- 1Department of Cardiology, The Second Hospital of Shanxi Medical University, Taiyuan, China
- 2Academy of Medical Sciences, The Shanxi Medical University, Taiyuan, China
- 3Department of Neonatology, The Second Hospital of Shanxi Medical University, Taiyuan, China
- 4State Key Laboratory of Oral Diseases, National Clinical Research Center for Oral Diseases, Department of Cariology and Endodontics, West China Hospital of Stomatology, Sichuan University, Chengdu, China
Objective: This study aimed to explore the changes in gut microbiota and its metabolites in different pathophysiological stages of doxorubicin (DOX)-induced heart failure (DIHF) and the relationship between gut microbiota and metabolites in various degrees of DIHF.
Materials and methods: C57BL/6 J mice were injected intraperitoneally with 5 mg/kg of DOX once a week for 5 consecutive weeks. At different times after injection, the cardiac function and histopathological analysis was conducted, the serum levels of creatine kinase (CK), CK-MB, lactic dehydrogenase, and cardiac troponin T were determined. 16S rRNA gene sequencing of feces and the nontargeted metabolomics analysis of serum were performed. Multi-omics analyses were used to explore the correlation between gut microbiota and serum metabolites.
Results: The results showed that DOX caused cardiac contractile dysfunction and left ventricular (LV) dilation. The levels of myocardial enzymes significantly increase in 3 and 5 weeks after DOX injection. DOX-treated mice showed significant differences in the composition and abundance of gut microorganisms, and the levels of serum metabolites at different times of treatment. Multi-omics analyses showed that intestinal bacteria were significantly correlated with the differential metabolites. Some bacteria and metabolites can be used as biomarkers of DIHF (AUC > 0.8). KEGG analyses showed the involvement of different metabolic pathways in various degrees of DIHF.
Conclusion: Marked differences were found in the composition and abundance of gut microorganisms, the levels of serum metabolites and metabolic pathways in different degrees of DIHF. The intestinal bacteria were significantly correlated with differential metabolites in different degrees of DIHF. The gut microbiota may serve as new targets for the treatment of DIHF.
1 Introduction
Doxorubicin (DOX) is a representative anthracycline antibiotic with a broad antitumor spectrum and potent action, and is widely used for treating various solid tumors and hematologic malignancies (Arcamone et al., 1969). However, DOX suffers from severe dose-dependent cardiotoxicity that leads to irreversible congestive heart failure (HF), thus limiting its clinical application (Yu et al., 2018; Wang et al., 2021). Research has shown that the DOX can induce myocardial toxicity via various mechanisms such as oxidative stress, iron metabolism, inflammation, and Ca2+overload (Yu et al., 2020; Christidi and Brunham, 2021; Zhu et al., 2010; Arai et al., 2000). Thus, a variety of strategies including limitation of cumulative DOX doses, use of antioxidant drugs, and standard anti-heart failure therapies have been proposed to ameliorate DOX-induced HF (DIHF), however, these strategies is not much satisfying (Huang et al., 2022). Therefore, it is crucial to better understand the pathological mechanisms and established novel strategies to prevent and treat DOX-induced myocardial toxicity.
Gut microbes has been found to play an essential role in the pathophysiological process of atherosclerosis, dyslipidemia, hypertension, heart failure, coronary heart disease (CAD), and obesity through immune response, inflammatory response, and oxidative stress (Kazemian et al., 2020; Rahman et al., 2022). The potential role of gut microbiota in DIHF has received widespread attention in recent years (Huang et al., 2022; Fan et al., 2023). The composition imbalance and functional changes of the gut microbiota can be one of the underlying etiological mechanisms of DIHF (Huang et al., 2022). However, how the gut microbiota change in different pathophysiological stages of HF under the action of DOX is still not fully clarified.
The gut microbiota related metabolites have been implicated in the progression of cardiovascular diseases (CVDs). Gut microbes provide nutrients and energy to the host through the digestion of the ingested food and metabolism, which subsequently producing biologically active signaling molecules to maintain the body’s health. Diseases can also be triggered when the metabolism of the intestinal flora is disturbed. Several studies have reported on the relationship between metabolites of some intestinal microorganisms and cardiovascular diseases (Kazemian et al., 2020; Rahman et al., 2022; Liu et al., 2019; Yousuf et al., 2022) However, whether gut microorganisms regulate the metabolites of DIHF is still not fully clarified.
We constructed a model of HF established by intraperitoneal injection of DOX. Comprehensive analyses of their gut microbiota and metabolomic profiles were conducted using 16S amplicon sequencing and liquid chromatography combined with tandem mass spectrometry (LC–MS/MS) to address the aforementioned issues. Furthermore, we assessed the correlation between gut microorganisms and serum metabolites and explored whether gut microbiota regulated the metabolism in DOX-treated mice. In addition, we screened the biomarkers of microflora and metabolites related to DIHF. This study might provide new targets and new ideas for treating DIHF.
2 Materials and methods
2.1 Materials
DOX was purchased from Shenzhen Wanle Pharmaceutical Co., Ltd. (Wanle, Shenzhen, China). The kits for lactate dehydrogenase (LDH), creatine kinase (CK), creatine kinase isozyme (CK-MB), and cardiac troponin T (cTnT) were acquired from Jiancheng Technology Co. (Nanjing, China).
2.2 Animals and treatment
The animal experiments in this study was approved by the Institutional Animal Care and Use Committee at Second Hospital of Shanxi Medical University (DW2022070) and conformed to the Guide for the Care and Use of Laboratory Animals published by the US National Institute of Health. Healthy male C57BL/6 J mice (aged 8 weeks) were purchased from the Shanxi Medical University (Taiyuan, China, SCXK Jin 2019-0004) and housed in the Specified Pathogen Free (SPF) Laboratory of the Second Hospital of Shanxi Medical University (Taiyuan, China, SYXK Jin 2021-0001). All C57BL/6 J mice were randomly divided into control group (n = 13), DOX-1w group (DOX treatment for 1 week, n = 13), DOX-3w group (DOX treatment for 3 week, n = 13), and DOX-5w group (DOX treatment for 5 week, n = 13) after 1 week adaptive feeding. DOX was configured with a concentration of 1 mg/mL, and the mice in the DOX-1w, DOX-3w, and DOX-5w groups were injected intraperitoneally with DOX (5 mg/kg) once a week for 1 week, 3 and 5 consecutive weeks, respectively. The cumulative doses of DOX in DOX-1w, DOX-3w and DOX-5w groups were 5 mg/kg, 15 mg/kg, and 25 mg/kg, respectively. The mice in the control group were intraperitoneally injected with an equal volume of normal saline once a week for 5 weeks. After the treatment of DOX, ten mice survived in the DOX-5w group, 12 mice survived in the DOX-3w group, and 13 mice survived in control and DOX-1w groups.
2.3 Cardiac echocardiography
At 1, 3, and 5 week after the injection of DOX, the mice were anesthetized with 1.5% isoflurane, and echocardiograms were performed with a MX-550D probe at the papillary muscle level near the sternum of mice for five consecutive cardiac cycles with the Vevo 3100 high-resolution imaging system (Fujifilm VisualSonics, Canada). The left ventricular ejection fraction (LVEF), left ventricular short-axis shortening rate (LVFS), left ventricular internal diameter at end-systole (LVIDs), and left ventricle posterior wall thickness at end-systole (LVPWs) were recorded.
2.4 Determination of serum biochemical indices
The serum levels of LDH, CK, CK-MB, and cTnT are direct signals of myocardium damage. The serum of mice was obtained by centrifugation at 3,000 rpm for 10 min. The serum levels of LDH, CK, CK-MB, and cTnT were detected using relevant commercial kits following the manufacturer’s instructions (Nanjing Jiancheng Bioengineering Institute, China). The nontargeted metabonomics was detected using LC-MS/MS.
2.5 Histological examination
After performing cardiac echocardiography and extracting blood, the mice were sacrificed with intravenous pentobarbital sodium (100 mg/kg) at the end of study.
The LV myocardial tissue was harvested, fixed in 4% formaldehyde, subsequently embedded in paraffin, and then routinely sectioned. Then hematoxylin–eosin (H-E) staining was performed, and Masson trichome staining was used to determine myocardial fibrosis.
2.6 Collection of fecal samples and DNA extraction
The feces were removed from mice in a sterile state and immediately placed in a sterile tube. Approximately 200–500 mg was taken from each tube, frozen in liquid nitrogen for 15 min, and then immediately stored in a refrigerator at −80°C. DNA was extracted from the gut microbes using a PowerFecal DNA isolation kit (MAGEN, Guangzhou, China) following the manufacturer’s protocols. DNA concentration and purity were assessed using a NanoDrop 2000 micro-ultraviolet spectrophotometer (Thermo Fisher Scientific, MA, United States) and quantified with a Qubit fluorometer using a Qubit dsDNA BR assay kit (Invitrogen, CA, United States). DNA quality was checked by running an aliquot on 1% agarose gel. The detection and analysis were performed by Shenzhen Huada Gene Technology Co., Ltd. (Shenzhen, China).
2.7 Gut microbiota 16S rRNA sequencing and data analysis
Variable regions, V3–V4, of the bacterial 16S rDNA gene were amplified with polymerase chain reaction primers, 338F (5′-ACTCCTACGGGAGGCAGCAG-3′) and 806R (5′-GGACT ACHVGGGTWTCTAAT-3′), and the products were purified using Agencourt AMPure XP beads and eluted with the elution buffer. The libraries were qualified using an Agilent Technologies 2100 bioanalyzer (Agilent Technologies, CA, United States) and sequenced using an Illumina HiSeq 2500 platform (BGI, Shenzhen, China), following the manufacturer’s protocols. Then, paired-end reads were generated. The raw reads were filtered and paired-end reads were tagged for clustering into operational taxonomic units (OTUs) with a cutoff value of 97%.
The OTUs representative sequences were taxonomically classified using Ribosomal Database Project Classifier v.2.2 with a minimum confidence threshold of 0.6 and trained on the Greengenes database v201305 using Quantitative Insights Into Microbial Ecology (QIIME) v1.8.0. (United States). Alpha and beta diversities were estimated using Mothur (v1.31.2) (https://www.mothur.org, United States) and QIIME (v1.8.0), respectively. R package 3.4.1 and R package “gg plots” were used to plot various clusters. The Wilcoxon test was used to ascertain significant species with R v3.4.1.
2.8 Serum nontargeted metabolomics analysis
The untargeted metabolomics analysis was conducted using LC–MS/MS. A Q-Exactive high-resolution mass spectrometer (Thermo Fisher Scientific, MA, United States) was used to collect data from both positive ions (pos) and negative ions (neg) to improve metabolite coverage. LC–MS/MS data were processed using the Compound Discoverer 3.1 software (Thermo Fisher Scientific, MA, United States), mainly including peak extraction, peak alignment, and compound identification. Data pre-processing, statistical analysis, metabolite classification annotations, and functional annotations were executed using the metabolomics R package metaX (Shenzhen Huada Gene Technology, China) and the metabolome bioinformatic analysis pipeline. The multivariate raw data were dimensionally reduced using Principal Component Analysis (PCA) to analyze the groupings, trends (intra-and inter-group similarities and differences), and outliers of the observed variables in the dataset (whether an abnormal sample was present). We used partial least squares–discriminant analysis (PLS-DA) to combine the variable importance in projection (VIP) values of the first two principal components of the model with the variability analysis, fold change, and Student t test to screen for differential metabolites. The metabolic pathway enrichment analysis of differential metabolites was performed based on the Kyoto Encyclopedia of Genes and Genomes (KEGG) database. A p value <0.05 was considered statistically significant.
2.9 Statistical analysis
The experimental data were analyzed using SPSS 26.0 and GraphPad Prism 8.0 statistical software. Prior to analysis, normality and homogeneity of variance tests were conducted on the data. When the data met the criteria of normal distribution and variance homogeneity, data were expressed as mean ± standard deviation (SD), and the t-test was employed. In cases where the data did not conform to normal distribution and variance homogeneity, the Wilcoxon rank-sum test was used. A p value <0.05 was considered statistically significant.
3 Results
3.1 Echocardiography and serum cardiac enzymes
Echocardiography results illustrated that the LVEF, LVFS and LVPWs significantly decreased, and LVIDs significantly increased in the DOX-5w group compared with the control, DOX-1w, and DOX-3w groups. Moreover, LVEF, LVFS and LVPWs in the DOX-3w group were significantly lower than those in the DOX-1w group, whereas LVIDs in the DOX-3w group were significantly higher than those in the DOX-1w group (p < 0.05) (Figure 1A). These results suggested that the cardiac function deteriorate with the increase in the accumulation of drugs in mice.
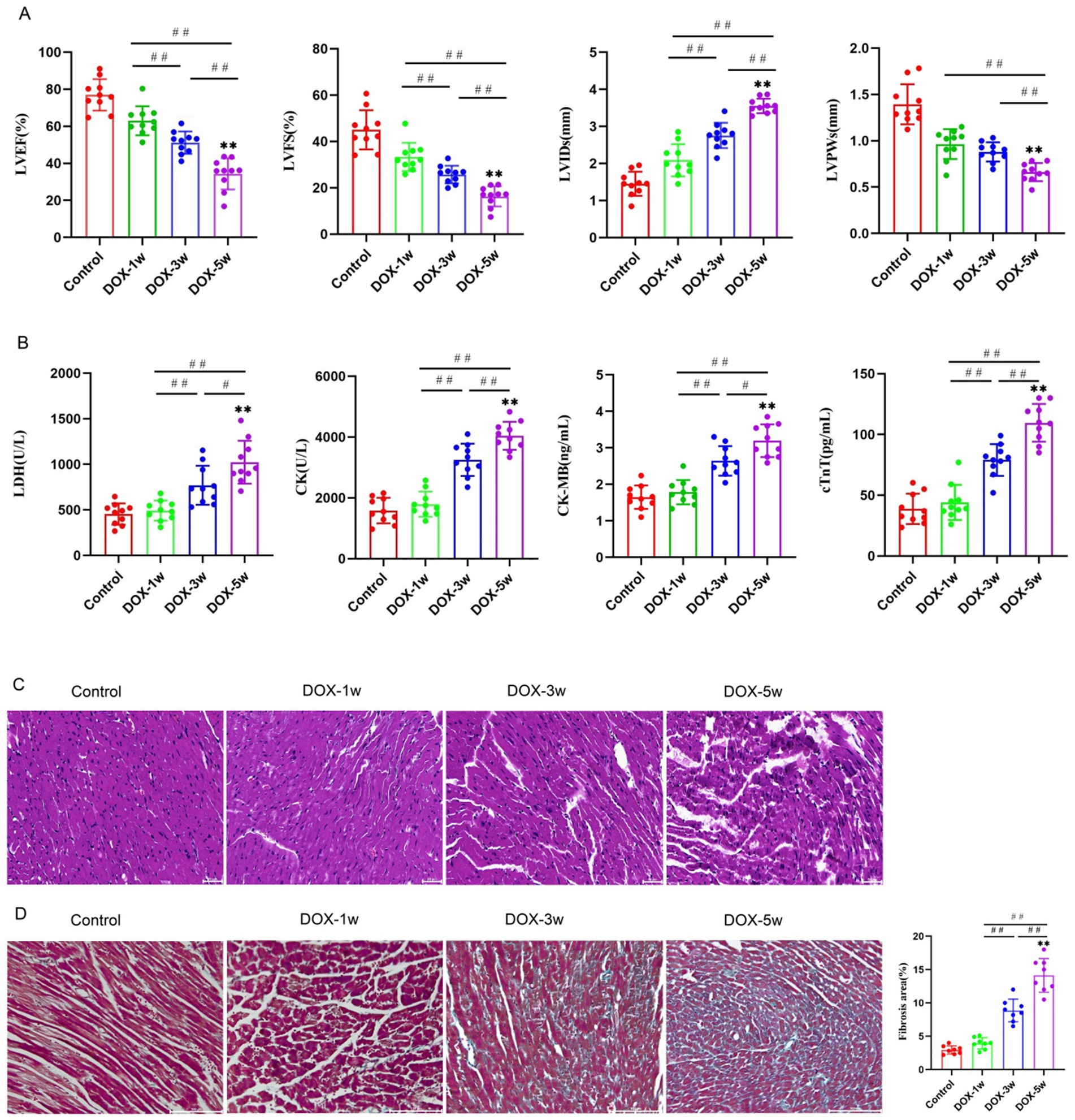
Figure 1. DOX-induced cardiac dysfunction, myocardial necrosis (increase in the levels of myocardial enzymes) and histological injury. (A) Comparison of echocardiography parameters. Effects of DOX on the echocardiography parameters in different groups. n = 10. (B) Serum levels of CK, CK-MB, LDH, and cTnT in different groups. n = 10. (C) Representative photographs of H&E staining (scale bar = 50 μm). (D) Representative photographs and quantitative data of Masson trichrome staining of heart sections (scale bar = 100 μm). n = 8. EF%, Left ventricular ejection fraction; FS%, Left ventricular fractional shortening; LVIDs, Left ventricular internal diameter at end-systole; LVPWs, Left ventricle posterior wall thickness at end-systole. DOX, Doxorubicin; CK, Creatine kinase; CK-MB, Creatine kinase-MB; LDH, Lactic dehydrogenase; and cTnT, Cardiac troponin T. Data are presented as mean ± SD. **p < 0.01 vs. Control group; #p < 0.05, ##p < 0.01 vs. DOX-1w or DOX-3w.
As depicted in Figure 1B, LDH, CK, CK-MB, and cTnT were significantly increased in the DOX-5w group compared with the control group, DOX-1w group and the DOX-3w group (p < 0.05). Moreover, LDH, CK, CK-MB, and cTnT were significantly increased in the DOX-3w group compared with the DOX-1w group (p < 0.05).
3.2 Histological examination of mice heart
HE staining was performed to further clarify the histological changes in the heart caused by DOX. As shown in Figure 1C, we observed that the myocardial fiber of the control group mice were distributed regularly and tightly. The myocardial fiber arrangement was partially disordered in DOX-1w mice. The myocardium was slightly congested, the disorder of myocardial fiber arrangement was serious, edema was visible, and the plasma and fibrin in the vein were exuded in DOX-3w mice. Myocardial congestion was serious, myoplasm was dissolved, myocardial texture was not clear, and obvious swelling, degeneration, or necrosis of myocardial cells could be seen in the DOX-5w mice. Myocardial fibrosis was detected by Masson’s trichrome staining. There was no apparent myocardial fibrosis in the DOX-1w group, but the myocardial fibrosis significantly increased in DOX-3w group compared with the DOX-1w group. The most severe myocardial fibrosis was observed in the DOX-5w group (Figure 1D). The aforementioned results suggested that the cardiac toxicity caused by DOX increased with the increase in the accumulation of drugs in mice, and the DOX-5w group had the most serious myocardial damage.
3.3 Amplicon sequencing results of mice gut microbiota
3.3.1 Analysis of bacterial diversity
Alpha diversity is used to describe the diversity of microbial communities within a sample. No significant differences were observed in alpha diversity between different groups. This suggested no difference in microbial community diversity within samples. Beta diversity is used to describe the similarity or dissimilarity in species composition of gut microbial communities in different study subject groups.
Principal Coordinate Analysis (PCoA) of weighted and unweighted UniFrac distances is used to assess the beta diversity. The unweighted-unifrac-based beta diversity significantly differed between the control and DOX-5w groups (p = 0.0035), the DOX-1w and DOX-3w groups (p < 0.0001), DOX-1 and DOX-5 groups (p < 0.0001), and the DOX-3w and DOX-5w groups (p < 0.0001) (Figure 2A).
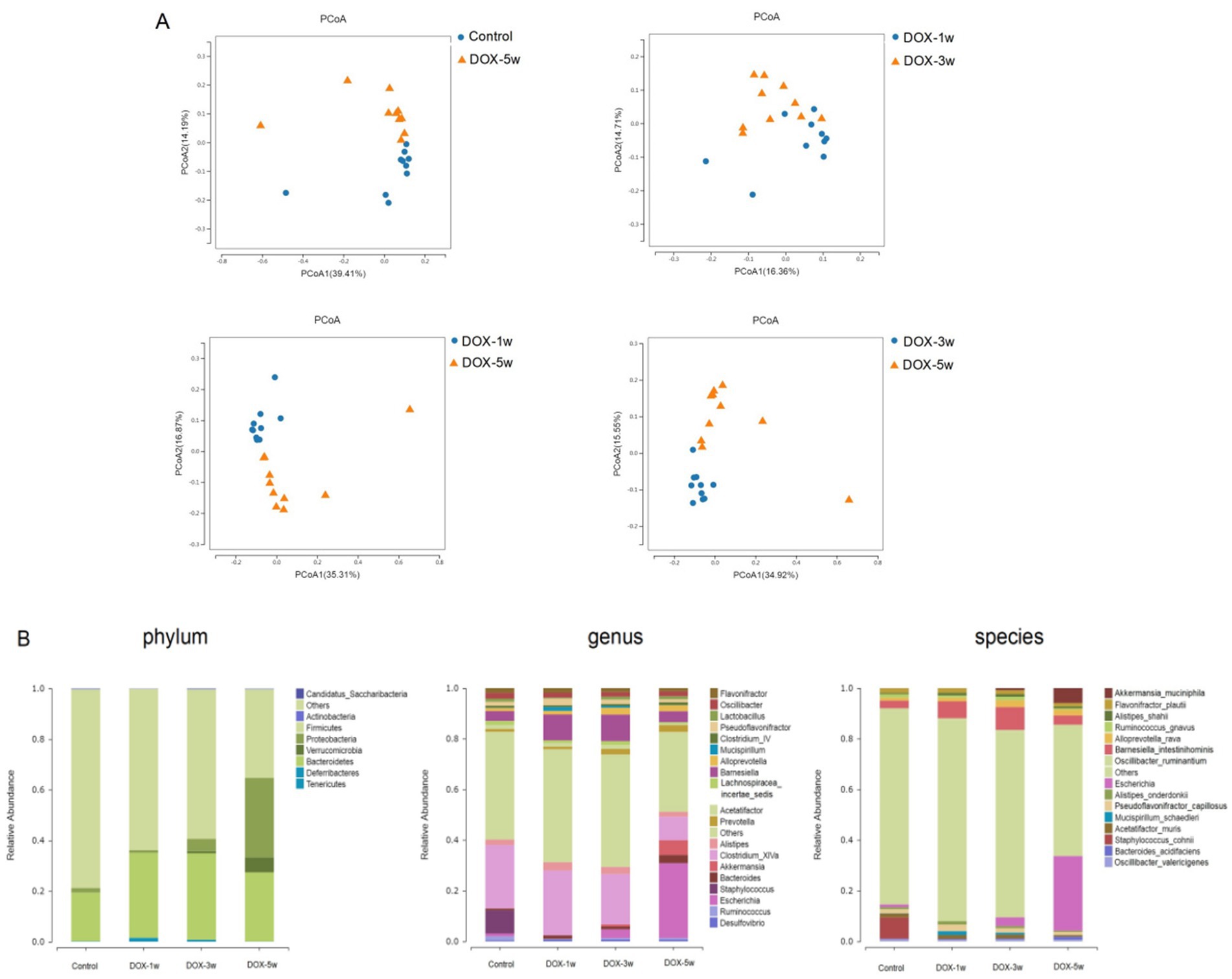
Figure 2. Beta diversity analysis of intestinal flora and species composition distribution. (A) PCoA plot based on the unweighted UniFrac distance of gut microbiota samples from Control vs. DOX-5 group (p = 0.0035), DOX-3 vs. DOX-1 group (p < 0.0001), DOX-5 vs. DOX-1 group (p < 0.0001), DOX-5 vs. DOX-3 group (p < 0.0001). (B) Species composition distribution at the phylum, genus, and species levels in different groups. n = 10.
These results suggested that with the extension of DOX treatment time and the cumulative measurement increased, the difference in the composition of intestinal microorganisms between different DOX treatment groups increased significantly.
3.3.2 Microbial composition analysis
Based on the species annotation analysis of OTUs, we found eight phylum of fecal flora sequence in the control, DOX-1w, DOX-3w, and DOX-5w groups, we found that the dominant phyla of the four groups were identical, which included Firmicutes, Bacteroidota, and Proteobacteria. At the phylum level, the relative abundances of Firmicutes, Bacteroidetes, and Proteobacteria were > 93% in all groups. Firmicutes was most higher in the DOX-1w group (approximately 63.62%), but decreased to 58.99 and 34.92% in the DOX-3w and DOX-5w groups, respectively. In contrast, Proteobacteria increased from 1.75% in the DOX-1w group to 4.81% in the DOX-3w, and even up to 31.41% in the DOX-5w group (Figure 2B).
The species composition bar chart at the genus level shows the top 20 species with the highest abundance (Figure 2B). The genus Prevotella increased from 0.98% in the DOX-1w group to 2.20 and 2.71% in the DOX-3w and DOX-5w groups, respectively, and the genus Bacteroides increased from 1.4 and 1.1% in DOX-1w and DOX-3w groups to 3.1% in the DOX-5w group.
The species composition bar chart at the species level shows the top 20 species with the highest abundance (Figure 2B). The species Bacteroides acidifaciens from 0.38% in the DOX-3w group to 1.10% in DOX-5w group, the species Escherichia from 0.008% in the DOX-1w group to 3.4 and 29% in DOX-3w and DOX-5w groups; While species Akkermansia muciniphila from 0.02% in the DOX-1w group to 0.08% in DOX-3w group, and even up to 5.8% in the DOX-5w group.
3.3.3 Differential analysis of intestinal microflora
Based on the abundance profiles, the features with significant differential abundance across groups were determined using the Wilcoxon rank-sum test. At the phylum level, the abundance of Verrucomicrobia in the DOX-3w group significantly increased compared with the DOX-1w group (p < 0.05). Also, the abundance of Proteobacteria significantly increased in the DOX-5w group compared with the control group and DOX-1w group (p < 0.05), while the abundance of Firmicutes in the DOX-5w group significantly decreased compared with the control group and DOX-1w group (p < 0.05). No difference was found in the DOX-5w group compared with the DOX-3w group. These results showed that the intestinal flora of mice changed significantly at the phylum level with the increase in the DOX dose (Supplementary Table S1).
At the genus level, 12 intestinal microorganisms were screened in the DOX-5w group compared with the control group, among which, the abundance of Bifidobacterium, Romboutsia, Escherichia, Mycoplasma, Turiciactor, Clostridium XVIII, Butyrisimonas, and Clostridium sensu stricto significantly increased, and that of Roseburia, Asaccharacter, Gemmiger, and Peptococcus significantly decreased.
Moreover, at the genus level, compared with the DOX-1w group, one intestinal microorganisms were screened in the DOX-3w group, and the abundance of Clostridium sensu stricto significantly increased in the DOX-3w group. However, 14 intestinal microorganisms were screened in the DOX-5w group compared with the DOX-1w group, among which, the abundance of Turicibacter, Escherichia, Butyricimonas, Klebsiella, Romboutsia, Mycoplasma, Staphylococcus, Bifidobacterium, and Clostridium sensu stricto significantly increased, while Roseburia, Mucispirillum, Butyricicoccus, Clostridium XlVa, and Rikenella significantly decreased. Compared with the DOX-3w group, DOX-5w group screened 6 intestinal microorganisms, among which, the abundance of Turiciactor, Bifidobacterium, Butyricomimonas, Faecalibacterium, Mycoplasma, and Klebsiella significantly increased. These results indicated that the gut microbiota significantly changed at the genus level with the increase in the DOX dose (Supplementary Table S2). Linear discriminant analysis effect size (LEfSe) was used to detect biomarkers (Figure 3).
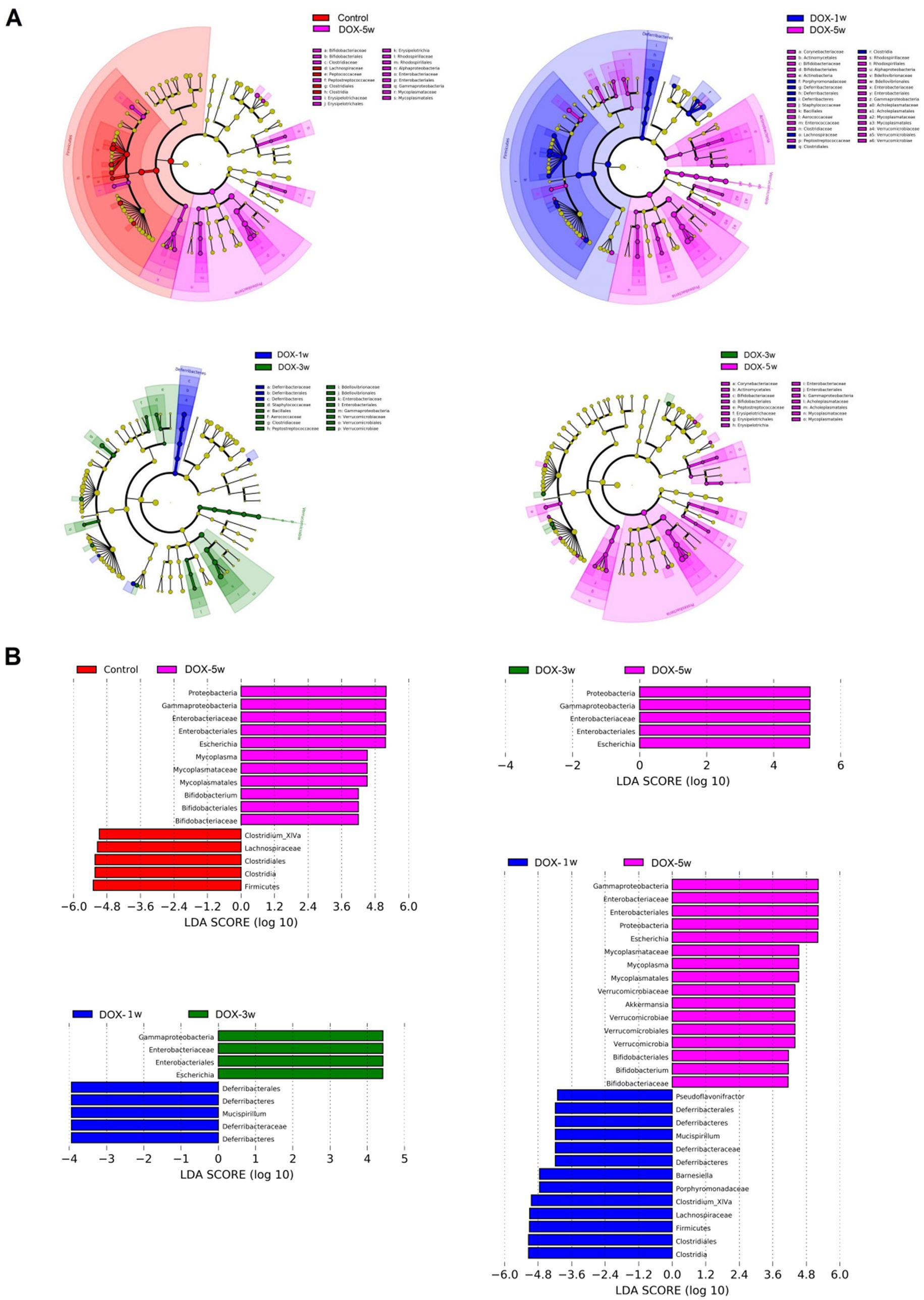
Figure 3. LEfSe analysis between different groups. (A) LEfSe cladogram from phylum to genus levels between different groups. Different color nodes represent the microbiota that are significantly enriched in the corresponding group and have significant differences between the different groups. (B) Histogram analyzed by LEfSe showed that the microbiota with significant differences between the different groups. (LDA score > 4.0). p < 0.05. n = 10. LDA, Linear discriminant analysis; LEfSe, Linear discriminant analysis effect size.
At the species level, nine different bacteria were screened in the DOX-5w group compared with the control group, among which, the abundance of Bifidobacterium pseudolongum, Romboutsia sedimentorum, Escherichia, Mycoplasma hyorhinis, Turicibacter sanguinis, and Clostridium. cocleatum significantly increased and that of Roseburia faecis, Faecalicoccus pleomorphus, and Asaccharobacter celatus significantly reduced.
At the species level, no difference was observed in the DOX-3w group compared with the DOX-1w group. However, 13 intestinal microorganisms were screened in the DOX-5w group compared with the DOX-1w group, among which, the abundance of Clostridium disporicum, Turicibacter sanguinis, Escherichia, Butyricimonas virosa, Romboutsia sedimentorum, Bacteroides caccae, Staphylococcus cohnii, Mycoplasma hyorhinis, Bifidobacterium pseudolongum, and Bacteroides nordii significantly increased, while Roseburia faecis, Mucispirillum schaedleri, and Butyricicoccus pullicaecorum significantly decreased. Compared with the DOX-3w group, the abundance of Turicibacter sanguinis, Bacteroides caccae, Bifidobacterium pseudolongum, Faecalibacterium prausnitzii, and Mycoplasma hyorhinis significantly increased and that of Roseburia faecis significantly decreased in the DOX-5w group (Supplementary Table S3).
3.4 Analysis of untargeted metabolomics results in mice serum
3.4.1 PLS-DA analysis
Partial least squares–discriminant analysis (PLS-DA) uses partial least squares regression to establish the relationship model between metabolite expression and sample category. The analysis results are demonstrated in Figure 4A. Significantly different separation trends of serum metabolites were observed among three groups (DOX-1w group, DOX-3w group, and DOX-5w group), indicating significant differences in metabolites between different treatment groups.
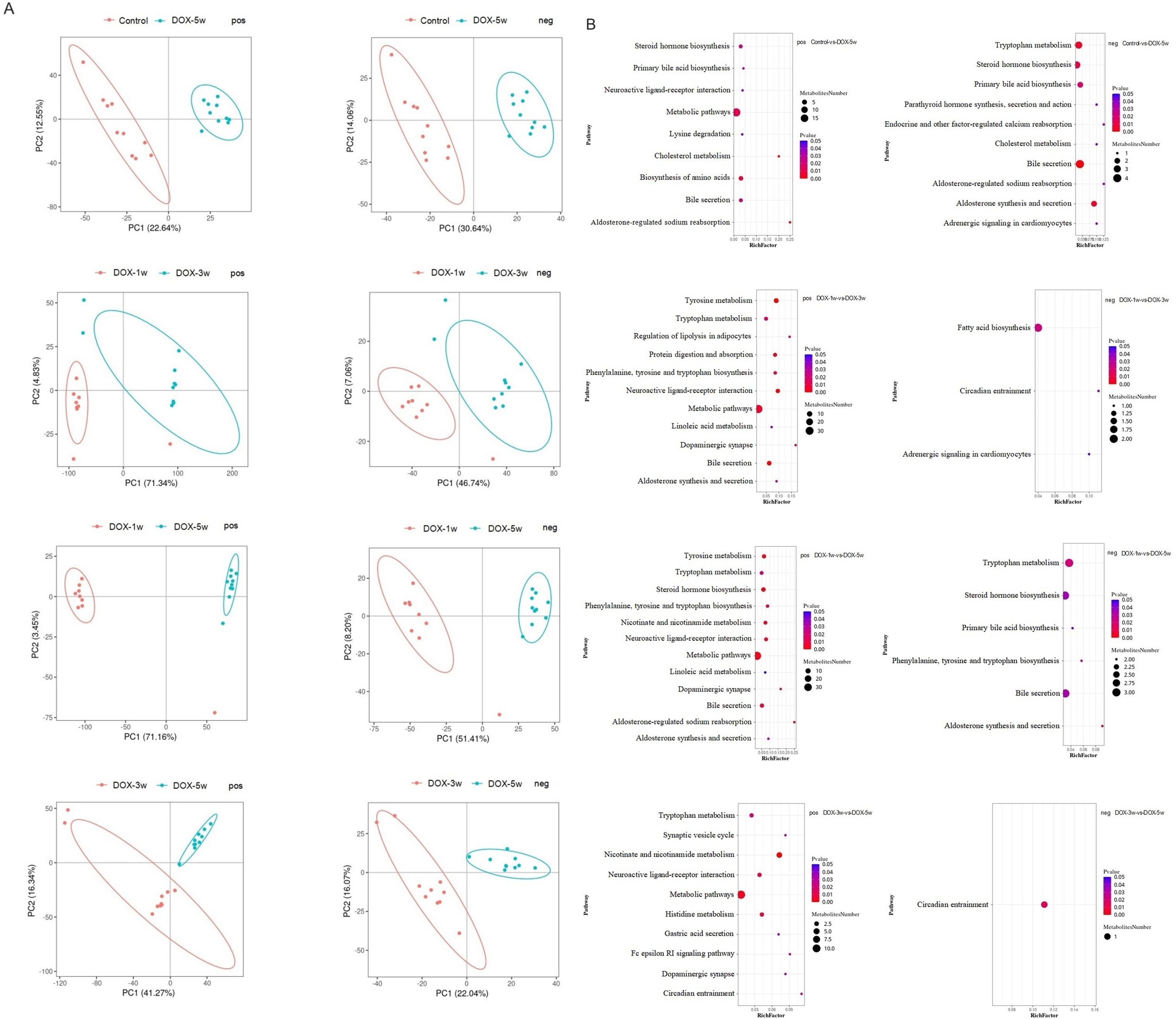
Figure 4. PLS-DA score graph of positive and negative ion modes and metabolic pathway enrichment analysis. (A) PLS-DA score graph of positive and negative ion modes in different groups. (B) Bubble plots for metabolic pathway enrichment analysis. n = 10, p < 0.05. pos, positive ion mode; neg, negative ion mode.
3.4.2 Serum differential metabolites
We conducted nontargeted LC–MS/MS metabolomics analysis on the serum samples from different groups. We observed significantly different metabolite profiles based on the PLS-DA models of metabolite profiling data. A total of 149 substances were upregulated and 219 substances were downregulated in the DOX-5w group compared with the control group, among which, the levels of tauroursodeoxycholic acid (TUDCA), deoxycholic acid (DCA), indole-3-acrylic acid, cystine, and l-histidine significantly increased and that of gluconic acid, aceglutamide, and creatine significantly decreased. Further, 269 substances were upregulated and 348 substances were downregulated in the DOX-3w group compared with the DOX-1w group, among which, the levels of TUDCA, docosahexaenoic acid, l-tryptophan, and d-ornithine significantly increased and that of his-tyr, l-gulonolactone and 3-hydroxysebacic acid significantly decreased. Also, 365 substances were upregulated and 409 substances were downregulated in the DOX-5w group compared with the DOX-1w group, among which, the levels of indole-3-acrylic acid, jasmonic acid, arg-gln, and d-ornithine significantly increased and that of dl-arginine, leu-leu, and sphingosine-1-phosphate (d16:1) significantly decreased. Moreover, 112 substances were upregulated and 51 substances were downregulated in the DOX-5w group compared with the DOX-3w group, among which, the levels of cholic acid, nicotinic acid, and indole-3-acrylic acid significantly increased and that of lysolecithin, prohydrojasmon, and 3,10-dihydroxydecanoic acid significantly decreased (VIP ≥ 1, level ≤ 4, fold-change ≥1.2 or ≤ 0.83, and p value <0.05 were used as the screening conditions for differential metabolites).
We performed receiver operating characteristic (ROC) analysis on the metabolites identified in different groups to find the metabolic biomarkers of varying degrees of HF in serum. The metabolites with predictive value in the control vs. DOX-5w group were as follows: tyrosyltyrosine (AUC = 0.94), aldosterone (AUC = 0.90), DCA (AUC = 0.87), cystine (AUC = 0.86), n-acetyl-L-phenylalanine (AUC = 0.96), trimethylamine N-oxide (TMAO, AUC = 0.93), taurocholic acid (AUC = 0.90), indole-3-acrylic acid (AUC = 0.91), l-histidine (AUC = 0.89), and TUDCA (AUC = 0.81), among others (Supplementary Table S4).
The metabolites with predictive value in the DOX-1w vs. DOX-3w group were as follows: daminozide (AUC = 0.99), lycopsamine (AUC = 0.99), phthalic acid (AUC = 0.97), choline (AUC = 0.94), taurocholic acid (AUC = 0.88), l-tryptophan (AUC = 0.84), and TUDCA (AUC = 0.92), among others (Supplementary Table S5).
The metabolites with predictive value in the DOX-1w vs. DOX-5w group were as follows: d-ornithine (AUC = 0.99), 3-dehydroshikimate (AUC = 0.99), promegestone (AUC = 0.99), l-tryptophan (AUC = 0.91), and indole-3-acrylic acid (AUC = 0.99), among others (Supplementary Table S6).
The metabolites with predictive value in the DOX-3w vs. DOX-5w group were as follows: eslicarbazepine (AUC = 0.98), 4-hydroxybenzaldehyde (AUC = 0.90), indole-3-acrylic acid (AUC = 0.88), and cholic acid (AUC = 0.90) (Supplementary Table S7).
We could accurately distinguish the two groups and predict the disease by screening the metabolites with predictive value.
3.4.3 Metabolic pathway analysis
Based on the KEGG database, metabolic pathway enrichment analysis was performed on different groups. Fourteen metabolic pathways with p value <0.05 were significantly enriched by differential metabolites in the DOX-5w group compared with the control group. These metabolic pathways mainly included aldosterone-regulated sodium reabsorption; biosynthesis of amino acid; bile secretion; primary bile acid biosynthesis; aldosterone synthesis and secretion; tryptophan metabolism; and adrenergic signaling in cardiomyocytes pathways, among others. The differential metabolites involved in these KEGG metabolic pathways mainly included taurocholic acid, l-histidine, cholic acid, glycocholic acid, DCA, aldosterone, corticosterone, corticosterone, and tetrahydrocortisone, among others (Figure 4B; Supplementary Table S8).
Fourteen metabolic pathways with p value <0.05 were significantly enriched by differential metabolites in the DOX-3w group compared with the DOX-1w group. These metabolic pathways mainly included bile secretion; phenylalanine, tyrosine and tryptophan biosynthesis; aldosterone synthesis and secretion; tryptophan metabolism; linoleic acid metabolism; and adrenergic signaling in cardiomyocytes pathways, among others. The differential metabolites involved in these KEGG metabolic pathways mainly included choline, dopamine, cortisol, taurocholic acid, cholate, 3-dehydroquinic acid, l-tryptophan, corticosterone, and arachidonic acid, among others (Figure 4B; Supplementary Table S9).
Thirteen metabolic pathways with p-value <0.05 were significantly enriched by differential metabolites in the DOX-5w group compared with the DOX-1w group. These metabolic pathways mainly included aldosterone-regulated sodium reabsorption; neuroactive ligand-receptor interaction; nicotinate and nicotinamide metabolism; bile secretion; phenylalanine, tyrosine and tryptophan biosynthesis; tryptophan metabolism; and aldosterone synthesis and secretion, among others. The differential metabolites involved in these KEGG metabolic pathways mainly included cortisone, cortisol, dopamine, tyramine, d-(−)-morphine, spermidine, glycocholate, d-(−)-quinic acid, l-tryptophan, 3-dehydroshikimate, corticosterone, desoxycortone, among others (Figure 4B; Supplementary Table S10).
Ten metabolic pathways with p-value <0.05 were significantly enriched by differential metabolites in the DOX-5w group compared with the DOX-3w group. These metabolic pathways mainly included nicotinate and nicotinamide metabolism; histidine metabolism; neuroactive ligand-receptor interaction; and gastric acid secretion, among others. The differential metabolites involved in these KEGG metabolic pathways mainly included histamine; urocanate; melatonin and nicotinic acid, among others (Figure 4B; Supplementary Table S11).
3.5 Multi-omics analysis revealed the relationship between gut microbiota and serum metabolites and cardiac enzymes in DOX-treated mice
We further examined the correlation between intestinal microbiota and serum metabolites to further determine whether intestinal microbes were involved in body metabolism. We performed Spearman correlation analysis on the identified intestinal microbiota and differential metabolites in serum between different groups. The results demonstrated that the differential microorganisms in the gut were significantly correlated with the identified differential metabolites with predictive value for DIHF.
The differential metabolites aldosterone, cholic acid, cystine, DCA, ethyl docosahexaenoate, glycocholate, glycocholic acid, l-histidine, lithocholic acid taurine conjugate, n-acetyl-l-phenylalanine, orotic acid, taurocholic acid, TUDCA, TMAO, trithionic acid, and tyrosyltyrosine were positively correlated with bacterial strains Bifidobacterium pseudolongum, Clostridium cocleatum, Escherichia, Mycoplasma hyorhinis, Romboutsia sedimentorum, and Turicibacter sanguinis, and negatively correlated with Asaccharobacter celatus, Roseburia faecis, and Faecalicoccus pleomorphus in DOX-5w group compared with control group (Figure 5A).
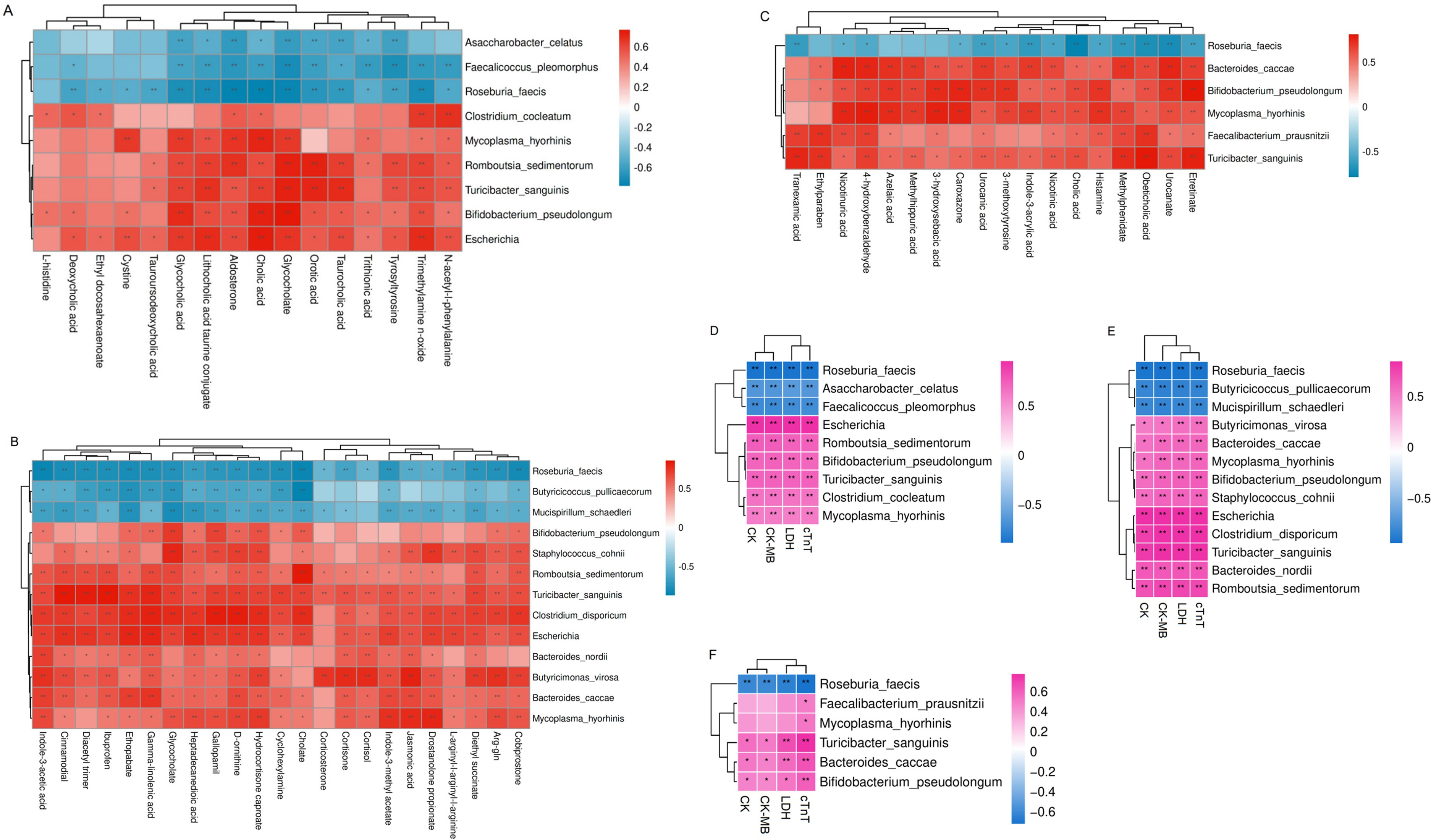
Figure 5. Multi-omics joint analysis. (A) Spearman’s correlation heatmap of gut microbiota and differential metabolites in the DOX-5w vs. Control group. (B) Spearman’s correlation heatmap of gut microbiota and differential metabolites in the DOX-5w vs. DOX-1w group. (C) Spearman’s correlation heatmap of gut microbiota and differential metabolites in the DOX-5w vs. DOX-3w group. (D) Spearman correlation heatmap of gut microbiota and serum levels of CK, CK-MB, LDH, and cTnT in the DOX-5w vs. Control group. (E) Spearman correlation heatmap of gut microbiota and serum levels of CK, CK-MB, LDH, and cTnT in the DOX-5w vs. DOX-1w group. (F) Spearman correlation heatmap of gut microbiota and serum levels of CK, CK-MB, LDH, and cTnT in the DOX-5w vs. DOX-3w group. The color blocks represent the correlation coefficient. The darker the color, the stronger the correlation between the microbial groups and the different metabolites. Red represents a positive correlation, and blue represents a negative correlation. CK, Creatine kinase; CK-MB, Creatine kinase-MB; LDH, Lactic dehydrogenase; cTnT, Cardiac troponin T. n = 10. *p < 0.05, **p < 0.01.
The differential metabolites arg-gln, cholate, cortisol, cortisone, d-ornithine, daidzin, cyclohexylamine, corticosterone, diethyl succinate, drostanolone propionate, ethopabate, gamma-linolenic acid, glycocholate, heptadecanedioic acid, indole-3-acetic acid, indole-3-methyl acetate, jasmonic acid, indole-3-acrylic acid, and l-arginyl-l-arginyl-l-arginine were positively correlated with Bacteroides caccae, Bacteroides nordii, Bifidobacterium pseudolongum, Butyricimonas virosa, Clostridium disporicum, Escherichia, Mycoplasma hyorhinis, Romboutsia sedimentorum, Staphylococcus cohnii, and Turicibacter sanguinis, and negatively correlated with Butyricicoccus pullicaecorum, Mucispirillum schaedleri, and Roseburia faecis in DOX-5w group compared with DOX-1w group (Figure 5B).
The differential metabolites 3-hydroxysebacic acid, 3-methoxytyrosine, 4-hydroxy-3-methoxyphenylglycol sulfate, 4-hydroxybenzaldehyde, azelaic acid, caroxazone, cholic acid, ethylparaben, etretinate, histamine, indole-3-acrylic acid, methylhippuric acid, methylphenidate, nicotinic acid, nicotinuric acid, obeticholic acid, tranexamic acid, urocanate and urocanic acid were positively correlated with Bacteroides caccae, Bifidobacterium pseudolongum, Faecalibacterium prausnitzii, Mycoplasma hyorhinis and Turicibacter sanguinis, and negatively correlated with Roseburia faecis in DOX-5w group compared with DOX-3w group (Figure 5C).
The myocardial necrosis markers LDH, CK, CK-MB, and cTnT were positively correlated with Bifidobacterium pseudolongum, Clostridium cocleatum, Escherichia, Mycoplasma hyorhinis, Romboutsia sedimentorum, and Turicibacter sanguinis, and negatively correlated with Asaccharobacter celatus, Roseburia faecis, and Faecalicoccus pleomorphus in the DOX-5w group compared with the control group (Figure 5D).
The myocardial necrosis markers LDH, CK, CK-MB, and cTnT were positively correlated with Bacteroides caccae, Bacteroides nordii, Bifidobacterium pseudolongum, Butyricimonas virosa, Clostridium disporicum, Escherichia, Mycoplasma hyorhinis, Romboutsia sedimentorum, Staphylococcus cohnii and Turicibacter sanguinis, and negatively correlated with Butyricicoccus pullicaecorum, Mucispirillum schaedleri and Roseburia faecis in DOX-5w group compared with DOX-1w group (Figure 5E).
The myocardial necrosis markers LDH, CK, CK-MB, and cTnT were positively correlated with Bacteroides caccae, Bifidobacterium pseudolongum, Faecalibacterium prausnitzii, Mycoplasma hyorhinis, and Turicibacter sanguinis, and negatively correlated with Roseburia faecis in DOX-5w group compared with DOX-3w group (Figure 5F).
4 Discussion
Our study found that the LVEF, LVFS, and LVPWs significantly decreased, LVIDs significantly increased, and the levels of myocardial necrosis markers increased with the extension of DOX treatment time and the cumulative measurement increased. Our study also found that the abundance of Firmicutes significantly decreased whereas that of Proteobacteria significantly increased in intestine with the prolonged use of DOX and aggravation of myocardial injury in the DOX-5w group. At the genus level, the abundance of Clostridium XVIII, Butyricimonas, and Clostridium sensu stricto in intestine increased significantly with the aggravation of myocardial injury. Further, the abundance of Roseburia, Asaccharobacter, Gemmiger, and Peptococcus significantly decreased. At the species level, no difference in intestinal flora was detected in the DOX-3w group compared with the DOX-1w group. However, the mice developed severe HF, EF further decreased, and intestinal flora disorder was significantly aggravated with DOX dose accumulation (DOX-5w group). Thirteen different bacteria were screened in the DOX-5w group compared with the DOX-1w group, among which, the abundance of Clostridium disporicum, Turicibacter sanguinis, Escherichia, Butyricimonas virosa, Romboutsia sedimentorum, Bacteroides caccae, Staphylococcus cohnii, Mycoplasma hyorhinis, Bifidobacterium pseudolongum, and Bacteroides nordii increased significantly and that of Roseburia faecis, Mucispirillum schaedleri, and Butyricicoccus pullicaecorum decreased significantly. Six different bacteria were screened in the DOX-5w group compared with the DOX-3w group, among which, the abundance of Turicibacter sanguinis, Bacteroides caccae, Bifidobacterium pseudolongum, Faecalibacterium prausnitzii, and Mycoplasma hyorhinis significantly increased and that of Roseburia faecis significantly decreased. These results showed that the myocardial injury was aggravated, and the composition and abundance of the intestinal flora in mice significantly changed with the DOX dose accumulation.
In addition, the serum metabolites of mice significantly changed in different degrees of DIHF. Bile acids play an essential role in regulating energy homeostasis and lipid and glucose metabolism. Studies have found that secondary bile acid levels are closely related to HF, atrial fibrillation, hypercholesterolemia, coronary heart disease, and various metabolic diseases (Mayerhofer et al., 2017). In vitro, the secondary bile acid DCA significantly induced the release of tumor necrosis factor (TNF-α) mRNA from macrophages in a dose-dependent manner (Yan et al., 2021). TUDCA is a conjugated bile acid derivative and has anti-apoptosis and neuroprotective activities (Grant and DeMorrow, 2020). Díaz et al. (2021) found that endoplasmic reticulum (ER) stress inhibitor TUDCA could improve cardiac autonomic balance and reduce the incidence of arrhythmias and abnormal respiratory patterns; that is, it improved cardiac function by inhibiting endoplasmic reticulum stress. TUDCA also alleviated paraquat-induced cardiomyocyte dysfunction (Ge et al., 2010). Our research showed that the levels of DCA and TUDCA showed significantly increased in the DOX-5w group compared with the control group, also, they had a significant predictive value in the DOX-5w group. Moreover, the levels of TUDCA increased more significantly in the DOX-3w group (the early stage of myocardial injury) compared with the DOX-1w group. Further research showed that the DCA and TUDCA were positively correlated with Bifidobacterium pseudolongum, Clostridium cocleatum, Escherichia, Mycoplasma hyorhinis, Romboutsia sedimentorum, and Turicibacter sanguinis, and negatively correlated with Asaccharobacter celatus, Roseburia faecis, and Faecalicoccus pleomorphus in the DOX-5w group compared with the control group. These results suggested that DOX regulated DCA and TUDCA levels by increasing or decreasing the abundance of specific gut bacteria, thus affecting the occurrence and development of HF.
TMAO is a metabolite of intestinal flora. Previous studies have confirmed that TMAO can accumulate in the heart, kidney, or other tissues, and participate in a series of biochemical reactions, including activating platelet aggregation, increasing the formation of foam cells, inducing inflammatory reactions, and so forth. These reactions can accelerate atherosclerosis (Din et al., 2019; Yousuf et al., 2022), cardiac remodeling, and the progression of chronic kidney disease to some extent. Previous studies showed that the intestinal flora related to TMAO metabolism mainly included Anaerococcus, Clostridium, Desulfitobacter, Enterococcus, Streptococcus, and Proteus (Al-Obaide et al., 2017). Our study also found a significant increase in the levels of TMAO in the DOX-5w mice compared with the control group, also, TMAO had a significant predictive value in the DOX-5w group. In addition, we found that TMAO was positively correlated with Bifidobacterium pseudolongum, Clostridium cocleatum, Escherichia, Mycoplasma hyorhinis, Romboutsia sedimentorum, and Turicibacter sanguinis, and negatively correlated with Asaccharobacter celatus, Roseburia faecis, and Faecalicoccus pleomorphus in the DOX-5w group compared with the control group. These results suggested that DOX regulated TMAO levels by increasing the abundance of trimethylamine-producing bacteria, thus affecting the occurrence and development of HF.
The changes in cardiac energy metabolism can aggravate the development of HF, and the decrease in mitochondrial oxidation capacity is the main reason for the energy deficiency of the heart in the HF state. The decrease in glucose and amino acid oxidation and the increase in ketone oxidation and other factors can cause abnormal changes in mitochondrial energy (Lopaschuk et al., 2021). Amino acids are not only the building blocks of proteins but also intermediate metabolites that drive multiple biosynthetic pathways. In our studies, the levels of cystine and l-histidine increased significantly in DOX-5w group compared with the control group, the levels of l-tryptophan increased significantly in the DOX-3w group and DOX-5w group compared with the Dox-1w group, and indole-3-acrylic acid (a tryptophan metabolite secreted by gut microbiota) increased significantly in the DOX-5w group compared with the control, DOX-1w and DOX-3w groups, suggesting that the amino acid synthesis and utilization disorders aggravate with the DOX accumulation. Further our research showed that the intestinal differential bacteria Bifidobacterium pseudolongum and Clostridium cocleatum were significantly correlated with l-histidine, and Bifidobacterium pseudolongum, Escherichia, Mycoplasma hyorhinis and Roseburia faecis were significantly correlated with cystine in DOX-5w group compared with the control group. Moreover, our research showed that the Bifidobacterium pseudolongum, Mycoplasma hyorhinis, Turicibacter sanguinis, Bacteroides caccae, and Roseburia faecis were significantly correlated with Indole-3-acrylic acid in DOX-5w group compared with the DOX-1w group and DOX-3w group, suggesting that the different gut microbiota affected different amino acid metabolism and subsequently myocardial energy metabolism in different degrees of DIHF.
Our studies also showed that different metabolic pathways with significant enrichment of differential metabolites participated in different degrees of DIHF. The KEGG metabolic pathways, such as bile secretion; tyrosine metabolism; neuroactive ligand-receptor interaction; phenylalanine, tyrosine and tryptophan biosynthesis; aldosterone synthesis and secretion; and tryptophan metabolism were significantly enriched by differential metabolites in DOX-3w and DOX-5w groups compared with the DOX-1w group. In addition, the aldosterone-regulated sodium reabsorption; nicotinate and nicotinamide metabolism; steroid hormone biosynthesis; and primary bile acid biosynthesis also were significantly enriched by differential metabolites in DOX-5w group compared with the DOX-1w group, nicotinate and nicotinamide metabolism; histidine metabolism; neuroactive ligand-receptor interaction; and tryptophan metabolism were significantly enriched by differential metabolites in DOX-5w group compared with the DOX-3w. These results showed that different accumulated doses of DOX induced varying degrees of myocardial injury through different enrichment pathways.
5 Conclusion
In conclusion, the intestinal flora and serum metabolic profile changed significantly with the increase in the cumulative dose of DOX. The intestinal flora may participate in different pathophysiological process in DIHF by causing abnormal metabolism and their metabolites downstream signaling pathways. These gut microbiota and serum metabolites can be used as markers of myocardial damage in different degree of DIHF.
Data availability statement
The data presented in the study are deposited in the NCBI Sequence Read Archive repository, accession number: PRJNA1150914.
Ethics statement
The animal experiments in this study was approved by the Institutional Animal Care and Use Committee at Second Hospital of Shanxi Medical University (DW2022070) and conformed to the Guide for the Care and Use of Laboratory Animals published by the US National Institute of Health. The study was conducted in accordance with the local legislation and institutional requirements.
Author contributions
QW: Data curation, Formal analysis, Investigation, Software, Writing – review & editing. ML: Conceptualization, Data curation, Formal analysis, Funding acquisition, Investigation, Methodology, Software, Writing – original draft, Writing – review & editing. TL: Conceptualization, Data curation, Formal analysis, Funding acquisition, Investigation, Methodology, Software, Writing – original draft, Writing – review & editing. LL: Data curation, Formal analysis, Investigation, Software, Writing – review & editing. CW: Formal analysis, Methodology, Software, Writing – review & editing. XW: Conceptualization, Data curation, Formal analysis, Funding acquisition, Investigation, Methodology, Project administration, Supervision, Software, Writing – original draft, Writing – review & editing. SR: Conceptualization, Data curation, Funding acquisition, Investigation, Methodology, Software, Writing – original draft, Writing – review & editing. XZ: Conceptualization, Data curation, Formal analysis, Funding acquisition, Methodology, Project administration, Software, Supervision, Writing – original draft, Writing – review & editing.
Funding
The author(s) declare that financial support was received for the research, authorship, and/or publication of this article. This work was supported by the Research Project of Shanxi Provincial Health and Family Planning Commission (Nos. 2020068), the Key Medical Research Projects of Shanxi Province (Nos. 2021XM09 and 2023XM029), and the Shanxi Medical Science and Technology Innovation Team (Nos. 2020TD01).
Conflict of interest
The authors declare that the research was conducted in the absence of any commercial or financial relationships that could be construed as a potential conflict of interest.
Publisher’s note
All claims expressed in this article are solely those of the authors and do not necessarily represent those of their affiliated organizations, or those of the publisher, the editors and the reviewers. Any product that may be evaluated in this article, or claim that may be made by its manufacturer, is not guaranteed or endorsed by the publisher.
Supplementary material
The Supplementary material for this article can be found online at: https://www.frontiersin.org/articles/10.3389/fmicb.2024.1348403/full#supplementary-material
References
Al-Obaide, M. A. I., Singh, R., Datta, P., Rewers-Felkins, K. A., Salguero, M. V., Al-Obaidi, I., et al. (2017). Gut microbiota-dependent trimethylamine-N-oxide and serum biomarkers in patients with T2DM and advanced CKD. J. Clin. Med. 6:86. doi: 10.3390/jcm6090086
Arai, M., Yoguchi, A., Takizawa, T., Yokoyama, T., Kanda, T., Kurabayashi, M., et al. (2000). Mechanism of doxorubicin-induced inhibition of sarcoplasmic reticulum Ca(2+)-ATPase gene transcription. Circ. Res. 86, 8–14. doi: 10.1161/01.RES.86.1.8
Arcamone, F., Cassinelli, G., Fantini, G., Grein, A., Orezzi, P., Pol, C., et al. (1969). Adriamycin, 14-hydroxydaunomycin, a new antitumor antibiotic from S. peucetius var. caesius. Biotechnol. Bioeng. 11, 1101–1110. doi: 10.1002/bit.260110607
Christidi, E., and Brunham, L. R. (2021). Regulated cell death pathways in doxorubicin-induced cardiotoxicity. Cell Death Dis. 12:339. doi: 10.1038/s41419-021-03614-x
Díaz, H. S., Andrade, D. C., Toledo, C., Schwarz, K. G., Pereyra, K. V., Díaz-Jara, E., et al. (2021). Inhibition of brainstem endoplasmic reticulum stress rescues cardiorespiratory dysfunction in high output heart failure. Hypertension 77, 718–728. doi: 10.1161/HYPERTENSIONAHA.120.16056
Din, A. U., Hassan, A., Zhu, Y., Yin, T., Gregersen, H., and Wang, G. (2019). Amelioration of TMAO through probiotics and its potential role in atherosclerosis. Appl. Microbiol. Biotechnol. 103, 9217–9228. doi: 10.1007/s00253-019-10142-4
Fan, Y., Liang, L., Tang, X., Zhu, J., Mu, L., Wang, M., et al. (2023). Changes in the gut microbiota structure and function in rats with doxorubicin-induced heart failure. Front. Cell. Infect. Microbiol. 13:1135428. doi: 10.3389/fcimb.2023.1135428
Ge, W., Zhang, Y., Han, X., and Ren, J. (2010). Cardiac-specific overexpression of catalase attenuates paraquat-induced myocardial geometric and contractile alteration: role of ER stress. Free Radic. Biol. Med. 49, 2068–2077. doi: 10.1016/j.freeradbiomed.2010.10.686
Grant, S. M., and DeMorrow, S. (2020). Bile acid signaling in neurodegenerative and neurological disorders. Int. J. Mol. Sci. 21:5982. doi: 10.3390/ijms21175982
Huang, J., Wei, S., Jiang, C., Xiao, Z., Liu, J., Peng, W., et al. (2022). Involvement of abnormal gut microbiota composition and function in doxorubicin induced cardiotoxicity. Front. Cell. Infect. Microbiol. 12:808837. doi: 10.3389/fcimb.2022.808837
Kazemian, N., Mahmoudi, M., Halperin, F., Wu, J. C., and Pakpour, S. (2020). Gut microbiota and cardiovascular disease: opportunities and challenges. Microbiome 8:36. doi: 10.1186/s40168-020-00821-0
Liu, H., Chen, X., Hu, X., Niu, H., Tian, R., Wang, H., et al. (2019). Alterations in the gut microbiome and metabolism with coronary artery disease severity. Microbiome 7:68. doi: 10.1186/s40168-019-0683-9
Lopaschuk, G. D., Karwi, Q. G., Tian, R., Wende, A. R., and Abel, E. D. (2021). Cardiac energy metabolism in heart failure. Circ. Res. 128, 1487–1513. doi: 10.1161/CIRCRESAHA.121.318241
Mayerhofer, C. C. K., Ueland, T., Broch, K., Vincent, R. P., Cross, G. F., Dahl, C. P., et al. (2017). Increased secondary/primary bile acid ratio in chronic heart failure. J. Card. Fail. 23, 666–671. doi: 10.1016/j.cardfail.2017.06.007
Rahman, M. M., Islam, F., Or-Rashid, M. H., Mamun, A. A., Rahaman, M. S., Islam, M. M., et al. (2022). The gut microbiota (Microbiome) in cardiovascular disease and its therapeutic regulation. Front. Cell. Infect. Microbiol. 12:903570. doi: 10.3389/fcimb.2022.903570
Wang, A. J., Zhang, J., Xiao, M., Wang, S., Wang, B. J., Guo, Y., et al. (2021). Molecular mechanisms of doxorubicin-induced cardiotoxicity: novel roles of sirtuin 1-mediated signaling pathways. Cell. Mol. Life Sci. 78, 3105–3125. doi: 10.1007/s00018-020-03729-y
Yan, T., Yan, N., Wang, H., Yagai, T., Luo, Y., Takahashi, S., et al. (2021). FXR-deoxycholic acid-TNF-α axis modulates acetaminophen-induced hepatotoxicity. Toxicol. Sci. 181, 273–284. doi: 10.1093/toxsci/kfab027
Yousuf, A., McVey, D. G., and Ye, S. (2022). Relationship between red meat metabolite trimethylamine N-oxide and cardiovascular disease. Heart Mind. 6, 3–9. doi: 10.4103/hm.hm_8_21
Yu, X., Ruan, Y., Huang, X., Dou, L., Lan, M., Cui, J., et al. (2020). Dexrazoxane ameliorates doxorubicin-induced cardiotoxicity by inhibiting both apoptosis and necroptosis in cardiomyocytes. Biochem. Biophys. Res. Commun. 523, 140–146. doi: 10.1016/j.bbrc.2019.12.027
Yu, J., Wang, C., Kong, Q., Wu, X., Lu, J. J., and Chen, X. (2018). Recent progress in doxorubicin-induced cardiotoxicity and protective potential of natural products. Phytomedicine 40, 125–139. doi: 10.1016/j.phymed.2018.01.009
Keywords: doxorubicin, heart failure, gut microbiota, 16S rRNA gene sequencing, metabolomics, multivariate analysis
Citation: Wang Q, Liu M, Liu T, Li L, Wang C, Wang X, Rong S and Zhou X (2024) Alterations in the gut microbiome and metabolism with doxorubicin-induced heart failure severity. Front. Microbiol. 15:1348403. doi: 10.3389/fmicb.2024.1348403
Edited by:
Eugenia Bezirtzoglou, Democritus University of Thrace, GreeceReviewed by:
Dongliang Li, Shandong University, ChinaXiaoyan Liu, Capital Medical University, China
Copyright © 2024 Wang, Liu, Liu, Li, Wang, Wang, Rong and Zhou. This is an open-access article distributed under the terms of the Creative Commons Attribution License (CC BY). The use, distribution or reproduction in other forums is permitted, provided the original author(s) and the copyright owner(s) are credited and that the original publication in this journal is cited, in accordance with accepted academic practice. No use, distribution or reproduction is permitted which does not comply with these terms.
*Correspondence: Shuling Rong, MjMwNjY3MzgxN0BxcS5jb20=; Xiaolin Wang, d2FuZ3hpYW9saW5Ac3htdS5lZHUuY24=; Xuedong Zhou, emhvdXhkQHNjdS5lZHUuY24=