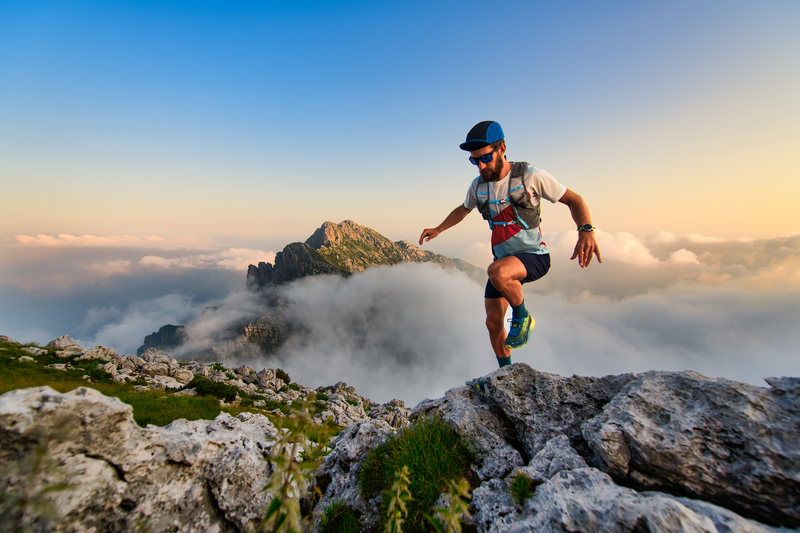
95% of researchers rate our articles as excellent or good
Learn more about the work of our research integrity team to safeguard the quality of each article we publish.
Find out more
ORIGINAL RESEARCH article
Front. Microbiol. , 16 November 2023
Sec. Microbial Symbioses
Volume 14 - 2023 | https://doi.org/10.3389/fmicb.2023.1298632
This article is part of the Research Topic The Gut-Skin Axis: Interaction of Gut Microbiome and Skin Diseases View all 12 articles
Skin is a diverse ecosystem that provides a habitat for microorganisms. The skin condition and the skin microbiome interact each other under diverse environmental conditions. This study was conducted on 10 study participants for a one-year, from September 2020 to August 2021, to investigate the variability of skin microbiome and skin biophysical parameters [TEWL, hydration, and elasticity (R5)] according to season, and to understand the interplay between skin microbiome and skin characteristics. We identified that Cutibacterium, Corynebacterium, Staphyloccocus, unclassified genus within Neisseriaceae, and Streptococcus were major skin microbial taxa at the genus level, and fluctuated with the seasons. Cutibacterium was more abundant in winter, while Corynebacterium, Staphylococcus, and Streptococcus were more abundant in summer. Notably, Cutibacterium and skin barrier parameter, TEWL, exhibited a co-decreasing pattern from winter to summer and showed a significant association between Cutibacterium and TEWL. Furthermore, functional profiling using KEGG provided clues on the impact of Cutibacterium on the host skin barrier. This study enhances our understanding of the skin microbiome and its interplay with skin characteristics and highlights the importance of seasonal dynamics in shaping skin microbial composition.
The skin is a primary physical barrier against the invasion of pathogens, and also is a diverse ecosystem that can be a habitat for microorganisms including bacteria and fungi (Chiller et al., 2001; Fredricks, 2001). The ecology of the skin is varied topographically and influenced by various elements including environment and host factors (Fierer et al., 2008; Grice et al., 2009; Isler et al., 2023). The host’s characteristics, such as age and sex, contribute to the diversity of the skin microbiome (Somerville, 1969; Grice and Segre, 2011). On the other hand, the environmental factors such as temperature and humidity, have been reported as stimuli of the growth of skin microbiome (Duncan et al., 1969). The ultraviolet radiation stimulates the overproduction of oil glands and thickens the outermost layer of skin (Pearse et al., 1987; Lesnik et al., 1992), which may lead to the growth of lipophilic microorganisms (Dréno et al., 2018; Kobayashi et al., 2019).
Recent studies have revealed that the skin microbiome plays an important role in maintaining skin health under specific environmental conditions (Gallo and Nakatsuji, 2011; Byrd et al., 2018). The commensal skin microbiome produces substances similar to antimicrobial peptides that contribute to the development and maintenance of the skin’s immune system (Cogen et al., 2010). The disruption of the balance between commensal and pathogenic microorganisms can lead to the onset of skin diseases (Fitz-Gibbon et al., 2013; Liu et al., 2020). Additionally, the barrier function of the skin can be influenced by skin microbiome that produces metabolites activating aryl hydrocarbon receptor, which promotes epithelial differentiation and integrity (Uberoi et al., 2021). Typically, studies investigating the associations between microbial community and specific skin phenotypes divide subjects into case and control groups. However, even within these groups, individual physiological variations persist, potentially impacting research outcomes. Intra-individual microbiome assessments are therefore useful for examining substantial associations between microbial variation and the host’s skin condition by controlling for individual intrinsic traits. A longitudinal examination of the skin microbiome may reveal the interplay between microbial dynamics and skin characteristics.
To investigate compositional variation of skin microbiome, several studies analyzed bacterial and fungal communities of human skin over various time ranges. The longitudinal studies conducted within 6 months demonstrated that composition and diversity of skin microbiome can vary temporally depending on the skin sites and subjects (Grice et al., 2009; Flores et al., 2014). On the other hand, studies conducted over a longer period of time (1–2 years), which are the most extended time scale for investigation of skin microbiome, showed that the compositional features of the skin microbiome tend to remain relatively stable (Oh et al., 2016; Hillebrand et al., 2021; Schmid et al., 2022). However, although several longitudinal studies on the skin microbiome have been performed, understanding of the complex relationship between skin microbiome and skin characteristics is still limited. This is largely due to the fact that most long-term studies on skin characteristics have been conducted independently of those on the skin microbiome (Youn et al., 2005; Nam et al., 2015; Dolečková et al., 2021), which has resulted in a lack of comprehensive understanding of their interplay.
To investigate the interactions between skin microbiome and skin characteristics, we conducted a longitudinal study tracking changes in the facial skin microbiome and various skin biophysical parameters, over a period of 1 year. We collected microbiome data at weekly intervals with a higher frequency compared to previous researches, enabling the capture of detailed changes in the microbial community and mitigating potential biases in microbiome sampling. Additionally, the skin characteristics of subjects were collected at monthly intervals simultaneously. Our study aims to offer a more comprehensive understanding of longitudinal alterations in microbiome composition and skin properties. It may help understand the interplay between these two traits and estimate the impact of microbial changes.
Participants with the following characteristics were excluded: (1) those who used systemic or topical antibiotics within 3 months prior to the study, (2) those who had cutaneous disease on the skin, and (3) those who had sensitive skin. A total of 10 healthy Korean participants (7 males and 3 females) voluntarily contributed in study. The mean age of the participants was 32.4 years. During the study period (12 months, from September 2020 to August 2021), all study participants wore a mask at least 8 h a day due to COVID-19. Facial skin microbiome samples were collected by having subjects swab their left cheek once a week using a Copan swab 480 CE (Copan, Brescia, Italy), following an infographic-guided procedure: (1) sampling right after waking up and before washing face, (2) rubbing the left cheek for 1 min. The use of cosmetics was prohibited at least 8 h before skin microbiome sample collection. All participants were required to wear a mask under routine office work conditions and to maintain cosmetic and hygiene routines as consistently as possible. All samples were stored at −80°C until further processing. Three facial skin biophysical parameters of study participants were evaluated once a month with several measurements as follows: Skin surface TEWL was measured using Tewameter® TM 300 (Courage and Khazaka GmbH, Cologne, Germany) and was expressed in grams per square meter per hour (g/m^2/h) (Gardien et al., 2016). Skin hydration was calculated using Corneomter CM 825 (Courage and Khazaka GmbH, Cologne, Germany). Changes in the capacitance of the stratum corneum were measured and were expressed in arbitrary units (CM) (Dąbrowska and Nowak, 2021). Skin surface elasticity was quantified using Cutometer MPA 580 (Courage and Khazaka GmbH, Cologne, Germany). R5 [immediate retraction (Ur)/immediate distension (Ue)], representing the net elasticity of the skin was used as elasticity index (Ohshima et al., 2013). This study was approved by the institutional review board at the LG H&H Research Center (Seoul, South Korea) and all study participants provided an institutional review board-approved written consent form (No. LGHH-20201210-AB-03).
Genomic DNA was extracted from swab sample using the QIAamp mini kit (QIAGEN, Hilden, Germany). Quantity of extracted DNA was measured with a Qubit 4 Fluorometer (Invitrogen, Massachusetts, USA). 16S rRNA gene amplicon libraries were constructed following the instructions of Illumina’s 16S rRNA metagenomics sequencing library preparations with some adaptation (Illumina, 2014) as follows: (1) locus specific amplification with two specific primers for v3- v4 variable regions of 16S rRNA gene, 341F (5′ - CCTACGGGNGGCWGCAG - 3′) and 805R (5′ - GACTACHVGGGTATCTAATCC - 3′), (2) purification of the amplicon with AMPure XP beads (Beckman Coulter, Krefeld, Germany), (3) amplification for sample indexing with Nextera XT Index kit (Illumina, California, USA), (4) purification of the amplicon with AMPure XP beads (Beckman Coulter, Krefeld, Germany), (5) validation of constructed library quantity and quality (size and integrity) with Qubit 4 Fluorometer and 2100 Bioanalyzer (Agilent, California, USA), respectively. Final library of each sample was then normalized and pooled. Pooled final library was loaded into MiSeq Reagent Kit v3 (600-cycle) (Illumina) and was sequenced on an Illumina MiSeq with 2 × 300 bp paired-end read chemistry (Illumina) (Fadrosh et al., 2014). Quality of raw sequence reads were checked using the FastQC (Babraham Institute, Cambridge, UK) and Sequencing Analysis Viewer (Illumina).
Demultiplexing of sequence reads was conducted by Illumina Miseq Reporter Software automatically. Subsequent pre-processing and clustering of demultiplexed paired-end sequence reads to obtain the clean amplicon sequence variants were processed by Quantitative Insights into Microbial Ecology 2 pipeline (2021.2.0) (Bolyen et al., 2019). In detail, the primer sequences used to amplify the v3- v4 variable regions of 16S rRNA gene were trimmed using q2-cutadapt plugin based on Cutadapt (2021.2.0) (Kechin et al., 2017). After trimming, the denoising of paired-end sequence reads, removal of chimeric sequences, and read fusion were conducted through q2-dada2 plugin based on DADA2 (2021.2.0) (Callahan et al., 2016). With the scikit-learn naïve-bayes model based pre-trained taxonomy classifier on GreenGene 13.8 99% reference database, all processed reads were matched to proper microbiome taxonomy. Further statistical analysis for the facial microbiome in this study was conducted on genus level and skin microbiome samples with low sequencing quality (read count ≦ 12,000, passing filter ≦ 80%) were excluded.
All statistical analyses were performed using R version 3.6.0. Relative abundance of microbiome was obtained by dividing the number of reads of one genus by the number of reads of all genus per subject. In order to obtain robust data, the mean value of the relative abundance of the microbiome within each participant per month (each participant was sampled per week, accumulating 4–5 samples in 1 month) was used, and four seasons were classified according to the month (spring: March, April, May; summer: June, July, August; fall: September, October, November; winter: December, January, February). The objective of the study was to observe changes in the overall microbiome. Therefore, the major five genera were selected based on their average relative abundance, which was greater than 1% in at least one-third of the samples. To compare the relative abundance of major taxa between summer and winter, Wilcoxon rank sum test was used.
Shannon diversity was calculated to examine microbiome evenness and richness of each sample (α-diversity) (Olszewski, 2004). Jensen-Shannon distance was also calculated for measuring dissimilarity between microbiome compositions of each sample (β-diversity) (Lin, 1991). To assess the statistical significance of β-diversity, permutational analysis of variance was used on the Jensen-Shannon distance matrices with 999 permutations in vegan 2.5–7 package in R (Anderson, 2001).
Normalization through Z-score transformation was conducted on measured skin parameters and the relative abundance of Cutibacterium, Corynebacterium, Staphylococcus, unclassified genus within Neisseriaceae (F), and Streptococcus. Canonical Correspondence Analysis (CCA) (Braak, 1986) was conducted to explain the dispersion of the microbiome communities with reference to factors including weather information, skin biophysical parameters and relationships between these factors. Jensen-Shannon distance matrix was used in microbiome 1.8.0 (Lahti and Shetty, 2017), vegan 2.5-7 (Oksanen et al., 2022), phyloseq 1.30.0 packages in R (McMurdie and Holmes, 2013). All statistical analyses were performed with R software (version 3.6.3.), and the ggplot2 package was used to visualize results (Wickham, 2009). To elucidate the association of skin biophysical parameters and the relative abundance of each five genera, linear regression analysis was conducted while adjusting for individual differences. Linear regression analysis adjusted for weather information (temperature, humidity: downloaded from Korea Meteorological Administration) (Supplementary Figure S1) was also performed. We considered p-value significant if less than 0.05. p-values were adjusted using the false discovery rate correction method by Benjamini-Hochberg procedure for multiple testing. Kruskal-Wallis test was used to test for significant differences in α-diversity between four season groups. Pairwise Wilcoxon rank sum test was used to compare the relative abundance of each major genus or skin biophysical parameters between pairs of seasons.
Functional profiling of the skin microbiome was conducted using q2-picrust2 (2021.2), which is based on PICRUSt2 (Douglas et al., 2020). PICRUSt2, tool for predicting functional abundances based on marker gene sequences, predicted the enriched pathway by inferring the functional profile of the facial skin microbiome based on Kyoto Encyclopedia of Genes and Genomes (KEGG) orthology gene family database (Kanehisa et al., 2004, 2012). For the purpose of identifying the pathway that can explain the difference between groups, the process was applied: comparing the KEGG orthology annotation results from PICRUSt analysis between the groups using the Kruskal-Wallis test for whole seasons, and using Wilcoxon rank-sum test for comparing summer and winter while adjusting individual factors.
We collected a total of 358 skin microbiome data from ten participants by weekly swab sampling and measured individual skin characteristics monthly. The workflow of this study is presented in Supplementary Figure S2 and a summary of the skin biophysical parameters of the study participants is presented in Supplementary Table S1. The skin microbiome compositions at the genus level of study participants on a monthly basis for 1 year are shown in Figure 1. The microbiome composition varied longitudinally, with each individual exhibiting a distinct microbial profile. Among various microbiome genera, Cutibacterium was the most dominant genus among 9 participants, with an average relative abundance of from 34 to 74% per individual. Additionally, one individual showed unclassified genus within Neisseriaceae (classified only at the family level) is the most dominant taxa.
Figure 1. The skin microbiome composition of study participants by month of the year. Average per month the relative abundance of major skin microbiome genera (Cutibacterium, Corynebacterium, Staphylococcus, unclassified genus within Neisseriaceae (classified only at the family level), and Streptococcus) of study participants. Microbial taxa which are found in less than one-third of study participants and having low abundance taxa (<1%) are grouped as ‘others’. HI, healthy individual.
At the genus level, Cutibacterium, Corynebacterium, Staphylococcus, unclassified genus within Neisseriaceae (F) and Streptococcus were major skin microbial taxa, which accounted for approximately 90% of the skin microbial community composition, and exhibited seasonal fluctuations (Figures 1, 2). In particular, the relative abundance of Cutibacterium was statistically significantly more abundant in winter than in summer (63.97 and 45.50%, respectively, p-value = 2.5E-10), while Corynebacterium, Staphylococcus, and Streptococcus were more abundant in summer than in winter (p-value <0.05).
Figure 2. Seasonal variation of the top five skin microbiome at the genus level. Boxplots of the relative abundance for the five major genera: (A) Cutibacterium, (B) Corynebacterium, (C) Staphylococcus, (D) unclassified genus within Neisseriaceae (classified only at the family level) and (E) Streptococcus. A polynomial local regression line was added to boxplots to enhance visualization of the trend. Relative abundances of panel (D) unclassified genus within Neisseriaceae and (E) Streptococcus were converted to a logarithmic scale (log10), to increase legibility.
The α-diversity of the microbial community exhibited seasonal variation (Figure 3A), with significant differences observed between the four seasons (p-value = 1.6E-10, Figure 3B). Notably, there were significant differences between most pairs of seasons (p-value <0.05) except for comparing spring 2021 and summer 2021 (Figure 3B). The β-diversity based on Jensen-Shannon distance plots according to season, month, and individuals are presented in Supplementary Figure S3. To examine skin microbiome composition varied longitudinally, a permutational analysis of variance (PERMANOVA) was performed by season and month, respectively (Supplementary Tables S2, S3). The results of the PERMANOVA analysis showed that there were significant differences in the microbiome compositions among the four seasons for all pairwise comparisons, except for the comparison between fall 2020 and winter 2021 (p-value <0.05; Supplementary Table S2). Furthermore, the distance within seasons is significantly closer than the distance between seasons, indicating the clustering of microbial communities by season (Wilcoxon rank sum test p-value <2.2E-16). Also, the microbiome compositions were clearly separated by each individual (p-value <0.05) (Supplementary Table S4).
Figure 3. Seasonal variation of α-diversity at the genus level. Boxplots of α-diversity based on panel (A) month and (B) season. In panel (A), a polynomial local regression line was added to boxplots to enhance trend visualization. In panel (B), significant differences in pairwise comparison are marked with stars (*p-value <0.05, **p-value < 0.01, ***p-value < 0.001, and ****p-value < 0.0001).
Seasonal variations were also observed in skin biophysical parameters, including TEWL, hydration, and elasticity (Figure 4; Supplementary Table S1). Especially, TEWL, which is a measure of skin barrier function, was significantly higher in the winter than in the summer (22.33 and 15.08, respectively, p-value = 5.0E-05). No significant differences in hydration were found between seasons, but elasticity showed significant differences in all pairs of seasons (Figures 4B,C). We noted longitudinal variations in both the five major microbial taxa and skin biophysical parameters, with remarkable differences between the two seasons (Figure 5). Notably, the abundance of Cutibacterium and TEWL exhibited a co-decreasing pattern from winter to summer and while the abundance of Corynebacterium and Staphylococcus showed the opposite pattern to TEWL (Figures 5A,D,G). In terms of hydration, there were coordinated patterns with Corynebacterium and Staphylococcus across the seasons, but an opposite pattern was observed in Cutibacterium with regards to hydration (Figures 5B,E,H). Elasticity showed co-increasing patterns with Staphylococcus and Streptococcus from winter to summer, but conversely, the opposite pattern was observed with Cutibacterium (Figures 5C,I,O).
Figure 4. Boxplot of skin biophysical parameters based on the season. Boxplots of panel (A) skin surface transepidermal water loss, (B) skin hydration, and (C) skin surface elasticity (R5, Ur/Ue) based on the season. Ur: immediate retraction, Ue: immediate distension. Significant differences in pairwise comparison are marked with stars (*p-value < 0.05, **p-value < 0.01, ***p-value < 0.001, and ****p-value < 0.0001).
Figure 5. Seasonal variation of the microbial taxa and skin biophysical parameters. The longitudinal movement of the relative abundance of panels (A–C) Cutibacterium, (D–F) Corynebacterium, (G–I) Staphylococcus, (J–L) Neisseriaceae (classified only at the family level), and (M–O) Streptococcus at the genus level were compared with skin biophysical parameters [(A,D,G,J,M): TEWL, (B,E,H,K,N): skin hydration, (C,F,I,L,O): skin elasticity]. The relative abundance of microbial taxa and skin biophysical parameters were normalized to the Z-score; each point represents the mean value for each season.
The Canonical Correspondence Analysis (CCA) was used for investigating the correlation between skin microbiome composition, weather conditions, and skin biophysical parameters (Supplementary Figure S4). The total inertia was 0.94 and the constrained inertia was 0.18, of which 9.6% was explained by the CCA1 axis and 3.8% by CCA2 axis. The length of each arrow reflects the strength of the variable in explaining the observed dispersion of the microbiome. Specifically, TEWL and hydration had a substantial impact on the dispersion of the microbiome.
To investigate the association between skin parameters and the skin microbiome that changes with seasonal changes, linear regression analyses were conducted using the whole collected skin microbiome data and skin biophysical data in study period. As a result, TEWL was significantly associated with the relative abundance of Cutibacterium, Corynebacterium, and Staphylococcus, respectively (p-value <0.05) (Table 1). Elasticity (R5) was also significantly associated with the relative abundance of Cutibacterium. After adjusting with false discovery rate, only Cutibacterium was significantly associated with TEWL (false discovery rate-adjusted p-value = 1.5E-05). Cutibacterium was also significantly associated with TEWL after adjusting for individual, temperature, and humidity (p-value = 0.02).
Table 1. Association analysis of the relative abundance of microbial taxa at the genus level with skin biophysical parameters.
To identify functional differences in the skin microbiome across different seasons, we conducted a predictive analysis of metagenome function by inferring enriched pathways based on sequences using PICRUSt2, which is based on the Kyoto Encyclopedia of Genes and Genomes (KEGG) database. At the second level of analysis, among 40 pathways, 36 pathways showed significant differences between seasons (false discovery rate-adjusted p-value <0.05) (Supplementary Figure S5). In particular, 33 pathways showed noticeable differences between summer and winter (Supplementary Table S5). For instance, pathways related to cell motility, the circulatory system, aging, and signal transduction were enriched in summer (Supplementary Figure S5). At the third level of analysis, among 390 pathways, 264 pathways were significantly different between summer and winter. Notably, pathways related to glycosaminoglycan degradation, oxidative phosphorylation, and porphyrin and chlorophyll metabolism were enriched in winter (Supplementary Table S6).
Understanding the skin microbiome is important as it plays an essential role in maintaining skin’s homeostasis by protecting against pathogens or controlling the immune system (Schommer and Gallo, 2013; Kobayashi et al., 2019). The impact of external environmental factors on both the skin condition and skin microbiome has been extensively investigated in previous studies (Leeming et al., 1984; Grice and Segre, 2011; Kobayashi et al., 2019; Isler et al., 2023). Since temperature and humidity obviously fluctuate with the season (Supplementary Figure S1), it is important to investigate skin microbiome and skin biophysical parameters along with seasonal changes. However, most previous studies have not investigated skin conditions and skin microbiome together longitudinally. Therefore, we conducted a one-year longitudinal study on skin biophysical parameters and skin microbiome, simultaneously.
Previous studies suggested that the bacterial community of the skin remains stable for approximately 1–2 years. However, these studies performed sampling only once or twice a year, which was insufficient to observe the annual fluctuations of the microbial community (Flores et al., 2014; Hillebrand et al., 2021). In a recent longitudinal study conducted by Oh et al. (2016), the annual variation in the mycobiota of healthy individuals was examined by collecting samples once a month, revealing that the mycobiota remained relatively stable throughout the year. However, it should be noted that this study focused solely on fungi and did not investigate bacteria and skin characteristics. Although these findings provide valuable insight into mycobiota dynamics in healthy individuals, further research is needed to fully understand the complex interplay between the microbiome and skin health.
In our study, we collected skin microbiome samples once a week and skin biophysical parameters once a month, which allowed us to collect a large amount of data and ensure the accuracy of our study results to study the relationship between skin and skin microbiome. As a result, we discovered the composition of the skin microbiome fluctuated with the seasons, in particular, that observed in the major skin microbial taxa, including Cutibacterium, Corynebacterium, and Staphylococcus. Especially, the HI-10 participant exhibited a distinct most abundant taxon, an unclassified genus within Neisseriaceae, which has been reported as one of the dominant microbial taxa in human skin (Qiao et al., 2021). The β-diversity plot also showed a distinct cluster of HI-10 samples from the others. Functional studies should be conducted further to untangle the potential relationships between dominant taxa and human skin properties. The α-diversity showed seasonal variation and β-diversity analysis revealed that microbial communities were distinct by season. Moreover, the Jensen-Shannon distance within seasons is significantly closer than between seasons suggests that microbial communities undergo seasonal changes, highlighting the importance of seasonal dynamics in shaping skin microbial composition. Seasonal variations were also observed in skin biophysical parameters, including TEWL, hydration, and elasticity. The relative abundance of Cutibacterium and TEWL was significantly high in winter and low in summer. In particular, our study’s CCA and linear regression result provide clues that can explain the association between skin microbiome and skin biophysical characteristics. The results of CCA analysis demonstrate that TEWL and hydration have more impacts on microbiome dispersion, and the relative abundance of Cutibacterium is correlated with TEWL. Especially, there was a significant association between Cutibacterium and TEWL, even after adjusting for individual differences and weather indicating a dependent relationship.
The epidermal barrier function is known to vary depending on the season, and the difference in the integrity of the skin barrier is mainly caused by epidermal ceramide, which is a major component of the skin barrier (Coderch et al., 2003; Wei et al., 2016; Pappas et al., 2018). The level of epidermal ceramide is widely recognized to be regulated by various factors such as skin microbiome and skin disease (Zheng et al., 2022; Schachner et al., 2023). Several studies have demonstrated that the skin microbiome can have an impact on the physical barrier of the skin (Liu et al., 2020; Harris-Tryon and Grice, 2022). Considering previous studies, we found that the major taxa that fluctuated with the seasons, may regulate the epidermal ceramide levels and subsequently impact the skin barrier function. S. epidermidis, one of the Staphylococcus species, helps maintain skin barrier integrity through the secretion of sphingomyelinase, which is crucial for the production of ceramide (Zheng et al., 2022). C. acnes is known to account for more than 90% of Cutibacterium in human skin (Li et al., 2021) and can partially hydrolyze triglycerides in sebum which acts as a pathway for water diffusion into the epidermis (Riegels-Nielsen et al., 1989; Grice and Segre, 2011; Rocha and Bagatin, 2018; Kobayashi et al., 2019). In addition, we performed functional profiling of the skin microbiome across seasons using KEGG to interpret how the skin microbiome may affect skin barrier function. As a result, it was possible to partially interpret and infer the effect of Cutibacterium, which accounts for more than half of the total individual’s microbiome composition, on the host skin barrier. For instance, porphyrin metabolism was elevated in winter, which is known to be produced by Cutibacterium acnes, can induce oxidative stress and cause skin inflammation (Barnard et al., 2020; Spittaels et al., 2021; Stødkilde et al., 2022). Although the resulting pathways operate at the cellular level and the functional relationship is not clear, the increase in glycosaminoglycan degradation pathway during winter may play an important role in the elevated loss of water content in the epidermal skin. Hyaluronic acid, one of the extracellular matrix components in the epidermis and dermis, is a type of glycosaminoglycan and is known to be degraded by hyaluronate lyase produced by C. acnes (Nazipi et al., 2017; Li et al., 2021; Mayslich et al., 2021). Moreover, a previous anti-acne treatment study has reported improvement in skin barrier function (reduced TEWL) and simultaneously the relative abundance of Cutibacterium (formerly Propionibacterium) tended to decrease (Shao et al., 2023). These results can partially explain the impact of the skin microbiome on skin characteristic such as skin barrier function.
Our study has the advantage of longitudinally observing variations by concurrently collecting skin microbiome and skin biophysical characteristics. This approach enables us to explore the intricate association between skin microbiome and host skin characteristics. Nonetheless, it is imperative to acknowledge the limitations of our study. The composition of the microbiome can be influenced by various factors such as the surrounding environment and host’s lifestyle (Prescott et al., 2017; Moitinho-Silva et al., 2021). While information about environments regarding humidity, and temperature, is available, details about the host’s lifestyle are lacking in our study. Since we consistently collected skin microbial samples and conducted skin measurements in the same facial area, we consider that the aspects related to personal hygiene habits such as mask-wearing and cosmetics were controlled. Specifically, throughout the entire duration of the study, mask-wearing was required and their cosmetic and hygiene routines were maintained consistently. However, to investigate a more systemic relationship between host and skin ecology, we propose the collection of survey data about host lifestyle information. By considering such factors, we anticipate a more comprehensive understanding of the relationship between host skin and skin microbiome.
In conclusion, our long-term study found that there was seasonal variation in skin microbial composition while maintaining an individual’s unique microbiome profile, and a significant association between the abundance of Cutibacterium and skin barrier parameter, TEWL. This study can contributed to a better comprehension of the skin microbiome and its intricate interplay with skin characteristics. Further functional studies on the changes in microbiome composition and skin barrier functions are needed for a deeper understanding of the skin microbiome’s contributions to skin biophysical characteristics.
The datasets presented in this study are deposited in the NCBI repository under accession number PRJNA1034158.
The studies involving humans were approved by the institutional review board at the LG H&H Research Center. The studies were conducted in accordance with the local legislation and institutional requirements. The participants provided their written informed consent to participate in this study.
JS: Conceptualization, Data curation, Investigation, Methodology, Validation, Visualization, Writing – original draft, Writing – review & editing. SY: Conceptualization, Data curation, Formal analysis, Investigation, Methodology, Validation, Visualization, Writing – original draft, Writing – review & editing. K-NG: Conceptualization, Data curation, Formal analysis, Investigation, Methodology, Validation, Visualization, Writing – original draft, Writing – review & editing. HK: Conceptualization, Investigation, Validation, Visualization, Writing – review & editing. J-GS: Conceptualization, Investigation, Methodology, Validation, Writing – original draft, Writing – review & editing. SL: Conceptualization, Formal analysis, Investigation, Methodology, Validation, Writing – original draft, Writing – review & editing. BH: Conceptualization, Methodology, Validation, Writing – review & editing. YK: Conceptualization, Funding acquisition, Project administration, Resources, Supervision, Writing – review & editing. NK: Conceptualization, Funding acquisition, Project administration, Resources, Supervision, Writing – review & editing.
The author(s) declare financial support was received for the research, authorship, and/or publication of this article. This study was supported by a grant funded by LG H&H.
Supplementary Figure S2 was created with BioRender.com (license number MC258I2B6Y).
JS, SY, K-NG, HK, J-GS, SL, BH, YK, and NK were employed by LG H&H Co., Ltd.
All claims expressed in this article are solely those of the authors and do not necessarily represent those of their affiliated organizations, or those of the publisher, the editors and the reviewers. Any product that may be evaluated in this article, or claim that may be made by its manufacturer, is not guaranteed or endorsed by the publisher.
The Supplementary material for this article can be found online at: https://www.frontiersin.org/articles/10.3389/fmicb.2023.1298632/full#supplementary-material
TEWL, transepidermal water loss; CCA, Canonical Correspondence Analysis; PERMANOVA, permutational analysis of variance; KEGG, Kyoto Encyclopedia of Genes and Genomes.
Anderson, M. J. (2001). A new method for non-parametric multivariate analysis of variance. Austral Ecol. 26, 32–46.
Barnard, E., Johnson, T., Ngo, T., Arora, U., Leuterio, G., McDowell, A., et al. (2020). Porphyrin production and regulation in cutaneous propionibacteria. mSphere. 5, e00793–e00719. doi: 10.1128/mSphere.00793-19
Bolyen, E., Rideout, J. R., Dillon, M. R., Bokulich, N. A., Abnet, C. C., Al-Ghalith, G. A., et al. (2019). Reproducible, interactive, scalable and extensible microbiome data science using QIIME 2. Nat. Biotechnol. 37, 852–857. doi: 10.1038/s41587-019-0209-9
Braak, C. J. F. T. (1986). Canonical correspondence analysis: a new eigenvector technique for multivariate direct gradient analysis. Ecology 67, 1167–1179. doi: 10.2307/1938672
Byrd, A. L., Belkaid, Y., and Segre, J. A. (2018). The human skin microbiome. Nat. Rev. Microbiol. 16, 143–155. doi: 10.1038/nrmicro.2017.157
Callahan, B. J., McMurdie, P. J., Rosen, M. J., Han, A. W., Johnson, A. J. A., and Holmes, S. P. (2016). DADA2: high-resolution sample inference from Illumina amplicon data. Nat. Methods 13, 581–583. doi: 10.1038/nmeth.3869
Chiller, K., Selkin, B. A., and Murakawa, G. J. (2001). Skin microflora and bacterial infections of the skin. J. Investig. Dermatol. Symp. Proc. 6, 170–174. doi: 10.1046/j.0022-202x.2001.00043.x
Coderch, L., López, O., de la Maza, A., and Parra, J. L. (2003). Ceramides and skin function. Am. J. Clin. Dermatol. 4, 107–129. doi: 10.2165/00128071-200304020-00004
Cogen, A. L., Yamasaki, K., Sanchez, K. M., Dorschner, R. A., Lai, Y., Mac Leod, D. T., et al. (2010). Selective antimicrobial action is provided by phenol-soluble modulins derived from Staphylococcus epidermidis, a normal resident of the skin. J. Invest. Dermatol. 130, 192–200. doi: 10.1038/jid.2009.243
Dąbrowska, M., and Nowak, I. (2021). Noninvasive evaluation of the influence of aucubin-containing cosmetic macroemulsion on selected skin parameters. J. Cosmet. Dermatol. 20, 1022–1030. doi: 10.1111/jocd.13649
Dolečková, I., Čápová, A., Machková, L., Moravčíková, S., Marešová, M., and Velebný, V. (2021). Seasonal variations in the skin parameters of Caucasian women from Central Europe. Skin Res. Technol. 27, 358–369. doi: 10.1111/srt.12951
Douglas, G. M., Maffei, V. J., Zaneveld, J. R., Yurgel, S. N., Brown, J. R., Taylor, C. M., et al. (2020). PICRUSt2 for prediction of metagenome functions. Nat. Biotechnol. 38, 685–688. doi: 10.1038/s41587-020-0548-6
Dréno, B., Pécastaings, S., Corvec, S., Veraldi, S., Khammari, A., and Roques, C. (2018). Cutibacterium acnes (Propionibacterium acnes) and acne vulgaris: a brief look at the latest updates. J. Eur. Acad. Dermatol. Venereol. 32, 5–14. doi: 10.1111/jdv.15043
Duncan, W. C., McBride, M. E., and Knox, J. M. (1969). Bacterial flora. The role of environmental factors. J. Invest. Dermatol. 52, 479–484. doi: 10.1038/jid.1969.81
Fadrosh, D. W., Ma, B., Gajer, P., Sengamalay, N., Ott, S., Brotman, R. M., et al. (2014). An improved dual-indexing approach for multiplexed 16S rRNA gene sequencing on the Illumina MiSeq platform. Microbiome 2:6. doi: 10.1186/2049-2618-2-6
Fierer, N., Hamady, M., Lauber, C. L., and Knight, R. (2008). The influence of sex, handedness, and washing on the diversity of hand surface bacteria. Proc. Natl. Acad. Sci. U. S. A. 105, 17994–17999. doi: 10.1073/pnas.0807920105
Fitz-Gibbon, S., Tomida, S., Chiu, B. H., Nguyen, L., Du, C., Liu, M., et al. (2013). Propionibacterium acnes strain populations in the human skin microbiome associated with acne. J. Invest. Dermatol. 133, 2152–2160. doi: 10.1038/jid.2013.21
Flores, G. E., Caporaso, J. G., Henley, J. B., Rideout, J. R., Domogala, D., Chase, J., et al. (2014). Temporal variability is a personalized feature of the human microbiome. Genome Biol. 15:531. doi: 10.1186/s13059-014-0531-y
Fredricks, D. N. (2001). Microbial ecology of human skin in health and disease. J. Investig. Dermatol. Symp. Proc. 6, 167–169. doi: 10.1046/j.0022-202x.2001.00039.x
Gallo, R. L., and Nakatsuji, T. (2011). Microbial symbiosis with the innate immune defense system of the skin. J. Invest. Dermatol. 131, 1974–1980. doi: 10.1038/jid.2011.182
Gardien, K. L. M., Baas, D. C., de Vet, H. C. W., and Middelkoop, E. (2016). Transepidermal water loss measured with the Tewameter TM300 in burn scars. Burns 42, 1455–1462. doi: 10.1016/j.burns.2016.04.018
Grice, E. A., Kong, H. H., Conlan, S., Deming, C. B., Davis, J., Young, A. C., et al. (2009). Topographical and temporal diversity of the human skin microbiome. Science 324, 1190–1192. doi: 10.1126/science.1171700
Grice, E. A., and Segre, J. A. (2011). The skin microbiome. Nat. Rev. Microbiol. 9, 244–253. doi: 10.1038/nrmicro2537
Harris-Tryon, T. A., and Grice, E. A. (2022). Microbiota and maintenance of skin barrier function. Science 376, 940–945. doi: 10.1126/science.abo0693
Hillebrand, G. G., Dimitriu, P., Malik, K., Park, Y., Qu, D., Mohn, W. W., et al. (2021). Temporal variation of the facial skin microbiome: a 2-year longitudinal study in healthy adults. Plast. Reconstr. Surg. 147, 50S–61S. doi: 10.1097/PRS.0000000000007621
Isler, M. F., Coates, S. J., and Boos, M. D. (2023). Climate change, the cutaneous microbiome and skin disease: implications for a warming world. Int. J. Dermatol. 62, 337–345. doi: 10.1111/ijd.16297
Kanehisa, M., Goto, S., Kawashima, S., Okuno, Y., and Hattori, M. (2004). The KEGG resource for deciphering the genome. Nucleic Acids Res. 32, 277D–2280D. doi: 10.1093/nar/gkh063
Kanehisa, M., Goto, S., Sato, Y., Furumichi, M., and Tanabe, M. (2012). KEGG for integration and interpretation of large-scale molecular data sets. Nucleic Acids Res. 40, D109–D114. doi: 10.1093/nar/gkr988
Kechin, A., Boyarskikh, U., Kel, A., and Filipenko, M. (2017). Cut primers: a new tool for accurate cutting of primers from reads of targeted next generation Sequencing. J. Comput. Biol. 24, 1138–1143. doi: 10.1089/cmb.2017.0096
Kobayashi, T., Voisin, B., Kim, D. Y., Kennedy, E. A., Jo, J. H., Shih, H. Y., et al. (2019). Homeostatic control of sebaceous glands by innate lymphoid cells regulates commensal bacteria equilibrium. Cells 176, 982–997.e16. doi: 10.1016/j.cell.2018.12.031
Lahti, L, and Shetty, S. (2017). Microbiome R package. Available at: https://doi.org/10.18129/B9.bioc.microbiome
Leeming, J. P., Holland, K. T., and Cunliffe, W. J. (1984). The microbial ecology of pilosebaceous units isolated from human skin. J. Gen. Microbiol. 130, 803–807. doi: 10.1099/00221287-130-4-803
Lesnik, R. H., Kligman, L. H., and Kligman, A. M. (1992). Agents that cause enlargement of sebaceous glands in hairless mice. Arch. Dermatol. Res. 284, 106–108. doi: 10.1007/BF00373379
Li, Z., Xia, J., Jiang, L., Tan, Y., An, Y., Zhu, X., et al. (2021). Characterization of the human skin resistome and identification of two microbiota cutotypes. Microbiome. 9:47. doi: 10.1186/s40168-020-00995-7
Lin, J. (1991). Divergence measures based on the Shannon entropy. IEEE Trans. Inf. Theory 37, 145–151. doi: 10.1109/18.61115
Liu, Y., Wang, S., Dai, W., Liang, Y., Shen, C., Li, Y., et al. (2020). Distinct skin microbiota imbalance and responses to clinical treatment in children with atopic dermatitis. Front. Cell. Infect. Microbiol. 10:336. doi: 10.3389/fcimb.2020.00336
Mayslich, C., Grange, P. A., and Dupin, N. (2021). Cutibacterium acnes as an opportunistic pathogen: an update of its virulence-associated factors. Microorganisms 9:303. doi: 10.3390/microorganisms9020303
McMurdie, P. J., and Holmes, S. (2013). phyloseq: an R package for reproducible interactive analysis and graphics of microbiome census data. PLoS One 8:e61217. doi: 10.1371/journal.pone.0061217
Moitinho-Silva, L., Boraczynski, N., Emmert, H., Baurecht, H., Szymczak, S., Schulz, H., et al. (2021). Host traits, lifestyle and environment are associated with human skin bacteria. Br. J. Dermatol. 185, 573–584. doi: 10.1111/bjd.20072
Nam, G. W., Baek, J. H., Koh, J. S., and Hwang, J. K. (2015). The seasonal variation in skin hydration, sebum, scaliness, brightness and elasticity in Korean females. Skin Res. Technol. 21, 1–8. doi: 10.1111/srt.12145
Nazipi, S., Stødkilde-Jørgensen, K., Scavenius, C., and Brüggemann, H. (2017). The skin bacterium Propionibacterium acnes employs two variants of hyaluronate lyase with distinct properties. Microorganisms 5:57. doi: 10.3390/microorganisms5030057
Oh, J., Byrd, A. L., Park, M., Comparative Sequencing Program, N. I. S. C., Kong, H. H., and Segre, J. A. (2016). Temporal stability of the human skin microbiome. Cells 165, 854–866. doi: 10.1016/j.cell.2016.04.008
Ohshima, H., Kinoshita, S., Oyobikawa, M., Futagawa, M., Takiwaki, H., Ishiko, A., et al. (2013). Use of Cutometer area parameters in evaluating age-related changes in the skin elasticity of the cheek. Skin Res. Technol. 19, e238–e242. doi: 10.1111/j.1600-0846.2012.00634.x
Oksanen, J, Simpson, GL, Blanchet, FG, Kindt, R, Legendre, P, Minchin, PR, et al. vegan: community ecology package [Internet]. (2022) Available at: https://cran.r-project.org/web/packages/vegan/ (Accessed September 12, 2023).
Olszewski, T. D. (2004). A unified mathematical framework for the measurement of richness and evenness within and among multiple communities. Oikos 104, 377–387. doi: 10.1111/j.0030-1299.2004.12519.x
Pappas, A., Kendall, A. C., Brownbridge, L. C., Batchvarova, N., and Nicolaou, A. (2018). Seasonal changes in epidermal ceramides are linked to impaired barrier function in acne patients. Exp. Dermatol. 27, 833–836. doi: 10.1111/exd.13499
Pearse, A. D., Gaskell, S. A., and Marks, R. (1987). Epidermal changes in human skin following irradiation with either UVB or UVA. J. Invest. Dermatol. 88, 83–87. doi: 10.1111/1523-1747.ep12465094
Prescott, S. L., Larcombe, D. L., Logan, A. C., West, C., Burks, W., Caraballo, L., et al. (2017). The skin microbiome: impact of modern environments on skin ecology, barrier integrity, and systemic immune programming. World Allergy Organ J. 10:29. doi: 10.1186/s40413-017-0160-5
Qiao, Z., Huang, S., Leng, F., Bei, Y., Chen, Y., Chen, M., et al. (2021). Analysis of the bacterial flora of sensitive facial skin among women in Guangzhou. Clin. Cosmet. Investig. Dermatol. 14, 655–664. doi: 10.2147/CCID.S307668
Riegels-Nielsen, P., Frimodt-Møller, N., Sørensen, M., and Jensen, J. S. (1989). Antibiotic treatment insufficient for established septic arthritis. Staphylococcus aureus Experiments in rabbits. Acta Orthop. Scand. 60, 113–115. doi: 10.3109/17453678909150107
Rocha, M. A., and Bagatin, E. (2018). Skin barrier and microbiome in acne. Arch. Dermatol. Res. 310, 181–185. doi: 10.1007/s00403-017-1795-3
Schachner, L. A., Alexis, A. F., Andriessen, A., Berson, D., Gold, M., Goldberg, D. J., et al. (2023). Insights into acne and the skin barrier: optimizing treatment regimens with ceramide-containing skincare. J. Cosmet. Dermatol. 22, 2902–2909. doi: 10.1111/jocd.15946
Schmid, B., Künstner, A., Fähnrich, A., Busch, H., Glatz, M., and Bosshard, P. P. (2022). Longitudinal characterization of the fungal skin microbiota in healthy subjects over a period of 1 year. J. Invest. Dermatol. 142, 2766–2772.e8. doi: 10.1016/j.jid.2022.03.014
Schommer, N. N., and Gallo, R. L. (2013). Structure and function of the human skin microbiome. Trends Microbiol. 21, 660–668. doi: 10.1016/j.tim.2013.10.001
Shao, X., Chen, Y., Zhang, L., Zhang, Y., Ariyawati, A., Chen, T., et al. (2023). Effect of 30% supramolecular salicylic acid peel on skin microbiota and inflammation in patients with moderate-to-severe acne vulgaris. Dermatol Ther (Heidelb). 13, 155–168. doi: 10.1007/s13555-022-00844-5
Somerville, D. A. (1969). The normal flora of the skin in different age groups. Br. J. Dermatol. 81, 248–258. doi: 10.1111/j.1365-2133.1969.tb13976.x
Spittaels, K. J., van Uytfanghe, K., Zouboulis, C. C., Stove, C., Crabbé, A., and Coenye, T. (2021). Porphyrins produced by acneic Cutibacterium acnes strains activate the inflammasome by inducing K+ leakage. iScience 24:102575. doi: 10.1016/j.isci.2021.102575
Stødkilde, K., Nielsen, J. T., Petersen, S. V., Paetzold, B., Brüggemann, H., Mulder, F. A. A., et al. (2022). Solution structure of the Cutibacterium acnes-specific protein Rox P and insights into its antioxidant activity. Front. Cell. Infect. Microbiol. 12:803004. doi: 10.3389/fcimb.2022.803004
Uberoi, A., Bartow-McKenney, C., Zheng, Q., Flowers, L., Campbell, A., Knight, S. A. B., et al. (2021). Commensal microbiota regulates skin barrier function and repair via signaling through the aryl hydrocarbon receptor. Cell Host Microbe 29, 1235–1248.e8. doi: 10.1016/j.chom.2021.05.011
Wei, K. S., Stella, C., Wehmeyer, K. R., Christman, J., Altemeier, A., Spruell, R., et al. (2016). Effects of season stratum corneum barrier function and skin biomarkers. J. Cosmet. Sci. 67, 185–203.
Youn, S. W., Na, J. I., Choi, S. Y., Huh, C. H., and Park, K. C. (2005). Regional and seasonal variations in facial sebum secretions: a proposal for the definition of combination skin type. Skin Res. Technol. 11, 189–195. doi: 10.1111/j.1600-0846.2005.00119.x
Keywords: skin microbiome, TEWL, skin biophysical characteristics, Cutibacterium, Corynebacterium, Staphylococcus, Neisseriaceae, Streptococcus
Citation: Seo JY, You SW, Gu K-N, Kim H, Shin J-G, Leem S, Hwang BK, Kim Y and Kang NG (2023) Longitudinal study of the interplay between the skin barrier and facial microbiome over 1 year. Front. Microbiol. 14:1298632. doi: 10.3389/fmicb.2023.1298632
Received: 22 September 2023; Accepted: 30 October 2023;
Published: 16 November 2023.
Edited by:
Jianmin Chai, Foshan University, ChinaReviewed by:
Mi-Ju Kim, Kyung Hee University, Republic of KoreaCopyright © 2023 Seo, You, Gu, Kim, Shin, Leem, Hwang, Kim and Kang. This is an open-access article distributed under the terms of the Creative Commons Attribution License (CC BY). The use, distribution or reproduction in other forums is permitted, provided the original author(s) and the copyright owner(s) are credited and that the original publication in this journal is cited, in accordance with accepted academic practice. No use, distribution or reproduction is permitted which does not comply with these terms.
*Correspondence: Yunkwan Kim, a2lteW9vbmt3YW5AbGdobmguY29t; Nae Gyu Kang, bmdrYW5nQGxnaG5oLmNvbQ==
†These authors have contributed equally to this work and share first authorship
‡ORCID: Jung Yeon Seo, https://orcid.org/0000-0003-2016-2974
Seung Won You, https://orcid.org/0000-0003-3786-418X
Ki-Nam Gu, https://orcid.org/0000-0002-3445-644X
Hanji Kim, https://orcid.org/0000-0001-9635-6084
Joong-Gon Shin, https://orcid.org/0000-0002-7435-0463
Sangseob Leem, https://orcid.org/0000-0001-9911-9279
Bo Kyoung Hwang, https://orcid.org/0009-0009-2080-2602
Yunkwan Kim, https://orcid.org/0000-0003-1353-4784
Nae Gyu Kang, https://orcid.org/0000-0002-3318-3873
Disclaimer: All claims expressed in this article are solely those of the authors and do not necessarily represent those of their affiliated organizations, or those of the publisher, the editors and the reviewers. Any product that may be evaluated in this article or claim that may be made by its manufacturer is not guaranteed or endorsed by the publisher.
Research integrity at Frontiers
Learn more about the work of our research integrity team to safeguard the quality of each article we publish.