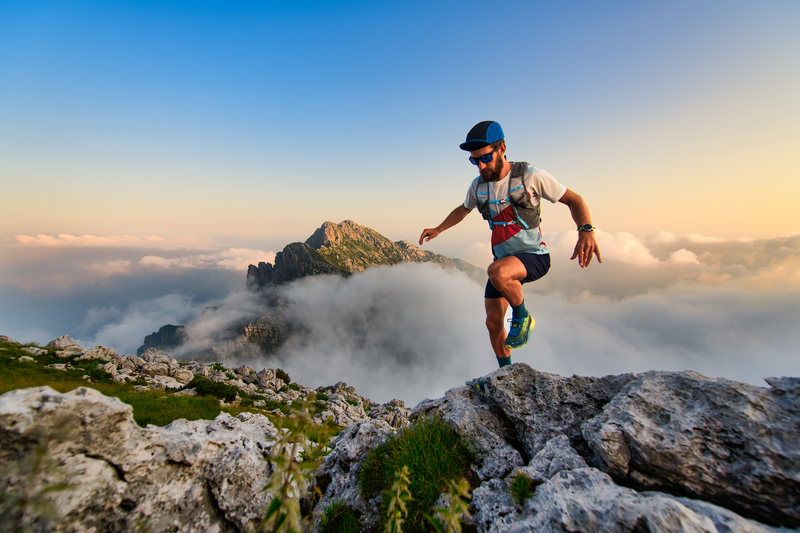
94% of researchers rate our articles as excellent or good
Learn more about the work of our research integrity team to safeguard the quality of each article we publish.
Find out more
ORIGINAL RESEARCH article
Front. Microbiol. , 03 April 2024
Sec. Microorganisms in Vertebrate Digestive Systems
Volume 15 - 2024 | https://doi.org/10.3389/fmicb.2024.1356478
This article is part of the Research Topic Untangle the Broad Connections and Tight Interactions Between Human Microbiota and Complex Diseases Through Data-Driven Approaches, Volume II View all 32 articles
Background: Observational studies and some experimental investigations have indicated that gut microbiota are closely associated with the incidence and progression of chronic renal failure. However, the causal relationship between gut microbiota and chronic renal failure remains unclear. The present study employs a two-sample Mendelian randomization approach to infer the causal relationship between gut microbiota and chronic renal failure at the genetic level. This research aims to determine whether there is a causal effect of gut microbiota on the risk of chronic renal failure, aiming to provide new evidence to support targeted gut therapy for the treatment of chronic renal failure.
Methods: Employing genome-wide association study (GWAS) data from the public MiBioGen and IEU OpenGWAS platform, a two-sample Mendelian randomization analysis was conducted. The causal relationship between gut microbiota and chronic renal failure was inferred using five different methods: Inverse Variance Weighted, MR-Egger, Weighted Median, Simple Mode, and Weighted Mode. The study incorporated sensitivity analyses that encompassed evaluations for pleiotropy and heterogeneity. Subsequently, the results of the Mendelian randomization analysis underwent a stringent correction for multiple testing, employing the False Discovery Rate method to enhance the validity of our findings.
Results: According to the results from the Inverse Variance Weighted method, seven bacterial genera show a significant association with the outcome variable chronic renal failure. Of these, Ruminococcus (gauvreauii group) (OR = 0.82, 95% CI = 0.71–0.94, p = 0.004) may act as a protective factor against chronic renal failure, while the genera Escherichia-Shigella (OR = 1.22, 95% CI = 1.08–1.38, p = 0.001), Lactococcus (OR = 1.1, 95% CI = 1.02–1.19, p = 0.013), Odoribacter (OR = 1.23, 95% CI = 1.03–1.49, p = 0.026), Enterorhabdus (OR = 1.14, 95% CI = 1.00–1.29, p = 0.047), Eubacterium (eligens group) (OR = 1.18, 95% CI = 1.02–1.37, p = 0.024), and Howardella (OR = 1.18, 95% CI = 1.09–1.28, p < 0.001) may be risk factors for chronic renal failure. However, after correction for multiple comparisons using False Discovery Rate, only the associations with Escherichia-Shigella and Howardella remain significant, indicating that the other genera have suggestive associations. Sensitivity analyses did not reveal any pleiotropy or heterogeneity.
Conclusion: Our two-sample Mendelian randomization study suggests that the genera Escherichia-Shigella and Howardella are risk factors for chronic renal failure, and they may serve as potential targets for future therapeutic interventions. However, the exact mechanisms of action are not yet clear, necessitating further research to elucidate their precise roles fully.
Chronic renal failure (CRF) refers to a state in which the kidneys gradually lose function and can no longer maintain the normal internal environment of the body. It is the consequence of the progression of various chronic kidney diseases (CKD), with some patients having to wait for renal replacement therapy. The pathogenesis of CRF is relatively complex, as CKD can typically cause chronic inflammation of the renal tubules and interstitium. This inflammatory response leads to kidney damage and promotes fibrosis, ultimately resulting in the deterioration of renal function (Peppa et al., 2004; Fang et al., 2023). Patients with CRF are also affected by oxidative stress; an excess generation of reactive oxygen species causes an imbalance between oxidation and antioxidation in the body, ultimately leading to pathologies such as lipid peroxidation of cell membranes, renal mitochondrial homeostasis disturbance, and tissue damage (Ho and Shirakawa, 2022; Piko et al., 2023). Additionally, the onset of CRF involves genetic susceptibility and epigenetics (Smyth et al., 2014). The 2023 ISN Global Kidney Health Atlas (ISN-GKHA) released by the International Society of Nephrology (ISN) shows that the global median prevalence of CKD is 9.5%, and the global median mortality rate is 2.4%. Due to the high cost of renal disease treatment and its significant impacts on health, the global burden of renal failure remains substantial. However, there are currently no methods to fully control the progression of CRF. For some renal failure patients, particularly those entering end-stage renal disease (ESRD), treatment still relies mainly on dialysis. Given the expense and complexity of CRF treatment, actively exploring new potential intervention measures to delay the progression of the disease is particularly important.
The gut microbiome is not only crucial for intestinal health but also affects the function of numerous other organs in the body. An increasing body of evidence supports the association between the human gut microbiome and extraintestinal organ function, including a close correlation between the gut microbiome and CRF (Lozupone et al., 2012). Studies have indicated that patients with CKD exhibit dysbiosis of the gut microbiota, with an increase in pathogenic bacteria, impaired gut barrier function, increased intestinal permeability, and generation of uremic toxins such as indoles (e.g., indoxyl sulfate), phenols (e.g., p-cresyl sulfate), and amines (e.g., trimethylamine-N-oxide) through the fermentation of undigested products in the colon by the gut microbiota. These uremic toxins and the impairment of gut barrier function are closely related to inflammatory responses and oxidative stress, which further lead to the progression of the disease and the occurrence of related complications (Mafra et al., 2014; Cigarran Guldris et al., 2017). The gut and kidney can be interconnected through a Metabolism-dependent pathway and an Immune pathway, forming the “gut-kidney axis” (Yang et al., 2018). With the proposal of the “gut-kidney axis” concept, targeting the gut for treatment may become an emerging potential therapeutic strategy for CRF (Martínez-Hernández et al., 2023). While some observational studies and experiments suggest that the gut microbiota plays a significant role in the development and progression of CRF (Tian et al., 2022), the specific causal relationship between the gut microbiota and CRF remains unclear, and there is inconsistency in the findings of gut microbiota studies among dialysis patients, nondialysis patients, and those undergoing hemodialysis or peritoneal dialysis (Stadlbauer et al., 2017; Hu J. et al., 2020). Therefore, in this study, a two-sample Mendelian randomization approach was employed from a genetic perspective to clarify the causal relationship between gut microbiota and CRF, providing new evidence to support targeted gut therapy for CRF.
Mendelian randomization (MR) studies are commonly used to assess the causal relationships between exposures or risk factors and outcomes (Sekula et al., 2016). Observational studies are often subject to confounding by extraneous factors, whereas MR uses genetic variants as instrumental variables to infer causal effects of exposures or risk factors on outcomes. According to Mendel’s laws, alleles of genetic variants are randomly allocated and less likely to be confounded by other factors; thus, MR serves as an analytical method that sits between randomized controlled trials and observational studies (Ference et al., 2021). It can circumvent the confounding inherent to observational studies and overcome issues such as the costly nature and practical challenges associated with randomized trials. To date, research integrating gut microbiota with MR has been widely applied, including in areas such as gut microbiota and cancer (Long et al., 2023), gut microbiota and autoimmune diseases (Xu et al., 2021), and gut microbiota and mental disorders (Ni et al., 2021). This study employs MR to infer the causal relationship between the gut microbiota and CRF.
Mendelian randomization analysis must satisfy three critical assumptions: (1) Relevance assumption: the genetic variants chosen as instrumental variables should be associated with the exposure; (2) Independence assumption: the instrumental variables used should not be associated with any confounders; (3) Exclusion-restriction assumption: the instrumental variables must not have a direct effect on the outcome but should only influence the outcome through the exposure. In this study, gut microbiota is considered the exposure, and chronic renal failure is the outcome. Significant single-nucleotide polymorphisms (SNPs) are selected as instrumental variables (IVs). Two-sample Mendelian randomization methods are employed for the causal inference, supplemented by sensitivity analyses such as horizontal pleiotropy and heterogeneity tests. The specific study flowchart can be seen in Figure 1.
The gut microbiota GWAS data are obtained from the MiBioGen consortium data (Kurilshikov et al., 2021).1 Researchers employed high-throughput gene sequencing technologies to analyze the gut microbiota of a large cohort of individuals, classifying the microbiota into respective taxonomic groups based on their genetic information by targeting three different variable regions (V4, V3–V4, and V1–V2) of the 16S rRNA gene. This dataset on gut microbiome comprises 24 cohorts, encompassing a total of 18,340 participants. Predominantly, the study population consisted of Europeans, accounting for more than 70% of the dataset (16 cohorts, N = 13,266). The GWAS data on CRF was sourced from the IEU OpenGWAS platform2 based on the research data by Sakaue et al. (2021). This CRF dataset included 482,858 individuals, with 8,287 cases and 474,571 controls, featuring 24,185,976 SNPs, with the study population being of European descent. These GWAS datasets are largely independent of each other, and all original research data have been ethically approved and consented to by the participants.
In this study, the gut microbiome GWAS data encompassed 211 taxonomic groups, including 131 genera, 35 families, 20 orders, 16 classes, and 9 phyla, with phyla representing the highest taxonomic level and genera being the lowest. The lower the taxonomic rank, the more common characteristics and similarities are shared among the microorganisms. Here, we conducted Mendelian randomization analyses using the genera level of gut microbiota, excluding 12 genera with unknown bacterial names (Kurilshikov et al., 2021), resulting in the inclusion of the remaining 119 genera in the study. The selection of suitable SNPs was based on the following criteria: (1) To ensure a sufficient number of instrumental variables, SNPs significantly associated at p < 1.0 × 10−5 were selected; (2) The chosen SNPs were required not to be in linkage disequilibrium with each other, generally measured by the parameters r2 and distance in kb. In this study, we set r2 < 0.001 and distance at 10,000 kb, thus removing SNPs within a 10,000 kb range that had an r2 > 0.001 with the most significant SNP to reduce the impact of linkage disequilibrium and retaining more independent SNPs; (3) The F-Statistic was used to assess the statistical strength of the association between SNPs and the exposure factor, where an F > 10 suggested a strong relationship with the exposure, and instrumental variables with an F < 10 were excluded. Finally, we harmonized SNPs with the same alleles in the GWAS data for gut microbiota and CRF, removing incompatible and palindromic SNPs to align the effect sizes for the exposure and outcome.
This study primarily utilized the “TwoSampleMR” package within R language (version 4.3.1) for analysis. The causal relationship between gut microbiota and CRF was assessed using five MR methods: Inverse Variance Weighted (IVW), MR-Egger, Weighted Median, Simple Mode, and Weighted Mode. Each statistical method relies on its specific model assumptions, and if these prerequisites are not met, the statistical power may be weakened or even rendered ineffective. The IVW method weights the effects between SNPs and causal traits by the inverse of their variance and combines them to estimate the overall effect. The results of IVW will be unbiased in the absence of horizontal pleiotropy (Burgess et al., 2015). The MR Egger method, based on the Egger regression in MR analyses, tests for horizontal pleiotropy through the MR-Egger regression. A significant intercept in the MR-Egger analysis, with p < 0.05, suggests the presence of horizontal pleiotropy. If the intercept is close to zero, the MR-Egger estimate will be close to the IVW estimate (Burgess and Thompson, 2017). The Weighted Median method can provide consistent estimates even if up to 50% of the SNP instruments are invalid, estimating the causal effect by weighting the effects of different SNPs (Bowden et al., 2016). The Simple Mode is a straightforward method of combining MR results by directly taking the mode of effect size estimates from different studies for a combined result. The Weighted Mode is an improved mode method that adds a step of weighting the effects of different studies on the basis of Simple Mode, calculating the combined result according to each study’s sample size or weight. Under certain conditions, the IVW method is more capable of effectively handling the synthesis of estimates, providing more precise results and demonstrating higher efficacy compared to other statistical methods (Bowden et al., 2016). Therefore, we primarily employed the IVW method for our analysis, with the remaining four methods serving as supplementary approaches. MR-PRESSO was utilized to detect significant outliers among the SNPs, and if the MR-PRESSO global test statistic p < 0.05, it indicates the presence of significant outliers that should be removed before repeating the MR analysis. Cochran’s Q test was applied to assess if there was significant heterogeneity among the SNPs. A statistically significant Cochran’s Q test (p < 0.05) indicates significant heterogeneity in the results, necessitating a discussion of the possible sources of heterogeneity. Leave-one-out sensitivity analysis was conducted, systematically excluding each SNP and calculating the combined effect of the remaining SNPs, to evaluate the impact of any single SNP on the relationship between exposure and outcome. Finally, the risk association between gut microbiota and CRF was expressed in terms of the odds ratio (OR) and its 95% confidence interval (CI), where p < 0.05 provided evidence for a potential causal relationship. Additionally, as the p-value threshold for SNP selection was set at p < 1.0 × 10−5, not meeting the conventional GWAS significance threshold of p < 1.0 × 10−8, False Discovery Rate (FDR) correction was applied for multiple testing to minimize the likelihood of type I statistical errors, setting the threshold for the FDR-adjusted q_value at 0.1. If p < 0.05 and q_value < 0.1, this suggests a significant association; if p < 0.05 but q_value > 0.1, it indicates an association of suggestive significance (Storey and Tibshirani, 2003).
Based on the selection criteria for instrumental variables, a total of 1,480 SNPs were ultimately included as instrumental variables for MR analysis; all SNPs had an F-value greater than 10, and details can be found in Supplementary Table 1. MR analysis revealed that among the gut microbiota of 119 bacterial genera, according to the results of the IVW method (p < 0.05), 7 genera were closely related to the outcome variable CRF. Among these, Ruminococcus (gauvreauii group) (OR = 0.82, 95% CI = 0.71–0.94, p = 0.004) may be a protective factor for CRF. In contrast, Escherichia-Shigella (OR = 1.22, 95% CI = 1.08–1.38, p = 0.001), Lactococcus (OR = 1.1, 95% CI = 1.02–1.19, p = 0.013), Odoribacter (OR = 1.23, 95% CI = 1.03–1.49, p = 0.026), Enterorhabdus (OR = 1.14, 95% CI = 1.00–1.29, p = 0.047), Eubacterium (eligens group) (OR = 1.18, 95% CI = 1.02–1.37, p = 0.024), and Howardella (OR = 1.18, 95% CI = 1.09–1.28, p < 0.001) may be risk factors for CRF. However, after FDR correction, only the genera Escherichia-Shigella and Howardella had significant positive associations, while the other genera showed suggestive correlations. The detailed analysis results are available in Table 1. The complete MR analysis results of gut microbiota from 119 genera related to CRF can be found in Supplementary Table 2. Sensitivity analysis was conducted on the seven genera closely related to CRF, and based on the results of MR-Egger regression analysis and Cochran’s Q test, it was evident that there was no horizontal pleiotropy or heterogeneity among these genera, with the MR-PRESSO analysis yielding a global test p > 0.05, indicating no significant outliers. Specific results of the sensitivity analysis are presented in Table 2. The results of the pleiotropy and heterogeneity tests can be found in Supplementary Tables 3, 4. A leave-one-out analysis was performed for these seven genera (Figure 2), which showed that the results did not change significantly after sequentially removing individual SNPs, reflecting the robustness of the study findings to some extent. Scatter plots, Funnel plots, and Forest plots for these seven genera can be found in Supplementary Figures 1–3, respectively. Table 3 displays the relevant information of SNPs for the genera Escherichia-Shigella and Howardella used in this study. We further annotated the SNPs of these two genera using the VannoPortal database (Huang et al., 2022).
Table 3. Summary information on SNPs of Escherichia-Shigella and Howardella used for the Mendelian randomization analyses.
Previous studies on the gut microbiome and CKD have primarily utilized animal models (Vaziri et al., 2013), with observations often showing inconsistencies. While there have been reports on the causal relationship between the gut microbiome and CKD (Li et al., 2023; Ren et al., 2023), significant variations in the richness and evenness (α-diversity) of bacterial species across different CKD stages have been observed (Wu et al., 2020). CRF represents a more severe stage of kidney pathology. This study focuses on the later stages of the disease, CRF, and evaluates the causal relationship between the gut microbiota of 119 genera and CRF at a genetic level, utilizing large-scale GWAS data. After MR analysis and FDR correction of the results, we ultimately discovered that Escherichia-Shigella and Howardella may be risk factors for CRF, as they exhibited a significant association with CRF.
Escherichia and Shigella both belong to the family Enterobacteriaceae, which is part of the phylum Proteobacteria, and are Gram-negative rod-shaped bacteria. In many classification systems, Escherichia and Shigella are treated as separate genera; however, due to their high genomic similarity and the potential inaccuracy of current metagenomic sequencing techniques in distinguishing members of the two genera, some bioinformatics analyses classify them as a single genus, especially in genomic studies of microbial communities, where these two genera are commonly represented together as “Escherichia-Shigella.” Certain strains of the Escherichia-Shigella genus are pathogenic, and thus this genus is often reported as a pathogen (Kamada et al., 2013). The genus Escherichia is most commonly represented by Escherichia coli (Tenaillon et al., 2010). It has been reported that Shiga toxin-producing E. coli can cause classic hemolytic uremic syndrome (HUS), which is the most common cause of acute renal failure in children. This condition is caused by endothelial cell damage and leukocyte activation due to the production of Shiga-like toxins and lipopolysaccharides, leading to the formation of glomerular capillary thrombosis through the interaction of platelets with endothelial cells, ultimately affecting renal hemodynamics and causing acute kidney injury (Palermo et al., 2014; Fakhouri et al., 2017). CRF is also one of the complications of HUS (Razzaq, 2006). Shigella typically causes bacterial dysentery, also known as Shigellosis, and is genetically related to E. coli (Beld and Reubsaet, 2011). Wu et al. (2020) discovered through 16S rRNA gene sequencing that the genus Escherichia-Shigella is associated with various stages of CKD, particularly enriched in CRF, and that the abundance of Escherichia-Shigella is highly correlated with the levels of serum Indoxyl Sulfate. Research by Hu X. et al. (2020) indicates an increase in the quantities of the genus Escherichia-Shigella and the phylum Proteobacteria in the gut of CRF patients, especially prominent at stage 5 of the disease, with several genera including Escherichia-Shigella showing high sensitivity and specificity in distinguishing CKD patients from healthy controls. Furthermore, Bao et al. (2023) also found a close association between the genus Escherichia-Shigella and vascular calcification in hemodialysis patients, identifying it as a risk factor for vascular calcification in these patients.
Howardella is a Gram-positive anaerobic bacterium that can produce ATP by decomposing urea (Cook et al., 2007). However, while some gut microbes hydrolyze urea through urease, they can generate large amounts of ammonia, which may affect the growth of the intestinal microbiota (Ramezani and Raj, 2014). Moreover, increased levels of ammonia are associated with tubulointerstitial damage in the kidneys. The rise in renal ammonia levels can potentially activate the complement alternative pathway, resulting in the production of chemotactic and lytic complement components that lead to tubulointerstitial inflammation (Nath et al., 1985). Additionally, the production of ammonia by the intestinal microbiota through urea hydrolysis can also lead to the disruption of the structure and function of the intestinal epithelium. Consequently, this promotes the translocation of uremic toxins, endotoxins, antigens, and other microbial metabolites into the circulation, which could be an important pathway for endogenous infections (Simões-Silva et al., 2018). Studies have reported an increase in the abundance of the genus Howardella associated with prediabetes (Yang et al., 2015), while a lower abundance of Howardella has been observed in the gut of patients with depression (Barandouzi et al., 2020). Furthermore, Yang et al. (2023) found a positive correlation between exposure to nitrogen dioxide and the abundance of the genus Howardella. However, there are limited reports on the association between the genus Howardella and CRF, warranting further in-depth research in the future.
Increasing research indicates the presence of gut microbiota dysbiosis in patients with CRF. Vaziri et al. (2013) observed a significant increase in the abundance of genera such as Brachybacterium and Catenibacterium in CRF patients, while Jiang et al. found a decrease in the abundance of butyrate-producing bacteria, including Roseburia, Faecalibacterium, Clostridium, Coprococcus, and Prevotella in CRF patients. They also discovered a negative correlation between bacteria such as Roseburia spp., Faecalibacterium, Prevotella, and markers like C-reactive protein and Cystatin C (Jiang et al., 2017). Furthermore, a systematic review reported a decrease in microbial diversity in CKD patients compared to healthy individuals, with a consistent reduction in Roseburia abundance, especially in patients with end-stage kidney disease (Voroneanu et al., 2023). An increase in the abundance of the phylum Proteobacteria is a potential microbial diagnostic marker of dysbiosis (Shin et al., 2015), which is mainly associated with an increase in the Enterobacteriaceae family and the genus Escherichia. The inflammation-driven overgrowth of Enterobacteriaceae is closely linked to the development of various host diseases (Lupp et al., 2007). The findings on the gut microbiota of CRF patients are varied, possibly limited by the nature of observational studies, and these studies have not further explored their causal relationships. This study addresses the gap by investigating the causal relationship between bacterial genera and CRF at the genus level, addressing the current inadequacies in gut microbiota research in CRF. However, the mechanisms of action remain unclear. Based on the gut-kidney axis concept and current research, we hypothesize that the mechanisms of action of the gut microbiota on CRF relate to the following aspects: (1) Microbiome: The microbial communities within the gut can affect intestinal health and the systemic inflammatory state, and they can influence renal function through their metabolic products. (2) Metabolic Products: Urea and other nitrogenous wastes produced in the gut can affect kidney health through the circulatory system. (3) Mucosal Barrier Function and Intestinal Permeability: Impairment of the gut barrier may allow bacteria and endotoxins access to the bloodstream, from where they can reach the kidneys and potentially cause inflammatory responses. (4) Immune System: Immune cells in the gut may impact kidney function by promoting systemic inflammatory responses. Therefore, correcting gut microbial dysbiosis, improving gut barrier function, and reducing endotoxin load, among other measures, may contribute to better kidney health and slow the progression of CRF.
In the gut of CRF patients, there is a significant increase in the number of bacterial families capable of producing urease as well as enzymes that generate indoles and p-cresol, whereas the number of bacterial families that can convert dietary fiber into short-chain fatty acids (such as butyrate) significantly decreases (Wong et al., 2014). Urease can break down urea into ammonia and carbon dioxide. In CRF patients, this could lead to excessive production of ammonia, thereby increasing the concentration of ammonia in the gut and blood, potentially exacerbating symptoms of uremia. Enzymes that produce indoles and p-cresol are involved in the metabolism of tryptophan and phenolic compounds in the intestine, ultimately generating toxic substances to the human body, such as Indoxyl Sulfate (IS) and p-Cresyl Sulfate (pCS). IS and pCS are metabolites in the blood associated with renal failure. They are commonly used as biomarkers to measure kidney function and damage extent. Studies have shown that high levels of IS and pCS play a crucial role in the progression of CKD, with their accumulation closely linked to accelerated renal fibrosis, reduced kidney function, and CKD progression (Meijers and Evenepoel, 2011; Mutsaers et al., 2015). Indoxyl sulfate can trigger cellular oxidative stress response by generating reactive oxygen species, activate NF-κB promoting cellular senescence, and activate p53 accelerating the aging process of proximal tubular cells, ultimately exacerbating CRF progression (Shimizu et al., 2011). In CRF patients, due to renal function decline, the accumulation of these compounds in the serum further aggravates disease progression. The accumulation of these toxins causes damage not only to the kidneys but also to other tissues and organs of the body. Short-chain fatty acids (SCFAs), particularly butyrate, are extremely important for intestinal health, providing energy for intestinal cells, regulating local and systemic immune responses, and promoting the integrity of the gut barrier. In CRF patients, the reduction in this probiotic conversion capability may impact gut health, increase inflammation and intestinal permeability, thereby further affecting the overall health of the patients. Gut microbiota dysbiosis, especially in the context of kidney disease, by reducing beneficial microbes producing SCFAs and increasing protein-fermenting microbes, may lead to the production of uremic toxins, endotoxemia, systemic inflammation, and various complications associated with CKD, thus exacerbating the progression of kidney disease (Pan and Kang, 2017). However, research by Mitrović et al. has shown that the use of Synbiotic can effectively reduce the serum levels of Indoxyl Sulfate and high-sensitivity C-reactive protein in patients with CRF. This finding supports the effectiveness of Synbiotic targeted gut therapy in reducing uremic toxin levels and improving the micro-inflammatory state in CRF patients, proving it to be a safe and effective treatment strategy (Mitrović et al., 2023). This indicates that correcting gut microbiota dysbiosis, improving gut barrier function, and reducing endotoxin load can all contribute to improving kidney health and delaying the progression of CRF.
It is noteworthy that the gut microbiota encompasses bacteria, fungi, viruses, archaea, and other microorganisms, together constituting a complex ecosystem. Bacteria are the most abundant microorganisms in the gut microbiota, and hence, current research on gut microbiota primarily focuses on the bacterial component. However, other microorganisms can also influence the health and disease states of the host under certain conditions.
The gut mycobiome, a component of the gut microbiome, plays an indispensable role in maintaining the balance of the intestinal environment and host health, despite fungi being less numerous than bacteria in the gut. Research by Qiu et al. has identified significant alterations in the composition of the gut fungi in individuals with both hypertension and CKD. Compared to healthy controls, this specific population exhibited increased richness and diversity of gut fungi, particularly elevated levels of the genera Apiotrichum and Saccharomyces, while the abundance of Candida decreased. Further analysis revealed a negative correlation between Candida and tumor necrosis factor alpha (TNF-α), and a positive correlation was found between the filtration rate of the glomeruli and the genera Apiotrichum, Ophiocordyceps, Saccharomyces, Nakaseomyces, and Septoria (Qiu et al., 2023). These findings suggest that these specific gut fungi may play an important role in protecting kidney function. Research by Ren et al. also found that patients with ESRD have a higher diversity of gut fungi compared to healthy individuals, characterized by a decrease in Saccharomyces cerevisiae and an increase in various opportunistic pathogens (such as Aspergillus fumigatus, Exophiala spinifera, Hortaea werneckii, etc.). Further metabolomic analysis revealed that the enriched opportunistic pathogens correlated positively with levels of serum creatinine, homocysteine, and phenylacetylglycine, while the yeast community negatively correlated with the levels of toxic metabolites in feces (Ren et al., 2024). Additionally, studies have shown a certain link between the risk of inflammatory bowel disease and CKD (Vajravelu et al., 2020), while Saccharomyces cerevisiae has been found to inhibit and reduce chemically induced colonic inflammation (Sivignon et al., 2015). Hu et al. (2022) also discovered a correlation between yeast and serum levels of gamma light chains. These findings indicate that the gut mycobiome is closely associated with the inflammatory state, immune response, and toxin levels in patients with CRF.
Viral infections are involved in a variety of glomerular diseases (Sallustio et al., 2023). The gut virome, particularly the part dominated by bacteriophages, plays a crucial role in the ecological balance of gut microbiota. These bacteriophages, by invading and replicating within bacterial hosts, not only regulate the population of specific bacterial groups to maintain microbial diversity but also significantly influence the host’s immune system regulation. Studies have reported that bacteriophages can activate the host’s immune response via the TLR9 sensing pathway, promoting the production of cytokines such as IL-12, IL-6, IL-10, and IFN-γ (Gogokhia et al., 2019; Sweere et al., 2019). Bacteriophages can also enter the systemic circulation through intestinal epithelial cells and then affect the human immune system by modulating the release of cytokines and the activity of T and B cells (Popescu et al., 2021). Research by Fan et al. (2023) revealed an imbalance in the gut virome in patients with diabetic nephropathy (DN), characterized by a significant decrease in overall viral richness and diversity. Notably, levels of Shigella phage and Xylella phage were elevated in the guts of DN patients. Further analysis of the functional aspects of the gut virome found multiple viral functional losses in DN patients, particularly a weakened ability of bacteriophages to lyse their bacterial hosts. This reduction in function could have important implications for the ecological balance of the gut microbiota.
Archaea represent a unique form of life, distinct from bacteria and eukaryotes, possessing special biological characteristics and metabolic pathways. Despite their relatively low abundance in the human body, archaea play a crucial role in maintaining human health and environmental balance (Mafra et al., 2022). Particularly in the human gut, methanogenic archaea, such as Methanobrevibacter smithii, dominate. These archaea not only promote the development of other microbes by consuming hydrogen, maintaining the stability and diversity of the gut microbial community, but also regulate the structure and function of the gut microbiome through their unique metabolic products (e.g., methane), significantly impacting host health. Trimethylamine-N-oxide (TMAO) has been shown to promote oxidative stress, inflammation, and the progression of CKD (Borges et al., 2016; Castillo-Rodriguez et al., 2018), and is an independent predictor of the burden of coronary artery atherosclerosis and mortality (Stubbs et al., 2016). Research has reported that methanogenic archaea can reduce the conversion of trimethylamine (TMA) to TMAO (Brugère et al., 2013), thereby decreasing the production of TMAO associated with health issues such as cardiovascular diseases CKD, and trimethylaminuria. Therefore, modulating the metabolic activity of archaea, especially increasing beneficial archaeal populations, may help mitigate inflammation and treat related disease states. This reveals the potential positive role of archaea in regulating the gut microbiome, reducing the generation of harmful metabolic products, and combating chronic inflammation and related diseases through possible other mechanisms, such as modulating host immune responses, offering a new perspective for the treatment of related diseases.
In summary, our study addresses the current gaps in research on the gut microbiota in CRF and identifies gut genera with potential causal relationships to CRF, offering more possibilities for future targeted gut interventions to prevent or treat CRF. However, our research has certain limitations. Firstly, since our data were derived from public databases, which only provided aggregated data related to single nucleotide polymorphisms without information on individual confounding factors such as age, gender, and antibiotic usage, we were unable to conduct more detailed subgroup analyses. Secondly, our study merely infers the causal relationship between gut microbiota and CRF from a theoretical perspective, with a focus on the correlation analysis between gut genera and CRF. The gut microbiome is a complex system influenced by diet, medication, environment, and other factors (Stepkowski et al., 2017; Asnicar et al., 2021). Future large-scale randomized controlled trials incorporating metagenomics, metabolomics, genomics, and in vitro cultures are needed to further investigate its specific mechanisms of action. Lastly, the GWAS data we used primarily involved European populations, which may not be generalizable to other ethnic groups. Moreover, our exposure data focused on the genus level of microbial communities, indicating that future research should delve deeper into species or strain-level analyses. We believe that as research progresses, modern medicine may develop new therapeutic strategies to prevent or treat various kidney diseases, including CRF, by improving the interaction between the gut and the kidney axis.
The results of this study suggest that the genera Escherichia-Shigella and Howardella are potential risk factors for CRF, highlighting their significance in CRF. Future interventions for patients with CRF could potentially include dietary modifications, probiotics, and fecal transplantation to slow the progression of the disease. However, our research can only infer part of the causation. The onset and progression of CRF are also related to many other factors beyond the gut microbiota, and further in-depth, large-scale studies are needed to fully elucidate their specific mechanisms of action.
The original contributions presented in the study are included in the article/Supplementary material, further inquiries can be directed to the corresponding author.
XL: Conceptualization, Data curation, Methodology, Writing – original draft, Writing – review & editing. JM: Data curation, Methodology, Writing – review & editing. XY: Formal analysis, Methodology, Writing – review & editing. LP: Conceptualization, Writing – review & editing. YoZ: Formal analysis, Writing – review & editing. YiZ: Supervision, Writing – review & editing. GS: Supervision, Writing – review & editing.
The author(s) declare that no financial support was received for the research, authorship, and/or publication of this article.
We want to acknowledge all the volunteers who participated in this study, and we also thank MiBioGen consortium and large-scale consortia or studies for providing gut microbiota and chronic renal failure GWAS summary statistics data for our analyses.
The authors declare that the research was conducted in the absence of any commercial or financial relationships that could be construed as a potential conflict of interest.
All claims expressed in this article are solely those of the authors and do not necessarily represent those of their affiliated organizations, or those of the publisher, the editors and the reviewers. Any product that may be evaluated in this article, or claim that may be made by its manufacturer, is not guaranteed or endorsed by the publisher.
The Supplementary material for this article can be found online at: https://www.frontiersin.org/articles/10.3389/fmicb.2024.1356478/full#supplementary-material
Asnicar, F., Berry, S. E., Valdes, A. M., Nguyen, L. H., Piccinno, G., Drew, D. A., et al. (2021). Microbiome connections with host metabolism and habitual diet from 1,098 deeply phenotyped individuals. Nat. Med. 27, 321–332. doi: 10.1038/s41591-020-01183-8
Bao, W.-H., Yang, W.-L., Su, C.-Y., Lu, X.-H., He, L., and Zhang, A.-H. (2023). Relationship between gut microbiota and vascular calcification in hemodialysis patients. Ren. Fail. 45:2148538. doi: 10.1080/0886022X.2022.2148538
Barandouzi, Z. A., Starkweather, A. R., Henderson, W. A., Gyamfi, A., and Cong, X. S. (2020). Altered composition of gut microbiota in depression: a systematic review. Front. Psych. 11:541. doi: 10.3389/fpsyt.2020.00541
Beld, M. J. C., and Reubsaet, F. A. G. (2011). Differentiation between Shigella, enteroinvasive Escherichia coli (EIEC) and noninvasive Escherichia coli. Eur. J. Clin. Microbiol. Infect. Dis. 31, 899–904. doi: 10.1007/s10096-011-1395-7
Borges, N. A., Barros, A. F., Nakao, L. S., Dolenga, C. J., Fouque, D., and Mafra, D. (2016). Protein-bound uremic toxins from gut microbiota and inflammatory markers in chronic kidney disease. J. Ren. Nutr. 26, 396–400. doi: 10.1053/j.jrn.2016.07.005
Bowden, J., Davey Smith, G., Haycock, P. C., and Burgess, S. (2016). Consistent estimation in Mendelian randomization with some invalid instruments using a weighted median estimator. Genet. Epidemiol. 40, 304–314. doi: 10.1002/gepi.21965
Brugère, J.-F., Borrel, G., Gaci, N., Tottey, W., O’Toole, P. W., and Malpuech-Brugère, C. (2013). Archaebiotics. Gut Microbes 5, 5–10. doi: 10.4161/gmic.26749
Burgess, S., Dudbridge, F., and Thompson, S. G. (2015). Combining information on multiple instrumental variables in Mendelian randomization: comparison of allele score and summarized data methods. Stat. Med. 35, 1880–1906. doi: 10.1002/sim.6835
Burgess, S., and Thompson, S. G. (2017). Interpreting findings from Mendelian randomization using the MR-egger method. Eur. J. Epidemiol. 32, 377–389. doi: 10.1007/s10654-017-0255-x
Castillo-Rodriguez, E., Fernandez-Prado, R., Esteras, R., Perez-Gomez, M. V., Gracia-Iguacel, C., Fernandez-Fernandez, B., et al. (2018). Impact of altered intestinal microbiota on chronic kidney disease progression. Toxins (Basel) 10:300. doi: 10.3390/toxins10070300
Cigarran Guldris, S., González Parra, E., and Cases Amenós, A. (2017). Microbiota intestinal en la enfermedad renal crónica. Nefrologia 37, 9–19. doi: 10.1016/j.nefro.2016.05.008
Cook, A. R., Riley, P. W., Murdoch, H., Evans, P. N., and McDonald, I. R. (2007). Howardella ureilytica gen. nov., sp. nov., a gram-positive, coccoid-shaped bacterium from a sheep rumen. Int. J. Syst. Evol. Microbiol. 57, 57, 2940–2945. doi: 10.1099/ijs.0.64819-0
Fakhouri, F., Zuber, J., Frémeaux-Bacchi, V., and Loirat, C. (2017). Haemolytic uraemic syndrome. Lancet 390, 681–696. doi: 10.1016/S0140-6736(17)30062-4
Fan, G., Cao, F., Kuang, T., Yi, H., Zhao, C., Wang, L., et al. (2023). Alterations in the gut virome are associated with type 2 diabetes and diabetic nephropathy. Gut Microbes 15:2226925. doi: 10.1080/19490976.2023.2226925
Fang, J., Guo, Y., Yin, W., Zhang, L., Li, G., Ma, J., et al. (2023). Neoxanthin alleviates the chronic renal failure-induced aging and fibrosis by regulating inflammatory process. Int. Immunopharmacol. 114:109429. doi: 10.1016/j.intimp.2022.109429
Ference, B. A., Holmes, M. V., and Smith, G. D. (2021). Using Mendelian randomization to improve the Design of Randomized Trials. Cold Spring Harb. Perspect. Med. 11:a040980. doi: 10.1101/cshperspect.a040980
Gogokhia, L., Buhrke, K., Bell, R., Hoffman, B., Brown, D. G., Hanke-Gogokhia, C., et al. (2019). Expansion of bacteriophages is linked to aggravated intestinal inflammation and colitis. Cell Host Microbe 25, 285–299.e8. doi: 10.1016/j.chom.2019.01.008
Ho, H.-J., and Shirakawa, H. (2022). Oxidative stress and mitochondrial dysfunction in chronic kidney disease. Cells 12:88. doi: 10.3390/cells12010088
Hu, X., Ouyang, S., Xie, Y., Gong, Z., and Du, J. (2020). Characterizing the gut microbiota in patients with chronic kidney disease. Postgrad. Med. 132, 495–505. doi: 10.1080/00325481.2020.1744335
Hu, J., Wei, S., Gu, Y., Wang, Y., Feng, Y., Sheng, J., et al. (2022). Gut Mycobiome in patients with chronic kidney disease was altered and associated with immunological profiles. Front. Immunol. 13:843695. doi: 10.3389/fimmu.2022.843695
Hu, J., Zhong, X., Yan, J., Zhou, D., Qin, D., Xiao, X., et al. (2020). High-throughput sequencing analysis of intestinal flora changes in ESRD and CKD patients. BMC Nephrol. 21:12. doi: 10.1186/s12882-019-1668-4
Huang, D., Zhou, Y., Yi, X., Fan, X., Wang, J., Yao, H., et al. (2022). VannoPortal: multiscale functional annotation of human genetic variants for interrogating molecular mechanism of traits and diseases. Nucleic Acids Res. 50, D1408–D1416. doi: 10.1093/nar/gkab853
Jiang, S., Xie, S., Lv, D., Wang, P., He, H., Zhang, T., et al. (2017). Alteration of the gut microbiota in Chinese population with chronic kidney disease. Sci. Rep. 7:2870. doi: 10.1038/s41598-017-02989-2
Kamada, N., Seo, S.-U., Chen, G. Y., and Núñez, G. (2013). Role of the gut microbiota in immunity and inflammatory disease. Nat. Rev. Immunol. 13, 321–335. doi: 10.1038/nri3430
Kurilshikov, A., Medina-Gomez, C., Bacigalupe, R., Radjabzadeh, D., Wang, J., Demirkan, A., et al. (2021). Large-scale association analyses identify host factors influencing human gut microbiome composition. Nat. Genet. 53, 156–165. doi: 10.1038/s41588-020-00763-1
Li, N., Wang, Y., Wei, P., Min, Y., Yu, M., Zhou, G., et al. (2023). Causal effects of specific gut microbiota on chronic kidney diseases and renal function—a two-sample Mendelian randomization study. Nutrients 15:360. doi: 10.3390/nu15020360
Long, Y., Tang, L., Zhou, Y., Zhao, S., and Zhu, H. (2023). Causal relationship between gut microbiota and cancers: a two-sample Mendelian randomisation study. BMC Med. 21:66. doi: 10.1186/s12916-023-02761-6
Lozupone, C. A., Stombaugh, J. I., Gordon, J. I., Jansson, J. K., and Knight, R. (2012). Diversity, stability and resilience of the human gut microbiota. Nature 489, 220–230. doi: 10.1038/nature11550
Lupp, C., Robertson, M. L., Wickham, M. E., Sekirov, I., Champion, O. L., Gaynor, E. C., et al. (2007). Host-mediated inflammation disrupts the intestinal microbiota and promotes the overgrowth of Enterobacteriaceae. Cell Host Microbe 2, 119–129. doi: 10.1016/j.chom.2007.06.010
Mafra, D., Lobo, J. C., Barros, A. F., Koppe, L., Vaziri, N. D., and Fouque, D. (2014). Role of altered intestinal microbiota in systemic inflammation and cardiovascular disease in chronic kidney disease. Future Microbiol. 9, 399–410. doi: 10.2217/fmb.13.165
Mafra, D., Ribeiro, M., Fonseca, L., Regis, B., Cardozo, L., Fragoso Dos Santos, H., et al. (2022). Archaea from the gut microbiota of humans: could be linked to chronic diseases? Anaerobe 77:102629. doi: 10.1016/j.anaerobe.2022.102629
Martínez-Hernández, S., Muñoz-Ortega, M., Ávila-Blanco, M., Medina-Pizaño, M., and Ventura-Juárez, J. (2023). Novel approaches in chronic renal failure without renal replacement therapy: a review. Biomedicines 11:2828. doi: 10.3390/biomedicines11102828
Meijers, B. K., and Evenepoel, P. (2011). The gut-kidney axis: indoxyl sulfate, p-cresyl sulfate and CKD progression. Nephrol. Dial. Transplant. 26, 759–761. doi: 10.1093/ndt/gfq818
Mitrović, M., Stanković-Popović, V., Tolinački, M., Golić, N., Soković Bajić, S., Veljović, K., et al. (2023). The impact of synbiotic treatment on the levels of gut-derived uremic toxins, inflammation, and gut microbiome of chronic kidney disease patients—a randomized trial. J. Ren. Nutr. 33, 278–288. doi: 10.1053/j.jrn.2022.07.008
Mutsaers, H. A. M., Stribos, E. G. D., Glorieux, G., Vanholder, R., and Olinga, P. (2015). Chronic kidney disease and fibrosis: the role of uremic retention solutes. Front. Med. 2:60. doi: 10.3389/fmed.2015.00060
Nath, K. A., Hostetter, M. K., and Hostetter, T. H. (1985). Pathophysiology of chronic tubulo-interstitial disease in rats. Interactions of dietary acid load, ammonia, and complement component C3. J. Clin. Invest. 76, 667–675. doi: 10.1172/JCI112020
Ni, J. J., Xu, Q., Yan, S. S., Han, B. X., Zhang, H., Wei, X. T., et al. (2021). Gut microbiota and psychiatric disorders: a two-sample Mendelian randomization study. Front. Microbiol. 12:737197. doi: 10.3389/fmicb.2021.737197
Palermo, M. S., Exeni, R. A., and Fernández, G. C. (2014). Hemolytic uremic syndrome: pathogenesis and update of interventions. Expert Rev. Anti-Infect. Ther. 7, 697–707. doi: 10.1586/eri.09.49
Pan, W., and Kang, Y. (2017). Gut microbiota and chronic kidney disease: implications for novel mechanistic insights and therapeutic strategies. Int. Urol. Nephrol. 50, 289–299. doi: 10.1007/s11255-017-1689-5
Peppa, M., Uribarri, J., Cai, W., Lu, M., and Vlassara, H. (2004). Glycoxidation and inflammation in renal failure patients. Am. J. Kidney Dis. 43, 690–695. doi: 10.1053/j.ajkd.2003.11.022
Piko, N., Bevc, S., Hojs, R., and Ekart, R. (2023). The role of oxidative stress in kidney injury. Antioxidants 12:1772. doi: 10.3390/antiox12091772
Popescu, M., Van Belleghem, J. D., Khosravi, A., and Bollyky, P. L. (2021). Bacteriophages and the immune system. Ann. Rev. Virol. 8, 415–435. doi: 10.1146/annurev-virology-091919-074551
Qiu, J., Zhao, L., Cheng, Y., Chen, Q., Xu, Y., Lu, Y., et al. (2023). Exploring the gut mycobiome: differential composition and clinical associations in hypertension, chronic kidney disease, and their comorbidity. Front. Immunol. 14:1317809. doi: 10.3389/fimmu.2023.1317809
Ramezani, A., and Raj, D. S. (2014). The gut microbiome, kidney disease, and targeted interventions. J. Am. Soc. Nephrol. 25, 657–670. doi: 10.1681/ASN.2013080905
Razzaq, S. (2006). Hemolytic uremic syndrome: an emerging health risk. Am. Fam. Physician 74, 991–996.
Ren, Y., Chen, L., Guo, R., Ma, S., Li, S., Zhang, Y., et al. (2024). Altered gut mycobiome in patients with end-stage renal disease and its correlations with serum and fecal metabolomes. J. Transl. Med. 22:202. doi: 10.1186/s12967-024-05004-1
Ren, F., Jin, Q., Jin, Q., Qian, Y., Ren, X., Liu, T., et al. (2023). Genetic evidence supporting the causal role of gut microbiota in chronic kidney disease and chronic systemic inflammation in CKD: a bilateral two-sample Mendelian randomization study. Front. Immunol. 14:1287698. doi: 10.3389/fimmu.2023.1287698
Sakaue, S., Kanai, M., Tanigawa, Y., Karjalainen, J., Kurki, M., Koshiba, S., et al. (2021). A cross-population atlas of genetic associations for 220 human phenotypes. Nat. Genet. 53, 1415–1424. doi: 10.1038/s41588-021-00931-x
Sallustio, F., Picerno, A., Montenegro, F., Cimmarusti, M. T., Di Leo, V., and Gesualdo, L. (2023). The human Virome and its crosslink with glomerulonephritis and IgA nephropathy. Int. J. Mol. Sci. 24:3897. doi: 10.3390/ijms24043897
Sekula, P., Del Greco, M. F., Pattaro, C., and Kottgen, A. (2016). Mendelian randomization as an approach to assess causality using observational data. J. Am. Soc. Nephrol. 27, 3253–3265. doi: 10.1681/ASN.2016010098
Shimizu, H., Bolati, D., Adijiang, A., Muteliefu, G., Enomoto, A., Nishijima, F., et al. (2011). NF-κB plays an important role in indoxyl sulfate-induced cellular senescence, fibrotic gene expression, and inhibition of proliferation in proximal tubular cells. Am. J. Phys. Cell Phys. 301, C1201–C1212. doi: 10.1152/ajpcell.00471.2010
Shin, N.-R., Whon, T. W., and Bae, J.-W. (2015). Proteobacteria: microbial signature of dysbiosis in gut microbiota. Trends Biotechnol. 33, 496–503. doi: 10.1016/j.tibtech.2015.06.011
Simões-Silva, L., Araujo, R., Pestana, M., Soares-Silva, I., and Sampaio-Maia, B. (2018). The microbiome in chronic kidney disease patients undergoing hemodialysis and peritoneal dialysis. Pharmacol. Res. 130, 143–151. doi: 10.1016/j.phrs.2018.02.011
Sivignon, A., de Vallée, A., Barnich, N., Denizot, J., Darcha, C., Pignède, G., et al. (2015). Saccharomyces cerevisiae CNCM I-3856 prevents colitis induced by AIEC Bacteria in the transgenic mouse model mimicking Crohnʼs disease. Inflamm. Bowel Dis. 21, 276–286. doi: 10.1097/MIB.0000000000000280
Smyth, L. J., Duffy, S., Maxwell, A. P., and McKnight, A. J. (2014). Genetic and epigenetic factors influencing chronic kidney disease. Am. J. Physiol. Renal Physiol. 307, F757–F776. doi: 10.1152/ajprenal.00306.2014
Stadlbauer, V., Horvath, A., Ribitsch, W., Schmerböck, B., Schilcher, G., Lemesch, S., et al. (2017). Structural and functional differences in gut microbiome composition in patients undergoing haemodialysis or peritoneal dialysis. Sci. Rep. 7:15601. doi: 10.1038/s41598-017-15650-9
Stepkowski, S., Zaza, G., Gassa, A. D., Felis, G., Granata, S., Torriani, S., et al. (2017). Impact of maintenance immunosuppressive therapy on the fecal microbiome of renal transplant recipients: comparison between an everolimus- and a standard tacrolimus-based regimen. PLoS One 12:e0178228. doi: 10.1371/journal.pone.0178228
Storey, J. D., and Tibshirani, R. (2003). Statistical significance for genomewide studies. Proc. Natl. Acad. Sci. 100, 9440–9445. doi: 10.1073/pnas.1530509100
Stubbs, J. R., House, J. A., Ocque, A. J., Zhang, S., Johnson, C., Kimber, C., et al. (2016). Serum trimethylamine-N-oxide is elevated in CKD and correlates with coronary atherosclerosis burden. J. Am. Soc. Nephrol. 27, 305–313. doi: 10.1681/ASN.2014111063
Sweere, J. M., Van Belleghem, J. D., Ishak, H., Bach, M. S., Popescu, M., Sunkari, V., et al. (2019). Bacteriophage trigger antiviral immunity and prevent clearance of bacterial infection. Science 363:eaat9691. doi: 10.1126/science.aat9691
Tenaillon, O., Skurnik, D., Picard, B., and Denamur, E. (2010). The population genetics of commensal Escherichia coli. Nat. Rev. Microbiol. 8, 207–217. doi: 10.1038/nrmicro2298
Tian, N., Li, L., Ng, J. K.-C., and Li, P. K.-T. (2022). The potential benefits and controversies of probiotics use in patients at different stages of chronic kidney disease. Nutrients 14:4044. doi: 10.3390/nu14194044
Vajravelu, R. K., Copelovitch, L., Osterman, M. T., Scott, F. I., Mamtani, R., Lewis, J. D., et al. (2020). Inflammatory bowel diseases are associated with an increased risk for chronic kidney disease, which decreases with age. Clin. Gastroenterol. Hepatol. 18, 2262–2268. doi: 10.1016/j.cgh.2019.10.043
Vaziri, N. D., Wong, J., Pahl, M., Piceno, Y. M., Yuan, J., DeSantis, T. Z., et al. (2013). Chronic kidney disease alters intestinal microbial flora. Kidney Int. 83, 308–315. doi: 10.1038/ki.2012.345
Voroneanu, L., Burlacu, A., Brinza, C., Covic, A., Balan, G. G., Nistor, I., et al. (2023). Gut microbiota in chronic kidney disease: from composition to modulation towards better outcomes-a systematic review. J. Clin. Med. 12:1948. doi: 10.3390/jcm12051948
Wong, J., Piceno, Y. M., DeSantis, T. Z., Pahl, M., Andersen, G. L., and Vaziri, N. D. (2014). Expansion of urease- and Uricase-containing, indole- and p-cresol-forming and contraction of short-chain fatty acid-producing intestinal microbiota in ESRD. Am. J. Nephrol. 39, 230–237. doi: 10.1159/000360010
Wu, I. W., Lin, C.-Y., Chang, L.-C., Lee, C.-C., Chiu, C.-Y., Hsu, H.-J., et al. (2020). Gut microbiota as diagnostic tools for mirroring disease progression and circulating nephrotoxin levels in chronic kidney disease: discovery and validation study. Int. J. Biol. Sci. 16, 420–434. doi: 10.7150/ijbs.37421
Xu, Q., Ni, J. J., Han, B. X., Yan, S. S., Wei, X. T., Feng, G. J., et al. (2021). Causal relationship between gut microbiota and autoimmune diseases: a two-sample Mendelian randomization study. Front. Immunol. 12:746998. doi: 10.3389/fimmu.2021.746998
Yang, T., Richards, E. M., Pepine, C. J., and Raizada, M. K. (2018). The gut microbiota and the brain-gut-kidney axis in hypertension and chronic kidney disease. Nat. Rev. Nephrol. 14, 442–456. doi: 10.1038/s41581-018-0018-2
Yang, H., Shi, P., Li, M., Kong, L., Liu, S., Jiang, L., et al. (2023). Mendelian-randomization study reveals causal relationships between nitrogen dioxide and gut microbiota. Ecotoxicol. Environ. Saf. 267:115660. doi: 10.1016/j.ecoenv.2023.115660
Keywords: chronic renal failure, gut microbiota, gut-kidney axis, Mendelian randomization, causal inference
Citation: Liu X, Mo J, Yang X, Peng L, Zeng Y, Zheng Y and Song G (2024) Causal relationship between gut microbiota and chronic renal failure: a two-sample Mendelian randomization study. Front. Microbiol. 15:1356478. doi: 10.3389/fmicb.2024.1356478
Received: 19 December 2023; Accepted: 20 March 2024;
Published: 03 April 2024.
Edited by:
Liang Wang, Guangdong Provincial People’s Hospital, ChinaReviewed by:
Yi Xiao Liu, Peking University, ChinaCopyright © 2024 Liu, Mo, Yang, Peng, Zeng, Zheng and Song. This is an open-access article distributed under the terms of the Creative Commons Attribution License (CC BY). The use, distribution or reproduction in other forums is permitted, provided the original author(s) and the copyright owner(s) are credited and that the original publication in this journal is cited, in accordance with accepted academic practice. No use, distribution or reproduction is permitted which does not comply with these terms.
*Correspondence: Gaofeng Song, c29uZ2dhb2Zlbmc4MDExMTNAMTYzLmNvbQ==
Disclaimer: All claims expressed in this article are solely those of the authors and do not necessarily represent those of their affiliated organizations, or those of the publisher, the editors and the reviewers. Any product that may be evaluated in this article or claim that may be made by its manufacturer is not guaranteed or endorsed by the publisher.
Research integrity at Frontiers
Learn more about the work of our research integrity team to safeguard the quality of each article we publish.