- 1Department of Biochemistry and Immunology, Institute of Biological Sciences, Federal University of Minas Gerais, Belo Horizonte, Minas Gerais, Brazil
- 2Laboratory of Simulation and Computational Biology — SIMBIC, High Performance Computing Center — CCAD, Federal University of Pará, Belém, Pará, Brazil
- 3Laboratory of Bioinformatics and Genomics of Microorganisms, Institute of Biological Sciences, Federal University of Pará, Belém, Pará, Brazil
Freshwater availability is essential, and its maintenance has become an enormous challenge. Due to population growth and climate changes, freshwater sources are becoming scarce, imposing the need for strategies for its reuse. Currently, the constant discharge of waste into water bodies from human activities leads to the dissemination of pathogenic bacteria, negatively impacting water quality from the source to the infrastructure required for treatment, such as the accumulation of biofilms. Current water treatment methods cannot keep pace with bacterial evolution, which increasingly exhibits a profile of multidrug resistance to antibiotics. Furthermore, using more powerful disinfectants may affect the balance of aquatic ecosystems. Therefore, there is a need to explore sustainable ways to control the spreading of pathogenic bacteria. Bacteriophages can infect bacteria and archaea, hijacking their host machinery to favor their replication. They are widely abundant globally and provide a biological alternative to bacterial treatment with antibiotics. In contrast to common disinfectants and antibiotics, bacteriophages are highly specific, minimizing adverse effects on aquatic microbial communities and offering a lower cost–benefit ratio in production compared to antibiotics. However, due to the difficulty involving cultivating and identifying environmental bacteriophages, alternative approaches using NGS metagenomics in combination with some bioinformatic tools can help identify new bacteriophages that can be useful as an alternative treatment against resistant bacteria. In this review, we discuss advances in exploring the virome of freshwater, as well as current applications of bacteriophages in freshwater treatment, along with current challenges and future perspectives.
1 Introduction
Freshwater is an indispensable resource for maintaining life on Earth and has been consistently impacted by the increasing anthropogenic influence. Urban and rural expansion around water bodies, coupled with waste disposal from hospitals, water treatment systems, industry, agriculture, and residences, contribute to rivers and lakes becoming hotspots for the proliferation of pathogenic microorganisms (Reddy et al., 2022). Water disinfection methods have become limited due to the growing demand for water reuse and the inefficiency of a significant portion of antibiotics against the spread of antibiotic-resistant bacteria (Mathieu et al., 2019). Therefore, there is a pressing need to explore natural compounds to control multidrug-resistant bacteria, such as bacteriophages.
Bacteriophages (or phages) are the most abundant biological entities globally. They were first described in the early 1900s, and by now, we know they are widespread in the environment, with estimates of ~1031 phages present in the biosphere (Twort, 1915; Rohwer and Edwards, 2002). Phages act as natural predators of bacteria and archaea, and exploit host machinery favoring their own replication (Dion et al., 2020). Phages may interact with bacterial or archaeal hosts by transferring genes that might be ecologically relevant, thus favoring the host genetic fitness through horizontal gene transfer (HGT) (Touchon et al., 2017; De Mandal et al., 2021). When associated with their hosts as prophages, phages may introduce auxiliary metabolism genes that potentially enhance host adaptability (Luo et al., 2022). The initial discovery that phages were highly abundant in aquatic samples (Bergh et al., 1989) laid the groundwork for the eventual determination of their pivotal impact on the ecosystem.
The paramount significance of phages arises from the viral shunt phenomenon, wherein organic matter is recycled through the lysis of host cells, driving global-scale biogeochemical cycles (Breitbart et al., 2018). Bacteriophages represent an ecological alternative to the use of antibiotics, with a lower cost–benefit ratio of production, and exhibit high specificity to their hosts, minimizing dysbiosis (Romero-Calle et al., 2019). They have been employed for at least a century in controlling bacterial infections in humans (Rohde et al., 2018), and have recently been advocated for applications in freshwater environments (Naknaen et al., 2021; Ben Saad et al., 2022; Hu et al., 2023).
Phages can be classified into three groups: (1) virulent bacteriophages that solely undergo the lytic cycle, leading to the lysis of the host cell; (2) temperate phages that can suffer lysogenic cycles, remaining dormant within the host cell (prophages) but can be induced to switch to the lytic or chronic cycle; and (3) filamentous phages: go through a chronic cycle in which viral replication occurs without host cell lysis (Chevallereau et al., 2022; Zhang et al., 2022). Lytic phages are the most desirable due to their cell lysis capability and lower risk of horizontal gene transfer.
Classical studies of phages relied on isolation and culture methods for their identification (Hyman, 2019). Currently, with the advancement of culture-independent methodologies such as metagenomics, databases are increasingly enriched with viral data, enabling a more comprehensive understanding at the taxonomic level and potential interactions of phages with their hosts (Santiago-Rodriguez and Hollister, 2023) showing that bioinformatics tools for mining viral data can be a powerful aid in discovering bacteriophages.
This review discusses the identification of phages in freshwater environments, the primary in silico tools used for phage data exploration, and types of phage applications in freshwater. We also discuss the possible challenges and future possibilities for the field.
2 Identification of phages in freshwater
The metaviromics field (phage metagenomics) essentially is a shotgun metagenomic approach focused on studying the genomes of viral populations from the environment (Hurwitz and Sullivan, 2013; Coutinho et al., 2017; Moon and Cho, 2021), and due to the importance of freshwater bodies as sources of drinking water, recreation, and commerce, more recent studies have dedicated their efforts to freshwater systems (Bruder et al., 2016). Since water chemistry and hydrological factors can contribute to a dynamic environment on a microbial level, likely to be reflected in the indigenous phage populations, the exploration of metagenomic data sampled from freshwater sources from different biomes and places in the world is bound to reveal a plethora of yet unknown and undocumented species of phages (Hayes et al., 2017; Alanazi et al., 2022).
Previous studies have explored how nutrient availability, seasonality, temperature, and human activity influence freshwater viral communities (Bruder et al., 2016). By example, the study of Mohiuddin and Schellhorn (2015) observed that geographic location does not appear to have had a major impact on viral abundance and diversity for two freshwater lakes of the lower Great Lakes region, Lake Ontario and Lake Erie, since the virome composition of both lakes were found to be similar. However, temporal variation in taxonomic composition was observed for both lakes after a year apart sampling.
Another interesting relationship against phage diversity are the possibly related effects of anthropogenic actions on the microbial environment. The study of Green et al., (2015) of the Virginian Lake Matoaka found viral species richness and diversity to be negatively correlated with the level of human activity at the sampling sites, observing the highest levels of diversity and species richness at the main body of the lake, the area least affected by human activity. Another study, conducted by Fancello et al. (2013), observed that the most anthropogenically influenced out of four perennial ponds of the Mauritanian Sahara presented the lowest amount of viral diversity, and higher abundance of heterotrophic microorganisms and human pathogens.
Freshwater viral metagenomics studies also can assist in tackling significant threats to global health, such as the spread of antibiotic resistance. Not only antibiotic resistance genes (ARGs) can spread across different bacterial populations through horizontal gene transfer mediated by bacteriophages, but bacteriophage-carried ARGs are especially threatening due to their prolonged persistence in the environment, fast replication rates, and ability to infect diverse hosts (Brown-Jaque et al., 2015). Moon et al. (2020) explored ARGs recovered from urban surface water viral metagenome data, revealing novel phage-borne antibiotic resistance genes that were also found in bacterial metagenomes, indicating that they were harbored by actively infecting phages. These results suggest that those environmental bacteriophages could act as reservoirs of unknown ARGs that could be widely disseminated via virus-host interactions and illustrate the potential of the viral metagenomics for the discovery of phages involved in spreading antimicrobial resistance on the environment.
In addition, freshwater metagenomic data can also be used to study the viral ecology in the context of other organisms. Chen et al. (2019) investigated and revealed a worldwide distribution of distinct phage genotypes that may infect Fonsibacter, one the most abundant bacterioplankton in freshwater ecosystems, suggesting their substantial role in shaping indigenous microbial communities and potentially significant influence on biogeochemical cycling.
3 In silico phage mining with bioinformatic tools
Due to the advances in sequencing technologies and in viral databases, we selected some of the currently most used tools developed to analyze the viral community on metaviromic data. A classic virome analysis pipeline include tools for (i) assembly, (ii) viral sequence prediction, (iii) quality check, (iv) annotation, (v) taxonomy classification, (vi) phage-host prediction tools and (vii) viral microdiversity analysis (Table 1), some being also present in general metagenomic studies (steps i, iv and v). They are essential to understand the diversity of viruses and know their function in the environment, and can be used to identify new uncultivated viral genomes (UViGs) (Green et al., 2015; Moon and Cho, 2021; Naknaen et al., 2021).
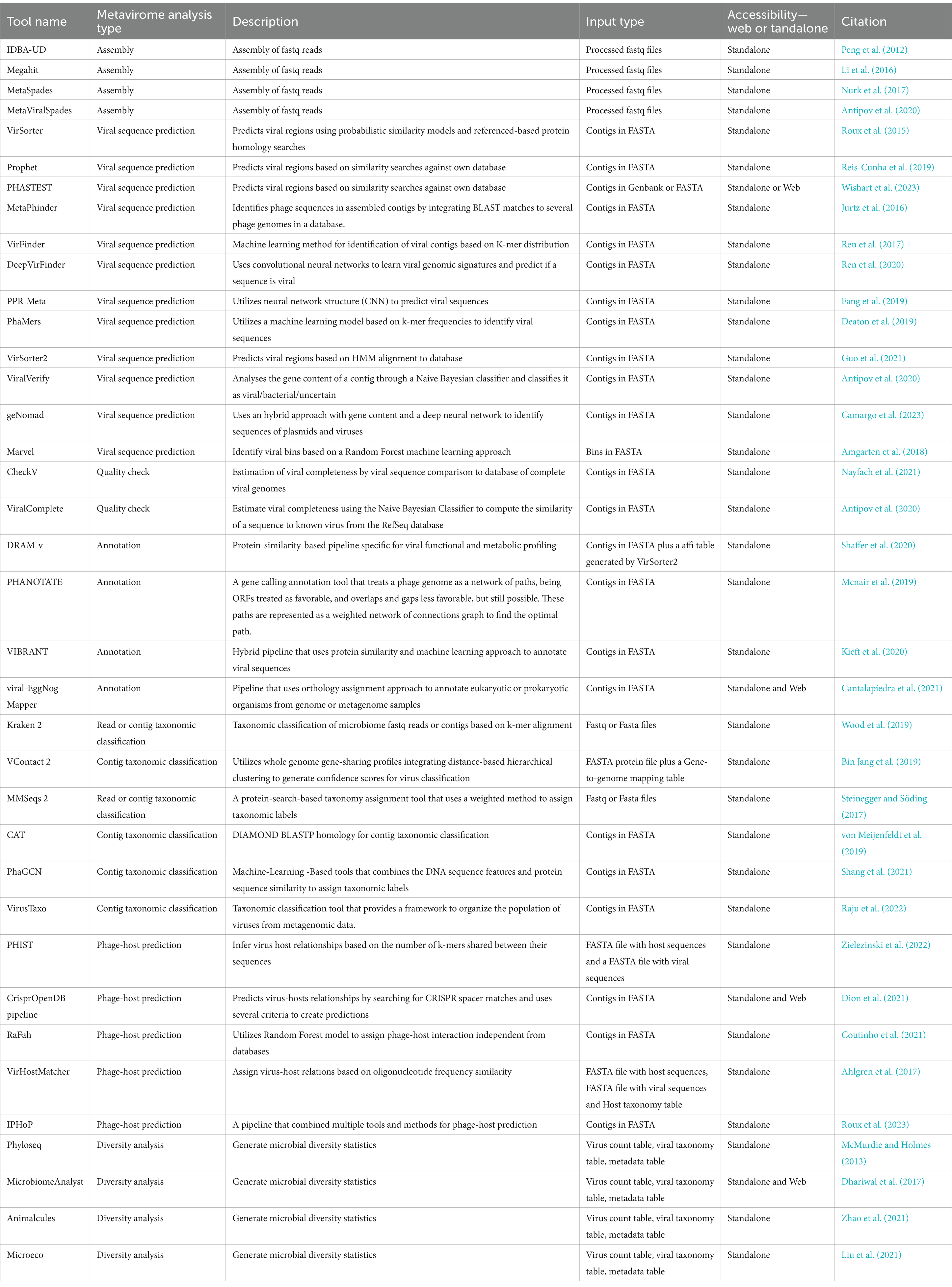
Table 1. Tools available for metagenomic analysis of data for viral identification from environmental data.
In 2017, Roux et al. (2017), identified IDBA-UD (Peng et al., 2012), Megahit (Li et al., 2016), and MetaSpades (Nurk et al., 2017) as the best available options for assembly of viral contigs from short reads. Later on Sutton et al. (2019) analyzed a set of simulated, mocked, and human gut virome with 16 assemblers and identified MetaSpades as the most efficient. However, it showed less effectiveness in reconstructing microdiversity, being more useful to study the mutation rates of the virome. Additionally, although not present in the previous study for being later published, MetaViralSpades (Antipov et al., 2020), a variation of MetaSpades (Nurk et al., 2017), outperformed it in an analysis of 18 real virome data sets, where the contig completeness was superior in 12 cases (Antipov et al., 2020).
After the assembly, a viral sequence prediction analysis can be applied to filter out phages’ host sequences from the metagenomic data. There are three main approaches (Andrade-Martínez et al., 2022) which includes tools that uses protein homology searches to databases: VirSorter (Roux et al., 2015), Prophet (Reis-Cunha et al., 2019), PHASTEST (Wishart et al., 2023), MetaPhinder (Jurtz et al., 2016); machine learning based tools that employs reference-free viral genomic features detection: VirFinder (Ren et al., 2017), DeepVirFinder (Ren et al., 2020), PPR-Meta (Fang et al., 2019), PhaMers (Deaton et al., 2019); and hybrid tools that employ machine learning classification reference based or reference independent: VirSorter2 (Nurk et al., 2017; Guo et al., 2021), ViralVerify (Nurk et al., 2017), geNomad (Camargo et al., 2023), Marvel (Amgarten et al., 2018), and VIBRANT (Kieft et al., 2020) (which can do the steps of identify viral sequences, annotation, and determine genome quality and completeness) (Table 1). Each methodology will have its limitations, and, for machine learning, is related to how updated is the training dataset, the alignment-based tools may also be limited by how updated are the datasets and the difficulty to handle large data. The best approach would be a combination of results from tools that utilize different methodologies for phage sequence prediction (Andrade-Martínez et al., 2022).
Contigs obtained from short-read metagenomic sequencing are normally segmented and it might have misleading information, making it difficult to perform further analysis. To help with this issue, the use of tools such as CheckV (Nayfach et al., 2021), ViralComplete (Antipov et al., 2020), or VIBRANT (Kieft et al., 2020), that identify the completeness and possible host contamination on viral genomes is essential, but yet, still need improvements due to be dependable of the database of virus and the tools used (Green et al., 2015; Sutton et al., 2019). In terms of annotation, some of the most known tools to predict ORFs (Open Reading Frames) are prodigal (Hyatt et al., 2010), Glimmer (Delcher et al., 2007) and GeneMarks (Besemer et al., 2001; Andrade-Martínez et al., 2022), but there are other more specific tools for virus annotations such as VIBRANT (Kieft et al., 2020), viral-Eggnog-mapper (Cantalapiedra et al., 2021), DRAM-v (Shaffer et al., 2020), and PHANOTATE (Mcnair et al., 2019; Table 1). They are suitable for viral annotation and can be applied in manual curation of possible viral false positive results taking into account characteristics such as number of viral and cellular genes hits, bitscores, absence of viral hallmark genes, and presence of plasmid genes (Guo et al., 2021).
For taxonomic classification, it is currently a challenge to find tools that can classify viral sequences under the latest ICTV taxonomy framework, given the high variability, lack of universally conserved genes, and unknown regarding viruses. Kraken 2 (Wood et al., 2019), is a powerful tool for virus taxonomy and identification, and a study performed by Ho et al. (2023) detected a high F1 score of 0.86 in the correct detection of sequences of a viral mock community of characterized viruses. However, it has limited homology to the reference used, so it’s a good option for the identification of known viruses, and when discovery of new viruses is considered, the use of Kraken 2 combined with other tools is advised (Ho et al., 2023). Among the tools that do taxonomy analysis MMSeqs 2 (Steinegger and Söding, 2017), and CAT (von Meijenfeldt et al., 2019), perform protein homology searches to own databases, VContact 2 (Bin Jang et al., 2019), who employs clustering of viral contigs based on shared genes, PhaGCN (Shang et al., 2021), a deep learning classifier based on gene-sharing networks, and VirusTaxo (Raju et al., 2022), that uses a k-mer enrichment database approach (Table 1). All of these tools have customizable databases or the option to retrain their machine-learning models with the latest ICTV taxonomy, which is essential since the ICTV taxonomy is frequently changing (Zhu et al., 2022).
Considering that freshwater environments are expected to have a considerable percentage of new uncultivated viral genomes (UViGs), if a researcher needs to identify its possible host, it is necessary to perform a phage-host prediction. Current methods include mainly similar oligonucleotide frequency (ONF) analysis (VirHostMatcher) (Ahlgren et al., 2017), k-mer similarity (PHIST) (Zielezinski et al., 2022), CRISPR spacer alignment (Dion et al., 2021), and machine learning algorithms (RAFaH) (Coutinho et al., 2021). For researchers new to metavirome analysis it might helpful to use a software that computes the results of other tools such as iPHoP (Roux et al., 2023), which computes the results of six tools utilizing different methodologies and summarizes the putative taxonomy of phage hosts in a table.
The high volume of data produced by the metagenomic studies stimulated the development of tools to simplify the analysis of metagenomic data that also can be applied to metaviromic datasets. Among them, packages such as Phyloseq (McMurdie and Holmes, 2013), MicrobiomeAnalyst (Dhariwal et al., 2017), Animalcules (Zhao et al., 2021), and Microeco (Liu et al., 2021) are some of the most known integrated R packages available (Wen et al., 2023) and offer great set of graphics to support analysis of environmental viruses and their role through metagenomics.
4 Applications of phages in freshwater
Safe drinking water is a high demand limited resource that gains more attention in research as water resources get scarcer worldwide, and multi-resistant water-borne pathogens and overall pollution grows as an even bigger threat to society over the years (Mathieu et al., 2019). Approximately one-ninth of the global population reportedly lacks access to safe drinking water (Jassim et al., 2016). Given the capacity of phages to infect bacterial hosts, they have recently been used as novel tools in water pollution control, to monitor and treat fresh and wastewater (Ji et al., 2021).
4.1 Bacteriophages as pollution indicators in water
There have been a few applied methods using phages to evaluate water quality as properties indicators to monitor pathogenic bacteria in wastewater. Immobilized phages have been used on an electrode surface as biorecognition elements, through a technique known as electrochemical impedance spectroscopy (EIS), to detect bacteria, such as E. coli, Staphylococcus aureus, and Pseudomonas aeruginosa (Yue et al., 2017; Zhou et al., 2017; Richter et al., 2018). Phages have also been employed as capture elements by other alternative combinations with nanoparticles for bacterial pathogen detection (Richter et al., 2018), and as biomechanisms to assess membrane performance and monitor membrane integrity in water treatment facilities (McMinn et al., 2017; Wu et al., 2017; Dias et al., 2018).
A specific group of bacteriophages named crAssphages have been proposed as potential universal human feces viral indicators in water bodies (Farkas et al., 2019; Mafumo et al., 2023). CrAssphages were described by Dutilh et al. (2014) as the most abundant phages in the human gut virome. Further studies identified that crAssphages are highly specific and abundant to human feces (Sabar et al., 2022), highly prevalent in sewage samples (Stachler et al., 2017), and maintain correlation to the presence of human enteric viruses in water (Jennings et al., 2020). Given the previous characteristics, crAssphages have been preconized in favor of currently used fecal indicator bacteria (FIB), which poorly explain viral pathogen dynamics in water and have low host specificity, making difficult the identification of the source of contamination (Ward et al., 2020; Toribio-Avedillo et al., 2021; Mafumo et al., 2023). CrAssphage applicability has been evaluated in several countries (Crank et al., 2020; Ward et al., 2020; Sangkaew et al., 2021; Nam et al., 2022) and shows promising possibilities for human fecal contamination detection in freshwater.
4.2 Bacteriophages in water treatment
Another challenge that greatly affects the operation of wastewater treatment systems is the formation of flocs and sludge bulking by filamentous microorganisms that proliferate excessively that form thick, viscous foams (Aracic et al., 2015). The study conducted by Petrovski et al. (2011a,b) showed how phages that can lyse multiple host bacteria can circumvent the stability of foams. Additionally, Liu et al. (2015) performed tests in a simulated aeration tank system using isolated Gordonia phages, achieving significant reduction in the sludge sedimentation volume. However, all these methods are still experimental as current research still focuses on evaluating and monitoring the behavior of potential phage candidates on wastewater treatment systems (Reisoglu and Aydin, 2023).
Other lines of research have employed phages as low-cost biological control agents to treat specific pathogenic bacteria in sewage. Studies reported the successful inhibition and lysis of drug-resistant A. baumannii (Lin et al., 2010), waterborne disease-causing Vibrio cholerae (Wei et al., 2011), and dysentery-causing Shigella (Jun et al., 2016) through the combination of different phages in co-culture essays. Also, some studies act on the biological control of cyanobacteria, harmful prokaryotes often causing water blooms on green or red tides and producing cyanotoxins, which endanger the surrounding wildlife, aquatic farming animals, threaten human health and can cause tremendous economic losses (Jassim and Limoges, 2013). The strategy in some of those studies is to isolate and employ cyanophages that effectively reduce phycobilisome proteins and destroy the thylakoid structure of cyanobacteria (Gao et al., 2012; Yoshida-Takashima et al., 2012).
However, for both cases, some problems still emerge in the practical application of phage-based biological control, with the emergence of host-resistant mutants, the reduction of cyanophage infectivity caused by sunlight irradiation, and the feasibility of multiple-host approaches are still challenges to be overcome. Nonetheless, phage-based technology also has the advantage of reducing the use of chemical reagents, thus reinforcing the appeal of such strategies and interest in their future development (Mathieu et al., 2019; Ji et al., 2021).
5 Current limitations and perspectives
The study of viral sequences in environmental samples is challenging due to the low representativity or fragmentation of DNA in short sequencing data, the high error rate and the large amount of DNA necessary for long-read sequencing (Warwick-Dugdale et al., 2019). As technology advances, improved read length and sequencing quality have partially addressed this issue. This progress has also opened up the opportunity to implement hybrid approaches for sequencing, combining short and long reads that might allow better environmental virus detection, characterization, and understanding of the microdiversity of virus populations (Warwick-Dugdale et al., 2019; Pratama et al., 2021; Andrade-Martínez et al., 2022).
For the identification of phages, common tools employ distinct methods, such as sequence composition, sequence similarity, and machine learning approaches (Titus Brown and Irber, 2016; Fang et al., 2019; Kieft et al., 2020), but there is no standardization for these techniques. Currently, each method yields slightly different results, and phage identification still relies heavily on trial and error usage of software packages. It is crucial that a golden standard be established to ensure the robustness of methodologies and techniques, thereby enhancing the replicability and reliability of phage identification.
An alternative for an assembly-free, culture-independent study of phages is the analysis of the whole genome of phages by using long-read sequencing technologies, like Oxford nanopore or PacBio technologies (Warwick-Dugdale et al., 2019; Zaragoza-Solas et al., 2022). The advantages of this approach are avoiding over-fragmentation of sequencing data and adopting portable sequencing technologies, allowing the researcher to identify phages from natural sources in situ (Warwick-Dugdale et al., 2019). This opportunity leads to the study of phages directly from their natural environment, allowing for the identification of phages and the analysis of the samples in real time, which is a significant and desirable feature for the genomic surveillance field (Lisotto et al., 2021).
Most virus databases are derived from uncultivated viral genomes (UViGs) representing >95% of public databases (Roux et al., 2019), leading to another significant problem: most of the phage-host interactions are obtained solely from in silico predictions of the study of metagenomes. This lack of lab-studied observations implies the absence of a clear understanding of host-phage dynamics in nature (Coclet and Roux, 2021). In addition to avoiding the intrinsic wet lab biases, such as the identification of false positive or negative viruses due to contamination, the increase of biases related to the process of sample collection, storage, genetic material extraction, purification, and sequencing (Cantalupo and Pipas, 2019). However, the lack of this holistic vision might affect the build of future databases and the scientific interpretations from related results, so it is vital to keep these current limitations presented by bioinformatics tools in mind and apply different combinations of analysis to confirm the identity of phages coming from metagenomic data (Roux et al., 2013, 2019).
Author contributions
CD: Writing – original draft. DM: Writing – original draft. WN: Writing – original draft. OA: Formal analysis, Supervision, Writing – review & editing. RR: Conceptualization, Formal analysis, Supervision, Writing – review & editing.
Funding
The author(s) declare that financial support was received for this article’s research, authorship, and publication. The authors would like to thank the funding agencies Coordenação de Aperfeiçoamento de Pessoal de Nível Superior (CAPES) and National Council for Scientific and Technological Development (CNPq), the support by the CNPq project #312316/2022-4, the Secretary of State for Science, Technology, and Professional and Technological Education (SECTET), the Dean’s Office for Research and Graduate Studies/Federal University of Pará–PROPESP/UFPA (PAPQ), and the partnership SECTEC/UFPA/FADESP for the financial support on this work.
Conflict of interest
The authors declare that the research was conducted in the absence of any commercial or financial relationships that could be construed as a potential conflict of interest.
Publisher's note
All claims expressed in this article are solely those of the authors and do not necessarily represent those of their affiliated organizations, or those of the publisher, the editors and the reviewers. Any product that may be evaluated in this article, or claim that may be made by its manufacturer, is not guaranteed or endorsed by the publisher.
Supplementary material
The Supplementary material for this article can be found online at: https://www.frontiersin.org/articles/10.3389/fmicb.2024.1390726/full#supplementary-material
References
Ahlgren, N. A., Ren, J., Lu, Y. Y., Fuhrman, J. A., and Sun, F. (2017). Alignment-free $d_2^*$ oligonucleotide frequency dissimilarity measure improves prediction of hosts from metagenomically-derived viral sequences. Nucleic Acids Res. 45, 39–53. doi: 10.1093/nar/gkw1002
Alanazi, F., Nour, I., Hanif, A., Al-Ashkar, I., Aljowaie, R. M., and Eifan, S. (2022). Novel findings in context of molecular diversity and abundance of bacteriophages in wastewater environments of Riyadh, Saudi Arabia. PLoS One 17:e0273343. doi: 10.1371/journal.pone.0273343
Amgarten, D., Braga, L. P. P., da Silva, A. M., and Setubal, J. C. (2018). MARVEL, a tool for prediction of bacteriophage sequences in metagenomic bins. Front. Genet. 9:304. doi: 10.3389/fgene.2018.00304
Andrade-Martínez, J. S., Camelo Valera, L. C., Chica Cárdenas, L. A., Forero-Junco, L., López-Leal, G., Moreno-Gallego, J. L., et al. (2022). Computational tools for the analysis of uncultivated phage genomes. Microbiol. Mol. Biol. Rev. 86:e0000421. doi: 10.1128/mmbr.00004-21
Antipov, D., Raiko, M., Lapidus, A., and Pevzner, P. A. (2020). Metaviral SPAdes: assembly of viruses from metagenomic data. Bioinformatics 36, 4126–4129. doi: 10.1093/bioinformatics/btaa490
Aracic, S., Manna, S., Petrovski, S., Wiltshire, J. L., Mann, G., and Franks, A. E. (2015). Innovative biological approaches for monitoring and improving water quality. Front. Microbiol. 6:826. doi: 10.3389/fmicb.2015.00826
Ben Saad, M., Ben Said, M., Bousselmi, L., and Ghrabi, A. (2022). Use of bacteriophage to inactivate pathogenic bacteria from wastewater. J. Environ. Sci. Health A 57, 111–116. doi: 10.1080/10934529.2022.2036551
Bergh, Ø., KY, B. Ø., Bratbak, G., and Heldal, M. (1989). High abundance of viruses found in aquatic environments. Nature 340, 467–468. doi: 10.1038/340467a0
Besemer, J., Lomsadze, A., and Borodovsky, M. (2001). GeneMarkS: a self-training method for prediction of gene starts in microbial genomes. Implications for finding sequence motifs in regulatory regions. Nucleic Acids Res. 29, 2607–2618. doi: 10.1093/nar/29.12.2607
Bin Jang, H., Bolduc, B., Zablocki, O., Kuhn, J. H., Roux, S., Adriaenssens, E. M., et al. (2019). Taxonomic assignment of uncultivated prokaryotic virus genomes is enabled by gene-sharing networks. Nat. Biotechnol. 37, 632–639. doi: 10.1038/s41587-019-0100-8
Breitbart, M., Bonnain, C., Malki, K., and Sawaya, N. A. (2018). Phage puppet masters of the marine microbial realm. Nat. Microbiol.. Nature Publishing Group 3, 754–766. doi: 10.1038/s41564-018-0166-y
Brown-Jaque, M., Calero-Cáceres, W., and Muniesa, M. (2015). Transfer of antibiotic-resistance genes via phage-related mobile elements. Plasmid 79, 1–7. doi: 10.1016/j.plasmid.2015.01.001
Bruder, K., Malki, K., Cooper, A., Sible, E., Shapiro, J. W., Watkins, S. C., et al. (2016). Freshwater metaviromics and bacteriophages: a current assessment of the state of the art in relation to bioinformatic challenges. Evol. Bioinforma. 12, 25–33. doi: 10.4137/EBO.S38549
Camargo, A. P., Roux, S., Schulz, F., Babinski, M., Xu, Y., Hu, B., et al. (2023). Identification of mobile genetic elements with geNomad. Nat. Biotechnol. doi: 10.1038/s41587-023-01953-y
Cantalapiedra, C. P., Hernández-Plaza, A., Letunic, I., Bork, P., and Huerta-Cepas, J. (2021). eggNOG-mapper v2: functional annotation, orthology assignments, and domain prediction at the metagenomic scale. Mol. Biol. Evol. 38, 5825–5829. doi: 10.1093/molbev/msab293
Cantalupo, P. G., and Pipas, J. M. (2019). Detecting viral sequences in NGS data. Curr. Opin. Virol. 39, 41–48. doi: 10.1016/j.coviro.2019.07.010
Chen, L. X., Zhao, Y., McMahon, K. D., Mori, J. F., Jessen, G. L., Nelson, T. C., et al. (2019). Wide distribution of phage that infect freshwater SAR11 Bacteria. mSystems. 4:e00410-19. doi: 10.1128/mSystems.00410-19
Chevallereau, A., Pons, B. J., van Houte, S., and Westra, E. R. (2022). Interactions between bacterial and phage communities in natural environments. Nat Rev Microbiol 20, 49–62. doi: 10.1038/s41579-021-00602-y
Coclet, C., and Roux, S. (2021). Global overview and major challenges of host prediction methods for uncultivated phages. Curr. Opin. Virol. 49, 117–126. doi: 10.1016/j.coviro.2021.05.003
Coutinho, F. H., Silveira, C. B., Gregoracci, G. B., Thompson, C. C., Edwards, R. A., Brussaard, C. P. D., et al. (2017). Marine viruses discovered via metagenomics shed light on viral strategies throughout the oceans. Nat. Commun. 8:15955. doi: 10.1038/ncomms15955
Coutinho, F. H., Zaragoza-Solas, A., López-Pérez, M., Barylski, J., Zielezinski, A., Dutilh, B. E., et al. (2021). RaFAH: host prediction for viruses of Bacteria and Archaea based on protein content. Patterns. 2:100274. doi: 10.1016/j.patter.2021.100274
Crank, K., Li, X., North, D., Ferraro, G. B., Iaconelli, M., Mancini, P., et al. (2020). CrAssphage abundance and correlation with molecular viral markers in Italian wastewater. Water Res. 184:116161. doi: 10.1016/j.watres.2020.116161
De Mandal, S., Panda, A. K., Kumar, N. S., Bisht, S. S., and Jin, F. (2021). Metagenomics and microbial ecology. Boca Raton: CRC Press.
Deaton, J., Yu, F. B., and Quake, S. R. (2019). Mini-metagenomics and nucleotide composition aid the identification and host Association of Novel Bacteriophage Sequences. Adv Biosyst 3:e1900108. doi: 10.1002/adbi.201900108
Delcher, A. L., Bratke, K. A., Powers, E. C., and Salzberg, S. L. (2007). Identifying bacterial genes and endosymbiont DNA with glimmer. Bioinformatics 23, 673–679. doi: 10.1093/bioinformatics/btm009
Dhariwal, A., Chong, J., Habib, S., King, I. L., Agellon, L. B., and Xia, J. (2017). MicrobiomeAnalyst: a web-based tool for comprehensive statistical, visual and meta-analysis of microbiome data. Nucleic Acids Res. 45, W180–W188. doi: 10.1093/nar/gkx295
Dias, E., Ebdon, J., and Taylor, H. (2018). The application of bacteriophages as novel indicators of viral pathogens in wastewater treatment systems. Water Res. 129, 172–179. doi: 10.1016/j.watres.2017.11.022
Dion, M. B., Oechslin, F., and Moineau, S. (2020). Phage diversity, genomics and phylogeny. Nat. Rev. Microbiol. 18, 125–138. doi: 10.1038/s41579-019-0311-5
Dion, M. B., Plante, P. L., Zufferey, E., Shah, S. A., Corbeil, J., and Moineau, S. (2021). Streamlining CRISPR spacer-based bacterial host predictions to decipher the viral dark matter. Nucleic Acids Res. 49, 3127–3138. doi: 10.1093/nar/gkab133
Dutilh, B. E., Cassman, N., McNair, K., Sanchez, S. E., Silva, G. G. Z., Boling, L., et al. (2014). A highly abundant bacteriophage discovered in the unknown sequences of human faecal metagenomes. Nat. Commun. 5:4498. doi: 10.1038/ncomms5498
Fancello, L., Trape, S., Robert, C., Boyer, M., Popgeorgiev, N., Raoult, D., et al. (2013). Viruses in the desert: a metagenomic survey of viral communities in four perennial ponds of the Mauritanian Sahara. ISME J. 7, 359–369. doi: 10.1038/ismej.2012.101
Fang, Z., Tan, J., Wu, S., Li, M., Xu, C., Xie, Z., et al. (2019). PPR-meta: a tool for identifying phages and plasmids from metagenomic fragments using deep learning. Gigascience 8:giz066. doi: 10.1093/gigascience/giz066
Farkas, K., Adriaenssens, E. M., Walker, D. I., McDonald, J. E., Malham, S. K., and Jones, D. L. (2019). Critical evaluation of CrAssphage as a molecular marker for human-derived wastewater contamination in the aquatic environment. Food Environ Virol 11, 113–119. doi: 10.1007/s12560-019-09369-1
Gao, E. B., Gui, J. F., and Zhang, Q. Y. (2012). A novel Cyanophage with a cyanobacterial nonbleaching protein a gene in the genome. J. Virol. 86, 236–245. doi: 10.1128/JVI.06282-11
Green, J., Rahman, F., Saxton, M., and Williamson, K. (2015). Metagenomic assessment of viral diversity in Lake Matoaka, a temperate, eutrophic freshwater lake in southeastern Virginia, USA. Aquat. Microb. Ecol. 75, 117–128. doi: 10.3354/ame01752
Guo, J., Bolduc, B., Zayed, A. A., Varsani, A., Dominguez-Huerta, G., Delmont, T. O., et al. (2021). VirSorter2: a multi-classifier, expert-guided approach to detect diverse DNA and RNA viruses. Microbiome 9:37. doi: 10.1186/s40168-020-00990-y
Guo, J., Vik, D., Pratama, A. A., Roux, S., and Sullivan, M.. Viral sequence identification SOP with VirSorter2. protocols.io. (2021). Available at: https://www.protocols.io/view/viral-sequence-identification-sop-with-virsorter2-5qpvoyqebg4o/v3 (Accessed Jan 5, 2024).
Hayes, S., Mahony, J., Nauta, A., and van Sinderen, D. (2017). Metagenomic approaches to assess bacteriophages in various environmental niches. Viruses 9:127. doi: 10.3390/v9060127
Ho, S. F. S., Wheeler, N. E., Millard, A. D., and van Schaik, W. (2023). Gauge your phage: benchmarking of bacteriophage identification tools in metagenomic sequencing data. Microbiome 11:84. doi: 10.1186/s40168-023-01533-x
Hu, M., Xing, B., Yang, M., Han, R., Pan, H., Guo, H., et al. (2023). Characterization of a novel genus of jumbo phages and their application in wastewater treatment. iScience 26:106947. doi: 10.1016/j.isci.2023.106947
Hurwitz, B. L., and Sullivan, M. B. (2013). The Pacific Ocean Virome (POV): a marine viral metagenomic dataset and associated protein clusters for quantitative viral ecology. PLoS One 8:e57355. doi: 10.1371/journal.pone.0057355
Hyatt, D., Chen, G. L., LoCascio, P. F., Land, M. L., Larimer, F. W., and Hauser, L. J. (2010). Prodigal: prokaryotic gene recognition and translation initiation site identification. BMC Bioinformatics 11:119. doi: 10.1186/1471-2105-11-119
Hyman, P. (2019). Phages for phage therapy: isolation, characterization, and host range breadth. Pharmaceuticals 12:35. doi: 10.3390/ph12010035
Jassim, S. A. A., and Limoges, R. G. (2013). Impact of external forces on cyanophage–host interactions in aquatic ecosystems. World J. Microbiol. Biotechnol. 29, 1751–1762. doi: 10.1007/s11274-013-1358-5
Jassim, S. A. A., Limoges, R. G., and El-Cheikh, H. (2016). Bacteriophage biocontrol in wastewater treatment. World J. Microbiol. Biotechnol. 32:70. doi: 10.1007/s11274-016-2028-1
Jennings, W. C., Gálvez-Arango, E., Prieto, A. L., and Boehm, A. B. (2020). CrAssphage for fecal source tracking in Chile: covariation with norovirus, HF183, and bacterial indicators. Water Res X 9:100071. doi: 10.1016/j.wroa.2020.100071
Ji, M., Liu, Z., Sun, K., Li, Z., Fan, X., and Li, Q. (2021). Bacteriophages in water pollution control: advantages and limitations. Front. Environ. Sci. Eng. 15:84. doi: 10.1007/s11783-020-1378-y
Jun, J. W., Giri, S. S., Kim, H. J., Yun, S. K., Chi, C., Chai, J. Y., et al. (2016). Bacteriophage application to control the contaminated water with Shigella. Sci. Rep. 6:22636. doi: 10.1038/srep22636
Jurtz, V. I., Villarroel, J., Lund, O., Voldby Larsen, M., and Nielsen, M. (2016). MetaPhinder—identifying bacteriophage sequences in metagenomic data sets. PLoS One 11:e0163111. doi: 10.1371/journal.pone.0163111
Kieft, K., Zhou, Z., and Anantharaman, K. (2020). VIBRANT: automated recovery, annotation and curation of microbial viruses, and evaluation of viral community function from genomic sequences. Microbiome 8:90. doi: 10.1186/s40168-020-00867-0
Li, D., Luo, R., Liu, C. M., Leung, C. M., Ting, H. F., Sadakane, K., et al. (2016). MEGAHIT v1.0: a fast and scalable metagenome assembler driven by advanced methodologies and community practices. Methods 102, 3–11. doi: 10.1016/j.ymeth.2016.02.020
Lin, N. T., Chiou, P. Y., Chang, K. C., Chen, L. K., and Lai, M. J. (2010). Isolation and characterization of ϕAB2: a novel bacteriophage of Acinetobacter baumannii. Res. Microbiol. 161, 308–314. doi: 10.1016/j.resmic.2010.03.007
Lisotto, P., Raangs, E. C., Couto, N., Rosema, S., Lokate, M., Zhou, X., et al. (2021). Long-read sequencing-based in silico phage typing of vancomycin-resistant Enterococcus faecium. BMC Genomics 22:758. doi: 10.1186/s12864-021-08080-5
Liu, C., Cui, Y., Li, X., and Yao, M. (2021). Microeco: an R package for data mining in microbial community ecology. FEMS Microbiol. Ecol. 97:fiaa255. doi: 10.1093/femsec/fiaa255
Liu, M., Gill, J. J., Young, R., and Summer, E. J. (2015). Bacteriophages of wastewater foaming-associated filamentous Gordonia reduce host levels in raw activated sludge. Sci. Rep. 5:13754. doi: 10.1038/srep13754
Luo, X. Q., Wang, P., Li, J. L., Ahmad, M., Duan, L., Yin, L. Z., et al. (2022). Viral community-wide auxiliary metabolic genes differ by lifestyles, habitats, and hosts. Microbiome 10:190. doi: 10.1186/s40168-022-01384-y
Mafumo, N., Bezuidt, O. K. I., le Roux, W., and Makhalanyane, T. P. (2023). CrAssphage may be viable markers of contamination in pristine and Contaminated River water. mSystems 8:e0128222. doi: 10.1128/msystems.01282-22
Mathieu, J., Yu, P., Zuo, P., Da Silva, M. L. B., and Alvarez, P. J. J. (2019). Going viral: emerging opportunities for phage-based bacterial control in water treatment and reuse. Acc. Chem. Res. 52, 849–857. doi: 10.1021/acs.accounts.8b00576
McMinn, B. R., Ashbolt, N. J., and Korajkic, A. (2017). Bacteriophages as indicators of faecal pollution and enteric virus removal. Lett. Appl. Microbiol. 65, 11–26. doi: 10.1111/lam.12736
McMurdie, P. J., and Holmes, S. (2013). Phyloseq: an R package for reproducible interactive analysis and graphics of microbiome census data. PLoS One 8:e61217. doi: 10.1371/journal.pone.0061217
Mcnair, K., Zhou, C., Dinsdale, E. A., Souza, B., and Edwards, R. A. (2019). PHANOTATE: a novel approach to gene identification in phage genomes. Bioinformatics 35, 4537–4542. doi: 10.1093/bioinformatics/btz265
Mohiuddin, M., and Schellhorn, H. E. (2015). Spatial and temporal dynamics of virus occurrence in two freshwater lakes captured through metagenomic analysis. Front. Microbiol. 6:960. doi: 10.3389/fmicb.2015.00960
Moon, K., and Cho, J. C. (2021). Metaviromics coupled with phage-host identification to open the viral ‘black box.’. J. Microbiol. 59, 311–323. doi: 10.1007/s12275-021-1016-9
Moon, K., Jeon, J. H., Kang, I., Park, K. S., Lee, K., Cha, C. J., et al. (2020). Freshwater viral metagenome reveals novel and functional phage-borne antibiotic resistance genes. Microbiome 8:75. doi: 10.1186/s40168-020-00863-4
Naknaen, A., Suttinun, O., Surachat, K., Khan, E., and Pomwised, R. (2021). A novel jumbo phage PhiMa05 inhibits harmful Microcystis sp. Front. Microbiol. 12:660351. doi: 10.3389/fmicb.2021.660351
Nam, S. J., Hu, W. S., and Koo, O. K. (2022). Evaluation of crAssphage as a human-specific microbial source-tracking marker in the Republic of Korea. Environ. Monit. Assess. 194:367. doi: 10.1007/s10661-022-09918-5
Nayfach, S., Camargo, A. P., Schulz, F., Eloe-Fadrosh, E., Roux, S., and Kyrpides, N. C. (2021). CheckV assesses the quality and completeness of metagenome-assembled viral genomes. Nat. Biotechnol. 39, 578–585. doi: 10.1038/s41587-020-00774-7
Nurk, S., Meleshko, D., Korobeynikov, A., and Pevzner, P. A. (2017). metaSPAdes: a new versatile metagenomic assembler. Genome Res. 27, 824–834. doi: 10.1101/gr.213959.116
Peng, Y., Leung, H. C. M., Yiu, S. M., and Chin, F. Y. L. (2012). IDBA-UD: a de novo assembler for single-cell and metagenomic sequencing data with highly uneven depth. Bioinformatics 28, 1420–1428. doi: 10.1093/bioinformatics/bts174
Petrovski, S., Seviour, R. J., and Tillett, D. (2011a). Prevention of Gordonia and Nocardia stabilized foam formation by using bacteriophage GTE7. Appl. Environ. Microbiol. 77, 7864–7867. doi: 10.1128/AEM.05692-11
Petrovski, S., Seviour, R. J., and Tillett, D. (2011b). Characterization of the genome of the polyvalent lytic bacteriophage GTE2, which has potential for biocontrol of Gordonia-, Rhodococcus-, and Nocardia-stabilized foams in activated sludge plants. Appl. Environ. Microbiol. 77, 3923–3929. doi: 10.1128/AEM.00025-11
Pratama, A. A., Bolduc, B., Zayed, A. A., Zhong, Z. P., Guo, J., Vik, D. R., et al. (2021). Expanding standards in viromics: in silico evaluation of dsDNA viral genome identification, classification, and auxiliary metabolic gene curation. PeerJ 9:e11447. doi: 10.7717/peerj.11447
Raju, R. S., Al Nahid, A., Chondrow Dev, P., and Islam, R. (2022). VirusTaxo: taxonomic classification of viruses from the genome sequence using k-mer enrichment. Genomics 114:110414. doi: 10.1016/j.ygeno.2022.110414
Reddy, S., Kaur, K., Barathe, P., Shriram, V., Govarthanan, M., and Kumar, V. (2022). Antimicrobial resistance in urban river ecosystems. Microbiol. Res. 263:127135. doi: 10.1016/j.micres.2022.127135
Reis-Cunha, J. L., Bartholomeu, D. C., Manson, A. L., Earl, A. M., and Cerqueira, G. C. (2019). ProphET, prophage estimation tool: a stand-alone prophage sequence prediction tool with self-updating reference database. PLoS One 14:e0223364. doi: 10.1371/journal.pone.0223364
Reisoglu, Ş., and Aydin, S. (2023). Bacteriophages as a promising approach for the biocontrol of antibiotic resistant pathogens and the reconstruction of microbial interaction networks in wastewater treatment systems: a review. Sci. Total Environ. 890:164291. doi: 10.1016/j.scitotenv.2023.164291
Ren, J., Ahlgren, N. A., Lu, Y. Y., Fuhrman, J. A., and Sun, F. (2017). VirFinder: a novel k-mer based tool for identifying viral sequences from assembled metagenomic data. Microbiome 5:69. doi: 10.1186/s40168-017-0283-5
Ren, J., Song, K., Deng, C., Ahlgren, N. A., Fuhrman, J. A., Li, Y., et al. (2020). Identifying viruses from metagenomic data using deep learning. Quant. Biol. 8, 64–77. doi: 10.1007/s40484-019-0187-4
Richter, Ł., Janczuk-Richter, M., Niedziółka-Jönsson, J., Paczesny, J., and Hołyst, R. (2018). Recent advances in bacteriophage-based methods for bacteria detection. Drug Discov. Today 23, 448–455. doi: 10.1016/j.drudis.2017.11.007
Rohde, C., Resch, G., Pirnay, J. P., Blasdel, B., Debarbieux, L., Gelman, D., et al. (2018). Expert opinion on three phage therapy related topics: bacterial phage resistance, phage training and prophages in bacterial production strains. Viruses 10:178. doi: 10.3390/v10040178
Rohwer, F., and Edwards, R. (2002). The phage proteomic tree: a genome-based taxonomy for phage. J. Bacteriol. 184, 4529–4535. doi: 10.1128/JB.184.16.4529-4535.2002
Romero-Calle, D., Guimarães Benevides, R., Góes-Neto, A., and Billington, C. (2019). Bacteriophages as alternatives to antibiotics in clinical care. Antibiotics 8:138. doi: 10.3390/antibiotics8030138
Roux, S., Adriaenssens, E. M., Dutilh, B. E., Koonin, E. V., Kropinski, A. M., Krupovic, M., et al. (2019). Minimum information about an uncultivated virus genome (MIUViG). Nat. Biotechnol. 37, 29–37. doi: 10.1038/nbt.4306
Roux, S., Camargo, A. P., Coutinho, F. H., Dabdoub, S. M., Dutilh, B. E., Nayfach, S., et al. (2023). iPHoP: an integrated machine learning framework to maximize host prediction for metagenome-derived viruses of archaea and bacteria. PLoS Biol. 21:e3002083. doi: 10.1371/journal.pbio.3002083
Roux, S., Emerson, J. B., Eloe-Fadrosh, E. A., and Sullivan, M. B. (2017). Benchmarking viromics: an in silico evaluation of metagenome-enabled estimates of viral community composition and diversity. PeerJ 5:e3817. doi: 10.7717/peerj.3817
Roux, S., Enault, F., Hurwitz, B. L., and Sullivan, M. B. (2015). VirSorter: mining viral signal from microbial genomic data. PeerJ 3:e985. doi: 10.7717/peerj.985
Roux, S., Krupovic, M., Debroas, D., Forterre, P., and Enault, F. (2013). Assessment of viral community functional potential from viral metagenomes may be hampered by contamination with cellular sequences. Open Biol. 3:130160. doi: 10.1098/rsob.130160
Sabar, M. A., Honda, R., and Haramoto, E. (2022). CrAssphage as an indicator of human-fecal contamination in water environment and virus reduction in wastewater treatment. Water Res.. Elsevier Ltd 221:118827. doi: 10.1016/j.watres.2022.118827
Sangkaew, W., Kongprajug, A., Chyerochana, N., Ahmed, W., Rattanakul, S., Denpetkul, T., et al. (2021). Performance of viral and bacterial genetic markers for sewage pollution tracking in tropical Thailand. Water Res. 190:116706. doi: 10.1016/j.watres.2020.116706
Santiago-Rodriguez, T. M., and Hollister, E. B. (2023). Viral metagenomics as a tool to track sources of fecal contamination: a one health approach. Viruses. MDPI 15:236. doi: 10.3390/v15010236
Shaffer, M., Borton, M. A., McGivern, B. B., Zayed, A. A., La Rosa, S. L., Solden, L. M., et al. (2020). DRAM for distilling microbial metabolism to automate the curation of microbiome function. Nucleic Acids Res. 48, 8883–8900. doi: 10.1093/nar/gkaa621
Shang, J., Jiang, J., and Sun, Y. (2021). Bacteriophage classification for assembled contigs using graph convolutional network. Bioinformatics 37, i25–i33. doi: 10.1093/bioinformatics/btab293
Stachler, E., Kelty, C., Sivaganesan, M., Li, X., Bibby, K., and Shanks, O. C. (2017). Quantitative CrAssphage PCR assays for human fecal pollution measurement. Environ. Sci. Technol. 51, 9146–9154. doi: 10.1021/acs.est.7b02703
Steinegger, M., and Söding, J. (2017). MMseqs2 enables sensitive protein sequence searching for the analysis of massive data sets. Nat. Biotechnol. 35, 1026–1028. doi: 10.1038/nbt.3988
Sutton, T. D. S., Clooney, A. G., Ryan, F. J., Ross, R. P., and Hill, C. (2019). Choice of assembly software has a critical impact on virome characterisation. Microbiome 7:12. doi: 10.1186/s40168-019-0626-5
Titus Brown, C., and Irber, L. (2016). Sourmash: a library for MinHash sketching of DNA. J. Open Source Softw. 1:27. doi: 10.21105/joss.00027
Toribio-Avedillo, D., Blanch, A. R., Muniesa, M., and Rodríguez-Rubio, L. (2021). Bacteriophages as fecal pollution indicators. Viruses 13:1089. doi: 10.3390/v13061089
Touchon, M., Moura de Sousa, J. A., and Rocha, E. P. (2017). Embracing the enemy: the diversification of microbial gene repertoires by phage-mediated horizontal gene transfer. Curr. Opin. Microbiol. 38, 66–73. doi: 10.1016/j.mib.2017.04.010
Twort, F. W. (1915). An investigation on the nature of ultra-microscopic viruses. Lancet 186, 1241–1243. doi: 10.1016/S0140-6736(01)20383-3
von Meijenfeldt, F. A. B., Arkhipova, K., Cambuy, D. D., Coutinho, F. H., and Dutilh, B. E. (2019). Robust taxonomic classification of uncharted microbial sequences and bins with CAT and BAT. Genome Biol. 20:217. doi: 10.1186/s13059-019-1817-x
Ward, L. M., Ghaju Shrestha, R., Tandukar, S., Sherchand, J. B., Haramoto, E., and Sherchan, S. P. (2020). Evaluation of CrAssphage marker for tracking fecal contamination in river water in Nepal. Water Air Soil Pollut. 231:282. doi: 10.1007/s11270-020-04648-1
Warwick-Dugdale, J., Solonenko, N., Moore, K., Chittick, L., Gregory, A. C., Allen, M. J., et al. (2019). Long-read viral metagenomics captures abundant and microdiverse viral populations and their niche-defining genomic islands. PeerJ 7:e6800. doi: 10.7717/peerj.6800
Wei, Y., Kirby, A., and Levin, B. R. (2011). The population and evolutionary dynamics of vibrio cholerae and its bacteriophage: conditions for maintaining phage-limited communities. Am. Nat. 178, 715–725. doi: 10.1086/662677
Wen, T., Niu, G., Chen, T., Shen, Q., Yuan, J., and Liu, Y. X. (2023). The best practice for microbiome analysis using R. Protein Cell 14, 713–725. doi: 10.1093/procel/pwad024
Wishart, D. S., Han, S., Saha, S., Oler, E., Peters, H., Grant, J. R., et al. (2023). PHASTEST: faster than PHASTER, better than PHAST. Nucleic Acids Res. 51, W443–W450. doi: 10.1093/nar/gkad382
Wood, D. E., Lu, J., and Langmead, B. (2019). Improved metagenomic analysis with kraken 2. Genome Biol. 20:257. doi: 10.1186/s13059-019-1891-0
Wu, B., Wang, R., and Fane, A. G. (2017). The roles of bacteriophages in membrane-based water and wastewater treatment processes: a review. Water Res. 110, 120–132. doi: 10.1016/j.watres.2016.12.004
Yoshida-Takashima, Y., Yoshida, M., Ogata, H., Nagasaki, K., Hiroishi, S., and Yoshida, T. (2012). Cyanophage infection in the bloom-forming Cyanobacteria <i>Microcystis aeruginosa</i> in surface freshwater. Microbes Environ. 27, 350–355. doi: 10.1264/jsme2.ME12037
Yue, H., He, Y., Fan, E., Wang, L., Lu, S., and Fu, Z. (2017). Label-free electrochemiluminescent biosensor for rapid and sensitive detection of pseudomonas aeruginosa using phage as highly specific recognition agent. Biosens. Bioelectron. 94, 429–432. doi: 10.1016/j.bios.2017.03.033
Zaragoza-Solas, A., Haro-Moreno, J. M., Rodriguez-Valera, F., and López-Pérez, M. (2022). Long-read metagenomics improves the recovery of viral diversity from complex natural marine samples. mSystems 7:e0019222:202228. doi: 10.1128/msystems.00192-22
Zhang, M., Zhang, T., Yu, M., Chen, Y. L., and Jin, M. (2022). The life cycle transitions of temperate phages: regulating factors and potential ecological implications. Viruses 14, 14:1904:1904. doi: 10.3390/v14122818
Zhao, Y., Federico, A., Faits, T., Manimaran, S., Segrè, D., Monti, S., et al. (2021). Animalcules: interactive microbiome analytics and visualization in R. Microbiome 9:76. doi: 10.1186/s40168-021-01013-0
Zhou, Y., Marar, A., Kner, P., and Ramasamy, R. P. (2017). Charge-directed immobilization of bacteriophage on nanostructured electrode for whole-cell electrochemical biosensors. Anal. Chem. 89, 5734–5741. doi: 10.1021/acs.analchem.6b03751
Zhu, Y., Shang, J., Peng, C., and Sun, Y. (2022). Phage family classification under Caudoviricetes: a review of current tools using the latest ICTV classification framework. Front. Microbiol. 13:1032186. doi: 10.3389/fmicb.2022.1032186
Keywords: freshwater, phage, bacteriophage, cyanophage, virome
Citation: Dantas CWD, Martins DT, Nogueira WG, Alegria OVC and Ramos RTJ (2024) Tools and methodology to in silico phage discovery in freshwater environments. Front. Microbiol. 15:1390726. doi: 10.3389/fmicb.2024.1390726
Edited by:
Marcin Łoś, University of Gdansk, PolandReviewed by:
Przemyslaw Decewicz, University of Warsaw, PolandCopyright © 2024 Dantas, Martins, Nogueira, Alegria and Ramos. This is an open-access article distributed under the terms of the Creative Commons Attribution License (CC BY). The use, distribution or reproduction in other forums is permitted, provided the original author(s) and the copyright owner(s) are credited and that the original publication in this journal is cited, in accordance with accepted academic practice. No use, distribution or reproduction is permitted which does not comply with these terms.
*Correspondence: Rommel Thiago Jucá Ramos, cm9tbWVsdGhpYWdvQGdtYWlsLmNvbQ==
†These authors have contributed equally to this work and share first authorship