- 1Key Laboratory of Breeding Biotechnology and Sustainable Aquaculture, Institute of Hydrobiology, Chinese Academy of Sciences, Wuhan, Hubei, China
- 2College of Advanced Agricultural Sciences, University of Chinese Academy of Sciences, Beijing, China
Introduction: Parasitic ciliates are protozoans with a global distribution. Along with the gut microbiota, they have formed a micro-ecosystem that affects the host’s nutrition, metabolism, and immunity. The interactions and relationships among the three components of this microecosystem (protozoa, gut microbiota, and host) remain only partially understood. Xenocypris fish and the unique ciliate Balantidium polyvacuolum in its hindgut are good materials to study the interplay.
Methods: In this study, 16S rRNA gene amplicon sequencing and short-chain fatty acids (SCFAs) identification were used. Network was also constructed to understand their relationships.
Results: We found that the gut microbiota of B. polyvacuolum-infected X. davidi and X. argentea had higher diversity, richness, and evenness than uninfected ones. B. polyvacuolum could lead to an increase of Fusobacterium and Chloroflexi in both X. davidi and X. argentea, while significantly increase the abundance of genera Romboutsia and Clostridium in X. argentea. Besides, B. polyvacuolum could significantly increase the content of total SCFAs and acetic acid in X. davidi and increase the concentrations of propionic, isobutyric and butanoic acids in X. argentea. Furthermore, correlation analyses showed that B. polyvacuolum may alter SCFAs by affecting key SCFAs-producing bacteria such as Clostridium and Cetobacterium.
Discussion: This study greatly expands our understanding of relationships among B. polyvacuolum, gut microbiota and host Xenocypris fish, which sheds new insights into the mechanism of interaction among protozoa, gut microbiota and host.
1 Introduction
Protozoa are single-celled organisms with a wide distribution, many of whom have adopted a parasitic lifestyle. These parasitic protozoa are capable of infecting a diverse array of hosts, including humans (Burgess et al., 2017), birds, reptiles (Koèíková et al., 2018), amphibians (Zhao et al., 2022a), fish (Zhao et al., 2022b), and insects (de Graaf et al., 2011). They normally live in the intestinal ecosystems, and cause a significant impact on the host (Burgess et al., 2017; Li et al., 2018). These intestinal parasites directly interact with the densely populated gut symbiotic bacteria, which may influence the composition of the gut microbiota. Evidence shows that protists from the genus Blastocystis can increase bacterial alpha diversity in the human intestine (Nieves-Ramírez et al., 2018). Colonization by the protozoan Tritrichomonas musculis can lead to inflammasome activation and thus contribute to the host’s protection against mucosal bacterial infections (Chudnovskiy et al., 2016). Other types of parasites, like helminths and cestodes, can also affect the diversity and structure of gut microbiota (Kreisinger et al., 2015; Brealey et al., 2022). Overall, the relationship between parasitic protozoa and gut microbiota remains incompletely understood, and further investigations are needed.
Gut microbiota plays a critical role in homeostasis by creating a mucosal barrier, offering defense against pathogens, and influencing the hosts’ metabolism, nutrition, and immunity (Leung et al., 2018; Ulusan Bagci and Caner, 2022). Most of its contribution to host physiology is related to its metabolism (Consortium, 2012). The metabolism of substrates can produce beneficial metabolites like bile acids, choline, and short-chain fatty acids (SCFAs), which are essential for the host’s health and can influence the host’s immune system (Nicholson et al., 2012). The SCFAs are produced via fermentation of amino acids or carbohydrates like glucose, starch, fiber, etc. (Horiuchi et al., 2020; Krautkramer et al., 2021; Wang et al., 2022). This fermentation can strongly expand the host’s metabolic capacity (Kaoutari et al., 2013; Krautkramer et al., 2021). Considering the cohabitation of gut microbiota and intestinal protozoa, we hypothesize that there must be some complex connection among the three components of this microecosystem (protozoa, gut microbiota, and host), which remains unknown.
Balantidium polyvacuolum is a single-celled organism characterized by numerous contractile vacuoles inside its body (Li et al., 2009). It has strict host specificity to Xenocyprinae fishes and mainly inhabits the mucosal folds of the hindgut (Li et al., 2009), so it’s a good material to study the interplay among protozoa, gut microbiota, and host. Up to now, there was only one report about the gut microbial communities of X. argentea (Yang et al., 2022). Herein, we applied the 16S rRNA gene amplicon sequencing of the gut microbiota and SCFAs identification in X. davidi and X. argentea, and then applied microbial co-occurrence network, random forest models, and correlation analysis to explore the relationships among the three components.
2 Materials and methods
2.1 Sample collection
Host fish X. davidi (infection n = 6, control n = 4, average weight 113.9 ± 17.4 g) and X. argentea (infection n = 6, control n = 3, average weight 123.6 ± 29.2 g) were collected from the Wuhan Section of the Yangtze River in Xianning City, Hubei Province, China, in July 2022. Fishes were anesthetized using 0.02% tricaine methane sulfonate (MS-222, Sigma) according to the manufacturer’s protocol and dissected in accordance with the protocols approved by the Animal Ethics Committee of Institute of Hydrobiology, Chinese Academy of Sciences (IHB/LL/2023036). The hindguts were removed under aseptic conditions. After microscopic examination to confirm the presence of B. polyvacuolum, the contents of the hindgut were harvested, and subsequently used for the microbiota analysis and SCFAs analysis.
2.2 DNA extraction and 16S rRNA gene amplicon sequencing
Intestinal microbiota DNA was extracted using the QIAamp DNA Stool Mini Kit (Qiagen, Germany) according to the manufacturer’s instructions. The quality and concentration of DNA were determined by 1.0% agarose gel electrophoresis and a NanoDrop® ND-2000 spectrophotometer (Thermo Scientific Inc., USA). The DNA samples were kept at −80°C until further use. The hypervariable region V3-V4 of the bacterial 16S rRNA gene was amplified with primer pairs 338F and 806R using an ABI GeneAmp® 9700 PCR thermocycler (ABI, CA, USA). Purified amplicons were pooled in equimolar amounts and paired-end sequenced on an Illumina NovaSeq PE250 platform according to the standard protocols by Majorbio Bio-Pharm Technology Co. Ltd. (Shanghai, China). The raw sequencing reads were deposited into the NCBI Sequence Read Archive (SRA) database under the BioSample number PRJNA981639.
2.3 Extraction and identification of SCFAs
For the SCFAs extraction, 20 mg of intestinal contents were placed into 2 mL grinding tubes and 800 μL of water containing 0.5% phosphoric acid was added. The samples were frozen and ground at 50 Hz for 3 min repeated twice, followed by ultrasonic for 10 min, and centrifugation at 4°C and 13000 × g for 15 min. Two hundred μL of the supernatant aqueous solution was removed into a 1.5 mL centrifuge tube. After that, 200 μL of N-butanol solvent containing internal standard 2-ethylbutyric acid (10 μg/mL) was added. After vortexing for 10 s, exposing to ultrasound at a low temperature for 10 min, and centrifuging at 4°C and 13000 × g for 5 min, the supernatant was carefully transferred to sample vials. The gas chromatography-mass spectrometry (GC-MS) analysis was conducted on an Agilent 8890B gas chromatography coupled to an Agilent 8890B/5977B mass selective detector with an inert electron impact (EI) ionization source and the ionization voltage of 70eV (Agilent, USA). Analyte compounds were separated with an HP-FFAP (30 m × 0.25 mm × 0.25 μm) capillary column, using 99.999% helium as a carrier gas at a constant flow rate (1 mL/min). The GC column temperature was programmed to hold at 80°C and rise to 120°C at a rate of 20°C per minute, then rise to 160°C at a rate of 5°C per minute, and finally hold at 220°C for 3 min. The injection volume of samples was 1 μL and introduced in splitting mode (10:1) with the inlet temperature of 180°C. The ion sources temperature was 230°C and the quadrupole temperature was 150°C. GC-MS was in selected-ion monitoring mode. Compounds were identified and quantified by the Masshunter software (v10.0.707.0, Agilent, USA). The mass spectrum peak area of the analyte was used as the ordinate and the concentration of the analyte as the abscissa to draw a linear regression standard curve for sample concentration calculation: the mass spectrum peak area of the sample analyte was substituted into the linear equation to calculate the concentration result.
2.4 Analysis of 16S rRNA gene sequences
The data were analyzed through the free online platform of majorbio choud platform.1 Low-quality reads (length <50 bp or quality score of <20) were removed from raw reads by fastp V.0.19.6 (Chen et al., 2018). The clean data were then assembled using FLASH V.1.2.11 (Magoè and Salzberg, 2011). Then, the optimized sequences were clustered into operational taxonomic units (OTUs) using UPARSE V.7.1 (Edgar, 2013) at a 97% sequence similarity level. The most abundant sequence for each OTU was selected as a representative sequence. The taxonomy of each OTU representative sequence was analyzed by RDP Classifier V.2.2 (Wang et al., 2007) against the Silva database V.123 using a confidence threshold of 0.7.
The following analysis and visualization were performed in RStudio V.4.1.1 (R Core Team, 2019). First, the relative abundance of microbial taxa was compared among different groups at phylum and genus levels. Then, the α diversity indices including Richness, ACE, and Shannon were calculated. For the β diversity analysis, non-metric multi-dimensional scale analysis (NMDS) based on the Bray-Curtis distance algorithm also was used to show the distribution and relationship among samples. Linear discriminant analysis coupled with effect size (LEfSe) was used to find species with significant differences at the genus level. Meanwhile, random forest analysis (RFA) was used to classify the most discriminant genera. Finally, Spearman’s correlation between the discriminant genera of infected and uninfected groups selected by RFA was analyzed.
2.5 Construction of the microbial network
The Molecular Ecological Network Analysis (MENA) pipeline2 was used to construct networks as previously described (Deng et al., 2012). First, only the top 100 dominant OTUs in each group were used for the network construction. Second, the Pearson correlation was calculated based on the log-transformed OTU abundances and then only relationships with a correlation coefficient r ≥ 0.2 and p-value < 0.05 were used to construct networks.
Global network indexes, including the average clustering coefficient, average path length, connectivity, and modularity were calculated as described previously (Deng et al., 2012). The topological roles of nodes in networks were evaluated and divided into four types based on the within-module connectivity (Zi) and among-module connectivity (Pi), which were network hubs (Zi > 2.5, Pi > 0.62), module hubs (Zi > 2.5, Pi ≤ 0.62), connectors (Zi ≤ 2.5, Pi > 0.62), and peripheral nodes (Zi ≤ 2.5, Pi ≤ 0.62). Network hubs, module hubs, and connectors can be seen as key nodes in network structuring (Olesen et al., 2007). Networks were visualized by Gephi software V0.10 (Bastian et al., 2009).
2.6 Analysis of SCFAs
The Shapiro and Bartlett tests were used to test the normality and homogeneity of variances, respectively. Then appropriate testing methods were chosen for the inter-group difference testing. Results were visualized with the ggplot2 package (Villanueva and Chen, 2019) in R (R Core Team, 2019).
2.7 Correlation analysis of microbiota and SCFAs
First, we used only the discriminant genera selected by RFA for the network construction. Second, Spearman’s correlation coefficient was calculated and only relationships with the p-value ≤ 0.05 were kept for the following analysis. Then, node properties were calculated. The correlation networks were visualized by the igraph package (Csardi and Nepusz, 2006) in R (R Core Team, 2019).
3 Results
3.1 Impact of B. polyvacuolum on gut microbiota
At the phylum level, Firmicutes, Proteobacteria, Actinobacteriota, Cyanobacteria and Chloroflexi were the top five relatively abundant taxa in both B. polyvacuolum-infected X. davidi (Xd+) and uninfected X. davidi (Xd-). In the B. polyvacuolum-infected X. argentea (Xa+), Firmicutes, Proteobacteria, Fusobacteriota, Actinobacteriota, and Chloroflexi were the top five relatively abundant taxa, while Firmicutes and Proteobacteria took the large part in the uninfected X. argentea (Xa-) (Figure 1A). The relative abundance of Chloroflexi was significantly higher (p = 0.034) in Xa+ than in Xa-, while Fusobacteriota was higher in both Xd+ and Xa+. Regardless of the B. polyvacuolum infections, the relative abundance of Proteobacteria was higher in X. argentea compared to X. davidi.
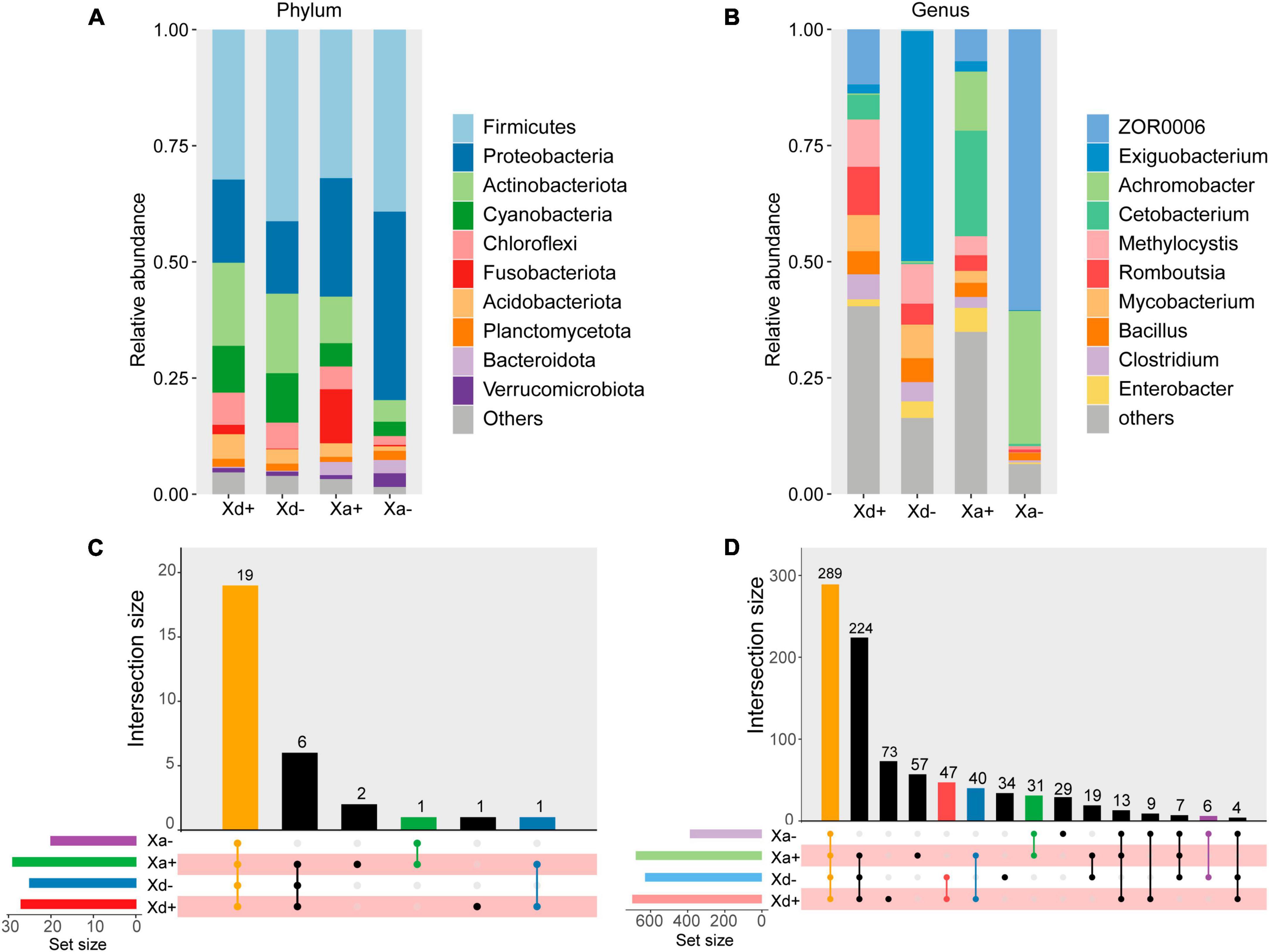
Figure 1. The relative abundance of microbial taxa at phylum and genus levels and the species composition of each group. (A) X. davidi infected with B. polyvacuolum (Xd+) and without B. polyvacuolum (Xd-). “Others” comprises the sum of different taxa, aside from the top 10 most abundant categories. (B) X. argentea infected with B. polyvacuolum (Xa+) and without B. polyvacuolum (Xa-). “Others” comprises unclassified genera in the top eighteen abundant categories. Upset diagrams showing the species composition in four groups at the phylum (C) and genus (D) levels. The data for drawing can be found in Supplementary Tables 1–4.
At the genus level, ZOR0006, Methylocystis, Romboutsia, and Mycobacterium were the top four relatively abundant taxa in Xd+ , while Exiguobacterium was abundant in Xd-. In Xa+, Cetobacterium, Achromobacter, and ZOR0006 were the top three relatively abundant taxa, while ZOR0006 and Achromobacter were abundant in Xa- (Figure 1B). The abundance of Romboutsia and Clostridium was significantly higher in Xa+ than in Xa- (p = 0.048 and 0.033, respectively), while the level of ZOR006 was significantly higher in Xa- (p = 0.024). The abundance of Exiguobacterium was significantly higher in Xd- than in Xd+ (p = 0.019). The four groups had 19 phyla and 289 genera in common (Figures 1C, D). The number of genera shared by Xd+ and Xa+ was 40. The total numbers of genera identified in groups Xd+, Xd-, Xa+, and Xa- were 458, 395, 436, and 364, respectively.
As for the alpha diversity, richness, ACE, and Shannon indices were calculated (Figures 2A–C). There were no significant differences between Xd+ and Xd- or between Xa+ and Xa-. Same for the beta diversity (Figure 2D). NMDS results showed that samples from different groups were scattered in different regions, except for partial overlaps (Figure 2E). Most of these overlaps occurred between the same species of fish (Xd+ /Xd- or Xa+ /Xa-). The ANOSM results also showed significant differences between groups (Figure 2F).
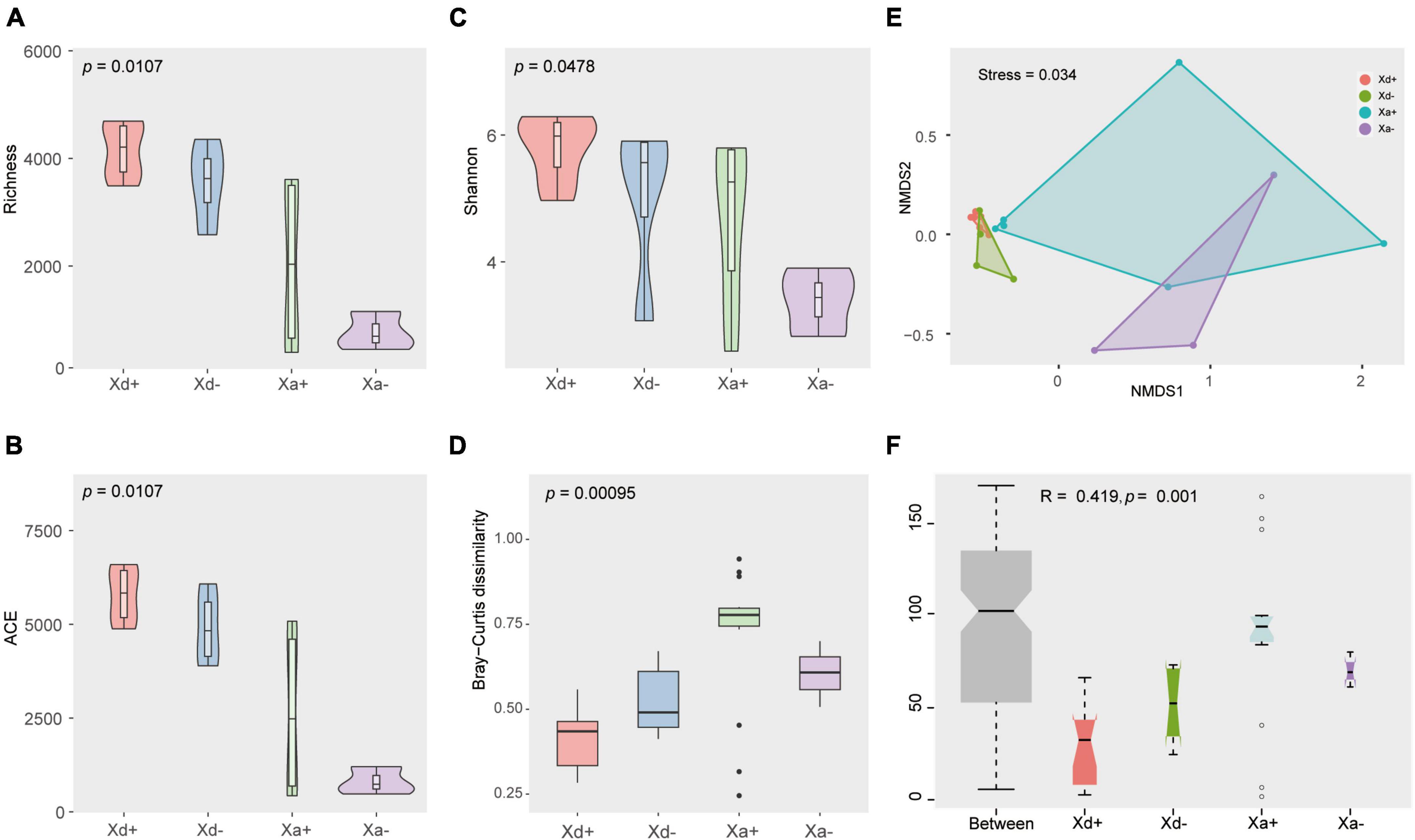
Figure 2. The structural features of the gut microbiota in four groups. Richness (A), ACE (B), and Shannon (C) indices of the alpha diversity. (D) Beta diversity. (E) Non-metric multi-dimensional scale analysis (NMDS) showing the distribution and relationship among samples. Dots represent samples. (F) ANOSIM results. p < 0.05 indicates a significant difference. The data for drawing can be found in Supplementary Tables 5–7.
Genera exhibiting significant differences among groups were screened by the two different methods LEfSe and RFA. The LEfSe analysis found that 18 taxa (Xd+ vs. Xd-) and 20 taxa (Xa+ vs. Xa-) showed significantly differences (Figures 3A, B). Meanwhile, the RFA showed that the significantly different taxa were 25 (Xd+ vs. Xd-) and 23 (Xa+ vs. Xa-), respectively (Figures 3C, D). Both analyses identified Hyphomicrobium, Methylomagnum, Pedomicrobium, Caldibacillus, and Collinsella (Xd+ vs. Xd-), and Crossiella, Defluviimonas, Geminocystis, and Phreatobacter (Xa+ vs. Xa-) as significantly different genera. Compared to respective control group, most of the genus were negatively correlated in Xd+ (Figures 4A, B), while positively corelated in Xa+ (Figures 4C, D).
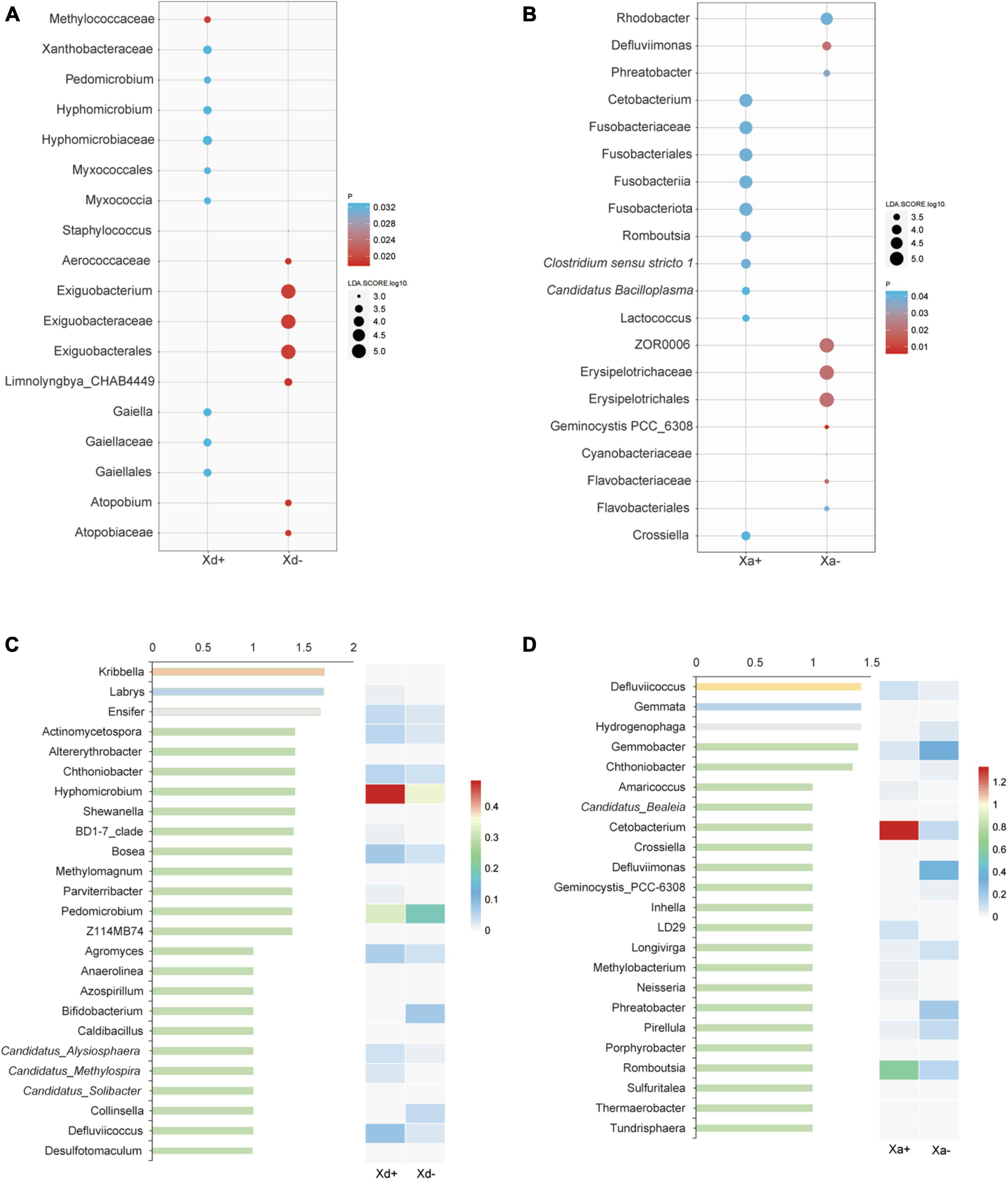
Figure 3. The most discriminant genera of uninfected and infected groups selected by LEfSe (A,B) and the random forest model (C,D). (A,C) panels show groups Xd+ and Xd-, whereas (B,D) show groups Xa+ and Xa-. The x-axis of the bar plot represents IncMSE (increase in mean squared error) and heat map based on the relative abundance of genera in (C,D). The data for drawing can be found in Supplementary Tables 8, 9.
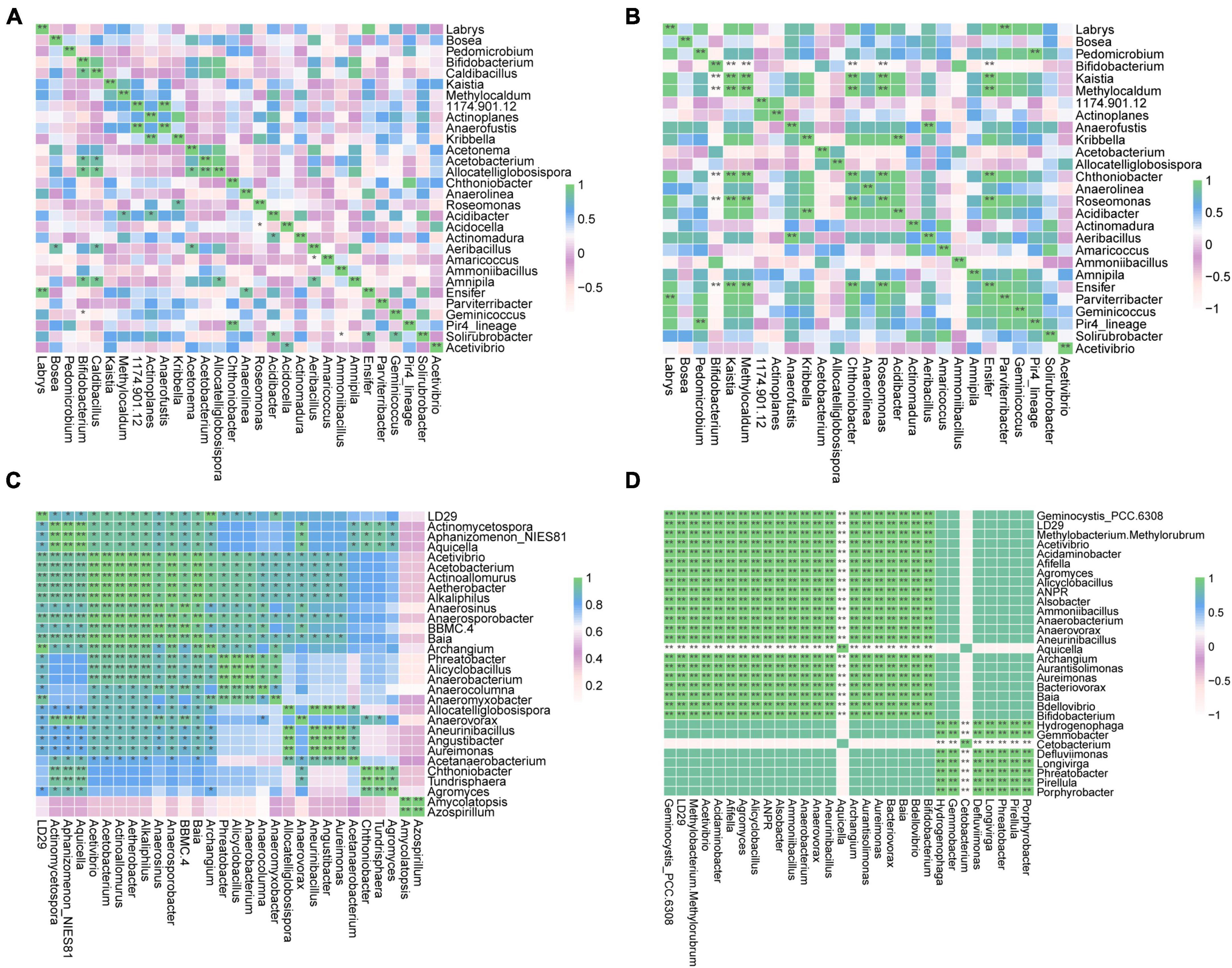
Figure 4. Heatmap of the Spearman’s correlation between the discriminant genera of uninfected and infected groups selected by a random forest model (*FDR < 0.05, **FDR < 0.01). (A) Group Xd+. (B) Group Xd-. (C) Group Xa+. (D) Group Xa-. The data for drawing can be found in Supplementary Table 10.
3.2 The co-abundance network of the gut microbiota
We sought to determine the co-occurrence patterns of the microbiota in the four groups. Results showed significant differences in network properties among groups (Table 1 and Figure 5). Xd+ has higher modularity and fewer network edges, compared with Xd-. Its network nodes mainly consisted of Firmicutes, Actinobacteriota, and Proteobacteria (Figure 5A), and the largest node belonged to Actinobacteriota in Xd+ , and to Firmicutes in Xd- (Figures 5A, B). As for Xa+ , it had more network nodes and higher modularity than Xa- (Figures 5C, D), and the main network nodes of it were Firmicutes, Actinobacteriota, and Proteobacteria (Figure 5C). Only one module hub was detected in Xa+ and no connectors were detected in the four groups (Figures 5E, F).
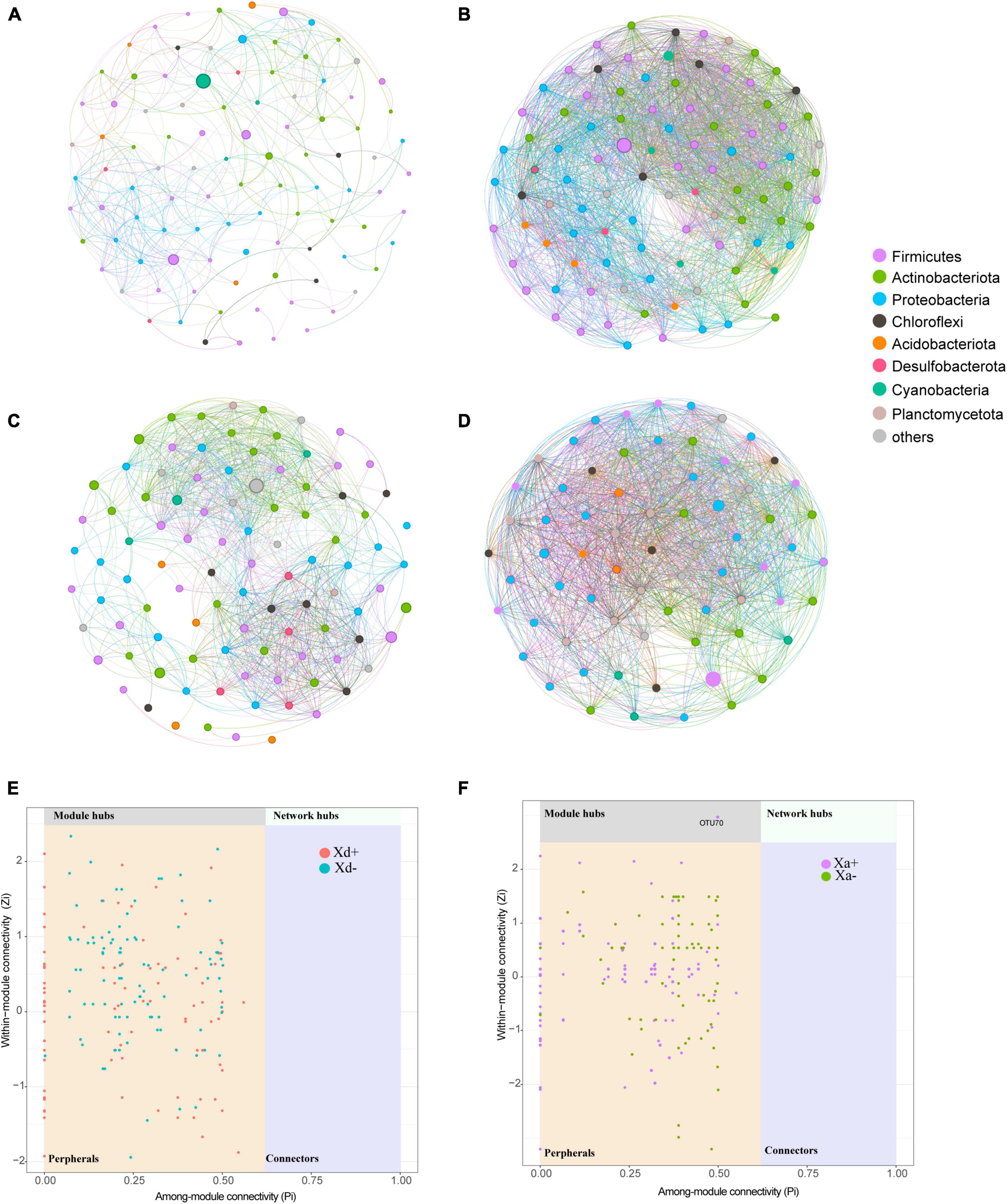
Figure 5. Co-occurrence networks based on the top 100 dominant genera. Node size represents the node degree and color represents the phylum. (A) Network of group Xd+. (B) Network of group Xd-. (C) Network of group Xa+. (D) Network of group Xa-. (E) Classification of nodes in Xd+ and Xd- networks. (F) Classification of nodes in Xa+ and Xa- networks. The data for drawing can be found in Supplementary Table 11.
3.3 Distribution of SCFAs in the infected and uninfected groups
The content of acetic acid was the highest among the eight SFCAs, followed by propanoic acid and butanoic acid (Figure 6). The total SCFAs, acetic acid and isovaleric acid significantly differed between Xd+ and Xd- (Figures 6A, B, F), while the total SCFAs and acetic acid also showed significant differences between Xa+ and Xa- (Figures 6A, B).
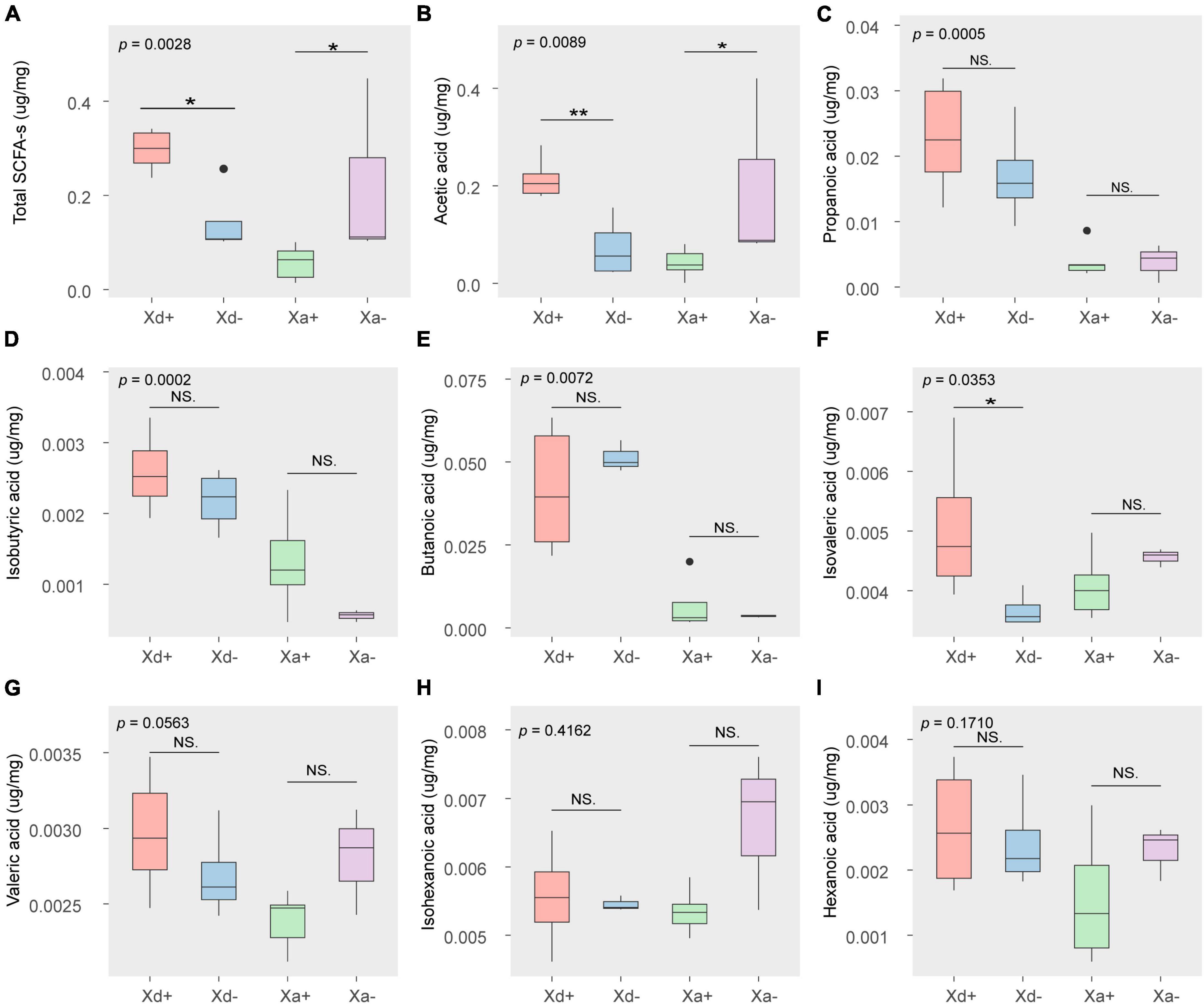
Figure 6. The concentration of SCFAs in different groups (μg/mg wet weight). (A) the total amount of SCFAs. The amount of acetic acid (B), propanoic acid (C), isobutyric acid (D), butanoic acid (E), isovaleric acid (F), valeric acid (G), isohexanoic acid (H), and hexanoic acid (I). The data for drawing can be found in Supplementary Table 12
3.4 The correlation between the SCFAs and most discriminant genera in uninfected and infected groups
In order to explore the relationship between the intestinal microbiota and SCFAs, we conducted a network analysis. It indicated a significant correlation between gut microbiota and SCFAs (Figure 7). Proteobacteria and Actinobacteria dominated the large modules in the networks of Xd+ and Xd- (Figures 7A, B). The total SCFAs and acetic acid in Xd+ had a significantly positive correlation with Collinsella (Figure 7A). Besides, more SCFAs in Xd+ significantly correlated with gut microbiota than that in Xd-. The Xd+ network had more connectors than the Xd- network (Figures 7A, B). Proteobacteria was the only dominant module in both Xa+ and Xa- (Figures 7C, D). Network nodes in Xa+ was fewer than that in Xa-, and most correlations in Xa+ were positive (Figure 7C).
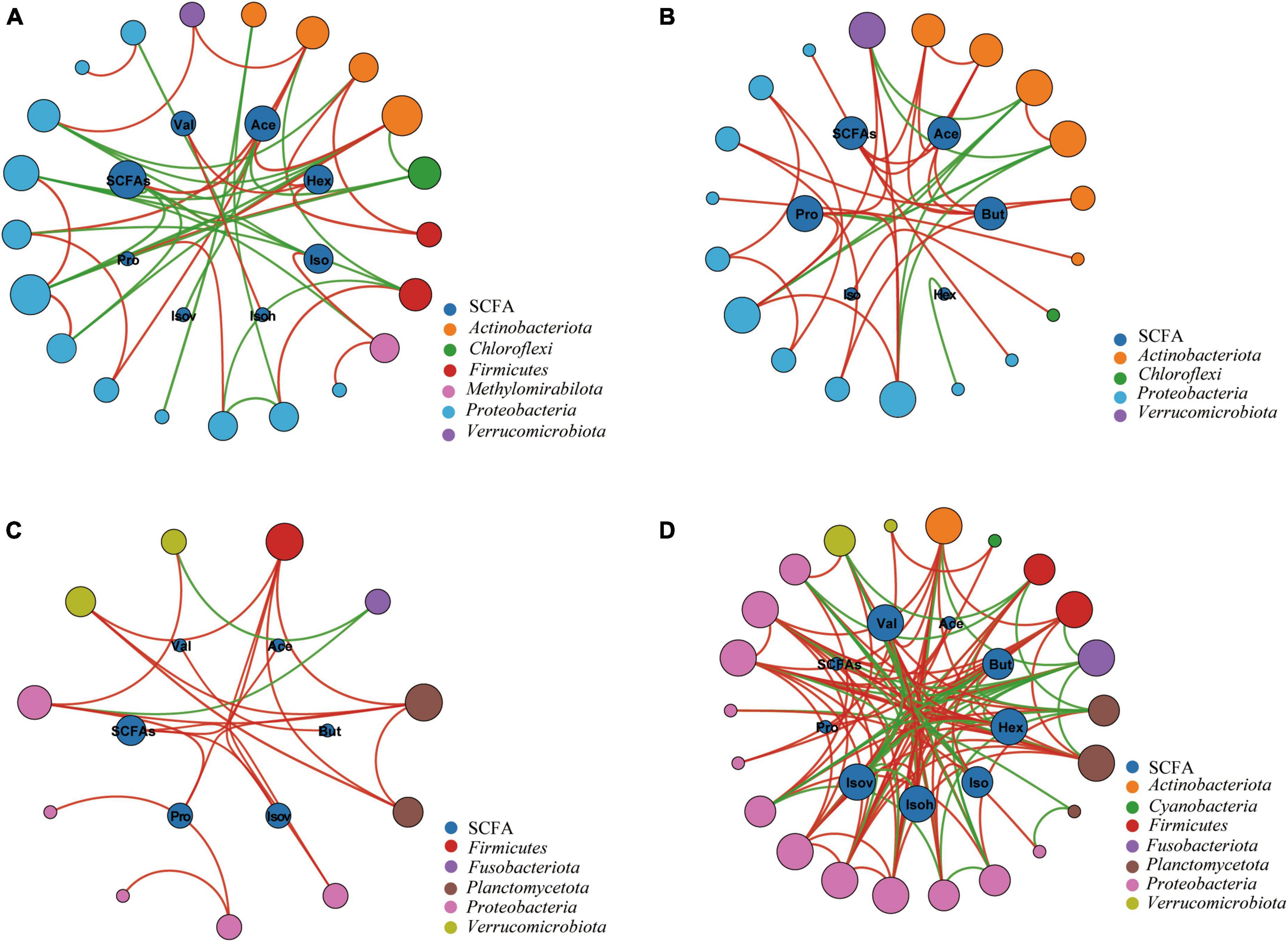
Figure 7. Networks between the SCFAs and the most highly discriminant genera selected by the RFA analysis. Node size is proportional to node connectivity. Outer nodes in different colors represent different phyla and inner nodes represent different SCFAs. Red lines indicate positive interactions and green lines indicate negative interactions. (A) Group Xd+. (B) Group Xd-. (C) Group Xa+. (D) Group Xa-. The data for drawing can be found in Supplementary Table 13
4 Discussion
4.1 B. polyvacuolum affects the composition of gut microbiota in X. davidi and X. argentea
In this study, we used X. davidi and X. argentea to analyze in detail the gut microbial composition and structure in response to infection with B. polyvacuolum. Results showed that Firmicutes, Proteobacteria, and Actinobacteriota were the predominant microbial phyla in X. davidi and X. argentea’s hindgut, which corresponds to previous reports in other fish species (Wu et al., 2012; Baldo et al., 2015; Belkova et al., 2017). Infection with B. polyvacuolum can cause an increase in the overall diversity of gut microbiota, as well as an increase in the relative abundance of Fusobacteria and Chloroflexi, in both X. davidi and X. argentea. Previous studies showed that Fusobacteria and Cetobacterium were the dominant phylum and genus, respectively, in the fish gut (Roeselers et al., 2011; Liu et al., 2016). Chloroflexi was also found in domesticated zebrafish and in the Nile tilapia (Oreochromis niloticus) (Pham et al., 2008; Bereded et al., 2020). The diversity and relative abundance of Fusobacteria was positively correlated with antibody production and resistance to infections (Knutie et al., 2017), it can be inferred that X. davidi and X. argentea with B. polyvacuolum may be healthier than the uninfected ones.
We also observed a significant increase of genera Romboutsia and Clostridium in B. polyvacuolum-infected X. argentea. Romboutsia plays an important role in carbohydrate utilization, single amino acids fermentation, and anaerobic respiration (Gerritsen et al., 2019). This genera was proved to be closely related to numerous species of Clostridium (Wang et al., 2015). Clostridium is commonly abundant in the gut of herbivorous and omnivorous fish, which has the ability to degrade cellulose (Liu et al., 2016; Yukgehnaish et al., 2020). Considering that X. davidi and X. argentea mainly feed on plant debris and algae (Sifa, 2000), it can be inferred that Clostridium in the hindgut of X. davidi and X. argentea also plays an important role in degrading cellulose.
In addition, we also found that among groups without B. polyvacuolum infection, ZOR006 and Exiguobacterium were dominant genera in X. davidi and X. argentea, respectively. Meanwhile, the alpha diversity showed that the gut microbiota of B. polyvacuolum-infected X. davidi and X. argentea had higher diversity, richness, and evenness than the uninfected ones. It may indicate that B. polyvacuolum had a beneficial effect on the balance of gut microbiota. Higher microbial diversity levels and more even communities could be signs of good gut health, which could be beneficial for the productivity of aquaculture (Infante-Villamil et al., 2021). It can be inferred that B. polyvacuolum contributed to the richness and evenness of the gut microbial community and made the gut microenvironment more diverse and stable. We also observed higher modularity in the B. polyvacuolum-infected X. davidi. Modularity is important for the stability of ecological networks (Olesen et al., 2007). Higher modularity indicated that the microbial ecosystem was more stable in B. polyvacuolum-infected X. davidi.
4.2 B. polyvacuolum may affect SCFAs synthesis in X. davidi and X. argentea through gut microbiota
Sequencing and short-chain fatty acids are the end products of gut microbial fermentation, mainly concentrated in the hindgut of aquatic animals (Clements et al., 1994). Gut microbial-derived SCFAs mainly include acetic, propionic, butyric, valeric, and caproic acids, and the first three are commonly the most abundant SCFAs (Trachsel et al., 2016). On one hand, the synthesis of SCFAs is correlated with microbial composition and environmental conditions (Louis et al., 2014), though it can also be affected by the species of fish. Normally, carnivorous fish have relatively higher SCFA concentrations than herbivorous and omnivorous species (Clements et al., 2014).
With B. polyvacuolum infection, the abundance of total SCFAs, acetic acid, and isovaleric acid in X. davidi and propionic, isobutyric and butanoic acids in X. argentea were significantly increased. In other words, the presence of B. polyvacuolum could lead to an increase in SFCAs, as the increased SCFAs may be a direct product of the increased diversity of gut microbiota. Research has shown that propionic acid could decrease the levels of fatty acids in liver and plasma, suppress appetite, and exhibit immunosuppressive effects (Sa’ad et al., 2010). Considering the crucial role of SCFAs in maintaining gut homoeostasis and monitoring the relationship with the host, its immune system and intestinal commensal microbes (Trachsel et al., 2016; Tran et al., 2020), the higher total SCFAs may indicate improved gut health in the B. polyvacuolum-infected X. davidi and X. argentea.
The high concentration of SCFAs in the B. polyvacuolum-infected X. davidi and X. argentea may be related to the high abundance of gut microbiota. In other words, there was a positive relationship between the diversity and composition of gut microbiota and the production of SCFAs. For instance, the abundance of Clostridium was significantly higher in the B. polyvacuolum-infected X. argentea. Clostridium is considered to be a probiotic and the main acetic acid-producing bacterium (Guo et al., 2019; Xu et al., 2020). It can be used by colonocytes to generate energy, maintain gut anaerobic conditions, preserve gut barrier integrity, and limit pro-inflammatory cytokines (Singh et al., 2023). It was reported that Clostridium butyricum can modulate intestinal metabolic capacities and improve the intestinal SCFA content in Marsupenaeus japonicus (Duan et al., 2018). It can also enhance the production of butyric acid and propanoic acid, as well as the activity of catalase and lysozyme in the intestine of common carp (Meng et al., 2021).
We also found an increased abundance of Cetobacterium in the B. polyvacuolum-infected X. davidi and X. argentea. Cetobacterium is also a good SCFAs producer and its metabolites, such as acetic, propionic, and butanoic acid, can improve fish health (Bhute et al., 2020; Wang et al., 2021; Xie et al., 2021), so the increased Cetobacterium may be the sign that B. polyvacuolum-infected X. davidi and X. argentea are in good health. Bacteria from the genus Collinsella were also correlated with the total SCFAs and acetic acid in X. davidi. This genus is also proved to be an effective SCFA producer (Yin et al., 2013). All these findings showed that B. polyvacuolum can regulate the levels of SCFAs by altering the gut microbiota composition.
5 Conclusion
This study revealed the interactions among parasitic protozoa, gut microbiota, and host metabolism. The results showed that B. polyvacuolum can affect the abundance and structure of gut microbiota in X. davidi and X. argentea. It increased the diversity, richness, and evenness of gut microbiota and enhanced its stability, which could be the sign that host with B. polyvacuolum are in good health. Meanwhile, SCFAs producers like Cetobacterium and Clostridium were also increased in B. polyvacuolum-infected X. davidi and X. argentea. Accordingly, the abundance of total SCFAs, acetic acid, and isovaleric acid significantly increased in B. polyvacuolum-infected X. davidi, while the concentrations of propionic, isobutyric and butanoic acids also increased in the B. polyvacuolum-infected X. argentea. These findings may indicate that B. polyvacuolum were a commensal member of the gut. Our findings provided valuable insights into the relationships between parasitic ciliates, gut microbiota, and host metabolism. This study may deepen the understanding of the impact of parasitic protozoa on host and gut microbiota.
Data availability statement
The datasets presented in this study can be found in online repositories. The names of the repository/repositories and accession number(s) can be found below: https://www.ncbi.nlm.nih.gov/bioproject/PRJNA981639/.
Ethics statement
The animal studies were approved by the Animal Ethics Committee of Institute of Hydrobiology, Chinese Academy of Sciences. The studies were conducted in accordance with the local legislation and institutional requirements. Written informed consent was obtained from the owners for the participation of their animals in this study.
Author contributions
XB: Formal analysis, Writing – original draft. ZL: Software, Visualization, Writing – review and editing. WZ: Funding acquisition, Software, Writing – review and editing. QZ: Software, Writing – original draft. YC: Writing – review and editing. WL: Writing – review and editing. HZ: Writing – review and editing. ML: Funding acquisition, Supervision, Writing – review and editing. GW: Funding acquisition, Supervision, Writing – review and editing.
Funding
The author(s) declare financial support was received for the research, authorship, and/or publication of this article. This work was supported by the National Natural Science Foundation of China (Nos. 32170437, 32230109, and 32303066), the Second Tibetan Plateau Scientific Expedition and Research Program (STEP) (No. 2019QZKK0304), the earmarked fund for CARS (No. CARS-45), the Protist 10,000 Genomics Project (P10K) Consortium, and the National Aquatic Biological Resource Center (NABRC).
Acknowledgments
We would like to thank Ivan Jakovlić for the help of language and the research was supported by the Wuhan Branch, Supercomputing Center, Chinese Academy of Sciences, China.
Conflict of interest
The authors declare that the research was conducted in the absence of any commercial or financial relationships that could be construed as a potential conflict of interest.
Publisher’s note
All claims expressed in this article are solely those of the authors and do not necessarily represent those of their affiliated organizations, or those of the publisher, the editors and the reviewers. Any product that may be evaluated in this article, or claim that may be made by its manufacturer, is not guaranteed or endorsed by the publisher.
Supplementary material
The Supplementary Material for this article can be found online at: https://www.frontiersin.org/articles/10.3389/fmicb.2023.1295456/full#supplementary-material
Footnotes
References
Baldo, L., Riera, J. L., Tooming-Klunderud, A., Albà, M. M., and Salzburger, W. (2015). Gut microbiota dynamics during dietary shift in eastern African cichlid fishes. PLoS One 10:e0127462. doi: 10.1371/journal.pone.0127462
Bastian, M., Heymann, S., and Jacomy, M. (2009). “Gephi: An open source software for exploring and manipulating networks,” in Proceedings of the international AAAI conference on web and social media (San José, CA), 361–362.
Belkova, N. L., Sidorova, T. V., Glyzina, O. Y., Yakchnenko, V. M., Sapozhnikova, Y. P., Bukin, Y. S., et al. (2017). Gut microbiome of juvenile coregonid fishes: Comparison of sympatric species and their F1 hybrids. Fundamental Appl. Limnol. 189, 279–290.
Bereded, N. K., Curto, M., Domig, K. J., Abebe, G. B., Fanta, S. W., Waidbacher, H., et al. (2020). Metabarcoding analyses of gut microbiota of Nile Tilapia (Oreochromis niloticus) from Lake Awassa and Lake Chamo, Ethiopia. Microorganisms 8:1040. doi: 10.3390/microorganisms8071040
Bhute, S. S., Escobedo, B., Haider, M., Mekonen, Y., Ferrer, D., Hillyard, S. D., et al. (2020). The gut microbiome and its potential role in paradoxical anaerobism in pupfishes of the Mojave Desert. Anim. Microb. 2, 1–9. doi: 10.1186/s42523-020-00037-5
Brealey, J. C., Lecaudey, L. A., Kodama, M., Rasmussen, J. A., Sveier, H., Dheilly, N. M., et al. (2022). Microbiome “inception”: An intestinal cestode shapes a hierarchy of microbial communities nested within the host. mBio 13, e679–e622. doi: 10.1128/mbio.00679-22
Burgess, S. L., Gilchrist, C. A., Lynn, T. C., and Petri, W. A. (2017). Parasitic protozoa and interactions with the host intestinal microbiota. Infect. Immun. 85, e00101-17.
Chen, S., Zhou, Y., Chen, Y., and Gu, J. (2018). fastp: An ultra-fast all-in-one FASTQ preprocessor. Bioinformatics 34, i884–i890. doi: 10.1093/bioinformatics/bty560
Chudnovskiy, A., Mortha, A., Kana, V., Kennard, A., Ramirez, J. D., Rahman, A., et al. (2016). Host-protozoan interactions protect from mucosal infections through activation of the inflammasome. Cell 167, 444.–456. doi: 10.1016/j.cell.2016.08.076
Clements, K. D., Angert, E. R., Montgomery, W. L., and Choat, J. H. (2014). Intestinal microbiota in fishes: What’s known and what’s not. Mol. Ecol. 23, 1891–1898.
Clements, K., Gleeson, V., and Slaytor, M. (1994). Short-chain fatty acid metabolism in temperate marine herbivorous fish. J. Comp. Physiol. B 164, 372–377.
Consortium, T. H. M. P. (2012). Structure, function and diversity of the healthy human microbiome. Nature 486, 207–214.
Csardi, G., and Nepusz, T. (2006). The igraph software package for complex network research. Interjournal Complex Syst. 1695, 1–9.
de Graaf, R. M., Ricard, G., van Alen, T. A., Duarte, I., Dutilh, B. E., Burgtorf, C., et al. (2011). The organellar genome and metabolic potential of the hydrogen-producing mitochondrion of Nyctotherus ovalis. Mol. Biol. Evol. 28, 2379–2391. doi: 10.1093/molbev/msr059
Deng, Y., Jiang, Y.-H., Yang, Y., He, Z., Luo, F., and Zhou, J. (2012). Molecular ecological network analyses. BMC Bioinform. 13:113. doi: 10.1186/1471-2105-13-113
Duan, Y., Dong, H., Wang, Y., Zhang, Y., and Zhang, J. (2018). Effects of the dietary probiotic Clostridium butyricum on intestine digestive and metabolic capacities, SCFA content and body composition in Marsupenaeus japonicus. J. Ocean Univ. China 17, 690–696.
Edgar, R. C. (2013). UPARSE: Highly accurate OTU sequences from microbial amplicon reads. Nat. Methods 10, 996–998. doi: 10.1038/nmeth.2604
Gerritsen, J., Hornung, B., Ritari, J., Paulin, L., Rijkers, G. T., Schaap, P. J., et al. (2019). A comparative and functional genomics analysis of the genus Romboutsia provides insight into adaptation to an intestinal lifestyle. BioRxiv [Preprint]. doi: 10.1101/845511
Guo, C.-J., Allen, B. M., Hiam, K. J., Dodd, D., Van Treuren, W., Higginbottom, S., et al. (2019). Depletion of microbiome-derived molecules in the host using Clostridium genetics. Science 366:eaav1282. doi: 10.1126/science.aav1282
Horiuchi, H., Kamikado, K., Aoki, R., Suganuma, N., Nishijima, T., Nakatani, A., et al. (2020). Bifidobacterium animalis subsp. lactis GCL2505 modulates host energy metabolism via the short-chain fatty acid receptor GPR43. Sci. Rep. 10:4158. doi: 10.1038/s41598-020-60984-6
Infante-Villamil, S., Huerlimann, R., and Jerry, D. R. (2021). Microbiome diversity and dysbiosis in aquaculture. Rev. Aquac. 13, 1077–1096.
Kaoutari, A. E., Armougom, F., Gordon, J. I., Raoult, D., and Henrissat, B. (2013). The abundance and variety of carbohydrate-active enzymes in the human gut microbiota. Nat. Rev. Microbiol. 11, 497–504.
Knutie, S. A., Wilkinson, C. L., Kohl, K. D., and Rohr, J. R. (2017). Early-life disruption of amphibian microbiota decreases later-life resistance to parasites. Nat. Commun. 8:86. doi: 10.1038/s41467-017-00119-0
Koèíková, B., Majláth, I., and Majláthová, V. (2018). The occurrence of protozoan parasites (Schellackia sp. Reichenow, 1919, Tritrichomonas sp. Kofoid, 1920, and Proteromonas sp. Kunstler, 1883) in Lacertid Lizards from selected localities in Europe. Comp. Parasitol. 85, 48–57.
Krautkramer, K. A., Fan, J., and Bäckhed, F. (2021). Gut microbial metabolites as multi-kingdom intermediates. Nat. Rev. Microbiol. 19, 77–94. doi: 10.1038/s41579-020-0438-4
Kreisinger, J., Bastien, G., Hauffe, H. C., Marchesi, J., and Perkins, S. E. (2015). Interactions between multiple helminths and the gut microbiota in wild rodents. Philos. Trans. R. Soc. B Biol. Sci. 370:20140295. doi: 10.1098/rstb.2014.0295
Leung, J. M., Graham, A. L., and Knowles, S. C. L. (2018). Parasite-microbiota interactions with the vertebrate gut: Synthesis through an ecological lens. Front. Microbiol. 9:843. doi: 10.3389/fmicb.2018.00843
Li, M., Wang, C., Wang, J. G., Li, A. H., Gong, X. N., and Ma, H. W. (2009). Redescription of Balantidium polyvacuolum Li 1963 (Class: Litostomatea) inhabiting the intestines of Xenocyprinae fishes in Hubei, China. Parasitol. Res. 106, 177–182. doi: 10.1007/s00436-009-1645-0
Li, M., Wang, R. Q., Gomes, G. B., Zou, H., Li, W. X., Wu, S. G., et al. (2018). Epidemiology and identification of two species of Chilodonella affecting farmed fishes in China. Vet. Parasitol. 264, 8–17. doi: 10.1016/j.vetpar.2018.10.009
Liu, H., Guo, X., Gooneratne, R., Lai, R., Zeng, C., Zhan, F., et al. (2016). The gut microbiome and degradation enzyme activity of wild freshwater fishes influenced by their trophic levels. Sci. Rep. 6:24340. doi: 10.1038/srep24340
Louis, P., Hold, G. L., and Flint, H. J. (2014). The gut microbiota, bacterial metabolites and colorectal cancer. Nat. Rev. Microbiol. 12, 661–672.
Magoè, T., and Salzberg, S. L. (2011). FLASH: Fast length adjustment of short reads to improve genome assemblies. Bioinformatics 27, 2957–2963. doi: 10.1093/bioinformatics/btr507
Meng, X., Wu, S., Hu, W., Zhu, Z., Yang, G., Zhang, Y., et al. (2021). Clostridium butyricum improves immune responses and remodels the intestinal microbiota of common carp (Cyprinus carpio L.). Aquaculture 530:735753.
Nicholson, J. K., Holmes, E., Kinross, J., Burcelin, R., Gibson, G., Jia, W., et al. (2012). Host-gut microbiota metabolic interactions. Science 336, 1262–1267.
Nieves-Ramírez, M., Partida-Rodríguez, O., Laforest-Lapointe, I., Reynolds, L., Brown, E., Valdez-Salazar, A., et al. (2018). Asymptomatic intestinal colonization with protist Blastocystis is strongly associated with distinct microbiome ecological patterns. Msystems 3, e00007–18. doi: 10.1128/mSystems.00007-18
Olesen, J. M., Bascompte, J., Dupont, Y. L., and Jordano, P. (2007). The modularity of pollination networks. Proc. Natl Acad. Sci. U.S.A. 104, 19891–19896.
Pham, L. N., Kanther, M., Semova, I., and Rawls, J. F. (2008). Methods for generating and colonizing gnotobiotic zebrafish. Nat. protoc. 3, 1862–1875.
R Core Team (2019). R: A language and environment for statistical computing. Vienna: R Foundation for Statistical Computing.
Roeselers, G., Mittge, E. K., Stephens, W. Z., Parichy, D. M., Cavanaugh, C. M., Guillemin, K., et al. (2011). Evidence for a core gut microbiota in the zebrafish. ISME J. 5, 1595–1608.
Sa’ad, H., Peppelenbosch, M. P., Roelofsen, H., Vonk, R. J., and Venema, K. (2010). Biological effects of propionic acid in humans; metabolism, potential applications and underlying mechanisms. Biochim. Biophys. Acta Mol. Cell Biol. Lipids 1801, 1175–1183.
Sifa, L. (2000). “The impact of large reservoirs on fish biodiversity and fisheries in China,” in Aciar proceedings, ACIAR, 1998, Shanghai.
Singh, V., Lee, G., Son, H., Koh, H., Kim, E. S., Unno, T., et al. (2023). Butyrate producers,“The Sentinel of Gut”: Their intestinal significance with and beyond butyrate, and prospective use as microbial therapeutics. Front. Microbiol. 13:1103836. doi: 10.3389/fmicb.2022.1103836
Trachsel, J., Bayles, D. O., Looft, T., Levine, U. Y., and Allen, H. K. (2016). Function and phylogeny of bacterial butyryl coenzyme a: Acetate transferases and their diversity in the proximal colon of swine. Appl. Environ. Microbiol. 82, 6788–6798. doi: 10.1128/AEM.02307-16
Tran, N. T., Li, Z., Wang, S., Zheng, H., Aweya, J. J., Wen, X., et al. (2020). Progress and perspectives of short-chain fatty acids in aquaculture. Rev. Aquac. 12, 283–298.
Ulusan Bagci, O., and Caner, A. (2022). The interaction of gut microbiota with parasitic protozoa. J. Parasitic Dis. 46, 8–11.
Villanueva, R. A. M., and Chen, Z. J. (2019). ggplot2: Elegant graphics for data analysis. Milton Park: Taylor & Francis.
Wang, A., Zhang, Z., Ding, Q., Yang, Y., Bindelle, J., Ran, C., et al. (2021). Intestinal Cetobacterium and acetate modify glucose homeostasis via parasympathetic activation in zebrafish. Gut Microbes 13, 1–15. doi: 10.1080/19490976.2021.1900996
Wang, Q., Garrity, G. M., Tiedje, J. M., and Cole, J. R. (2007). Naive Bayesian classifier for rapid assignment of rRNA sequences into the new bacterial taxonomy. Appl. Environ. Microbiol. 73, 5261–5267. doi: 10.1128/AEM.00062-07
Wang, Y., Dong, Q., Hu, S., Zou, H., Wu, T., Shi, J., et al. (2022). Decoding microbial genomes to understand their functional roles in human complex diseases. iMeta 1:e14.
Wang, Y., Song, J., Zhai, Y., Zhang, C., Gerritsen, J., Wang, H., et al. (2015). Romboutsia sedimentorum sp. nov., isolated from an alkaline-saline lake sediment and emended description of the genus Romboutsia. Int. J. Syst. Evol. Microbiol. 65, 1193–1198.
Wu, S., Wang, G., Angert, E. R., Wang, W., Li, W., and Zou, H. (2012). Composition, diversity, and origin of the bacterial community in grass carp intestine. PLoS One 7:e30440. doi: 10.1371/journal.pone.0030440
Xie, M., Zhou, W., Xie, Y., Li, Y., Zhang, Z., Yang, Y., et al. (2021). Effects of Cetobacterium somerae fermentation product on gut and liver health of common carp (Cyprinus carpio) fed diet supplemented with ultra-micro ground mixed plant proteins. Aquaculture 543:736943.
Xu, Y., Zhu, Y., Li, X., and Sun, B. (2020). Dynamic balancing of intestinal short-chain fatty acids: The crucial role of bacterial metabolism. Trends Food Sci. Technol. 100, 118–130.
Yang, H., Wu, J., Du, H., Zhang, H., Li, J., and Wei, Q. (2022). Quantifying the colonization of environmental microbes in the fish gut: A casestudy of wildfish populations in the Yangtze River. Front. Microbiol. 12:4367. doi: 10.3389/fmicb.2021.828409
Yin, X., Peng, J., Zhao, L., Yu, Y., Zhang, X., Liu, P., et al. (2013). Structural changes of gut microbiota in a rat non-alcoholic fatty liver disease model treated with a Chinese herbal formula. Syst. Appl. Microbiol. 36, 188–196. doi: 10.1016/j.syapm.2012.12.009
Yukgehnaish, K., Kumar, P., Sivachandran, P., Marimuthu, K., Arshad, A., Paray, B. A., et al. (2020). Gut microbiota metagenomics in aquaculture: Factors influencing gut microbiome and its physiological role in fish. Rev. Aquac. 12, 1903–1927.
Zhao, W. S., Bu, X. L., Zou, H., Li, W. X., Wu, S. G., Li, M., et al. (2022a). The genome of the mitochondrion-related organelle in Cepedea longa, a large endosymbiotic Opalinid inhabiting the recta of frogs. Int. J. Mol. Sci. 23:13472.
Keywords: Balantidium polyvacuolum, gut microbiota, short-chain fatty acids (SCFAs), protozoa, co-occurrence network
Citation: Bu X, Li Z, Zhao W, Zeng Q, Chen Y, Li W, Zou H, Li M and Wang G (2023) Alterations of gut microbiota and short-chain fatty acids induced by Balantidium polyvacuolum in the hindgut of Xenocyprinae fishes providing new insights into the relationship among protozoa, gut microbiota and host. Front. Microbiol. 14:1295456. doi: 10.3389/fmicb.2023.1295456
Received: 16 September 2023; Accepted: 30 October 2023;
Published: 21 November 2023.
Edited by:
Jun-Seob Kim, Incheon National University, Republic of KoreaReviewed by:
Jonghyeok Shin, Korea Research Institute of Bioscience and Biotechnology, Republic of KoreaVineet Singh, Kyungpook National University, Republic of Korea
Copyright © 2023 Bu, Li, Zhao, Zeng, Chen, Li, Zou, Li and Wang. This is an open-access article distributed under the terms of the Creative Commons Attribution License (CC BY). The use, distribution or reproduction in other forums is permitted, provided the original author(s) and the copyright owner(s) are credited and that the original publication in this journal is cited, in accordance with accepted academic practice. No use, distribution or reproduction is permitted which does not comply with these terms.
*Correspondence: Ming Li, bGltaW5nQGloYi5hYy5jbg==