- 1Faculty of Chemistry and Earth Sciences, Institute for Inorganic and Analytical Chemistry, Friedrich Schiller University Jena, Jena, Germany
- 2Cluster of Excellence Balance of the Microverse, Friedrich Schiller University Jena, Jena, Germany
- 3iDiv - German Centre for Integrative Biodiversity Research, Halle-Jena-Leipzig, Leipzig, Germany
- 4Geobotany and Botanical Gardens, Martin-Luther University of Halle-Wittenberg, Halle, Germany
- 5Institute of Plant Biochemistry, Leibniz Institute of Plant Biochemistry, Halle, Germany
- 6Pharmaceuticals Division, Research & Development, Data Science and Artificial Intelligence, AG Bayer, Berlin, Germany
Diatoms (Bacillariophyceae) are aquatic photosynthetic microalgae with an ecological role as primary producers in the aquatic food web. They account substantially for global carbon, nitrogen, and silicon cycling. Elucidating the chemical space of diatoms is crucial to understanding their physiology and ecology. To expand the known chemical space of a cosmopolitan marine diatom, Skeletonema marinoi, we performed High-Resolution Liquid Chromatography-Tandem Mass Spectrometry (LC-MS2) for untargeted metabolomics data acquisition. The spectral data from LC-MS2 was used as input for the Metabolome Annotation Workflow (MAW) to obtain putative annotations for all measured features. A suspect list of metabolites previously identified in the Skeletonema spp. was generated to verify the results. These known metabolites were then added to the putative candidate list from LC-MS2 data to represent an expanded catalog of 1970 metabolites estimated to be produced by S. marinoi. The most prevalent chemical superclasses, based on the ChemONT ontology in this expanded dataset, were organic acids and derivatives, organoheterocyclic compounds, lipids and lipid-like molecules, and organic oxygen compounds. The metabolic profile from this study can aid the bioprospecting of marine microalgae for medicine, biofuel production, agriculture, and environmental conservation. The proposed analysis can be applicable for assessing the chemical space of other microalgae, which can also provide molecular insights into the interaction between marine organisms and their role in the functioning of ecosystems.
1 Introduction
Unicellular microalgae, forming the phytoplankton, are primary producers in the marine food web, contributing to biodiversity and affecting the biogeochemical cycles, particularly the oceanic carbon cycling (Field et al., 1998; Litchman et al., 2015; Qian et al., 2016). Among the phytoplankton community, diatoms (Bacillariophyceae) are found in the euphotic layer of the ocean and have the largest contribution to the aquatic biosphere (Malviya et al., 2016). These eukaryotic microalgae survive under extreme environmental conditions such as varying light, temperature, nutrition, and salinity. They produce unique natural products (NP) with distinct biological activities displaying various chemical classes (Stonik and Stonik, 2015). As the primary producers, diatoms serve as a substantial biological source that can generate diverse natural products with applications in various NP-driven industries, such as food technology, cosmetics, biofuel, nanotechnology, and drug discovery (Lordan et al., 2011; Hildebrand et al., 2012; Martins et al., 2014; Khan et al., n.d.). However, the marine diatom community remains a large reservoir of unknown natural products (Nieri et al., 2023).
To expand the record of identified marine natural products or metabolites, we used Skeletonema marinoi (NCBI Taxonomy ID: 267567) as a model organism for diatoms. The strain used was taxonomically revised from Skeletonema costatum (Sarno et al., 2005). S. marinoi is a marine unicellular, centric diatom that lives in chain-like colonies consisting of up to 30 cells, with 1–2 chloroplasts in each cell located near the siliceous cell wall or frustule (Sarno et al., 2005). Skeletonema marinoi is considered a non-toxic species and generally safe (Silva et al., 2022). S. marinoi has been evolutionarily successful, and its genome has been able to encode certain bacterial, plant, and animal-like metabolic pathways (Di Dato et al., 2017). It can also adapt to varying environmental conditions (Milito et al., 2020). Given the optimal availability of nutrients, S. marinoi produces massive phytoplankton algal blooms in the coastal regions (Godhe and Härnström, 2010), releasing oxylipins that act as toxins for predators and other phytoplankton members (Fontana et al., 2007b; Ribalet et al., 2007). Although much effort has been made toward the chemical characterization of lipids in S. marinoi, as they impact growth, reproduction, stress response, and defense mechanism in the diatoms (Rousch et al., 2003; Fontana et al., 2007a; Popovich et al., 2012; Sayanova et al., 2017), much of its metabolome is still unexplored compared to other model species.
Mass spectrometry (MS)-based metabolomics is the key technology for detecting these secondary metabolites and covers the analysis of compounds with distinct structural heterogeneity (Bauermeister et al., 2021). In this study, we performed the metabolome annotation of the mass spectrometry data obtained from S. marinoi to comprehend the chemical composition of the primary and specialized metabolites produced by the diatom. We annotated molecular structures, chemical classes, and molecular formulae to MS features obtained with High-resolution Liquid Chromatography-Electrospray Ionization-Tandem Mass Spectrometry (LC-ESI-Orbitrap MS2) using Metabolome Annotation Workflow (MAW) (Zulfiqar et al., 2023). The findings of this study contribute to the expansion of known marine natural products derived from S. marinoi.
2 Methods and materials
2.1 Suspect list development and curation
For this study (Zulfiqar, 2022, 2023a,b), a suspect list was generated manually from different databases and literary resources (not considering the extraction and acquisition methods) to represent a collection of known metabolites produced by Skeletonema marinoi and its phylogenetically closely related species, Skeletonema costatum. The list contained primary metabolites, as well as the secondary metabolites that have been reported in S. marinoi and S. costatum. The 893 compounds in the list were collected and assembled from databases such as KEGG (Kanehisa and Goto, 2000; Kanehisa, 2019; Kanehisa et al., 2023), ChEBI (Hastings et al., 2016), PubChem (Kim et al., 2021), MetaCyc (Caspi et al., 2014), UniProtKB (The UniProt Consortium, 2021), BRENDA (Chang et al., 2021), LOTUS (Rutz et al., 2022), and several publications (Derenbach and Pesando, 1986; Pennington et al., 1988; Brown and Jeffrey, 1995; Anning et al., 2000; Ianora et al., 2004; Fontana et al., 2007a; Ribalet et al., 2007; Snoeijs et al., 2011; Vidoudez and Pohnert, 2012; Lauritano et al., 2015; Orefice et al., 2016; Di Dato et al., 2017; Amato et al., 2018; Jing et al., 2019; Smerilli et al., 2019; Cardoso et al., 2020; Gallo et al., 2020; Russo et al., 2021; Nikitashina et al., 2022). For curation and additional metadata on each entry, PubChemPy (Swain, n.d.) and RDKit (Landrum, 2016) were used by adding any missing synonyms, IUPAC names, and molecular weights. Entries with no identifier or structural notation were discarded. Lastly, pybatchclassyfire (Allard, 2020) was used to add classification based on ChemONT 2.1 (subclass, class, superclass) to the suspect list using SMILES (Djoumbou Feunang et al., 2016).
2.2 Sample preparation and metabolite extraction
For the whole metabolome extraction from LC-MS samples, methanol, ethanol, chloroform, acetonitrile, and water were used as reagents. S. marinoi RCC 75 strain used in this study was obtained from Roscoff Culture Collection (Roscoff, France). These cultures were grown in 40 mL artificial seawater (ASW) inside 50 mL cell culture flasks. The cultures were kept at the optimum conditions for the growth of this strain under a standard temperature of 13°C with 55–65 μmol photons m−2 s−1 lighting, keeping the 14:10 h light:dark regime. The cultures were incubated in a shaking incubator at 80 rpm. Cultures were collected on Whatman GF/C filters with 1.2 μm pore size (GE Healthcare, US) under vacuum (750 mbar). These conditions provide a filtering speed of ~1.5 mL/min. The filters were then submerged in an ice-cold extraction mixture of methanol:ethanol:chloroform, 1:3:1, v:v:v. Samples were ultrasonicated for 10 min, and the solutions were transferred to new Eppendorf tubes without the filters. The Eppendorf tubes with the solutions were then centrifuged at 30,000 g at 4°C for 15 min. The aliquots for LC-MS analyses were separated and evaporated using a vacuum. Samples with aliquots that contained 3×10E6 cells were prepared. Media blanks included the average volume for the culture's samples. The volumes varied from 25 to 40 μL depending on cell number in the cultures. The dried samples were stored in argon at −24°C. For LC-MS analysis, the dried samples were dissolved in 150 μL of the mixture of methanol:acetonitrile:water, 5:9:1, v:v:v.
2.3 LC-MS2 measurement
Samples separation with chromatography was performed on the SeQuant ZIC-HILIC column (5 μm, 200 Å, 150 × 2.1 mm, Merck, Germany) with a flow rate of 0.6 mL/min. The samples were measured with the Dionex Ultimate 3000 system coupled to a Q-Exactive Plus Orbitrap mass spectrometer (Thermo Scientific, Bremen, Germany). Using electrospray ionization, the molecular ions were obtained in both positive and negative modes, with a scan range of 80–1,200 m/z. The duration of the method was 12.5 min, and MS runtime ranged from 0.5–9 min. The separation was performed on the SeQuant ZIC-HILIC column (5 μm, 200 Å, 150 × 2.1 mm, Merck, Germany), equipped with SeQuant ZIC-HILIC Guard (20 × 2.1 mm, Merck, Germany). Solution A consisted of water with 2% acetonitrile and 0.1% formic acid, and solution B consisted of 90% acetonitrile with 10% water and 1 mmol/L-1 ammonium acetate. The flow rate was set to 0.6 mL/min-1. The sample was run through a solvent mixture comprising of 15% solvent A for a duration of 4 min. Afterward, the solvent was gradually increased to 100% solvent A at 5 min and was maintained at this level until 7 min. Following this, solvent B was gradually increased to 100% until 8 min and held at this level until 10 minutes. Finally, the solvent was gradually decreased to 15% solvent A at 10.5 min and maintained at this level until 12.5 min.
MS1 measurements were conducted with the following settings: automatic gain control target−3E6, the maximum ion injection time−200 ms, and scan range from 80 to 1,200 m/z, resolution 70,000. MS2 had the following settings: automatic gain control target 2E5, maximum ion injection time 100 ms, three-stepped normalized collision energy 15, 30, 45, and scan range from 80 to 1,200 m/z, resolution 70,000. MS1 and MS2 measurements were performed in parallel. A target list of all detected compounds was created with Compound Discoverer (Thermo Fisher Scientific) and was manually separated into 20 inclusion lists. We used retention time-based PRM and defined a narrow retention time window of 0.2 min for each feature in the inclusion list. All MS2 spectra were acquired within 20 consecutive injections of the same sample.
2.4 Metabolic profiling with Metabolome Annotation Workflow
The RAW MS files obtained from the Orbitrap Mass Spectrometer were preprocessed using Compound Discoverer. The standard workflow (Untargeted Metabolomics with Statistics Detect Unknowns with ID Using Online Databases and mzLogic) was used with default options (Zulfiqar and Schmidt, 2021; Zulfiqar and Stettin, 2023a,b). The RAW MS2 files were converted to .mzML using Proteowizard MS-Convert Suite (Chambers et al., 2012). MAW (version 1.1) was used for dereplication against spectral and compound databases. For the spectral database dereplication, the input query spectra were matched against the experimental spectra from GNPS (Wang et al., 2016), Massbank (Horai et al., 2010), and HMDB (Wishart et al., 2007, 2018). For the compound database dereplication, we used SIRIUS4 (version 4.9.12) (Dührkop et al., 2015, 2019). SIRIUS performed the dereplication against the database “ALL,” which refers to all databases integrated within the software. CANOPUS (Dührkop et al., 2021) and ClassyFire (Djoumbou Feunang et al., 2016) predicted the chemical class of the candidates. The candidate selection module was used to list the most probable (high-scoring) candidates from spectral databases and SIRIUS. The sunburst function generated sunburst diagrams based on the chemical classes of top-ranking candidates. Figure 1 explains the untargeted approach to extracting the whole metabolome of the diatom and the application of MAW on the annotation of metabolites from the whole metabolome.
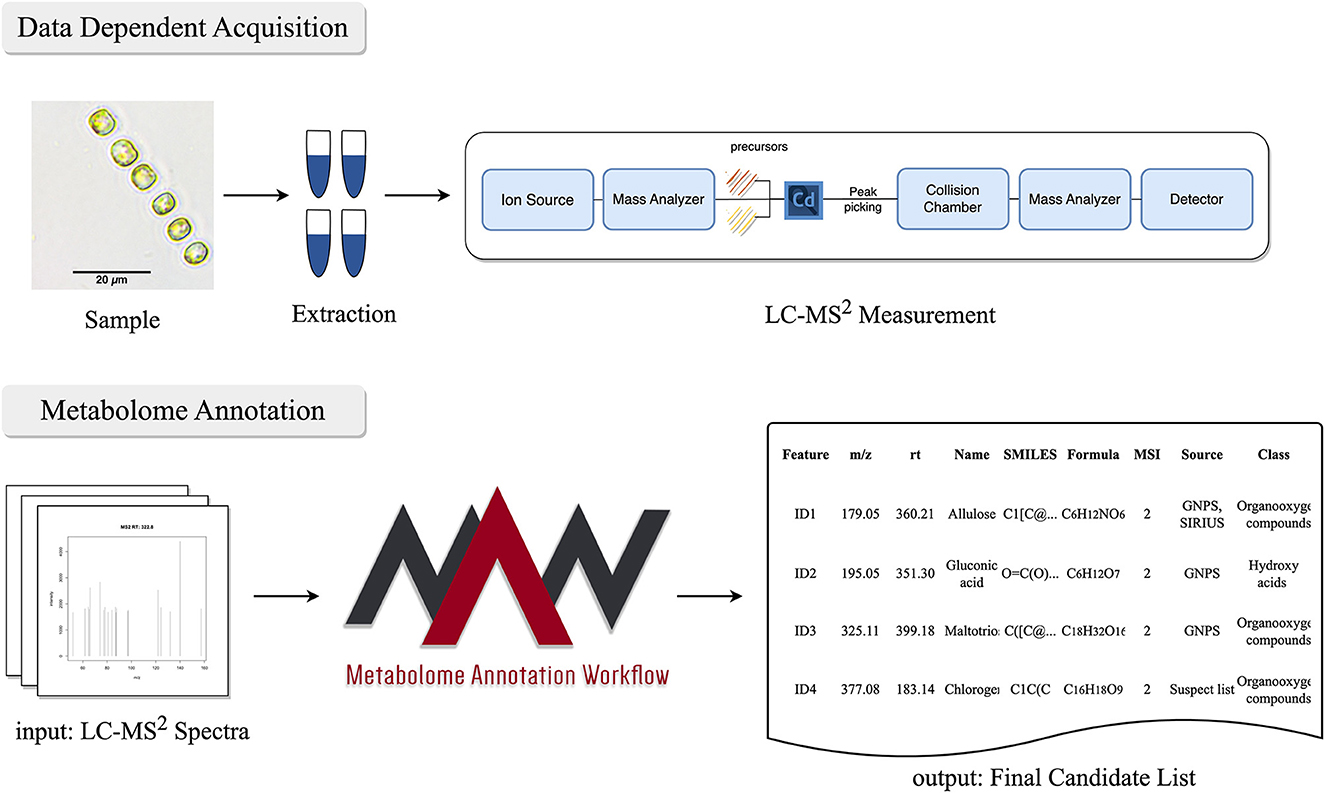
Figure 1. Workflow for untargeted mass spectrometry approach to acquire the metabolome from Skeletonema marinoi. After the sample collection and metabolic extraction, LC-MS2 measurements are performed using an orbitrap mass spectrometer. The data obtained from the LC-MS2 is then subjected to MAW, which performs the annotation of metabolites and generates a final list of metabolites extracted from the samples.
3 Results
3.1 Suspect list of known S. marinoi metabolites
A suspect list of known metabolites produced by S. marinoi and the closely related S. costatum was created using different databases and literature articles, described in the methodology section, for the purpose of dereplication of known metabolites annotated from the MS2 data acquired from the precursor masses (m/z) in the inclusion lists. The curated suspect list contained names, formulae, species, SMILES, InChIs, monoisotopic masses, PubChem identifiers, source databases and references, IUPAC names, synonyms, subclasses, classes, and superclasses of 893 compounds. For the classification of these metabolites, ClassyFire (Djoumbou Feunang et al., 2016) was used, which annotated 851 compounds from the suspect list. ClassyFire uses ChemONT, which is a structure-based taxonomy system used to annotate chemical compounds to a hierarchical system of classification. Metabolites belong to the class of organic compounds and can be categorized into more detailed superclasses (generic), classes (specific), and subclasses (highly specific). The most abundant superclasses in the suspect list, according to ChemONT, were organoheterocyclic compounds, organic acids and derivatives, organic oxygen compounds, and lipids and lipid-like molecules (see Supplementary Figure 1).
To comprehend the structural similarity among the known metabolites from the suspect list, we clustered together the chemically similar compounds from the abundant superclasses into a network (shown in Supplementary Figure 2). This chemical similarity network visually represents the structural similarity between different compounds, which can reveal joint functional regulation. A group of compounds in a cluster can represent a biochemical pathway or otherwise connected regulations. The chemical similarity network was created using an “all vs. all” approach, where the SMILES of all chemical structures in the suspect list were converted to Morgan Fingerprints (Morgan, 1965; Rogers and Hahn, 2010) and then were compared against one another using a Tanimoto similarity score, with a given threshold of ≥0.85. This calculation was carried out with RDKit (Landrum, 2016) using the MAW-defined function ChemMN (https://github.com/zmahnoor14/MAW/blob/main/cwl/Workflow_Python_Script_all.py#L2948). The chemical superclass with the highest internal similarity in the suspect list was the lipids and lipid-like molecules, exhibiting the polyunsaturated fatty acids (PUFA), polyunsaturated aldehydes (PUA), and precursor molecules such as acetyl CoA and malonyl CoA which drive the citric acid cycle and fatty acid biosynthesis (Kelly and Hughes, 2001). Organoheterocyclic compounds were also highly interconnected, representing different tetrapyrroles, such as chlorophyll A. The other two superclasses were amino acid derivatives and coenzymes and organic oxygen compounds belonging to polyphenols.
3.2 Structure annotation and classification of tandem mass spectrometry data from S. marinoi
To broaden the known chemical space of S. marinoi, we acquired untargeted metabolomics data from S. marinoi samples using High-Resolution Liquid Chromatography-Tandem Mass Spectrometry (HR-LC-MS2). The data was measured in both positive and negative modes, which resulted in 1,014 and 839 features, respectively, making a total of 1,853 distinct metabolic features with unique precursor masses (m/z) and retention time (seconds), out of which 1,153 were identified as unique metabolites. This difference in unique features and unique compounds can be attributed to the presence of various isotopes, adducts, and fragments from the same compound (Lu et al., 2020). The Metabolome Annotation Workflow was used to perform dereplication using spectral databases [GNPS (Wang et al., 2016), Massbank (Horai et al., 2010), and HMDB (Wishart et al., 2007, 2018)], and compound databases integrated with SIRIUS4 (Dührkop et al., 2015, 2019). The results were post-processed, and each distinct feature was assigned a list of putative structures with Metabolomics Standards Initiative (MSI) confidence levels of identification (Sumner et al., 2007; Schymanski et al., 2014; Zulfiqar et al., 2023). The standards used for this dataset included betaine, pipecolinic acid, cysteinolic acid, methionine sulfoxide, N,N-dimethylarginine, O-acetyl-L-carnitine, O-propanoyl-L-carnitine, O-butanoyl-L-carnitine, and isovalerylcarnitine, were annotated using MAW and were assigned MSI level 1 in the workflow article (Zulfiqar et al., 2023). Table 1 shows the number of unique features annotated within each MSI level.
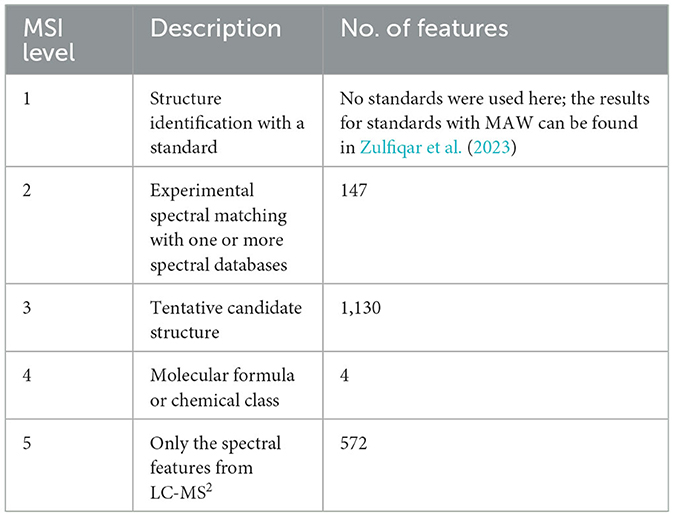
Table 1. Number of features from the LC-MS2 data of S. marinoi, annotated with MAW, divided into different MSI levels.
To assess the metabolic profile of S. marinoi from LC-MS2 data, the high-scoring candidates with MSI levels 2 and 3 were analyzed. The annotations contained both primary metabolites, such as amino acids, and their derivatives (pipecolic acid, ornithine, carnitine), nucleic acids and analogs (adenosine, guanosine, cyclic AMP), carbohydrates (alpha-lactose and glucose-1-phosphate), fatty acids (eicosapentaenoic acid, myristic acid, stearic acid), and different other organic acids (gluconic acid, chlorogenic acid, and ascorbic acid). The composition of compound superclasses, classes, and subclasses in this experimental dataset is represented by a sunburst plot in Supplementary Figure 3. A total of 1,036 annotations were assigned a chemical superclass using CANOPUS (Dührkop et al., 2021) and ClassyFire. This dataset shows the same abundant superclasses as the suspect list.
3.3 Expansion of known metabolome of S. marinoi
Lastly, the putative candidate list from the LC-MS2 data was compared with the suspect list to extract metabolites that were common in both datasets with a Tanimoto similarity score of 1, which led to 57 common compounds. The most prevalent class was carboxylic acids and derivatives, particularly amino acids and analogs, as most of the common metabolites were primary metabolites (Supplementary Figure 4). The compounds from the suspect list and LC-MS2 data were then combined into a single union set of metabolites, which resulted in 1997 compounds. Manual curation was performed to remove expectedly misannotated metabolites originating from plant, fungal, or animal origins, which then reduced the list to 1970 compounds that represented the expanded chemical space of compounds produced by S. marinoi. Figure 2 represents a sunburst plot of the chemical classification of 1,691 compounds from this expanded chemical space of compounds from S. marinoi.
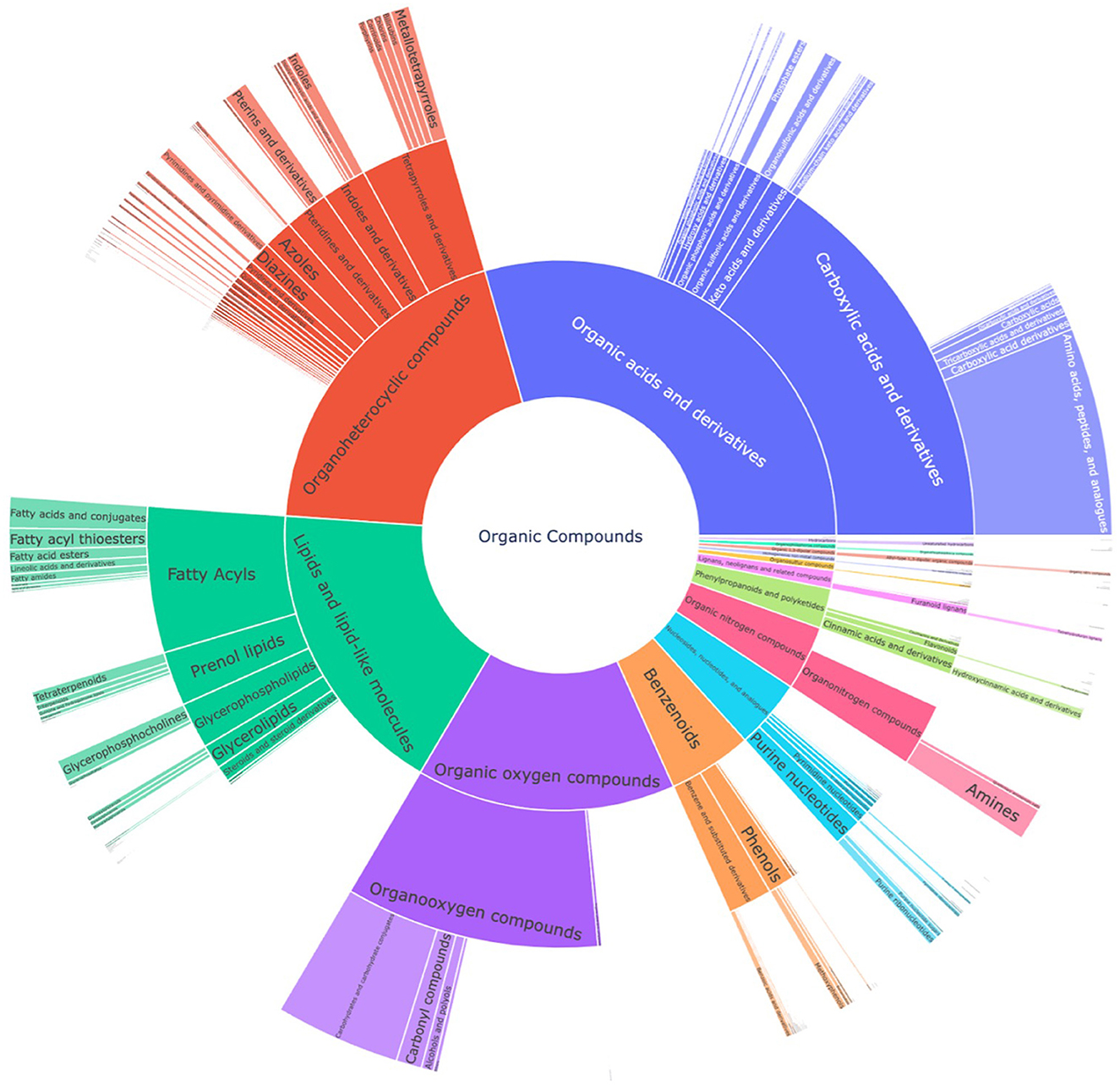
Figure 2. Sunburst plot for chemical classification of the union set from the suspect list and the LC-MS2 data from S. marinoi, which shows the expanded known metabolome of the diatom. The innermost section of the kingdom of organic compounds is classified into superclasses, classes, and subclasses. The most prevalent superclasses are organic acids and derivatives (28.1%), organoheterocyclic compounds (20.3%), lipids and lipid-like molecules (17.5%), and organic oxygen compounds (14.5%).
The metabolites from the LC-MS2 data were incorporated into the chemical similarity network of compounds from the suspect list, shown in Supplementary Figure 2, to demonstrate the expansion in the chemical similarity, which indicated the metabolites previously not reported or missing from the chemical similarity network or the known metabolic pathways of the diatom (as depicted in Figure 3). The hexagonal-shaped metabolite nodes in Figure 3 represent the putative candidates from the LC-MS2 data. Figure 3 shows that the lipids and lipid-like molecules superclass had again the largest expansion representing newly added metabolites such as saturated oxo-fatty acid (SOFAs), fatty acid amides (FAAs), and glycerophospholipids. The organic oxygen compounds superclass expanded with building blocks of polysaccharides for the diatom cell wall. Cyanodecanoic and cyanododecanoic acids were added to the chemical similarity network of the organic acids and derivatives superclass.
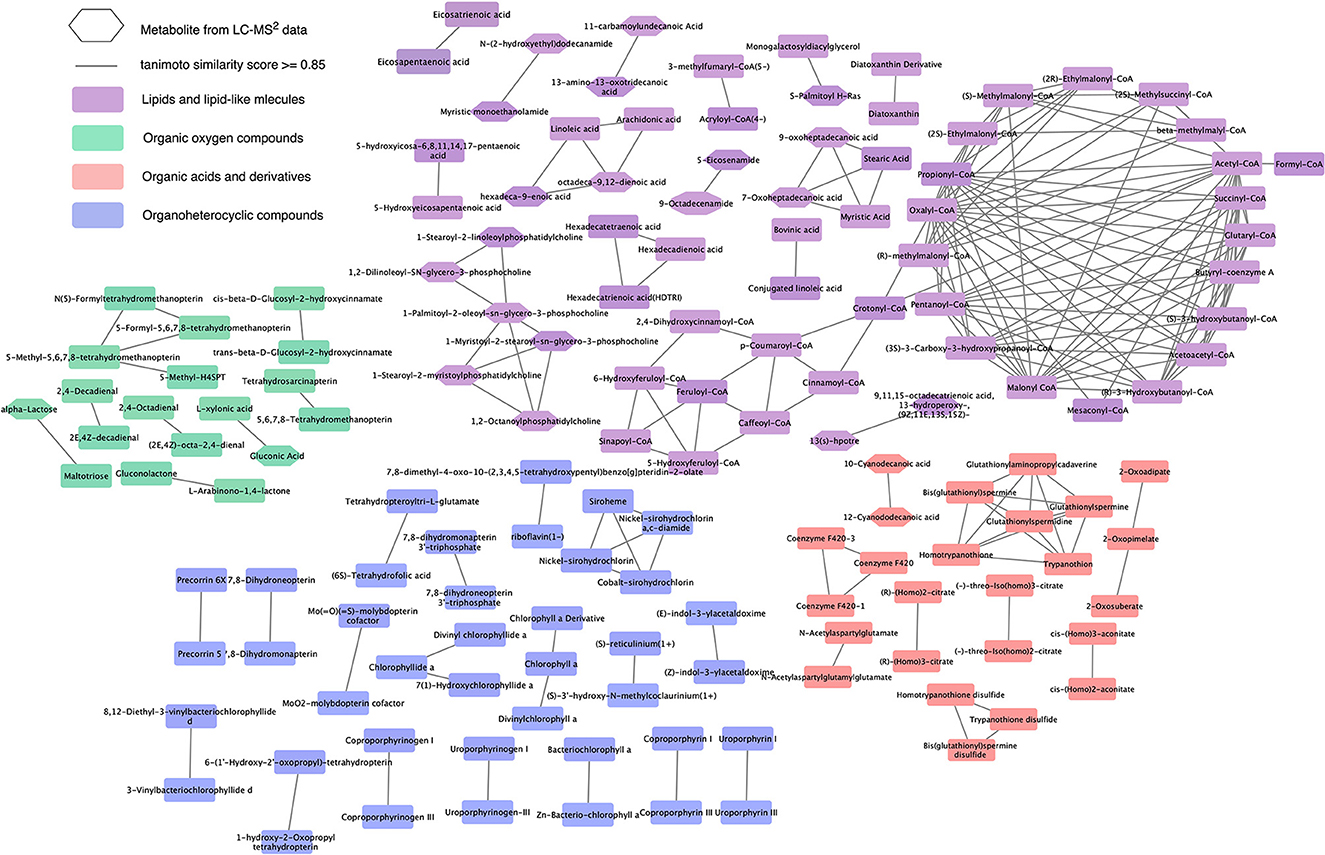
Figure 3. Expansion of chemical similarity network of the suspect list with the candidate structures from LC-MS2 data. This network represents the expanded chemical similarity space of the suspect list from Supplementary Figure 2; the new metabolites from the LC-MS2 data are represented with a hexagonal shape. This network shows the most abundant superclasses and the structural similarities between the metabolites from these superclasses.
4 Discussion
Marine natural product reservoirs are still partly underexplored/ unexploited (Nieri et al., 2023). The marine diatom S. marinoi, for example, has been extensively studied for its lipid metabolism; however, the chemical diversity has not yet been fully explored for other natural products. To understand the biochemical mechanisms occurring within ubiquitous diatoms, we expanded the chemical space of known metabolites from S. marinoi using a whole untargeted metabolomics approach. With our automated MAW, we annotated 69% of the metabolic features from the LC-MS2 data with a chemical structure or a molecular formula (see Table 1). We verified the presence of previously identified metabolites using the suspect list of compounds from S. marinoi as the source of known metabolites (see Supplementary Figures 1, 2). Here, we discuss metabolites annotated in this study, of which some have not been previously identified in S. marinoi.
4.1 Secondary metabolites in the expanded chemical space of S. marinoi
Using the LC-MS2 data, MAW annotated some of the known metabolites produced by S. marinoi, many of which were amino acids, analogs, and their derivatives such as betaine, pipecolic acid, 4-hydroxyproline, methionine sulfoxide, and N,N-dimethylarginine. Such polar compounds alleviate salt stress in S. marinoi and might act as osmoprotectants (Nikitashina et al., 2022). S. marinoi is also known to produce a rich portfolio of lipids and lipid-like molecules. With MAW, we annotated several polyunsaturated fatty acids (PUFA), such as eicosapentaenoic and eicosatrienoic acids, saturated fatty acids (SFA), such as myristic acid and stearic acid, and fatty acid esters (FAE) such as isovalerylcarnitine, propionylcarnitine, and butyrylcarnitine, which also act as stress protectants (Nikitashina et al., 2022). Being a photosynthetic organism, S. marinoi is also known to produce terpenoid pigments, such as astaxanthin and tocopherol, which were also verified with MAW (Silva et al., 2022).
MAW annotated 1,072 unique structures to metabolites that were not present in the suspect list and hence represented the expansion of known metabolites produced by S. marinoi. Only a few of these compounds show chemical similarity with the previously known natural products produced by S. marinoi (shown in Figure 3 as hexagonal nodes), among which most of the metabolites belonged to the lipids and lipid-like molecules superclass. Lipids in diatoms have been studied extensively due to their role as membrane constituents, regulators of gene expression, and sources of energy for the cells via fatty acid beta-oxidation (Yi et al., 2017; Vuong et al., 2020). In Figure 3, one of the chemical similarity clusters showed two saturated fatty acids (SFA)—myristic (14:0) and stearic acids (18:0)—and two saturated oxo-fatty acids (SOFA)−7-oxoheptadecanoic acid and 9-oxoheptadecanoic acid (derivatives of heptadecanoic acid). SOFAs are SFAs with a ketone group. This remains an understudied class of lipids with unknown pathways (Batsika et al., 2021) and hence cannot be linked back to the known diatom metabolism. However, the SFA heptadecanoic acid is present in algae, plants, and bacteria (Wikidata Contributors, n.d.). In a study done on S. costatum, the fatty acid profile in the stationary growth phase showed minute levels of heptadecanoic acid (Popovich et al., 2012), indicating the measurable presence of this SFA after the end of the cell growth period. Heptadecanoic acid has been exploited for its medicinal use against type 2 diabetes and skin cancer (Forouhi et al., 2014; Kathiresan, 2020). 4-Oxo sebacic acid, which is likely derived from sebacic acid (a dicarboxylic SFA), was also annotated using MAW; however, no previous records of sebacic acid in diatoms have been reported so far.
Another class of detected lipids is the fatty acid amide (FAA). A chemical similarity pair was formed between two FAA derivatives of myristic and lauric acid—myristic monoethanolamide and N-(2-hydroxyethyl)dodecanamide. Both are N-functionalized long-chain-acyl ethanolamines resulting from the condensation of the carboxy group of an SFA with the amino group of ethanolamine. N-(long-chain-acyl)ethanolamines are converted to SFAs by FAA hydrolases (Rhea-Fatty Acid Amide hydrolase ID: 45456, n.d.). Another pair of FAAs with high structural similarity was 5-eicosenamide (an unsaturated eicosanoic acid derivative) and 9-octadecanamide (a stearic acid derivative). Two other high-scoring examples (from Table 2) are hexadecanamide (derived from palmitic acid) and tetradecanamide (derived from myristic acid). Carboxamides also undergo conversion back to SFA. SFAs are present in the lipid profile of many diatoms (Pratiwi et al., 2009); however, there is no systematic research done on their FAA derivatives reported in this study. FAAs, such as oleamide from oleic acid, have anti-biofouling effects (Fiorini et al., 2020) on the accumulated microalgal biofilms on various surfaces, but this result has to be verified in the light of our finding that microalgae contribute themselves to the compound class.
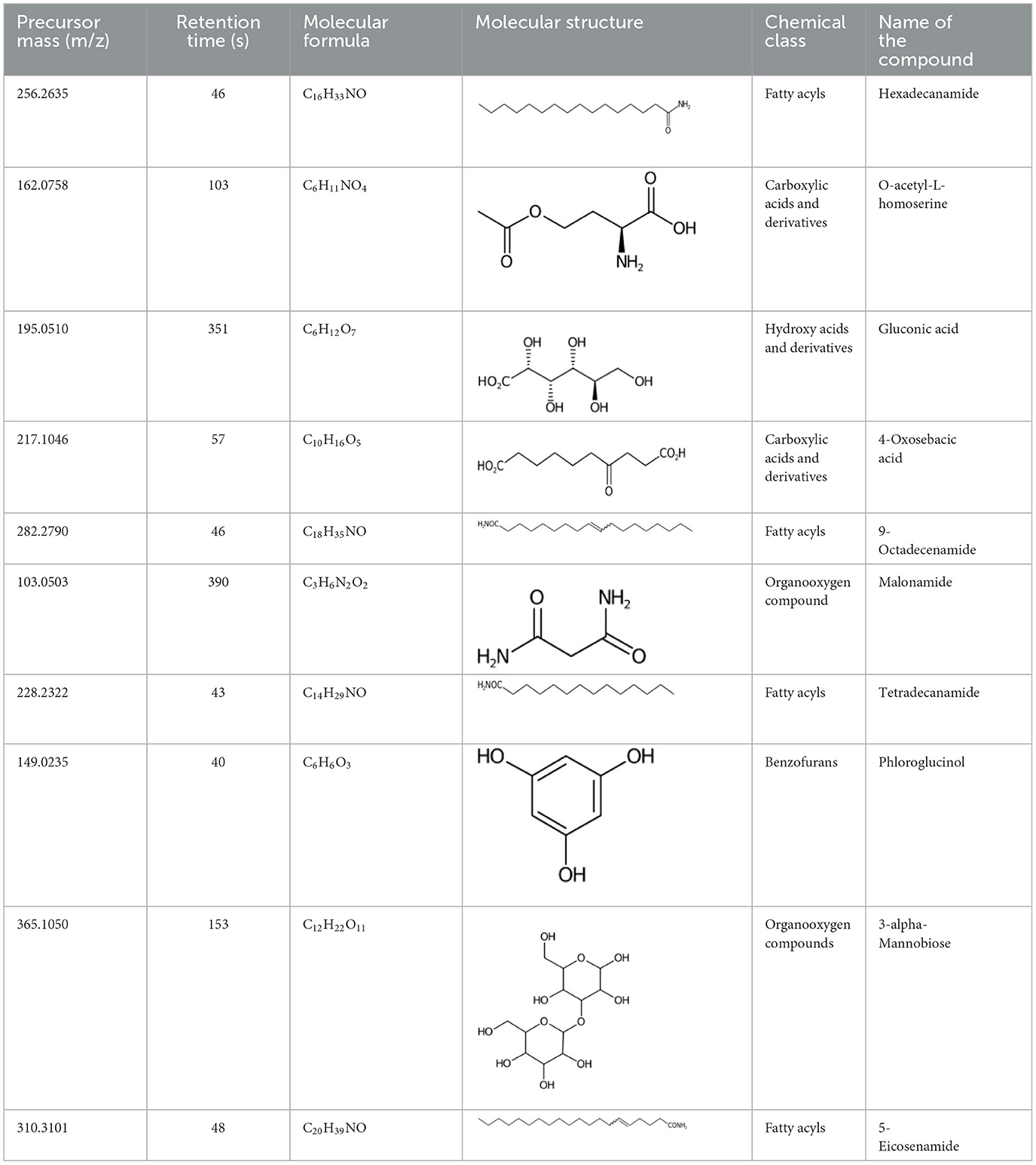
Table 2. List of top-ranking metabolites (based on MAW candidate selection criterion) from the LC-MS2 data annotated with MAW from MSI levels 2 and 3.
One larger cluster in the chemical similarity network in Figure 3 is the group of six glycerophospholipids, mainly phosphocholines and phosphatidylcholines, with two fatty acyl groups. Glycerophospholipids are integral to the membrane structure (Niu et al., 2013) and have also been found in the silica deposition vesicles that form the silica shells in the diatom Thalassiosira pseudonana (Schwarz et al., 2022). Enzymatic transformation of these metabolites, releasing choline, occurs through hydrolysis via phospholipase D, where choline acts as a downstream signaling molecule (Hausmann et al., 2011). Another reaction is the acyltransferase reaction, which catalyzes the release of fatty acids from the 1 or 2 acyl group position via phospholipase A (Duncan et al., 2008). These enzymatic activities have also been reported in diatoms (Pohnert, 2002; Beligni et al., 2015) but not with the glycerophospholipids found in this study. It is noteworthy that the spectral features annotated with these glycerophospholipids had smaller precursor masses as compared to the monoisotopic mass of the molecules, which can be attributed to the in-source fragmentation where one fatty acyl chain fragmented from the whole molecule, and so the annotations, in this case, are not extremely reliable.
Diatoms produce extracellular polysaccharides as part of their silica shell/frustules formed by monosaccharide building blocks. Generally, the cell wall of diatoms also contains mannose and glucuronic acid as main constituents and varying amounts of fucose and xylose (Gügi et al., 2015). A recent study showed that the cell wall of the diatom Phaeodactylum tricornutum contains α –(1 → 3)-mannan backbone, which could explain the presence of 3-alpha-mannobiose in diatoms as annotated with MAW (Le Costaouëc et al., 2017). Furthermore, xylose, maltotriose, and allulose were annotated with MAW and had a chemical similarity with three sugar molecules from the suspect list, arabinose, maltose, and sorbose, with a Tanimoto similarity score of 1. MAW annotated maltotriose, which is found in pennate diatom biofilms (Wigglesworth-Cooksey and Cooksey, 2005). Another monosaccharide allulose was annotated with MAW, which is rarely found in natural carbohydrate plants and macroalgae, with applications such as anti-atherosclerosis, anti-hyperlipidemic, and neuroprotective (Mu et al., 2018).
A few other metabolites annotated with MAW were phloroglucinol, malonamide, and two polyphenols (chlorogenate and 4-hydroxycinnamic acid). Phloroglucinol is a growth promoter produced by marine algae Phaeophyceae and Fucaceae and is known to increase the levels of the carotenoid pigment fucoxanthin in microalgae (Liu et al., n.d.). Malonamide is a dicarboxylic acid diamide, a derivative of malonic acid. Malonamide can be converted back to malonic acid via amidase. Malonamide derivatives (MAMDs) have been utilized as anticancer and antibiotic drugs (Purgatorio et al., 2022); however, not many studies have been conducted on the role of malonamide as a metabolite specifically in diatoms.
Diatoms, in general, are rich sources of polyphenolic compounds exhibiting antioxidant properties (Goiris et al., 2012). Studies show that diatom Phaeodactylum tricornutum, under copper stress, releases high concentrations of polyphenols such as 4-hydroxycinnamic acid and chlorogenic acid (Rico et al., 2013; Santiago-Díaz et al., 2023).
4.2 Prokaryote-associated metabolites
S. marinoi forms synergistic interactions with marine prokaryotes. The phycosphere of the marine diatom, which represents the diffusion-limited mucus-containing layer around the cells, hosts several bacterial species that stimulate the growth of the diatoms (Johansson et al., 2019). The bacteria provide the S. marinoi with vitamins and organic sulfur metabolites and get the nitrogen and carbon sources in return (Bruckner et al., 2011; Seymour et al., 2017). In this study, pure but not axenic S. marinoi strain was used for inoculation. S. marinoi grew with its associated microbiome that never became dominant in terms of biomass, as monitored by microscopy. Thus, we cannot rule out that some of the metabolites detected arise from metabolism by the associated bacteria, and hence, bacterial-produced metabolites were also measured and annotated. Here, we discuss a few high-scoring metabolites annotated with MAW reported in marine prokaryotes.
A sugar-based organic acid, gluconic acid, has been found in marine bacteria connected to the release of domoic acid as a toxin in blooms of the diatom Pseudo-nitzschia multiseries (Stewart et al., 1997). A benzenoid from the statin family, which is known for its therapeutic properties, fluostatin J, is generally found in marine actinobacteria (Zhang et al., 2012). Indole is produced by symbiotic bacteria supporting the growth of Skeletonema marinoi (Johansson et al., 2019). Marine bacteria and some marine algae execute the biodegradation of sulfoacetate to produce sulfoacetaldehyde, which is a major organic solute in marine organisms (Weinitschke et al., 2010). 6-Chloropurine riboside, derived from the bacterium Geobacillus stearothermophilus, is used as an antiviral and anti-tumoral therapeutic agent (Rivero et al., 2012).
4.3 Assessment of data quality and Metabolome Annotation Workflows
The metabolites annotated with MAW revealed a large number of chemical compounds from Skeletonema marinoi, which have also been previously reported in other diatoms. However, we also observed metabolites from prokaryotic, plant, and fungal origin (the latter two were discarded during the curation). Many of the metabolites from non-diatomic sources were also marine natural products, suggesting that both fungi and diatoms may produce similar compounds.
However, the annotation of certain spectral features to compounds of fungal origin could also be due to misannotation attributed to the limitations of mass spectrometry techniques and current annotation tools and databases and the complexity of chemical data. Mass spectrometry (MS) is currently the main viable option for untargeted metabolomics, followed by manual curation and verification (Chaleckis et al., 2019). The MS-based search for metabolite annotation rarely leads to unique biochemical structures due to the presence of compound isomers in the databases used for dereplication and the limitations in the mass accuracy of the MS system used (Xiao et al., 2012). The MS1 peaks chosen for MS2 fragmentation have varying optimal fragmentation energy, so not all MS2 spectra are informative. Features obtained with an untargeted LC-MS2 approach, which do not have an MSI level 1 confidence score (based on the known internal standard measurement), cannot provide absolute certainty about the assigned chemical structure for a feature due to the lack of our current knowledge of the chemical dark space. Moreover, the current databases also only favor well-studied model organisms and may not cover the entire metabolome of S. marinoi, which is why it is important to elucidate the metabolome of this diatom.
To overcome a fraction of these challenges, MAW automatized the annotation process and performed dereplication in both spectral and compound databases while integrating prior biochemical knowledge in the form of a suspect list, which is also important for making accurate annotations. To give each annotation a confidence score, the Metabolomics Standards Initiative (MSI) levels of confidence were implemented in MAW to follow the standards set by the metabolomics scientific community. Automated workflows, such as MAW, aid in the standardization and reproducibility and reduce the manual work in the analysis. Metabolomics is a relatively new omics field, and the standardization in metabolomics is not widely adopted, which leads to missing metadata and reproducibility issues. However, it is challenging to follow a set of standards in metabolomics because of the different combinations of chromatography and data acquisition methods of a diverse set of metabolites. Moreover, although using ChemONT, which is a standardized vocabulary for chemical classification, one metabolite with a carboxylic group and an amine group can be categorized as either an organic acid or an organic nitrogen compound. As of now, MAW follows the currently available standards and ontologies for the spectral data acquired from data-dependent acquisition (DDA) LC-MS2 data, associating one chemical class to each annotation.
4.4 Future perspective
The catalog of metabolites presented in this study exhibits a wide range of coverage provided by untargeted LC-MS-metabolomics. However, this approach does not provide validity for structure identification. To overcome this limitation, it is recommended to conduct targeted studies using some of the biologically important NPs. To further improve the accuracy of metabolite annotation in future studies, techniques such as nuclear magnetic resonance (NMR) spectroscopy can be employed to provide additional structural information for metabolite identification. The development of more comprehensive annotation tools and submission of new experimental spectra to natural product databases like GNPS will facilitate better metabolite annotation and identification, contributing to the expansion of available metabolites. Re-annotation with new updates of the databases can also enhance the annotation results. An integrative study of genomics and metabolomics from this diatom can also reveal the production of secondary metabolites involved in different biomechanisms in the marine ecosystem, providing a systems-level understanding. Being the model organism for diatoms, this could hold the potential for elucidating biochemical mechanisms in other diatom species and unveiling marine-centric metabolic pathways (Edison et al., 2016).
Data availability statement
The datasets presented in this study can be found in online repositories. The RAW and mzML metabolome data and the identifications can be found here: European Molecular Biology Laboratory's European Bioinformatics Institute (EMBL-EBI) MetaboLights, https://www.ebi.ac.uk/metabolights/, MTBLS2892 (Haug et al., 2020). The mzML files can be found here: Zenodo, https://zenodo.org/, doi: 10.5281/zenodo.7515842 and doi: 10.5281/zenodo.7515829. The currently available versions of GNPS, HMDB, and MassBank can be found here: Zenodo, https://zenodo.org/, doi: 10.5281/zenodo.6528931. The suspect list for Skeletonema spp. can be found here: Zenodo, https://zenodo.org/, doi: 10.5281/zenodo.5772755. The scripts used to generate the list of metabolites using MAW version 1.1 and for the suspect list curation can be found here: GitHub, https://github.com/, zmahnoor14/MAW-Diatom. The list of annotated metabolites from MAW, intersection and union lists, along with the corresponding sunburst plots can be found here: Zenodo, https://zenodo.org/, doi: 10.5281/zenodo.7798782.
Author contributions
MZ: Data curation, Formal analysis, Methodology, Software, Validation, Writing—original draft, Writing—review & editing. DS: Formal analysis, Investigation, Methodology, Writing—original draft, Writing—review & editing. SS: Formal analysis, Methodology, Writing—review & editing. VN: Formal analysis, Methodology, Writing—review & editing. GP: Conceptualization, Funding acquisition, Project administration, Resources, Supervision, Writing—review & editing. CS: Conceptualization, Funding acquisition, Project administration, Resources, Supervision, Writing—review & editing. KP: Project administration, Supervision, Writing—review & editing. MS: Conceptualization, Investigation, Project administration, Supervision, Writing—review & editing.
Funding
The author(s) declare financial support was received for the research, authorship, and/or publication of this article. Funded by the Deutsche Forschungsgemeinschaft (DFG, German Research Foundation) under Germany's Excellence Strategy—EXC 2051—Project-ID 390713860.
Conflict of interest
The authors declare that the research was conducted in the absence of any commercial or financial relationships that could be construed as a potential conflict of interest.
Publisher's note
All claims expressed in this article are solely those of the authors and do not necessarily represent those of their affiliated organizations, or those of the publisher, the editors and the reviewers. Any product that may be evaluated in this article, or claim that may be made by its manufacturer, is not guaranteed or endorsed by the publisher.
Supplementary material
The Supplementary Material for this article can be found online at: https://www.frontiersin.org/articles/10.3389/fmicb.2023.1295994/full#supplementary-material
References
Allard, P.-M. (2020). pybatchclassyfire. GitLab. Available online at: https://gitlab.unige.ch/Pierre-Marie.Allard/pybatchclassyfire (accessed September 22, 2022).
Amato, A., Sabatino, V., Nylund, G. M., Bergkvist, J., Basu, S., Andersson, M. X., et al. (2018). Grazer-induced transcriptomic and metabolomic response of the chain-forming diatom Skeletonema marinoi. ISME J. 12, 1594–1604. doi: 10.1038/s41396-018-0094-0
Anning, T., MacIntyre, H. L., Pratt, S. M., Sammes, P. J., Gibb, S., and Geider, R. J. (2000). Photoacclimation in the marine diatom Skeletonema costatum. Limnol. Oceanogr. 45, 1807–1817. doi: 10.4319/lo.2000.45.8.1807
Batsika, C. S., Mantzourani, C., Gkikas, D., Kokotou, M. G., Mountanea, O. G., Kokotos, C. G., et al. (2021). Saturated oxo fatty acids (SOFAs): a previously unrecognized class of endogenous bioactive lipids exhibiting a cell growth inhibitory activity. J. Med. Chem. 64, 5654–5666. doi: 10.1021/acs.jmedchem.0c02058
Bauermeister, A., Mannochio-Russo, H., Costa-Lotufo, L. V., Jarmusch, A. K., and Dorrestein, P. C. (2021). Mass spectrometry-based metabolomics in microbiome investigations. Nat. Rev. Microbiol. 20, 143–160. doi: 10.1038/s41579-021-00621-9 Available online at: https://www.nature.com/articles/s41579-021-00621-9
Beligni, M. V., Bagnato, C., Prados, M. B., Bondino, H., Laxalt, A. M., Munnik, T., et al. (2015). The diversity of algal phospholipase D homologs revealed by biocomputational analysis. J. Phycol. 51, 943–962. doi: 10.1111/jpy.12334
Brown, M. R., and Jeffrey, S. W. (1995). The amino acid and gross composition of marine diatoms potentially useful for mariculture. J. Appl. Phycol. 7, 521–527. doi: 10.1007/BF00003938
Bruckner, C. G., Rehm, C., Grossart, H.-P., and Kroth, P. G. (2011). Growth and release of extracellular organic compounds by benthic diatoms depend on interactions with bacteria. Environ. Microbiol. 13, 1052–1063. doi: 10.1111/j.1462-2920.2010.02411.x
Cardoso, C., Pereira, H., Franca, J., Matos, J., Monteiro, I., Pousão-Ferreira, P., et al. (2020). Lipid composition and some bioactivities of 3 newly isolated microalgae (Tetraselmis sp. IMP3, Tetraselmis sp. CTP4, and Skeletonema sp.). Aquac. Int. 28, 711–727. doi: 10.1007/s10499-019-00489-w
Caspi, R., Altman, T., Billington, R., Dreher, K., Foerster, H., Fulcher, C. A., et al. (2014). The MetaCyc database of metabolic pathways and enzymes and the BioCyc collection of Pathway/Genome Databases. Nucleic Acids Res. 42, D459–D471. doi: 10.1093/nar/gkt1103
Chaleckis, R., Meister, I., Zhang, P., and Wheelock, C. E. (2019). Challenges, progress and promises of metabolite annotation for LC–MS-based metabolomics. Curr. Opin. Biotechnol. 55, 44–50. doi: 10.1016/j.copbio.2018.07.010
Chambers, M. C., Maclean, B., Burke, R., Amodei, D., Ruderman, D. L., Neumann, S., et al. (2012). A cross-platform toolkit for mass spectrometry and proteomics. Nat. Biotechnol. 30, 918–920. doi: 10.1038/nbt.2377
Chang, A., Jeske, L., Ulbrich, S., Hofmann, J., Koblitz, J., Schomburg, I., et al. (2021). BRENDA, the ELIXIR core data resource in 2021: new developments and updates. Nucleic Acids Res. 49, D498–D508. doi: 10.1093/nar/gkaa1025
Derenbach, J. B., and Pesando, D. (1986). Investigations into a small fraction of volatile hydrocarbons: III. Two diatom cultures produce ectocarpene, a pheromone of brown algae. Mar. Chem. 19, 337–341. doi: 10.1016/0304-4203(86)90054-X
Di Dato, V., Orefice, I., Amato, A., Fontanarosa, C., Amoresano, A., Cutignano, A., et al. (2017). Animal-like prostaglandins in marine microalgae. ISME J. 11, 1722–1726. doi: 10.1038/ismej.2017.27
Djoumbou Feunang, Y., Eisner, R., Knox, C., Chepelev, L., Hastings, J., Owen, G., et al. (2016). ClassyFire: automated chemical classification with a comprehensive, computable taxonomy. J. Cheminformatics 8, 61. doi: 10.1186/s13321-016-0174-y
Dührkop, K., Fleischauer, M., Ludwig, M., Aksenov, A. A., Melnik, A. V., Meusel, M., et al. (2019). SIRIUS 4: a rapid tool for turning tandem mass spectra into metabolite structure information. Nat. Methods 16, 299–302. doi: 10.1038/s41592-019-0344-8
Dührkop, K., Nothias, L.-F., Fleischauer, M., Reher, R., Ludwig, M., Hoffmann, M. A., et al. (2021). Systematic classification of unknown metabolites using high-resolution fragmentation mass spectra. Nat. Biotechnol. 39, 462–471. doi: 10.1038/s41587-020-0740-8
Dührkop, K., Shen, H., Meusel, M., Rousu, J., and Böcker, S. (2015). Searching molecular structure databases with tandem mass spectra using CSI:FingerID. Proc. Natl. Acad. Sci. U. S. A. 112, 12580–12585. doi: 10.1073/pnas.1509788112
Duncan, R. E., Sarkadi-Nagy, E., Jaworski, K., Ahmadian, M., and Sul, H. S. (2008). Identification and functional characterization of adipose-specific phospholipase A2 (AdPLA). J. Biol. Chem. 283, 25428–25436. doi: 10.1074/jbc.M804146200
Edison, A., Hall, R., Junot, C., Karp, P., Kurland, I., Mistrik, R., et al. (2016). The time is right to focus on model organism metabolomes. Metabolites 6, 8. doi: 10.3390/metabo6010008
Field, C. B., Behrenfeld, M. J., Randerson, J. T., and Falkowski, P. (1998). Primary production of the biosphere: integrating terrestrial and oceanic components. Science. 281, 237–240. doi: 10.1126/science.281.5374.237
Fiorini, F., Borgonuovo, C., Ferrante, M. I., and Brönstrup, M. (2020). A metabolomics exploration of the sexual phase in the marine diatom Pseudo-nitzschia multistriata. Mar. Drugs 18, 313. doi: 10.3390/md18060313
Fontana, A., d'Ippolito, G., Cutignano, A., Miralto, A., Ianora, A., Romano, G., et al. (2007a). Chemistry of oxylipin pathways in marine diatoms. Pure Appl. Chem. 79, 481–490. doi: 10.1351/pac200779040481
Fontana, A., d'Ippolito, G., Cutignano, A., Romano, G., Lamari, N., Massa Gallucci, A., et al. (2007b). LOX-induced lipid peroxidation mechanism responsible for the detrimental effect of marine diatoms on zooplankton grazers. Chembiochem 8, 1810–1818. doi: 10.1002/cbic.200700269
Forouhi, N. G., Koulman, A., Sharp, S. J., Imamura, F., Kröger, J., Schulze, M. B., et al. (2014). Differences in the prospective association between individual plasma phospholipid saturated fatty acids and incident type 2 diabetes: the EPIC-InterAct case-cohort study. Lancet Diabetes Endocrinol. 2, 810–818. doi: 10.1016/S2213-8587(14)70146-9
Gallo, C., Landi, S., d'Ippolito, G., Nuzzo, G., Manzo, E., Sardo, A., et al. (2020). Diatoms synthesize sterols by inclusion of animal and fungal genes in the plant pathway. Sci. Rep. 10, 4204. doi: 10.1038/s41598-020-60993-5
Godhe, A., and Härnström, K. (2010). Linking the planktonic and benthic habitat: genetic structure of the marine diatom Skeletonema marinoi. Mol. Ecol. 19, 4478–4490. doi: 10.1111/j.1365-294X.2010.04841.x
Goiris, K., Muylaert, K., Fraeye, I., Foubert, I., De Brabanter, J., and De Cooman, L. (2012). Antioxidant potential of microalgae in relation to their phenolic and carotenoid content. J. Appl. Phycol. 24, 1477–1486. doi: 10.1007/s10811-012-9804-6
Gügi, B., Le Costaouec, T., Burel, C., Lerouge, P., Helbert, W., and Bardor, M. (2015). Diatom-specific oligosaccharide and polysaccharide structures help to unravel biosynthetic capabilities in diatoms. Mar. Drugs 13, 5993–6018. doi: 10.3390/md13095993
Hastings, J., Owen, G., Dekker, A., Ennis, M., Kale, N., Muthukrishnan, V., et al. (2016). ChEBI in 2016: improved services and an expanding collection of metabolites. Nucleic Acids Res. 44, D1214–D1219. doi: 10.1093/nar/gkv1031
Haug, K., Cochrane, K., Nainala, V. C., Williams, M., Chang, J., Jayaseelan, K. V., et al. (2020). MetaboLights: a resource evolving in response to the needs of its scientific community. Nucleic Acids Res. 48, D440–D444. doi: 10.1093/nar/gkz1019
Hausmann, J., Kamtekar, S., Christodoulou, E., Day, J. E., Wu, T., Fulkerson, Z., et al. (2011). Structural basis for substrate discrimination and integrin binding by autotaxin. Nat. Struct. Mol. Biol. 18, 198–204. doi: 10.1038/nsmb.1980
Hildebrand, M., Davis, A. K., Smith, S. R., Traller, J. C., and Abbriano, R. (2012). The place of diatoms in the biofuels industry. Biofuels 3, 221–240. doi: 10.4155/bfs.11.157
Horai, H., Arita, M., Kanaya, S., Nihei, Y., Ikeda, T., Suwa, K., et al. (2010). MassBank: a public repository for sharing mass spectral data for life sciences. J. Mass Spectrom. 45, 703–714. doi: 10.1002/jms.1777
Ianora, A., Miralto, A., Poulet, S. A., Carotenuto, Y., Buttino, I., Romano, G., et al. (2004). Aldehyde suppression of copepod recruitment in blooms of a ubiquitous planktonic diatom. Nature 429, 403–407. doi: 10.1038/nature02526
Jing, X., Mi, T., Zhen, Y., Wang, H., and Yu, Z. (2019). Influence of N, P, Fe nutrients availability on nitrogen metabolism- relevant genes expression in Skeletonema marinoi. J. Ocean Univ. China 18, 239–252. doi: 10.1007/s11802-019-3500-y
Johansson, O. N., Pinder, M. I. M., Ohlsson, F., Egardt, J., Töpel, M., and Clarke, A. K. (2019). Friends with benefits: exploring the phycosphere of the marine diatom Skeletonema marinoi. Front. Microbiol. 10, e01828. doi: 10.3389/fmicb.2019.01828
Kanehisa, M. (2019). Toward understanding the origin and evolution of cellular organisms. Protein Sci. Publ. Protein Soc. 28, 1947–1951. doi: 10.1002/pro.3715
Kanehisa, M., Furumichi, M., Sato, Y., Kawashima, M., and Ishiguro-Watanabe, M. (2023). KEGG for taxonomy-based analysis of pathways and genomes. Nucleic Acids Res. 51, D587–D592. doi: 10.1093/nar/gkac963
Kanehisa, M., and Goto, S. (2000). KEGG: kyoto encyclopedia of genes and genomes. Nucleic Acids Res. 28, 27–30. doi: 10.1093/nar/28.1.27
Kathiresan, K. (2020). “Chapter 8 - bioprospecting potential of mangrove resources,” in Biotechnological Utilization of Mangrove Resources, eds J. K. Patra, R. R. Mishra, and H. Thatoi (Academic Press), 225–241. Available online at: https://www.sciencedirect.com/science/article/abs/pii/B9780128195321000081?via%3Dihub
Kelly, D. J., and Hughes, N. J. (2001). “The citric acid cycle and fatty acid biosynthesis,” in Helicobacter pylori: Physiology and Genetics (ASM Press). Available online at: https://www.ncbi.nlm.nih.gov/books/NBK2413/ (accessed May 7, 2023).
Khan, M. J., Rai, A., Ahirwar, A., Sirotiya, V., Mourya, M., and Mishra, S., et al. (n.d.). Diatom microalgae as smart nanocontainers for biosensing wastewater pollutants: recent trends innovations. Bioengineered 12, 9531–9549. doi: 10.1080/21655979.2021.1996748
Kim, S., Chen, J., Cheng, T., Gindulyte, A., He, J., He, S., et al. (2021). PubChem in 2021: new data content and improved web interfaces. Nucleic Acids Res. 49, D1388–D1395. doi: 10.1093/nar/gkaa971
Landrum, G. (2016). RDKit: Open-Source Cheminformatics Software. Available online at: http://www.rdkit.org/; https://github.com/rdkit/rdkit (accessed November 23, 2023).
Lauritano, C., Orefice, I., Procaccini, G., Romano, G., and Ianora, A. (2015). Key genes as stress indicators in the ubiquitous diatom Skeletonema marinoi. BMC Genom. 16, 411. doi: 10.1186/s12864-015-1574-5
Le Costaouëc, T., Unamunzaga, C., Mantecon, L., and Helbert, W. (2017). New structural insights into the cell-wall polysaccharide of the diatom Phaeodactylum tricornutum. Algal Res. 26, 172–179. doi: 10.1016/j.algal.2017.07.021
Litchman, E., de Tezanos Pinto, P., Edwards, K. F., Klausmeier, C. A., Kremer, C. T., and Thomas, M. K. (2015). Global biogeochemical impacts of phytoplankton: a trait-based perspective. J. Ecol. 103, 1384–1396. doi: 10.1111/1365-2745.12438
Liu, Y., Li, Y., Zhao, H., Zhu, B., Xu, J., and Xu, F., et al. (n.d.). Phloroglucinol promotes fucoxanthin synthesis by activating the cis-zeatin brassinolide pathways in Thalassiosira pseudonana. Appl. Environ. Microbiol. 88, e02160-21. doi: 10.1128/aem.02160-21
Lordan, S., Ross, R. P., and Stanton, C. (2011). Marine bioactives as functional food ingredients: potential to reduce the incidence of chronic diseases. Mar. Drugs 9, 1056–1100. doi: 10.3390/md9061056
Lu, W., Xing, X., Wang, L., Chen, L., Zhang, S., McReynolds, M. R., et al. (2020). Improved annotation of untargeted metabolomics data through buffer modifications that shift adduct mass and intensity. Anal. Chem. 92, 11573–11581. doi: 10.1021/acs.analchem.0c00985
Malviya, S., Scalco, E., Audic, S., Vincent, F., Veluchamy, A., Poulain, J., et al. (2016). Insights into global diatom distribution and diversity in the world's ocean. Proc. Natl. Acad. Sci. U. S. A. 113, E1516–E1525. doi: 10.1073/pnas.1509523113
Martins, A., Vieira, H., Gaspar, H., and Santos, S. (2014). Marketed marine natural products in the pharmaceutical and cosmeceutical industries: tips for success. Mar. Drugs 12, 1066–1101. doi: 10.3390/md12021066
Milito, A., Orefice, I., Smerilli, A., Castellano, I., Napolitano, A., Brunet, C., et al. (2020). Insights into the light response of Skeletonema marinoi: involvement of ovothiol. Mar. Drugs 18, 477. doi: 10.3390/md18090477
Morgan, H. L. (1965). The generation of a unique machine description for chemical structures-a technique developed at chemical abstracts service. J. Chem. Doc. 5, 107–113. doi: 10.1021/c160017a018
Mu, W., Hassanin, H. A. M., Zhou, L., and Jiang, B. (2018). Chemistry behind rare sugars and bioprocessing. J. Agric. Food Chem. 66, 13343–13345. doi: 10.1021/acs.jafc.8b06293
Nieri, P., Carpi, S., Esposito, R., Costantini, M., and Zupo, V. (2023). Bioactive molecules from marine diatoms and their value for the nutraceutical industry. Nutrients 15, 464. doi: 10.3390/nu15020464
Nikitashina, V., Stettin, D., and Pohnert, G. (2022). Metabolic adaptation of diatoms to hypersalinity. Phytochemistry 201, 113267. doi: 10.1016/j.phytochem.2022.113267
Niu, Y.-F., Zhang, M.-H., Li, D.-W., Yang, W.-D., Liu, J.-S., Bai, W.-B., et al. (2013). Improvement of neutral lipid and polyunsaturated fatty acid biosynthesis by overexpressing a type 2 diacylglycerol acyltransferase in marine diatom Phaeodactylum tricornutum. Mar. Drugs 11, 4558–4569. doi: 10.3390/md11114558
Orefice, I., Chandrasekaran, R., Smerilli, A., Corato, F., Caruso, T., Casillo, A., et al. (2016). Light-induced changes in the photosynthetic physiology and biochemistry in the diatom Skeletonema marinoi. Algal Res. 17, 1–13. doi: 10.1016/j.algal.2016.04.013
Pennington, F., Guillard, R. R. L., and Liaaen-Jensen, S. (1988). Carotenoid distribution patterns in bacillariophyceae (diatoms). Biochem. Syst. Ecol. 16, 589–592. doi: 10.1016/0305-1978(88)90067-1
Pohnert, G. (2002). Phospholipase A2 activity triggers the wound-activated chemical defense in the diatom Thalassiosira rotula. Plant Physiol. 129, 103–111. doi: 10.1104/pp.010974
Popovich, C. A., Damiani, C., Constenla, D., and Leonardi, P. I. (2012). Lipid quality of the diatoms Skeletonema costatum and Navicula gregaria from the South Atlantic Coast (Argentina): evaluation of its suitability as biodiesel feedstock. J. Appl. Phycol. 24, 1–10. doi: 10.1007/s10811-010-9639-y
Pratiwi, A. R., Syah, D., Hardjito, L., Panggabean, L. M. G., and Suhartono, M. T. (2009). Fatty acid synthesis bindonesian marine diatom, Chaetoceros gracilis. HAYATI J. Biosci. 16, 151–156. doi: 10.4308/hjb.16.4.151
Purgatorio, R., Gambacorta, N., Samarelli, F., Lopopolo, G., de Candia, M., Catto, M., et al. (2022). Assessing the role of a malonamide linker in the design of potent dual inhibitors of factor Xa and cholinesterases. Molecules 27, 4269. doi: 10.3390/molecules27134269
Qian, L., Tiezhu, M, Yu, Z., Hualong, W., and Zhigang, Y. (2016). Description of carbon fixation pathway based on Skeletonema marinoi transcriptome. Sci. Bull. 61, 2483–2493. doi: 10.1360/N972016-00046
Rhea-Fatty Acid Amide hyrolase ID: 45456 (n.d.). Available online at: https://www.rhea-db.org/rhea/45456 (accessed May 31, 2023).
Ribalet, F., Wichard, T., Pohnert, G., Ianora, A., Miralto, A., and Casotti, R. (2007). Age and nutrient limitation enhance polyunsaturated aldehyde production in marine diatoms. Phytochemistry 68, 2059–2067. doi: 10.1016/j.phytochem.2007.05.012
Rico, M., López, A., Santana-Casiano, J. M., Gonzàlez, A. G., and Gonzàlez-Dàvila, M. (2013). Variability of the phenolic profile in the diatom Phaeodactylum tricornutum growing under copper and iron stress. Limnol. Oceanogr. 58, 144–152. doi: 10.4319/lo.2013.58.1.0144
Rivero, C. W., De Benedetti, E. C., Sambeth, J. E., Lozano, M. E., and Trelles, J. A. (2012). Biosynthesis of anti-HCV compounds using thermophilic microorganisms. Bioorg. Med. Chem. Lett. 22, 6059–6062. doi: 10.1016/j.bmcl.2012.08.045
Rogers, D., and Hahn, M. (2010). Extended-connectivity fingerprints. J. Chem. Inf. Model. 50, 742–754. doi: 10.1021/ci100050t
Rousch, J. M., Bingham, S. E., and Sommerfeld, M. R. (2003). Changes in fatty acid profiles of thermo-intolerant and thermo-tolerant marine diatoms during temperature stress. J. Exp. Mar. Biol. Ecol. 295, 145–156. doi: 10.1016/S0022-0981(03)00293-4
Russo, E., Campos, A. M., d'Ippolito, G., Manzo, E., Carotenuto, Y., Fontana, A., et al. (2021). Implementation in lipid extraction and analysis from phytoplankton: Skeletonema marinoi as case study. Mar. Chem. 232, 103964. doi: 10.1016/j.marchem.2021.103964
Rutz, A., Sorokina, M., Galgonek, J., Mietchen, D., Willighagen, E., Gaudry, A., et al. (2022). The LOTUS initiative for open knowledge management in natural products research. Elife 11, e70780. doi: 10.7554/eLife.70780.sa2
Santiago-Díaz, P., Rivero, A., Rico, M., González González, A., González-Dávila, M., and Santana-Casiano, M. (2023). Copper toxicity leads to accumulation of free amino acids and polyphenols in Phaeodactylum tricornutum diatoms. Environ. Sci. Pollut. Res. 30, 51261–51270. doi: 10.1007/s11356-023-25939-0
Sarno, D., Kooistra, W. H. C. F., Medlin, L. K., Percopo, I., and Zingone, A. (2005). Diversity in the genus Skeletonema (bacillariophyceae). II. an assessment of the taxonomy of S. costatum-like species with the description of four new species. J. Phycol. 41, 151–176. doi: 10.1111/j.1529-8817.2005.04067.x
Sayanova, O., Mimouni, V., Ulmann, L., Morant-Manceau, A., Pasquet, V., Schoefs, B., et al. (2017). Modulation of lipid biosynthesis by stress in diatoms. Philos. Trans. R. Soc. B Biol. Sci. 372, 20160407. doi: 10.1098/rstb.2016.0407
Schwarz, P., Herrfurth, C., Steinem, C., and Feussner, I. (2022). Lipidomics of Thalassiosira pseudonana as a function of valve SDV synthesis. J. Appl. Phycol. 34, 1471–1481. doi: 10.1007/s10811-022-02715-0
Schymanski, E. L., Jeon, J., Gulde, R., Fenner, K., Ruff, M., Singer, H. P., et al. (2014). Identifying small molecules via high resolution mass spectrometry: communicating confidence. Environ. Sci. Technol. 48, 2097–2098. doi: 10.1021/es5002105
Seymour, J. R., Amin, S. A., Raina, J.-B., and Stocker, R. (2017). Zooming in on the phycosphere: the ecological interface for phytoplankton–bacteria relationships. Nat. Microbiol. 2, 1–12. doi: 10.1038/nmicrobiol.2017.65
Silva, M., Kamberovic, F., Uota, S. T., Kovan, I.-M., Viegas, C. S. B., Simes, D. C., et al. (2022). Microalgae as potential sources of bioactive compounds for functional foods and pharmaceuticals. Appl. Sci. 12, 5877. doi: 10.3390/app12125877
Smerilli, A., Balzano, S., Maselli, M., Blasio, M., Orefice, I., Galasso, C., et al. (2019). Antioxidant and photoprotection networking in the coastal diatom Skeletonema marinoi. Antioxidants 8, 154. doi: 10.3390/antiox8060154
Snoeijs, P., Sylvander, P., and H?ubner, N. (2011). “Oxidative stress in aquatic primary producers as a driving force for ecosystem responses to large-scale environmental changes,” in Oxidative Stress in Aquatic Ecosystems (JohnWiley & Sons Ltd.), 72–88. doi: 10.1002/9781444345988.ch5
Stewart, J. E., Marks, L. J., Wood, C. R., Risser, S. M., and Gray, S. (1997). Symbiotic relations between bacteria and the domoic acid producing diatom Pseudo-nitzschia multiseries and the capacity of these bacteria for gluconic acid/gluconolactone formation. Aquat. Microb. Ecol. 12, 211–221. doi: 10.3354/ame012211
Stonik, V., and Stonik, I. (2015). Low-molecular-weight metabolites from diatoms: structures, biological roles and biosynthesis. Mar. Drugs 13, 3672–3709. doi: 10.3390/md13063672
Sumner, L. W., Amberg, A., Barrett, D., Beale, M. H., Beger, R., Daykin, C. A., et al. (2007). Proposed minimum reporting standards for chemical analysis. Metabolomics 3, 211–221. doi: 10.1007/s11306-007-0082-2
Swain M. (n.d.). Introduction — PubChemPy 1.0.4 Documentation. Available online at: https://pubchempy.readthedocs.io/en/latest/guide/introduction.html#pubchempy-license (accessed November 18, 2022).
The UniProt Consortium (2021). UniProt: the universal protein knowledgebase in 2021. Nucl. Acids Res. 49, D480–D489. doi: 10.1093/nar/gkaa1100
Vidoudez, C., and Pohnert, G. (2012). Comparative metabolomics of the diatom Skeletonema marinoi in different growth phases. Metabolomics 8, 654–669. doi: 10.1007/s11306-011-0356-6
Vuong, T. T., Kwon, B.-R., Eom, J.-I., Shin, B.-K., and Kim, S. M. (2020). Interaction between marine bacterium Stappia sp. K01 and diatom Phaeodactylum tricornutum through extracellular fatty acids. J. Appl. Phycol. 32, 71–82. doi: 10.1007/s10811-019-01931-5
Wang, M., Carver, J. J., Phelan, V. V., Sanchez, L. M., Garg, N., Peng, Y., et al. (2016). Sharing and community curation of mass spectrometry data with global natural products social molecular networking. Nat. Biotechnol. 34, 828–837. doi: 10.1038/nbt.3597
Weinitschke, S., Hollemeyer, K., Kusian, B., Bowien, B., Smits, T. H. M., and Cook, A. M. (2010). Sulfoacetate is degraded via a novel pathway involving sulfoacetyl-CoA and sulfoacetaldehyde in Cupriavidus necator H16. J. Biol. Chem. 285, 35249–35254. doi: 10.1074/jbc.M110.127043
Wigglesworth-Cooksey, B., and Cooksey, K. E. (2005). Use of fluorophore-conjugated lectins to study cell-cell interactions in model marine biofilms. Appl. Environ. Microbiol. 71, 428–435. doi: 10.1128/AEM.71.1.428-435.2005
Wikidata Contributors (n.d.). Available online at: https://www.wikidata.org/w/index.php?title=Q902204&oldid=1903039391 (accessed May 29, 2023).
Wishart, D. S., Feunang, Y. D., Marcu, A., Guo, A. C., Liang, K., Vázquez-Fresno, R., et al. (2018). HMDB 4.0: the human metabolome database for 2018. Nucleic Acids Res. 46, D608–D617. doi: 10.1093/nar/gkx1089
Wishart, D. S., Tzur, D., Knox, C., Eisner, R., Guo, A. C., Young, N., et al. (2007). HMDB: the human metabolome database. Nucleic Acids Res. 35, D521–526. doi: 10.1093/nar/gkl923
Xiao, J. F., Zhou, B., and Ressom, H. W. (2012). Metabolite identification and quantitation in LC-MS/MS-based metabolomics. Trends Anal. Chem. TRAC 32, 1–14. doi: 10.1016/j.trac.2011.08.009
Yi, Z., Xu, M., Di, X., Brynjolfsson, S., and Fu, W. (2017). Exploring valuable lipids in diatoms. Front. Mar. Sci. 4, 17. doi: 10.3389/fmars.2017.00017
Zhang, W., Liu, Z., Li, S., Lu, Y., Chen, Y., Zhang, H., et al. (2012). Fluostatins I–K from the South China sea-derived Micromonospora rosaria SCSIO N160. J. Nat. Prod. 75, 1937–1943. doi: 10.1021/np300505y (accessed November 23, 2023).
Zulfiqar, M. (2022). Spectral Libraries for MAW. MAW Database Download. Available online at: https://zenodo.org/record/6528931 (accessed November 23, 2023).
Zulfiqar, M. (2023a). GitHub Repository for Metabolic Profiling of Skeletonema marinoi. Metab. Profiling Skeletonema Mar. Using MAW. Available online at: https://github.com/zmahnoor14/MAW-Diatom (accessed April 23, 2023).
Zulfiqar, M., Gadelha, L., Steinbeck, C., Sorokina, M., and Peters, K. (2023). MAW: the reproducible metabolome annotation workflow for untargeted tandem mass spectrometry. J. Cheminformat. 15, 32. doi: 10.1186/s13321-023-00695-y
Keywords: diatom, Skeletonema marinoi, Skeletonema, untargeted metabolomics, metabolome annotation, chemical classification
Citation: Zulfiqar M, Stettin D, Schmidt S, Nikitashina V, Pohnert G, Steinbeck C, Peters K and Sorokina M (2023) Untargeted metabolomics to expand the chemical space of the marine diatom Skeletonema marinoi. Front. Microbiol. 14:1295994. doi: 10.3389/fmicb.2023.1295994
Received: 17 September 2023; Accepted: 31 October 2023;
Published: 05 December 2023.
Edited by:
Yizhi Sheng, China University of Geosciences, ChinaReviewed by:
Nishikant Wase, University of Virginia, United StatesGiovanna Romano, Anton Dohrn Zoological Station, Italy
Copyright © 2023 Zulfiqar, Stettin, Schmidt, Nikitashina, Pohnert, Steinbeck, Peters and Sorokina. This is an open-access article distributed under the terms of the Creative Commons Attribution License (CC BY). The use, distribution or reproduction in other forums is permitted, provided the original author(s) and the copyright owner(s) are credited and that the original publication in this journal is cited, in accordance with accepted academic practice. No use, distribution or reproduction is permitted which does not comply with these terms.
*Correspondence: Christoph Steinbeck, christoph.steinbeck@uni-jena.de; Maria Sorokina, maria.sorokina@bayer.com