Dubois, L., Asnicar, F., Maharjan, S., et al. (2021). Integrating taxonomic, functional, and strain-level profiling of diverse microbial communities with bioBakery 3. eLife 10:e65088. doi: 10.7554/eLife.65088
Benjamini, Y., and Hochberg, Y. (1995). Controlling the false discovery rate: a practical and powerful approach to multiple testing. J R Stat Soc Series B 57, 289–300. doi: 10.1111/j.2517-6161.1995.tb02031.x
Berni Canani, R., Sangwan, N., Stefka, A. T., Nocerino, R., Paparo, L., Aitoro, R., et al. (2016). Lactobacillus rhamnosus GG-supplemented formula expands butyrate-producing bacterial strains in food allergic infants. ISME J. 10, 742–750. doi: 10.1038/ismej.2015.151
Blazewicz, S. J., Barnard, R. L., Daly, R. A., and Firestone, M. K. (2013). Evaluating rRNA as an indicator of microbial activity in environmental communities: limitations and uses. ISME J. 7, 2061–2068. doi: 10.1038/ismej.2013.102
Bobo, L. D., Dubberke, E. R., and Kollef, M. (2011). Clostridium difficile in the ICU. Chest 140, 1643–1653. doi: 10.1378/chest.11-0556
Bolyen, E., Rideout, J. R., Dillon, M. R., Bokulich, N. A., Abnet, C. C., Al-Ghalith, G. A., et al. (2019). Reproducible, interactive, scalable and extensible microbiome data science using QIIME 2. Nat. Biotechnol. 37, 852–857. doi: 10.1038/s41587-019-0209-9
Brown, M. B. (1975). 400: a method for combining non-independent, one-sided tests of significance. Biometrics 31, 987–992. doi: 10.2307/2529826
Buffie, C. G., Bucci, V., Stein, R. R., McKenney, P. T., Ling, L., Gobourne, A., et al. (2015). Precision microbiome reconstitution restores bile acid mediated resistance to Clostridium difficile. Nature 517, 205–208. doi: 10.1038/nature13828
Carucci, L., Nocerino, R., Paparo, L., De Filippis, F., Coppola, S., Giglio, V., et al. (2022). Therapeutic effects elicited by the probiotic Lacticaseibacillus rhamnosus GG in children with atopic dermatitis. The results of the ProPAD trial. Pediatr. Allergy Immunol. 33:e13836. doi: 10.1111/pai.13836
Chen, S., Zhou, Y., Chen, Y., and Gu, J. (2018). Fastp: an ultra-fast all-in-one FASTQ preprocessor. Bioinformatics 34, i884–i890. doi: 10.1093/bioinformatics/bty560
Choi, H. K., Kim, K. H., Lee, S. H., and Lee, S. J. (2011). Risk factors for recurrence of Clostridium difficile infection: effect of vancomycin-resistant enterococci colonization. J. Korean Med. Sci. 26, 859–864. doi: 10.3346/jkms.2011.26.7.859
Crost, E. H., Coletto, E., Bell, A., and Juge, N. (2023). Ruminococcus gnavus: friend or foe for human health. FEMS Microbiol. Rev. 47:fuad014. doi: 10.1093/femsre/fuad014
Douglas, G. M., Maffei, V. J., Zaneveld, J. R., Yurgel, S. N., Brown, J. R., Taylor, C. M., et al. (2020). PICRUSt2 for prediction of metagenome functions. Nat. Biotechnol. 38, 685–688. doi: 10.1038/s41587-020-0548-6
Dubberke, E. R., Butler, A. M., Reske, K. A., Agniel, D., Olsen, M. A., D’Angelo, G., et al. (2008). Attributable outcomes of endemic Clostridium difficile–associated disease in nonsurgical patients. Emerg. Infect. Dis. 14, 1031–1038. doi: 10.3201/eid1407.070867
Dubberke, E. R., and Olsen, M. A. (2012). Burden of Clostridium difficile on the healthcare system. Clin. Infect. Dis. 55, S88–S92. doi: 10.1093/cid/cis335
Dubois, T., Tremblay, Y. D. N., Hamiot, A., Martin-Verstraete, I., Deschamps, J., Monot, M., et al. (2019). A microbiota-generated bile salt induces biofilm formation in Clostridium difficile. NPJ Biofilms Microbiomes 5:14. doi: 10.1038/s41522-019-0087-4
Dudzicz, S., Kujawa-Szewieczek, A., Kwiecień, K., Więcek, A., and Adamczak, M. (2018). Lactobacillus plantarum 299v reduces the incidence of Clostridium difficile infection in nephrology and transplantation Ward—results of one year extended study. Nutrients 10:1574. doi: 10.3390/nu10111574
Engevik, M. A., Engevik, A. C., Engevik, K. A., Auchtung, J. M., Chang-Graham, A. L., Ruan, W., et al. (2021). Mucin-degrading microbes release monosaccharides that chemoattract Clostridioides difficile and facilitate colonization of the human intestinal mucus layer. ACS Infect Dis 7, 1126–1142. doi: 10.1021/acsinfecdis.0c00634
Ezeji, J. C., Sarikonda, D. K., Hopperton, A., Erkkila, H. L., Cohen, D. E., Martinez, S. P., et al. (2021). Parabacteroides distasonis: intriguing aerotolerant gut anaerobe with emerging antimicrobial resistance and pathogenic and probiotic roles in human health. Gut Microbes 13:1922241. doi: 10.1080/19490976.2021.1922241
Fachi, J. L., Felipe, J. S., Pral, L. P., da Silva, B. K., Corrêa, R. O., de Andrade, M. C. P., et al. (2019). Butyrate protects mice from Clostridium difficile-induced colitis through an HIF-1-dependent mechanism. Cell Rep. 27, 750–761.e7. doi: 10.1016/j.celrep.2019.03.054
Faust, K., and Raes, J. (2016). CoNet app: inference of biological association networks using Cytoscape. F1000Res 5:1519. doi: 10.12688/f1000research.9050.2
Franzosa, E. A., Sirota-Madi, A., Avila-Pacheco, J., Fornelos, N., Haiser, H. J., Reinker, S., et al. (2019). Gut microbiome structure and metabolic activity in inflammatory bowel disease. Nat. Microbiol. 4, 293–305. doi: 10.1038/s41564-018-0306-4
Goldenberg, J. Z., Yap, C., Lytvyn, L., Lo, C. K.-F., Beardsley, J., Mertz, D., et al. (2017). Probiotics for the prevention of Clostridium difficile-associated diarrhea in adults and children. Cochrane Database Syst. Rev. 2017:CD006095. doi: 10.1002/14651858.CD006095.pub4
Greathouse, K. L., Harris, C. C., and Bultman, S. J. (2015). Dysfunctional families: Clostridium scindens and secondary bile acids inhibit the growth of Clostridium difficile. Cell Metab. 21, 9–10. doi: 10.1016/j.cmet.2014.12.016
Hagihara, M., Ariyoshi, T., Kuroki, Y., Eguchi, S., Higashi, S., Mori, T., et al. (2021). Clostridium butyricum enhances colonization resistance against Clostridioides difficile by metabolic and immune modulation. Sci. Rep. 11:15007. doi: 10.1038/s41598-021-94572-z
Hayashi, A., Nagao-Kitamoto, H., Kitamoto, S., Kim, C. H., and Kamada, N. (2021). The butyrate-producing bacterium Clostridium butyricum suppresses Clostridioides difficile infection via neutrophil- and antimicrobial cytokine–dependent but GPR43/109a-independent mechanisms. J. Immunol. 206, 1576–1585. doi: 10.4049/jimmunol.2000353
Henke, M. T., Kenny, D. J., Cassilly, C. D., Vlamakis, H., Xavier, R. J., and Clardy, J. (2019). Ruminococcus gnavus, a member of the human gut microbiome associated with Crohn’s disease, produces an inflammatory polysaccharide. Proc. Natl. Acad. Sci. 116, 12672–12677. doi: 10.1073/pnas.1904099116
Huerta-Cepas, J., Forslund, K., Coelho, L. P., Szklarczyk, D., Jensen, L. J., von Mering, C., et al. (2017). Fast genome-wide functional annotation through Orthology assignment by eggNOG-mapper. Mol. Biol. Evol. 34, 2115–2122. doi: 10.1093/molbev/msx148
Huerta-Cepas, J., Szklarczyk, D., Heller, D., Hernández-Plaza, A., Forslund, S. K., Cook, H., et al. (2019). eggNOG 5.0: a hierarchical, functionally and phylogenetically annotated orthology resource based on 5090 organisms and 2502 viruses. Nucleic Acids Res. 47, D309–D314. doi: 10.1093/nar/gky1085
Kang, J. D., Myers, C. J., Harris, S. C., Kakiyama, G., Lee, I.-K., Yun, B.-S., et al. (2019). Bile acid 7α-dehydroxylating gut bacteria secrete antibiotics that inhibit Clostridium difficile: role of secondary bile acids. Cell Chem Biol 26, 27–34.e4. doi: 10.1016/j.chembiol.2018.10.003
Kassambara, A. (2020). rstatix: Pipe-friendly Framework for Basic Statistical Tests in R. Available at: https://github.com/kassambara/rstatix (Accessed 21 January 2021).
Khanna, S., Pardi, D. S., Kelly, C. R., Kraft, C. S., Dhere, T., Henn, M. R., et al. (2016). A novel microbiome therapeutic increases gut microbial diversity and prevents recurrent Clostridium difficile infection. J Infect Dis 214, 173–181. doi: 10.1093/infdis/jiv766
Klarin, B., Wullt, M., Palmquist, I., Molin, G., Larsson, A., and Jeppsson, B. (2008). Lactobacillus plantarum 299v reduces colonisation of Clostridium difficile in critically ill patients treated with antibiotics. Acta Anaesthesiol. Scand. 52, 1096–1102. doi: 10.1111/j.1399-6576.2008.01748.x
Kujawa-Szewieczek, A., Adamczak, M., Kwiecień, K., Dudzicz, S., Gazda, M., and Więcek, A. (2015). The effect of Lactobacillus plantarum 299v on the incidence of Clostridium difficile infection in high risk patients treated with antibiotics. Nutrients 7, 10179–10188. doi: 10.3390/nu7125526
Kullback, S., and Leibler, R. A. (1951). On information and sufficiency. Ann. Math. Stat. 22, 79–86. doi: 10.1214/aoms/1177729694
Lamendella, R., Wright, J. R., Hackman, J., McLimans, C., Toole, D. R., Rubio, W. B., et al. (2018). Antibiotic treatments for Clostridium difficile infection are associated with distinct bacterial and fungal community structures. mSphere 3:17. doi: 10.1128/mSphere.00572-17
Lee, J.-C., Chiu, C.-W., Tsai, P.-J., Lee, C.-C., Huang, I.-H., Ko, W.-C., et al. (2022). Clostridium butyricum therapy for mild-moderate Clostridioides difficile infection and the impact of diabetes mellitus. Biosci Microbiota Food Health 41, 37–44. doi: 10.12938/bmfh.2021-049
Lessa, F. C., Mu, Y., Bamberg, W. M., Beldavs, Z. G., Dumyati, G. K., Dunn, J. R., et al. (2015). Burden of Clostridium difficile infection in the United States. N. Engl. J. Med. 372, 825–834. doi: 10.1056/NEJMoa1408913
Levett, P. N. (1984). Detection of Clostridium difficile in faeces by direct gas liquid chromatography. J. Clin. Pathol. 37, 117–119. doi: 10.1136/jcp.37.2.117
Lin, R., Sun, Y., Mu, P., Zheng, T., Mu, H., Deng, F., et al. (2020). Lactobacillus rhamnosus GG supplementation modulates the gut microbiota to promote butyrate production, protecting against deoxynivalenol exposure in nude mice. Biochem. Pharmacol. 175:113868. doi: 10.1016/j.bcp.2020.113868
Louis, P., and Flint, H. J. (2009). Diversity, metabolism and microbial ecology of butyrate-producing bacteria from the human large intestine. FEMS Microbiol. Lett. 294, 1–8. doi: 10.1111/j.1574-6968.2009.01514.x
Ma, S., Ren, B., Mallick, H., Moon, Y. S., Schwager, E., Maharjan, S., et al. (2021). A statistical model for describing and simulating microbial community profiles. PLoS Comput. Biol. 17:e1008913. doi: 10.1371/journal.pcbi.1008913
Magdy Wasfy, R., Mbaye, B., Borentain, P., Tidjani Alou, M., Murillo Ruiz, M. L., Caputo, A., et al. (2023). Ethanol-producing Enterocloster bolteae is enriched in chronic hepatitis B-associated gut dysbiosis: a case–control Culturomics study. Microorganisms 11:2437. doi: 10.3390/microorganisms11102437
Malek, M., Ibragimov, R., Albrecht, M., and Baumbach, J. (2016). CytoGEDEVO—global alignment of biological networks with Cytoscape. Bioinformatics 32, 1259–1261. doi: 10.1093/bioinformatics/btv732
Mallick, H., Franzosa, E. A., Mclver, L. J., Banerjee, S., Sirota-Madi, A., Kostic, A. D., et al. (2019). Predictive metabolomic profiling of microbial communities using amplicon or metagenomic sequences. Nat. Commun. 10:3136. doi: 10.1038/s41467-019-10927-1
Markey, L., Shaban, L., Green, E. R., Lemon, K. P., Mecsas, J., and Kumamoto, C. A. (2018). Pre-colonization with the commensal fungus Candida albicans reduces murine susceptibility to Clostridium difficile infection. Gut Microbes 9, 497–509. doi: 10.1080/19490976.2018.1465158
Mohandas, S., Soma, V. L., Tran, T. D. B., Sodergren, E., Ambooken, T., Goldman, D. L., et al. (2020). Differences in gut microbiome in hospitalized immunocompetent vs. immunocompromised children, including those with sickle cell disease. Front. Pediatr. 8:446. doi: 10.3389/fped.2020.583446
Moretto, M. M., Harrow, D. I., Hawley, T. S., and Khan, I. A. (2015). Interleukin-12-producing CD103+ CD11b− CD8+ dendritic cells are responsible for eliciting gut intraepithelial lymphocyte response against encephalitozoon cuniculi. Infect. Immun. 83, 4719–4730. doi: 10.1128/iai.00820-15
Nie, K., Ma, K., Luo, W., Shen, Z., Yang, Z., Xiao, M., et al. (2021). Roseburia intestinalis: a beneficial gut organism from the discoveries in genus and species. Front. Cell. Infect. Microbiol. 11:757718. doi: 10.3389/fcimb.2021.757718
Oka, K., Osaki, T., Hanawa, T., Kurata, S., Sugiyama, E., Takahashi, M., et al. (2018). Establishment of an endogenous Clostridium difficile rat infection model and evaluation of the effects of Clostridium butyricum MIYAIRI 588 probiotic strain. Front. Microbiol. 9:1264. doi: 10.3389/fmicb.2018.01264
Pandit, L., Cox, L. M., Malli, C., D’Cunha, A., Rooney, T., Lokhande, H., et al. (2021). Clostridium bolteae is elevated in neuromyelitis optica spectrum disorder in India and shares sequence similarity with AQP4. Neurol Neuroimmunol Neuroinflamm 8:907. doi: 10.1212/NXI.0000000000000907
Paredes-Sabja, D., Shen, A., and Sorg, J. A. (2014). Clostridium difficile spore biology: sporulation, germination, and spore structural proteins. Trends Microbiol. 22, 406–416. doi: 10.1016/j.tim.2014.04.003
Pedregosa, F., Varoquaux, G., Gramfort, A., Michel, V., Thirion, B., Grisel, O., et al. (2011). Scikit-learn: machine learning in python. J. Mach. Learn. Res. 12, 2825–2830.
Pensinger, D. A., Fisher, A. T., Dobrila, H. A., Van Treuren, W., Gardner, J. O., Higginbottom, S. K., et al. (2023). Butyrate differentiates permissiveness to Clostridioides difficile infection and influences growth of Diverse C. difficile isolates. Infect. Immun. 91:e0057022. doi: 10.1128/iai.00570-22
Pielou, E. C. (1966). The measurement of diversity in different types of biological collections. J. Theor. Biol. 13, 131–144. doi: 10.1016/0022-5193(66)90013-0
Polage, C. R., Gyorke, C. E., Kennedy, M. A., Leslie, J. L., Chin, D. L., Wang, S., et al. (2015). Overdiagnosis of Clostridium difficile infection in the molecular test era. JAMA Intern. Med. 175, 1792–1801. doi: 10.1001/jamainternmed.2015.4114
Poquet, I., Saujet, L., Canette, A., Monot, M., Mihajlovic, J., Ghigo, J.-M., et al. (2018). Clostridium difficile biofilm: Remodeling metabolism and cell surface to build a sparse and heterogeneously aggregated architecture. Front. Microbiol. 9:2084. doi: 10.3389/fmicb.2018.02084
Quensen, J. (2020). ggordiplots. Available at: https://github.com/jfq3/ggordiplots (Accessed 31 May 2021).
R Core Team (2023). R: A language and environment for statistical computing. Available at: https://www.R-project.org/
Resch, A., Rosenstein, R., Nerz, C., and Götz, F. (2005). Differential gene expression profiling of Staphylococcus aureus cultivated under biofilm and planktonic conditions. Appl. Environ. Microbiol. 71, 2663–2676. doi: 10.1128/AEM.71.5.2663-2676.2005
Rognes, T., Flouri, T., Nichols, B., Quince, C., and Mahé, F. (2016). VSEARCH: a versatile open source tool for metagenomics. PeerJ 4:e2584. doi: 10.7717/peerj.2584
Rossez, Y., Wolfson, E. B., Holmes, A., Gally, D. L., and Holden, N. J. (2015). Bacterial flagella: twist and stick, or dodge across the kingdoms. PLoS Pathog. 11:e1004483. doi: 10.1371/journal.ppat.1004483
Sangster, W., Hegarty, J. P., Schieffer, K. M., Wright, J. R., Hackman, J., Toole, D. R., et al. (2016). Bacterial and fungal microbiota changes Distinguish C. difficile infection from other forms of Diarrhea: results of a prospective inpatient study. Front. Microbiol. 7:789. doi: 10.3389/fmicb.2016.00789
Segata, N., Izard, J., Waldron, L., Gevers, D., Miropolsky, L., Garrett, W. S., et al. (2011). Metagenomic biomarker discovery and explanation. Genome Biol. 12:R60. doi: 10.1186/gb-2011-12-6-r60
Shannon, P., Markiel, A., Ozier, O., Baliga, N. S., Wang, J. T., Ramage, D., et al. (2003). Cytoscape: a software environment for integrated models of biomolecular interaction networks. Genome Res. 13, 2498–2504. doi: 10.1101/gr.1239303
Shivashankar, R., Khanna, S., Kammer, P. P., Scott Harmsen, W., Zinsmeister, A. R., Baddour, L. M., et al. (2014). Clinical predictors of recurrent Clostridium difficile infection in out-patients. Aliment. Pharmacol. Ther. 40, 518–522. doi: 10.1111/apt.12864
Sokol, H., Jegou, S., McQuitty, C., Straub, M., Leducq, V., Landman, C., et al. (2017). Specificities of the intestinal microbiota in patients with inflammatory bowel disease and Clostridium difficile infection. Gut Microbes 9, 55–60. doi: 10.1080/19490976.2017.1361092
Sørensen, T. J. (1948). A method of establishing groups of equal amplitude in plant sociology based on similarity of species content and its application to analyses of the vegetation on Danish commons. København: I kommission hos E. Munksgaard.
Spearman Rank Correlation Coefficient (2008). The concise Encyclopedia of statistics, (New York, NY: Springer), 502–505.
Stewart, D. B., Wright, J. R., Fowler, M., McLimans, C. J., Tokarev, V., Amaniera, I., et al. (2019). Integrated meta-omics reveals a fungus-associated bacteriome and distinct functional pathways in Clostridioides difficile infection. mSphere 4:19. doi: 10.1128/mSphere.00454-19
Wemheuer, F., Taylor, J. A., Daniel, R., Johnston, E., Meinicke, P., Thomas, T., et al. (2020). Tax4Fun2: prediction of habitat-specific functional profiles and functional redundancy based on 16S rRNA gene sequences. Environ Microbiome 15:11. doi: 10.1186/s40793-020-00358-7
Wood, D. E., Lu, J., and Langmead, B. (2019). Improved metagenomic analysis with Kraken 2. bioRxiv [Preprint], bioRxiv:762302.
Zhang, J., Kobert, K., Flouri, T., and Stamatakis, A. (2014). PEAR: a fast and accurate Illumina paired-end reAd mergeR. Bioinformatics 30, 614–620. doi: 10.1093/bioinformatics/btt593
Zhang, T., Liu, Z., Zhao, J., Zhang, H., Chen, W., Li, H., et al. (2023). Multi-omics analysis of the biofilm forming mechanism of Bifidobacterium longum. LWT 188:115415. doi: 10.1016/j.lwt.2023.115415
Keywords: Clostridioides difficile , metatranscriptomics, fungi, human microbiome, mycobiome
Citation: Chen See JR, Leister J, Wright JR, Kruse PI, Khedekar MV, Besch CE, Kumamoto CA, Madden GR, Stewart DB and Lamendella R (2024) Clostridioides difficile infection is associated with differences in transcriptionally active microbial communities. Front. Microbiol. 15:1398018. doi: 10.3389/fmicb.2024.1398018
Edited by:
Meina Neumann-Schaal, German Collection of Microorganisms and Cell Cultures GmbH (DSMZ), GermanyReviewed by:
Maria Dzunkova, University of Valencia, SpainAlexandro Rodríguez-Rojas, University of Veterinary Medicine Vienna, Austria
Mindy Engevik, Medical University of South Carolina, United States
Copyright © 2024 Chen See, Leister, Wright, Kruse, Khedekar, Besch, Kumamoto, Madden, Stewart and Lamendella. This is an open-access article distributed under the terms of the Creative Commons Attribution License (CC BY). The use, distribution or reproduction in other forums is permitted, provided the original author(s) and the copyright owner(s) are credited and that the original publication in this journal is cited, in accordance with accepted academic practice. No use, distribution or reproduction is permitted which does not comply with these terms.
*Correspondence: Regina Lamendella, bGFtZW5kZWxsYUBqdW5pYXRhLmVkdQ==