- 1College of Veterinary Medicine, Shanxi Agricultural University, Jinzhong, China
- 2Single Molecule Nanometry Laboratory (Sinmolab), Nanjing Agricultural University, Nanjing, China
Coinfection of porcine epidemic diarrhea virus (PEDV) and porcine deltacoronavirus (PDCoV) is common in pig farms, but there is currently no effective vaccine to prevent this co-infection. In this study, we used immunoinformatics tools to design a multi-epitope vaccine against PEDV and PDCoV co-infection. The epitopes were screened through a filtering pipeline comprised of antigenic, immunogenic, toxic, and allergenic properties. A new multi-epitope vaccine named rPPMEV, comprising cytotoxic T lymphocyte-, helper T lymphocyte-, and B cell epitopes, was constructed. To enhance immunogenicity, the TLR2 agonist Pam2Cys and the TLR4 agonist RS09 were added to rPPMEV. Molecular docking and dynamics simulation were performed to reveal the stable interactions between rPPMEV and TLR2 as well as TLR4. Additionally, the immune stimulation prediction indicated that rPPMEV could stimulate T and B lymphocytes to induce a robust immune response. Finally, to ensure the expression of the vaccine protein, the sequence of rPPMEV was optimized and further performed in silico cloning. These studies suggest that rPPMEV has the potential to be a vaccine candidate against PEDV and PDCoV co-infection as well as a new strategy for interrupting the spread of both viruses.
1 Introduction
Currently, the alphacoronavirus porcine epidemic diarrhea virus (PEDV) and deltacoronavirus porcine delta coronavirus (PDCoV) are two main swine enteric coronaviruses (Koonpaew et al., 2019; Hou et al., 2023): the former can infect swine of all ages and cause watery diarrhea, vomiting, and dehydration, and the latter causes acute diarrhea, vomiting, and dehydration in neonatal piglets (Tang et al., 2021; Hou et al., 2023). Especially, the co-infection of the two viruses, which both continue to emerge and reemerge worldwide, causing more severe mortality and economic losses.
To rapidly and efficiently prevent and control PEDV and PDCoV co-infection, the vaccine is a valuable means (Trovato et al., 2020). As a vaccine development route, the traditional methods are time-consuming and labor-intensive (Nabel, 2002), and such vaccines often contain large proteins or the entire organism, resulting in an unnecessary antigenic load and increasing the likelihood of eliciting an allergic reaction (Chauhan et al., 2019). These problems can be solved by using peptide-based vaccines, which are made up of brief immunogenic peptide fragments that can elicit highly targeted immune responses, thereby reducing the likelihood of an allergic reaction. In peptide-based vaccine development, effective screening and immunogen design are major challenges since short peptides typically have weak immunogenic effects due to their small molecular weights (Sun et al., 2022). According to reported works, the coronavirus S protein plays an important role in viral entry and virus-host interaction, and it is the primary target for stimulating the host cell immune response and inducing neutralizing antibodies (Sun et al., 2008; Li et al., 2021). Furthermore, it was also reported that the S proteins of PEDV and PDCoV have good immunity and the potential for vaccine development (Wang et al., 2016; Zhai et al., 2023). Therefore, the S protein is the preferred region for immunogen screening and the design of the PEDV and PDCoV vaccine. For immunogen screening, the typical epitope screening is to insert a peptide with the target epitope into the plasmid and verify the immune effect of the epitope through large experiments (De Groot et al., 2001). Immunoinformatics approaches, which can eliminate the need for time-consuming and expensive manipulation as well as complex procedures, have emerged as a crucial tool for epitope localization and are playing an increasingly important role in epitope discovery as well as in successful vaccine design (Khan et al., 2018; Dong et al., 2020). Khan et al. (2021) used immunoinformatics methods to design a universal multi-epitope vaccine against SARS-CoV-2. Rowaiye et al. (2023) developed a multiepitope vaccine candidate to curb the outbreaks of African swine fever virus using the immunoinformatics. Therefore, these immunoinformatics approaches can be employed for vaccine design for the PEDV and PDCoV co-infection.
In this study, we employed immunoinformatic approaches to predict and design a safe and effective multi-epitope candidate vaccine derived from the S protein for prevalent PEDV and PDCoV variants. The designed vaccine named rPPMEV comprises a range of predicted epitopes, can interact with TLRs, and has the potential to stimulate T and B lymphocytes to induce a strong immunological response. The findings of this study provide a new vaccine candidate for the prevention of PEDV and PDCoV co-infection.
2 Materials and methods
To predict and design a safe and effective multi-epitope candidate vaccine for PEDV and PDCoV co-infection, procedures listed in Figure 1 were implemented. In this section, these procedures are briefly mentioned below.
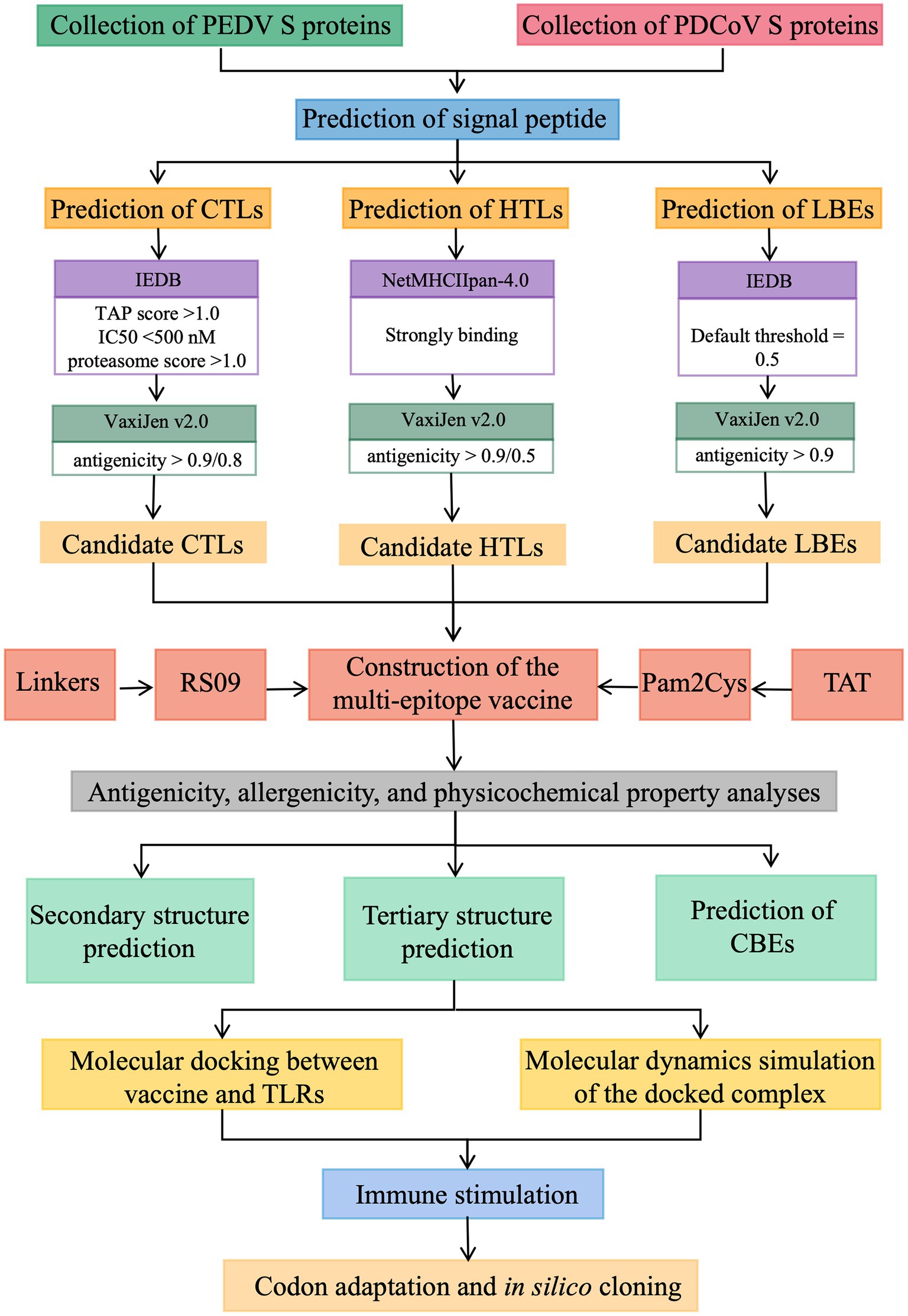
Figure 1. The prediction procedures of the multi-epitope candidate vaccine for PEDV and PDCoV co-infection.
2.1 Identification of target antigens
PEDV strains CH/HLJJS/2022, CH/HBXT/2018, and SXSL were selected since they have been highly pathogenic in recent years (Zhang et al., 2019; Liu et al., 2022; Yao et al., 2023). The spike (S) glycoproteins UUT43943.1, AZL49329.1, and UWU45211.1 of these three PEDV strains were selected as candidate antigens for epitope prediction due to their significant usefulness in PEDV vaccines (Zhang et al., 2019, Liu et al., 2022, Yao et al., 2023). In addition, PDCoV strains CH-HLJ-20, HNZK-02, Swine/CHN/SC/2018/1, CHN/Sichuan/2019-MK993519.1, and CHN-TS1-2019-MT663769.1 were selected for vaccine development as these have been prevalent in recent years (Kong et al., 2022). The S glycoproteins QZA57171.1, AXP32216.1, QCO76963.1, QGZ00525.1, and QZX45753.1 of these five PDCoV strains were selected as preparatory antigens because the S protein has good immunity for PDCoV vaccine design (Zhai et al., 2023). The sequences of all S proteins were obtained from the National Center for Biotechnology Information (NCBI) database.1
2.2 Prediction of signal peptide
To determine whether the signal peptide is present in the candidate antigen proteins, the signal peptide of the S protein was predicted using SignalP-5.0 server2 (Almagro Armenteros et al., 2019). The following T and B cell epitope predictions all need to remove the signal peptides.
2.3 Prediction of cytotoxic T lymphocyte epitopes
The Immune Epitope Database (IEDB) server3 was used to predict cytotoxic T lymphocyte epitopes (CTLs) (Fleri et al., 2017). The epitope length of 9 residues was used to predict the epitope through 45 common swine leukocyte antigen (SLA) class I molecules by running the “IEDB-recommended” method. Then, the epitopes were screened using a TAP score >1.0, an IC50 <500 nM, and a proteasome score >1.0. The dominant epitopes, which simultaneously appeared in at least three SLA-I alleles in each viral strain and had high antigenicity (> 0.9 for PEDV, > 0.8 for PDCoV), were further predicted by using VaxiJen v2.0.4 Finally, the common dominant epitopes of the PEDV and PDCoV strains were used to construct the final vaccine.
2.4 Prediction of helper T lymphocyte epitopes
The online server NetMHCIIpan 4.05 was used to predict helper T lymphocyte epitopes (HTLs). A length of 15 amino acid residues was used for epitope prediction through 27 high-frequency human MHC II (HLA-II) alleles (Ros-Lucas et al., 2020). The threshold for strongly binding peptides was set to its default value. The dominant epitopes, which simultaneously appeared in at least three HLA-II alleles in each viral strain and had high antigenicity (> 0.9 for PEDV, > 0.5 for PDCoV), were further predicted by using VaxiJen v2.0. Finally, the common dominant epitopes of the PEDV and PDCoV strains were used to construct the final vaccine.
2.5 Prediction of linear B cell epitopes
For the prediction of linear B cell epitopes (LBEs), the IEDB server with the method of Bepipred Linear Epitope Prediction 2.0 at the default threshold of 0.5 was used. Then, the predicted epitopes were screened using VaxiJen v2.0. Finally, the common epitopes with high antigenicity (>0.9) of the PEDV or PDCoV strains were used for vaccine construction.
2.6 Construction of the multi-epitope vaccine
The final subunit vaccine was constructed by sequentially combining the generated peptide sequences with appropriate linkers. To improve the antigenicity and immunogenicity of the vaccine, the toll-like receptor 4 (TLR4) agonist RS09 and the TLR2 agonist dipalmitoyl-S-glycero-cysteine (Pam2Cys) were added to the N-terminal and C-terminal via the EAAAK linker, respectively (Jackson et al., 2004; Meza et al., 2017; Albutti, 2021). The CTLs, HTLs, and B cell epitopes were joined by AAY, GPGPG, and KK, respectively. In addition, the TAT sequence (11 aa) was added to its carboxyl terminus to enhance the intracellular delivery of the vaccine (Frankel and Pabo, 1988).
2.7 Antigenicity, allergenicity, and physicochemical property analyses of the multi-epitope vaccine
The antigenicity and allergenicity of the multi-epitope vaccine were analyzed using the online software VaxiJen v2.0 and AllerTop v. 2.0,6 respectively. The physicochemical characteristics of the multi-epitope vaccine, such as its molecular weight, atomic composition, theoretical isoelectric point (PI), half-life, stability, hydropathicity, and other properties, were predicted using the online program Protparam.7
2.8 Prediction of the secondary and tertiary structures of the multi-epitope vaccine
The secondary structure of the multi-epitope vaccine was predicted using SOPMA online analysis software8 (Deléage, 2017). The initial tertiary structure was predicted by Robetta server9 (Baek et al., 2021). After primary 3D modeling, the initial tertiary structure was further optimized by GalaxyRefine server10 (Yu et al., 2022). Later, the refined structure was validated using two online tools: SWISS-MODEL workspace11 and ProSA-web.12 The SWISS-MODEL workspace was used to evaluate the quality of protein by analyzing the Ramachandran plot (Waterhouse et al., 2018). The ProSA-web was used for protein validation by generating a z-score (Wiederstein and Sippl, 2007).
2.9 Prediction of conformational B cell epitopes
The conformational B cell epitopes (CBEs) of the multi-epitope vaccine were predicted using the online software IEDB ElliPro tool13 with the default parameters of a minimum score of 0.5 and a maximum distance of 6 angstrom (Ponomarenko et al., 2008), and visualized with PyMol.
2.10 Molecular docking between the multi-epitope vaccine and TLRs
The molecular docking between the vaccine construct and the TLRs was performed using ClusPro server14 (Kozakov et al., 2017). The receptors were TLR2 (PDB ID: 6NIG) and TLR4 (PDB ID: 4G8A), and the ligand was the multi-epitope vaccine. The PDB file of the docking results was loaded into Ligplot and PyMol to analyze the interaction interface residues.
2.11 Molecular dynamics simulation of the docked complex
To understand any state changes in a given biological environment, the molecular dynamics (MD) simulation was applied to the TLR2-Vaccine and TLR4-Vaccine complexes using GROMACS (GROningen MAchine for Chemical Simulations) (He et al., 2021). First, in all MD simulations, the protein-ligand complex architecture was generated using the AMBER99 force field. The protein was then solvated in a cubic box of TIP3P waters (Grifoni et al., 2020), with a minimum distance of 1.0 nm (TLR2-Vaccine, TLR4-Vaccine) between the protein and box edge (Ismail et al., 2020). The charged protein complex was neutralized by the addition of ions using a genion tool (Shukla et al., 2018). Additionally, the solvated electroneutral system was relaxed through energy minimization in order to avoid steric conflicts and inappropriate geometry. Then, 100 ps of NVT [substance (N), volume (V), and temperature (T)] equilibration and 100 ps of NPT [substance (N), pressure (P), and temperature (T)] equilibration were used to acclimate the system without restrictions. After proper minimizations and equilibrations, a productive MD run of 20 ns was performed for all the complex systems, and the parameters, root mean square deviation (RMSD) and root mean square fluctuation (RMSF), which define the stability of the docked complex on simulation, were computed.
2.12 Immune stimulation
To detect the immune response of the multi-epitope vaccine to the host, the C-ImmSim server15 was used for the immune simulation (Rapin et al., 2010). The time steps were set at 1, 84, and 168 (one time step corresponds to 8 h). The number of simulation steps was set at 1,050 (Bhatnager et al., 2021). The other parameters were used as the default simulation parameters.
2.13 Codon adaptation and in silico cloning
To achieve superior expression of recombinant protein, the codon adaptation of the multi-epitope vaccine was performed by the online tool Java Codon Adaptation Tool (JCat)16 (Grote et al., 2005). Escherichia coli (Strain K12) was chosen to express the vaccine protein. The indicators, codon adaptation index (CAI) the ideal value is (1) and percentage GC content (the ideal range is 30%–70%), were analyzed (Puigbo et al., 2007). For in silico cloning of the vaccine construct, pET28a (+) was selected as the vector. The codon-optimized sequence of the vaccine was cloned into the vector through the XhoI and BamHI restriction sites by SnapGene tool.17
3 Results and discussion
3.1 The acquisition of vaccine-candidate antigens
Nowadays, the co-infection of PEDV and PDCoV, which both continue to emerge and reemerge worldwide, causes massive economic losses to the swine industry globally (Jiao et al., 2021). To rapidly and efficiently prevent and control virus infection, the development of vaccines has become imperative. In this study, we used immunoinformatics to discover and design a multivalent epitope vaccine to combat PEDV and PDCoV. The schematic procedure of the multi-epitope selection and the final vaccine construction is shown in Figure 2. The development of a new vaccine derived from a highly virulent virus provides cross-protection against low-virulence virus infection (Yao et al., 2023), and a vaccine developed from the strains responsible for the current outbreak will be successful in preventing viral infection (Rock, 2017; Borca et al., 2020; Moise et al., 2020). Thus, the three highly pathogenic PEDV strains and five prevalent PDCoV strains were selected for vaccine development. Furthermore, because the S proteins of PEDV and PDCoV strains have strong immunogenicity and the potential to generate vaccines (Wang et al., 2016; Zhai et al., 2023), the S proteins were selected as the preparatory antigens for immunogen screening and the design of a new PEDV and PDCoV vaccine. The GeneBank accession numbers of the three PEDV strains and five PDCoV strains, as well as the antigenicity of all S proteins, are shown in Supplementary Table S1, and the amino acid sequence of all S proteins is shown in Supplementary Data.
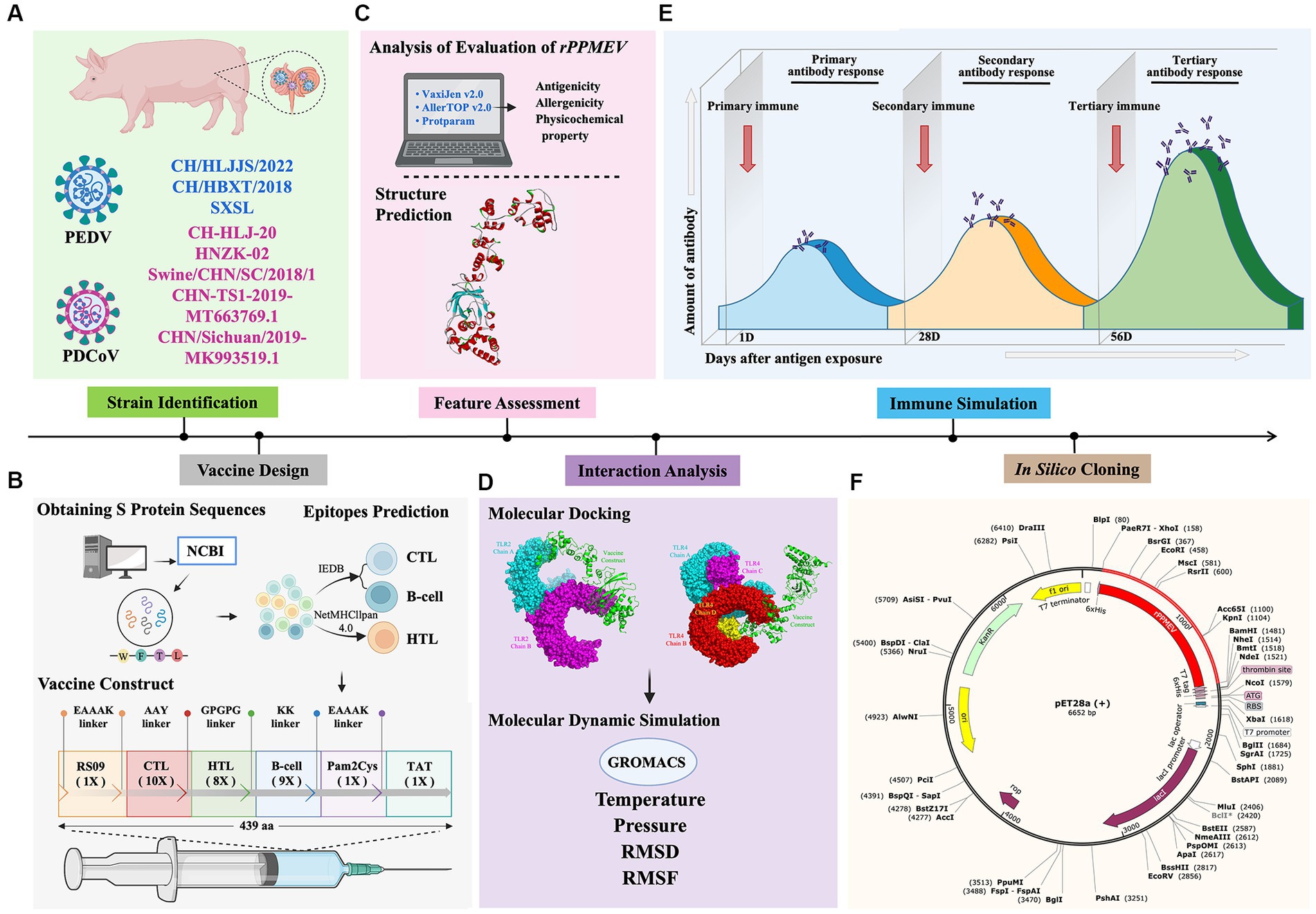
Figure 2. Flow chart of the multi-epitope selection and the final rPPMEV construction. The rPPMEV was designed in six steps with different colors, including PEDV and PDCoV strain identification (A), vaccine design (B), rPPMEV feature assessment (C), the interaction analysis of rPPMEV with TLR2 and TLR4 immune receptors (D), rPPMEV immunological characteristics analysis (E), and in silico cloning (F).
To prepare an epitope vaccine, obtaining the epitopes of the relative antigen is the key point (Li et al., 2013). Firstly, to determine whether these S proteins contain signal peptide regions, the signal peptide was examined before the epitope prediction. The findings reveal that the signal peptide sequence of PEDV is 1–18 (MKSLTYFWLLLPVLSTLS), while the signal peptide region of PDCoV is 1–19 (MQRALLIMTLLCLVRAKFA) (Supplementary Table S1). Then, to avoid specifying or inhibiting protein localization, the signal peptide sequences were removed from the epitope prediction of all S proteins (Mahmud et al., 2021). Secondly, it was reported that cytotoxic T cells are important for specific antigen recognition and the helper T cells are an essential component of adaptive immunity, which function in activating B cells, macrophages, and even cytotoxic T cells (Dimitrov et al., 2013; Gupta et al., 2013), the two types of epitopes, CTLs and HTLs, of T cell epitopes were predicted in this study. Furthermore, the B cell epitope was also screened for the vaccine construct since it could trigger the production of antigen-specific immunoglobulins, which are crucial components of adaptive immunity (Sarkar et al., 2022). After epitope prediction, the inclusion criteria for immunodominant epitopes are as follows: (1) the common dominant CTLs simultaneous appearance in at least three SLA-I alleles of each PEDV strain or PDCoV strain and with a high antigenicity score; (2) the common dominant HTLs simultaneous appearance in at least three HLA-II alleles in each PEDV strain or PDCoV strain and with a high antigenicity score; (3) the common dominant LBEs simultaneous appearance in each PEDV or PDCoV strain and with high antigenicity. Finally, 10 CTLs, 8 HTLs, and 9 LBEs were chosen for constructing the new multi-epitope vaccine rPPMEV (Supplementary Table S2). Then, according to the reports, TLRs are constitutively expressed in innate immune cells and play a vital role in viral recognition, leading to antiviral signaling cascades. Specifically, cell membrane receptors TLR2 and TLR4 play an important role in recognizing envelope glycoproteins (Kurt-Jones et al., 2000; Boehme and Compton, 2004; Takeda and Akira, 2004; Barton, 2007; Temeeyasen et al., 2018). To significantly improve the immunogenicity and antigenicity of rPPMEV, the TLR2 agonist Pam2Cys (FNNFTVSFWLRVPKVSASHLE) and TLR4 agonist RS-09 (APPHALS), which can trigger activation of TLR2 and TLR4 signaling, respectively (Jiang et al., 2023), were incorporated into the vaccine design. Additionally, to conjugate and enhance expression function and prevent the production of neo-epitopes, linker selection is a significant concern in the development of multi-epitope vaccines (Chen et al., 2013). The EAAAK linker, which can effectively separate and decrease interaction between vaccines as well as increase the thermal stability of the chimeric protein (Saadi et al., 2017), was used to join the adjuvants RS-09 and Pam2Cys. The AAY, GPGPG, and KK linkers were utilized to join the CTLs, HTLs, and B cell epitopes, respectively. The TAT sequence was attached to the C-terminal of the vaccine construct to enhance vaccine intercellular delivery. Finally, the new multivalent vaccine, rPPMEV, which was designed using immunoinformatics techniques, has a length of 439 amino acids, as illustrated in Figure 3.
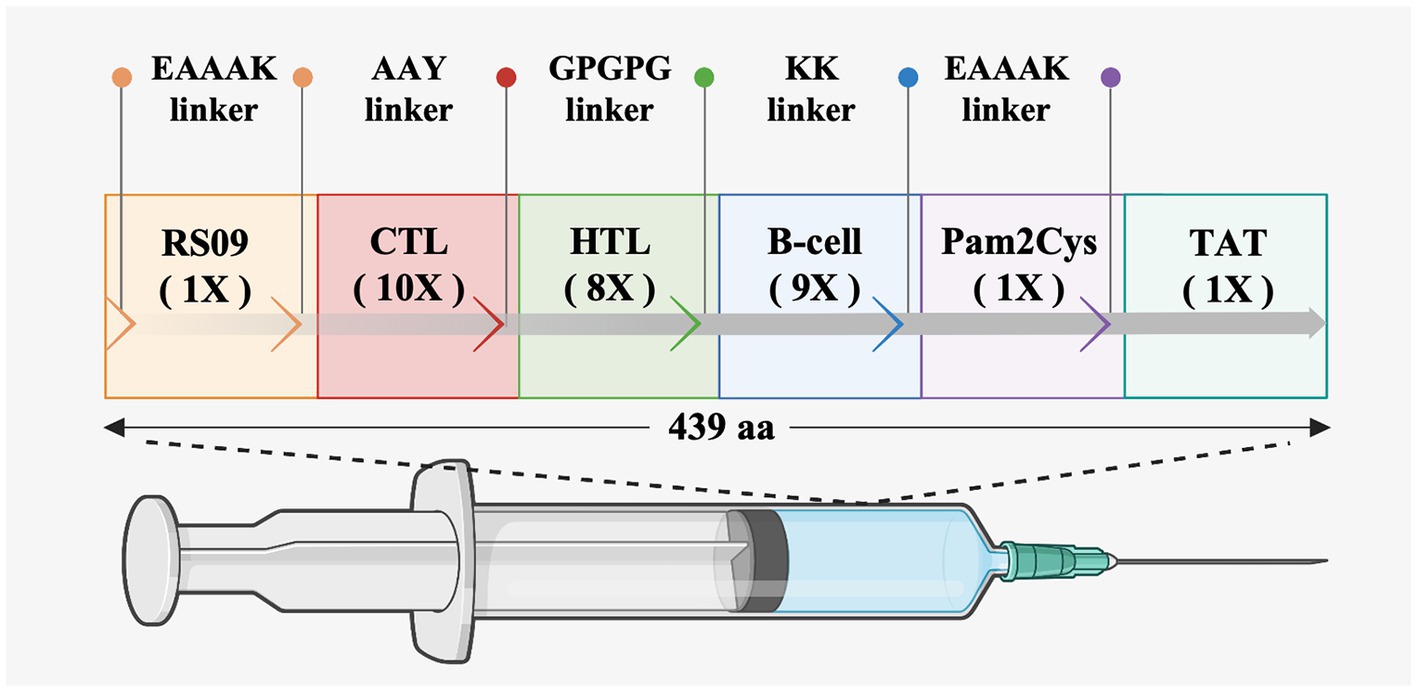
Figure 3. The design and construction of rPPMEV. The rPPMEV contains 439 amino acids, and the components needed in rPPMEV construction are represented in different colors.
3.2 Allergenicity, antigenicity, and physicochemical property analyses of rPPMEV
To evaluate the safety of rPPMEV, an allergenicity analysis was carried out using the AllerTop v. 2.0 server. The results show that rPPMEV and its closest protein (UniProtKB accession number O14514) are non-allergic. Moreover, the antigenicity analysis of VaxiJen v2.0 reveals that rPPMEV exhibits strong antigenicity with a score of 0.7241, which is above the threshold of 0.4. These results demonstrate that rPPMEV is safe for administration to swine. Additionally, the physicochemical properties of rPPMEV were also analyzed, as the physical properties of proteins significantly affect their immune function (Ikai, 1980). The finding shows that rPPMEV has 439 amino acids, 6,617 total atoms, the formula C2154H3274N554O627S8, and a molecular weight of 47 KD, which can be easily purified since the molecular weight of the protein is less than 110 KD (Barh et al., 2013). The theoretical pI of rPPMEV is 9.39, and it includes 28 negatively charged residues and 46 positively charged residues. The instability index of rPPMEV was calculated to be 33.81 (a value below the threshold value of 40 means that the protein is stable), indicating that rPPMEV should be stable upon expression in host systems. Furthermore, the aliphatic index of rPPMEV is 68.54, and the Grand average of hydropathicity (GRAVY) of rPPMEV is-0.249 (the range of GRAVY is −2 to 2, a negative value means that protein is hydrophilic) (Sha et al., 2020), showing that rPPMEV is hydrophilic.
3.3 The prediction of rPPMEV secondary and tertiary structure
An ideal peptide-based vaccination designed using immunoinformatics techniques should trigger a strong immunological response without having any negative side effects (Tahir ul Qamar et al., 2020; Shantier et al., 2022). The secondary structure determines the stability of protein structure, which is essential for antigen proteolysis, presentation, and activation of T and B cells (Scheiblhofer et al., 2017), and the tertiary structure determines the molecular recognition by the TCR (Greenbaum et al., 2007). As a result of secondary prediction, there is 31.89% alpha helix, 25.06% extended strand, 7.52% beta turn, and 35.54% random coil in rPPMEV, as shown in Figure 4A. Among these regions, the naturally unfolding protein regions and alpha-helical coiled coils, as basic types of “structural antigens,” can induce antibody recognition after infection (Corradin et al., 2007). Subsequently, the tertiary structure of the vaccine was predicted using the Robetta server. There are five models outputted in the result. The z-score was calculated on all models through ProSA-web. The z-scores of models 1–5 are −7.43, −7.64, −6.92, −6.19, and −6.59, respectively, as shown in Supplementary Figure S1. Model 2 was selected as the initial model of rPPMEV (Figure 4B) since it has the highest quality with the lowest z-score (Figure 4C). The Ramachandran plot shows that Model 2 has 91.53% favored region, 1.60% outlier region, and 0.00% rotamer region (Figure 4D). To improve the structure quality and protein stability, the initial model was refined by the GalaxyRefine server. As a result, five optimized 3D models are presented. The z-scores of these optimized models 1–5 are −7.84, −7.69, −7.62, −7.93, and −7.66, respectively (Supplementary Figure S2). Similar to the initial model selection, the optimized Model 4 was adopted as the final tertiary structure of rPPMEV (Figure 4E), which has the lowest z-score (Figure 4F) and performs at 93.59%, 1.14%, and 1.16% in the favored, outlier, and rotamer regions, respectively (Figure 4G).
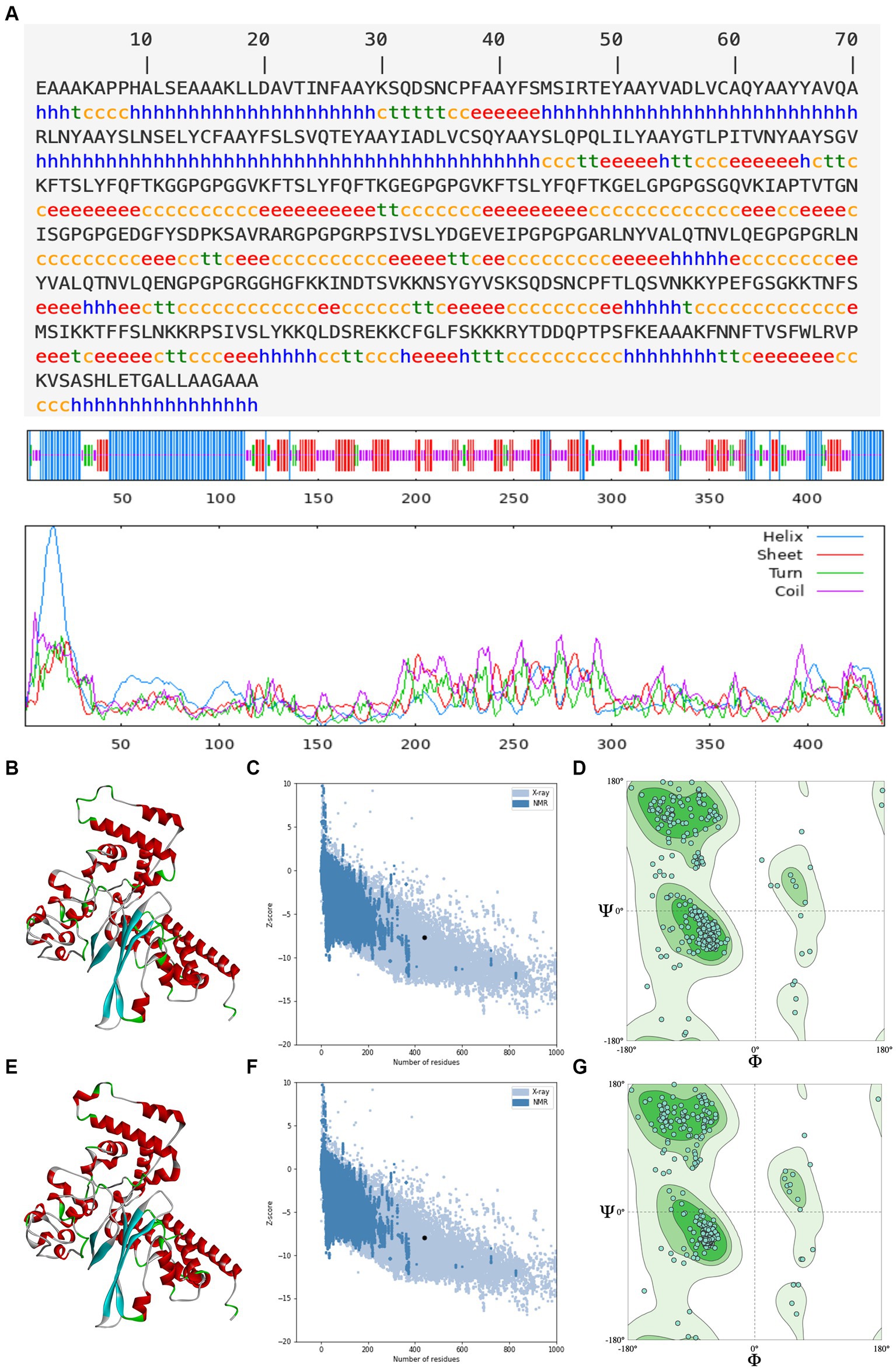
Figure 4. The prediction of rPPMEV secondary and tertiary structure. (A) The prediction of rPPMEV secondary structure. The blue “h” represents the alpha helix, the red “e” represents the extended strand, the green “t” represents the beta turn, and the yellow “c” represents the random coil. (B) The prediction of rPPMEV initial tertiary structure. The alpha helix, extended strand, beta turn, and random coil are marked in the 3D model with the colors of red, cyan, green, and gray, respectively. (C) The z-score of the rPPMEV initial tertiary structure. (D) The Ramachandran plots of the rPPMEV initial tertiary structure. (E) The prediction of the rPPMEV final tertiary structure. In the 3D model, the “red,” “cyan,” “green,” and “gray” parts represent alpha helix, extended strand, beta turn, and random coil, respectively. (F) The z-score of the rPPMEV final tertiary structure. (G) The Ramachandran plots of the rPPMEV final tertiary structure.
3.4 Prediction of conformational B cell epitopes
To predict CBEs, the rPPMEV was analyzed through the ElliPro server. The results show that there are 238 residues, with values ranging from 0.676 to 0.842, distributed across the eight B cell epitopes in rPPMEV. The epitopes range from 11 to 77 amino acid residues, as shown in Figure 5 and Supplementary Table S3.
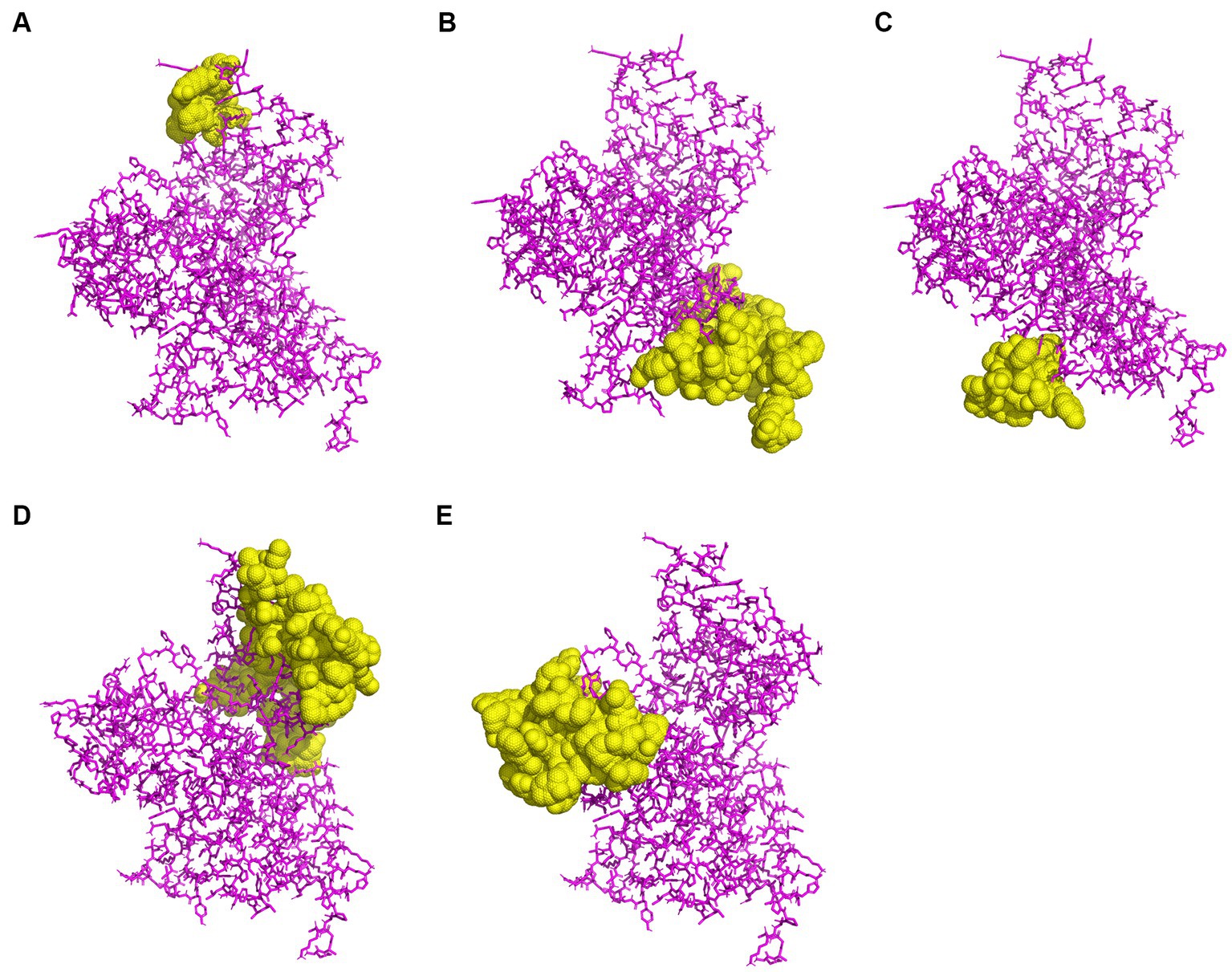
Figure 5. The prediction of conformational B cell epitopes. (A) to (E) display the five CBEs of rPPMEV. The “yellow” regions are the predicted conformational B cell epitopes of rPPMEV.
3.5 Molecular docking between rPPMEV and TLRs
To prevent and control viruses, the ability of vaccines to induce a brisk and consistent immune response is critical. To achieve the objective of the proposed work, it is necessary to design a vaccine that can interact with the target immune cell receptors (Choudhury et al., 2022). The TLRs are a class of essential protein molecules involved in innate immunity as well as a link between nonspecific and specific immunity (Takeda and Akira, 2004). TLR2 and TLR4 can recognize viral structural glycoproteins, resulting in the production of inflammatory cytokines (Choudhury et al., 2022). To evaluate the interaction and binding consistency between rPPMEV and TLRs, molecular docking was performed with the rPPMEV ligand and TLR2 as well as TLR4 receptors, respectively. The results show that there are 30 docking results of rPPMEV-TLR2 (Supplementary Table S4) and rPPMEV-TLR4 (Supplementary Table S5), respectively. The conformation of the docked rPPMEV-TLR2 with the lowest interaction energy (−1119.4 kcal/mol) is shown in Figure 6A. The interaction interface residues of this rPPMEV-TLR2 complex were analyzed by PyMol in 3D and Ligplot in 2D, respectively. The findings reveal that the complex subunits interact through one ionic bond and 11 hydrogen bonds, as illustrated in Figures 6B,C. Similar to the rPPMEV-TLR2, the rPPMEV-TLR4 model was selected according to its lowest energy weighted score (−1032.7 kcal/mol), as shown in Figures 7A,D. The interaction interface residue analysis in 3D and 2D formats reveal that there are 2 ionic bonds and 25 hydrogen bonds at the docking interface of rPPMEV and the TLR4 Chain B, as shown in Figures 7B,C, one hydrogen bond at the docking interface of rPPMEV and the TLR4 Chain C, as shown in Figures 7E,F, and 4 hydrogen bonds at the docking interface of rPPMEV and the TLR4 Chain D, as shown in Figures 7G,H. These results indicate that rPPMEV has excellent performance in tightly binding to TLR2 and TLR4 to trigger a strong immune response.
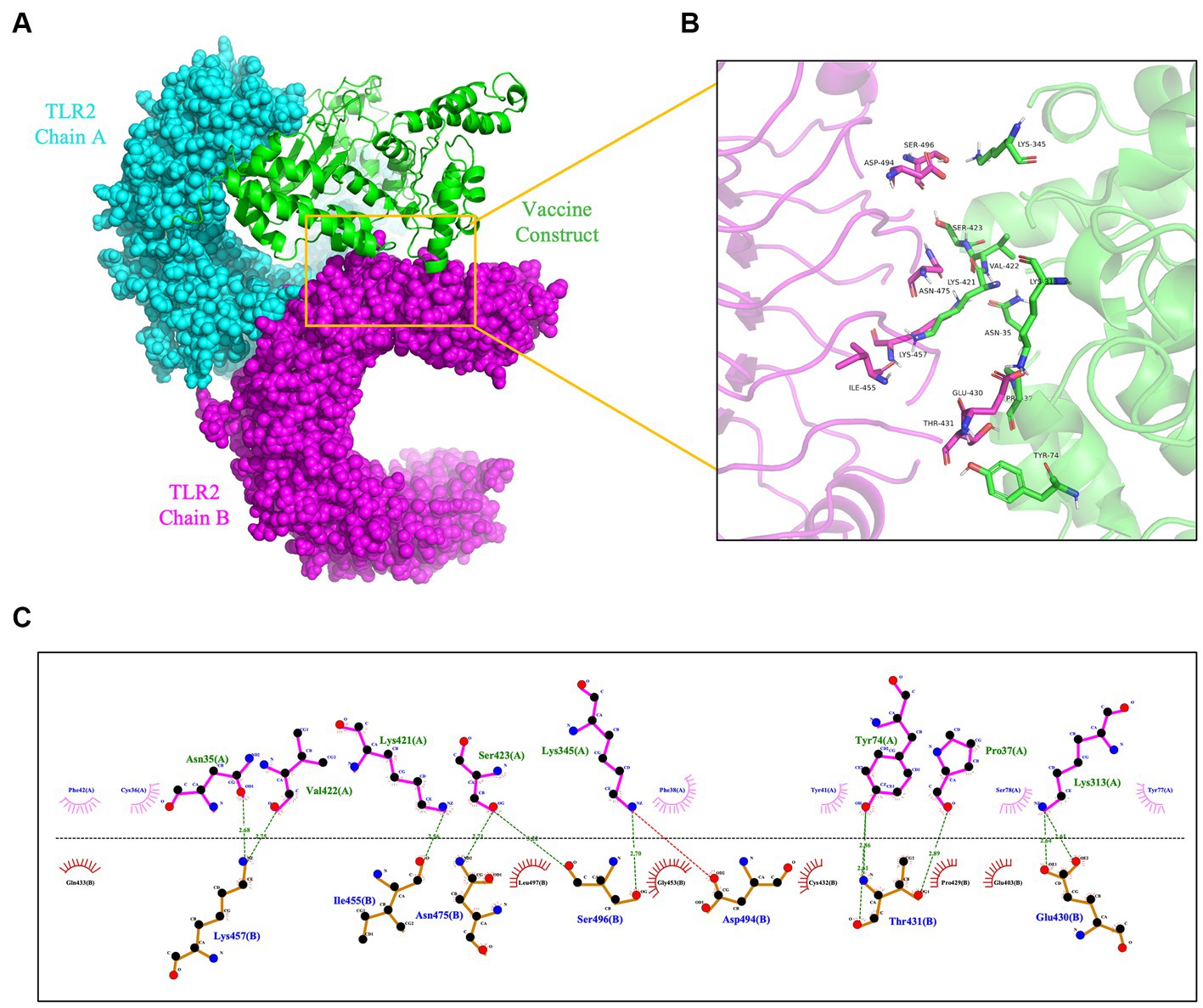
Figure 6. The molecular docking of rPPMEV and TLR2. (A) The docked complexes of rPPMEV and TLR2 with the lowest interaction energy. (B) The interaction interface residues of rPPMEV and TLR2 predicted by PyMol in 3D. (C) The interaction interface residues of rPPMEV and TLR2 predicted by Ligplot in 2D. The green dotted line represents the hydrogen bond, and the red dotted line represents the ionic bond.
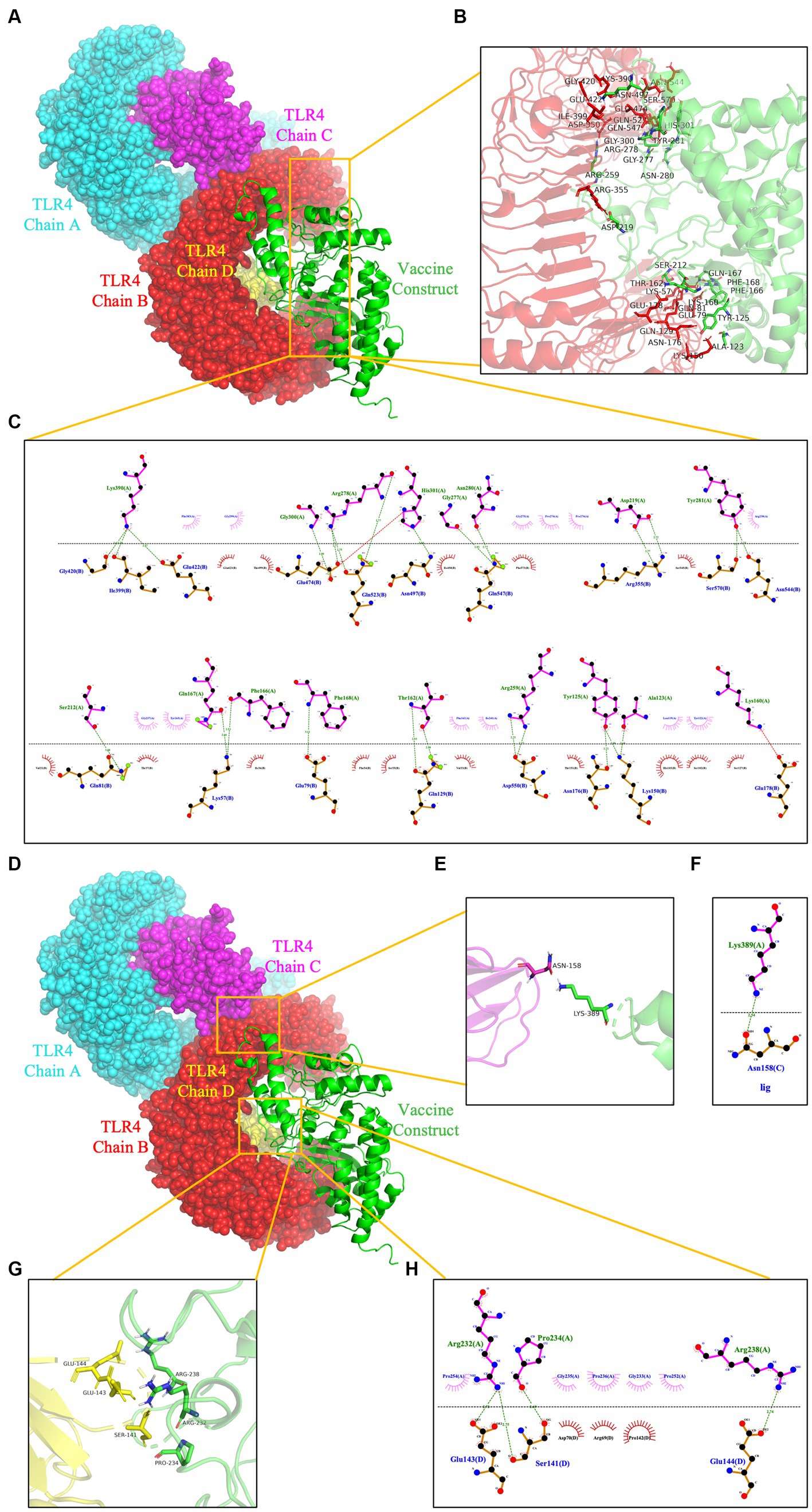
Figure 7. The molecular docking of rPPMEV and TLR4. The docked complexes of rPPMEV and TLR4 with the lowest interaction energy (A,D). The interaction interface residues predicted by PyMol in 3D of rPPMEV with TLR4 Chain B (B), TLR4 Chain C (E), and TLR4 Chain D (G). The interaction interface residues predicted by Ligplot in 2D of rPPMEV with TLR4 Chain B (C), TLR4 Chain C (F), and TLR4 Chain D (H). The green dotted line represents the hydrogen bond, and the red dotted line represents the ionic bond.
3.6 Molecular dynamics simulations between rPPMEV and TLRs
To evaluate the structural stability of the rPPMEV-TLR2 and rPPMEV-TLR4 complexes, the MD simulation was conducted using GROMACS. The results of MD simulations of the rPPMEV-TLR2 and rPPMEV-TLR4 complexes are presented in Figure 8. With 100 ps of the time interval, the temperatures of the two simulation systems (rPPMEV-TLR2, rPPMEV-TLR4) are both around 300 K (Figures 8A,B), and the pressure of the two systems is around 1.4 atmosphere (Figure 8C) and 0.75 atmospheres (Figure 8D), respectively. These results indicate that the system is stable, and the MD operation is successful. In addition, during a 20 ns MD simulation, the RMSD value of the rPPMEV-TLR2 complex rises sharply to 0.4 nm in 2 ns and then remains at 0.43 nm (Figure 8E), while the RMSD value of the rPPMEV-TLR4 complex reveals a large fluctuation between 0 and 2 ns before being constant around 0.5 nm (Figure 8F). It was reported that the RMSD of the ligand is considered to be fixed within 1 nm, stable below 2 nm, and unstable above 2 nm during molecular docking (Eweas et al., 2021). The RMSD results of rPPMEV-TLR2 and rPPMEV-TLR4 are both less than 1 nm within 20 ns, indicating that the interaction of the two complexes at the docking interface is fixed. Furthermore, RMSF values demonstrate that the RMSF profiles of most amino acid residues of the rPPMEV-TLR2 complex (Figure 8G) and the rPPMEV-TLR4 complex (Figure 8H) are below 0.45 nm, and only a few residues have significant changes. These results prove that the two complexes have stability and stiffness.
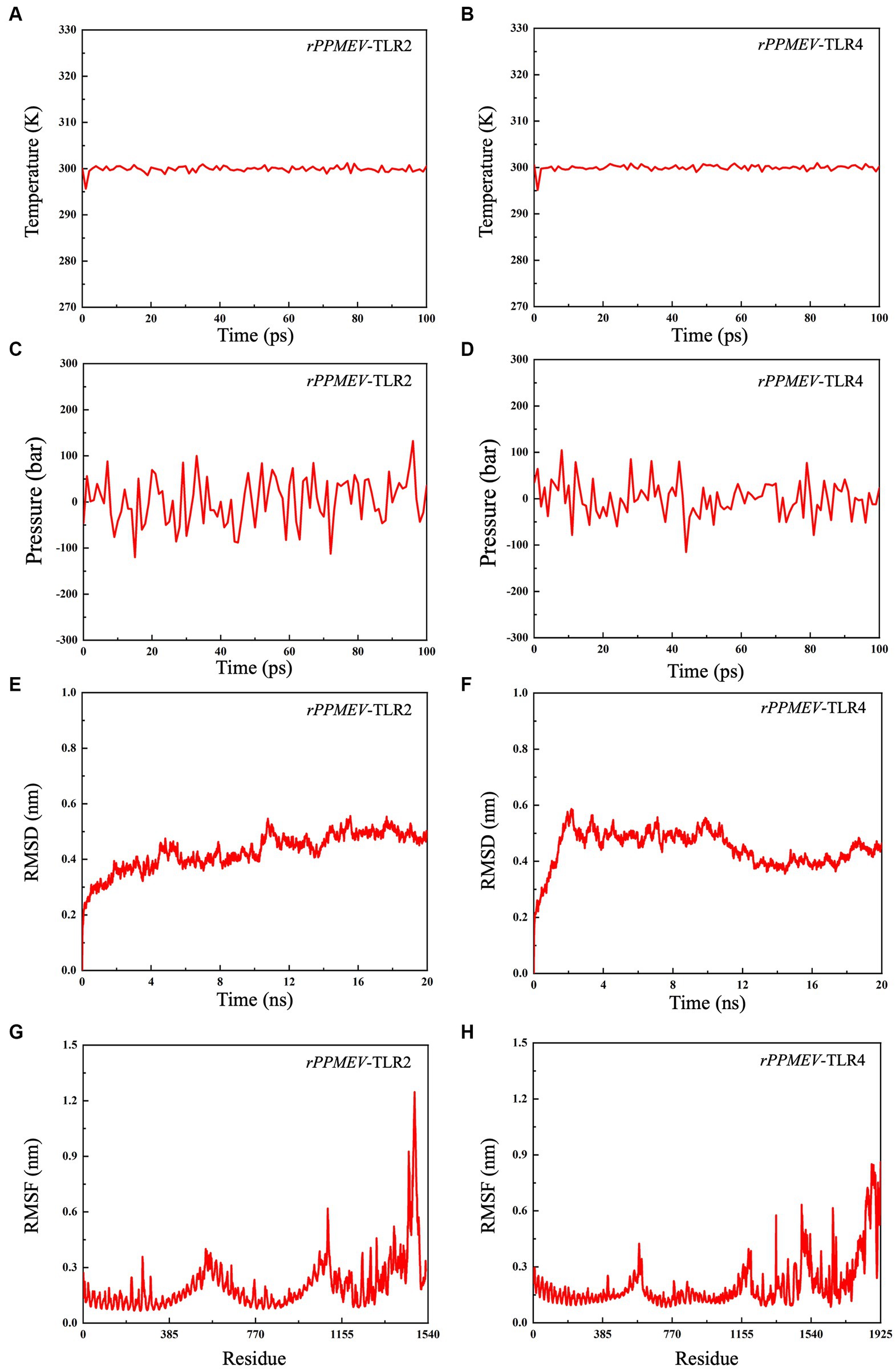
Figure 8. Molecular dynamics simulations between rPPMEV and TLRs. The temperature plots of the rPPMEV-TLR2 complex (A) and the rPPMEV-TLR4 complex (B). The pressure plots of the rPPMEV-TLR2 complex (C) and the rPPMEV-TLR4 complex (D). The RMSD analysis of the rPPMEV-TLR2 complex (E) and the rPPMEV-TLR4 complex (F). The RMSF analysis of the rPPMEV-TLR2 complex (G) and the rPPMEV-TLR4 complex (H).
3.7 Immune simulation
As an intracellular pathogen, cellular and humoral immunity induced by vaccines is essential for killing and eliminating viruses. To evaluate the immunological efficacy of rPPMEV, the immune stimulation of rPPMEV was performed by the C-ImmSim Server. The results show that the rPPMEV can induce three peaks in antibody levels after three vaccine doses, as shown in Figure 9A. The antibodies IgM + IgG, IgM, and IgG2 are found in the primary immunization. Further, as immune responses enhance, the levels of total IgM + IgG, IgM, and IgG1 + IgG2 antibodies elevate, indicating that antibody titers increase after the second and third injections. These increasing levels of antibodies in the immune response are mainly attributed to the increase in the total count of B-lymphocytes and T-lymphocytes. As shown in Figure 9B, the B cell population is highly stimulated upon immunization. Apart from B-lymphocytes, rPPMEV also induces the formation of three gradually rising peaks in the T helper (TH) (Figure 9C) cell and active TH cell (Figure 9D) populations after three injections, respectively. Moreover, the active cytotoxic T lymphocyte (TC cell) count sustains growth after each immunization (Figure 9E). In the end, as shown in Figure 9F, the concentrations of IFN-γ and IL-2 are both at high levels during each injection, indicating that rPPMEV may have the ability to induce a sufficient immune response (Kar et al., 2020).
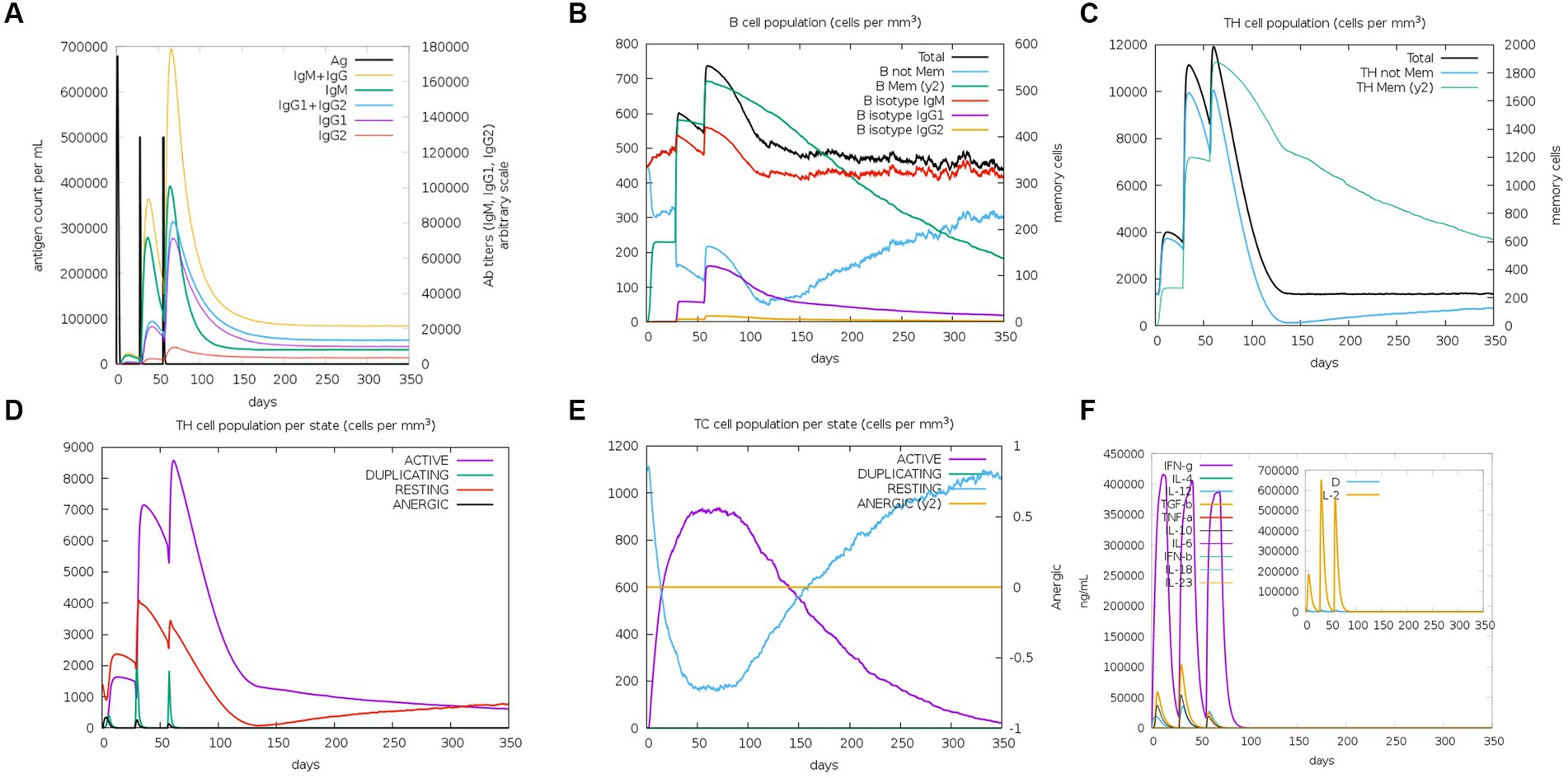
Figure 9. The immune simulated response spectrum of rPPMEV in the C-ImmSim server. (A) The production of various types of antibodies after vaccination. (B) The population of B cells after vaccination. (C) The population of helper T cells after vaccination. (D) The population of helper T cells in various states. (E) The population of cytotoxic T cells in various states. (F) Secretion levels of cytokines after vaccination.
3.8 Codon optimization and in silico cloning
To generate an appropriate plasmid construct harboring the vaccine construct sequence, codon optimization was embarked upon, as shown in Figure 10A. The results show that the improved sequence has a codon adaptation index (CAI) value of 0.98 and a GC content of 50.87, indicating that the protein of the vaccine has a high potential to be well expressed in E. coli (Ali et al., 2017). Subsequently, the improved sequence of 1,317 bases was cloned into the pET28a (+) vector between the XhoI and BamHI restriction sites using Snap-Gene software, as shown in Figure 10B.
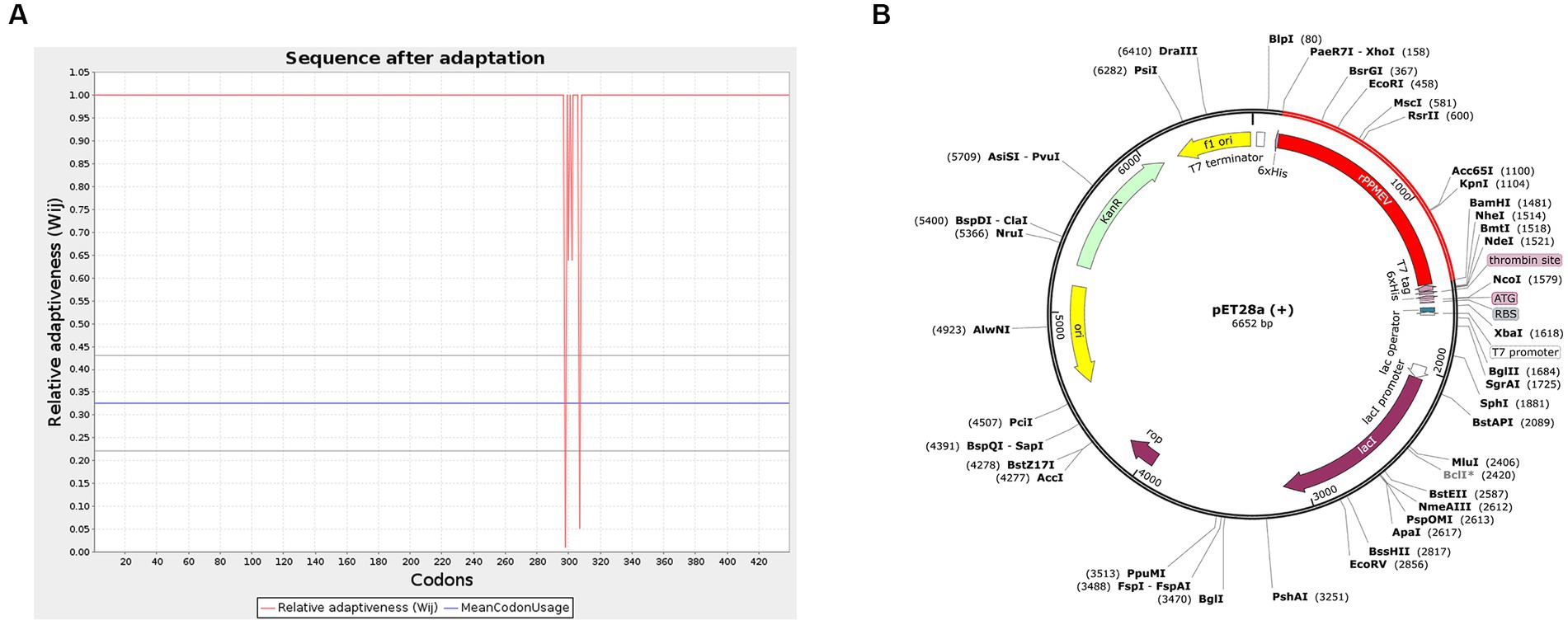
Figure 10. Codon optimization and in silico cloning. (A) The codon optimization of rPPMEV. (B) In silico cloning of rPPMEV in the pET28a (+) vector. The red areas represent the rPPMEV, while the black areas represent the pET28a (+) expression vector.
4 Conclusion
In summary, our study highlights a promising vaccine for PEDV and PDCoV prevention. The vaccine has several advantages. (1) The peptides of the vaccine, derived from the S proteins with the good immune activity of the current common PEDV and PDCoV strains, have a more promising protective effect for the host in either the case of the current epidemic PEDV or PDCoV infection alone or in the case of co-infection than the original peptide molecules for the prevention of PEDV or PDCoV alone. (2) The vaccine, which contains multiple MHC epitopes, the TLR2 agonist Pam2Cys, and The TLR4 agonist RS-09, could target antigen-presenting cells to initiate innate immune responses and provide high levels of either antibody production or cytotoxic cellular response. (3) The vaccine has strong immunogenicity, antigenicity, non-toxicity, and non-sensitization properties. The physicochemical and immunological properties of the vaccine are based on bioinformatics analysis. Although it was reported that the vaccines designed by this method have been proven to produce protective effects in vivo and some of them have entered the clinical trial stage (Mahmud et al., 2021), the efficacy evaluation of the vaccine rPPMEV still needs to be evaluated by in vivo and in vitro tests to finally prove the efficacy of this vaccine.
Data availability statement
The original contributions presented in the study are included in the article/Supplementary material, further inquiries can be directed to the corresponding author.
Author contributions
WeiH: Conceptualization, Funding acquisition, Investigation, Methodology, Writing – original draft, Writing – review & editing. HeW: Investigation, Writing – review & editing. WW: Investigation, Writing – review & editing. RW: Investigation, Writing – review & editing. WH: Investigation, Writing – review & editing. SW: Investigation, Writing – review & editing. BW: Investigation, Writing – review & editing. HaiW: Conceptualization, Funding acquisition, Investigation, Writing – review & editing.
Funding
The author(s) declare financial support was received for the research, authorship, and/or publication of this article. This study was supported by Fundamental Research Program of Shanxi Province (No. 202103021223168), the Shanxi Province Excellent Doctoral Work Award-Scientific Research Project (No. SXBYKY2022053), the Start-up Fund for Doctoral Research, Shanxi Agricultural University (No. 2021BQ71), Shanxi Provincial Key Research and Development Program (No. 202102140601020), the “Six New” Project of Agriculture and Rural Department of Shanxi Province, the Fund for Shanxi “1331 Project” Key Innovative Research Team (No. 20211331-16), the Fund for Shanxi “1331 Project” (No. 20211331-12).
Conflict of interest
The authors declare that the research was conducted in the absence of any commercial or financial relationships that could be construed as a potential conflict of interest.
Publisher’s note
All claims expressed in this article are solely those of the authors and do not necessarily represent those of their affiliated organizations, or those of the publisher, the editors and the reviewers. Any product that may be evaluated in this article, or claim that may be made by its manufacturer, is not guaranteed or endorsed by the publisher.
Supplementary material
The Supplementary material for this article can be found online at: https://www.frontiersin.org/articles/10.3389/fmicb.2023.1295678/full#supplementary-material
Footnotes
1. ^https://www.ncbi.nlm.nih.gov/gene/
2. ^http://www.cbs.dtu.dk/services/SignalP/
4. ^http://www.ddg-pharmfac.net/vaxijen/VaxiJen/VaxiJen.html
5. ^https://services.healthtech.dtu.dk/service.php?NetMHCIIpan-4.0
6. ^http://www.ddg-pharmfac.net/AllerTOP
7. ^https://web.expasy.org/protparam/
8. ^https://npsa-prabi.ibcp.fr/cgi-bin/npsa_automat.pl?page=npsa_sopma.html
9. ^https://robetta.bakerlab.org
10. ^http://galaxy.seoklab.org/
11. ^http://swissmodel/expasy.org/assess
12. ^https://prosa.services.came.sbg.ac.at/prosa.php
13. ^http://tools.iedb.org/ellipro/
14. ^https://cluspro.bu.edu/login.php?redir/queue.php
References
Albutti, A. (2021). An integrated computational framework to design a multi-epitopes vaccine against Mycobacterium tuberculosis. Sci. Rep. 11:21929. doi: 10.1038/s41598-021-01283-6
Ali, M., Pandey, R. K., Khatoon, N., Narula, A., Mishra, A., and Prajapati, V. K. (2017). Exploring dengue genome to construct a multi-epitope based subunit vaccine by utilizing immunoinformatics approach to battle against dengue infection. Sci. Rep. 7:9232. doi: 10.1038/s41598-017-09199-w
Almagro Armenteros, J. J., Tsirigos, K. D., Sønderby, C. K., Petersen, T. N., Winther, O., Brunak, S., et al. (2019). SignalP 5.0 improves signal peptide predictions using deep neural networks. Nat. Biotechnol. 37, 420–423. doi: 10.1038/s41587-019-0036-z
Baek, M., Dimaio, F., Anishchenko, I., Dauparas, J., Ovchinnikov, S., Lee, G. R., et al. (2021). Accurate prediction of protein structures and interactions using a three-track neural network. Science 373, 871–876. doi: 10.1126/science.abj8754
Barh, D., Barve, N., Gupta, K., Chandra, S., Jain, N., Tiwari, S., et al. (2013). Exoproteome and secretome derived broad spectrum novel drug and vaccine candidates in Vibrio cholerae targeted by Piper betel derived compounds. PLoS One 8:e52773. doi: 10.1371/journal.pone.0052773
Barton, G. M. (2007). Viral recognition by toll-like receptors. Semin. Immunol. 19, 33–40. doi: 10.1016/j.smim.2007.01.003
Bhatnager, R., Bhasin, M., Arora, J., and Dang, A. S. (2021). Epitope based peptide vaccine against SARS-COV2: an immune-informatics approach. J. Biomol. Struct. Dyn. 39, 5690–5705. doi: 10.1080/07391102.2020.1787227
Boehme, K. W., and Compton, T. (2004). Innate sensing of viruses by toll-like receptors. J. Virol. 78, 7867–7873. doi: 10.1128/JVI.78.15.7867-7873.2004
Borca, M. V., Ramirez-Medina, E., Silva, E., Vuono, E., Rai, A., Pruitt, S., et al. (2020). Development of a highly effective African swine fever virus vaccine by deletion of the I177L gene results in sterile immunity against the current epidemic Eurasia strain. J. Virol. 94, e02017–e02019. doi: 10.1128/JVI.02017-19
Chauhan, V., Rungta, T., Goyal, K., and Singh, M. P. (2019). Designing a multi-epitope based vaccine to combat Kaposi sarcoma utilizing immunoinformatics approach. Sci. Rep. 9:2517. doi: 10.1038/s41598-019-39299-8
Chen, X., Zaro, J. L., and Shen, W.-C. (2013). Fusion protein linkers: property, design and functionality. Adv. Drug Deliv. Rev. 65, 1357–1369. doi: 10.1016/j.addr.2012.09.039
Choudhury, A., Gupta, P. S. S., Panda, S. K., Rana, M. K., and Mukherjee, S. (2022). Designing AbhiSCoVac-A single potential vaccine for all “corona culprits”: Immunoinformatics and immune simulation approaches. J. Mol. Liq. 351:118633. doi: 10.1016/j.molliq.2022.118633
Corradin, G., Villard, V., and Kajava, A. V. (2007). Protein structure based strategies for antigen discovery and vaccine development against malaria and other pathogens. Endocr. Metab. Immune. Disord. Drug. Targets 7, 259–265. doi: 10.2174/187153007782794371
De Groot, A. S., Bosma, A., Chinai, N., Frost, J., Jesdale, B. M., Gonzalez, M. A., et al. (2001). From genome to vaccine: in silico predictions, ex vivo verification. Vaccine 19, 4385–4395. doi: 10.1016/s0264-410x(01)00145-1
Deléage, G. (2017). Alignsec: viewing protein secondary structure predictions within large multiple sequence alignments. Bioinformatics 33, 3991–3992. doi: 10.1093/bioinformatics/btx521
Dimitrov, I., Flower, D. R., and Doytchinova, I. (2013). AllerTOP-a server for in silico prediction of allergens. BMC Bioinformatics :S4. doi: 10.1186/1471-2105-14-S6-S4
Dong, R., Chu, Z., Yu, F., and Zha, Y. (2020). Contriving multi-epitope subunit of vaccine for COVID-19: immunoinformatics approaches. Front. Immunol. 11:1784. doi: 10.3389/fimmu.2020.01784
Eweas, A. F., Alhossary, A. A., and Abdel-Moneim, A. S. (2021). Molecular docking reveals ivermectin and remdesivir as potential repurposed drugs against SARS-CoV-2. Front. Microbiol. 11:592908. doi: 10.3389/fmicb.2020.592908
Fleri, W., Paul, S., Dhanda, S. K., Mahajan, S., Xu, X., Peters, B., et al. (2017). The immune epitope database and analysis resource in epitope discovery and synthetic vaccine design. Front. Immunol. 8:278. doi: 10.3389/fimmu.2017.00278
Frankel, A. D., and Pabo, C. O. (1988). Cellular uptake of the tat protein from human immunodeficiency virus. Cells 55, 1189–1193. doi: 10.1016/0092-8674(88)90263-2
Greenbaum, J. A., Andersen, P. H., Blythe, M., Bui, H. H., Cachau, R. E., Crowe, J., et al. (2007). Towards a consensus on datasets and evaluation metrics for developing B-cell epitope prediction tools. J. Mol. Recognit. 20, 75–82. doi: 10.1002/jmr.815
Grifoni, A., Sidney, J., Zhang, Y., Scheuermann, R. H., Peters, B., and Sette, A. (2020). A sequence homology and bioinformatic approach can predict candidate targets for immune responses to SARS-CoV-2. Cell Host Microbe 27, 671–680.e2. doi: 10.1016/j.chom.2020.03.002
Grote, A., Hiller, K., Scheer, M., Münch, R., Nörtemann, B., Hempel, D. C., et al. (2005). JCat: a novel tool to adapt codon usage of a target gene to its potential expression host. Nucleic Acids Res. 33, W526–W531. doi: 10.1093/nar/gki376
Gupta, S., Kapoor, P., Chaudhary, K., Gautam, A., Kumar, R., Consortium, O. S. D. D., et al. (2013). In silico approach for predicting toxicity of peptides and proteins. PLoS One 8:e73957. doi: 10.1371/journal.pone.0073957
He, J., Huang, F., Zhang, J., Chen, Q., Zheng, Z., Zhou, Q., et al. (2021). Vaccine design based on 16 epitopes of SARS-CoV-2 spike protein. J. Med. Virol. 93, 2115–2131. doi: 10.1002/jmv.26596
Hou, W., Wu, H., Wang, S., Wang, W., Wang, B., and Wang, H. (2023). Designing a multi-epitope vaccine to control porcine epidemic diarrhea virus infection using immunoinformatics approaches. Front. Microbiol. 14:1264612. doi: 10.3389/fmicb.2023.1264612
Ikai, A. (1980). Thermostability and aliphatic index of globular proteins. J. Biochem. 88, 1895–1898.
Ismail, S., Ahmad, S., and Azam, S. S. (2020). Immunoinformatics characterization of SARS-CoV-2 spike glycoprotein for prioritization of epitope based multivalent peptide vaccine. J. Mol. Liq. 314:113612. doi: 10.1016/j.molliq.2020.113612
Jackson, D. C., Lau, Y. F., Le, T., Suhrbier, A., Deliyannis, G., Cheers, C., et al. (2004). A totally synthetic vaccine of generic structure that targets toll-like receptor 2 on dendritic cells and promotes antibody or cytotoxic T cell responses. Proc. Natl. Acad. Sci. U. S. A. 101, 15440–15445. doi: 10.1073/pnas.0406740101
Jiang, F., Liu, Y., Xue, Y., Cheng, P., Wang, J., Lian, J., et al. (2023). Developing a multiepitope vaccine for the prevention of SARS-CoV-2 and monkeypox virus co-infection: a reverse vaccinology analysis. Int. Immunopharmacol. 115:109728. doi: 10.1016/j.intimp.2023.109728
Jiao, Z., Liang, J., Yang, Y., Li, Y., Yan, Z., Hu, G., et al. (2021). Coinfection of porcine deltacoronavirus and porcine epidemic diarrhea virus altered viral tropism in gastrointestinal tract in a piglet model. Virology 558, 119–125. doi: 10.1016/j.virol.2021.03.006
Kar, T., Narsaria, U., Basak, S., Deb, D., Castiglione, F., Mueller, D. M., et al. (2020). A candidate multi-epitope vaccine against SARS-CoV-2. Sci. Rep. 10:10895. doi: 10.1038/s41598-020-67749-1
Khan, M. T., Islam, M. J., Parihar, A., Islam, R., Jerin, T. J., Dhote, R., et al. (2021). Immunoinformatics and molecular modeling approach to design universal multi-epitope vaccine for SARS-CoV-2. Inform. Med. Unlocked 24:100578. doi: 10.1016/j.imu.2021.100578
Khan, A., Junaid, M., Kaushik, A. C., Ali, A., Ali, S. S., Mehmood, A., et al. (2018). Computational identification, characterization and validation of potential antigenic peptide vaccines from hrHPVs E6 proteins using immunoinformatics and computational systems biology approaches. PLoS One 13:e0196484. doi: 10.1371/journal.pone.0196484
Kong, F., Wang, Q., Kenney, S. P., Jung, K., Vlasova, A. N., and Saif, L. J. (2022). Porcine deltacoronaviruses: origin, evolution, cross-species transmission and zoonotic potential. Pathogens 11:79. doi: 10.3390/pathogens11010079
Koonpaew, S., Teeravechyan, S., Frantz, P. N., Chailangkarn, T., and Jongkaewwattana, A. (2019). PEDV and PDCoV pathogenesis: the interplay between host innate immune responses and porcine enteric coronaviruses. Front. Vet. Sci. 6:34. doi: 10.3389/fvets.2019.00034
Kozakov, D., Hall, D. R., Xia, B., Porter, K. A., Padhorny, D., Yueh, C., et al. (2017). The ClusPro web server for protein–protein docking. Nat. Protoc. 12, 255–278. doi: 10.1038/nprot.2016.169
Kurt-Jones, E. A., Popova, L., Kwinn, L., Haynes, L. M., Jones, L. P., Tripp, R. A., et al. (2000). Pattern recognition receptors TLR4 and CD14 mediate response to respiratory syncytial virus. Nat. Immunol. 1, 398–401. doi: 10.1038/80833
Li, Y., Liu, X., Zhu, Y., Zhou, X., Cao, C., Hu, X., et al. (2013). Bioinformatic prediction of epitopes in the Emy162 antigen of Echinococcus multilocularis. Exp. Ther. Med. 6, 335–340. doi: 10.3892/etm.2013.1142
Li, Y., Wang, J., Hou, W., Shan, Y., Wang, S., and Liu, F. (2021). Dynamic dissection of the endocytosis of porcine epidemic diarrhea coronavirus cooperatively mediated by clathrin and caveolae as visualized by single-virus tracking. mBio 12, e00256–e00221. doi: 10.1128/mBio.00256-21
Liu, H. A.-O., Yin, X., Tian, H., Qiu, Y., Wang, Z., Chen, J., et al. (2022). The S protein of a novel recombinant PEDV strain promotes the infectivity and pathogenicity of PEDV in mid-West China. Transbound. Emerg. Dis. 69, 3704–3723. doi: 10.1111/tbed.14740
Mahmud, S., Rafi, M. O., Paul, G. K., Promi, M. M., Shimu, M., Sultana, S., et al. (2021). Designing a multi-epitope vaccine candidate to combat MERS-CoV by employing an immunoinformatics approach. Sci. Rep. 11:15431. doi: 10.1038/s41598-021-92176-1
Meza, B., Ascencio, F., Sierra-Beltrán, A. P., Torres, J., and Angulo, C. (2017). A novel design of a multi-antigenic, multistage and multi-epitope vaccine against Helicobacter pylori: an in silico approach. Infect. Genet. Evol. 49, 309–317. doi: 10.1016/j.meegid.2017.02.007
Moise, L., Gutiérrez, A. H., Khan, S., Tan, S., Ardito, M., Martin, W. D., et al. (2020). New immunoinformatics tools for swine: designing epitope-driven vaccines, predicting vaccine efficacy, and making vaccines on demand. Front. Immunol. 11:563362. doi: 10.3389/fimmu.2020.563362
Nabel, G. J. (2002). HIV vaccine strategies. Vaccine 20, 1945–1947. doi: 10.1016/s0264-410x(02)00074-9
Ponomarenko, J., Bui, H.-H., Li, W., Fusseder, N., Bourne, P. E., Sette, A., et al. (2008). ElliPro: a new structure-based tool for the prediction of antibody epitopes. BMC Bioinformatics 9:514. doi: 10.1186/1471-2105-9-514
Puigbo, P., Romeu, A., and Garcia-Vallve, S. (2007). HEG-DB: a database of predicted highly expressed genes in prokaryotic complete genomes under translational selection. Nucleic Acids Res. 36, D524–D527. doi: 10.1093/nar/gkm831
Rapin, N., Lund, O., Bernaschi, M., and Castiglione, F. (2010). Computational immunology meets bioinformatics: the use of prediction tools for molecular binding in the simulation of the immune system. PLoS One 5:e9862. doi: 10.1371/journal.pone.0009862
Rock, D. (2017). Challenges for African swine fever vaccine development—… perhaps the end of the beginning. Vet. Microbiol. 206, 52–58. doi: 10.1016/j.vetmic.2016.10.003
Ros-Lucas, A., Correa-Fiz, F., Bosch-Camós, L., Rodriguez, F., and Alonso-Padilla, J. (2020). Computational analysis of African swine fever virus protein space for the design of an epitope-based vaccine ensemble. Pathogens 9:1078. doi: 10.3390/pathogens9121078
Rowaiye, A. B., Oli, A. N., Asala, M. T., Nwonu, E. J., Njoku, M. O., Asala, O. O., et al. (2023). Design of multiepitope vaccine candidate from a major capsid protein of the African swine fever virus. Vet. Vaccine 2:100013. doi: 10.1016/j.vetvac.2023.100013
Saadi, M., Karkhah, A., and Nouri, H. R. (2017). Development of a multi-epitope peptide vaccine inducing robust T cell responses against brucellosis using immunoinformatics based approaches. Infect. Genet. Evol. 51, 227–234. doi: 10.1016/j.meegid.2017.04.009
Sarkar, B., Ullah, M. A., Araf, Y., Islam, N. N., and Zohora, U. S. (2022). Immunoinformatics-guided designing and in silico analysis of epitope-based polyvalent vaccines against multiple strains of human coronavirus (HCoV). Expert Rev. Vaccines 21, 1851–1871. doi: 10.1080/14760584.2021.1874925
Scheiblhofer, S., Laimer, J., Machado, Y., Weiss, R., and Thalhamer, J. (2017). Influence of protein fold stability on immunogenicity and its implications for vaccine design. Expert Rev. Vaccines 16, 479–489. doi: 10.1080/14760584.2017.1306441
Sha, T., Li, Z., Zhang, C., Zhao, X., Chen, Z., Zhang, F., et al. (2020). Bioinformatics analysis of candidate proteins Omp2b, P39 and BLS for Brucella multivalent epitope vaccines. Microb. Pathog. 147:104318. doi: 10.1016/j.micpath.2020.104318
Shantier, S. W., Mustafa, M. I., Abdelmoneim, A. H., Fadl, H. A., Elbager, S. G., and Makhawi, A. M. (2022). Novel multi epitope-based vaccine against monkeypox virus: vaccinomic approach. Sci. Rep. 12:15983. doi: 10.1038/s41598-022-20397-z
Shukla, R., Shukla, H., Sonkar, A., Pandey, T., and Tripathi, T. (2018). Structure-based screening and molecular dynamics simulations offer novel natural compounds as potential inhibitors of Mycobacterium tuberculosis isocitrate lyase. J. Biomol. Struct. Dyn. 36, 2045–2057. doi: 10.1080/07391102.2017.1341337
Sun, D., Feng, L., Shi, H., Chen, J., Cui, X., Chen, H., et al. (2008). Identification of two novel B cell epitopes on porcine epidemic diarrhea virus spike protein. Vet. Microbiol. 131, 73–81. doi: 10.1016/j.vetmic.2008.02.022
Sun, Q., Huang, Z., Yang, S., Li, Y., Ma, Y., Yang, F., et al. (2022). Bioinformatics-based SARS-CoV-2 epitopes design and the impact of spike protein mutants on epitope humoral immunities. Immunobiology 227:152287. doi: 10.1016/j.imbio.2022.152287
Tahir ul Qamar, M., Shokat, M., Muneer I, Z., Ashfaq, U. A., Javed, H., Bari, A. F., et al. (2020). Multiepitope-based subunit vaccine design and evaluation against respiratory syncytial virus using reverse vaccinology approach. Vaccines 8:288. doi: 10.3390/vaccines8020288
Takeda, K., and Akira, S. (2004). TLR signaling pathways. Semin. Immunol. 16, 3–9. doi: 10.1016/j.smim.2003.10.003
Tang, P., Cui, E., Song, Y., Yan, R., and Wang, J. (2021). Porcine deltacoronavirus and its prevalence in China: a review of epidemiology, evolution, and vaccine development. Arch. Virol. 166, 2975–2988. doi: 10.1007/s00705-021-05226-4
Temeeyasen, G., Sinha, A., Gimenez-Lirola, L., Zhang, J., and Piñeyro, P. (2018). Differential gene modulation of pattern-recognition receptor TLR and RIG-I-like and downstream mediators on intestinal mucosa of pigs infected with PEDV non S-INDEL and PEDV S-INDEL strains. Virology 517, 188–198. doi: 10.1016/j.virol.2017.11.024
Trovato, M., Sartorius, R., D’Apice, L., Manco, R., and De Berardinis, P. (2020). Viral emerging diseases: challenges in developing vaccination strategies. Front. Immunol. 11:2130. doi: 10.3389/fimmu.2020.02130
Wang, D., Fang, L., and Xiao, S. (2016). Porcine epidemic diarrhea in China. Virus Res. 226, 7–13. doi: 10.1016/j.virusres.2016.05.026
Waterhouse, A., Bertoni, M., Bienert, S., Studer, G., Tauriello, G., Gumienny, R., et al. (2018). Swiss-model: homology modelling of protein structures and complexes. Nucleic Acids Res. 46, W296–W303. doi: 10.1093/nar/gky427
Wiederstein, M., and Sippl, M. J. (2007). ProSA-web: interactive web service for the recognition of errors in three-dimensional structures of proteins. Nucleic Acids Res. 35, W407–W410. doi: 10.1093/nar/gkm290
Yao, X., Qiao, W.-T., Zhang, Y.-Q., Lu, W.-H., Wang, Z.-W., Li, H.-X., et al. (2023). A new PEDV strain CH/HLJJS/2022 can challenge current detection methods and vaccines. Virol. J. 20:13. doi: 10.1186/s12985-023-01961-z
Yu, M., Zhu, Y., Li, Y., Chen, Z., Li, Z., Wang, J., et al. (2022). Design of a recombinant multivalent epitope vaccine based on SARS-CoV-2 and its variants in immunoinformatics approaches. Front. Immunol. 13:884433. doi: 10.3389/fimmu.2022.884433
Zhai, K., Zhang, Z., Liu, X., Lv, J., Zhang, L., Li, J., et al. (2023). Mucosal immune responses induced by oral administration of recombinant Lactococcus lactis expressing the S1 protein of PDCoV. Virology 578, 180–189. doi: 10.1016/j.virol.2022.12.010
Keywords: PEDV, PDCoV, vaccine, multi-epitope, immunoinformatics
Citation: Hou W, Wu H, Wang W, Wang R, Han W, Wang S, Wang B and Wang H (2023) Developing a multi-epitope vaccine candidate to combat porcine epidemic diarrhea virus and porcine deltacoronavirus co-infection by employing an immunoinformatics approach. Front. Microbiol. 14:1295678. doi: 10.3389/fmicb.2023.1295678
Edited by:
Rajesh Kumar Pathak, Chung-Ang University, Republic of KoreaReviewed by:
Kalpana Singh, Guru Angad Dev Veterinary and Animal Sciences University, IndiaNadeem Shabir, Sher-e-Kashmir University of Agricultural Sciences and Technology, India
Mugunthan Susithra Priyadarshni, Thiruvalluvar University, India
Copyright © 2023 Hou, Wu, Wang, Wang, Han, Wang, Wang and Wang. This is an open-access article distributed under the terms of the Creative Commons Attribution License (CC BY). The use, distribution or reproduction in other forums is permitted, provided the original author(s) and the copyright owner(s) are credited and that the original publication in this journal is cited, in accordance with accepted academic practice. No use, distribution or reproduction is permitted which does not comply with these terms.
*Correspondence: Haidong Wang, wanghaidong@sxau.edu.cn