- Medical Readiness Systems Biology, CMPN, WRAIR, Silver Spring, MD, United States
Meta-organisms encompassing the host and resident microbiota play a significant role in combatting diseases and responding to stress. Hence, there is growing traction to build a knowledge base about this ecosystem, particularly to characterize the bidirectional relationship between the host and microbiota. In this context, metabolomics has emerged as the major converging node of this entire ecosystem. Systematic comprehension of this resourceful omics component can elucidate the organism-specific response trajectory and the communication grid across the ecosystem embodying meta-organisms. Translating this knowledge into designing nutraceuticals and next-generation therapy are ongoing. Its major hindrance is a significant knowledge gap about the underlying mechanisms maintaining a delicate balance within this ecosystem. To bridge this knowledge gap, a holistic picture of the available information has been presented with a primary focus on the microbiota-metabolite relationship dynamics. The central theme of this article is the gut-brain axis and the participating microbial metabolites that impact cerebral functions.
Introduction
The total number of resident microbiota, or the “collection of microorganisms living in or on the human body,” (Merriam-Webster, n.d.) marginally exceeds the number of human cells (Sender et al., 2016). A 70 kg adult male is estimated to have 39 trillion bacteria that live with 30 trillion human cells, which includes both nucleated and non-nucleated cells, e.g., red blood cells (Sender et al., 2016). The ratio between bacterial and host cells varies from 1.3 in adult males to 2.2 in adult females (Sender et al., 2016). The higher microbial cellular load in adult females is attributed to a unique and complex ecosystem of microbes colonized in the female genital tract (Punzon-Jimenez and Labarta, 2021) and vagina (Fu et al., 2020; Srinivasan et al., 2022), and this ecosystem alters with the menstruation cycle (Krog et al., 2022) and during pregnancy (Punzon-Jimenez and Labarta, 2021). Hence, it is essential to consider this diverse microbial ecosystem across the genders (Auriemma et al., 2021) to make any inference in this field of study.
The endogenous microbiota of the human body is mostly concentrated inside the intestine. The gut microbial community is predominantly enriched by bacteria (109–1011 cells/g) (Sender et al., 2016; Cani, 2018) and archaea (108–1010 cells/g) (Kim et al., 2020). Another major microbe that colonizes the intestinal lumen is the virus, an intracellular parasite, and the bacteriophage is a major viral species that controls the bacterial diversity in the host (Scanlan, 2017). The bacteria outnumber viruses by approximately 10 to 1, as there are 109–1010 virus-like particles (VLP) per gram of human feces (Shkoporov and Hill, 2019). While the human genome contains approximately 20,000 genes, the hologenome, a combination of the host and resident microbes, contains over 33 million genes (Lloyd-Price et al., 2016). Ninety-three percent of these genes belong to bacteria (Sender et al., 2016), while viruses claim the second largest share, e.g., 5.8% of total DNA (Arumugam et al., 2011). Together, this gut microbiota fosters a balanced ecosystem with its host, and this interactive milieu is the core feature of the meta-organism (Theis et al., 2016) or holobiont concept (Simon et al., 2019). In theory, the meta-organism or holobiont concept is about studying the holistic host-microbiota interactive sphere that includes biology, ecology, and evolution of both host and resident-microbiota. For the present purpose, we will focus on the biology of meta-organisms.
The functional microbiome (Lam et al., 2015; Berg et al., 2020) is constituted by different omics layers linked to the microbe, namely metagenomics, metatranscriptomics, metaproteomics, and meta-metabolomics, often called metabolomics (Zhang et al., 2019; Zou et al., 2019; Salvato et al., 2021). One of the significant operations of a functional microbiome is to maintain a robust crosstalk between the resident microbe and the host’s peripheral tissues, such as the heart, lungs, kidney, and brain. The gut-brain axis, possibly the most studied subject in this context, embodies the bidirectional communication between the host’s brain and gut commensals that control several brain functions, such as neuroinflammation, neurodegeneration, neurotoxicity, and behavioral, emotional, and memory constructs (Mayer et al., 2015; Cryan et al., 2020).
Eubiosis, or the balanced abundance profile of resident microbiota (Iebba et al., 2016; Al-Rashidi, 2022) fosters a symbiotic relationship with the host when the resources available to the host become systematically shared with its resident microbiota. Eubiotic microbial composition controls inflammation and maintains energy homeostasis and a robust gut-brain axis (Lloyd-Price et al., 2016). In contrast, stressful conditions, such as changes in lifestyle or challenges from foreign elements, elevate the host’s demand for the resources, eventually forcing the host and microbiota to compete for resources from a shared pool. Consequently, as the microbial diversity alters, the adaptive and facultative microbiota proliferate, and the overall ecosystem shifts into dysbiosis that could disrupt the host-microbial communication, including the gut-brain axis (Chen and Devaraj, 2018; Dabke et al., 2019; Louis-Jean and Martirosyan, 2019; Cryan et al., 2020; Xu et al., 2020).
Metabolites are the key information hub of meta-organisms since the host-microbiota communication grid, including the gut-brain axis, is built upon the exchange of metabolites (Ramautar et al., 2013; Wishart, 2019; Wachsmuth et al., 2022). Being the intermediate and derivatives of the biological networks in host cells and microorganisms alike, metabolites appear to be the converging node of the ecosystem (Krautkramer et al., 2021). Illustrating this concept, Figure 1A depicts metabolites as the major node of the crosstalk between the host and its resident microbiota. Figure 1B shows the sizes of various metabolite superfamilies; these metabolite superfamilies are linked to the host, environment, resident microbiome, and their interphases. Microbial metabolites are the smallest in number (Krautkramer et al., 2021).
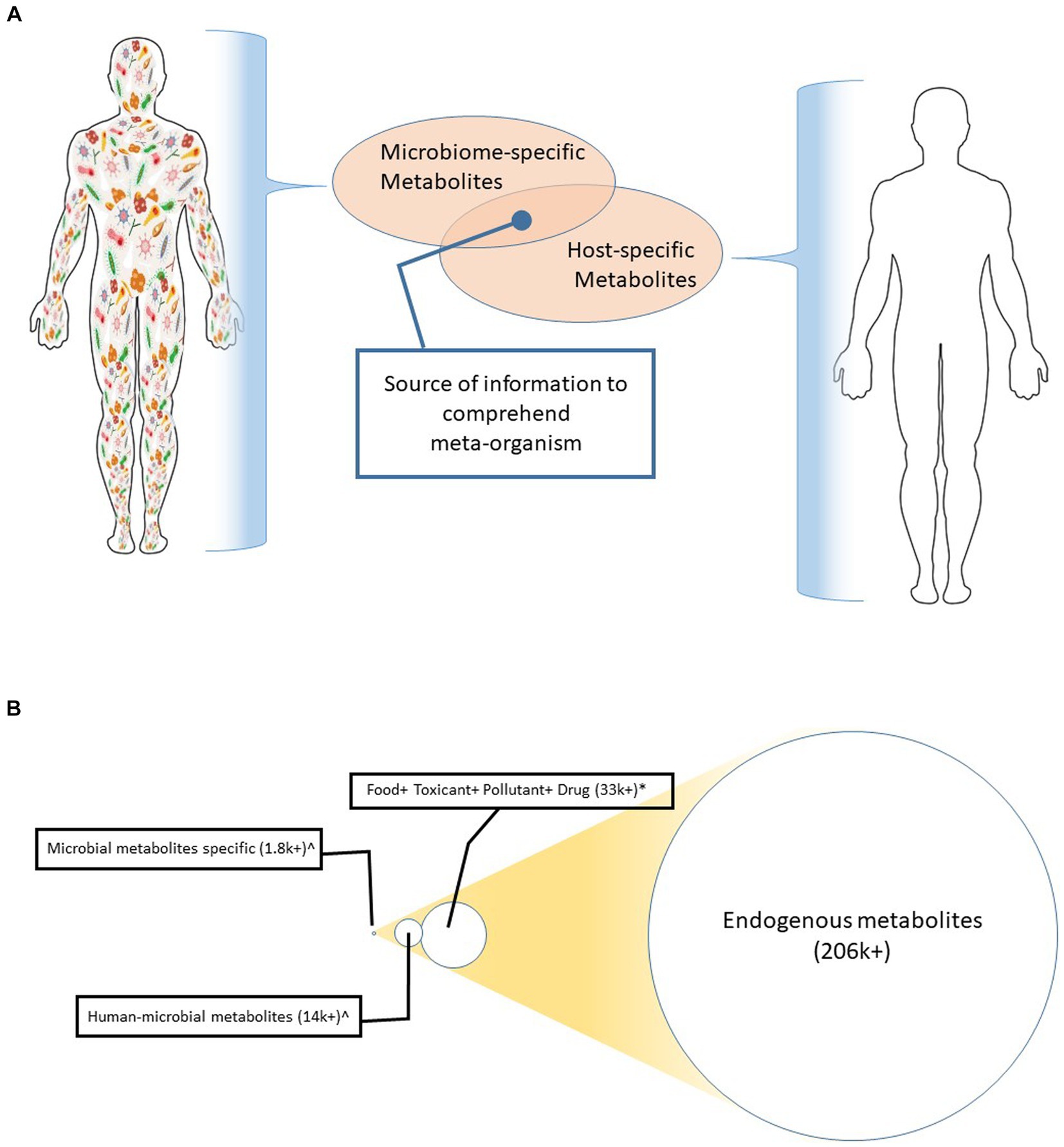
Figure 1. (A) Metabolite as a converging node of multiple kingdoms. This illustration depicts that the host (e.g., human in this case) produces a metabolite cluster. Likewise, the resident microbiota generates another set of metabolite clusters. Characterization of the functional interaction of these two clusters can inform the underlying mechanisms that control the meta-organism. (B) A comparative number of the metabolites generated from humans, the environment, and those microorganisms that colonize in or on humans. The circles are drawn in scale to give an eye estimation of the differences in their abundances.
Rather than curating citations via preset exclusion-inclusion criteria, the present review article selected citations driven by its hypothesis and crafted the review in the following order. The first chapter briefly describes metabolomics, focusing on the available detection tools and pertinent databases to illuminate the metabolites’ association with other omics components. The second chapter describes the resident microbiome, its diversity profile, and how this ecosystem controls the host’s health. The third chapter focuses on the microbial metabolites, e.g., those synthesized by commensals colonized in or on the host. The fourth chapter discusses the gut-brain axis; the potential roles of metabolites and microbiome in supporting this communication framework, and the pathogenesis of the diseases linked to the central nervous system (CNS) are discussed in adjunct. The concluding section highlights the knowledge gap in comprehending meta-organisms and suggests future applications in healthcare and beyond. Significant terms used in this review article are defined in Box 1.
Metabolites: current perspective
Metabolites are the substrate, intermediate, and end product of the biological activities at the cells, tissues, or organ levels (Ramautar et al., 2013; Wishart, 2019). Metabolites are the typical downstream products of the host’s genomic, epigenomic, and proteomic activities. Present capabilities can detect more than 200,000 endogenous metabolites linked to ~1,900 metabolic enzymes encoded in the human genome (Kanehisa and Goto, 2000). In comparison, microbes produce approximately 16,000 metabolites, of which nearly 1,800 are exclusively produced by the microbe community, and the rest are produced by both humans and microbes. In addition, many metabolites are linked to the environment. For instance, ~32,000, ~900, and ~160 metabolites are associated with diet, toxins, and drugs, respectively (Metabolite Statistics, n.d.). Approximately 1 to 3 million xenobiotic metabolites or synthetic products represent drugs, cosmetics, food supplements, pollutants, etc. (Idle and Gonzalez, 2007; Johnson et al., 2012), and many overlap with those linked to the environment. A subset of these metabolites is classified as toxic substances and foreign stimulants to meta-organisms (Gonzalez-Sanchez and DeNicola, 2021).
Emerging knowledge has associated the metabolites’ roles with many biological functions, such as disease pathogenesis (Mamas et al., 2011), immune modulations (Levy et al., 2016), and trans-organ communications (Levy et al., 2016; Frezza, 2017). Indeed, the alterations of biochemical activities due to physiological and psychological stress, exposure to external stimulants, or shifts in diet or lifestyle changes alter the metabolite expressions (Liu et al., 2021) and ultimately influence the overall health of the meta-organism (Milovic et al., 2000; Amaral et al., 2009). Therefore, metabolomics holds time-sensitive information on the molecular activities that co-occur across multiple organisms comprising the host, its resident microbiota, and the overarching environment.
The technical capability of detecting the metabolite has reached a high standard of resolution and robustness in recent years due to the development of cutting-edge tools and highly enriched databases. Table 1 lists the leading tools available to detect and characterize metabolites. Mass spectroscopy-based tools are most popular due to their high throughput and highly sensitive detection capabilities (Dührkop et al., 2021). Recent technological developments in the miniaturization of spectrophotometers have revolutionized their applicability since these tools have become increasingly portable, affordable, and easy to operate in austere conditions (Alseekh et al., 2021). There are supplementary tools available that can further enrich our knowledge of metabolites. For instance, the nuclear magnetic resonance (NMR) spectroscopy can divulge the metabolites’ structures (Wishart et al., 2022). The capability of Raman spectroscopy in detecting metabolites with spatial resolution has recently been adapted to study single-cell metabolomics (Berry and Loy, 2018). A low throughput option, such as histochemistry, presents a unique scope to detect the spatial enrichment of targeted metabolites. The spatial information could be vital in mapping metabolites to the central nervous system (CNS). It can illuminate the differential metabolomic expressions across the blood-brain barrier (BBB). Speculatively, this knowledge can help to understand the roles of metabolites in the gut-brain axis (Mayer et al., 2015).
Development of pertinent databases remains an ongoing effort since the technological capabilities in identifying the metabolites remain a limiting step for constructing the databases. Table 2 lists the leading databases that can help in characterizing the metabolites in three ways. For instance, these databases can (1) find the association of the metabolites to the host’s bio functions, (2) find the association of the metabolites to microbial functions, and (3) facilitate systems integration to link the host and microbiota via metabolite-enriched bio networks and pathways. Of these three types of databases, the host-specific database is possibly at its most mature phase. Part of the reason is that we have yet to fully comprehend the taxonomic determinants of the entire microbial community (Zhu et al., 2019).
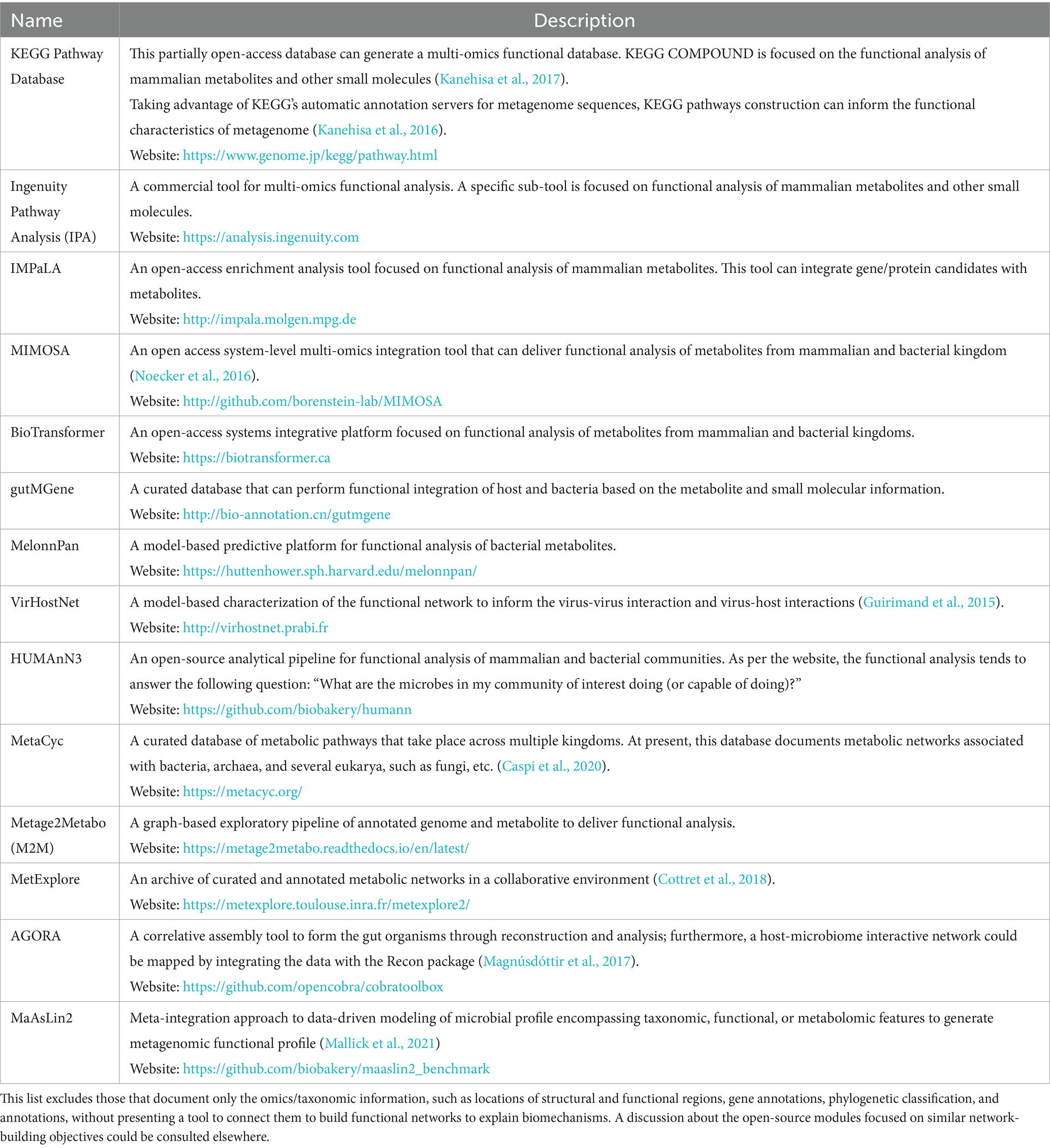
Table 2. Available computation tools for developing predictive functional models using metabolomics and functional metagenomics.
There are mounting efforts to comprehend the biological functions of these metabolites and integrate them across various kingdoms (mammals, bacteria, etc.) and viruses based on their functional and biological relationships. These relationships are typically deduced either by statistical methods (e.g., correlative or enrichment analysis of the co-expressed metabolites) or by garnering the biochemical information (Amara et al., 2022) via curating the available literature that helps linking the metabolites to certain diseases (e.g., carcinoma) or biological networks (e.g., HPA axis abnormalities) based on a priori information. Subsequently, this information converges to build ab initio metabolite network topology (Naake and Fernie, 2019). For instance, the IPA and KEGG pipeline listed in Table 2 statistically integrate a priori knowledge to infer a multi-omics associative matrix (Subramanian et al., 2020) with metabolomics as one of the network layers. Next, this network topology could be mapped across the meta-organism to gain insight. Table 2 lists MIMOSA (Noecker et al., 2016), BioTransformer, and gutMGene (Cheng et al., 2022) databases that can inform about meta-organisms by integrating metabolomics across different kingdoms and organisms. This knowledge can lead us to design therapeutic strategies (Shi et al., 2021).
To summarize this chapter, metabolomics is a key sub-discipline of the pan-omics family (Brestoff and Artis, 2015; Bae et al., 2019; Krautkramer et al., 2021; Spivak et al., 2022), and microbial metabolomics is one of its impactful, though small in size components (Krautkramer et al., 2021). Ongoing efforts aim to link the metabolites to their upstream and downstream regulators that could be potential therapeutic targets (Olivotto et al., 1984; Lee and Finkel, 2013). This information-gathering process needs customization since the expression levels of the metabolites are susceptible to the host’s disease pathology, diet, geographical location, and circadian rhythm (Jones et al., 2021). To elaborate, the abundance of trimethylamine-oxide (TMAO), a liver-oxidized product of gut bacteria-derived TMA, is significantly over-expressed not only by certain disease types, such as type-2 diabetes and hepatic/renal diseases but also among the population who consumes fish-enriched diet (Subramaniam and Fletcher, 2018). Further, the sensitivity of metabolite profile to geographic location is underlined by a cross-continent diversification of breast milk-induced metabolite and microbiota (Gómez-Gallego et al., 2018). The compositions and characteristics of fecal microbiota and corresponding metabolites are highly susceptible to many indigenous and exogenous factors. Hence, the accuracy and reproducibility of detecting fecal metabolites critically depend on the study design and sampling protocol (Weinstock, 2012; Scherz et al., 2022). Potentially optimized protocols for fecal sample collection are discussed here (Mathay et al., 2015; Vogtmann et al., 2017; Jones et al., 2021). It is possibly essential to longitudinally collect fecal samples (Jones et al., 2021; Zheng et al., 2022) since it is unlikely that a single sampling of fecal materials can accurately represent the dynamic nature of microbiota.
All these factors should be considered to understand the true impacts of metabolites. In conclusion, systems knowledge integration could be the key to elucidating the relationship dynamics among different kingdoms, ecosystems, and the longitudinal profile of functional microbiota. The following chapters illuminate how metabolites play key roles in determining functional metagenomics—the bidirectional relationship between the microbiota and the host.
Microbial ecosystem: diversity profile and disease pathogenesis
The hologenome encompasses nearly 1,600 times more microbial genes than the host genome (Lloyd-Price et al., 2016). This estimation alone can underscore the inherent complexity of meta-organism, which thrives on a symbiotic bidirectional relationship between host and resident microbiota. This host-microbiota crosstalk is shaped by and contributed to the host and its resident microbe’s coevolution, synchronized interactions with foreign elements, commensalistic association, and ecological or mutualistic collaboration (Ogunrinola et al., 2020); and its cumulative impacts are manifested in the microbial diversity profile (Manor et al., 2020). Therefore, it is contemplated that the quantitation of microorganismal diversity could throw light on disease etiology.
The estimation of microbial diversity primarily depends on the following two factors: richness (measures the number of independent species) and evenness (quantifies the relative abundances of different species). Alpha diversity measures the evenness and richness of microbial profiles within a community (Baczkowski et al., 1998). The routines, namely the Shannon diversity, Simpson diversity, and Chao 1 quantify different features of alpha diversity (GitHub, n.d.). Shannon diversity estimates the effective number of species colonized in a particular community, hence quantifying both evenness and richness (with a weight to evenness) of the microbial profile. Chao 1 estimates the number of species or the total richness of a particular community. Simpson diversity is primarily a dominance index, as this estimation gives more weight to the common or dominant species. On the other hand, beta diversity is the characteristic of the trans-community microbial profile (Baczkowski et al., 1998). The unweighted Unifrac quantifies the presence or absence of different taxa across the communities, whereas the weighted Unifrac considers the abundance of different taxa. The Bray–Curtis index estimates the abundance-based dissimilarity across the communities, while the Jaccard index measures the occurrence (presence vs. absence)-based diversity across the communities (GitHub, n.d.).
A shift from a balanced ecosystem or the dysbiotic ecosystem could be attributed to diet and lifestyle alterations (Ghosh et al., 2013), age (Mariat et al., 2009), obesity (Magne et al., 2020), circadian rhythm (Thaiss et al., 2014, 2015), and disease pathologies, including cancer (Sheflin et al., 2014; Biragyn and Ferrucci, 2018), cardiovascular disorder (Lau et al., 2017), immune dysfunction (de Oliveira et al., 2021), and several psychological illnesses (Sarkar et al., 2018; Parker et al., 2020). For instance, a reduced alpha diversity of gut microbiota was found in young adults (mean age: ~13 years) with attention-deficit hyperactivity disorder (ADHD). The measurement was estimated by Shannon diversity and Chao 1 index, which likely indicated a diminished richness of gut microbe linked to ADHD (Prehn-Kristensen et al., 2018). A contrasting picture emerged from an independent younger (mean age ~8 years) cohort, where Shannon diversity and Chao 1 index of subjects with ADHD emerged higher than that of the healthy baseline (Wang L. J. et al., 2020). These studies highlighted how multiple factors concurrently influence the microbial diversity.
Often, a combinatory analysis of alpha and beta diversity metrics is used to characterize the holistic changes in the microbial ecosystem. For instance, the alpha diversity in the fecal microbiota of cervical cancer patients showed no differences. Still, the beta diversity measured by the weighted Unifrac and Bray–Curtis algorithm revealed a significant difference that underscored a shift in trans-community microbial abundance, but not within a community (Wang Z. et al., 2019). Similarly, physiological stress caused significant alpha and beta diversity in gut microbes among young men. The Shannon dissimilarity and Chao 1 index suggested a shift in microbial richness within a community, while the Bray–Curtis analysis suggested a trans-community shift (Karl et al., 2017).
With the advancement of high-resolution detection technologies, we can now probe individual community members of the gut commensals. The abundance profile of a single microbe or its associative abundance profile with neighboring commensal(s) can deliver highly precise information. For instance, the shifting ratio of Bacteroidetes and Firmicutes has been linked to age (Mariat et al., 2009), obesity (Magne et al., 2020), and so forth. Linking the microbial abundance profile with circadian rhythm, the relative abundance of Lactobacillus was reported to escalate during the resting phase than during the active phase (Thaiss et al., 2014).
There is a growing appreciation for using the microbial diversity profile to develop the next generation intervention strategy. The torchbearer of the success story is the fecal microbiota transplantation (FMT) method that stalled tumor growth (Riquelme et al., 2019), ameliorated cardiovascular illness (Hu et al., 2019), and eliminated pathogenic insults (Hui et al., 2019) by maneuvering the microbial diversity. However, the potential of FMT as a treatment option is possibly limited due to the concerns about this intervention method’s traceability, safety, and standardization process (Osman et al., 2022; Vaughn et al., 2023). Driven by the hypothesis that the hypoxic condition inside the tumor is favorable for anaerobic microorganisms, systematic colonization of anaerobic bacteria successfully arrested the growth and metastasis of tumor cells (Drozdz et al., 2020). Further, the knowledge of dysbiosis helped to customize the diet supplements for immunotherapy to treat carcinoma (Routy et al., 2018).
Systematic manipulation of microbial colonies has emerged as a potential therapeutic option to combat several ailments; nevertheless, a comprehensive understanding of its molecular underpinnings is warranted to make this intervention process robust and effective. In this context, the next chapter highlights the microbial metabolites, which could play a critical role in designing a therapeutic strategy based on microbes. Once integrated with host metabolites, this knowledge could illuminate the biological underpinnings of their symbiotic relationship and lead to novel therapeutic options.
Microbial metabolites and their spectrum of bio functions
In a homeostatic condition, the expression level of microbial metabolites is controlled by the host’s genetic predisposition, age (Connell et al., 2022), and other environmental factors, such as geographical location, food habits, and various lifestyle traits (Gupta et al., 2020; Krautkramer et al., 2021). Interestingly, there are less than two thousand microbial-specific metabolites in human compared to more than two hundred thousand host metabolites. However, the size of the microbial genome far outnumbers the human genome (Lloyd-Price et al., 2016; Metabolite Statistics, n.d.). A small number of microbial metabolites is potentially attributed to a rather streamlined metabolic function performed by the microorganisms. Ahmed et al. (2022) and Krautkramer et al. (2021) extensively reviewed these microbial-derived metabolites and reported how their biological functions reach various peripheral tissues to ensure the host’s health and fitness.
Shifting microbial diversity from its eubiotic state is effectively mirrored by the microbial metabolite profile. Therefore, multiple ongoing efforts aim to manipulate the microbial metabolites to improve host’s health; however, these undertakings meet a significant challenge due to the lack of pertinent knowledge (Entwistle et al., 2019; Brunet et al., 2020). After screening several available literature (Yano et al., 2015; Rath et al., 2017; Fung et al., 2019; Wang S. P. et al., 2020; Krautkramer et al., 2021; Mou et al., 2021; Otaru et al., 2021; Ahmed et al., 2022), a bacteria-metabolite relationship network (Figure 2) was developed. This network informs the biological sites (e.g., bacteria) where a particular family of metabolites is synthesized. It is important to note that these networks are primarily built upon a priori knowledge; hence, the validity of such networks depends on continuous cross-checking of the literature and experimental feedback (Amara et al., 2022). Secondly, similar associative networks linking metabolites with other organisms, such as viruses and archaea, are essential to fully characterize the microbial metabolites’ functional outreach, and this aspect is yet underdeveloped. Table 2 presents the databases that aim to bridge this knowledge gap, and Figure 2 maps these microbial metabolites to their bacterial source.
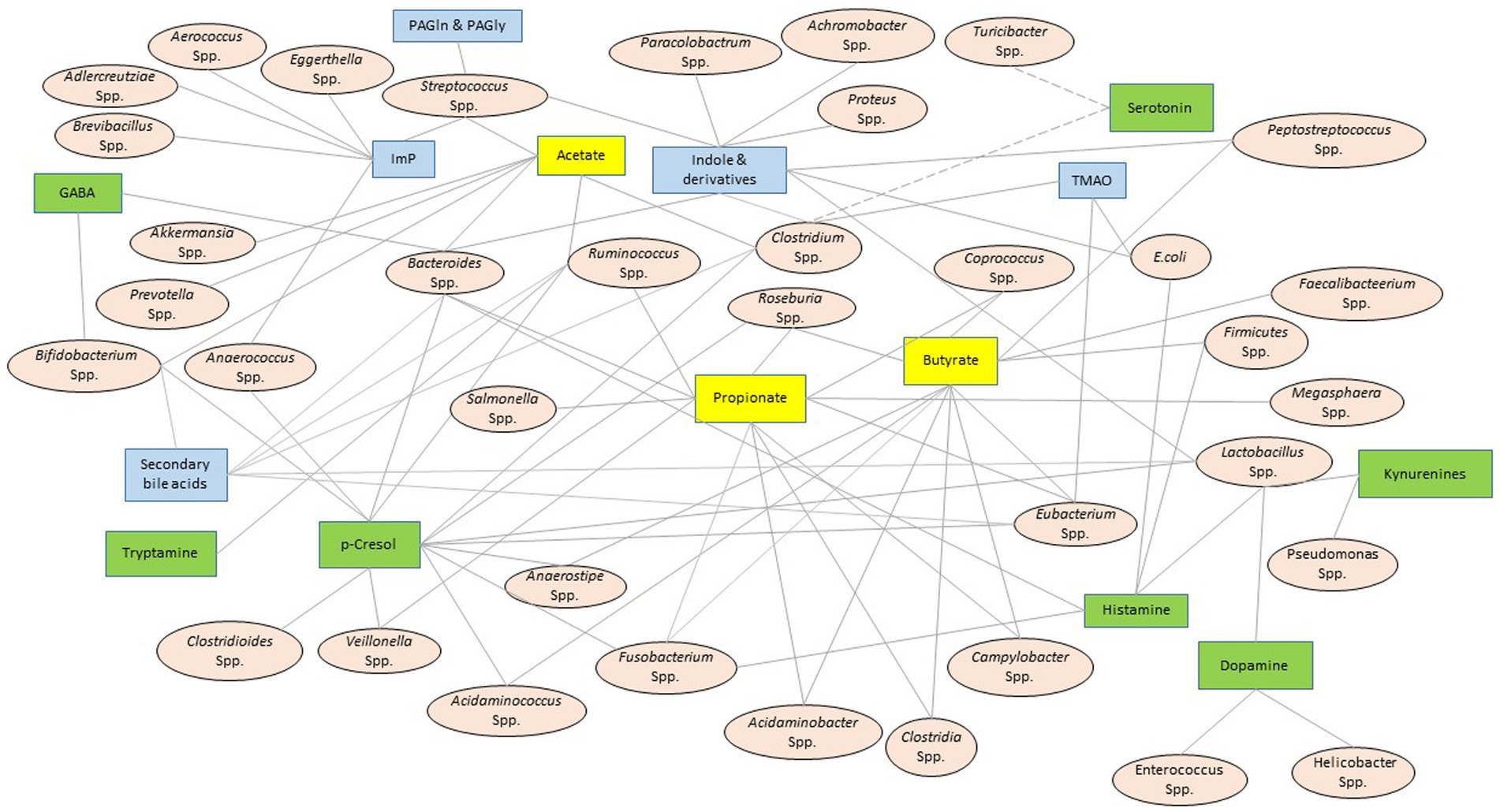
Figure 2. Bacteria-metabolite relationship network. The oval and square-shaped nodes represent the resident bacterial genera or species and the metabolite families, respectively. The solid edges connect the bacterial species or genera to their secretary metabolites. A set of broken edges that converge to serotonin connect it to those bacterial species that demonstrated correlative shifts in abundance, although there is no direct evidence of serotonin production from these bacteria (Yano et al., 2015; Fung et al., 2019). A double solid line connected GABA to Bacteroidetes to suggest that Bacteroidetes encodes an enzyme to produce GABA (Otaru et al., 2021). This network system was constructed after consulting a host of published literature (Pokusaeva et al., 2017; Rath et al., 2017; Wang et al., 2020; Krautkramer et al., 2021; Mou et al., 2021; Ahmed et al., 2022). The yellow, green, and blue boxes represent the metabolites linked to small chain fatty acids (SCFA), amino acids and their derivates, and others, respectively. GABA, γ-aminobutyric acid; TMA, trimethyl amine; Imp, imidazole propionate; PAGln, phenylacetylglutamine; PAGly, phenylacetylglycine.
Secondary bile acid (SBA) and short-chain fatty acids (SCFAs) are two major microbial metabolites in human. High-fat diets trigger the biosynthesis of SBA in the gut lumen (Ridlon et al., 2016; Zeng et al., 2019). Deoxycholic acid and lithocholic acid are two important SBAs produced by the dehydroxylation of primary bile acids (Ridlon et al., 2016; Zeng et al., 2019). In addition, gut microbiota epimerizes and oxidizes the primary and secondary bile acids to produce SBAs of iso-, alo-, oxo- and keto- families, which are comparatively low expressed entities, and their functional attributes are yet to be fully comprehended (Wahlstrom et al., 2016).
SCFAs are primarily comprised of acetate, propionate, and butyrate in an approximate molar ratio of 60:20:20, respectively, in a healthy host. Additional low-abundant SCFAs include fumarate, succinate, lactate, and pyruvate, which are used for cross-feeding among the different microbes (Macfarlane and Macfarlane, 2003; Silva et al., 2020). Anaerobic fermentation of non-digestible carbohydrates is the primary course of SCFAs production, as the pentose phosphate pathway and the Embden–Meyerhof–Parnas Glycolytic pathway catabolize five-carbon (e.g., xylene, pectins) and six-carbon (e.g., fructose, sucrose, starch, cellulose, etc.) substrates, respectively, to produce SCFA (Krautkramer et al., 2021). Briefly, the common end-product of the pentose phosphate pathway and Glycolytic pathway is phosphoenolpyruvate, which converts to pyruvate by endothermic cyclic conversion between NADPH and NAD+. Pyruvate is essentially the upstream substrate of all major SCFAs. For instance, the acetate and butyrate are produced from pyruvate via acetyl Co-A intermediate, with carbon dioxide and ethanol being the major byproducts, and propionate is generated via the succinate pathway utilizing carbon dioxide as the major co-factor (Fernandez-Veledo and Vendrell, 2019). Lactate and formate are additional downstream products from pyruvate metabolism. The production of these metabolites in microbes is typically controlled by access to dietary resources and stress factors. For instance, acetate and lactate are typically produced when carbohydrates are in excess; on the other hand, limited energy to the microbial community generally escalates the synthesis of propionate (Macfarlane and Macfarlane, 2003).
The scarcity of carbohydrates and/or high colonic pH induces the generation of SCFAs via protein fermentation (Neis et al., 2015). In addition to SCFAs, the protein fermentation in microbes produces folates, phenols, and indoles. Catabolism of branched-chain amino acids, such as leucine, isoleucine, and valine, produce isobutyrate and isovalerate, which are usually accumulated in low concentrations (Macfarlane and Macfarlane, 2003; Silva et al., 2020). Kynurenines and serotonin are the major products of the catabolism of tryptophan, an essential amino acid (Ostapiuk and Urbanska, 2022). Nearly 90% of the serotonin in the human body is produced by the colon, particularly the enterochromaffin cells on colonic epithelia. Currently, there is no direct evidence that microbiota produces serotonin, although such a possibility cannot be overruled since the microbiome encodes some contigs that typically contribute to the serotonergic network (Yano et al., 2015; Fung et al., 2019). In addition, a reduced concentration of serotonin was reported in concurrence with an increased abundance of tryptophan in germ-free mice, which indirectly suggests a microbial influence on serotonin production (Strasser et al., 2016). Another essential amino acid, namely histidine, undergoes decarboxylation in various bacterial species to produce histamine, and this recent discovery could have great potential in the field of allergic and immune therapy (Mou et al., 2021). Non-essential amino acids, such as tyrosine and L-dopa, and SCFAs, particularly butyrate, are metabolized in various bacterial species to produce dopamine, a key modulator of the gut-brain axis (Villageliu and Lyte, 2018).
Microbial metabolites could have beneficial or toxic roles depending on the metabolites’ expression levels and the target organ. For instance, low expression of SBAs (5–50 μM) promotes proliferation and invasiveness of colon cancer cells. Still, at higher expression levels (>50 μM), SBAs inhibit the colonic cell cycle and activate cancer cell apoptosis (Milovic et al., 2000; Amaral et al., 2009). Kynurenic acid operates differentially in different organs; in the CNS, kynurenic acid acts as a neuroprotective agent but inhibits insulin synthesis in the liver and kidney (Ostapiuk and Urbanska, 2022). SCFAs have various functions in bioenergy production, maintaining gut integrity, and promoting anti-inflammation via reactive oxygen species production (Tan et al., 2014). On the other hand, TMAO, a phosphatidylcholine derivative, is linked to oxidative stress, hyperlipidemia, and pro-inflammation (Agus et al., 2021).
In this context, there is growing traction about how gut microbiota communicate with peripheral organs. For instance, the dysbiosis of gut commensal has been linked to pulmonary health and asthma (Hufnagl et al., 2020), liver immunology (Trebicka et al., 2021), kidney failure (Zaky et al., 2021), and multiple cancer pathogenesis (Alexander and Turnbaugh, 2020; Sánchez-Alcoholado et al., 2020; Zheng et al., 2020; Kandalai et al., 2023; Xia et al., 2023). Indeed, an in vivo model suggested how microbial metabolites are associated with circadian rhythm and its disruption (Tahara et al., 2018). The roles of microbial metabolites in bioenergy production, somatic inflammation (Alexander and Turnbaugh, 2020), and physiological performances (Borrego-Ruiz and Borrego, 2024) have been studied extensively. Because psychological issues have become a prevalent health concern in the modern world, the study on the gut-brain axis is at the epicenter of the field of host-microbiome study. Figure 3 illustrates the functional association of these microbial metabolites to various brain diseases and co-morbidities, and the next chapter is focused on the gut microbiome-metabolite-brain axis.
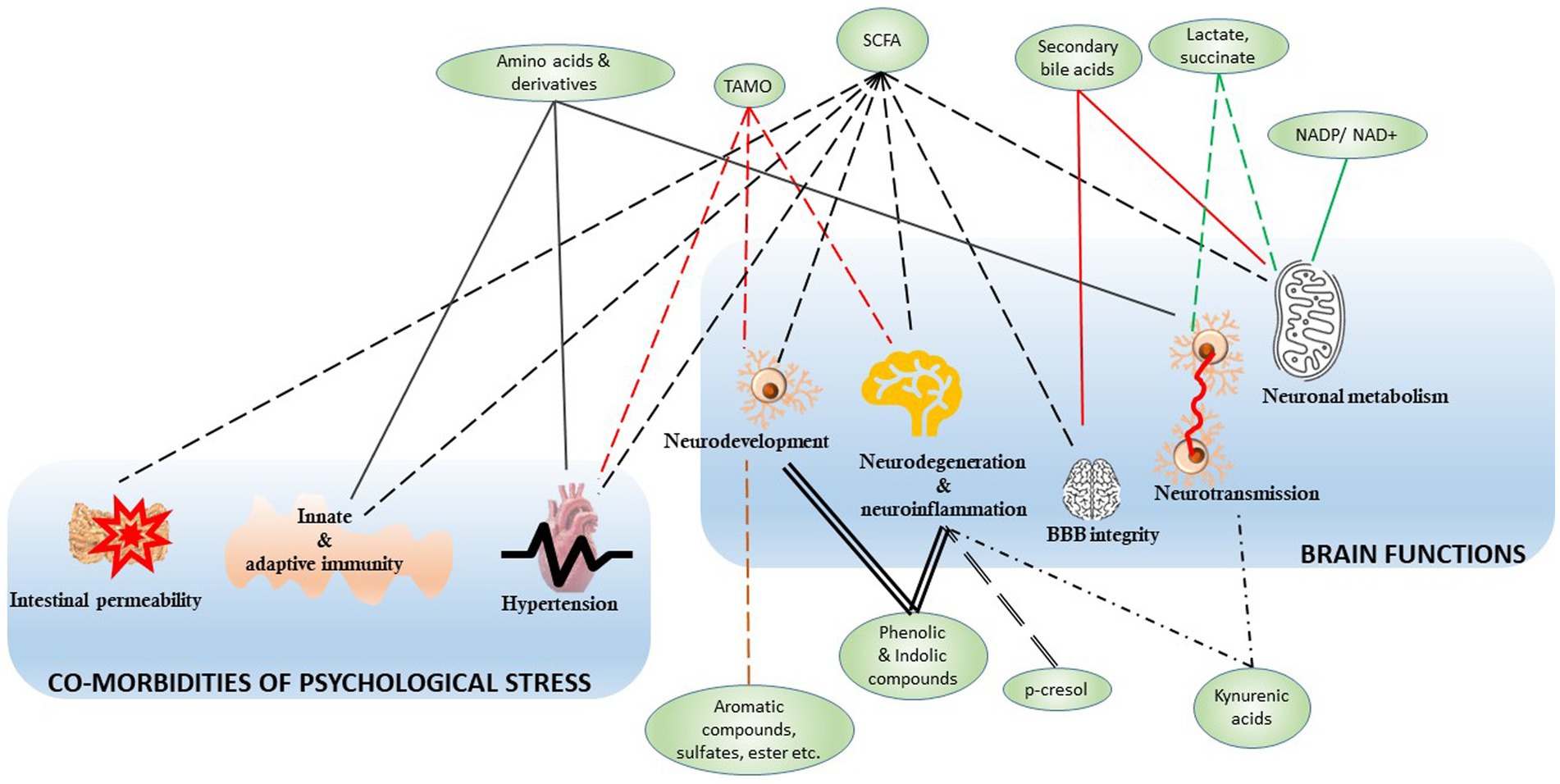
Figure 3. The microbial metabolites and associated brain functions and co-morbidities. The green nodes represent the microbial metabolites mapped in Figure 2 to associate with their generators, like the bacterial genera and species. The edges represent the connection between the metabolite and various functions, and all the edges were crafted differently to highlight their distinct connective modalities. The functions are clustered into two groups, namely, the brain functions and the co-morbidities of psychological stress. SCFA, small chain fatty acids; TMAO, trimethylamine N-oxide; NADP, nicotinamide adenine dinucleotide phosphate; NAD+, nicotinamide adenine dinucleotide.
Gut microbiota-metabolite-brain axis
The gut microbiota-brain axis is a feedback-controlled circuit that recruits metabolites as the primary messenger between the gut and brain (Mayer et al., 2015; Ahmed et al., 2022). Due to the limited access to the human brain, the concept of the gut-brain axis has thus far been primarily built upon animal models. Mounting evidence shows that the gut microbiota-metabolite-brain axis controls the neurophysiology and signaling networks and regulates a spectrum of behavioral constructs. The association between the resident commensals and cerebral health was suggested by interrogating a colony of germ-free mice that demonstrated inhibited expression of tight junction proteins on BBB coupled with escalated BBB permeability (Braniste et al., 2014; Parker et al., 2020). Similarly, longitudinal antibiotic treatment reduced the abundance of gut commensals in mice, and this cohort demonstrated a cognitive deficiency and memory loss, as well as diminished neurogenesis in the hippocampus, the primary brain region to process memory (Fröhlich et al., 2016). Increased activity of a major component of the sympathetic nervous system in germ-free mice, namely the hypothalamic-pituitary-adrenal (HPA) axis, potentially orchestrated elevated Bdnf gene expressions (Manca et al., 2020) and accumulation of corticosterone in the bloodstream (Neufeld et al., 2011). Linking the gut commensal to social skill development, Desbonnet et al. (2014) reported that the germ-free mice showed increased social avoidance and self-grooming along with decreased social engagement. This negative outcome was reversed by administrating probiotic diets that helped growing the commensals in the host (Desbonnet et al., 2014; Fröhlich et al., 2016).
Differential microbial signatures, such as a shift in the diversity of the entire ecosystem (Uronis et al., 2011; Prehn-Kristensen et al., 2018; Tran et al., 2019; Wang L. J. et al., 2020) or a change in the comparative abundance of selected taxonomic groups (e.g., Firmicutes and Bacteroidetes ratio) (Mariat et al., 2009; Magne et al., 2020) are linked to various psychological deficiencies and co-morbidities. To elaborate, traumatic brain injury and post-traumatic stress disorder—the two most prevalent psychological disorders of modern warfare—are linked to fecal microbiota. For instance, a social stress mouse model stimulating PTSD-like traits caused a time-independent shift in the overall abundance profile of gut bacteria alongside a time-resolved alteration of the Firmicutes and Bacteroidetes ratio (Gautam et al., 2018; Hoke et al., 2022). Concurrently, these stressed mice displayed behavioral deficiencies, metabolic dysfunction, neurotoxicity (Muhie et al., 2017), and a shift in the neuronal morphology in the hippocampus, amygdala, and prefrontal cortex (Anilkumar et al., 2022). In fecal samples, the total abundance of bacterial phyla, namely Actinobacteria, Lentisphaerae, and Verrucomicrobia, was directly associated with the increased PTSD index (Hemmings et al., 2017). The proliferation of obligate anaerobic bacteria such as Deferribacteres in fecal samples of traumatic brain injury (TBI) patients potentially highlights that TBI triggers a hypoxic condition in the colonic lumen due to endogenous energy deprivation (Nicholson et al., 2019; Angoa-Pérez et al., 2020).
Gut-brain communication is facilitated by transferring neurotransmitters and neuromodulators via the bloodstream or lymphatic systems and synaptic transmission via the vagus nerve (VN). Most bloodborne microbial metabolites cross the BBB via carrier proteins and receptors expressed on BBB or by compromising BBB integrity (Ahmed et al., 2022). VN, a significant component of the parasympathetic nervous system, constitutes a parallel communication channel between the gut microbiota and the nervous system, encompassing the CNS and enteric nervous system (ENS) (Parker et al., 2020). In vivo studies observed that the microbial population was not directly connected to the VN because the nerve fibers do not penetrate the gut epithelia. Instead, the changes in the microbial ecosystem and corresponding shifts in metabolite levels perturb the neuroendocrine and endocrine signals of the host gastrointestinal tract; downstream signal travels to the brain via a series of receptors on the vagal afferents and neuropod cell-mediated synaptic transmission (Parker et al., 2020; Ahmed et al., 2022).
The intestinal epithelia play an integral role in the communication between brain and gut microbiota. Perturbed by the stress and environmental shifts, the neurons, immune cells, and mucosal cells of the intestinal epithelia release catecholamines, serotonin, dynorphin, and cytokines into the gut lumen. Playing a critical role in this process, the enterochromaffin cells in intestinal epithelia potentially act as the first line of the recipient of information about food intake, as it rapidly transmits this knowledge to the brain via activating VNs [sometimes via a single synapse within a millisecond timeframe (Kaelberer et al., 2018)] and/or sending bloodborne messengers to the hypothalamus; henceforth these cells regulate food intake and glycemia (Bohórquez et al., 2015; Gribble and Reimann, 2016). This enterochromaffin cell-mediated serotonin production is modulated by microorganisms such as Clostridium spp. and their metabolites, such as indole, norepinephrine, and butyric acid (Yano et al., 2015; Fung et al., 2019). This serotonin pool, often called the peripheral serotonin (El-Merahbi et al., 2015) functions independently of the serotonin pool generated inside the CNS since serotonin cannot cross BBB (Berger et al., 2009). The role of brain serotonin in controlling mood, sleep, and stress response is comparatively well studied (Berger et al., 2009; Strasser et al., 2016), while we have not fully comprehended the role of peripheral serotonin (El-Merahbi et al., 2015). The SBAs are another major microbial metabolite that cannot cross the BBB, although preliminary indications suggest certain roles for SBAs in modulating the brain functions (Monteiro-Cardoso et al., 2021).
The stress signals released into the intestinal lumen via the gut epithelial cells alter the pH and viscosity of gastrointestinal fluid along with the intestinal lumen’s temperature and pressure (Parker et al., 2020; Ahmed et al., 2022). As a result, the microbial compositions and their motility adapt to best utilize the available resources, such as excess lactate or reduced bioenergy, to survive in unfavorable environments. If the changes in the gut microbial ecosystem exceed normal homeostatic ranges, a therapeutic intervention becomes necessary; furthermore, if these processes are left unchecked, the intestinal epithelia become permeable, allowing the gut microbiome to enter the bloodstream- this condition is known as gut leakage (Chakaroun et al., 2020). Given changes in the functional metagenomics is mirrored by the differentially expressed microbial metabolites.
The altered ecology of commensals differentially secrete a wide variety of neurotransmitters derived from aromatic amino acids, which act on the brain functions as excitatory (e.g., glutamate, dopamine, and acetylcholine) or inhibitory neurotransmitters (e.g., GABA and glycine) (Spivak et al., 2022). It is also important to note that the synthesis and biofunctions of these neurotransmitters are controlled by various co-factors (e.g., age) and multiple peripheral tissues (e.g., kidney and liver). Still, the degree of influences of these co-factors on the gut commensals remains largely unexplained.
Glutamate, the most prominent neurotransmitter in the cortex region of the brain, is regulated by kynurenic acid, and colonic Lactobacillus and Pseudomonas spp. contribute a significant portion of whole-body kynurenic acid accumulation via tryptophan metabolism (Ostapiuk and Urbanska, 2022). The synthesis of dopamine, another excitatory neurotransmitter, is controlled by p-cresol and synthesized in several peripheral tissues and colonic Lactobacillus and Clostridium spp. (Pascucci et al., 2020). In addition to modulating the dopaminergic network, p-cresol regulates the oxytocinergic and opioidergic networks and guides behavioral plasticity (Putnam and Chang, 1858). On the other hand, Bacteroidetes, one of the most abundant fecal microorganisms, encodes glutamate decarboxylase-encoding gene, which is linked to the production of GABA, the major inhibitory neurotransmitter of brain (Otaru et al., 2021).
Clostridia, Fusobacterium, and Acidaminobacter are the primary producers of SCFAs, while Firmicutes, a highly abundant fecal microorganism, mainly secretes butyrate (Eeckhaut et al., 2011). SCFAs, primarily propionate and butyrate, participate in many health-beneficial function (Louis and Flint, 2017); for instance, they control the adaptive immune systems (Louis and Flint, 2017) and, in this process, mitigate neuroinflammation by inhibiting the production of histone deacetylase 1, a proinflammatory cytokine (Song et al., 2022).
In a healthy gut milieu, lactate is used to cross-feed the microbiota for unrelenting SCFA production. In homeostatic conditions, many gut microbes, including Firmicutes, convert lactate to propionate and butyrate (Abedi and Hashemi, 2020), which helps maintain a low accumulation of lactate in the intestinal lumen (Duncan et al., 2004). Increased lactate production is typically pH mediated and often identified as the marker of dysbiosis when Firmicutes and Bacteroidetes get replaced by lactate-producing Actinobacteria, Lactobacillus, and Proteobacteria. This event inhibits the synthesis of butyrate and propionate (Abedi and Hashemi, 2020; Wang S. P. et al., 2020). Interestingly, increased lactate production by gut anaerobes during exercise potentially supports the host’s mitochondrial respiration (Brooks, 2020) and indirectly facilitates cerebral BDNF production via upregulating the Sirtuin1 network. This is likely a dose-dependent impact of lactate on brain, as the mice under the regime of physical exercise showed increased learning and memory retention power (El Hayek et al., 2019).
In addition to lactate, several other microbial metabolites actively participate in neuronal bioenergetics and glucose homeostasis (Zhang et al., 2021). Potentially directed by enterochromaffin cells, microorganisms inside the intestinal lumen produce glucose via gluconeogenesis (Soty et al., 2017), which switches to lactate production during energy deficiency and hypoxia (Brooks, 2020). Although the microbe-induced glucose metabolism has been comparatively well studied (van Olden et al., 2015; Utzschneider et al., 2016), some pertinent aspects still need additional probing. For instance, the energy-modulating hormones, such as peptide YY and GLP-1, are found to be expressed on intestinal epithelia, and corresponding receptors are reportedly expressed in the hypothalamus (Steinert et al., 2011), although the mode of exchange of these hormones between the gut and brains is yet obscured.
Finally, recent studies have suggested a potential association between the host microbial community and the glymphatic system (Hablitz and Nedergaard, 2021), a novel concept that explains the waste clearance pathways exclusively from the mammalian CNS (Meyerhoff et al., 2022). Glymphatic systems’ close associations with BBB and sleep construction, and therefore with the tryptophan pathway and vagal retrograde signaling network, intrigue us to link the gut microbiome to glymphatic systems (Hablitz and Nedergaard, 2021); however, much work is needed to completely understand this mechanism.
Challenges and path forward
Systems interrogation and knowledge integration of functional metagenome, meta-organism, and host omics are gaining traction to uncover the holistic molecular mechanism that drives the host’s stress response. Most of the available data, particularly those focused on the gut-brain axis, are built upon animal models due to the limited availability of the human brain. However, the translational potential of rodent metagenomic data is often contested. The brain morphology of humans and rodents is fundamentally different since the human gyrecephalic brain has distinct convolutions and expansions in the cortex, which facilitate handling a much wider range of emotional structures than that by the smooth-surfaced cortex in the lissencephalic brain of rodent (Sun and Hevner, 2014). Furthermore, the ecosystem of rodent microorganisms is distant from that of human; conversely, domestic animals, such as dogs, have higher metagenomic homology with humans (Wardeh et al., 2015). On the positive side, the enterochromaffin cells of rodents were found genetically homologous to that of human, although their functional homology, particularly under stress, is yet to be fully characterized (Roberts et al., 2019). Some of the customized rodent models, such as the germ-free model and gnotobiotic humanized model, are promoted for greater translational potential (Uzbay, 2019). These models could reduce the high variability of metagenomic data among the research laboratories. Nevertheless, germ-free mice are predisposed to various unique characteristics due to their atypical habitat, limited maternal care, and distinct lifestyles and diets (Mayer et al., 2015). Altogether, these traits potentially limit the translational potential of the outcome derived from germ-free mouse colonies (Mayer et al., 2015).
The true characteristics of the host-gut microbe relationship are still obscure; to begin with, we are still unsure if this relationship is causative or correlative in nature. Comprehension of the host-microbe relationship possibly depends on the following two fundamental pieces of information: (i) how the gut microbe communicates with peripheral organs and (ii) how different microorganisms in the gut lumen, such as bacteria, viruses, etc. crosstalk among themselves. To shed light on the gut microbe-organ axis, this review article has discussed in detail the topic of the gut microbe-brain axis, while the other relationship matrix with gut microbes and other peripheral organs, such as lungs, liver, and kidneys, were cursorily discussed. The inter-organism relationship, particularly the bacteria-virus mutualistic association, has been considered to be the controlling factor of host defense. Enteric virus is mostly represented by bacteriophage and eukaryotic virus (Li et al., 2021), although the characterization of the virus composition has been challenged by limited capability in mapping the virus gene sequences (Minot et al., 2011; Manrique et al., 2016). Given that the bacteriophage can obliterate bacteria, their relationship and comparative abundance in the intestine remains an interesting subject to moot. A significantly high ratio of bacteriophage-to-bacteria at the enteric mucosal surface in comparison to the rest of gut lumen (Barr et al., 2013) is a potential frontline of defense against bacterial infection and thereby regulates many antagonists and beneficial actions including the host defense and immune response (Almeida et al., 2019; Kirsch et al., 2021). Our understanding of additional inter-kingdom relationships, such as bacteria-archaea (Hoegenauer et al., 2022) and bacteria-protozoa (Dubik et al., 2022), are still at their early stages, although emerging studies identified their concerted efforts in disease pathogenesis (Kodio et al., 2020; Mafra et al., 2022).
Needless to say, we have yet to fully characterize the associations between microbial metabolites and disease pathology. One of the possible modalities to meet the knowledge gap is to systematically dissociate a sick gut from its sick host. The null hypothesis could be that the fecal microbe of a sick host cannot adversely affect a healthy host. To support this hypothesis, fecal samples could be collected from the stressed or sick rodents and allowed to colonize them in healthy gnotobiotic rodents using FMT (Wang J. W. et al., 2019). Subsequent analysis can throw light on how a sick microbiota can control the host’s health in the absence of the adverse condition. Concurrent FMT of healthy commensals (i.e., the fecal samples collected from healthy cohort) into sick rodents will help in getting the full scenario about how microbiota communicate with the host to regulate its overall health.
Following the same concept, a surgical deletion or chemical manipulation of VNs can highlight the role of this nervous system in sensing the dietary intake (Brown et al., 2011; Yao et al., 2018) and consequent impacts on humans, such as the change in body weight (Burneo et al., 2002).
The outcome of the abovementioned modalities has been used to customize the traditional nutraceuticals, such as prebiotic, postbiotic, and symbiotic diets, and more novel supplements, namely parabiotic (made from non-viable microorganisms) and postbiotic biotherapies (made from microbial derivatives, such as metabolites) (Nataraj et al., 2020); although these nomenclatures are contested in past (Zolkiewicz et al., 2020; Salminen et al., 2021). Additional therapeutic approaches include genetic domestication of the microbe of interest using gene-editing technology (e.g., CRISPR) or by implanting synthetic promoters in the gut lumen (Mimee et al., 2016; Inda et al., 2019). In the recent past, Bacteroidetes, one of the most abundant gut commensals, was successfully systems-engineered (Mimee et al., 2016). It is a step toward designing a universally applicable tool that can systematically alter the microorganism along with its neighbors (e.g., Firmicutes that co-habitats Bacteroidetes) and/or co-factors (e.g., diet source) to reinforce the host’s response (Wexler, 2007; Inda et al., 2019).
Disease diagnostic and prognostic capabilities based on meta-organism data have shown significant progress. A sophisticated colonoscopy with a miniature camera can monitor a wide range of physiological attributes of the colon, such as pH, temperatures, etc., in real-time (Yung et al., 2016; Kalantar-Zadeh et al., 2017). Ingestible sensor prototypes, such as the “digital pill,” have emerged at the forefront to the disease diagnosis platforms (Kalantar-Zadeh et al., 2017; Beardslee et al., 2020). These advanced sensors can determine the colon oxidation potentials due to the shifts in microbial abundance (Baltsavias et al., 2020) and profile the gases, such as oxygen and hydrogen, emitted inside the colon lumen (Kalantar-Zadeh et al., 2018). Box 2 documents these novel diagnostic concepts along with the more conventional prototypes that monitor microbe and microbial metabolites.
Shifting the focus to its surrounding environment, metagenome and metabolites have shown great potential to enable 360° surveillance outreach. Wastewater surveillance of the microbiome gave a longitudinal profile of spreading COVID-19 infection across different communities (Brumfield et al., 2022). Metabolite monitoring in bryophytes and fish larvae (Sanches-Fernandes et al., 2022) can act as biosensors of toxins or radio-biological attacks on the communities.
Microbial metabolites are the primary intermediatory of the crosstalk between host and microbiota. This is an emerging concept essentially refining the traditional view of postprandial neuronal and hormonal exchange between the brain and gut (Wachsmuth et al., 2022). The microbiome is critically associated with the pathophysiology of several diseases and the host’s adaptive response to stress. Hence, one can anticipate that systematic modulation and monitoring of the microbiome could be the key enabler to combat diseases. Overall, meta-organisms and functional microbiota are the subject of very active research, and we hope to see significant progress in the coming years.
Box 1. Definitions of the key terms used in this article. The “*” items are those whose definitions are sometimes contested; see the main text for details.
Box 2. An outlook of the conventional to futuristic tools/technologies that could readily benefit from the knowledge of meta-organisms and metabolites.
Author contributions
NC: Conceptualization, Data curation, Investigation, Writing – original draft, Writing – review & editing.
Funding
The author(s) declare that no financial support was received for the research, authorship, and/or publication of this article.
Acknowledgments
The author would like to sincerely acknowledge CPT Kevin Swift, Ph.D., and Rasha Hammamieh, Ph.D., for their scientific and editorial support.
Conflict of interest
The author declares that the research was conducted in the absence of any commercial or financial relationships that could be construed as a potential conflict of interest.
The handling editor BD declared a past collaboration with the author NC.
Publisher’s note
All claims expressed in this article are solely those of the authors and do not necessarily represent those of their affiliated organizations, or those of the publisher, the editors and the reviewers. Any product that may be evaluated in this article, or claim that may be made by its manufacturer, is not guaranteed or endorsed by the publisher.
Author disclaimer
Material has been reviewed by the Walter Reed Army Institute of Research. There is no objection to its presentation and/or publication. The opinions or assertions contained herein are the private views of the author and are not to be construed as official or as reflecting true views of the Department of the Army or the Department of Defense.
References
Abedi, E., and Hashemi, S. M. B. (2020). Lactic acid production-producing microorganisms and substrates sources-state of art. Heliyon 6:e04974. doi: 10.1016/j.heliyon.2020.e04974
Agus, A., Clement, K., and Sokol, H. (2021). Gut microbiota-derived metabolites as central regulators in metabolic disorders. Gut 70, 1174–1182. doi: 10.1136/gutjnl-2020-323071
Ahmed, H., Leyrolle, Q., Koistinen, V., Kärkkäinen, O., Layé, S., Delzenne, N., et al. (2022). Microbiota-derived metabolites as drivers of gut-brain communication. Gut Microbes 14:2102878. doi: 10.1080/19490976.2022.2102878
Alexander, M., and Turnbaugh, P. J. (2020). Deconstructing mechanisms of diet-microbiome-immune interactions. Immunity 53, 264–276. doi: 10.1016/j.immuni.2020.07.015
Almeida, G. M. F., Laanto, E., Ashrafi, R., and Sundberg, L. R. (2019). Bacteriophage adherence to mucus mediates preventive protection against pathogenic bacteria. mBio 10:e01984. doi: 10.1128/mBio.01984-19
Al-Rashidi, H. E. (2022). Gut microbiota and immunity relevance in eubiosis and dysbiosis. Saudi J. Biol. Sci. 29, 1628–1643. doi: 10.1016/j.sjbs.2021.10.068
Alseekh, S., Aharoni, A., Brotman, Y., Contrepois, K., D’Auria, J., Ewald, J., et al. (2021). Mass spectrometry-based metabolomics: a guide for annotation, quantification and best reporting practices. Nat. Methods 18, 747–756. doi: 10.1038/s41592-021-01197-1
Amara, A., Frainay, C., Jourdan, F., Naake, T., Neumann, S., Novoa-del-Toro, E. M., et al. (2022). Networks and graphs discovery in metabolomics data analysis and interpretation. Front. Mol. Biosci. 9:841373. doi: 10.3389/fmolb.2022.841373
Amaral, J. D., Viana, R. J., Ramalho, R. M., Steer, C. J., and Rodrigues, C. M. (2009). Bile acids: regulation of apoptosis by ursodeoxycholic acid. J. Lipid Res. 50, 1721–1734. doi: 10.1194/jlr.R900011-JLR200
Angoa-Pérez, M., Zagorac, B., Anneken, J. H., Briggs, D. I., Winters, A. D., Greenberg, J. M., et al. (2020). Repetitive, mild traumatic brain injury results in a progressive white matter pathology, cognitive deterioration, and a transient gut microbiota dysbiosis. Sci. Rep. 10:8949. doi: 10.1038/s41598-020-65972-4
Anilkumar, S., Chakraborty, N., Meyerhoff, J., Campbell, R., Muhie, S., Hammamieh, R., et al. (2022). Longitudinally divergent dendritic spine densities of the hippocampus and amygdala: an impact of aggressor-exposed social stress. Horz. Neurosci. Res. 46.
Arumugam, M., Raes, J., Pelletier, E., Le Paslier, D., Yamada, T., Mende, D. R., et al. (2011). Enterotypes of the human gut microbiome. Nature 473, 174–180. doi: 10.1038/nature09944
Auriemma, R. S., Scairati, R., Del Vecchio, G., Liccardi, A., Verde, N., Pirchio, R., et al. (2021). The vaginal microbiome: a long urogenital colonization throughout woman life. Front. Cell. Infect. Microbiol. 11:686167. doi: 10.3389/fcimb.2021.686167
Baczkowski, A. J., Joanes, D. N., and Shamia, G. M. (1998). Range of validity of alpha and beta for a generalized diversity index H (alpha, beta) due to good. Math. Biosci. 148, 115–128. doi: 10.1016/s0025-5564(97)10013-x
Bae, S. A., Fang, M. Z., Rustgi, V., Zarbl, H., and Androulakis, I. P. (2019). At the interface of lifestyle, behavior, and circadian rhythms: metabolic implications. Front. Nutr. 6:132. doi: 10.3389/fnut.2019.00132
Baltsavias, S., van Treuren, W., Weber, M. J., Charthad, J., Baker, S., Sonnenburg, J. L., et al. (2020). In vivo wireless sensors for gut microbiome redox monitoring. IEEE Trans. Biomed. Eng. 67, 1–1830. doi: 10.1109/TBME.2019.2948575
Barr, J. J., Auro, R., Furlan, M., Whiteson, K. L., Erb, M. L., Pogliano, J., et al. (2013). Bacteriophage adhering to mucus provide a non-host-derived immunity. Proc. Natl. Acad. Sci. U.S.A. 110, 10771–10776. doi: 10.1073/pnas.1305923110
Beardslee, L. A., Banis, G. E., Chu, S., Liu, S., Chapin, A. A., Stine, J. M., et al. (2020). Ingestible sensors and sensing systems for minimally invasive diagnosis and monitoring: the next frontier in minimally invasive screening. ACS Sens. 5, 891–910. doi: 10.1021/acssensors.9b02263
Berg, G., Rybakova, D., Fischer, D., Cernava, T., Vergès, M. C. C., Charles, T., et al. (2020). Microbiome definition re-visited: old concepts and new challenges. Microbiome 8:103. doi: 10.1186/s40168-020-00875-0
Berger, M., Gray, J. A., and Roth, B. L. (2009). The expanded biology of serotonin. Annu. Rev. Med. 60, 355–366. doi: 10.1146/annurev.med.60.042307.110802
Berry, D., and Loy, A. (2018). Stable-isotope probing of human and animal microbiome function. Trends Microbiol. 26, 999–1007. doi: 10.1016/j.tim.2018.06.004
Biragyn, A., and Ferrucci, L. (2018). Gut dysbiosis: a potential link between increased cancer risk in ageing and inflammaging. Lancet Oncol. 19, e295–e304. doi: 10.1016/S1470-2045(18)30095-0
Bohórquez, D. V., Shahid, R. A., Erdmann, A., Kreger, A. M., Wang, Y., Calakos, N., et al. (2015). Neuroepithelial circuit formed by innervation of sensory enteroendocrine cells. J. Clin. Invest. 125, 782–786. doi: 10.1172/JCI78361
Borrego-Ruiz, A., and Borrego, J. J. (2024). An updated overview on the relationship between human gut microbiome dysbiosis and psychiatric and psychological disorders. Prog. Neuro-Psychopharmacol. Biol. Psychiatry 128:110861. doi: 10.1016/j.pnpbp.2023.110861
Braniste, V., Al-Asmakh, M., Kowal, C., Anuar, F., Abbaspour, A., Tóth, M., et al. (2014). The gut microbiota influences blood-brain barrier permeability in mice. Sci. Transl. Med. 6:263ra158. doi: 10.1126/scitranslmed.3009759
Brestoff, J. R., and Artis, D. (2015). Immune regulation of metabolic homeostasis in health and disease. Cell 161, 146–160. doi: 10.1016/j.cell.2015.02.022
Brooks, G. A. (2020). Lactate as a fulcrum of metabolism. Redox Biol. 35:101454. doi: 10.1016/j.redox.2020.101454
Brown, T. A., Washington, M. C., Metcalf, S. A., and Sayegh, A. I. (2011). The feeding responses evoked by cholecystokinin are mediated by vagus and splanchnic nerves. Peptides 32, 1581–1586. doi: 10.1016/j.peptides.2011.06.024
Brumfield, K. D., Leddy, M., Usmani, M., Cotruvo, J. A., Tien, C. T., Dorsey, S., et al. (2022). Microbiome analysis for wastewater surveillance during COVID-19. mBio 13:e0059122. doi: 10.1128/mbio.00591-22
Brunet, M. A., Leblanc, S., and Roucou, X. (2020). Reconsidering proteomic diversity with functional investigation of small ORFs and alternative ORFs. Exp. Cell Res. 393:112057. doi: 10.1016/j.yexcr.2020.112057
Burneo, J. G., Faught, E., Knowlton, R., Morawetz, R., and Kuzniecky, R. (2002). Weight loss associated with vagus nerve stimulation. Neurology 59, 463–464. doi: 10.1212/wnl.59.3.463
Cani, P. D. (2018). Human gut microbiome: hopes, threats and promises. Gut 67, 1716–1725. doi: 10.1136/gutjnl-2018-316723
Caspi, R., Billington, R., Keseler, I. M., Kothari, A., Krummenacker, M., Midford, P. E., et al. (2020). The MetaCyc database of metabolic pathways and enzymes—a 2019 update. Nucleic Acids Res. 48, D445–D453. doi: 10.1093/nar/gkz862
Chakaroun, R. M., Massier, L., and Kovacs, P. (2020). Gut microbiome, intestinal permeability, and tissue bacteria in metabolic disease: perpetrators or bystanders? Nutrients 12:1082. doi: 10.3390/nu12041082
Chen, X., and Devaraj, S. (2018). Gut microbiome in obesity, metabolic syndrome, and diabetes. Curr. Diab. Rep. 18:129. doi: 10.1007/s11892-018-1104-3
Cheng, L., Qi, C., Yang, H., Lu, M., Cai, Y., Fu, T., et al. (2022). gutMGene: a comprehensive database for target genes of gut microbes and microbial metabolites. Nucleic Acids Res. 50, D795–D800. doi: 10.1093/nar/gkab786
Connell, E., Le Gall, G., Pontifex, M. G., Sami, S., Cryan, J. F., Clarke, G., et al. (2022). Microbial-derived metabolites as a risk factor of age-related cognitive decline and dementia. Mol. Neurodegener. 17:43. doi: 10.1186/s13024-022-00548-6
Cottret, L., Frainay, C., Chazalviel, M., Cabanettes, F., Gloaguen, Y., Camenen, E., et al. (2018). MetExplore: collaborative edition and exploration of metabolic networks. Nucleic Acids Res. 46, W495–W502. doi: 10.1093/nar/gky301
Cryan, J. F., O’Riordan, K. J., Sandhu, K., Peterson, V., and Dinan, T. G. (2020). The gut microbiome in neurological disorders. Lancet Neurol. 19, 179–194. doi: 10.1016/S1474-4422(19)30356-4
Dabke, K., Hendrick, G., and Devkota, S. (2019). The gut microbiome and metabolic syndrome. J. Clin. Invest. 129, 4050–4057. doi: 10.1172/JCI129194
de Oliveira, G. L. V., Cardoso, C. R. B., Taneja, V., and Fasano, A. (2021). Editorial: intestinal dysbiosis in inflammatory diseases. Front. Immunol. 12:727485. doi: 10.3389/fimmu.2021.727485
Desbonnet, L., Clarke, G., Shanahan, F., Dinan, T. G., and Cryan, J. F. (2014). Microbiota is essential for social development in the mouse. Mol. Psychiatry 19, 146–148. doi: 10.1038/mp.2013.65
Drozdz, M., Makuch, S., Cieniuch, G., Wozniak, M., and Ziolkowski, P. (2020). Obligate and facultative anaerobic bacteria in targeted cancer therapy: current strategies and clinical applications. Life Sci. 261:118296. doi: 10.1016/j.lfs.2020.118296
Dubik, M., Pilecki, B., and Moeller, J. B. (2022). Commensal intestinal protozoa-underestimated members of the gut microbial community. Biology 11:1742. doi: 10.3390/biology11121742
Dührkop, K., Nothias, L. F., Fleischauer, M., Reher, R., Ludwig, M., Hoffmann, M. A., et al. (2021). Systematic classification of unknown metabolites using high-resolution fragmentation mass spectra. Nat. Biotechnol. 39, 462–471. doi: 10.1038/s41587-020-0740-8
Duncan, S. H., Louis, P., and Flint, H. J. (2004). Lactate-utilizing bacteria, isolated from human feces, that produce butyrate as a major fermentation product. Appl. Environ. Microbiol. 70, 5810–5817. doi: 10.1128/AEM.70.10.5810-5817.2004
Eeckhaut, V., Van Immerseel, F., Croubels, S., De Baere, S., Haesebrouck, F., Ducatelle, R., et al. (2011). Butyrate production in phylogenetically diverse Firmicutes isolated from the chicken caecum. Microb. Biotechnol. 4, 503–512. doi: 10.1111/j.1751-7915.2010.00244.x
El Hayek, L., Khalifeh, M., Zibara, V., Abi Assaad, R., Emmanuel, N., Karnib, N., et al. (2019). Lactate mediates the effects of exercise on learning and memory through SIRT1-dependent activation of hippocampal brain-derived neurotrophic factor (BDNF). J. Neurosci. 39, 2369–2382. doi: 10.1523/JNEUROSCI.1661-18.2019
El-Merahbi, R., Loffler, M., Mayer, A., and Sumara, G. (2015). The roles of peripheral serotonin in metabolic homeostasis. FEBS Lett. 589, 1728–1734. doi: 10.1016/j.febslet.2015.05.054
Entwistle, S., Li, X., and Yin, Y. (2019). Orphan genes shared by pathogenic genomes are more associated with bacterial pathogenicity. mSystems 4:e00290. doi: 10.1128/mSystems.00290-18
Fernandez-Veledo, S., and Vendrell, J. (2019). Gut microbiota-derived succinate: friend or foe in human metabolic diseases? Rev. Endocr. Metab. Disord. 20, 439–447. doi: 10.1007/s11154-019-09513-z
Frezza, C. (2017). Mitochondrial metabolites: undercover signalling molecules. Interface Focus 7:20160100. doi: 10.1098/rsfs.2016.0100
Fröhlich, E. E., Farzi, A., Mayerhofer, R., Reichmann, F., Jačan, A., Wagner, B., et al. (2016). Cognitive impairment by antibiotic-induced gut dysbiosis: analysis of gut microbiota-brain communication. Brain Behav. Immun. 56, 140–155. doi: 10.1016/j.bbi.2016.02.020
Fu, M., Zhang, X., Liang, Y., Lin, S., Qian, W., and Fan, S. (2020). Alterations in vaginal microbiota and associated metabolome in women with recurrent implantation failure. mBio 11:e03242. doi: 10.1128/mBio.03242-19
Fung, T. C., Vuong, H. E., Luna, C. D. G., Pronovost, G. N., Aleksandrova, A. A., Riley, N. G., et al. (2019). Intestinal serotonin and fluoxetine exposure modulate bacterial colonization in the gut. Nat. Microbiol. 4, 2064–2073. doi: 10.1038/s41564-019-0540-4
Gautam, A., Kumar, R., Chakraborty, N., Muhie, S., Hoke, A., Hammamieh, R., et al. (2018). Altered fecal microbiota composition in all male aggressor-exposed rodent model simulating features of post-traumatic stress disorder. J. Neurosci. Res. 96, 1311–1323. doi: 10.1002/jnr.24229
Ghosh, S., Molcan, E., DeCoffe, D., Dai, C., and Gibson, D. L. (2013). Diets rich in n−6 PUFA induce intestinal microbial dysbiosis in aged mice. Br. J. Nutr. 110, 515–523. doi: 10.1017/S0007114512005326
GitHub. (n.d.). Chapter 6 microbiome diversity. Available at: https://microbiome.github.io/OMA/microbiome-diversity.html
Gómez-Gallego, C., Morales, J. M., Monleón, D., Du Toit, E., Kumar, H., Linderborg, K., et al. (2018). Human breast milk NMR metabolomic profile across specific geographical locations and its association with the milk microbiota. Nutrients 10:1355. doi: 10.3390/nu10101355
Gonzalez-Sanchez, P., and DeNicola, G. M. (2021). The microbiome(s) and cancer: know thy neighbor(s). J. Pathol. 254, 332–343. doi: 10.1002/path.5661
Gribble, F. M., and Reimann, F. (2016). Enteroendocrine cells: chemosensors in the intestinal epithelium. Annu. Rev. Physiol. 78, 277–299. doi: 10.1146/annurev-physiol-021115-105439
Guirimand, T., Delmotte, S., and Navratil, V. (2015). VirHostNet 2.0: surfing on the web of virus/host molecular interactions data. Nucleic Acids Res. 43, D583–D587. doi: 10.1093/nar/gku1121
Gupta, A., Osadchiy, V., and Mayer, E. A. (2020). Brain-gut-microbiome interactions in obesity and food addiction. Nat. Rev. Gastroenterol. Hepatol. 17, 655–672. doi: 10.1038/s41575-020-0341-5
Hablitz, L. M., and Nedergaard, M. (2021). The glymphatic system: a novel component of fundamental neurobiology. J. Neurosci. 41, 7698–7711. doi: 10.1523/JNEUROSCI.0619-21.2021
Hemmings, S. M. J., Malan-Müller, S., van den Heuvel, L. L., Demmitt, B. A., Stanislawski, M. A., Smith, D. G., et al. (2017). The microbiome in posttraumatic stress disorder and trauma-exposed controls: an exploratory study. Psychosom. Med. 79, 936–946. doi: 10.1097/PSY.0000000000000512
Hoegenauer, C., Hammer, H. F., Mahnert, A., and Moissl-Eichinger, C. (2022). Methanogenic archaea in the human gastrointestinal tract. Nat. Rev. Gastroenterol. Hepatol. 19, 805–813. doi: 10.1038/s41575-022-00673-z
Hoke, A., Chakraborty, N., Gautam, A., Hammamieh, R., and Jett, M. (2022). Acute and delayed effects of stress eliciting post-traumatic stress-like disorder differentially alters fecal microbiota composition in a male mouse model. Front. Cell. Infect. Microbiol. 12:810815. doi: 10.3389/fcimb.2022.810815
Hu, X. F., Zhang, W. Y., Wen, Q., Chen, W. J., Wang, Z. M., Chen, J., et al. (2019). Fecal microbiota transplantation alleviates myocardial damage in myocarditis by restoring the microbiota composition. Pharmacol. Res. 139, 412–421. doi: 10.1016/j.phrs.2018.11.042
Hufnagl, K., Pali-Scholl, I., Roth-Walter, F., and Jensen-Jarolim, E. (2020). Dysbiosis of the gut and lung microbiome has a role in asthma. Semin. Immunopathol. 42, 75–93. doi: 10.1007/s00281-019-00775-y
Hui, W., Li, T., Liu, W., Zhou, C., and Gao, F. (2019). Fecal microbiota transplantation for treatment of recurrent C. difficile infection: an updated randomized controlled trial meta-analysis. PLoS One 14:e0210016. doi: 10.1371/journal.pone.0210016
Idle, J. R., and Gonzalez, F. J. (2007). Metabolomics. Cell Metab. 6, 348–351. doi: 10.1016/j.cmet.2007.10.005
Iebba, V., Totino, V., Gagliardi, A., Santangelo, F., Cacciotti, F., Trancassini, M., et al. (2016). Eubiosis and dysbiosis: the two sides of the microbiota. New Microbiol. 39, 1–12.
Inda, M. E., Broset, E., Lu, T. K., and de la Fuente-Nunez, C. (2019). Emerging frontiers in microbiome engineering. Trends Immunol. 40, 952–973. doi: 10.1016/j.it.2019.08.007
Johnson, C. H., Patterson, A. D., Idle, J. R., and Gonzalez, F. J. (2012). Xenobiotic metabolomics: major impact on the metabolome. Annu. Rev. Pharmacol. Toxicol. 52, 37–56. doi: 10.1146/annurev-pharmtox-010611-134748
Jones, J., Reinke, S. N., Ali, A., Palmer, D. J., and Christophersen, C. T. (2021). Fecal sample collection methods and time of day impact microbiome composition and short chain fatty acid concentrations. Sci. Rep. 11:13964. doi: 10.1038/s41598-021-93031-z
Kaelberer, M. M., Buchanan, K. L., Klein, M. E., Barth, B. B., Montoya, M. M., Shen, X., et al. (2018). A gut-brain neural circuit for nutrient sensory transduction. Science 361:eaat5236. doi: 10.1126/science.aat5236
Kalantar-Zadeh, K., Berean, K. J., Ha, N., Chrimes, A. F., Xu, K., Grando, D., et al. (2018). A human pilot trial of ingestible electronic capsules capable of sensing different gases in the gut. Nat. Electron. 1, 79–87. doi: 10.1038/s41928-017-0004-x
Kalantar-Zadeh, K., Ha, N., Ou, J. Z., and Berean, K. J. (2017). Ingestible sensors. ACS Sens. 2, 468–483. doi: 10.1021/acssensors.7b00045
Kandalai, S., Li, H., Zhang, N., Peng, H., and Zheng, Q. (2023). The human microbiome and cancer: a diagnostic and therapeutic perspective. Cancer Biol. Ther. 24:2240084. doi: 10.1080/15384047.2023.2240084
Kanehisa, M., Furumichi, M., Tanabe, M., Sato, Y., and Morishima, K. (2017). KEGG: new perspectives on genomes, pathways, diseases and drugs. Nucleic Acids Res. 45, D353–D361. doi: 10.1093/nar/gkw1092
Kanehisa, M., and Goto, S. (2000). KEGG: Kyoto encyclopedia of genes and genomes. Nucleic Acids Res. 28, 27–30. doi: 10.1093/nar/28.1.27
Kanehisa, M., Sato, Y., and Morishima, K. (2016). BlastKOALA and GhostKOALA: KEGG tools for functional characterization of genome and metagenome sequences. J. Mol. Biol. 428, 726–731. doi: 10.1016/j.jmb.2015.11.006
Karl, J. P., Margolis, L. M., Madslien, E. H., Murphy, N. E., Castellani, J. W., Gundersen, Y., et al. (2017). Changes in intestinal microbiota composition and metabolism coincide with increased intestinal permeability in young adults under prolonged physiological stress. Am. J. Physiol. Gastrointest. Liver Physiol. 312, G559–G571. doi: 10.1152/ajpgi.00066.2017
Kim, J. Y., Whon, T. W., Lim, M. Y., Kim, Y. B., Kim, N., Kwon, M. S., et al. (2020). The human gut archaeome: identification of diverse haloarchaea in Korean subjects. Microbiome 8:114. doi: 10.1186/s40168-020-00894-x
Kirsch, J. M., Brzozowski, R. S., Faith, D., Round, J. L., Secor, P. R., and Duerkop, B. A. (2021). Bacteriophage-bacteria interactions in the gut: from invertebrates to mammals. Annu. Rev. Virol. 8, 95–113. doi: 10.1146/annurev-virology-091919-101238
Kodio, A., Menu, E., and Ranque, S. (2020). Eukaryotic and prokaryotic microbiota interactions. Microorganisms 8:12. doi: 10.3390/microorganisms8122018
Krautkramer, K. A., Fan, J., and Backhed, F. (2021). Gut microbial metabolites as multi-kingdom intermediates. Nat. Rev. Microbiol. 19, 77–94. doi: 10.1038/s41579-020-0438-4
Krog, M. C., Hugerth, L. W., Fransson, E., Bashir, Z., Nyboe Andersen, A., Edfeldt, G., et al. (2022). The healthy female microbiome across body sites: effect of hormonal contraceptives and the menstrual cycle. Hum. Reprod. 37, 1525–1543. doi: 10.1093/humrep/deac094
Lam, K. N., Cheng, J., Engel, K., Neufeld, J. D., and Charles, T. C. (2015). Current and future resources for functional metagenomics. Front. Microbiol. 6:1196. doi: 10.3389/fmicb.2015.01196
Lau, K., Srivatsav, V., Rizwan, A., Nashed, A., Liu, R., Shen, R., et al. (2017). Bridging the gap between gut microbial dysbiosis and cardiovascular diseases. Nutrients 9:859. doi: 10.3390/nu9080859
Lee, I. H., and Finkel, T. (2013). Metabolic regulation of the cell cycle. Curr. Opin. Cell Biol. 25, 724–729. doi: 10.1016/j.ceb.2013.07.002
Levy, M., Thaiss, C. A., and Elinav, E. (2016). Metabolites: messengers between the microbiota and the immune system. Genes Dev. 30, 1589–1597. doi: 10.1101/gad.284091.116
Li, Y., Handley, S. A., and Baldridge, M. T. (2021). The dark side of the gut: virome-host interactions in intestinal homeostasis and disease. J. Exp. Med. 218:e20201044. doi: 10.1084/jem.20201044
Liu, K. H., Owens, J. A., Saeedi, B., Cohen, C. E., Bellissimo, M. P., Naudin, C., et al. (2021). Microbial metabolite delta-valerobetaine is a diet-dependent obesogen. Nat. Metab. 3, 1694–1705. doi: 10.1038/s42255-021-00502-8
Lloyd-Price, J., Abu-Ali, G., and Huttenhower, C. (2016). The healthy human microbiome. Genome Med. 8:51. doi: 10.1186/s13073-016-0307-y
Louis, P., and Flint, H. J. (2017). Formation of propionate and butyrate by the human colonic microbiota. Environ. Microbiol. 19, 29–41. doi: 10.1111/1462-2920.13589
Louis-Jean, S., and Martirosyan, D. (2019). Nutritionally attenuating the human gut microbiome to prevent and manage metabolic syndrome. J. Agric. Food Chem. 67, 12675–12684. doi: 10.1021/acs.jafc.9b04879
Macfarlane, S., and Macfarlane, G. T. (2003). Regulation of short-chain fatty acid production. Proc. Nutr. Soc. 62, 67–72. doi: 10.1079/PNS2002207
Mafra, D., Ribeiro, M., Fonseca, L., Regis, B., Cardozo, L. F. M. F., Fragoso dos Santos, H., et al. (2022). Archaea from the gut microbiota of humans: could be linked to chronic diseases? Anaerobe 77:102629. doi: 10.1016/j.anaerobe.2022.102629
Magne, F., Gotteland, M., Gauthier, L., Zazueta, A., Pesoa, S., Navarrete, P., et al. (2020). The Firmicutes/Bacteroidetes ratio: a relevant marker of gut dysbiosis in obese patients? Nutrients 12:1474. doi: 10.3390/nu12051474
Magnúsdóttir, S., Heinken, A., Kutt, L., Ravcheev, D. A., Bauer, E., Noronha, A., et al. (2017). Generation of genome-scale metabolic reconstructions for 773 members of the human gut microbiota. Nat. Biotechnol. 35, 81–89. doi: 10.1038/nbt.3703
Mallick, H., Rahnavard, A., McIver, L. J., Ma, S., Zhang, Y., Nguyen, L. H., et al. (2021). Multivariable association discovery in population-scale meta-omics studies. PLoS Comput. Biol. 17:e1009442. doi: 10.1371/journal.pcbi.1009442
Mamas, M., Dunn, W. B., Neyses, L., and Goodacre, R. (2011). The role of metabolites and metabolomics in clinically applicable biomarkers of disease. Arch. Toxicol. 85, 5–17. doi: 10.1007/s00204-010-0609-6
Manca, C., Shen, M., Boubertakh, B., Martin, C., Flamand, N., Silvestri, C., et al. (2020). Alterations of brain endocannabinoidome signaling in germ-free mice. Biochim. Biophys. Acta Mol. Cell Biol. Lipids 1865:158786. doi: 10.1016/j.bbalip.2020.158786
Manor, O., Dai, C. L., Kornilov, S. A., Smith, B., Price, N. D., Lovejoy, J. C., et al. (2020). Health and disease markers correlate with gut microbiome composition across thousands of people. Nat. Commun. 11:5206. doi: 10.1038/s41467-020-18871-1
Manrique, P., Bolduc, B., Walk, S. T., van der Oost, J., de Vos, W. M., and Young, M. J. (2016). Healthy human gut phageome. Proc. Natl. Acad. Sci. U.S.A. 113, 10400–10405. doi: 10.1073/pnas.1601060113
Mariat, D., Firmesse, O., Levenez, F., Guimarăes, V. D., Sokol, H., Doré, J., et al. (2009). The Firmicutes/Bacteroidetes ratio of the human microbiota changes with age. BMC Microbiol. 9:123. doi: 10.1186/1471-2180-9-123
Mathay, C., Hamot, G., Henry, E., Georges, L., Bellora, C., Lebrun, L., et al. (2015). Method optimization for fecal sample collection and fecal DNA extraction. Biopreserv. Biobank. 13, 79–93. doi: 10.1089/bio.2014.0031
Mayer, E. A., Tillisch, K., and Gupta, A. (2015). Gut/brain axis and the microbiota. J. Clin. Invest. 125, 926–938. doi: 10.1172/JCI76304
Merriam-Webster. (n.d.). Merriam-Webster dictionary: definition of microbiome. Available at: https://www.merriam-webster.com/dictionary/microbiome
Metabolite Statistics. (n.d.). Available at: https://hmdb.ca/statistics
Meyerhoff, J., Chakraborty, N., and Hammamieh, R. (2022). Glymphatics: a transformative development in medical neuroscience relevant to injuries in military central nervous system. Mil. Med. 187, e1086–e1090. doi: 10.1093/milmed/usab344
Milovic, V., Stein, J., Odera, G., Gilani, S., and Murphy, G. M. (2000). Low-dose deoxycholic acid stimulates putrescine uptake in colon cancer cells (Caco-2). Cancer Lett. 154, 195–200. doi: 10.1016/s0304-3835(00)00400-6
Mimee, M., Tucker, A. C., Voigt, C. A., and Lu, T. K. (2016). Programming a human commensal bacterium, Bacteroides thetaiotaomicron, to sense and respond to stimuli in the murine gut microbiota. Cell Syst. 2:214. doi: 10.1016/j.cels.2016.03.007
Minot, S., Sinha, R., Chen, J., Li, H., Keilbaugh, S. A., Wu, G. D., et al. (2011). The human gut virome: inter-individual variation and dynamic response to diet. Genome Res. 21, 1616–1625. doi: 10.1101/gr.122705.111
Monteiro-Cardoso, V. F., Corliano, M., and Singaraja, R. R. (2021). Bile acids: a communication channel in the gut-brain axis. Neuromolecular Med. 23, 99–117. doi: 10.1007/s12017-020-08625-z
Mou, Z., Yang, Y., Hall, A. B., and Jiang, X. (2021). The taxonomic distribution of histamine-secreting bacteria in the human gut microbiome. BMC Genomics 22:695. doi: 10.1186/s12864-021-08004-3
Muhie, S., Gautam, A., Chakraborty, N., Hoke, A., Meyerhoff, J., Hammamieh, R., et al. (2017). Molecular indicators of stress-induced neuroinflammation in a mouse model simulating features of post-traumatic stress disorder. Transl. Psychiatry 7:e1135. doi: 10.1038/tp.2017.91
Naake, T., and Fernie, A. R. (2019). MetNet: metabolite network prediction from high-resolution mass spectrometry data in R aiding metabolite annotation. Anal. Chem. 91, 1768–1772. doi: 10.1021/acs.analchem.8b04096
Nataraj, B. H., Ali, S. A., Behare, P. V., and Yadav, H. (2020). Postbiotics-parabiotics: the new horizons in microbial biotherapy and functional foods. Microb. Cell Factories 19:168. doi: 10.1186/s12934-020-01426-w
Neis, E. P., Dejong, C. H., and Rensen, S. S. (2015). The role of microbial amino acid metabolism in host metabolism. Nutrients 7, 2930–2946. doi: 10.3390/nu7042930
Neufeld, K. M., Kang, N., Bienenstock, J., and Foster, J. A. (2011). Reduced anxiety-like behavior and central neurochemical change in germ-free mice. Neurogastroenterol. Motil. 23, 255–e119. doi: 10.1111/j.1365-2982.2010.01620.x
Nicholson, S. E., Watts, L. T., Burmeister, D. M., Merrill, D., Scroggins, S., Zou, Y., et al. (2019). Moderate traumatic brain injury alters the gastrointestinal microbiome in a time-dependent manner. Shock 52, 240–248. doi: 10.1097/SHK.0000000000001211
Noecker, C., Eng, A., Srinivasan, S., Theriot, C. M., Young, V. B., Jansson, J. K., et al. (2016). Metabolic model-based integration of microbiome taxonomic and metabolomic profiles elucidates mechanistic links between ecological and metabolic variation. mSystems 1:e00013. doi: 10.1128/mSystems.00013-15
Ogunrinola, G. A., Oyewale, J. O., Oshamika, O. O., and Olasehinde, G. I. (2020). The human microbiome and its impacts on health. Int. J. Microbiol. 2020, 8045646–8045647. doi: 10.1155/2020/8045646
Olivotto, M., Arcangeli, A., Caldini, R., Chevanne, M., Cipolleschi, M. G., and Dello Sbarba, P. (1984). Metabolic aspects of cell cycle regulation in normal and cancer cells. Toxicol. Pathol. 12, 369–373. doi: 10.1177/019262338401200411
Osman, M., Budree, S., Kelly, C. R., Panchal, P., Allegretti, J. R., Kassam, Z., et al. (2022). Effectiveness and safety of fecal microbiota transplantation for Clostridioides difficile infection: results from a 5344-patient cohort study. Gastroenterology 163, 319–322. doi: 10.1053/j.gastro.2022.03.051
Ostapiuk, A., and Urbanska, E. M. (2022). Kynurenic acid in neurodegenerative disorders-unique neuroprotection or double-edged sword? CNS Neurosci. Ther. 28, 19–35. doi: 10.1111/cns.13768
Otaru, N., Ye, K., Mujezinovic, D., Berchtold, L., Constancias, F., Cornejo, F. A., et al. (2021). GABA production by human intestinal Bacteroides spp.: prevalence, regulation, and role in acid stress tolerance. Front. Microbiol. 12:656895. doi: 10.3389/fmicb.2021.656895
Parker, A., Fonseca, S., and Carding, S. R. (2020). Gut microbes and metabolites as modulators of blood-brain barrier integrity and brain health. Gut Microbes 11, 135–157. doi: 10.1080/19490976.2019.1638722
Pascucci, T., Colamartino, M., Fiori, E., Sacco, R., Coviello, A., Ventura, R., et al. (2020). P-cresol alters brain dopamine metabolism and exacerbates autism-like behaviors in the BTBR mouse. Brain Sci. 10:233. doi: 10.3390/brainsci10040233
Pokusaeva, K., Johnson, C., Luk, B., Uribe, G., Fu, Y., Oezguen, N., et al. (2017). GABA-producing Bifidobacterium dentium modulates visceral sensitivity in the intestine. Neurogastroenterol. Motil. 29:e12904. doi: 10.1111/nmo.12904
Prehn-Kristensen, A., Zimmermann, A., Tittmann, L., Lieb, W., Schreiber, S., Baving, L., et al. (2018). Reduced microbiome alpha diversity in young patients with ADHD. PLoS One 13:e0200728. doi: 10.1371/journal.pone.0200728
Punzon-Jimenez, P., and Labarta, E. (2021). The impact of the female genital tract microbiome in women health and reproduction: a review. J. Assist. Reprod. Genet. 38, 2519–2541. doi: 10.1007/s10815-021-02247-5
Putnam, P. T., and Chang, S. W. C. (1858). Interplay between the oxytocin and opioid systems in regulating social behaviour. Philos. Trans. R. Soc. B 377:20210050. doi: 10.1098/rstb.2021.0050
Ramautar, R., Berger, R., van der Greef, J., and Hankemeier, T. (2013). Human metabolomics: strategies to understand biology. Curr. Opin. Chem. Biol. 17, 841–846. doi: 10.1016/j.cbpa.2013.06.015
Rath, S., Heidrich, B., Pieper, D. H., and Vital, M. (2017). Uncovering the trimethylamine-producing bacteria of the human gut microbiota. Microbiome 5:54. doi: 10.1186/s40168-017-0271-9
Ridlon, J. M., Harris, S. C., Bhowmik, S., Kang, D. J., and Hylemon, P. B. (2016). Consequences of bile salt biotransformations by intestinal bacteria. Gut Microbes 7, 22–39. doi: 10.1080/19490976.2015.1127483
Riquelme, E., Zhang, Y., Zhang, L., Montiel, M., Zoltan, M., Dong, W., et al. (2019). Tumor microbiome diversity and composition influence pancreatic cancer outcomes. Cell 178, 795–806.e12. doi: 10.1016/j.cell.2019.07.008
Roberts, G. P., Larraufie, P., Richards, P., Kay, R. G., Galvin, S. G., Miedzybrodzka, E. L., et al. (2019). Comparison of human and murine enteroendocrine cells by transcriptomic and peptidomic profiling. Diabetes 68, 1062–1072. doi: 10.2337/db18-0883
Routy, B., Le Chatelier, E., Derosa, L., Duong, C. P. M., Alou, M. T., Daillère, R., et al. (2018). Gut microbiome influences efficacy of PD-1-based immunotherapy against epithelial tumors. Science 359, 91–97. doi: 10.1126/science.aan3706
Salminen, S., Collado, M. C., Endo, A., Hill, C., Lebeer, S., Quigley, E. M. M., et al. (2021). The International Scientific Association of Probiotics and Prebiotics (ISAPP) consensus statement on the definition and scope of postbiotics. Nat. Rev. Gastroenterol. Hepatol. 18, 649–667. doi: 10.1038/s41575-021-00440-6
Salvato, F., Hettich, R. L., and Kleiner, M. (2021). Five key aspects of metaproteomics as a tool to understand functional interactions in host-associated microbiomes. PLoS Pathog. 17:e1009245. doi: 10.1371/journal.ppat.1009245
Sanches-Fernandes, G. M. M., Califano, G., Castanho, S., Soares, F., Ribeiro, L., Pousão-Ferreira, P., et al. (2022). Effects of live feed manipulation with algal-derived antimicrobial metabolites on fish larvae microbiome assembly: a molecular-based assessment. Aquac. Res. 3, 1062–1083. doi: 10.1111/are.15648
Sánchez-Alcoholado, L., Ramos-Molina, B., Otero, A., Laborda-Illanes, A., Ordóñez, R., Medina, J. A., et al. (2020). The role of the gut microbiome in colorectal cancer development and therapy response. Cancers 12:1406. doi: 10.3390/cancers12061406
Sarkar, A., Harty, S., Lehto, S. M., Moeller, A. H., Dinan, T. G., Dunbar, R. I. M., et al. (2018). The microbiome in psychology and cognitive neuroscience. Trends Cogn. Sci. 22, 611–636. doi: 10.1016/j.tics.2018.04.006
Scanlan, P. D. (2017). Bacteria-bacteriophage coevolution in the human gut: implications for microbial diversity and functionality. Trends Microbiol. 25, 614–623. doi: 10.1016/j.tim.2017.02.012
Scherz, V., Greub, G., and Bertelli, C. (2022). Building up a clinical microbiota profiling: a quality framework proposal. Crit. Rev. Microbiol. 48, 356–375. doi: 10.1080/1040841X.2021.1975642
Sender, R., Fuchs, S., and Milo, R. (2016). Revised estimates for the number of human and bacteria cells in the body. PLoS Biol. 14:e1002533. doi: 10.1371/journal.pbio.1002533
Sheflin, A. M., Whitney, A. K., and Weir, T. L. (2014). Cancer-promoting effects of microbial dysbiosis. Curr. Oncol. Rep. 16:406. doi: 10.1007/s11912-014-0406-0
Shi, Z., Li, X., Wang, X., Zhang, L., Li, L., Fu, X., et al. (2021). Characteristics and clinical implications of the nasal microbiota in extranodal NK/T-cell lymphoma nasal type. Front. Cell Infect. Microbiol. 11:686595. doi: 10.3389/fcimb.2021.686595
Shkoporov, A. N., and Hill, C. (2019). Bacteriophages of the human gut: the “known unknown” of the microbiome. Cell Host Microbe 25, 25, 195–209. doi: 10.1016/j.chom.2019.01.017
Silva, Y. P., Bernardi, A., and Frozza, R. L. (2020). The role of short-chain fatty acids from gut microbiota in gut-brain communication. Front. Endocrinol. 11:25. doi: 10.3389/fendo.2020.00025
Simon, J. C., Marchesi, J. R., Mougel, C., and Selosse, M. A. (2019). Host-microbiota interactions: from holobiont theory to analysis. Microbiome 7:5. doi: 10.1186/s40168-019-0619-4
Song, L., Sun, Q., Zheng, H., Zhang, Y., Wang, Y., Liu, S., et al. (2022). Roseburia hominis alleviates neuroinflammation via short-chain fatty acids through histone deacetylase inhibition. Mol. Nutr. Food Res. 66:e2200164. doi: 10.1002/mnfr.202200164
Soty, M., Gautier-Stein, A., Rajas, F., and Mithieux, G. (2017). Gut-brain glucose signaling in energy homeostasis. Cell Metab. 25, 1231–1242. doi: 10.1016/j.cmet.2017.04.032
Spivak, I., Fluhr, L., and Elinav, E. (2022). Local and systemic effects of microbiome-derived metabolites. EMBO Rep. 23:e55664. doi: 10.15252/embr.202255664
Srinivasan, S., Hua, X., Wu, M. C., Proll, S., Valint, D. J., Reed, S. D., et al. (2022). Impact of topical interventions on the vaginal microbiota and metabolome in postmenopausal women: a secondary analysis of a randomized clinical trial. JAMA Netw. Open 5:e225032. doi: 10.1001/jamanetworkopen.2022.5032
Steinert, R. E., Gerspach, A. C., Gutmann, H., Asarian, L., Drewe, J., and Beglinger, C. (2011). The functional involvement of gut-expressed sweet taste receptors in glucose-stimulated secretion of glucagon-like peptide-1 (GLP-1) and peptide YY (PYY). Clin. Nutr. 30, 524–532. doi: 10.1016/j.clnu.2011.01.007
Strasser, B., Gostner, J. M., and Fuchs, D. (2016). Mood, food, and cognition: role of tryptophan and serotonin. Curr. Opin. Clin. Nutr. Metab. Care 19, 55–61. doi: 10.1097/MCO.0000000000000237
Subramaniam, S., and Fletcher, C. (2018). Trimethylamine N-oxide: breathe new life. Br. J. Pharmacol. 175, 1344–1353. doi: 10.1111/bph.13959
Subramanian, I., Verma, S., Kumar, S., Jere, A., and Anamika, K. (2020). Multi-omics data integration, interpretation, and its application. Bioinform. Biol. Insights 14:1177932219899051. doi: 10.1177/1177932219899051
Sun, T., and Hevner, R. F. (2014). Growth and folding of the mammalian cerebral cortex: from molecules to malformations. Nat. Rev. Neurosci. 15, 217–232. doi: 10.1038/nrn3707
Tahara, Y., Yamazaki, M., Sukigara, H., Motohashi, H., Sasaki, H., Miyakawa, H., et al. (2018). Gut microbiota-derived short chain fatty acids induce circadian clock entrainment in mouse peripheral tissue. Sci. Rep. 8:1395. doi: 10.1038/s41598-018-19836-7
Tan, J., McKenzie, C., Potamitis, M., Thorburn, A. N., Mackay, C. R., and Macia, L. (2014). The role of short-chain fatty acids in health and disease. Adv. Immunol. 121, 91–119. doi: 10.1016/B978-0-12-800100-4.00003-9
Thaiss, C. A., Zeevi, D., Levy, M., Segal, E., and Elinav, E. (2015). A day in the life of the meta-organism: diurnal rhythms of the intestinal microbiome and its host. Gut Microbes 6, 137–142. doi: 10.1080/19490976.2015.1016690
Thaiss, C. A., Zeevi, D., Levy, M., Zilberman-Schapira, G., Suez, J., Tengeler, A. C., et al. (2014). Transkingdom control of microbiota diurnal oscillations promotes metabolic homeostasis. Cell 159, 514–529. doi: 10.1016/j.cell.2014.09.048
Theis, K. R., Dheilly, N. M., Klassen, J. L., Brucker, R. M., Baines, J. F., Bosch, T. C. G., et al. (2016). Getting the hologenome concept right: an eco-evolutionary framework for hosts and their microbiomes. mSystems 1. doi: 10.1128/mSystems.00028-16
Tran, T. T. T., Cousin, F. J., Lynch, D. B., Menon, R., Brulc, J., Brown, J. R. M., et al. (2019). Prebiotic supplementation in frail older people affects specific gut microbiota taxa but not global diversity. Microbiome 7:39. doi: 10.1186/s40168-019-0654-1
Trebicka, J., Bork, P., Krag, A., and Arumugam, M. (2021). Utilizing the gut microbiome in decompensated cirrhosis and acute-on-chronic liver failure. Nat. Rev. Gastroenterol. Hepatol. 18, 167–180. doi: 10.1038/s41575-020-00376-3
Uronis, J. M., Arthur, J. C., Keku, T., Fodor, A., Carroll, I. M., Cruz, M. L., et al. (2011). Gut microbial diversity is reduced by the probiotic VSL#3 and correlates with decreased TNBS-induced colitis. Inflamm. Bowel Dis. 17, 289–297. doi: 10.1002/ibd.21366
Utzschneider, K. M., Kratz, M., Damman, C. J., and Hullar, M. (2016). Mechanisms linking the gut microbiome and glucose metabolism. J. Clin. Endocrinol. Metab. 101, 1445–1454. doi: 10.1210/jc.2015-4251
Uzbay, T. (2019). Germ-free animal experiments in the gut microbiota studies. Curr. Opin. Pharmacol. 49, 6–10. doi: 10.1016/j.coph.2019.03.016
van Olden, C., Groen, A. K., and Nieuwdorp, M. (2015). Role of intestinal microbiome in lipid and glucose metabolism in diabetes mellitus. Clin. Ther. 37, 1172–1177. doi: 10.1016/j.clinthera.2015.03.008
Vaughn, B. P., Fischer, M., Kelly, C. R., Allegretti, J. R., Graiziger, C., Thomas, J., et al. (2023). Effectiveness and safety of colonic and capsule fecal microbiota transplantation for recurrent Clostridioides difficile infection. Clin. Gastroenterol. Hepatol. 21, 1330–1337.e2. doi: 10.1016/j.cgh.2022.09.008
Villageliu, D., and Lyte, M. (2018). Dopamine production in Enterococcus faecium: a microbial endocrinology-based mechanism for the selection of probiotics based on neurochemical-producing potential. PLoS One 13:e0207038. doi: 10.1371/journal.pone.0207038
Vogtmann, E., Chen, J., Amir, A., Shi, J., Abnet, C. C., Nelson, H., et al. (2017). Comparison of collection methods for fecal samples in microbiome studies. Am. J. Epidemiol. 185, 115–123. doi: 10.1093/aje/kww177
Wachsmuth, H. R., Weninger, S. N., and Duca, F. A. (2022). Role of the gut-brain axis in energy and glucose metabolism. Exp. Mol. Med. 54, 377–392. doi: 10.1038/s12276-021-00677-w
Wahlstrom, A., Sayin, S. I., Marschall, H. U., and Backhed, F. (2016). Intestinal crosstalk between bile acids and microbiota and its impact on host metabolism. Cell Metab. 24, 41–50. doi: 10.1016/j.cmet.2016.05.005
Wang, J. W., Kuo, C. H., Kuo, F. C., Wang, Y. K., Hsu, W. H., Yu, F. J., et al. (2019). Fecal microbiota transplantation: review and update. J. Formos. Med. Assoc. 118, S23–S31. doi: 10.1016/j.jfma.2018.08.011
Wang, S. P., Rubio, L. A., Duncan, S. H., Donachie, G. E., Holtrop, G., Lo, G., et al. (2020). Pivotal roles for pH, lactate, and lactate-utilizing bacteria in the stability of a human colonic microbial ecosystem. mSystems 5:e00645. doi: 10.1128/mSystems.00645-20
Wang, Z., Wang, Q., Zhao, J., Gong, L., Zhang, Y., Wang, X., et al. (2019). Altered diversity and composition of the gut microbiome in patients with cervical cancer. AMB Express 9:40. doi: 10.1186/s13568-019-0763-z
Wang, L. J., Yang, C. Y., Chou, W. J., Lee, M. J., Chou, M. C., Kuo, H. C., et al. (2020). Gut microbiota and dietary patterns in children with attention-deficit/hyperactivity disorder. Eur. Child Adolesc. Psychiatry 29, 287–297. doi: 10.1007/s00787-019-01352-2
Wardeh, M., Risley, C., McIntyre, M. K., Setzkorn, C., and Baylis, M. (2015). Database of host-pathogen and related species interactions, and their global distribution. Sci. Data 2:150049. doi: 10.1038/sdata.2015.49
Weinstock, G. M. (2012). Genomic approaches to studying the human microbiota. Nature 489, 250–256. doi: 10.1038/nature11553
Wexler, H. M. (2007). Bacteroides: the good, the bad, and the nitty-gritty. Clin. Microbiol. Rev. 20, 593–621. doi: 10.1128/CMR.00008-07
Wishart, D. S. (2019). Metabolomics for investigating physiological and pathophysiological processes. Physiol. Rev. 99, 1819–1875. doi: 10.1152/physrev.00035.2018
Wishart, D. S., Cheng, L. L., Copié, V., Edison, A. S., Eghbalnia, H. R., Hoch, J. C., et al. (2022). NMR and metabolomics-a roadmap for the future. Metabolites 12:678. doi: 10.3390/metabo12080678
Xia, B., Wang, J., Zhang, D., and Hu, X. (2023). The human microbiome links to prostate cancer risk and treatment (review). Oncol. Rep. 49:123. doi: 10.3892/or.2023.8560
Xu, C., Lee, S. K., Zhang, D., and Frenette, P. S. (2020). The gut microbiome regulates psychological-stress-induced inflammation. Immunity 53, 417–428.e4. doi: 10.1016/j.immuni.2020.06.025
Yano, J. M., Yu, K., Donaldson, G. P., Shastri, G. G., Ann, P., Ma, L., et al. (2015). Indigenous bacteria from the gut microbiota regulate host serotonin biosynthesis. Cell 161, 264–276. doi: 10.1016/j.cell.2015.02.047
Yao, G., Kang, L., Li, J., Long, Y., Wei, H., Ferreira, C. A., et al. (2018). Effective weight control via an implanted self-powered vagus nerve stimulation device. Nat. Commun. 9:5349. doi: 10.1038/s41467-018-07764-z
Yung, D. E., Rondonotti, E., and Koulaouzidis, A. (2016). Review: capsule colonoscopy-a concise clinical overview of current status. Ann. Transl. Med. 4:398. doi: 10.21037/atm.2016.10.71
Zaky, A., Glastras, S. J., Wong, M. Y. W., Pollock, C. A., and Saad, S. (2021). The role of the gut microbiome in diabetes and obesity-related kidney disease. Int. J. Mol. Sci. 22:9641. doi: 10.3390/ijms22179641
Zeng, H., Umar, S., Rust, B., Lazarova, D., and Bordonaro, M. (2019). Secondary bile acids and short chain fatty acids in the colon: a focus on colonic microbiome, cell proliferation, inflammation, and cancer. Int. J. Mol. Sci. 20:1214. doi: 10.3390/ijms20051214
Zhang, X., Li, L., Butcher, J., Stintzi, A., and Figeys, D. (2019). Advancing functional and translational microbiome research using meta-omics approaches. Microbiome 7:154. doi: 10.1186/s40168-019-0767-6
Zhang, L., Liu, C., Jiang, Q., and Yin, Y. (2021). Butyrate in energy metabolism: there is still more to learn. Trends Endocrinol. Metab. 32, 159–169. doi: 10.1016/j.tem.2020.12.003
Zheng, Y., Fang, Z., Xue, Y., Zhang, J., Zhu, J., Gao, R., et al. (2020). Specific gut microbiome signature predicts the early-stage lung cancer. Gut Microbes 11, 1030–1042. doi: 10.1080/19490976.2020.1737487
Zheng, W., Zhao, S., Yin, Y., Zhang, H., Needham, D. M., Evans, E. D., et al. (2022). High-throughput, single-microbe genomics with strain resolution, applied to a human gut microbiome. Science 376:eabm1483. doi: 10.1126/science.abm1483
Zhu, Z., Ren, J., Michail, S., and Sun, F. (2019). MicroPro: using metagenomic unmapped reads to provide insights into human microbiota and disease associations. Genome Biol. 20:154. doi: 10.1186/s13059-019-1773-5
Zolkiewicz, J., Marzec, A., Ruszczynski, M., and Feleszko, W. (2020). Postbiotics-a step beyond pre- and probiotics. Nutrients 12:2189. doi: 10.3390/nu12082189
Keywords: metabolite, microbiome, metagenomics, holobiont, fecal microbes, gut brain axis, microbial diversity, small molecules
Citation: Chakraborty N (2024) Metabolites: a converging node of host and microbe to explain meta-organism. Front. Microbiol. 15:1337368. doi: 10.3389/fmicb.2024.1337368
Edited by:
Bernie Daigle, University of Memphis, United StatesCopyright © 2024 Chakraborty. This is an open-access article distributed under the terms of the Creative Commons Attribution License (CC BY). The use, distribution or reproduction in other forums is permitted, provided the original author(s) and the copyright owner(s) are credited and that the original publication in this journal is cited, in accordance with accepted academic practice. No use, distribution or reproduction is permitted which does not comply with these terms.
*Correspondence: Nabarun Chakraborty, TmFiYXJ1bi5DaGFrcmFib3J0eTIuY2l2QGhlYWx0aC5taWw=