- 1Department of Horticulture, Auburn University, Auburn, AL, United States
- 2Department of Food Science and Technology, University of Georgia, Athens, GA, United States
Fresh produce can be contaminated by enteric pathogens throughout crop production, including through contact with contaminated agricultural water. The most common outbreaks and recalls in fresh produce are due to contamination by Salmonella enterica and Shiga toxin-producing E. coli (STEC). Thus, the objectives of this study were to investigate the prevalence of markers for STEC (wzy, hly, fliC, eaeA, rfbE, stx-I, stx-II) and Salmonella (invA) in surface water sources (n = 8) from produce farms in Southwest Georgia and to determine correlations among the prevalence of virulence markers for STEC, water nutrient profile, and environmental factors. Water samples (500 mL) from eight irrigation ponds were collected from February to December 2021 (n = 88). Polymerase chain reaction (PCR) was used to screen for Salmonella and STEC genes, and Salmonella samples were confirmed by culture-based methods. Positive samples for Salmonella were further serotyped. Particularly, Salmonella was detected in 6/88 (6.81%) water samples from all ponds, and the following 4 serotypes were detected: Saintpaul 3/6 (50%), Montevideo 1/6 (16.66%), Mississippi 1/6 (16.66%), and Bareilly 1/6 (16.66%). Salmonella isolates were only found in the summer months (May-Aug.). The most prevalent STEC genes were hly 77/88 (87.50%) and stx-I 75/88 (85.22%), followed by fliC 54/88 (61.63%), stx-II 41/88 (46.59%), rfbE 31/88 (35.22%), and eaeA 28/88 (31.81%). The wzy gene was not detected in any of the samples. Based on a logistic regression analysis, the odds of codetection for STEC virulence markers (stx-I, stx-II, and eaeA) were negatively correlated with calcium and relative humidity (p < 0.05). A conditional forest analysis was performed to assess predictive performance (AUC = 0.921), and the top predictors included humidity, nitrate, calcium, and solar radiation. Overall, information from this research adds to a growing body of knowledge regarding the risk that surface water sources pose to produce grown in subtropical environmental conditions and emphasizes the importance of understanding the use of abiotic factors as a holistic approach to understanding the microbial quality of water.
Introduction
The consumption of fruits and vegetables has significantly increased over the past few decades as people have become more health-conscious and aware of the benefits of a balanced diet (Machado-moreira et al., 2019; Balali et al., 2020). Fresh produce is often consumed raw and without a cooking step, which increases the risk of foodborne infections (Falardeau et al., 2017; Quintanilla Portillo et al., 2022). The increase in fruit and vegetable production also increases the number of recalls and foodborne outbreaks associated with fresh produce that are commonly reported due to microbial contamination (Wadamori et al., 2017). During crop production, several factors can contribute to microbial contamination, including animals as natural carriers of enteric pathogens or humans if good agricultural practices are not properly followed. Those pathogens can end up in the soil (i.e., manure) and agricultural water (leakage of septic tanks) (Strawn et al., 2013b). Particularly, water is essential for plant development, and the monitoring of microbial quality, as well as good agricultural practices, is critical to minimize risks associated with microbial contamination. This is relatively potentiated with the establishment of food safety practices to protect the safety of fresh produce (Truitt et al., 2018; Devarajan et al., 2023). Previous studies have investigated the prevalence of foodborne pathogens in irrigation water sources for vegetable crops (McEgan et al., 2013; Strawn et al., 2013b; Falardeau et al., 2017; Topalcengiz et al., 2017; Truitt et al., 2018; Weller et al., 2020a, 2020b; Belias et al., 2021; Murphy et al., 2022). Although there is a substantial body of research available, the existing studies exhibit a notable degree of inconsistency, often contradicting each other, especially when evaluating abiotic factors and pathogen prevalence. Our research seeks to better understand the use of abiotic factors as predictors of pathogens by using machine learning models and, more specifically, in subtropical environmental conditions characterized by frequent rainfall events during hot summers and dry periods during the winter, which can significantly influence crop management practices and pathogens’ survival.
Surface water is the most susceptible water source used in irrigation systems to contamination with biological hazards, including enteric pathogens like Salmonella enterica (S. enterica) and Escherichia coli (E. coli) (Rodrigues et al., 2020; Gurtler and Gibson, 2022). Once contaminated water is used in the production of fresh produce, there is a significant food safety risk and a public health concern. Finding the sources of bacterial contamination in produce whenever an outbreak occurs can be complex due to several factors, including the short shelf life of the produce, the need for patients to recall their food consumption history, and tracing back the origin of the bacteria strain recovered from the patient back to its source (e.g., water), therefore, addressing produce contamination remains challenging. Nonetheless, agricultural water has been identified as a source of contamination in numerous outbreaks and from a variety of produce, including tomatoes, cucumbers, and leafy greens (Ackers et al., 1998; Greene et al., 2008; Laughlin et al., 2019; CDC, 2019a,b). Water has been associated with two of the most recent significant outbreaks in the United States linked to fresh produce. One of these outbreaks was caused by Salmonella Newport infections linked to onions (CDC, 2020) that sickened over 1,000 people, while the other was caused by E. coli O157:H7 infections associated with romaine lettuce that sickened over 200 people (CDC, 2019b).
The southeastern United States is an important vegetable production region, especially the state of Georgia, contributing to 2.8 billion dollars to the state’s economy. Some of the most common vegetables grown in the study region include cucumbers, bell peppers, broccoli, watermelons, onions, and other fresh produce (Kane, 2023). To provide water for the extensive vegetable cultivated area, Georgia relies on a daily irrigation demand of over two billion liters, of which surface water is one of the primary sources (Painter, 2019; Kane, 2023). Previous studies on the microbial quality of water have been conducted across Georgia (Jenkins et al., 2012; Antaki et al., 2016; Harris et al., 2018) and other southern states, including Florida (Strawn et al., 2014; Murphy et al., 2022) and Virginia (Truitt et al., 2018; Gu et al., 2019). None of these studies implemented source tracking methodologies and only credited the presence of Salmonella in water due to factors like geography, land use, ecology, and seasonality (Rajabi et al., 2011; Li et al., 2014; Luo et al., 2015; Maurer et al., 2015).
Further studies have assessed the presence and concentration of Salmonella, Shiga toxin-producing Escherichia coli (STEC), and generic E. coli in irrigation water in Southern Georgia and Virginia and analyzed the relationship with different environmental factors, including total rainfall, pH, water temperature, dissolved oxygen concentration, oxidation–reduction potential, turbidity, and humidity (Gu et al., 2013, 2019; Antaki et al., 2016). In water sources from Georgia, Antaki et al. (2016) did not find correlations between generic E. coli and Salmonella. On the contrary, Gu et al. (2013) indicated positive correlations between E. coli O157 and fecal coliforms, temperature, and rainfall. Similar results were reported in Virginia, where Salmonella increased after the rising of air temperature and the presence of rainfall events (Gu et al., 2019).
All these aforementioned studies have identified the prevalence of pathogens and correlations with environmental factors; however, only one study has evaluated the codetection of virulence factors of STEC (Gu et al., 2013), and none of the previous studies have evaluated the genetic markers (fliC, wzy, rfbE, and hly) with a wide range of nutrients and environmental factors in ponds used for irrigation. Understanding the behavior and prevalence of STEC and Salmonella with abiotic factors at the farm level can be beneficial for creating predictive models. Using physicochemical data in conjunction with microbial testing can provide insights into the potential presence of enteric pathogens in water and support science-based decision-making processes. In addition, corrective actions (i.e., use of microbial die-off, water treatment, or suspension of water use) can be taken in advance to prevent and reduce pathogen contribution to fresh produce. Considering that STEC and Salmonella remain common etiological agents for produce-related outbreaks (Belias et al., 2021). Implementing mitigation strategies for microbial prediction is critical to protecting public health. Recognizing that several water sources in the southeastern United States have been reported to be contaminated with foodborne pathogens, it is relevant to identify factors associated with the prevalence of foodborne pathogens in these water sources. Thus, the objectives of this study were (1) to investigate the prevalence of foodborne pathogens (STEC and Salmonella) in surface water sources from a large produce-growing area in the Southeastern United States and (2) to determine the correlation among the prevalence of foodborne pathogens, fecal indicator bacteria, and abiotic factors.
Materials and methods
Irrigation ponds and water sampling
Eight irrigation ponds from large produce farms located in Southwest Georgia, United States, were selected for water sampling. All ponds were located within a 32-km radius of each other. The agricultural and physical characteristics of each pond area are described in Table 1. Surface water samples (500 mL) were collected monthly with a sterile amber glass bottle and transported to the laboratory in a cooler with ice. Water samples were collected from February to December 2021 (n = 88). A volume of 150 mL from each sample was filtered via the membrane filtration method through a 47 mm diameter, 0.45 μm pore-size sterile filter (Pall Corporation, Ann Arbor, Michigan, United States) as described by Topalcengiz et al. (2017). Filters were placed in Whirl-Pak bags and stored at −80°C (no cryo-protectant was used), until further processing for identification of Salmonella and STEC markers. Enumeration of generic E. coli was conducted by using the IDEXX Colilert with Quanti-Tray® 2000 (IDEXX Laboratories, Westbrook, ME, United States) method, as described by Haley et al. (2022).
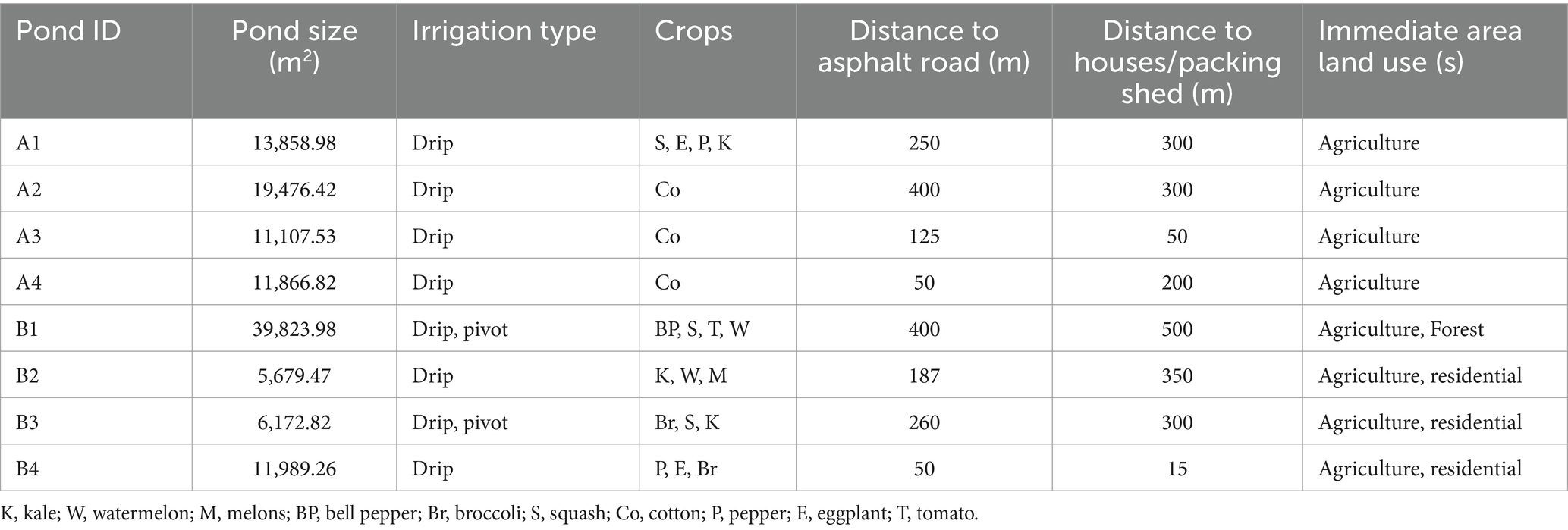
Table 1. Characteristics of the eight irrigation ponds surveyed for STEC markers and Salmonella in Southwest Georgia.
Isolation of Salmonella and STEC genes
The laboratory analysis was performed based on the methodology described by Topalcengiz et al. (2017). Briefly, to determine the prevalence of STEC, 25 mL of modified peptone water with pyruvate (mBPWp; Neogen, Lansing, MI, United States) was added to frozen filters and incubated at 35 ± 1°C for 24 h. For Salmonella, 100 μL of the pre-enrichment (mBPWp) was added into 10 mL of Rappaport-Vassiliadis (RV; Difco, Bectin, Dickinson, Sparks, MD, United States) and incubated at 42 ± 1°C for 48 h. Subsequently, DNA was extracted directly from mBPWp and RV using a DNeasy microbial kit (DNeasy® UltraClean® Microbial Kit, Qiagen, United States). Next, polymerase chain reaction (PCR) was performed to determine the presence of the markers for Salmonella (invA) and STEC (hly, rfbE, flic, stx-I, stx-II, eaeA, and wzy). The primer sequences used are listed in Table 2. Salmonella Braenderup (ATCC BAA-664) and E. coli O157:H7 (ATCC 43895) were used as positive and negative controls, respectively. A 50 mL PCR reaction for Salmonella and STEC markers consisted of 25 μL of Master Mix (GoTaq®Green Master Mix, Promega, Madison, WI, United States), 5 μL of each primer (reverse and forward), 5 μL of DNA template, and 10 μL of molecular biology grade water (Corning, Mediatech, Manassas, VA, United States). The PCR conditions for invA were: 1 min at 94°C for melting, followed by 35 cycles of 94°C for 1 min, 58.3°C for 30 s, and 72°C for 30 s with a final elongation of 72°C for 7 min, as previously described by Yanestria et al. (2019). For STEC, the PCR conditions were: 10 min at 95°C for melting, followed by 20 cycles of 94°C for 30 s, 59°C for 1 min with 0.5°C decreasing temperature per cycle, 72°C for 1 min, and a second 20 cycles of 94\u00B0C for 30 s, 49°C for 1 min, 72°C for 1 min and with a final elongation of 72°C for 7 min as previously described by Topalcengiz et al. (2017). For the gene wzy, the conditions used were the same as described by Iguchi et al. (2017): 25 cycles of 94°C for 30 s, 58°C for 30 s, and 72°C for 1 min. Lastly, gel electrophoresis was conducted on 1.5% agarose gel with 3 μL of ethidium bromide (Fisher Scientific, Fair Lawn, NJ, United States) 1X TAE buffer at 90 V for 35 min.
Salmonella isolation and serotyping
Salmonella-positive samples on PCR were enriched following the FDA Bacteriological Analytical Manual (BAM) to obtain a culture for further serotyping (Andrews et al., 2023). Samples were enriched by streaked onto bismuth sulfite agar (BSA; Difco, Becton, Dickinson, Sparks, MD, United States), xylose-lysine-desoxycholate (XLD; Difco, Becton, Dickinson, Sparks, MD, USA), and hektoen enteric agar (HE; Difco, Becton, Dickinson, Sparks, MD, United States). XLD and HE were incubated at 35 ± 2°C for 24 h, and BSA was incubated at 35 ± 2°C for 48 h. Following incubation, if typical colonies were present, they were transferred onto triple sugar iron agar (TSI; Difco, Becton, Dickinson, Sparks, MD, United States) and lysine iron agar (LIA; Difco, Becton, Dickinson, Sparks, MD, United States) slants. For serotyping, isolates were streaked onto xylose-lysine-tergitol-4 agar (XLT4; Difco, Bectin, Dickinson, Sparks, MD, United States) and incubated at 35 ± 2°C for 24 h. All Salmonella isolates were also confirmed using a latex agglutination test (Oxoid, Hampshire, United Kingdom) and biochemical assays (urea, triple sugar iron agar, and lysine iron agar) before shipping isolates to the National Veterinary Services Laboratories (USDA, NVSL, Ames, Iowa, United States) for serotyping.
Nutrient analysis for water
A 50 mL volume of each water sample was sent to the Soil, Forage, and Water Testing Laboratory at Auburn University for nutrient analysis. The analyzed nutrients included routine elements by ICAP (calcium, magnesium, potassium, phosphorous, copper, iron, manganese, zinc, boron, aluminum, cadmium, chromium, lead, sodium, nickel, and nitrate-n). The laboratory also provided information on water pH, soluble salts, and electrical conductivity. However, boron, cadmium, and chromium were below the limit of detection (<0.1) and were not included in the statistical analysis.
Weather information collection
Weather information, including air temperature, solar radiation, and rain data, was obtained from the University of Georgia Weather Network.1 The closest weather stations to the ponds were located in Moultrie, Bowen, Tifton, and Ty Ty, GA.
Statistical analysis
All data cleaning, visualization, and statistical analyses were performed using R Statistical Software [version 4.2.3 (2023-04-21 ucrt)]. All the analyses were performed for STEC virulence markers codetection, which indicated that virulence genes were present in the same sample (stx-I, stx-II, and eaeA). Logistic regression was implemented to determine the association of abiotic factors with the odds of codetection for virulent markers of STEC, and conditional forest analysis was further implemented to estimate whether those variables could be used to predict the codetection of STEC markers.
For logistic regression, all the numerical variables were scaled using the “caret” package as described by Belias et al. (2021). All the variables used for the analysis are listed in the Supplementary material (see Supplementary Tables 1, 2). To prevent multicollinearity in the logistic regression, a correlation matrix (see Supplementary Figure 1) using the “corrplot” package (Wei et al., 2021) was developed, and variables with strong correlation (>0.60) were omitted from the overarching model. In instances where two or more variables displayed significant correlation, one of them was systematically excluded. The global model for logistic regression included the following variables: rainfall before sampling (24 h, 48 h, and 7 days), generic E. coli (data not shown), phosphorus, nitrate, calcium, pH, humidity, and solar radiation. Additionally, pond and month of sampling were used as random effects to include temporal and special autocorrelation. To determine the best model, a stepwise regression was used based on AIC (Akaike Information Criterion). The best model was determined by the lowest AIC (72.5) and included the variables: calcium, nitrate, humidity, and solar radiation (see Table 3, Supplementary Table 3). The variance influence factor (VIF) was used to assess multicollinearity.

Table 3. Logistic regression analysis for the association between codetection of STEC virulence markers, nutrients in water, and environmental factors.
Subsequently, using the “party” and “mlr” packages (Strobl et al., 2008; Belias et al., 2021; Hothorn et al., 2023), a conditional forest analysis was implemented. This analysis was conducted as an alternative option to predict STEC marker detection in water samples, as this analysis is robust to a large number of predictors and small sample sizes, as described by Belias et al. (2021). To maximize the area under the curve (AUC), repeated iterations of 10-fold cross-validation were used for tuning hyperparameters (mtry). For each forest, 20,000 trees were produced. Additionally, the variable of importance values was determined, and the top 10 predictors were extracted (Figure 1). Next, models were trained, tested, and cross-validated with our own data set. Although there are potential limitations of training the same data set, due to the nature of our experiment, this analysis represented a good fit, as we have various predictors and a small sample size.
Results
Farms included in this study represent large vegetable-growing operations in the region. Crops grown on these farms included tomatoes, zucchini, kale, cabbage, watermelon, peppers, cotton, and eggplant (Table 1). A total of 88 samples were collected and analyzed as a part of the study. As a result, data obtained include serotypes of Salmonella (Table 4), the detection of STEC markers (Table 5), and the relationship with abiotic factors (Table 3).
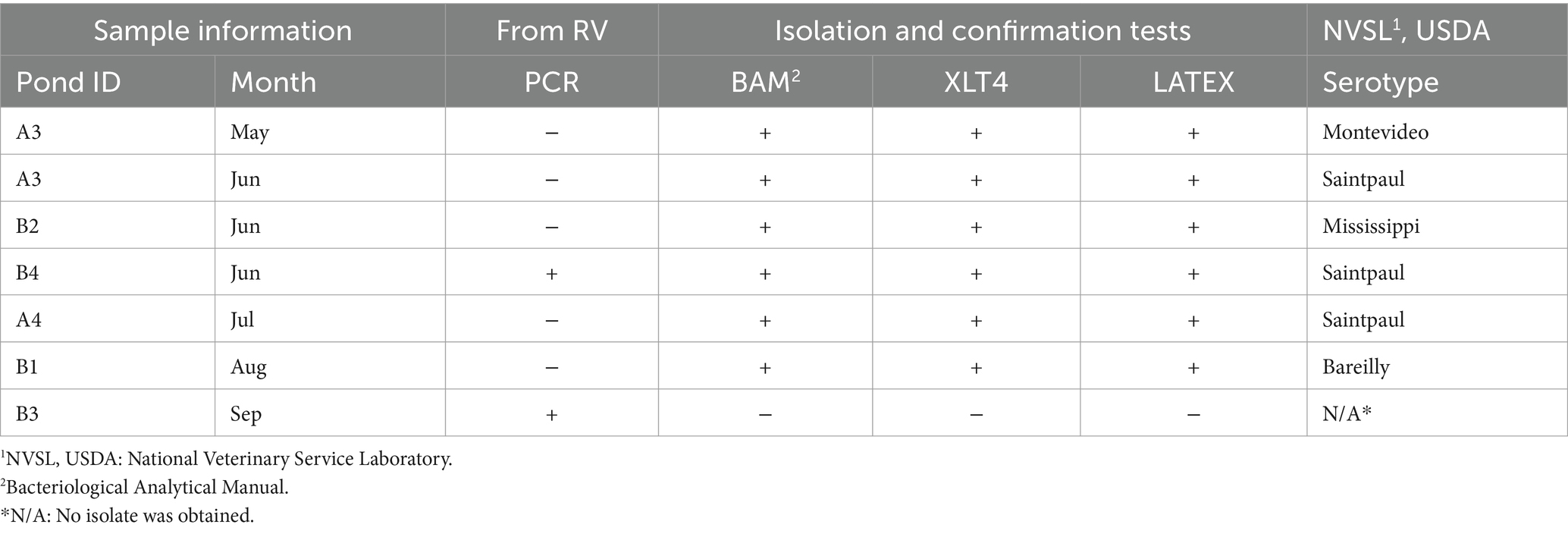
Table 4. List of Salmonella serotypes isolated from surface water and list of tests applied to invA positive samples.
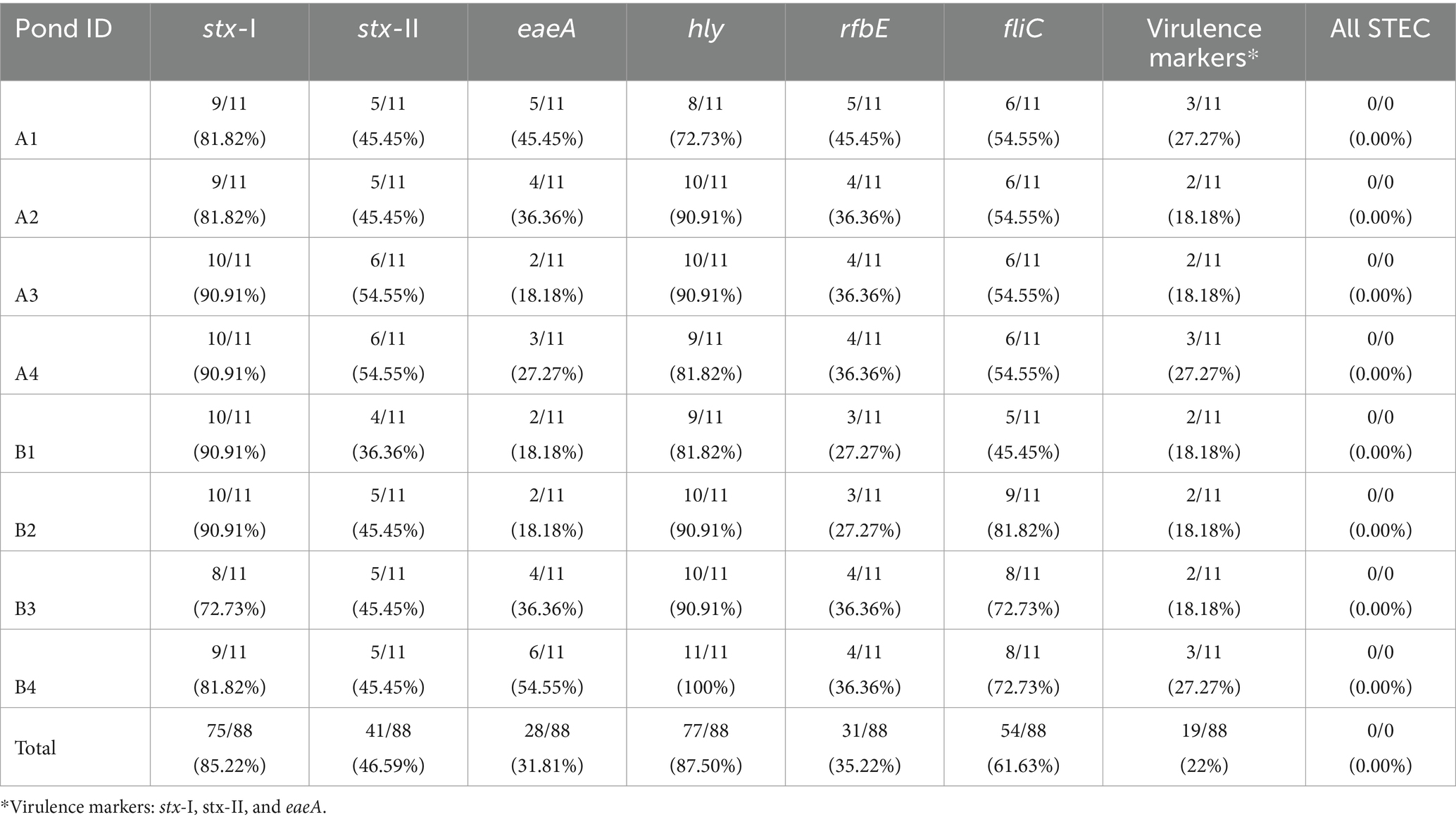
Table 5. Percentages of STEC markers (%) for each Southwest Georgia pond over 11 months of sampling.
Seasonal distribution of Salmonella
The overall prevalence of Salmonella in water samples was 6.81% (6/88 samples; Table 4). Four serovars were detected in 5 ponds (A3, A4, B1, B2, and B4). Pond A3 reported two serotypes of Salmonella (Montevideo and Saintpaul) for two consecutive months (May and June). Salmonella Bareilly and Mississippi were detected only once in ponds B1 and B2, respectively. A logistic regression was conducted; however, none of the parameters evaluated represented statistical significance (data not shown) for Salmonella. Discrepancies between methods were measured using PCR and culture-based methods. The PCR identified only two positive Salmonella samples, while culture-based methods identified 6 Salmonella-recovered samples.
STEC markers distribution from irrigation ponds
All STEC markers were detected throughout the sampling period (February–December 2021), except for the gene wzy (Table 5). None of the samples had all seven genes present simultaneously; however, at least one of the six genes was detected in 97.72% of the samples. Each pond had the following individual STEC genes with the corresponding percentage of detection: hly (87.50%), stx-I (85.22%), fliC (61.63%), stx-II (46.59%), rfbE (35.22), eaeA (31.81%), and wzy (0%). The frequency of individual genes varied among the samples, but the genes stx-I and hly were reported in over 70% of individual samples. The genes eaeA and rfbE were reported less frequently compared to the rest of the markers.
Codetection of STEC virulence markers (stx-I, stx-II, and eaeA)
Twenty-two percent (19/88) of the samples were screened positive for the genes eaeA and stx (stx-I and stx-II) (Table 5). The occurrence of STEC virulence markers (stx-I, stx-II, and eaeA) was correlated with abiotic factors, including nutrients in water (i.e., calcium and nitrate), solar radiation, and humidity (Table 3). The likelihood of STEC virulence markers codetection in the ponds was negatively correlated with calcium and humidity. There was no other significant correlation between the STEC virulence markers and abiotic factors or fecal indicator bacteria (generic E. coli). The area under the curve for the logistic regression model was 0.86. In addition, a conditional forest analysis was used to predict the codetection of STEC virulence markers and the top ten ranked predictors based on the variable of importance values (Figure 1). Among the top ten predictors, the following categories of abiotic factors were included: nutrients (i.e., nitrate, calcium, aluminum, and magnesium), weather-related predictors (solar radiation, humidity, air temperature, rainfall events 24 h and 7 days prior to sampling), and one water physicochemical parameter (soluble salts). From those 10 predictors, our global model for logistic regression included at least seven variables; the remaining (soluble salts, air temperature, and magnesium) were not included to prevent multicollinearity. In addition, our “best model” determined by the stepwise regression was able to select the four most important variables obtained in the conditional forest analysis. The AUC for the conditional forest analysis was 0.9214.
Discussion
This study was conducted to (i) investigate the prevalence of foodborne pathogens (i.e., STEC and Salmonella) in surface water sources from a large produce-growing area in the Southeastern United States and (ii) to determine the correlation among the prevalence of foodborne pathogens, fecal indicator bacteria (generic E. coli), and abiotic factors. Results provide insight into microbial water quality in Southwest Georgia, elucidating the use of abiotic factors as predictors of food safety hazards in surface agricultural water. A logistic regression was used to identify associations between abiotic factors and the presence of foodborne pathogens, and a conditional forest analysis was used as a prediction tool for the presence of STEC virulence markers. The results of this study can be further used to predict pathogen interaction with abiotic factors in subtropical environments.
Federal agencies like the Environmental Protection Agency (EPA) have established standards recommending the utilization of fecal indicator organisms like generic E. coli to determine microbial water quality. The Food Safety Modernization Act (FSMA) Produce Safety Rule previously stressed the use of microbial water testing by following EPA-approved methods, but with the new proposed Subpart E of the regulation, that is no longer a federal requirement, but it is still recommended as part of water assessment (US EPA, 2014; FDA, 2022). However, multiple studies have raised concerns about the poor correlation between generic E. coli and the presence of foodborne pathogens (Benjamin et al., 2013; Gu et al., 2013; Antaki et al., 2016; Weller et al., 2020b; Belias et al., 2021). Our results align with existing literature, as no correlation was measured between generic E. coli and the codetection of virulent genes for STEC and Salmonella, highlighting the limitations of solely relying on fecal indicators for pathogen incidence (Benjamin et al., 2013; Antaki et al., 2016; Falardeau et al., 2017; Topalcengiz et al., 2017; Liu et al., 2018; Gurtler and Gibson, 2022; Murphy et al., 2022; Xu et al., 2022). Findings in this study suggest that abiotic factors play a crucial role in the prevalence of foodborne pathogens and understanding them when conducting a holistic approach to agricultural water risk assessment is crucial.
Salmonella findings: prevalence and serotypes
The identification of Salmonella in six out of the eight assessed ponds, either through culture or PCR, underscores the complexity and challenges encountered in environmental sample analysis. While the initial focus was on PCR for Salmonella detection, only two samples yielded positive results, prompting the adoption of the culture method. Despite the validation of the PCR reaction with a control (Salmonella Braenderup), negative results were observed, a common situation in environmental samples characterized by a diverse microbial community (Sipos et al., 2010). Plausible explanations for these findings include potential sample contamination during processing, primer cross-reaction, and the presence of low concentrations of targeted DNA. On the contrary, it is plausible to infer that samples exclusively identified through PCR may represent viable but non-culturable (VBNC) Salmonella or even dead cells, adding a layer of complexity to the interpretation of results. This can happen in water sources as the bacteria are stressed due to harsh conditions (Liu et al., 2018; Weller et al., 2020b; Stocker et al., 2022). For this study, no correlations were identified between the prevalence of Salmonella and the abiotic factors. However, all the Salmonella isolates were identified only in the summer months (May-Sept), where there is often an increase in air temperatures. Results are consistent with previous studies in the region regarding seasonality and temperature (Cooley et al., 2014; Luo et al., 2015; Murphy et al., 2022). The serotypes identified in the present study have previously been listed among the top 20 most reported serotypes associated with Salmonella infections in humans and have been previously isolated from water sources from Georgia (Luo et al., 2015; Antaki et al., 2016; Harris et al., 2018; Deaven et al., 2021) and other states (Truitt et al., 2018). The prevalence of Salmonella in the ponds was substantially lower when compared to previous studies in Georgia and surrounding states; for instance, we isolated Salmonella from 8% (7/88) of the samples by filtering a volume of 150 mL of water, as previously described. In contrast, studies in southern Georgia reported higher rates, but these were observed in larger water volumes, with 11.0% (34/285) of samples containing Salmonella, each sample having a total of 880 mL of water (Antaki et al., 2016), and 49% (52/107) in studies where a total volume of 1.8 L was analyzed (Harris et al., 2018). However, the type of surface water might impact the microflora. For example, rivers, lakes, or ponds have different water flows and geological characteristics. In the Mid-Atlantic region of the United States, researchers reported a higher prevalence of Salmonella in water collected from rivers compared to ponds (Sharma et al., 2020), which may justify the lower numbers of Salmonella isolates detected in the ponds evaluated in the current study.
Additional factors such as land use, the presence of wild animals, and concentrated animal feeding operations (CAFO) are relevant factors for microbial contamination (Strawn et al., 2013b). As surface water is exposed to the environment and animals are more susceptible to microbial contamination, wild animal activity (e.g., birds, reptiles, wild hogs) was observed in all the ponds’ surrounding areas, which may have contributed to the ponds’ microflora (Rocha et al., 2022). Poultry operations are often with a source of Salmonella and Campylobacter jejuni (Vereen et al., 2013; Bardsley et al., 2021). Considering that poultry is one of the major commodities in Georgia (Kane, 2023), it is possible that the prevalence of Salmonella in Georgia could be attributed to the presence of such operations. In our study, at least three poultry farms were within 5–8 km of pond A and at least 5 km from pond B1.
Detection of Shiga toxin-producing Escherichia coli
E. coli O157:H7 might have been present in the water sources as 6/88 samples had rbfE and stx (stxI and stxII) genes simultaneously. Detecting both stx and O157 markers is a good indicator of the potential presence of E. coli O157:H7 (Franz et al., 2007; Feng et al., 2021). Studies conducted in southern Georgia isolated E. coli O157:H7 in surface water (Jenkins et al., 2012; Gu et al., 2013). STEC is frequently associated with ruminants, mainly with cattle (Jay et al., 2007; Jenkins et al., 2011; Munns et al., 2015). None of the farms in this study had animal operations within the farm, and the closest cattle operation was 7 km from Pond A and at least 5 km from Pond B1. STEC can also be associated with human fecal markers (Weller et al., 2020b). All evaluated ponds had either houses or packinghouses within less than 500 m of proximity, which may have impacted the water quality. The other genes were detected throughout all the sampling time and in different percentages (Table 4), but hly (77/88, 87.5%) and stx-I (75/88, 85.22%) were found with more frequency, indicating the presence of Shiga toxin (stx-I) and hemolysin which can also be found in other pathotypes of E. coli, including extraintestinal pathogenic E. coli (Wyborn et al., 2004). When comparing all the genes for STEC, the genes reported with less frequency were eaeA (28/88), followed by rbfE (31/88). This differs from the finding of Haymaker et al. (2019), who measured STEC genes in 10 liters of water and reported that the gene eaeA (88/510) was the most frequently detected in water sources from the Mid-Atlantic region of the United States.
Although this study exclusively aimed to detect specific STEC virulence markers without utilizing culture-based methods or whole-genome sequencing (WGS) to isolate and identify individual bacterial strains due to their complexity, this approach may limit the interpretation of PCR signals, especially in cases involving multiple serotypes. Therefore, it is crucial to exercise caution when interpreting PCR results, particularly in scenarios with the potential presence of multiple serotypes. For future studies, researchers can improve the accuracy and reliability of findings by integrating complementary techniques such as bacterial isolation and WGS, thereby facilitating a more comprehensive understanding of microbial populations and their associated virulence factors.
Codetection of STEC virulence markers (stx-I, stx-II, and eaeA)
The codetection of eaeA and stx genes (I and II) was evaluated in this study to determine the presence of Shiga toxin-producing E. coli genes. Although E. coli O157:H7 is frequently reported with contaminating produce, it was not confirmed in this study. Other non-O157 serogroups (i.e., O111, O26, O117, O121, and O145) can also produce Shiga toxins, carry virulence genes, and have been previously detected in surface water (Nadya et al., 2016). A recent study from the CDC found that consuming raw produce like lettuce and tomatoes is the leading cause of non-O157 outbreaks (Marder et al., 2023). In the past, E. coli O26:H11 has caused outbreaks and hemolytic-uremic syndrome (HUS; Alharbi et al., 2022). The virulence genes for STEC (eaeA and stx) have been primarily associated with patients with severe life-threatening complications with HUS (Eklund et al., 2002; Werber et al., 2008). STEC, when compared to other foodborne pathogens, has a lower infectious dose that results in high morbidity and mortality (Saxena et al., 2015). In the present study, 19/88 (21.59%) of collected samples were PCR-screened positive for the virulence genes. Similar results were reported by Belias et al. (2021), who reported a 21% (36/169) prevalence from canal water. Research conducted in agricultural water from New York, Arizona, and California identified different percentages of codetection, respectively, 2.7% (16/588), 48% (44/83), 88% (77/88), 57% (188/330) (Strawn et al., 2013a; Cooley et al., 2014; Weller et al., 2020b). It is relevant to emphasize that the use of the simultaneous detection of those genes without a culture or serotyping in a single sample may lead to an overestimation of STEC prevalence, as it has been suggested that their presence might indicate the presence of either a single organism possessing both genes or multiple organisms, each with one of the genes (Weller et al., 2020b; Belias et al., 2021). Thus, careful consideration of the limitations associated with gene detection methods is crucial in accurately assessing the prevalence of STEC, highlighting the need for complementary techniques such as culture or serotyping in future studies to distinguish between single organisms with both genes and multiple organisms.
Based on the logistic regression model, the odds of codetection of virulence genes of STEC were negatively associated with calcium and humidity (p-value >0.05). The best model failed to identify any substantial correlations with either nitrate or solar radiation (p-value >0.05). Findings in our study suggest that for each ppm increase in calcium, the odds of codetection decreased significantly (p < 0.05). The odds ratio (OR) of 0.27 indicates that higher calcium levels reduce the odds of codetection by 73%. The average calcium levels were 13.08 ppm with a median of 12.70 and a maximum of 41 ppm. Literature often associates the levels of calcium with oxidative stress in bacteria, the increase in calcium also can impact the lipid bilayer of E. coli (Dominguez, 2004) and biofilm formation (Bilecen and Yildiz, 2009; Hu et al., 2022). No documented literature was found on the codetection of virulent factors and their relationship with calcium concentrations in water used for irrigation.
There was a negative correlation between relative humidity and STEC virulence factors. The median relative humidity during the study was 79.08%, with a minimum of 63.25% and a maximum of 89.40%. Higher humidity levels were associated with a significant decrease in the odds of stx-aeaA codetection by a factor of 0.31. Limited information is available on the effect of relative humidity and pathogens in water sources. In a study conducted in Spain, authors did not find correlations between relative humidity and levels of E. coli in water sources (Weller et al., 2020a; Truchado et al., 2021), on the other hand, suggested that the average of relative humidity was classified as an important factor for Listeria monocytogenes isolation from water sources. In non-aquatic environments opposite findings have been reported suggesting that higher environmental moisture could enhance pathogen survival in soil, especially in subtropical and tropical environments (Zhang et al., 2010; Liu et al., 2013). While it is widely recognized that higher moisture levels generally promote pathogen survival, particularly in terrestrial environments, our findings suggest a more nuanced relationship within aquatic ecosystems. In aquatic environments, factors such as water flow, temperature fluctuations, and microbial interactions can significantly influence the survival and proliferation of pathogens. Therefore, while our results may seem contradictory to the general understanding of humidity’s effects on pathogen survival, they may reflect the complex interplay of various environmental factors unique to surface water ecosystems. This highlights the importance of considering the specific conditions of aquatic environments when interpreting microbial dynamics and virulence marker distribution.
Although solar radiation and nitrate were not statistically significant (p-value >0.05), biological significance can be inferred as our findings suggest that solar radiation was negatively associated with the codetection of virulent genes. The mean and median for solar radiation from this data set were 17.32 MJ/m2 and 16.97 MJ/m2, respectively. With each unit (MJ/m2) increase in solar radiation, the odds of codetection were reduced by approximately 60%. Previous studies have reported that solar radiation inactivates E. coli in water (Whitman et al., 2004; Kim et al., 2023). A study in Northeast Georgia reported similar results for generic E. coli but established that STEC (O157:H7) strains were resistant to solar radiation (Jenkins et al., 2011). Studies in New York and New Zealand report negative correlations between E. coli and solar radiation (Weller et al., 2020a; Bunyaga et al., 2023). Weller et al. (2020a, b) reported that in Arizona, the likelihood of codetection of eaeA-stx increased as solar radiation increased. Previous findings suggest that UV rays can impact E. coli as a function of water turbidity given by suspended particles, as higher concentrations of suspended particles reduce the UV rays’ penetration and impact on pathogens (Tousi et al., 2021). Further research must be conducted to determine biological interactions between solar radiation and foodborne pathogens in aquatic environments. Nitrate, on the other hand, was considered the second most important variable in our conditional forest analysis, and similarly to other nutrients like phosphorous, the literature suggests that this nutrient has biological significance in the presence of foodborne pathogens in water sources (Gu et al., 2013). Although not considered to be statistically significant according to our logistic regression analysis, it might represent biological significance as for each unit increase in nitrate, the odds of codetection increased by a factor of 3.37. In our study, the mean concentration for nitrates was 2.2 ppm, with a maximum of 19.1 ppm. Phosphorus and nitrogen are crucial nutrients for eutrophication processes, (US EPA, 2013). A cyanobacteria-like organism was occasionally observed in some of the ponds, which may have supported the growth of STEC and Salmonella, as they can form biofilms on aquatic plants or attach to suspended solids in aquatic ecosystems (Chekabab et al., 2013; Cho et al., 2022). Additional abiotic factors like physicochemical parameters are well known to impact waterborne bacteria, including water pH (Rodrigues et al., 2020), turbidity, water temperature, and conductivity have been previously associated with the presence of pathogens like L. monocytogenes and Salmonella (Chung et al., 2020); however, the outcomes from this study did not find a significant correlation between STEC virulence factors and other abiotic factors.
Conclusion
This study provides insight into the role of abiotic indicators in detecting microbial contamination in agricultural water sources located in the Southeast United States. Furthermore, significant associations between calcium and humidity are key factors for the codetection of virulence markers for STEC. This study reinforces the poor correlation between generic E. coli and foodborne pathogens. Further studies must be conducted to determine the serogroups of STEC present in surface water used for irrigation. Our study also suggests that although all the markers for E. coli O157:H7 were not detected simultaneously in a single sample, markers were detected throughout the study which might indicate the potential presence of other dangerous E. coli (enteropathogenic, enterohemorrhagic, STEC). The presence of Salmonella and STEC in agricultural water poses a public health concern as several outbreaks have been associated with the serovars detected at the evaluated farms.
Data availability statement
The raw data supporting the conclusions of this article will be made available by the authors, without undue reservation.
Author contributions
ZC: Data curation, Formal analysis, Methodology, Validation, Writing – original draft. LD: Conceptualization, Methodology, Visualization, Writing – review & editing. AS: Conceptualization, Data curation, Visualization, Writing – review & editing. CR: Conceptualization, Funding acquisition, Investigation, Methodology, Project administration, Resources, Supervision, Visualization, Writing – review & editing.
Funding
The author(s) declare financial support was received for the research, authorship, and/or publication of this article. This research was funded by the Alabama Agricultural Experiment Station at Auburn University.
Acknowledgments
The authors would like to thank Joara S. Candian, Fabricio Landim, and Michael Phillips for their assistance with collecting samples for the first portion of the study. The authors are also grateful to Stuart Price and Steven Kitchens for their assistance and guidance with laboratory work. Lastly, thanks to Nathan Barlett and Jessica Hofstetter for assisting with programming in the software “R.”
Conflict of interest
The authors declare that the research was conducted in the absence of any commercial or financial relationships that could be construed as a potential conflict of interest.
Publisher’s note
All claims expressed in this article are solely those of the authors and do not necessarily represent those of their affiliated organizations, or those of the publisher, the editors and the reviewers. Any product that may be evaluated in this article, or claim that may be made by its manufacturer, is not guaranteed or endorsed by the publisher.
Supplementary material
The Supplementary material for this article can be found online at: https://www.frontiersin.org/articles/10.3389/fmicb.2024.1320168/full#supplementary-material
Footnotes
References
Ackers, M.-L., Mahon, B. E., Leahy, E., Goode, B., Damrow, T., Hayes, P. S., et al. (1998). An outbreak of Escherichia coli O157:H7 infections associated with leaf lettuce consumption. J. Infect. Dis. 177, 1588–1593. doi: 10.1086/515323
Alharbi, M. G., Al-Hindi, R. R., Esmael, A., Alotibi, I. A., Azhari, S. A., Alseghayer, M. S., et al. (2022). The “big six”: hidden emerging foodborne bacterial pathogens. Trop. Med. Infect. Dis. 7:356. doi: 10.3390/tropicalmed7110356
Andrews, W., Wang, H., Jacobson, A., Ge, B., Zhang, G., and Hammack, T. (2023). BAM Chapter 5: Salmonella : FDA. https://www.fda.gov/food/laboratory-methods-food/bam-chapter-5-salmonella
Antaki, E. M., Vellidis, G., Harris, C., Aminabadi, P., Levy, K., and Jay-Russell, M. T. (2016). Low concentration of Salmonella enterica and generic Escherichia coli in farm ponds and irrigation distribution systems used for mixed produce production in southern Georgia. Foodborne Pathog. Dis. 13, 551–558. doi: 10.1089/fpd.2016.2117
Balali, G. I., Yar, D. D., Afua Dela, V. G., and Adjei-Kusi, P. (2020). Microbial contamination, an increasing threat to the consumption of fresh fruits and vegetables in Today’s world. Int. J. Microbiol. 2020:e3029295, 1–13. doi: 10.1155/2020/3029295
Bardsley, C. A., Weller, D. L., Ingram, D. T., Chen, Y., Oryang, D., Rideout, S. L., et al. (2021). Strain, soil-type, irrigation regimen, and poultry litter influence Salmonella survival and die-off in agricultural soils. Front. Microbiol. 12:12. doi: 10.3389/fmicb.2021.590303
Belias, A., Brassill, N., Roof, S., Rock, C., Wiedmann, M., and Weller, D. (2021). Cross-validation indicates predictive models May provide an alternative to Indicator organism monitoring for evaluating pathogen presence in southwestern US agricultural water. Front. Water 3:3. doi: 10.3389/frwa.2021.693631
Benjamin, L., Atwill, E. R., Jay-Russell, M., Cooley, M., Carychao, D., Gorski, L., et al. (2013). Occurrence of generic Escherichia coli, E. coli O157 and Salmonella spp. in water and sediment from leafy green produce farms and streams on the Central California coast. Int. J. Food Microbiol. 165, 65–76. doi: 10.1016/j.ijfoodmicro.2013.04.003
Bilecen, K., and Yildiz, F. H. (2009). Identification of a calcium-controlled negative regulatory system affecting Vibrio cholerae biofilm formation. Environ. Microbiol. 11, 2015–2029. doi: 10.1111/j.1462-2920.2009.01923.x
Bunyaga, A., Corner-Thomas, R., Draganova, I., Kenyon, P., and Burkitt, L. (2023). The behaviour of sheep around a natural waterway and impact on water quality during winter in New Zealand. Anim. Open Access J. MDPI 13:1461. doi: 10.3390/ani13091461
CDC (2019a). E. coli outbreak from fresh spinach | CDC foodborne and diarrheal diseases branch. Cent. Dis. Control Prev.,
CDC (2019b). Multistate outbreak of E. coli O157:H7 infections linked to Romaine lettuce (final update) | investigation notice: Multistate outbreak of E. coli O157:H7 infections April 2018 | E. coli | CDC [WWW document]. Available at: https://www.cdc.gov/ecoli/2018/o157h7-04-18/index.html (Accessed 27 February, 23).
CDC (2020). Outbreak of Salmonella Newport infections linked to onions | CDC [WWW document]. Cent. Dis. Control Prev.
Chekabab, S. M., Paquin-Veillette, J., Dozois, C. M., and Harel, J. (2013). The ecological habitat and transmission of Escherichia coli O157:H7. FEMS Microbiol. Lett. 341, 1–12. doi: 10.1111/1574-6968.12078
Cho, K. H., Wolny, J., Kase, J. A., Unno, T., and Pachepsky, Y. (2022). Interactions of E. coli with algae and aquatic vegetation in natural waters. Water Res. 209:117952. doi: 10.1016/j.watres.2021.117952
Chung, T., Weller, D. L., and Kovac, J. (2020). The composition of microbial communities in six streams, and its association with environmental conditions, and foodborne pathogen isolation. Front. Microbiol. 11:1757. doi: 10.3389/fmicb.2020.01757
Cooley, M. B., Quiñones, B., Oryang, D., Mandrell, R. E., and Gorski, L. (2014). Prevalence of Shiga toxin producing Escherichia coli, Salmonella enterica, and Listeria monocytogenes at public access watershed sites in a California central coast agricultural region. Front. Cell. Infect. Microbiol. 4:30. doi: 10.3389/fcimb.2014.00030
de Rocha, A. D., Ferrari, R. G., Pereira, W. E., de Lima, L. A., Givisiez, P. E. N., Moreno-Switt, A. I., et al. (2022). Revisiting the biological behavior of Salmonella enterica in hydric resources: a Meta-analysis study addressing the critical role of environmental water on food safety and public health. Front. Microbiol. 13:802625. doi: 10.3389/fmicb.2022.802625
Deaven, A. M., Ferreira, C. M., Reed, E. A., Chen See, J. R., Lee, N. A., Almaraz, E., et al. (2021). Salmonella genomics and population analyses reveal high inter-and Intraserovar diversity in freshwater. Appl. Environ. Microbiol. 87, e02594–e02520. doi: 10.1128/AEM.02594-20
Devarajan, N., Weller, D. L., Jones, M., Adell, A. D., Adhikari, A., Allende, A., et al. (2023). Evidence for the efficacy of pre-harvest agricultural practices in mitigating food-safety risks to fresh produce in North America. Front. Sustain. Food Syst. 7:1435. doi: 10.3389/fsufs.2023.1101435
Dominguez, D. C. (2004). Calcium signalling in bacteria. Mol. Microbiol. 54, 291–297. doi: 10.1111/j.1365-2958.2004.04276.x
Eklund, M., Leino, K., and Siitonen, A. (2002). Clinical Escherichia coli strains carrying stx genes: stx variants and stx-positive virulence profiles. J. Clin. Microbiol. 40, 4585–4593. doi: 10.1128/JCM.40.12.4585-4593.2002
Falardeau, J., Johnson, R. P., Pagotto, F., and Wang, S. (2017). Occurrence, characterization, and potential predictors of verotoxigenic Escherichia coli, listeria monocytogenes, and Salmonella in surface water used for produce irrigation in the lower mainland of British Columbia Canada. PLOS ONE 12:e0185437. doi: 10.1371/journal.pone.0185437
Feng, P., Weagant, S. D., and Jinnneman, K. (2021). BAM chapter 4A: Diarrheagenic Escherichia coli : FDA.
Franz, E., Klerks, M. M., De Vos, O. J., Termorshuizen, A. J., and van Bruggen, A. H. C. (2007). Prevalence of Shiga toxin-producing Escherichia coli stx1, stx2, eaeA, and rfbE genes and survival of E. coli O157:H7 in manure from organic and low-input conventional dairy farms. Appl. Environ. Microbiol. 73, 2180–2190. doi: 10.1128/AEM.01950-06
Greene, S. K., Daly, E. R., Talbot, E. A., Demma, L. J., Holzbauer, S., Patel, N. J., et al. (2008). Recurrent multistate outbreak of Salmonella Newport associated with tomatoes from contaminated fields, 2005. Epidemiol. Infect. 136, 157–165. doi: 10.1017/S095026880700859X
Gu, G., Luo, Z., Cevallos-Cevallos, J. M., Adams, P., Vellidis, G., Wright, A., et al. (2013). Factors affecting the occurrence of Escherichia coli O157 contamination in irrigation ponds on produce farms in the Suwannee River watershed. Can. J. Microbiol. 59, 175–182. doi: 10.1139/cjm-2012-0599
Gu, G., Strawn, L. K., Zheng, J., Reed, E. A., and Rideout, S. L. (2019). Diversity and dynamics of Salmonella enterica in water sources, poultry litters, and field soils amended with poultry litter in a major agricultural area of Virginia. Front. Microbiol. 10:868. doi: 10.3389/fmicb.2019.02868
Gurtler, J. B., and Gibson, K. E. (2022). Irrigation water and contamination of fresh produce with bacterial foodborne pathogens. Curr. Opin. Food Sci. 47:100889. doi: 10.1016/j.cofs.2022.100889
Haley, O. C., Zhao, Y., Maher, J. M., Gragg, S. E., Trinetta, V., Bhullar, M., et al. (2022). Comparative assessment of the microbial quality of agricultural water on Kansas and Missouri fresh produce farms. Food Prot. Trends 42, 186–193. doi: 10.4315/FPT-21-033
Harris, C. S., Tertuliano, M., Rajeev, S., Vellidis, G., and Levy, K. (2018). Impact of storm runoff on Salmonella and Escherichia coli prevalence in irrigation ponds of fresh produce farms in southern Georgia. J. Appl. Microbiol. 124, 910–921. doi: 10.1111/jam.13689
Haymaker, J., Sharma, M., Parveen, S., Hashem, F., May, E. B., Handy, E. T., et al. (2019). Prevalence of Shiga-toxigenic and atypical enteropathogenic Escherichia coli in untreated surface water and reclaimed water in the mid-Atlantic U.S. Environ. Res. 172, 630–636. doi: 10.1016/j.envres.2019.02.019
Hothorn, T., Hornik, K., Strobl, C., and Zeileis, A. (2023). Party: a Laboratory for Recursive Partytioning.
Hu, J., Lv, X., Niu, X., Yu, F., Zuo, J., Bao, Y., et al. (2022). Effect of nutritional and environmental conditions on biofilm formation of avian pathogenic Escherichia coli. J. Appl. Microbiol. 132, 4236–4251. doi: 10.1111/jam.15543
Hu, Y., Zhang, Q., and Meitzler, J. C. (1999). Rapid and sensitive detection of Escherichia coli O157:H7 in bovine faeces by a multiplex PCR. J. Appl. Microbiol. 87, 867–876. doi: 10.1046/j.1365-2672.1999.00938.x
Iguchi, A., von Mentzer, A., Kikuchi, T., and Thomson, N. R. (2017). An untypeable enterotoxigenic Escherichia coli represents one of the dominant types causing human disease. Microb. Genomics 3:e000121. doi: 10.1099/mgen.0.000121
Jay, M. T., Cooley, M., Carychao, D., Wiscomb, G. W., Sweitzer, R. A., Crawford-Miksza, L., et al. (2007). Escherichia coli O157:H7 in feral swine near spinach fields and cattle, Central California coast. Emerg. Infect. Dis. 13, 1908–1911. doi: 10.3201/eid1312.070763
Jenkins, M. B., Endale, D. M., Fisher, D. S., Paige Adams, M., Lowrance, R., Larry Newton, G., et al. (2012). Survival dynamics of fecal bacteria in ponds in agricultural watersheds of the Piedmont and coastal plain of Georgia. Water Res. 46, 176–186. doi: 10.1016/j.watres.2011.10.049
Jenkins, M. B., Fisher, D. S., Endale, D. M., and Adams, P. (2011). Comparative die-off of Escherichia coli 0157:H7 and fecal Indicator Bacteria in pond water. Environ. Sci. Technol. 45, 1853–1858. doi: 10.1021/es1032019
Kim, S., Pachepsky, Y., Micallef, S. A., Rosenberg Goldstein, R., Sapkota, A. R., Hashem, F., et al. (2023). Temporal stability of Salmonella enterica and Listeria monocytogenes in surface waters used for irrigation in the mid-Atlantic United States. J. Food Prot. 86:100058. doi: 10.1016/j.jfp.2023.100058
Laughlin, M., Bottichio, L., Weiss, J., Higa, J., McDonald, E., Sowadsky, R., et al. (2019). Multistate outbreak of Salmonella Poona infections associated with imported cucumbers, 2015–2016. Epidemiol. Infect. 147:e270. doi: 10.1017/S0950268819001596
Li, B., Vellidis, G., Liu, H., Jay-Russell, M., Zhao, S., Hu, Z., et al. (2014). Diversity and antimicrobial resistance of Salmonella enterica isolates from surface water in southeastern United States. Appl. Environ. Microbiol. 80, 6355–6365. doi: 10.1128/AEM.02063-14
Liu, C., Hofstra, N., and Franz, E. (2013). Impacts of climate change on the microbial safety of pre-harvest leafy green vegetables as indicated by Escherichia coli O157 and Salmonella spp. Int. J. Food Microbiol. 163, 119–128. doi: 10.1016/j.ijfoodmicro.2013.02.026
Liu, H., Whitehouse, C. A., and Li, B. (2018). Presence and persistence of Salmonella in water: the impact on microbial quality of water and food safety. Front. Public Health 6:159. doi: 10.3389/fpubh.2018.00159
Luo, Z., Gu, G., Ginn, A., Giurcanu, M. C., Adams, P., Vellidis, G., et al. (2015). Distribution and characterization of Salmonella enterica isolates from irrigation ponds in the southeastern United States. Appl. Environ. Microbiol. 81, 4376–4387. doi: 10.1128/AEM.04086-14
Machado-Moreira, B., Richards, K., Brennan, F., Abram, F., and Burgess, C. M. (2019). Microbial contamination of fresh produce: what, where, and how? Compr. Rev. Food Sci. Food Saf. 18, 1727–1750. doi: 10.1111/1541-4337.12487
Manuel, C. S. (2010). Molecular ecology of foodborne pathogens in primary production agriculture environments in northern Colorado and investigations in internalin a diversity in Listeria monocytogenes DUP-1039C strains (text) : Colorado State University. https://mountainscholar.org/handle/10217/70812
Marder, E. P., Cui, Z., Bruce, B. B., Richardson, L. C., Boyle, M. M., Cieslak, P. R., et al. (2023). Risk factors for non-O157 Shiga toxin-producing Escherichia coli infections, United States. Emerg. Infect. Dis. J. 29, 1183–1190. doi: 10.3201/eid2906.221521
Maurer, J. J., Martin, G., Hernandez, S., Cheng, Y., Gerner-Smidt, P., Hise, K. B., et al. (2015). Diversity and persistence of Salmonella enterica strains in rural landscapes in the southeastern United States. PLoS One 10:e0128937. doi: 10.1371/journal.pone.0128937
McEgan, R., Mootian, G., Goodridge, L. D., Schaffner, D. W., and Danyluk, M. D. (2013). Predicting Salmonella populations from biological, chemical, and physical indicators in Florida surface waters. Appl. Environ. Microbiol. 79, 4094–4105. doi: 10.1128/AEM.00777-13
Munns, K. D., Selinger, L. B., Stanford, K., Guan, L., Callaway, T. R., and McAllister, T. A. (2015). Perspectives on super-shedding of Escherichia coli O157:H7 by cattle. Foodborne Pathog. Dis. 12, 89–103. doi: 10.1089/fpd.2014.1829
Murphy, C. M., Strawn, L. K., Chapin, T. K., McEgan, R., Gopidi, S., Friedrich, L., et al. (2022). Factors associated with E. coli levels in and Salmonella contamination of agricultural water differed between north and South Florida waterways. Front. Water 3:673. doi: 10.3389/frwa.2021.750673
Nadya, S., Delaquis, P., Chen, J., Allen, K., Johnson, R. P., Ziebell, K., et al. (2016). Phenotypic and genotypic characteristics of Shiga toxin-producing Escherichia coli isolated from surface waters and sediments in a Canadian urban-agricultural landscape. Front. Cell. Infect. Microbiol. 6:36. doi: 10.3389/fcimb.2016.00036
Painter, J. A. (2019). Estimated use of water in Georgia for 2015 and water-use trends, 1985–2015 (USGS Numbered Series No. 2019–1086), Estimated use of water in Georgia for 2015 and water-use trends, 1985–2015, Open-File Report. Reston, VA: U.S. Geological Survey.
Quintanilla Portillo, J., Cheng, X., Belias, A. M., Weller, D. L., Wiedmann, M., and Stasiewicz, M. J. (2022). A validated Preharvest sampling simulation shows that sampling plans with a larger number of randomly located samples perform better than typical sampling plans in detecting representative point-source and widespread hazards in leafy Green fields. Appl. Environ. Microbiol. 88, e01015–e01022. doi: 10.1128/aem.01015-22
Rahn, K., De Grandis, S. A., Clarke, R. C., McEwen, S. A., Galán, J. E., Ginocchio, C., et al. (1992). Amplification of an invA gene sequence of Salmonella typhimurium by polymerase chain reaction as a specific method of detection of Salmonella. Mol. Cell. Probes 6, 271–279. doi: 10.1016/0890-8508(92)90002-F
Rajabi, M., Jones, M., Hubbard, M., Rodrick, G., and Wright, A. C. (2011). Distribution and genetic diversity of Salmonella enterica in the upper Suwannee River. Int. J. Microbiol. 2011, 1–9. doi: 10.1155/2011/461321
Rodrigues, C., da Silva, A. L. B. R., and Dunn, L. L. (2020). Factors impacting the prevalence of foodborne pathogens in agricultural water sources in the southeastern United States. Water 12:51. doi: 10.3390/w12010051
Saxena, T., Kaushik, P., and Krishna Mohan, M. (2015). Prevalence of E. coli O157:H7 in water sources: an overview on associated diseases, outbreaks and detection methods. Diagn. Microbiol. Infect. Dis. 82, 249–264. doi: 10.1016/j.diagmicrobio.2015.03.015
Sharma, M., Handy, E. T., East, C. L., Kim, S., Jiang, C., Callahan, M. T., et al. (2020). Prevalence of Salmonella and Listeria monocytogenes in non-traditional irrigation waters in the mid-Atlantic United States is affected by water type, season, and recovery method. PLoS One 15:e0229365. doi: 10.1371/journal.pone.0229365
Sipos, R., Székely, A., Révész, S., and Márialigeti, K. (2010). “Addressing PCR biases in environmental microbiology studies” in Bioremediation: Methods and protocols, methods in molecular biology. ed. S. P. Cummings (Totowa, NJ: Humana Press).
Stocker, M. D., Smith, J. E., Hill, R. L., and Pachepsky, Y. A. (2022). Intra-daily variation of Escherichia coli concentrations in agricultural irrigation ponds. J. Environ. Qual. 51, 719–730. doi: 10.1002/jeq2.20352
Strawn, L. K., Danyluk, M. D., Worobo, R. W., and Wiedmann, M. (2014). Distributions of Salmonella subtypes differ between two U.S. Produce-Grow. Reg. Appl. Environ. Microbiol. 80, 3982–3991. doi: 10.1128/AEM.00348-14
Strawn, L. K., Fortes, E. D., Bihn, E. A., Nightingale, K. K., Gröhn, Y. T., Worobo, R. W., et al. (2013a). Landscape and meteorological factors affecting prevalence of three food-borne pathogens in fruit and vegetable farms. Appl. Environ. Microbiol. 79, 588–600. doi: 10.1128/AEM.02491-12
Strawn, L. K., Gröhn, Y. T., Warchocki, S., Worobo, R. W., Bihn, E. A., and Wiedmann, M. (2013b). Risk factors associated with Salmonella and Listeria monocytogenes contamination of produce fields. Appl. Environ. Microbiol. 79, 7618–7627. doi: 10.1128/AEM.02831-13
Strobl, C., Boulesteix, A.-L., Kneib, T., Augustin, T., and Zeileis, A. (2008). Conditional variable importance for random forests. BMC Bioinform. 9:307. doi: 10.1186/1471-2105-9-307
Topalcengiz, Z., Strawn, L. K., and Danyluk, M. D. (2017). Microbial quality of agricultural water in Central Florida. PLoS One 12:e0174889. doi: 10.1371/journal.pone.0174889
Tousi, E. G., Duan, J. G., Gundy, P. M., Bright, K. R., and Gerba, C. P. (2021). Evaluation of E. coli in sediment for assessing irrigation water quality using machine learning. Sci. Total Environ. 799:149286. doi: 10.1016/j.scitotenv.2021.149286
Truchado, P., Gil, M. I., López, C., Garre, A., López-Aragón, R. F., Böhme, K., et al. (2021). New standards at European Union level on water reuse for agricultural irrigation: are the Spanish wastewater treatment plants ready to produce and distribute reclaimed water within the minimum quality requirements? Int. J. Food Microbiol. 356:109352. doi: 10.1016/j.ijfoodmicro.2021.109352
Truitt, L. N., Vazquez, K. M., Pfuntner, R. C., Rideout, S. L., Havelaar, A. H., and Strawn, L. K. (2018). Microbial quality of agricultural water used in produce Preharvest production on the eastern shore of Virginia. J. Food Prot. 81, 1661–1672. doi: 10.4315/0362-028X.JFP-18-185
US EPA (2013). Indicators: Nitrogen [WWW document]. Available at: https://www.epa.gov/national-aquatic-resource-surveys/indicators-nitrogen (Accessed 28 November, 2023).
US EPA (2014). Water quality standards handbook [WWW document]. Available at: https://www.epa.gov/wqs-tech/water-quality-standards-handbook (Accessed 6 September, 2023).
Vereen, E., Lowrance, R. R., Jenkins, M. B., Adams, P., Rajeev, S., and Lipp, E. K. (2013). Landscape and seasonal factors influence Salmonella and Campylobacter prevalence in a rural mixed use watershed. Water Res. 47, 6075–6085. doi: 10.1016/j.watres.2013.07.028
Wadamori, Y., Gooneratne, R., and Hussain, M. A. (2017). Outbreaks and factors influencing microbiological contamination of fresh produce. J. Sci. Food Agric. 97, 1396–1403. doi: 10.1002/jsfa.8125
Wei, T., Simko, V., Levy, M., Xie, Y., Jin, Y., Zemla, J., et al. (2021). Corrplot: Visualization of a correlation matrix.
Weller, D., Belias, A., Green, H., Roof, S., and Wiedmann, M. (2020a). Landscape, water quality, and weather factors associated with an increased likelihood of foodborne pathogen contamination of New York streams used to source water for produce production. Front. Sustain. Food Syst. 3:124. doi: 10.3389/fsufs.2019.00124
Weller, D., Brassill, N., Rock, C., Ivanek, R., Mudrak, E., Roof, S., et al. (2020b). Complex interactions between weather, and microbial and physicochemical water quality impact the likelihood of detecting foodborne pathogens in agricultural water. Front. Microbiol. 11:134. doi: 10.3389/fmicb.2020.00134
Werber, D., Beutin, L., Pichner, R., Stark, K., and Fruth, A. (2008). Shiga toxin–producing Escherichia coli serogroups in food and patients, Germany. Emerg. Infect. Dis. 14, 1803–1806. doi: 10.3201/eid1411.080361
Whitman, R. L., Nevers, M. B., Korinek, G. C., and Byappanahalli, M. N. (2004). Solar and temporal effects on Escherichia coli concentration at a Lake Michigan Swimming Beach. Appl. Environ. Microbiol. 70, 4276–4285. doi: 10.1128/AEM.70.7.4276-4285.2004
Wyborn, N. R., Clark, A., Roberts, R. E., Jamieson, S. J., Tzokov, S., Bullough, P. A., et al. (2004). Properties of haemolysin E (HlyE) from a pathogenic Escherichia coli avian isolate and studies of HlyE export. Microbiology 150, 1495–1505. doi: 10.1099/mic.0.26877-0
Xu, X., Rothrock, M. J., Reeves, J., Kumar, G. D., and Mishra, A. (2022). Using E. coli population to predict foodborne pathogens in pastured poultry farms. Food Microbiol. 108:104092. doi: 10.1016/j.fm.2022.104092
Yanestria, S. M., Rahmaniar, R. P., Wibisono, F. J., and Effendi, M. H. (2019). Detection of invA gene of Salmonella from milkfish (Chanos chanos) at Sidoarjo wet fish market, Indonesia, using polymerase chain reaction technique. Vet. World 12, 170–175. doi: 10.14202/vetworld.2019.170-175
Keywords: agricultural water, produce safety, irrigation, Escherichia coli , Salmonella enterica
Citation: Chevez ZR, Dunn LL, da Silva ALBR and Rodrigues C (2024) Prevalence of STEC virulence markers and Salmonella as a function of abiotic factors in agricultural water in the southeastern United States. Front. Microbiol. 15:1320168. doi: 10.3389/fmicb.2024.1320168
Edited by:
Dario De Medici, National Institute of Health (ISS), ItalyReviewed by:
Jay Paul Graham, University of California, Berkeley, United StatesAnna Szczerba-Turek, University of Warmia and Mazury in Olsztyn, Poland
Nicola Holden, The James Hutton Institute, United Kingdom
Copyright © 2024 Chevez, Dunn, da Silva and Rodrigues. This is an open-access article distributed under the terms of the Creative Commons Attribution License (CC BY). The use, distribution or reproduction in other forums is permitted, provided the original author(s) and the copyright owner(s) are credited and that the original publication in this journal is cited, in accordance with accepted academic practice. No use, distribution or reproduction is permitted which does not comply with these terms.
*Correspondence: Camila Rodrigues, czr0079@auburn.edu