- 1Zhejiang Cheery Bio-Technology Co., Ltd., Jiaxing, China
- 2College of Advanced Materials Engineering, Jiaxing Nanhu University, Jiaxing, China
- 3Zhejiang Institute of Advanced Technology Chinese Academy of Sciences, Jiaxing, China
- 4Sinochem Linyi Crop Nutrition Co., Ltd., Linyi, China
Introduction: Proteases exhibit a wide range of applications, and among them, alkaline proteases have become a prominent area of research due to their stability in highly alkaline environments. To optimize the production yield and activity of alkaline proteases, researchers are continuously exploring different fermentation conditions and culture medium components.
Methods: In this paper, the fermentation conditions of the alkaline protease (EC 3.4.21.14) production by Bacillus subtilis BS-QR-052 were optimized, and the effect of different nutrition and fermentation conditions was investigated. Based on the single-variable experiments, the Plackett–Burman design was used to explore the significant factors, and then the optimized fermentation conditions, as well as the interaction between these factors, were evaluated by response surface methodology through the Box–Behnken design.
Results and discussion: The results showed that 1.03% corn syrup powder, 0.05% MgSO4, 8.02% inoculation volume, 1:1.22 vvm airflow rate, as well as 0.5% corn starch, 0.05% MnSO4, 180 rpm agitation speed, 36°C fermentation temperature, 8.0 initial pH and 96 h incubation time were predicted to be the optimal fermentation conditions. The alkaline protease enzyme activity was estimated to be approximately 1787.91 U/mL, whereas subsequent experimental validation confirmed it reached 1780.03 U/mL, while that of 500 L scale-up fermentation reached 1798.33 U/mL. This study optimized the fermentation conditions for alkaline protease production by B. subtilis through systematic experimental design and data analysis, and the activity of the alkaline protease increased to 300.72% of its original level. The established model for predicting alkaline protease activity was validated, achieving significantly higher levels of enzymatic activity. The findings provide valuable references for further enhancing the yield and activity of alkaline protease, thereby holding substantial practical significance and economic benefits for industrial applications.
1 Introduction
Proteases can hydrolyze proteins into peptides, short-chain peptides and amino acids, and are categorized into neutral proteases, acid proteases and alkaline proteases according to their optimum reaction pH values. Among these, alkaline protease is the most highly demanded enzyme preparation in the market, with applications spanning various production sectors, including food processing, pharmaceuticals, the detergent industry, textiles, leather processing, and feed additives (Ito et al., 1998). So far, There are dozens of commercially produced alkaline proteases available, with the main source of production being the alkaline proteases (EC 3.4.21.14) produced through the metabolic activity of Bacillus subtilis (Sharma et al., 2017).
B. subtilis, one kind of Gram-positive bacteria (Vanitha et al., 2014), distributes widely on earth, like air and water, as well as guts of human beings and animals. When its survival environment, such as the acidity, temperature, humidity and nutrient substance, etc., has dramatically changed, it will generate spore to tackle these changes. During the stable phase of fermentation, a significant amount of proteases is synthesized as a result of substrate induction (Singh et al., 2015). B. subtilis has been scientifically validated to meet the requirements for biological safety (Harwood et al., 2018), which allows it to be legally approved by the Chinese Ministry of Agriculture for use as a feed additive. As a member of the probiotic bacteria, B. subtilis is utilized for the production of a microbial ecological formulation (Morikawa, 2006; Hashem et al., 2019). This eco-friendly, naturally derived, and non-polluting formulation has exhibited significant effectiveness, leading to its extensive application across various industries, including manufacturing, agriculture, pharmaceuticals, healthcare, and aquaculture.
Although B. subtilis exhibits good enzymatic activity, the existing strains are unable to meet the increasing demands driven by societal development. Consequently, researchers commonly employ methods such as wild strain isolation and screening, mutagenesis breeding and genetic engineering to enhance alkaline protease activity, stability, and yield. Through targeted modification and screening of strains, they aim to obtain optimal strains. The evaluation of these strains typically relies on the alkaline protease content and enzyme activity achieved through microbial fermentation. For example, Rekik achieved an alkaline protease enzyme activity of 9,000 U/mL in B. subtilis through the optimization of the culture medium (Rekik et al., 2019). Similarly, other researchers have also optimized the fermentation conditions for different alkaline protease-producing strains, resulting in significant improvements in alkaline protease activity (Qureshi et al., 2011; Pant et al., 2015).
Optimization of variables is a crucial aspect of scientific exploration. Typically, various approaches are employed, including single-variable experiments, orthogonal experimental design (Box and Wilson, 1992), response surface methodology (RSM) (Khuri and Mukhopadhyay, 2010), and so on. Among these, RSM is a widely used method in experimental design and statistical modeling. It is primarily employed to study the influence of multiple factors on a specific response variable and to identify operating conditions that optimize the response variable. Characteristics of RSM include a comprehensive examination of the impacts of multiple factors, the description of the relationship between factors and the response variable through mathematical models, and the determination of optimal operating conditions through the design of a series of experiments. Furthermore, RSM emphasizes the interactions between factors and provides visualization tools, such as response surface plots, to intuitively display these relationships. Through statistical inference, the significance and reliability of the model can be assessed, thereby providing support for the ultimate optimization decisions.
Research has shown that alkaline proteases possess strong hydrolytic and alkali-resistant capabilities, as well as significant thermal stability (Mei and Jiang, 2005). Currently, most of these alkaline proteases used in the industry rely on imports, which greatly increases the production costs of enterprises. This study focuses on the alkaline protease production ability of B. subtilis BS-QR-052, which has been purified from the environment. The main objective of the research is to optimize the composition of the fermentation medium based on conventional and cost-effective production fermentation media. This involves fine-tuning the culture conditions to enhance the alkaline protease yield and enzyme activity in the RSM. Ultimately, the study aims to establish a solid theoretical foundation for the future industrial applications of this enzyme and provide solutions to the problem of high enzyme production costs in the industry.
2 Materials and methods
2.1 Microbial strain and culture medium
B. subtilis BS-QR-052, isolated from environmental samples in the Key Microbiological Laboratory of Zhejiang Cheery Co., Ltd., was identified as a member of the Bacillus genus through phylogenetic analysis based on the 16S rDNA (Woese and Fox, 1977) sequence and the CVTree3 algorithm (Qi et al., 2004). Before use, it was stored in a glycerol tube at −40°C following lyophilization. Three main types of culture media, namely solid slant medium (in g/100 mL): 1.0 peptone, 0.5 yeast extract, 1.5 NaCl, and 1.5 agar at pH 10.0, liquid seed medium (in g/100 mL): 1.0 beef paste, 1.5 glucose, and 0.5 NaCl at natural pH, and basal fermentation medium (in g/100 mL): 0.5 glucose, 1.0 peptone, 0.05 NaCl at pH 10.0, were utilized throughout the entire research process. As designed, each research step included three biological replicates. All chemical reagents and culture medium nutrients were purchased from Shanghai Aladdin Biochemical Technology Co., Ltd.
2.2 Cultivation conditions
Fifty milliliters of liquid seed medium was incubated overnight at 37°C and 200 rpm to obtain a primary seed culture in an Erlenmeyer flask in the incubator shaker (HZQ-F160A, Shanghai Bluepard Instruments Co., Ltd.). For the secondary seed culture, 5% of the primary seed culture was inoculated into a new Erlenmeyer flask containing a liquid seed medium, following the same cultivation method as the primary seed culture.
2.3 The viable count and enzyme activity assay of basal fermentation
A basal fermentation medium was used to prepare a 50 L fermentation broth (BLBIO-50-500-2000, Shanghai Bailun Biological Technology Co., Ltd., China), with the initial pH adjusted to 7.0. The inoculation was performed using a flame, with 5% of the secondary seed culture inoculated into the fermentation broth. The fermentation was carried out at a temperature of 40°C, an airflow rate of 1:1.2 vvm (volume of air per volume of medium), and an agitation speed of 150 rpm for 120 h. Samples were taken every 2 h to monitor the viable cell count and alkaline protease production in the fermentation broth. After reaching the stable phase of fermentation, a certain amount of fermentation broth was taken and centrifuged at 7155 × g for 10 min. The supernatant was collected to measure the alkaline protease activity.
The National Standard of PRC (GBT 23527-2009) was employed to measure the enzyme activity of alkaline proteases (Huang et al., 2020). Here, One unit of enzyme activity is defined as the amount of enzyme that liberates 1 μg tyrosine from casein as a substrate at 45°C at pH 10.0 per minute. The 1% (10 g/L) casein solution was used as the reaction substrate. A standard curve was constructed by preparing L-tyrosine standard solutions with concentrations of 0, 10, 20, 30, 40, 50, 60, 70, and 80 μg/mL. A blank solution without L-tyrosine was prepared in a separate tube. According to the proposal, Folin’s reagent was used to react with L-tyrosine under alkaline conditions to produce a blue compound, and the absorbance of this product was measured at a wavelength of 680 nm. The enzyme activity of all samples is calculated by the following formula:
in this equation: X = The enzyme activity of samples, (u/g). A = Enzyme activity of the final dilution compared to the standard curve, (u/mL). V = The volume of the volumetric flask used to dissolve the samples, (mL). 4 = The total volume of the reaction mixture is 4 mL. n = The dilution factor of enzyme liquid of alkaline proteases. m = The mass of the samples, (g). 10 = The reaction time is 10 min.
2.4 One variable at a time experiments: physicochemical determinants for alkaline protease production
Based on the single-variable experiment, the effect of carbon sources, nitrogen sources, and metal ions on the production of alkaline protease by B. subtilis was studied in a basal fermentation medium. All carbon and nitrogen sources were tested at 1% and 0.5%, respectively. The carbon sources included sucrose, lactose, maltose, glucose, corn starch, maltodextrin, and cornmeal. The nitrogen sources included soybean meal, peptone, urea, yeast extract, beef paste, soybean cake powder, corn steep powder, NH4Cl, and bran. Metal ions such as 0.05% CaCl2, MgSO4, MnSO4, CuSO4, FeCl3, and K2HPO4 were also added by turns. The influence of these three components on alkaline protease production was investigated while keeping other culture conditions constant. The fermentation process lasted for 96 h at a temperature of 38°C, with an inoculation volume of 5%, pH set at 7.0, agitation speed of 150 rpm, and an airflow rate of 1:1 vvm. Then, the supernatant was collected by centrifugation, and the enzyme activity was measured to determine the most suitable combination for alkaline protease production by B. subtilis.
A 10 L glass fermenter (JD-GAFM-10L, Beijing Jiade Precision Technology Co., Ltd., China) with 70% liquid volume was used for fermentation experiments employing a basal fermentation medium. The fermentation was conducted under the following conditions: a temperature of 38°C, an inoculation volume of 5%, an initial pH of 7.0, an agitation speed of 150 rpm, and an airflow rate of 1:1 vvm. Single-variable experiments were performed to investigate the effects of fermentation temperature (28, 32, 36, 40, 44, 48%), agitation speed (140, 150, 160, 170, 180, 190, 200, 220 rpm), inoculation volume (2, 4, 6, 8, 10, 12, 14%), initial pH (6.0, 6.5, 7.0, 7.5, 8.0), and airflow rate (1:0.6, 1:0.8, 1:1.0, 1:1.2, 1:1.4, 1:1.6 vvm) on the fermentation process. After 96 h of fermentation, the supernatant was collected by centrifugation, and the enzyme activity was measured.
2.5 Multiple variables at a time experiments: optimal combination of physicochemical factors for alkaline protease production
Plackett and Burman (1946) designs were employed for the rapid screening of significant factors influencing the experimental process, with each variable coded at two levels, −1 and +1. Then, the factors that remarkably influence fermentation were screened out for Box–Behnken design (Ferreira et al., 2007), with each factor tested in three gradients, −1, 0 and +1, to conduct the RSM (Khuri and Mukhopadhyay, 2010).
2.6 The scale-up test of alkaline protease fermentation
Using an optimized fermentation medium, a 500 L fermentation broth (BLBIO-50-500-2000, Shanghai Bailun Biological Technology Co., Ltd., China) was prepared. The fermentation process was carried out under optimized conditions for a cultivation period of 96 h. Samples were collected every 2 h to measure the viable cell count and alkaline protease enzyme activity in the fermentation broth.
2.7 The software used for statistical analysis
Microsoft 365 (Microsoft Corporation, United States) is employed for the compilation of all experimental data and tables. Origin Pro (OriginLab Corporation, United States) is utilized for generating trend charts and bar graphs. Design Expert 11(Stat-Ease Corporation, United States) is applied for Plackett–Burman design, Box–Behnken design, and RSM. All exported graphs are assembled and refined in Adobe Illustrator (version 26.0, Adobe Corporation, United States).
3 Results
3.1 The viable count and alkaline protease activity in the basal fermentation medium
In Figure 1, the viable cell count of B. subtilis increased slowly within the first 10 h, and no detectable alkaline protease activity was observed according to the standard curve of the L-tyrosine (y = 0.01x + 0.0035, R2 = 0.9998) in Supplementary Figure S1. At 26 h of cultivation, alkaline protease activity of 6 U/mL was detected, accompanied by an increase in the cell count of B. subtilis, indicating rapid proliferation of the bacterial cells entering the logarithmic growth phase. In the early stages of fermentation, the enzyme production rate was relatively slow. When the fermentation time reached 96 h, the alkaline protease activity reached its highest value of 598 U/mL. Subsequently, there was no significant change in enzyme production with increasing fermentation time. This may be due to the fact that in the late stages of fermentation, substrate depletion becomes severe, leading to a decline in cell viability and enzyme production capacity. Therefore, the fermentation time for B. subtilis to produce alkaline protease is determined as 96 h.
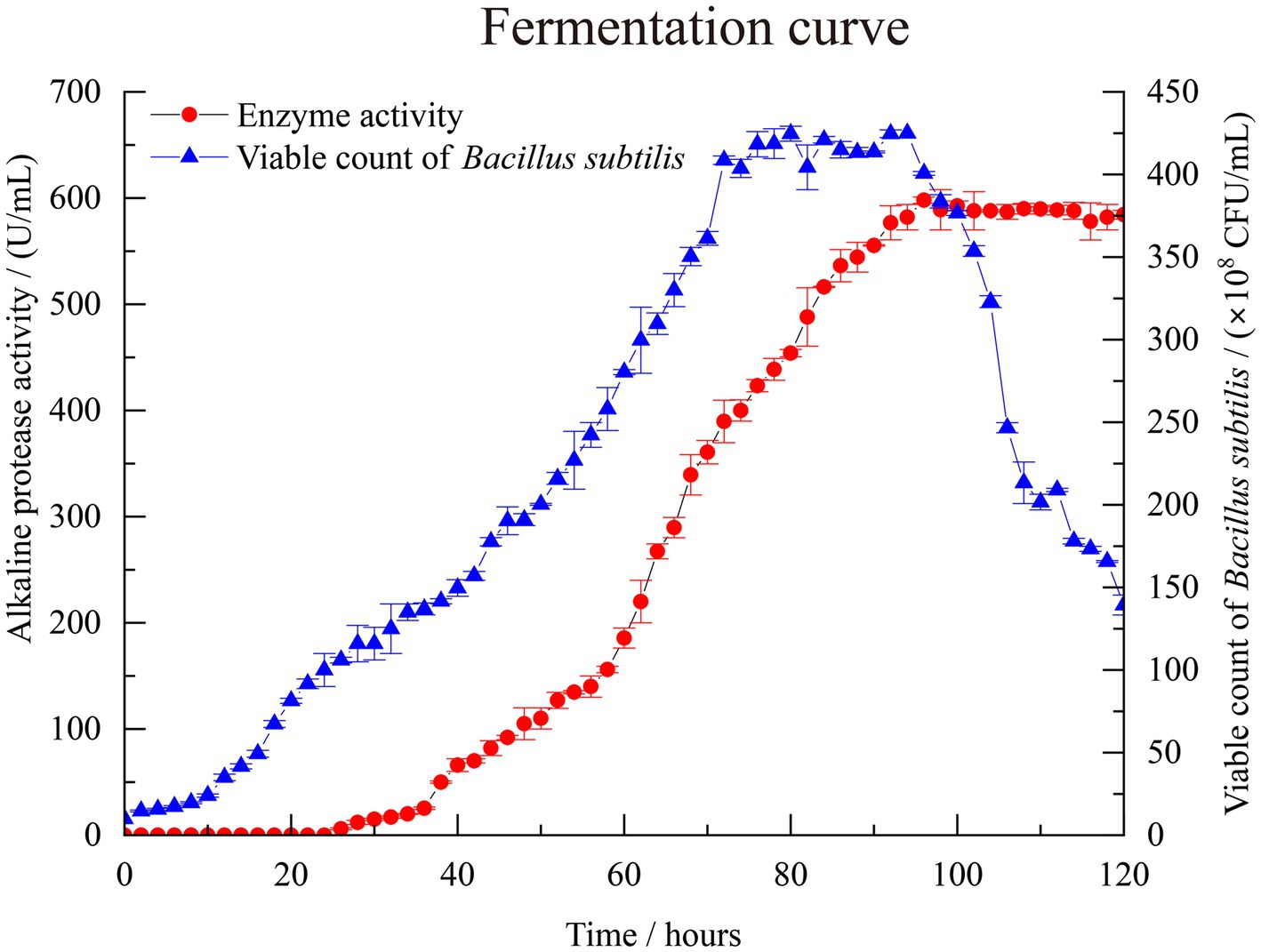
Figure 1. Bacillus subtilis BS-QR-052 growth and alkaline protease production in 50 L laboratory scale fermenter. Values are the mean of three replicas ǂ standard error or standard deviation.
3.2 The optimization of nutritional composition and fermentation conditions
Figure 2A illustrates that when using corn starch as the carbon source, B. subtilis exhibits the highest alkaline protease activity during the stationary phase, reaching 768 U/mL. This activity is significantly superior to that observed when using cornmeal (p-vlaue = 0.02) and maltodextrin (p-vlaue = 0.03) as alternative carbon sources. Similarly, when investigating different nitrogen sources (Figure 2B), the group supplemented with corn syrup powder demonstrated the highest alkaline protease activity, reaching 886 U/mL, which was significantly higher than the enzyme activity when soybean meal was used as a nitrogen source (p-value = 0.02). Following closely are soybean cake powder and bran, while urea and NH4Cl show the lowest activity. Furthermore, the influence of metal ions on alkaline protease production was studied. As depicted in Figure 2C, the groups treated with MgSO4 and MnSO4 display the highest alkaline protease production, reaching 625 U/mL and 620 U/mL, respectively. In contrast, the groups treated with K2HPO4 and CaCl2 show relatively lower protease production, with p-values of 0.058 and 0.002 against that of MgSO4, respectively. These results collectively indicate that corn starch and corn syrup powder are more suitable as carbon and nitrogen sources to enhance the alkaline protease production by B. subtilis. Additionally, the presence of Mg2+ and Mn2+ positively impacts alkaline protease proliferation and metabolism in B. subtilis.
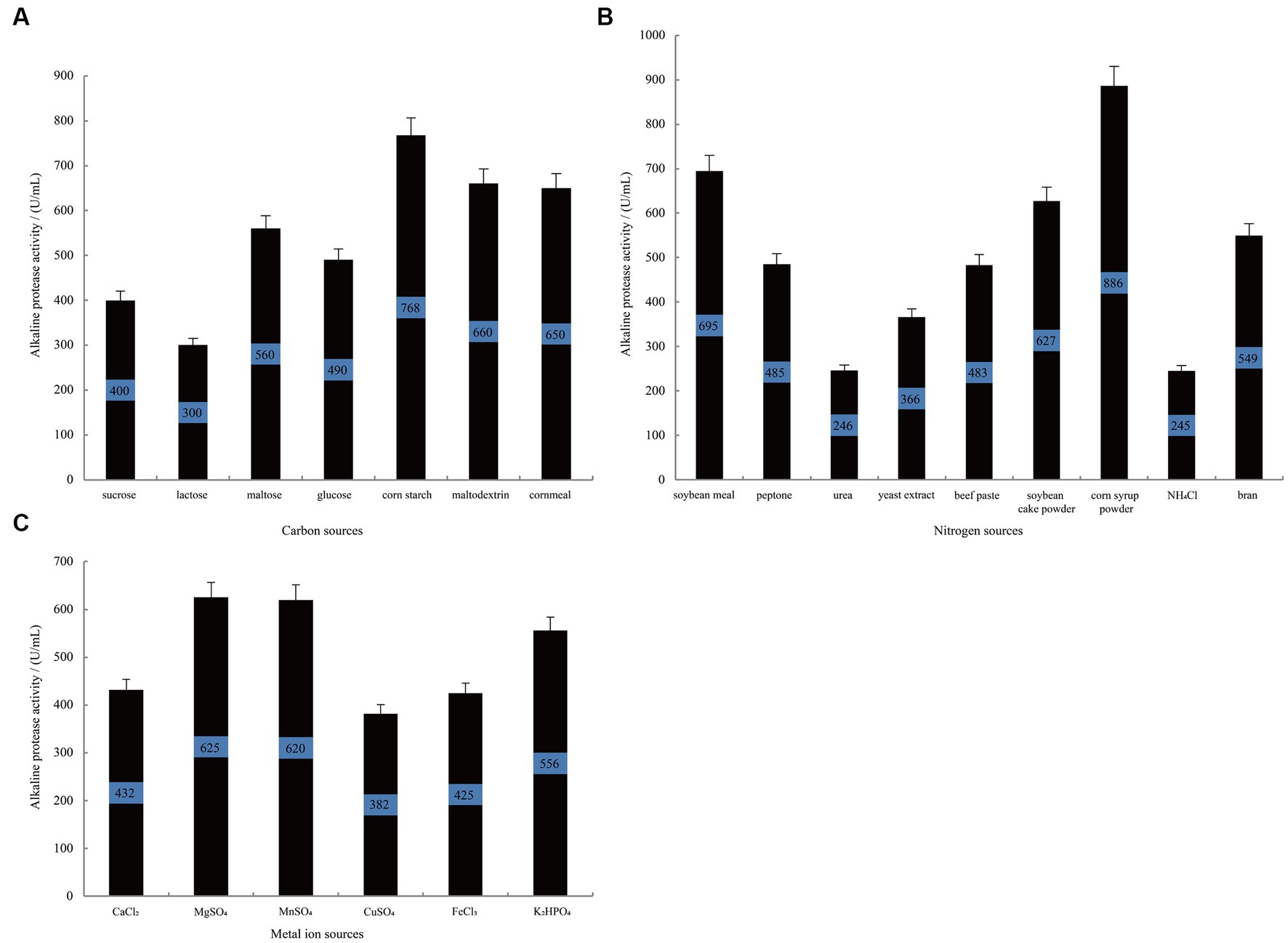
Figure 2. The single-variable experiments conducted to investigate the nutritional components. The effect of carbon source (A), nitrogen source (B), and metal ion (C) on the alkaline protease production by B. subtilis BS-QR-052. Values are the mean of three replicas ǂ standard error or standard deviation.
Single-variable experiments revealed that during the fermentation of B. subtilis at 36°C (Figure 3A), the alkaline protease activity reached a stable phase with a remarkable enzyme activity of 758 U/mL. This activity was significantly higher than that observed at 28°C and 32°C. However, an increase beyond 36°C resulted in a gradual decline in the alkaline protease activity. Among other investigated parameters, the highest alkaline protease activity of 600 U/mL was achieved at an agitation speed of 180 rpm (Figure 3B). Furthermore, when the inoculation volume was set at 8% (Figure 3C), the fermentation broth exhibited an optimal alkaline protease activity of 580 U/mL. Additionally, an initial pH of 8.0 (Figure 3D) yielded the maximum alkaline protease activity, measuring 640 U/mL. Moreover, the alkaline protease activity reached its peak value of 670 U/mL when the airflow rate was maintained at 1:1.2 vvm (Figure 3E). Consequently, the findings suggest that a fermentation temperature of 36°C, an inoculation volume of 8%, an initial pH of 8.0, an agitation speed of 180 rpm and an airflow rate of 1:1.2 vvm are considered optimal conditions for alkaline protease accumulation by B. subtilis.
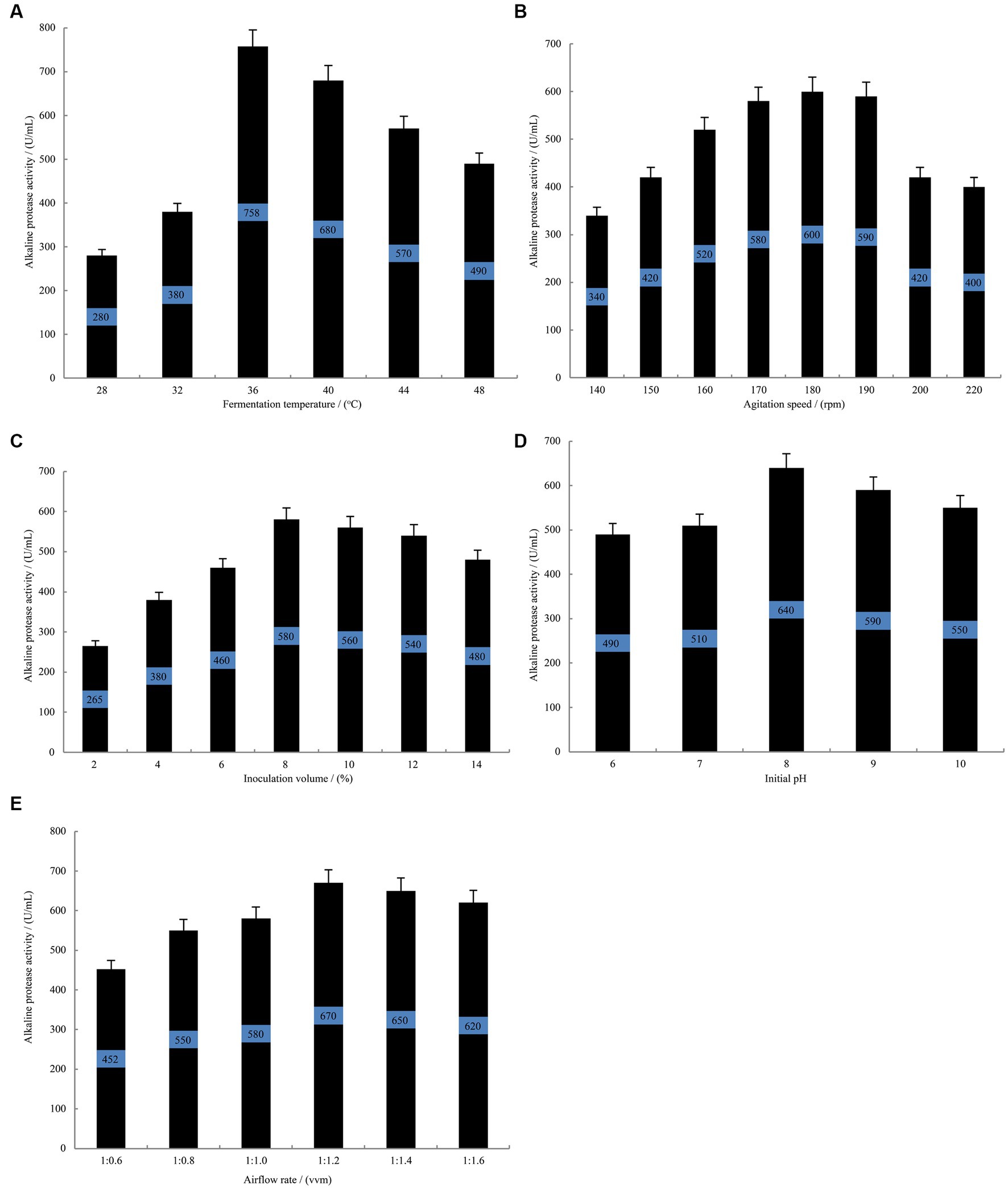
Figure 3. The single-variable experiments conducted to evaluate the fermentation conditions. The effect of fermentation temperature (A) agitation speed (B) inoculation volume (C) initial pH (D) airflow rate (E) on the alkaline protease production by B. subtilis BS-QR-052. Values are the mean of three replicas ǂ standard error or standard deviation.
3.3 The rapid screening of significant factors influencing enzyme activity
Based on the single-variable experiments, a comprehensive investigation of the interaction effects of variables, including corn starch (A), corn syrup powder (B), MgSO4 (C), MnSO4 (D), agitation speed (E), fermentation temperature (F), airflow rate (G), inoculation volume (H), and initial pH (I), was performed according to the Plackett–Burman design in Table 1. As resulted in Supplementary Table S1, the enzyme activity responses for all experimental combinations exceeded 1,000 U/mL, reaching a maximum of 1,258 U/mL. In Table 2, the model (in terms of coded factors: Y = 1103.50 + 12.17A-38.17B-17.50C-16.50D-7.17E+15.83F-21.17G + 23.83H + 10.17I) established in this experiment exhibits extremely high significance with a p-value <0.01. Corn syrup powder, MgSO4, inoculation volume, and airflow rate had significant effects at a significance level of 0.01 < p < 0.05. However, the remaining factors do not have a significant effect on the production of alkaline protease by B. subtilis at a significance level of p < 0.05. Therefore, these four factors need to be further explored.
3.4 Response surface methodology and experimental verification
In Supplementary Table S2, these four significant factors, namely corn syrup powder (X1), MgSO4 (X2), inoculation volume (X3) and airflow rate (X4), were selected to conduct Box–Behnken design, aiming to explore the fermentation process of B. subtilis in the production of alkaline protease. Other variables were maintained at constant levels: 0.5% corn starch, 0.05% MnSO4, fermentation temperature of 36°C, agitation speed of 180 rpm, and initial pH of 8.0. The Supplementary Table S3 obtained the results that the enzyme activity response values of most experimental groups exceeded 1,000 U/mL. Overall, when all four variables are set to the 0 level, the enzyme activity response value is approximately 1700, indicating a relatively optimal combination. Furthermore, the variance analysis of the regression model demonstrated that the regression equation prediction model can be represented as:
The significance of the experimental model’s effect on the response value Y is evident from Table 3, as indicated by its extremely low p-value (<0.0001), thus providing high credibility. The lack-of-fit item in the model exhibits a p-value of 0.7504, which is greater than the significance level of 0.05, implying that the model fit is satisfactory. Based on the p-values, it can be concluded that all square terms have highly significant effects on the activity of alkaline protease produced by B. subtilis (p < 0.01). This result once again confirms the significant impact of these four factors, among the nine factors studied, on the accumulation of alkaline protease. The linear terms of corn syrup powder, MgSO4, inoculation volume, and airflow rate have corresponding F values of 1.35, 7.10, 0.45, and 6.93, respectively. These values suggest that the factors influencing the accumulation of alkaline protease follow the order: MgSO4 > airflow rate > corn syrup powder > inoculation volume. When considering the F value and p-value of both the linear and quadratic terms, the influence of MgSO4 and airflow rate on enzyme activity is also likely to be more prominent compared to the inoculation volume and corn syrup powder. Additionally, the coefficient of determination (adjusted R2) for the model is 0.9294, demonstrating a strong correlation between the actual and predicted values. The estimated value of the predicted R2 is 0.7087, with a difference of 0.15 from the adjusted R2, which is less than 0.2. This indicates that the regression model can adequately explain the manufacturing process. A coefficient of variation (c.v.) value of 8.14% being below the threshold of 10% indicates high reliability and precision in the experiment. Furthermore, the residual diagnostic analysis provides the normal probability plot of residuals, the plot of residuals against predicted values, and the plot of predicted values against actual values. Supplementary Figure S2 shows that the normal probability plot of residuals is approximately linear, indicating a nearly normal distribution of residuals. The plot of residuals against predicted values does not exhibit any clear patterns, suggesting the absence of systematic errors. Additionally, the plot of predicted values against actual values also shows an ideal linear distribution. Overall, these findings indicate the accuracy of the regression model.
RSM overcomes the limitation of orthogonal experiments, which do not provide intuitive visual representations. It involves creating three-dimensional response surface plots and contour plots based on quadratic equations to illustrate the interactions between experimental factors. Generally, response surface plots and contour plots visually represent the impact of interactions on response values. Steeper surfaces and denser contour lines indicate more significant effects, and when contour lines form ellipses, it signifies a stronger interaction between the two factors. As depicted in Figure 4B, it indicates a significant interaction (p < 0.05) between corn syrup powder (X1) and inoculation volume (X3), resulting in a steeper response surface. This observation is consistent with the contour plot presented in Supplementary Figure S3B, where a discernible elliptical pattern is observed, indicating the presence of an interaction effect between the two factors. The contour plots of corn syrup powder (X1) and MgSO4 (X2) exhibit an elliptical trend. Although the regression model shows a p-value of 0.0915 > 0.05, not reaching the significance threshold, there still exists a certain degree of interaction effect (Supplementary Figure S3A). Except for these two combinations, the remaining four combinations did not exhibit strong interaction effects. The contour plots of the four combinations (Supplementary Figures S3C–F) all show approximately circular patterns. In contrast, the three-dimensional response surface plots for all six combinations (Figure 4) display distinct steep trends, indicating a significant impact of the four factors on alkaline protease. Overall, the experimental model effectively captures the variations in enzyme activity of alkaline protease produced by B. subtilis under different conditions. Consequently, it can be employed for the analysis and prediction of alkaline protease enzyme activity in B. subtilis fermentation.
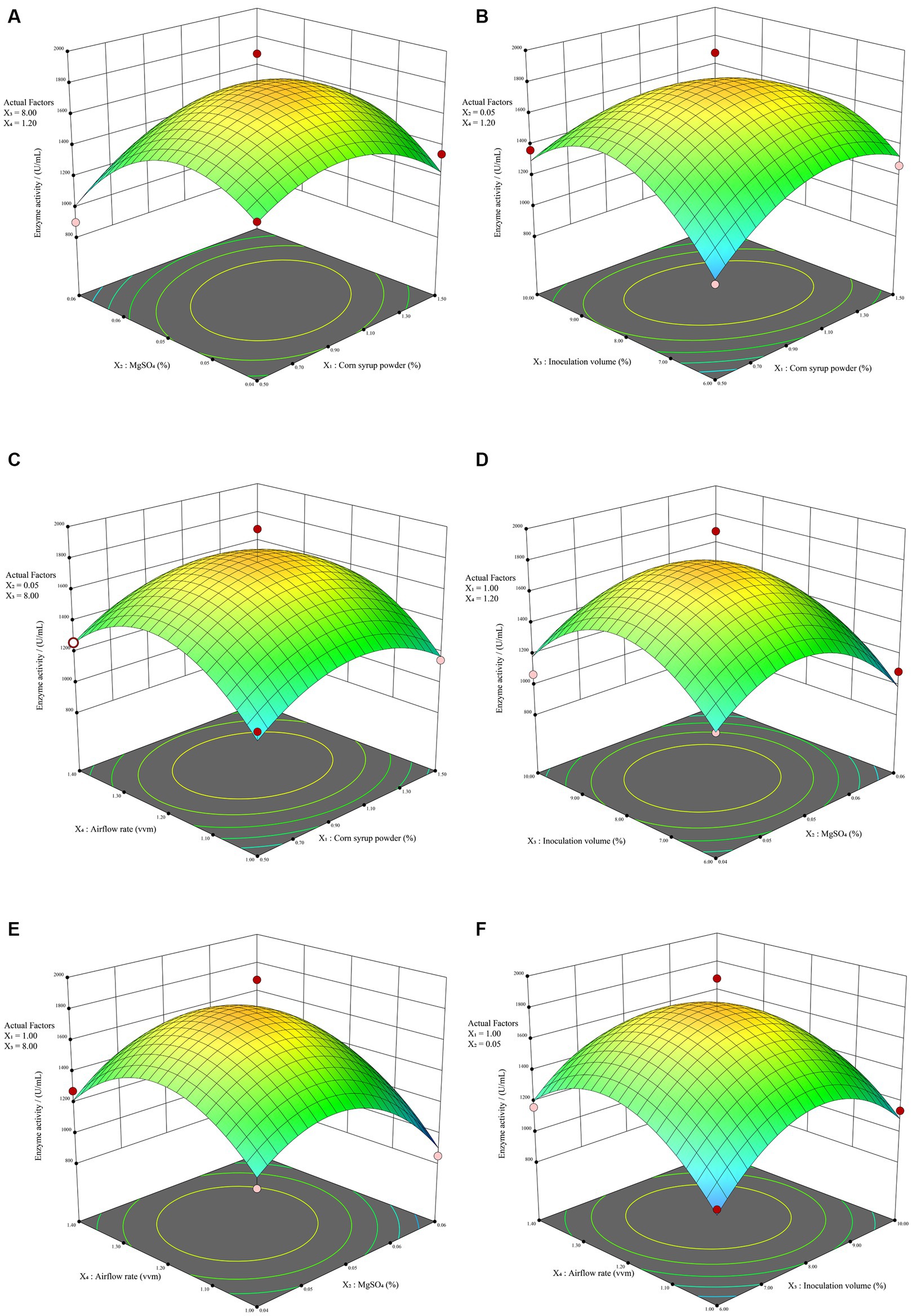
Figure 4. The RSM results. The response surface plot illustrating the impact of the interaction between corn syrup powder and MgSO4 (A), corn syrup powder and inoculation volume (B), corn syrup powder and airflow rate (C), MgSO4 and inoculation volume (D), MgSO4 and airflow rate (E), inoculation volume and airflow rate (F) on enzyme activity.
Finally, based on the optimal model mentioned above, the recommended combination of 1.03% corn syrup powder, 0.05% MgSO4, 8.02% inoculation volume, and 1:1.22 vvm airflow rate was adopted in the confirmatory trial. The other variables used for fermentation verification were maintained at 0.5% corn starch, 0.05% MnSO4, a fermentation temperature of 36°C, an agitation speed of 180 rpm and an initial pH of 8.0. As a result, the measured activity of alkaline protease during the verification was 1780.03 U/mL, which closely matched the predicted value of 1787.91 U/mL, demonstrating the reliability of the mathematical model established in this research.
3.5 The 500 L scale-up fermentation of Bacillus subtilis for producing alkaline protease
A scale-up test was conducted based on the previous fermentation optimization, using a 500 L fermentation system. Figure 5 illustrates the monitoring of control parameters throughout the fermentation test. The observed changes further validated the repeatability of the experimental results. During the initial 0–24 h, the population of B. subtilis increased slowly, and the enzyme activity remained close to 0, indicating a lag phase of the strain in the new medium environment. After 26 h, the number of viable bacteria in the fermentation broth began to increase rapidly. At about 70 h, the number of viable bacteria is close to the equilibrium period, about 490–500 × 108 CFU/mL. Concurrently, the enzyme activity of alkaline protease started to rise rapidly, along with the accumulation of secondary metabolites. By the 94 h mark, the enzyme activity reached 1798.33 U/mL, which signifies that the enzymatic activity of the alkaline protease after optimization reached 300.72% of the pre-optimized level. As the culture time extended, the number of viable bacteria in the fermentation broth gradually declined, accompanied by a significant production of spores. This period corresponded to the depletion of nutrients in the fermented liquid.
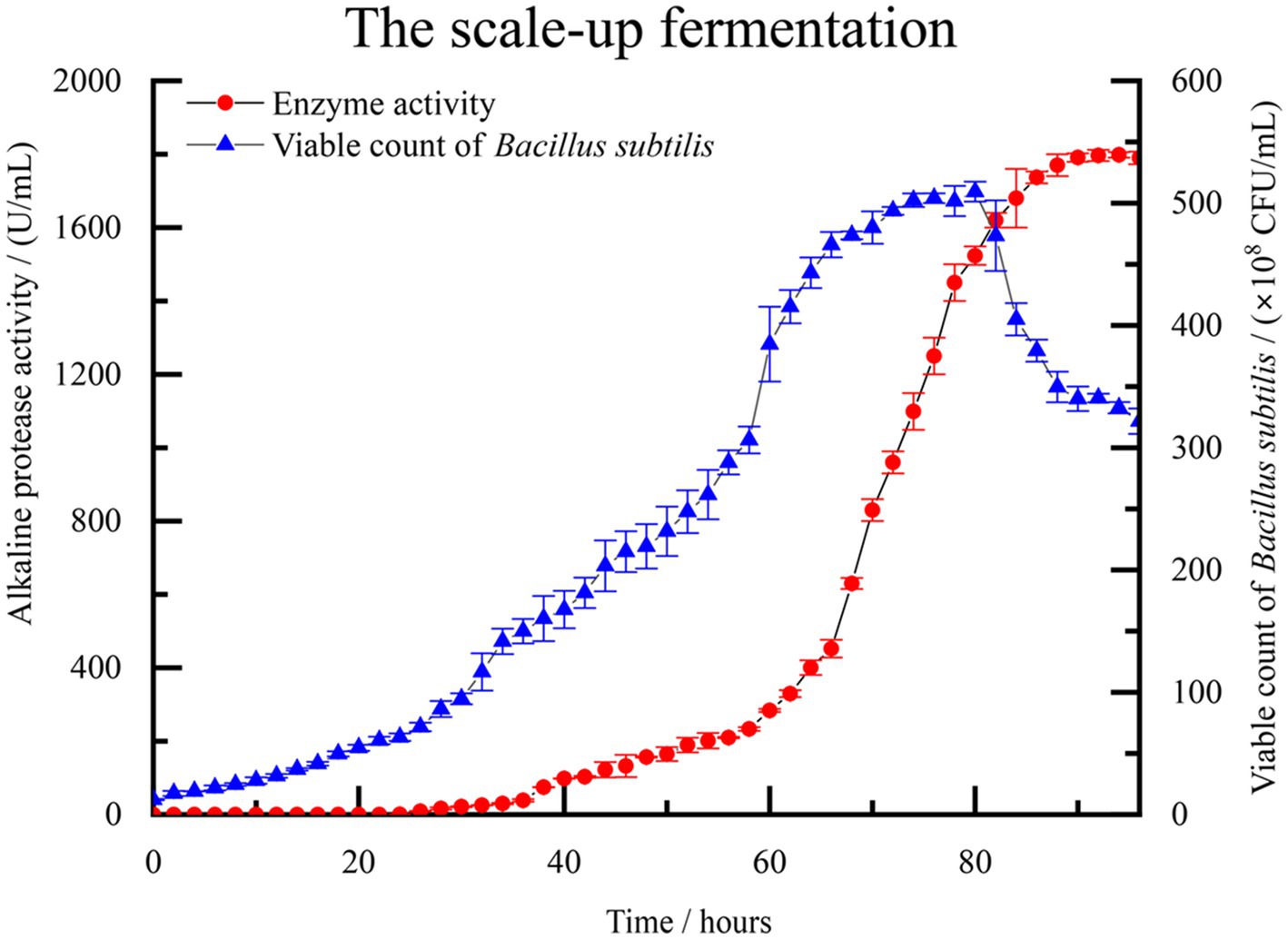
Figure 5. The fermentation curve of B. subtilis in 500 L scale-up fermentation. Enzyme activity is represented by solid red circles, while the count of viable B. subtilis spores is represented by solid blue triangles.
4 Discussion
4.1 Bacillus subtilis BS-QR-052 begins to accumulate alkaline protease at the beginning of the logarithmic phase and continues until the stationary phase or decline phase
Numerous studies have indicated that in most cultivation conditions, species of Bacillus, like Bacillus subtilis, produce extracellular alkaline proteinases during the late exponential and stationary phases of batch culture growth (Singh et al., 2004; Zhou et al., 2019; Jafari et al., 2023). This pattern aligns with the consistency observed in our study, both before and after the optimization of the medium and fermentation conditions. Furthermore, other microbial species, such as Pseudomonas aeruginosa (Saleem, 2012), also exhibit similar fermentation patterns when synthesizing alkaline proteases. The model demonstrates that in Bacillus fermentation processes with shorter cycles (10–30 h), the peak activity of alkaline protease occurs 2–12 h after the point of maximum biomass (Singh et al., 2004; Subba Rao et al., 2009), while in longer fermentation cycles (60–80 h), the peak activity of alkaline protease lags behind the time of highest cell density by 30–38 h (Saleem, 2012; Jafari et al., 2023). However, the stage of the cell growth cycle at which extracellular protease synthesis reaches its maximum has been a topic of ongoing debate. The situations can be categorized into two classes (Priest, 1977): (i) during the active growth phase, the secretion rate of extracellular proteases is very low, and subsequently increases during the late exponential and early stationary phases of growth; (ii) the synthesis and secretion of extracellular enzymes increase with growth and decrease as the culture enters the stationary phase. The possible reasons for this may be attributed to different carbon sources (Herbert, 1961) and spores (Mao et al., 1992). Cells cultured on a single carbon source experience a rapid decrease in metabolic activity and RNA content as they transition from the exponential to the stationary phase. However, when complex organic compounds are used as carbon or nitrogen sources, cells in the late exponential and early stationary phases can maintain a certain level of metabolic activity and RNA content, leading to continued accumulation of proteases (Herbert, 1961). Hence, in this research, the use of complex organic carbon and nitrogen sources is the primary factor contributing to this phenomenon.
Although this study resulted in a reduction of approximately 30 h in the fermentation cycle before and after optimization, and the delay in alkaline protease accumulation compared to cell proliferation was reduced from 20 h to 10 h, the overall fermentation cycle remains long at approximately 90 h. Generally, the synthesis and accumulation of microbial extracellular proteases are strongly influenced by the composition of the culture medium (Bhunia et al., 2012). Factors such as the C/N (carbon-to-nitrogen) ratio, the presence of easily metabolizable sugars (such as glucose) and metal ions (e.g., Mg2+ and Ca2+), and the availability of rapidly utilizable nitrogen sources, like amino acids, have been confirmed to influence on the synthesis of alkaline protease significantly. In this research, corn starch was used as a carbon source to avoid glucose catabolic repression. Corn syrup powder is used as an organic nitrogen source, which can promote higher protease production and avoid the inhibitory effect of rapidly available nitrogen sources on protease synthesis. The use of organic carbon and nitrogen sources reduces costs and avoids metabolic inhibition, but it prolongs the production cycle to a certain extent, which may be the main reason why the fermentation cycle lasted more than 90 h in this study.
4.2 Nutrition and conditions are important factors affecting the production of alkaline protease by Bacillus subtilis BS-QR-052
High productivity is one of the advantages of B. subtilis in producing alkaline protease. The enzyme activity of alkaline protease produced by wild strains ranges from 50 to 500 U/mL (Nisha and Divakaran, 2014; Bukhari et al., 2020), and can be increased to 500–1,000 U/mL through optimization of the culture medium or overexpression methods (Shafique et al., 2021; Emon et al., 2023). In this study, the enzyme activity was 598 U/mL and 1780.03 U/mL before and after optimization, respectively, representing a remarkable 198% improvement. While the fold increase achieved may be slightly lower compared to other process optimization strategies (Ozdenefe et al., 2017), the enzyme activity still reached a significantly high level. Additionally, implementing measures such as fed-batch fermentation (Chen et al., 2022) can further enhance the production of alkaline protease, highlighting the productivity advantage of B. subtilis in the field of alkaline protease production. Therefore, selecting suitable nutrients and optimizing various process conditions are crucial approaches to maximize the yield of alkaline protease produced through B. subtilis fermentation.
4.3 Composite sources are more effective, and inorganic salts and metal ions are essential
The cultivation medium for alkaline protease production in B. subtilis typically comprises carbon sources, nitrogen sources, inorganic salts, and metal ions. Common carbon sources include glucose, sucrose, lactose, maltose, corn starch, maltodextrin, and cornmeal. Nitrogen sources commonly used are soybean meal, peptone, urea, yeast extract, beef paste, soybean cake powder, and corn syrup powder. Previous research suggests that a combination of carbon and nitrogen sources may be more suitable for enhancing the fermentation process and maximizing alkaline protease production by B. subtilis (Krishnaveni et al., 2012; Nisha and Divakaran, 2014; Sathishkumar et al., 2015). In this study, we employed a composite carbon source (corn starch) and a composite nitrogen source (corn syrup powder) to optimize the production yield of alkaline protease. Through single-variable optimization, we achieved an impressive nearly 1-fold increase in production yield. Notably, corn syrup powder, as a composite nitrogen source, emerged as a significant factor influencing the yield, closely linked to the secretion of extracellular protease. Additionally, the inclusion of inorganic salts and metal ions contributed to the maintenance of extracellular alkaline protease stability, aligning with the pronounced impact observed with the addition of MgSO4 (Nisha and Divakaran, 2014). Hence, the search for an optimal combination of cultivation medium components holds great promise in promoting the maximum secretion of extracellular alkaline protease by B. subtilis.
4.4 Statistical modeling and response surface methodology have significant potential applications in the optimization processes of biochemical systems
RSM stands out as a powerful tool in the optimization processes of chemical and biochemical systems. Its considerable success in the realm of biotechnological industrial production is evident in various reports. Notably, RSM has proven effective in optimizing the production of microbial-synthesized products, including enzymes, pigments, and pectin oligosaccharides (Breig and Luti, 2021). For example, Lysinibacillus sphaericus AA6 EMCCN3080 achieved a remarkable 98-fold increase in alkaline protease (ALK-PR23) activity through RSM optimization (Matrawy et al., 2023b). In our study, a similar approach significantly enhanced alkaline protease activity, reaching an impressive 1798.33 U/mL. Other instances include the 7.58-fold increase in α-amylase (CA-AM21) yield in Glutamicibacter soli AM6 EMCCN 3074 (Matrawy et al., 2023a), and the 8.95 and 5-fold production boosts in xylanase for Penicillium chrysogenum A3 DSM105774 (Matrawy et al., 2021b) and Thermomyces lanuginosus A3-1 DSM 105773 (Matrawy et al., 2021a), respectively. Furthermore, the pectinase from Aspergillus exhibited a 13-fold improvement in producing pectin oligosaccharides during citrus pectin fermentation (Embaby et al., 2016). In the biotransformation of natural pigments, the application of RSM significantly elevated the pigment production efficiency in Monascus purpureus ATCC 16436 strain (Embaby et al., 2018). These findings underscore the versatile and impactful role of RSM in optimizing diverse biochemical processes for enhanced biotechnological outcomes.
5 Conclusion
In this study, a series of experiments were conducted to optimize the fermentation medium composition and process conditions for the production of B. subtilis BS-QR-052 alkaline protease. The results indicate that corn starch and corn syrup powder are suitable carbon and nitrogen sources, while the presence of Mg2+ and Mn2+ positively influences the production of alkaline protease. Moreover, specific process conditions were identified as optimal: a fermentation temperature of 36°C, an inoculation volume of 8%, an initial pH of 8.0, an agitation speed of 180 rpm, and an airflow rate of 1:1.2 vvm. Notably, the Plackett-Burman design revealed that corn syrup powder, MgSO4, inoculation volume, and airflow rate had significant impacts on the accumulation of alkaline protease. Through RSM, a prediction model was simulated, and the optimal values were predicted. Subsequently, the model was experimentally validated, and after optimization, the enzyme’s activity increased to 300.72% of its original level. This indicates that the findings of this study possess certain stability and repeatability, laying the foundation for further production and promotion of alkaline protease.
Data availability statement
The original contributions presented in the study are included in the article/Supplementary material, further inquiries can be directed to the corresponding author.
Author contributions
BS: Conceptualization, Funding acquisition, Methodology, Project administration, Resources, Writing – original draft. KZ: Conceptualization, Data curation, Investigation, Methodology, Project administration, Validation, Visualization, Writing – original draft, Writing – review & editing. YiZ: Data curation, Investigation, Methodology, Project administration, Resources, Validation, Writing – review & editing. YT: Conceptualization, Investigation, Methodology, Writing – review & editing. FZ: Formal analysis, Funding acquisition, Project administration, Resources, Supervision, Writing – review & editing. WC: Formal analysis, Project administration, Supervision, Writing – review & editing. XT: Data curation, Methodology, Validation, Writing – review & editing. CC: Data curation, Methodology, Validation, Writing – review & editing. YaZ: Data curation, Methodology, Validation, Writing – review & editing.
Funding
The author(s) declare financial support was received for the research, authorship, and/or publication of this article. This work was supported by the following grants: Nanhu District Science and Technology Plan Project of Jiaxing City (SQ2020000016), The “14th Five-Year Plan” Teaching Reform Project of Ordinary Undergraduate Universities in Zhejiang Province (jg20220697), Scientific Research Project of Jiaxing Nanhu University (62203ZL), and Research Start-up Funding Project of Jiaxing Nanhu University (QD61220020).
Conflict of interest
BS, YT, and FZ were employed by Zhejiang Cheery Bio-Technology Co., Ltd. YiZ was employed by Sinochem Linyi Crop Nutrition Co., Ltd.
The remaining authors declare that the research was conducted in the absence of any commercial or financial relationships that could be construed as a potential conflict of interest.
Publisher’s note
All claims expressed in this article are solely those of the authors and do not necessarily represent those of their affiliated organizations, or those of the publisher, the editors and the reviewers. Any product that may be evaluated in this article, or claim that may be made by its manufacturer, is not guaranteed or endorsed by the publisher.
Supplementary material
The Supplementary material for this article can be found online at: https://www.frontiersin.org/articles/10.3389/fmicb.2023.1301065/full#supplementary-material
References
Bhunia, B., Basak, B., and Dey, A. (2012). A review on production of serine alkaline protease by Bacillus spp. J. Biochem. Technol. 3, 448–457.
Box, G. E., and Wilson, K. B. (1992). “On the experimental attainment of optimum conditions” in Breakthroughs in statistics: methodology and distribution. eds. S. Kotz and N. L. Johnson (New York, NY: Springer), 270–310.
Breig, S. J. M., and Luti, K. J. K. (2021). Response surface methodology: a review on its applications and challenges in microbial cultures. Mater. Today: Proc. 42, 2277–2284. doi: 10.1016/j.matpr.2020.12.316
Bukhari, D. A., Barkat, A., and Rehman, A. (2020). Expression, purification, and molecular characterization of a full-length thermostable alkaline protease gene from Bacillus subtilis DMA-09. Biologia 76, 741–750. doi: 10.2478/s11756-020-00608-6
Chen, H., Wu, J., Huang, X., Feng, X., Ji, H., Zhao, L., et al. (2022). Overexpression of Bacillus circulans alkaline protease in Bacillus subtilis and its potential application for recovery of protein from soybean dregs. Front. Microbiol. 13:968439. doi: 10.3389/fmicb.2022.968439
Embaby, A. M., Hussein, M. N., and Hussein, A. (2018). Monascus orange and red pigments production by Monascus purpureus ATCC16436 through co-solid state fermentation of corn cob and glycerol: an eco-friendly environmental low cost approach. PLoS One 13:e0207755. doi: 10.1371/journal.pone.0207755
Embaby, A. M., Melika, R. R., Hussein, A., El-Kamel, A. H., and Marey, H. S. (2016). A novel non-cumbersome approach towards biosynthesis of pectic-oligosaccharides by non-aflatoxigenic Aspergillus sp. section Flavi strain EGY1 DSM 101520 through citrus pectin fermentation. PLoS One 11:e0167981. doi: 10.1371/journal.pone.0167981
Emon, T. H., Hakim, A., Chakraborthy, D., and Azad, A. K. (2023). Enhanced production of dehairing alkaline protease from Bacillus subtilis mutant E29 by consolidated bioprocessing using response surface modeling. Biomass Convers. Biorefin. doi: 10.1007/s13399-023-04244-3
Ferreira, S. L., Bruns, R. E., Ferreira, H. S., Matos, G. D., David, J. M., Brandao, G. C., et al. (2007). Box–Behnken design: an alternative for the optimization of analytical methods. Anal. Chim. Acta 597, 179–186. doi: 10.1016/j.aca.2007.07.011
Harwood, C. R., Mouillon, J. M., Pohl, S., and Arnau, J. (2018). Secondary metabolite production and the safety of industrially important members of the Bacillus subtilis group. FEMS Microbiol. Rev. 42, 721–738. doi: 10.1093/femsre/fuy028
Hashem, A., Tabassum, B., and Fathi Abd Allah, E. (2019). Bacillus subtilis: a plant-growth promoting rhizobacterium that also impacts biotic stress. Saudi J. Biol. Sci. 26, 1291–1297. doi: 10.1016/j.sjbs.2019.05.004
Herbert, D. (1961). The chemical composition of micro-organisms as a function of their environment. Symp. Soc. Gen. Microbiol. 11, 391–416.
Huang, Y., Wang, J., Hou, Y., and Hu, S.-Q. (2020). Production of yeast hydrolysates by Bacillus subtilis derived enzymes and antihypertensive activity in spontaneously hypertensive rats. Food Biotechnol. 34, 262–281. doi: 10.1080/08905436.2020.1791174
Ito, S., Kobayashi, T., Ara, K., Ozaki, K., Kawai, S., and Hatada, Y. (1998). Alkaline detergent enzymes from alkaliphiles: enzymatic properties, genetics, and structures. Extremophiles 2, 185–190. doi: 10.1007/s007920050059
Jafari, Z., Najafpour Darzi, G., and Zare, H. (2023). Growth media optimization for production of alkaline protease from industrial wastewater using Bacillus subtilis PTCC 1254. Int. J. Eng. 36, 513–522. doi: 10.5829/IJE.2023.36.03C.11
Khuri, A. I., and Mukhopadhyay, S. (2010). Response surface methodology. Wiley Interdiscip. Rev.: Comput. Stat. 2, 128–149. doi: 10.1002/wics.73
Krishnaveni, K., Kumar, D. M., Balakumaran, M., Ramesh, S., and Kalaichelvan, P. (2012). Production and optimization of extracellular alkaline protease from Bacillus subtilis isolated from dairy effluent. Pharm. Lett. 4, 98–109.
Mao, W., Pan, R., and Freedman, D. (1992). High production of alkaline protease by Bacillus licheniformis in a fed-batch fermentation using a synthetic medium. J. Ind. Microbiol. 11, 1–6. doi: 10.1007/BF01583724
Matrawy, A. A., Khalil, A. I., and Embaby, A. M. (2023a). Bioconversion of bread waste by marine psychrotolerant glutamicibacter soli strain AM6 to a value-added product: cold-adapted, salt-tolerant, and detergent-stable α-amylase (CA-AM21). Biomass Convers. Biorefin. 13, 12125–12142. doi: 10.1007/s13399-022-02325-3
Matrawy, A. A., Khalil, A. I., Marey, H. S., and Embaby, A. M. (2021a). Biovalorization of the raw agro-industrial waste rice husk through directed production of xylanase by Thermomyces lanuginosus strain A3-1 DSM 105773: a statistical sequential model. Biomass Convers. Biorefin. 11, 2177–2189. doi: 10.1007/s13399-020-00824-9
Matrawy, A. A., Khalil, A. I., Marey, H. S., and Embaby, A. M. (2021b). Use of wheat straw for value-added product xylanase by penicillium chrysogenum strain A3 DSM105774. J. Fungi 7:696. doi: 10.3390/jof7090696
Matrawy, A. A., Marey, H. S., and Embaby, A. M. (2023b). “The agro-industrial byproduct wheat bran as an inducer for alkaline protease (ALK-PR23) production by Pschyrotolerant strain AA6 EMCCN3080” in Waste biomass valorization, 1–16. doi: 10.1007/s12649-023-02283-5
Mei, C., and Jiang, X. (2005). A novel surfactant-and oxidation-stable alkaline protease from Vibrio metschnikovii DL 33-51. Process Biochem. 40, 2167–2172. doi: 10.1016/j.procbio.2004.08.007
Morikawa, M. (2006). Beneficial biofilm formation by industrial bacteria Bacillus subtilis and related species. J. Biosci. Bioeng. 101, 1–8. doi: 10.1263/jbb.101.1
Nisha, N., and Divakaran, J. (2014). Optimization of alkaline protease production from Bacillus subtilis NS isolated from sea water. Afr. J. Biotechnol. 13, 1707–1713. doi: 10.5897/AJB2014.13652
Ozdenefe, M. S., Dincer, S., Unal, M. U., Kayis, F. B., Takci, H. A. M., and Arkut, A. (2017). Optimization of culture conditions for alkaline protease production from waste breads using Bacillus subtilis. Rom. Biotechnol. Lett. 22, 12597–12610.
Pant, G., Prakash, A., Pavani, J., Bera, S., Deviram, G., Kumar, A., et al. (2015). Production, optimization and partial purification of protease from Bacillus subtilis. J. Taibah Univ. Sci. 9, 50–55. doi: 10.1016/j.jtusci.2014.04.010
Plackett, R. L., and Burman, J. P. (1946). The design of optimum multifactorial experiments. Biometrika 33, 305–325. doi: 10.1093/biomet/33.4.305
Priest, F. G. (1977). Extracellular enzyme synthesis in the genus Bacillus. Bacteriol. Rev. 41, 711–753. doi: 10.1128/br.41.3.711-753.1977
Qi, J., Wang, B., and Hao, B.-I. (2004). Whole proteome prokaryote phylogeny without sequence alignment: AK-string composition approach. J. Mol. Evol. 58, 1–11. doi: 10.1007/s00239-003-2493-7
Qureshi, A. S., Bhutto, M. A., Khushk, I., and Dahot, M. U. (2011). Optimization of cultural conditions for protease production by Bacillus subtilis EFRL 01. Afr. J. Biotechnol. 10, 5173–5181.
Rekik, H., Zarai Jaouadi, N., Gargouri, F., Bejar, W., Frikha, F., Jmal, N., et al. (2019). Production, purification and biochemical characterization of a novel detergent-stable serine alkaline protease from Bacillus safensis strain RH12. Int. J. Biol. Macromol. 121, 1227–1239. doi: 10.1016/j.ijbiomac.2018.10.139
Saleem, A. J. (2012). Relationship study between the alkaline protease production and the growth phases of Pseudomonas aeruginosa isolated from patients. Adv. Microbiol. 2, 354–357. doi: 10.4236/aim.2012.23043
Sathishkumar, R., Ananthan, G., and Arun, J. (2015). Production, purification and characterization of alkaline protease by ascidian associated Bacillus subtilis GA CAS8 using agricultural wastes. Biocatal. Agric. Biotechnol. 4, 214–220. doi: 10.1016/j.bcab.2014.12.003
Shafique, T., Shafique, J., Zahid, S., Kazi, M., Alnemer, O., and Ahmad, A. (2021). Screening, selection and development of Bacillus subtilis apr-IBL04 for hyper production of macromolecule alkaline protease. Saudi J. Biol. Sci. 28, 1494–1501. doi: 10.1016/j.sjbs.2020.11.079
Sharma, K. M., Kumar, R., Panwar, S., and Kumar, A. (2017). Microbial alkaline proteases: optimization of production parameters and their properties. J. Genet. Eng. Biotechnol. 15, 115–126. doi: 10.1016/j.jgeb.2017.02.001
Singh, P., Rani, A., and Chaudhary, N. (2015). Isolation and characterization of protease producing Bacillus sp from soil. Int. J. Pharm. Sci. Res. 6, 633–639.
Singh, J., Vohra, R., and Sahoo, D. (2004). Enhanced production of alkaline proteases by Bacillus sphaericus using fed-batch culture. Process Biochem. 39, 1093–1101. doi: 10.1016/S0032-9592(03)00217-6
Subba Rao, C., Sathish, T., Pendyala, B., Kumar, T. P., and Prakasham, R. S. (2009). Development of a mathematical model for Bacillus circulans growth and alkaline protease production kinetics. J. Chem. Technol. Biotechnol. 84, 302–307. doi: 10.1002/jctb.2040
Vanitha, N., Rajan, S., and Murugesan, A. (2014). Optimization and production of alkaline protease enzyme from Bacillus subtilis 168 isolated from food industry waste. Int. J. Curr. Microbiol. App. Sci. 3, 36–44.
Woese, C. R., and Fox, G. E. (1977). Phylogenetic structure of the prokaryotic domain: the primary kingdoms. Proc. Natl. Acad. Sci. U.S.A. 74, 5088–5090. doi: 10.1073/pnas.74.11.5088
Keywords: Bacillus subtilis , alkaline protease, fermentation optimization, single-variable experiments, response surface methodology
Citation: Sun B, Zou K, Zhao Y, Tang Y, Zhang F, Chen W, Tang X, Chang C and Zheng Y (2023) The fermentation optimization for alkaline protease production by Bacillus subtilis BS-QR-052. Front. Microbiol. 14:1301065. doi: 10.3389/fmicb.2023.1301065
Edited by:
Sikandar I. Mulla, REVA University, IndiaReviewed by:
Prashant Bhagwat, Durban University of Technology, South AfricaAsma Ansari, Karachi Institute of Biotechnology and Genetic Engineering (KIBGE), Pakistan
Amira Embaby, Alexandria University, Egypt
Copyright © 2023 Sun, Zou, Zhao, Tang, Zhang, Chen, Tang, Chang and Zheng. This is an open-access article distributed under the terms of the Creative Commons Attribution License (CC BY). The use, distribution or reproduction in other forums is permitted, provided the original author(s) and the copyright owner(s) are credited and that the original publication in this journal is cited, in accordance with accepted academic practice. No use, distribution or reproduction is permitted which does not comply with these terms.
*Correspondence: Kai Zou, em91a2FpQGp4bmh1LmVkdS5jbg==