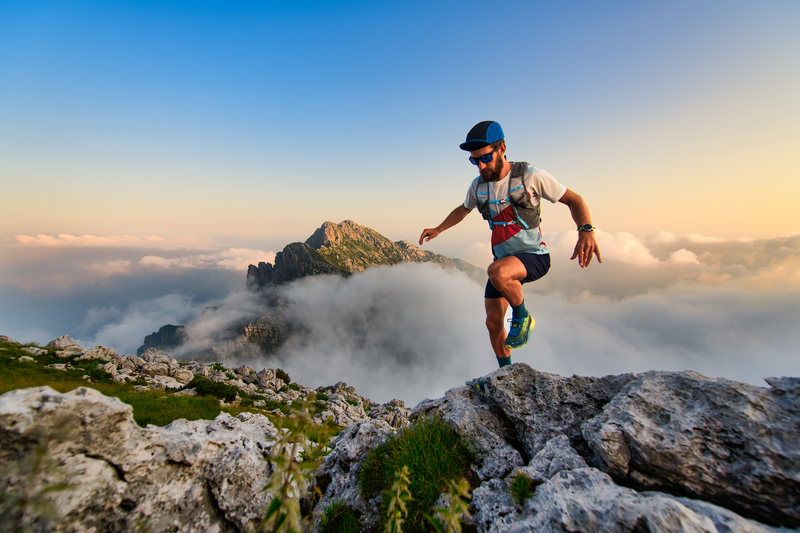
94% of researchers rate our articles as excellent or good
Learn more about the work of our research integrity team to safeguard the quality of each article we publish.
Find out more
ORIGINAL RESEARCH article
Front. Microbiol. , 02 April 2024
Sec. Microorganisms in Vertebrate Digestive Systems
Volume 15 - 2024 | https://doi.org/10.3389/fmicb.2024.1360445
This article is part of the Research Topic Untangle the Broad Connections and Tight Interactions Between Human Microbiota and Complex Diseases Through Data-Driven Approaches, Volume II View all 32 articles
Background: Androgenetic alopecia (AGA) is the most common type of androgen-associated hair loss. Previous studies have indicated an association between the gut microbiota and AGA. To delve deeper, we executed a two-sample Mendelian randomization (MR) analysis to investigate the potential causal relationship between the gut microbiota and AGA.
Methods: A two-sample MR investigation was utilized to delve into the intricate interplay between gut microbiota and AGA. Information regarding 211 gut microbial taxa was sourced from the MiBioGen consortium. The summary statistics of the genome-wide association studies (GWAS) for AGA were obtained from the FinnGen biobank, which included 195 cases and 201,019 controls. Various analytical approaches, including Inverse Variance Weighting (IVW), Weighted Median, MR-Egger, Weighted Mode, and Simple Mode were employed to evaluate the causal impact of gut microbiota on AGA. Sensitivity analyses were subsequently conducted to affirm the robustness of the findings.
Results: A two-sample MR investigation unveiled the genus Olsenella, genus Ruminococcaceae UCG-004, and genus Ruminococcaceae UCG-010 were identified as risk factors associated with AGA. In contrast, the family Acidaminococcaceae and genus Anaerofilum, along with the genus Ruminiclostridium 9, demonstrated a protective effect. The sensitivity analyses provided additional assurance that the findings of the current study were less susceptible to the influence of confounding variables and biases.
Conclusion: The MR study has established a link between specific gut microbiota and AGA, offering evidence for the identification of more precisely targeted probiotics. This discovery has the potential to aid in the prevention, control, and reversal of AGA progression.
Male Pattern Hair Loss (MPHL) and Female Pattern Hair Loss (FPHL) also referred to as Androgenetic alopecia (AGA) stand out as the most prevalent form of hair loss, impacting a substantial portion of the population. It is estimated that by the age of 70, at least 80% of men and 50% of women experience AGA (Devjani et al., 2023). AGA is marked by the gradual miniaturization of hair follicles, resulting in hair loss (Trüeb, 2002). Dealing with alopecia is a challenging and time-consuming process. Individuals experiencing hair loss often suffer from a diminished quality of life, including reduced self-confidence and heightened feelings of depression (Lee et al., 2002; Yeo et al., 2014; Marks et al., 2019). Consequently, the effective management of hair loss plays a crucial role in improving people’s overall wellbeing. To enhance our ability to prevent and treat AGA effectively, it is essential to acquire a more comprehensive understanding of the underlying mechanisms that contribute to its development. Nevertheless, the precise cause behind the escalating incidence rate of AGA has yet to be fully elucidated.
Numerous factors influence the initiation and progression of AGA, the interaction of endocrine factors and genetic predisposition is one of the primary factors (Lolli et al., 2017). Studies have confirmed that a range of external factors, such as metabolism, psychological changes, environmental exposure, dietary intake, and microorganisms, can have adverse effects on the lifespan of hair (Lai et al., 2013; Phillips et al., 2017; Ho et al., 2019; Suzuki et al., 2021). Recently, there is a study has shown that gut microbiota also is an essential factor in the development of AGA (Jung et al., 2022). However, in research related to AGA, there is a relatively limited study on the specific role of the gut microbiome in AGA. The conventional observational study is susceptible to the impact of numerous potential factors, including lifestyle and socioeconomic status, during the implementation process, rendering it prone to biases. Hence, we examined existing summary data from the results of genome-wide association studies (GWAS) to investigate the influence of gut microbiota on AGA.
Genome-wide association studies with large sample sizes has revealed some single nucleotide polymorphisms (SNPs) correlated with both AGA and gut microbiota (Wang et al., 2019). Mendelian randomization (MR) is a method that employs genetic variants linked to a hypothesized risk factor as proxies to ascertain the causal impact of that exposure on a specific outcome (Birney, 2022). In this study, we evaluated the causal effects of gut microbiota and AGA using a two-sample MR study design. Our results demonstrated a potential causal association between specific gut microbiota and AGA.
To explore the interaction between gut microbiota and AGA, we designated gut microbiota as the exposure variable, with AGA considered as outcomes. The MR study (Evans and Davey Smith, 2015) adhered to three crucial assumptions: (1) Instrumental variables (IVs) chosen from datasets were connected to the exposure variable; (2) they were independent of any unknown confounders related to the exposure; and (3) they exclusively influenced outcomes through exposure pathways (Davey Smith and Hemani, 2014). SNPs were employed as valid IVs in the MR study to assess the bidirectional causal relationship between the exposure and the outcome. The comprehensive flowchart for this MR study is illustrated in Figure 1.
Instrumental variables (IVs) for investigating the correlation between human genetic variants and the composition of the gut microbiome were identified from a GWAS dataset within the global collaborative project MiBioGen (Kurilshikov et al., 2021). This extensive, multi-ethnic GWAS involved the coordination of 16S ribosomal RNA gene sequencing profiles and genotyping data from 18,340 participants. A total of 211 taxa, comprising 131 genera, 35 families, 20 orders, 16 classes, and 9 phyla, were encompassed in the analysis (Kurilshikov et al., 2021; Cui et al., 2023). The dataset for AGA included 201,214 European participants sourced from the freely accessible website FinnGen biobank.1 The specific has been placed in the Supplementary Table 4.
We implemented a set of criteria to carefully select eligible genetic IVs:(1) Significance Threshold: Due to the limited number of IVs meeting the genome-wide significance threshold (p < 5 × 10–8) (Kurilshikov et al., 2021; Herbert et al., 2022), we opted for a relatively less stringent threshold (p < 1 × 10–5) based on previous research (Cui et al., 2023; Dai et al., 2023; Liu et al., 2023; Xiao et al., 2023). This less stringent threshold was selected to identify potential sets of variants that are likely to be enriched for association, allowing for a more comprehensive assessment and exploration of results. (2) Clumping Procedure: A clumping procedure was executed (R2 < 0.001, clumping distance = 10,000 kb) to eliminate variants in strong linkage disequilibrium (LD). This step ensured the independence of each SNP in our selection. (3) To mitigate bias stemming from weak IVs, it was deemed necessary to ensure that the F-statistic surpassed a threshold of 10. This threshold helps assess the strength of the instruments and provides confidence in the instrumental variable analysis results (Ardissino et al., 2024).
Clinical manifestations vary between genders. Males experience frontotemporal recession and vertex loss, while females retain the frontal hairline, with hair loss more uniformly across the frontal region post-hairline. Gender-atypical patterns can occur. Assessment of AGA commonly employs the Hamilton-Norwood scale (12 degrees), and the Ludwig scale (3 stages). Diagnostic criteria include miniaturized follicles (hair diameter <0.03 mm), decreased terminal and anagen hairs, increased vellus and telogen hairs, and perifollicular lymphohistiocytic inflammatory infiltrate around the infundibulum (Alessandrini et al., 2021).
We utilized five extensively employed Mendelian Randomization (MR) techniques to identify bidirectional causal connections between exposure and outcome. These methods include inverse variance weighting (IVW), weighted median, MR-Egger, weighted mode, and simple mode (Bowden et al., 2016; Hartwig et al., 2017; Liu et al., 2023). The IVW method calculates the causal impact of the exposure on the outcome by aggregating ratio estimates for each SNP. It was selected as the primary method due to its capacity to offer a robust and unbiased causal effect, especially in scenarios where no polymorphism or heterogeneity is identified (Mounier and Kutalik, 2023). A positive causal effect was confirmed if the IVW results were significant (p < 0.05), and the beta values from other methods were consistent in direction. Subsequently, we proceeded to visually represent the outcomes derived from the five Mendelian Randomization (MR) methods. The bidirectional causal effect was quantified as an odds ratio (OR) computed through MR analysis.
To enhance the robustness of our analysis regarding causality, we employed the Bonferroni’s method to establish distinct significance thresholds for multiple testing across various taxonomic levels. These thresholds were determined based on the number of bacteria within each taxonomic level. Specifically, we set the thresholds at 1.6 × 10–3 (0.05 divided by 32) for the family level and 4.2 × 10–4 (0.05 divided by 119) for the genus level. P-values falling below these thresholds were considered to indicate nominal significance (p < 0.05), suggesting potential causal effects (Su et al., 2023). This study is reported following the Strengthening the Reporting of Observational Studies in Epidemiology Using Mendelian Randomization guidelines (STROBE-MR) (Skrivankova et al., 2021).
Use some basic sensitivity analyses to validate the results. Cochran’s Q statistic is employed to assess heterogeneity among IVs (Bowden et al., 2018). A significance level of p < 0.05 was deemed indicative of the presence of heterogeneity. MR pleiotropy residuals and outlier analysis (MR-PRESSO) are also used to validate the potential pleiotropy of the selected IVs and the direct effects on outcomes. Subsequently, we apply the leave-one-out method to exclude each SNP from the IVs and use the IVW method to assess whether individual SNPs significantly affect the causal effect. All the aforementioned analyses are conducted using the MR and MR-PRESSO R packages with two-sample MR (Hemani et al., 2018; Verbanck et al., 2018).
We utilized GWAS Catalog2 to investigate whether SNPs were linked to potential risk factors. The factors encompass body mass index, obesity, alcohol consumption and neuropsychiatric diseases (Severi et al., 2003). We excluded SNPs associated with any of these potential confounders at genome-wide significance.
The power calculation for the IVW estimates was conducted using a web tool available at https://shiny.cnsgenomics.com/mRnd/. A further explanation of power calculation can be seen in “binary outcome derivations” in the web tool and the type I error rate (alpha) is set at 0.05 (Brion et al., 2013). Specifically, it was recommended to achieve a statistical power of over 80% for the analysis (Zhong et al., 2023).
In our analysis, 62 SNPs were used as IVs for AGA. The comprehensive characteristics of these IVs pertaining to microbial taxa were summarized in Supplementary Table 1. Notably, all SNPs included in our analysis exhibited F-statistics greater than 19 indicating the robustness of these instruments. Upon reviewing the GWAS Catalog, we observed that only one SNP exhibits pleiotropic effects, primarily linked to smoking. Previous research indicates no significant association between AGA and smoking (Kavadya and Mysore, 2022). Therefore, we exclude the confounded without removing the SNP. MR analysis was conducted to evaluate the potential causal relationship between specific categories of gut microbiota and the occurrence of AGA. There are 6 bacterial taxa had a correlated with AGA. According to the IVW estimate, the genus Olsenella (OR = 1.9663, 95% CI: 1.1245–3.4380, p = 0.0177), genus Ruminococcaceae UCG-004 (OR = 2.9609, 95% CI: 1.1504–7.6217, p = 0.0244), and genus Ruminococcaceae UCG-010 (OR = 3.9630, 95% CI: 1.1047–14.2160, p = 0.0346) were identified as risk factors, (Figure 2) suggesting a potential association with decreased hair growth function. On the other hand, the family Acidaminococcaceae (OR = 0.2105, 95% CI: 0.0696–0.6367, p = 0.0058) and genus Anaerofilum (OR = 0.4633, 95% CI: 0.2356–0.9110, p = 0.0257), along with the genus Ruminiclostridium 9 (OR = 0.2459, 95% CI: 0.0608–0.9955, p = 0.0493), demonstrated a protective effect, (Figure 2) implying a potential association with a reduced risk of AGA. Additionally, the estimates of causal effects obtained from the weighted median, MR-Egger, weighted mode, and simple mode methods demonstrated magnitudes and directions that were comparable to those derived from the previously mentioned IVW method, as detailed in Supplementary Table 2 and Figure 3. These findings provide insights into the specific gut microbiota components that may play a role in the development of AGA and its potential link to AGA.
Figure 2. The forest plot illustrates the causal effect of gut microbiota on AGA using the IVW method in MR. The error bars in the plot represent the 95% confidence interval of the odds ratio. In the plot, the blue dots represent outcomes related to AGA, while the red dots represent outcomes associated with AGA positivity. OR, odds ratio; MR, Mendelian randomization; IVW, inverse variance weighted.
In the sensitivity analysis, we employed Cochran’s Q statistics with both IVW and MR-Egger methodologies to evaluate heterogeneity. The results indicated no significant heterogeneity among the IVs, as evidenced by all p-values > 0.05 (Supplementary Table 3). Furthermore, both the MR-Egger intercept and the MR-PRESSO global test provided supporting evidence for the absence of statistically significant directional horizontal pleiotropy (all p-values > 0.05, Supplementary Table 3). Additionally, the leave-one-out analysis demonstrated the lack of influential IVs as illustrated in Figure 4.
These sensitivity analyses, encompassing Cochran’s Q statistics, MR-Egger intercept, MR-PRESSO global test, and leave-one-out analysis, collectively underscored the robustness of the Mendelian Randomization findings in the two-sample analysis. Furthermore, the presentation of the funnel plot and forest plots indicating the reliability of the results (see Supplementary Figures 1, 2).
Androgenetic alopecia is characterized by a progressive thinning of hair in specific areas, influenced by genetic and hormonal factors (Kwack et al., 2023). Prior research has hinted at a connection between AGA and the microbiome (Jung et al., 2022), but much remains unknown about the genetic factors driving this relationship. We employed a bidirectional two-sample MR analytical method, drawing data from GWAS databases encompassing both gut microbiota and AGA. Our inquiry delved into the intricate action of gut microbiota on AGA, unearthing significant insights into how gut microbiota influences AGA. Specifically, our findings spotlight the protective role of certain microbial—such as family Acidaminococcaceae, genus Anaerofilum, and genus Ruminiclostridium 9—in AGA. Conversely, genera like genus Olsenella, genus Ruminococcaceae UCG-004, and genus Ruminococcaceae UCG-010 were identified as risk factors associated with AGA.
The human microbiota has evolved in tandem with its host and serves as an essential component of the human body. Acquired at birth, the microbiota matures alongside the host and maintains significance throughout life, impacting various bodily functions from infancy to old age (Adak and Khan, 2019). Earlier investigations have indicated that in individuals with AGA, both the scalp and gut microbiomes exhibit greater complexity and density, as reflected by elevated values of network topological statistics such as degree centrality, vertices, and edges (Jung et al., 2022). Two patients with alopecia universalis experienced hair regrowth after undergoing fecal microbiota transplantation as a treatment for recurrent Clostridioides difficile infections (Rebello et al., 2017). Imbalanced gut microbiota, notably the proliferation of Lactobacillus murinus, followed by biotin deficiency, constitutes crucial factors contributing to the onset of alopecia (Hayashi et al., 2017). The transplantation of fecal microbiota or the use of a specific probiotic (Bifidobacterium longum HK003) obtained from the feces of healthy individuals could potentially stimulate hair regrowth (Lam et al., 2022). Concerning Ruminiclostridium 9, it has demonstrated its regulatory effects on lipid metabolism, inflammation reduction, enhancement of intestinal barrier function, weight gain reduction, and improved insulin sensitivity in mice, effectively countering obesity development (Wang et al., 2020). However, its specific role in AGA remains unstudied. Further research on the effect of the family Acidaminococcaceae, genus Anaerofilum, and genus Ruminiclostridium 9 on AGA may guide targeted probiotic applications. Our findings suggest that modulating the gut microbiota through probiotics could offer new avenues for the prevention and treatment of AGA.
Ruminococcaceae is a family of bacteria that belongs to the Bacillota (formerly known as Firmicutes) which is one of the major bacterial phyla observed in the human microbiome. Ruminococcaceae is known to be a significant component of the gut microbiota and plays important roles in various physiological processes (Hou et al., 2022). Ruminococcaceae were good predictors of folliculitis decalvans (Moreno-Arrones et al., 2023). Patients undergoing finasteride treatment exhibited a reduction in Ruminococcaceae levels compared to a healthy control group (Diviccaro et al., 2019; Borgo et al., 2021). The You-gui pill could increasing Ruminococcaceae UCG-007 and Ruminococcaceae UCG-010 in the intestine, thereby aiding in the treatment of kidney-yang deficiency syndrome (Chen et al., 2019). In our research, we identified that genus Ruminococcaceae UCG004 (with a statistical power of 98%) and genus Ruminococcaceae UCG010 (with a statistical power of 100%) are implicated as risk factors in association with AGA. The power levels exceeding 80% for both underscore the reliability of the involvement of genus Ruminococcaceae UCG004 and genus Ruminococcaceae UCG010 in AGA. Additional investigation is required to delve into the role of Ruminococcaceae in AGA.
The gut microbiota comprises a unique blend of various organisms, including bacteria, viruses, archaea, protozoa, and fungi, which interact bidirectionally with the central nervous system, forming what is known as the microbiome-gut-brain axis (Mayer, 2011; Sharon et al., 2016). This axis encompasses immune, neural, endocrine, and metabolic pathways, with steroid hormones playing a significant role. Thus far, the steroidogenic capacity remains incompletely understood. However, microbial species such as Clostridium scindens have the potential to convert glucocorticoids into androgens (Ridlon et al., 2013; Ferreira-Halder et al., 2017), thereby fostering the development of AGA. Additionally, Faecalibacterium spp. is a notable producer of butyrate (Ferreira-Halder et al., 2017), a short-chain fatty acid that plays a crucial role in gut microbiota-brain communication (Dalile et al., 2019) and has recently been proposed to influence sleep modulation (Szentirmai et al., 2019). Consequently, Faecalibacterium spp. may be a potential target for therapy AGA. Interestingly, certain members of the human microbiota (e.g., Bifidobacterium spp. and Lactobacillus spp.) encode genes involved in GABA production, suggesting microbial involvement in the production of this neurotransmitter within the gut (Barrett et al., 2012).
The precise biological mechanisms by which microbiotas influence AGA development remain unclear. The microbiota plays a major role in the endocrine system by interacting with estrogen, androgens, insulin, and other hormones (Qi et al., 2021). The androgens are a critical factor that leads to the gradual conversion of terminal hairs into intermediate and vellus hairs, resulting in the gradual hair thinning and hair loss seen in patients with AGA (Lolli et al., 2017). Different types of gut microbiota can produce enzymes essential for androgen metabolism, facilitating the synthesis and alteration of androgens. The microbial-mediated degradation of testosterone has been witnessed across various environmental contexts. For instance, Actinobacteria and Proteobacteria possess the capability to break down androgens (Qi et al., 2021). Additionally, Clostridium scindens, harboring the 20α-hydroxysteroid dehydrogenase (HSDH) enzyme in its genome, is a human gut microbe with significant potential to convert glucocorticoids into androgens (Ridlon et al., 2013). Meanwhile, microbiota-host communication is primarily facilitated through secreted exosomes (Li Q. et al., 2019). Exosomes, extracellular vesicles involved in cell communication, homeostasis, differentiation, and organogenesis (Li et al., 2018, 2021; Lai et al., 2022), are pivotal in hair morphogenesis and regeneration, holding potential for alopecia treatment (Hu et al., 2020). Plasma exosomes, originating from host cells or gut microbiota, mediate local or remote mutual regulation (Li Y. et al., 2019; Li et al., 2020). Intriguingly, exosomes have been observed carrying Wnt proteins on their surface, inducing β-catenin activation—a crucial signaling pathway in hair morphogenesis and regeneration (Gross et al., 2012). Exosomes from adipose-derived stem cells positively impact hair regrowth by enhancing DPC proliferation via upregulating the Wnt/β-catenin, TNF-α signaling pathways, and vascular endothelial growth factor expression (Hu et al., 2020). Further investigation is required to explore the functions of the six microbiotas mentioned in our article on the composition and decomposition of androgens. Elucidating the potential biological mechanisms at microbiotas that might influence AGA development is crucial.
Following Bonferroni’s correction, no distinct causal relationship between gut flora, metabolites, and the risk factors associated with heart failure was identified. This implies that further investigations are essential to validate and confirm the potential relationships between these elements. The recommended threshold for statistical power is over 80%. Notably, the power values for genus Olsenella (93%), genus Ruminococcaceae UCG004 (98%), and genus Ruminococcaceae UCG010 (100%) all exceed 80%, thereby reinforcing the validity of our findings. However, it is noteworthy that the overall power calculated by our research institute is not entirely satisfactory. The power values for family Acidaminococcaceae (29%), genus Anaerofilum (41%), and genus Ruminiclostridium 9 (31%) fall below the 80% threshold. This discrepancy might be attributed to the relatively small rate of cases/controls (195/201,019) in the GWAS data for AGA. To enhance the statistical power and obtain more accurate results in future studies, it is advisable to consider increasing the sample size of cases.
The systemic androgens and genetic factors are the primary causes of AGA, various external or exogenous factors also play a role in its development (Choi et al., 2017). Limited further studies due to inadequate summary data for various alopecia types, lack of stratification statistics for AGA degree, and absence of sex-based stratification data. Several limitations of our study exist. In conclusion, the intricate physiological mechanisms underlying the relationship between gut microbiota and AGA extend beyond the scope of our simplistic models. Subsequent research efforts should focus on identifying potential mechanisms to deepen our understanding of AGA for preventive measures.
Our study pioneered a two-sample MR analysis using GWAS summary statistics to probe a potential causal connection between gut microbiota and AGA. This analytical approach not only holds promise for developing effective prevention and intervention strategies for AGA but also offers innovative insights into the underlying mechanisms of AGA through the lens of gut microbiota.
The original contributions presented in this study are included in the article/Supplementary material, further inquiries can be directed to the corresponding authors.
HF: Conceptualization, Data curation, Investigation, Methodology, Project administration, Software, Validation, Visualization, Writing – original draft, Writing – review and editing. TX: Methodology, Writing – original draft. WZ: Methodology, Writing – original draft. LJ: Funding acquisition, Resources, Software, Writing – review and editing. SS: Funding acquisition, Resources, Supervision, Writing – review and editing.
The author(s) declare financial support was received for the research, authorship, and/or publication of this article. This work is supported by the Natural Science Foundation Committee of China (Grant No. 81972953), Fund for Less Developed Regions of the National Natural Science Foundation of China (Grant No. 82160601), Guizhou Provincial Health Commission Science and Technology Fund Program (Grant No. 2021XMSB00029829), and Guizhou Provincial Science and Technology Projects, ZK (2022) general 250.
We would like to thank the MiBioGen and FinnGen consortium for making summary level data from this consortium publicly available.
The authors declare that the research was conducted in the absence of any commercial or financial relationships that could be construed as a potential conflict of interest.
All claims expressed in this article are solely those of the authors and do not necessarily represent those of their affiliated organizations, or those of the publisher, the editors and the reviewers. Any product that may be evaluated in this article, or claim that may be made by its manufacturer, is not guaranteed or endorsed by the publisher.
The Supplementary Material for this article can be found online at: https://www.frontiersin.org/articles/10.3389/fmicb.2024.1360445/full#supplementary-material
Adak, A., and Khan, M. (2019). An insight into gut microbiota and its functionalities. Cell Mol. Life Sci. 76, 473–493. doi: 10.1007/s00018-018-2943-4
Alessandrini, A., Bruni, F., Piraccini, B., and Starace, M. (2021). Common causes of hair loss - clinical manifestations, trichoscopy and therapy. J. Eur. Acad. Dermatol. Venereol. 35, 629–640. doi: 10.1111/jdv.17079
Ardissino, M., Morley, A., Slob, E., Schuermans, A., Rayes, B., Raisi-Estabragh, Z., et al. (2024). Birth weight influences cardiac structure, function, and disease risk: Evidence of a causal association. Eur. Heart J. 45, 443–454. doi: 10.1093/eurheartj/ehad631
Barrett, E., Ross, R., O’Toole, P., Fitzgerald, G., and Stanton, C. (2012). γ-Aminobutyric acid production by culturable bacteria from the human intestine. J. Appl. Microbiol. 113, 411–417. doi: 10.1111/j.1365-2672.2012.05344.x
Birney, E. (2022). Mendelian randomization. Cold Spring Harb. Perspect. Med. 12:a041302. doi: 10.1101/cshperspect.a041302
Borgo, F., Macandog, A., Diviccaro, S., Falvo, E., Giatti, S., Cavaletti, G., et al. (2021). Alterations of gut microbiota composition in post-finasteride patients: A pilot study. J. Endocrinol. Invest. 44, 1263–1273. doi: 10.1007/s40618-020-01424-0
Bowden, J., Davey Smith, G., Haycock, P., and Burgess, S. (2016). Consistent estimation in mendelian randomization with some invalid instruments using a weighted median estimator. Genet. Epidemiol. 40, 304–314. doi: 10.1002/gepi.21965
Bowden, J., Spiller, W., Del Greco, M. F., Sheehan, N., Thompson, J., Minelli, C., et al. (2018). Improving the visualization, interpretation and analysis of two-sample summary data Mendelian randomization via the Radial plot and Radial regression. Int. J. Epidemiol. 47, 1264–1278. doi: 10.1093/ije/dyy101
Brion, M., Shakhbazov, K., and Visscher, P. (2013). Calculating statistical power in Mendelian randomization studies. Int. J. Epidemiol. 42, 1497–1501. doi: 10.1093/ije/dyt179
Chen, R., Wang, J., Zhan, R., Zhang, L., and Wang, X. (2019). Fecal metabonomics combined with 16S rRNA gene sequencing to analyze the changes of gut microbiota in rats with kidney-yang deficiency syndrome and the intervention effect of You-gui pill. J. Ethnopharmacol. 244:112139. doi: 10.1016/j.jep.2019.112139
Choi, J., Jun, M., Lee, S., Oh, S., and Lee, W. (2017). The association between exercise and androgenetic alopecia: A survey-based study. Ann. Dermatol. 29, 513–516. doi: 10.5021/ad.2017.29.4.513
Cui, G., Li, S., Ye, H., Yang, Y., Jia, X., Lin, M., et al. (2023). Gut microbiome and frailty: Insight from genetic correlation and mendelian randomization. Gut Microbes. 15:2282795. doi: 10.1080/19490976.2023.2282795
Dai, H., Hou, T., Wang, Q., Hou, Y., Zhu, Z., Zhu, Y., et al. (2023). Roles of gut microbiota in atrial fibrillation: Insights from Mendelian randomization analysis and genetic data from over 430,000 cohort study participants. Cardiovasc. Diabetol. 22:306. doi: 10.1186/s12933-023-02045-6
Dalile, B., Van Oudenhove, L., Vervliet, B., and Verbeke, K. (2019). The role of short-chain fatty acids in microbiota-gut-brain communication. Nat. Rev. Gastroenterol. Hepatol. 16, 461–478. doi: 10.1038/s41575-019-0157-3
Davey Smith, G., and Hemani, G. (2014). Mendelian randomization: Genetic anchors for causal inference in epidemiological studies. Hum. Mol. Genet. 23, R89–R98. doi: 10.1093/hmg/ddu328
Devjani, S., Ezemma, O., Kelley, K. J., Stratton, E., and Senna, M. (2023). Androgenetic alopecia: Therapy update. Drugs 83, 701–715.
Diviccaro, S., Giatti, S., Borgo, F., Barcella, M., Borghi, E., Trejo, J., et al. (2019). Treatment of male rats with finasteride, an inhibitor of 5alpha-reductase enzyme, induces long-lasting effects on depressive-like behavior, hippocampal neurogenesis, neuroinflammation and gut microbiota composition. Psychoneuroendocrinology 99, 206–215. doi: 10.1016/j.psyneuen.2018.09.021
Evans, D., and Davey Smith, G. (2015). Mendelian randomization: New applications in the coming age of hypothesis-free causality. Annu. Rev. Genomics Hum. Genet. 16, 327–350. doi: 10.1146/annurev-genom-090314-050016
Ferreira-Halder, C., Faria, A., and Andrade, S. (2017). Action and function of Faecalibacterium prausnitzii in health and disease. Best Pract. Res. Clin. Gastroenterol. 31, 643–648. doi: 10.1016/j.bpg.2017.09.011
Gross, J., Chaudhary, V., Bartscherer, K., and Boutros, M. (2012). Active Wnt proteins are secreted on exosomes. Nat. Cell Biol. 14, 1036–1045. doi: 10.1038/ncb2574
Hartwig, F., Davey Smith, G., and Bowden, J. (2017). Robust inference in summary data Mendelian randomization via the zero modal pleiotropy assumption. Int. J. Epidemiol. 46, 1985–1998. doi: 10.1093/ije/dyx102
Hayashi, A., Mikami, Y., Miyamoto, K., Kamada, N., Sato, T., Mizuno, S., et al. (2017). Intestinal dysbiosis and biotin deprivation induce alopecia through overgrowth of Lactobacillus murinus in Mice. Cell Rep. 20, 1513–1524. doi: 10.1016/j.celrep.2017.07.057
Hemani, G., Zheng, J., Elsworth, B., Wade, K., Haberland, V., Baird, D., et al. (2018). The MR-Base platform supports systematic causal inference across the human phenome. Elife 7:e34408. doi: 10.7554/eLife.34408
Herbert, A., Heron, J., Barnes, M., Barter, C., Feder, G., Meghrawi, K., et al. (2022). Exploring the causal role of intimate partner violence and abuse on depressive symptoms in young adults: A population-based cohort study. BMC Med. 20:1. doi: 10.1186/s12916-021-02182-3
Ho, B., Ho, E., Chu, C., Ramasamy, S., Bigliardi-Qi, M., de Sessions, P., et al. (2019). Microbiome in the hair follicle of androgenetic alopecia patients. PLoS One 14:e0216330. doi: 10.1371/journal.pone.0216330
Hou, K., Wu, Z., Chen, X., Wang, J., Zhang, D., Xiao, C., et al. (2022). Microbiota in health and diseases. Signal Transduct. Target Ther. 7:135. doi: 10.1038/s41392-022-00974-4
Hu, S., Li, Z., Lutz, H., Huang, K., Su, T., Cores, J., et al. (2020). Dermal exosomes containing miR-218-5p promote hair regeneration by regulating β-catenin signaling. Sci. Adv. 6:eaba1685. doi: 10.1126/sciadv.aba1685
Jung, D., Yoo, H., Kim, M., Singh, V., Park, S., Jeong, M., et al. (2022). Comparative analysis of scalp and gut microbiome in androgenetic alopecia: A Korean cross-sectional study. Front. Microbiol. 13:1076242. doi: 10.3389/fmicb.2022.1076242
Kavadya, Y., and Mysore, V. (2022). Role of smoking in androgenetic alopecia: A systematic review. Int. J. Trichol. 14, 41–48. doi: 10.4103/ijt.ijt_59_21
Kurilshikov, A., Medina-Gomez, C., Bacigalupe, R., Radjabzadeh, D., Wang, J., Demirkan, A., et al. (2021). Large-scale association analyses identify host factors influencing human gut microbiome composition. Nat. Genet. 53, 156–165. doi: 10.1038/s41588-020-00763-1
Kwack, M., Hamida, O., Kim, M., Kim, M., and Sung, Y. (2023). Establishment and characterization of matched immortalized human frontal and occipital scalp dermal papilla cell lines from androgenetic alopecia. Sci. Rep. 13:21421. doi: 10.1038/s41598-023-48942-4
Lai, C., Chu, N., Chang, C., Wang, S., Yang, H., Chu, C., et al. (2013). Androgenic alopecia is associated with less dietary soy, lower [corrected] blood vanadium and rs1160312 1 polymorphism in Taiwanese communities. PLoS One 8:e79789. doi: 10.1371/journal.pone.0079789
Lai, H., Li, Y., Zhang, H., Hu, J., Liao, J., Su, Y., et al. (2022). exoRBase 2.0: An atlas of mRNA, lncRNA and circRNA in extracellular vesicles from human biofluids. Nucleic Acids Res. 50, D118–D128. doi: 10.1093/nar/gkab1085
Lam, S., Zhang, J., Yang, K., Chu, L., Zhu, W., Tang, W., et al. (2022). Modulation of gut microbiota impacts diet-induced and drug-induced alopecia in mice. Gut 71, 2366–2369. doi: 10.1136/gutjnl-2021-326320
Lee, H., Ha, S., Kim, D., Kim, H., and Kim, J. (2002). Perception of men with androgenetic alopecia by women and nonbalding men in Korea: How the nonbald regard the bald. Int. J. Dermatol. 41, 867–869. doi: 10.1046/j.1365-4362.2002.01446.x
Li, Q., Ren, Y., and Fu, X. (2019). Inter-kingdom signaling between gut microbiota and their host. Cell Mol. Life Sci. 76, 2383–2389. doi: 10.1007/s00018-019-03076-7
Li, S., Li, Y., Chen, B., Zhao, J., Yu, S., Tang, Y., et al. (2018). exoRBase: A database of circRNA, lncRNA and mRNA in human blood exosomes. Nucleic Acids Res. 46, D106–D112. doi: 10.1093/nar/gkx891
Li, Y., He, X., Li, Q., Lai, H., Zhang, H., Hu, Z., et al. (2020). EV-origin: Enumerating the tissue-cellular origin of circulating extracellular vesicles using exLR profile. Comput. Struct. Biotechnol. J. 18, 2851–2859. doi: 10.1016/j.csbj.2020.10.002
Li, Y., Li, Y., Yu, S., Qian, L., Chen, K., Lai, H., et al. (2021). Circulating EVs long RNA-based subtyping and deconvolution enable prediction of immunogenic signatures and clinical outcome for PDAC. Mol. Ther. Nucleic Acids 26, 488–501. doi: 10.1016/j.omtn.2021.08.017
Li, Y., Zhao, J., Yu, S., Wang, Z., He, X., Su, Y., et al. (2019). Extracellular vesicles long RNA sequencing reveals abundant mRNA, circRNA, and lncRNA in human blood as potential biomarkers for cancer diagnosis. Clin. Chem. 65, 798–808. doi: 10.1373/clinchem.2018.301291
Liu, X., Liu, J., Zhang, T., Wang, Q., and Zhang, H. (2023). Complex relationship between gut microbiota and thyroid dysfunction: A bidirectional two-sample Mendelian randomization study. Front. Endocrinol. 14:1267383. doi: 10.3389/fendo.2023.1267383
Lolli, F., Pallotti, F., Rossi, A., Fortuna, M., Caro, G., Lenzi, A., et al. (2017). Androgenetic alopecia: A review. Endocrine 57, 9–17. doi: 10.1007/s12020-017-1280-y
Marks, D., Penzi, L., Ibler, E., Manatis-Lornell, A., Hagigeorges, D., Yasuda, M., et al. (2019). The medical and psychosocial associations of alopecia: Recognizing hair loss as more than a cosmetic concern. Am. J. Clin. Dermatol. 20, 195–200. doi: 10.1007/s40257-018-0405-2
Mayer, E. (2011). Gut feelings: The emerging biology of gut-brain communication. Nat. Rev. Neurosci. 12, 453–466. doi: 10.1038/nrn3071
Moreno-Arrones, O., Garcia-Hoz, C., Del Campo, R., Roy, G., Saceda-Corralo, D., Jimenez-Cauhe, J., et al. (2023). Folliculitis decalvans has a heterogeneous microbiological signature and impaired immunological response. Dermatology 239, 454–461. doi: 10.1159/000529301
Mounier, N., and Kutalik, Z. (2023). Bias correction for inverse variance weighting Mendelian randomization. Genet Epidemiol. 47, 314–331. doi: 10.1002/gepi.22522
Phillips, T., Slomiany, W., and Allison, R. (2017). Hair loss: Common causes and treatment. Am. Fam. Phys. 96, 371–378.
Qi, X., Yun, C., Pang, Y., and Qiao, J. (2021). The impact of the gut microbiota on the reproductive and metabolic endocrine system. Gut Microbes 13, 1–21. doi: 10.1080/19490976.2021.1894070
Rebello, D., Wang, E., Yen, E., Lio, P., and Kelly, C. (2017). Hair growth in two alopecia patients after fecal microbiota transplant. ACG Case Rep. J. 4:e107.
Ridlon, J., Ikegawa, S., Alves, J., Zhou, B., Kobayashi, A., Iida, T., et al. (2013). Clostridium scindens: A human gut microbe with a high potential to convert glucocorticoids into androgens. J. Lipid Res. 54, 2437–2449. doi: 10.1194/jlr.M038869
Severi, G., Sinclair, R., Hopper, J., English, D., McCredie, M., Boyle, P., et al. (2003). Androgenetic alopecia in men aged 40-69 years: Prevalence and risk factors. Br. J. Dermatol. 149, 1207–1213. doi: 10.1111/j.1365-2133.2003.05565.x
Sharon, G., Sampson, T., Geschwind, D., and Mazmanian, S. (2016). The central nervous system and the gut microbiome. Cell 167, 915–932. doi: 10.1016/j.cell.2016.10.027
Skrivankova, V., Richmond, R., Woolf, B., Davies, N., Swanson, S., VanderWeele, T., et al. (2021). Strengthening the reporting of observational studies in epidemiology using mendelian randomisation (STROBE-MR): Explanation and elaboration. BMJ 375:n2233. doi: 10.1136/bmj.n2233
Su, Q., Jin, C., Bo, Z., Yang, Y., Wang, J., Wang, J., et al. (2023). Association between gut microbiota and gastrointestinal cancer: A two-sample bi-directional Mendelian randomization study. Front. Microbiol. 14:1181328. doi: 10.3389/fmicb.2023.1181328
Suzuki, K., Inoue, M., Cho, O., Mizutani, R., Shimizu, Y., Nagahama, T., et al. (2021). Scalp microbiome and sebum composition in Japanese male individuals with and without androgenetic alopecia. Microorganisms 9:2132. doi: 10.3390/microorganisms9102132
Szentirmai, É, Millican, N., Massie, A., and Kapás, L. (2019). Butyrate, a metabolite of intestinal bacteria, enhances sleep. Sci. Rep. 9:7035.
Trüeb, R. (2002). Molecular mechanisms of androgenetic alopecia. Exp. Gerontol. 37, 981–990. doi: 10.1016/s0531-5565(02)00093-1
Verbanck, M., Chen, C., Neale, B., and Do, R. (2018). Detection of widespread horizontal pleiotropy in causal relationships inferred from Mendelian randomization between complex traits and diseases. Nat. Genet. 50, 693–698. doi: 10.1038/s41588-018-0099-7
Wang, M., Cordell, H., and Van Steen, K. (2019). Statistical methods for genome-wide association studies. Semin Cancer Biol. 55, 53–60. doi: 10.1016/j.semcancer.2018.04.008
Wang, P., Li, D., Ke, W., Liang, D., Hu, X., and Chen, F. (2020). Resveratrol-induced gut microbiota reduces obesity in high-fat diet-fed mice. Int. J. Obes. 44, 213–225. doi: 10.1038/s41366-019-0332-1
Xiao, L., Liu, S., Wu, Y., Huang, Y., Tao, S., Liu, Y., et al. (2023). The interactions between host genome and gut microbiome increase the risk of psychiatric disorders: Mendelian randomization and biological annotation. Brain Behav. Immun. 113, 389–400. doi: 10.1016/j.bbi.2023.08.003
Yeo, I., Jang, W., Min, P., Cho, H., Cho, S., Hong, N., et al. (2014). An epidemiological study of androgenic alopecia in 3114 Korean patients. Clin. Exp. Dermatol. 39, 25–29. doi: 10.1111/ced.12229
Keywords: androgenetic alopecia, causal relationship, genetic, gut microbiota, Mendelian randomization
Citation: Fu H, Xu T, Zhao W, Jiang L and Shan S (2024) Roles of gut microbiota in androgenetic alopecia: insights from Mendelian randomization analysis. Front. Microbiol. 15:1360445. doi: 10.3389/fmicb.2024.1360445
Received: 23 December 2023; Accepted: 13 March 2024;
Published: 02 April 2024.
Edited by:
Qi Zhao, University of Science and Technology Liaoning, ChinaReviewed by:
Chi-Yang Chiu, The University of Tennessee Health Science Center (UTHSC), United StatesCopyright © 2024 Fu, Xu, Zhao, Jiang and Shan. This is an open-access article distributed under the terms of the Creative Commons Attribution License (CC BY). The use, distribution or reproduction in other forums is permitted, provided the original author(s) and the copyright owner(s) are credited and that the original publication in this journal is cited, in accordance with accepted academic practice. No use, distribution or reproduction is permitted which does not comply with these terms.
*Correspondence: Shijun Shan, c2hhbnNoaWp1bjIwMjNAMTYzLmNvbQ==; Leiwei Jiang, bGVpd2VpamlhbmdAMTYzLmNvbQ==
Disclaimer: All claims expressed in this article are solely those of the authors and do not necessarily represent those of their affiliated organizations, or those of the publisher, the editors and the reviewers. Any product that may be evaluated in this article or claim that may be made by its manufacturer is not guaranteed or endorsed by the publisher.
Research integrity at Frontiers
Learn more about the work of our research integrity team to safeguard the quality of each article we publish.