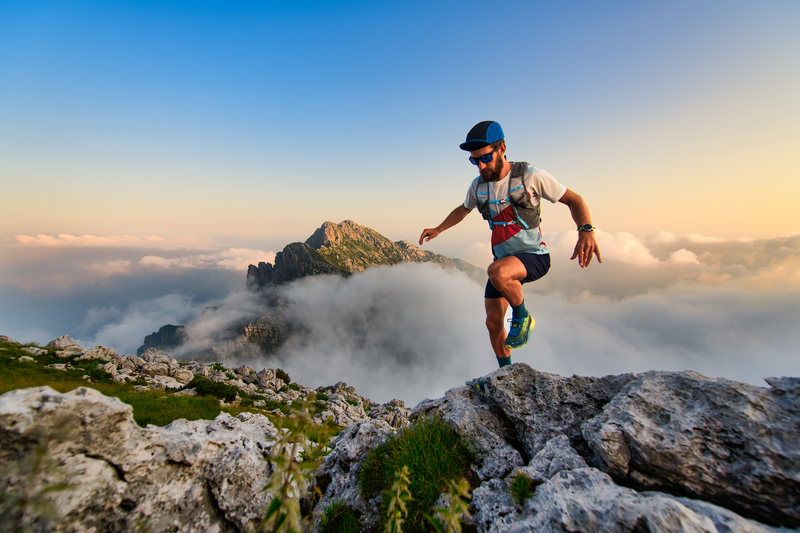
95% of researchers rate our articles as excellent or good
Learn more about the work of our research integrity team to safeguard the quality of each article we publish.
Find out more
ORIGINAL RESEARCH article
Front. Microbiol. , 18 December 2023
Sec. Terrestrial Microbiology
Volume 14 - 2023 | https://doi.org/10.3389/fmicb.2023.1293720
Grazing exclusion (GE) is an effective measure for restoring degraded grassland ecosystems. However, the effect of GE on methane (CH4) uptake and production remains unclear in dominant bacterial taxa, main metabolic pathways, and drivers of these pathways. This study aimed to determine CH4 flux in alpine meadow soil using the chamber method. The in situ composition of soil aerobic CH4-oxidizing bacteria (MOB) and CH4-producing archaea (MPA) as well as the relative abundance of their functional genes were analyzed in grazed and nongrazed (6 years) alpine meadows using metagenomic methods. The results revealed that CH4 fluxes in grazed and nongrazed plots were −34.10 and −22.82 μg‧m−2‧h−1, respectively. Overall, 23 and 10 species of Types I and II MOB were identified, respectively. Type II MOB comprised the dominant bacteria involved in CH4 uptake, with Methylocystis constituting the dominant taxa. With regard to MPA, 12 species were identified in grazed meadows and 3 in nongrazed meadows, with Methanobrevibacter constituting the dominant taxa. GE decreased the diversity of MPA but increased the relative abundance of dominated species Methanobrevibacter millerae from 1.47 to 4.69%. The proportions of type I MOB, type II MOB, and MPA that were considerably affected by vegetation and soil factors were 68.42, 21.05, and 10.53%, respectively. Furthermore, the structural equation models revealed that soil factors (available phosphorus, bulk density, and moisture) significantly affected CH4 flux more than vegetation factors (grass species number, grass aboveground biomass, grass root biomass, and litter biomass). CH4 flux was mainly regulated by serine and acetate pathways. The serine pathway was driven by soil factors (0.84, p < 0.001), whereas the acetate pathway was mainly driven by vegetation (−0.39, p < 0.05) and soil factors (0.25, p < 0.05). In conclusion, our findings revealed that alpine meadow soil is a CH4 sink. However, GE reduces the CH4 sink potential by altering vegetation structure and soil properties, especially soil physical properties.
Methane (CH4) is considered the second most important greenhouse gas following carbon dioxide (CO2). However, the potential of CH4 to affect global warming is ~28 times that of CO2 (Stocker et al., 2013). Furthermore, CH4 is responsible for ~20% of global warming effects, thus profoundly affecting the rate of global warming. In the global CH4 budget, terrestrial soil constitutes the most substantial biological sink of atmospheric CH4 (Dutaur and Verchot, 2007; De Bernardi et al., 2022). Grassland soil absorbs 3.73 Tg of CH4 annually (Yu et al., 2017), and alpine meadows on the Qinghai–Tibet Plateau exhibit the highest CH4 uptake (0.284 Tg of CH4 annually), accounting for ~44% of the total CH4 absorption by grassland soil in China (Wang et al., 2014). Therefore, CH4 metabolism in alpine meadows is critical for its effects on global climate change.
Methanotrophs catalyze CH4 oxidation, they are classified as aerobic CH4-oxidizing bacteria (MOB) and anaerobic methanotrophic archaea (ANME), which can offset the atmospheric effects of CH4 emissions (Knief, 2015; Schmitz et al., 2021; Wallenius et al., 2021). MOB can be categorized into the following four groups according to their physiological, phylogenetic, biochemical, and phenotypical characteristics: type I MOB (Gammaproteobacteria), type II MOB (Alphaproteobacteria), Verrucomicrobia, and NC10 (Jeremy et al., 2010; Knief, 2015; Padilla et al., 2016). Alphaproteobacteria and Gammaproteobacteria are abundant in CH4-metabolizing flora and have been studied for more than a century (Schmieder and Edwards, 2011). Types I and II MOB assimilate CH4 via the ribulose monophosphate pathway (RuMP) and serine pathway, respectively (Hanson and Hanson, 1996; Jeremy et al., 2010). ANME belong to the Euryarchaeota phylum and include ANME-1, ANME-2, and ANME-3. They are mainly found in anoxic environments such as coastal sediments, wetland soils, and water bodies (Wallenius et al., 2021). Methanogens, belonging to archaea, are CH4-producing microorganisms; therefore, they are also known as CH4-producing archaea (MPA). In terms of taxonomy, MPA was found within 8 orders, including Methanococcales, Methanopyrales, Methanobacteriales, Methanomicrobiales, Methanocellales, Methanonatronarchaeales, Methanosarcinales, and Methanomassiliicoccales (Lyu et al., 2018). Further, the following three methanogenic pathways have been reported (KEGG, 2022): CO2 to CH4 (CO2 pathway), methanol to CH4 (methanol pathway), and acetate to CH4 (acetate pathway). Analysis of functional genes is an effective means of explaining the taxonomic and functional changes in microbial communities. Recent studies have evaluated the CH4 uptake and production potential of microbial communities by detecting monooxygenase subunit A (pmoA) and methyl coenzyme M reductase A (mcrA) genes, respectively (Bhardwaj and Dubey, 2020; Zhang et al., 2022). Although analysis of pmoA and mcrA effectively indicates alterations in the abundance of MOB and MAP, such analysis cannot determine the differences between methanotrophic and methanogenic pathways. Recent evidence suggests that gene abundance determined using metagenomics can predict CH4 metabolism more effectively than community profiling (Zhang et al., 2022).
Overgrazing can convert an atmospheric CH4 sink to a CH4 source in the rangeland ecosystem, and CH4 emission is known to increase with increasing grazing intensity (Tang et al., 2019). This process can be reversed via grazing exclusion (GE), which alters the plant community structure and soil properties (Liu et al., 2015; Li et al., 2019; Dang et al., 2023). Vegetation and soil factors influence the composition and function of the soil microbiome (Upton et al., 2020; Wang et al., 2022). The physical properties of soil are vital for CH4 circulation. Priano et al. (2018) reported that CH4 metabolism is primarily related to soil texture and that CH4 diffusivity in soil is affected by differences in silt and clay contents and soil bulk density, which in turn affect CH4 emissions. A previous study showed that CH4 uptake is positively correlated with soil moisture, temperature, and precipitation (Yue et al., 2016). Additionally, livestock is an essential driver of grassland vegetation succession, and increased quantity of leguminous forage promotes CH4 emission (Khalsa et al., 2014). Thus, plant community structure and soil physicochemical properties regulate methanotrophic and methanogenic communities.
In the past decades, owing to heavy grazing, alpine meadows on the Qinghai–Tibet Plateau have been largely degraded. In 2014, we conducted a GE experiment to track the restoration of the ecosystem. Currently, vegetation biomass and soil fertility could be considerably restored after fencing (Li et al., 2018). However, the underlying mechanism of CH4 metabolism in the plant growing season remains unclear. In the present study, in situ CH4 flux was determined in August 2020, and metagenomics was employed to analyze the composition of methanotrophic and methanogenic communities as well as the relative abundance of their functional genes in the shallow soil of grazed and nongrazed (2014–2020) alpine meadows. A 6-year study is necessary because the stability of the vegetation community and soil properties in alpine meadows reaches an optimum level only after 6 years of GE (Li et al., 2018). The resultant metagenomic, vegetation, and soil data were combined to (1) determine the effects of GE on the dominant CH4-metabolizing taxa in degraded alpine meadows; (2) identify the main CH4 uptake and production pathways based on alterations in related functional genes; (3) explore the heterogeneous responses of methanotrophic and methanogenic communities to GE. By analyzing the response of CH4 metabolic communities and functional genes to grazing exclusion, we will elucidate the key environmental factors regulating CH4 bioprocesses and provide a theoretical guide for decision making in natural restoration of grasslands by fencing.
The study site was located at the northeastern edge of the Qinghai–Tibetan Plateau in Tianzhu Tibetan Autonomous County, Gansu Province, China (37°10′12.92″N, 102°47′12.12″E; 3042.4 m above sea level, Figure 1A). This site exhibits a considerable temperature difference between day and night, has a cold and wet climate, and has no absolute frost-free period (Wang et al., 2020). The annual rainfall is 467.9 mm, with 76% of the rainfall being concentrated from June to September. The average annual temperature is 1.1°C, with the average temperature in July (the warmest month) being 13.7°C and that in January (the coldest month) being −10.3°C (Wang et al., 2022). Plants grow for ~120 days throughout the year, and alpine meadows comprise the primary vegetation type at the study site. The dominant herbages are mainly from the Gramineae, Salicaceae, and Polygonaceae families, and the dominant species include Poa pratensis, Elymus nutans, Polygonum viviparum, Kobresia humilis, Blysmus sinocompressus, and Kobresia capillifolia. Based on vegetation and soil characteristics before 2014, the meadowland at the experimental site was heavily degraded, according to a standard for alpine meadow degradation by Ma et al. (2002). A region with uniform vegetation distribution in the alpine meadows was selected as the experimental site in May 2014. Grazed plots (GPs) and nongrazed plots (NPs) were established in the degraded alpine meadows. Six replicates were used per plot, each with an area of 100 m × 50 m (Figure 1B). The grazing intensity in GPs was four sheep/ha, and grazing occurred continuously throughout the year. All types of grazing were prohibited in NPs from 2014 to 2020.
Figure 1. Experimental design and steps. Grazed and nongrazed plots were established in alpine meadows on the eastern Qinghai-Tibet Plateau in May 2014 (A), with six replicates in grazed and nongrazed plots (B). (C) Shows the sampling diagram of vegetation survey and soil sampling in August 2020, and blue dots indicate sampling points. (D) Shows the vegetation landscape of grazed and nongrazed plots. (E) Shows the experimental steps, soil was collected at a depth of 0–10 cm at each sampling point (five collections) and mixed into one sample. The pooled soil samples were divided into four parts using the tetrad method: One part was used to measure moisture, one part was cryopreserved (−80°C) for DNA extraction and sequencing, one part was used to determine total nutrient content, and one part was used to determine available nutrient content. Further details are provided in the main text.
In August 2020, acrylic chambers were used to determine CH4 flux in situ in GPs and NPs. An acrylic chamber consists of two parts: a base (50 cm × 50 cm × 15 cm) inlaid into 10-cm deep soil and a cube box (50 × 50 × 50 cm3) covering the base. The connection between the base and the box was sealed with water. In the plots, 12 chambers were installed and vegetation was cut. CH4 flux was measured at 9:00–11:00 daily for 1 week, and the gas in the box was collected using a syringe every 15 min (Xing et al., 2022). The box was covered with a white insulating foam during sampling to avoid the warming effect of sunlight (Zhang et al., 2013). Subsequently, the gas samples were analyzed within 48 h using a gas chromatograph (Agilent 6820A, CA, United States). CH4 was tested using a flame ionization detector, and N2 was used as the carrier gas to remove O2 and water vapor (Chen et al., 2017). The CH4 flux was calculated according to the method reported by Xing et al. (2022).
Vegetation and soil indices were measured in GPs and NPs (Figure 1D). To reduce the differences caused by soil spatial heterogeneity, 5 sampling points were selected in each plot using the “X” sampling method and mixed into one sample (Figure 1C), thus obtaining a total of 12 samples. To avoid boundary effects, the distance between all sampling points and the fence was maintained at >5 m. Soil temperature (ST) was measured at a depth of 0–10 cm at each sampling site for a week under clear weather conditions. Vegetation and litter features were surveyed using a sample square (1 m2) at each sampling site. In the sampling square, grass species, individual numbers, plant height (using a tape measure), and coverage were recorded. The aboveground biomass (including mosses) was determined via mowing, and the litter was collected. Furthermore, a soil auger (inner diameter, 3.5 cm) was used to collect soil at a depth of 0–10 cm. From the same sampling point, five samples were collected and pooled into one sample. The quadrat method was used to divide the soil into four parts (Figure 1E). The soil indicators included soil organic matter (SOM), available nitrogen (AN), available phosphorus (AP), available potassium (AK), total nitrogen, total phosphorus (TP), and total potassium (TK), which were measured according to the methods described by Bao (2000). The soil samples were stored at −80°C for DNA extraction and sequencing (six repetitions). Additionally, soil was collected from the sample squares at a depth of 0–10 cm using a soil auger (diameter, 10 cm), packed in mesh bags, and rinsed with water to obtain the plant roots. Soil samples were also collected from the sample squares using a cutting ring (diameter, 5 cm; height, 5 cm) and dried to a constant weight at 105°C, following which soil bulk density (SBD) and soil moisture (SM) were determined. All aboveground plant, root, and litter samples were dried to a constant weight at 70°C and then weighed to calculate grass aboveground biomass (GAB), grass root biomass (GRB), and litter biomass (LB). The importance value of the grass species was calculated as follows (Ren et al., 1998):
where , , , and indicate the relative values of grass height, coverage, density, and GAB, respectively.
Genomic DNA was extracted from the soil samples using hexadecyltrimethyl ammonium bromide (Mackelprang et al., 2011; Hultman et al., 2015). DNA degradation and potential contamination were detected via 1% agarose gel electrophoresis. DNA concentration was accurately measured using Qubit dsDNA Assay Kit and Qubit 2.0 Fluorescence Quantification Instrument (Life Technologies, CA, United States). The tested DNA was randomly spliced to obtain ~350-bp fragments using Covaris ultrasonic fragmentation device. End repair, A-tail addition, sequencing connector addition, purification, and polymerase chain reaction (PCR) amplification were performed. Libraries were constructed using NEB Next Ultra DNA Library Prep Kit for Illumina (NEB, MA, United States). The PCR products were purified and the library size distribution was analyzed using Agilent 2100 Bioanalyzer. Indexed coded samples were clustered using cBot Cluster Generation System according to the manufacturer’s instructions. Library preparations were sequenced using the Illumina NovaSeq6000 platform, generating pair-end sequences.
Metagenome sequencing was performed using the Illumina NovaSeq high-throughput sequencing platform to obtain data regarding raw bacteria, fungi, and archaea from 0 to 10-cm deep soil samples. The raw sequences were preprocessed to ensure the reliability of the data. In particular, the sequencing junction sequences were removed from the raw data using cutadapt, and quality control of the data was performed using KneadData software. FastQC was used to assess the rationality and effectiveness of the quality control process (Schmieder and Edwards, 2011). Following quality control, the processed data were compared with the host genome data using Bowtie2, and sequences that were not comparable to those of the host were retained for subsequent analysis. The quality of the sequencing data is shown in Supplementary Table S1. Kraken2 and a self-constructed microbial database were used for species annotation to identify the species present in the samples, and Bracken was used to predict their relative abundance (Lu et al., 2017). Kraken2 is the latest comparison software based on K-mer, and the local Kraken2 database contained 16,799 known bacterial genomes. Finally, functional annotation was performed based on reads (Wood and Salzberg, 2014) using HUMAnN2 software to match the reads of the individual samples with those in the database (UniRef90). Based on this analysis, annotation information and relative abundance tables were obtained for each functional database. Microbial biomarkers were identified at the species level using the linear discriminant analysis (LDA) effect size (LEfSe) method based on an LDA score of >2. Additionally, reads per kilobase per million mapped read values were used to estimate the relative abundance of functional genes (Kim et al., 2016). Default parameters were used for the abovementioned analyses.
Data were tested for normality and homogeneity of variances. Independent samples t-test was conducted between the two groups (GPs and NPs) when the data followed a normal distribution in Shapiro–Wilk and Levene’s tests. The data are presented as means ± standard errors, and statistical significance was indicated by a p-value of <0.05. If the data followed a non-normal distribution in Shapiro–Wilk or Levene’s test, a nonparametric test (Mann–Whitney U test) was used. Additionally, redundancy analysis was performed to determine the potential associations of drivers with CH4-metabolizing microbial communities and their functions. The random forest method was used to select soil indicators and construct soil composite variables. Structural equation models (SEMs) were used to analyze the interactions among vegetation, soil, microbial taxa, metabolic pathways, and CH4 flux. Furthermore, Spearman’s correlation analysis and environmental factor mapping with microbial composition and function were performed. The abovementioned analysis was performed using R version 4.2.2 with the R packages randomForest, Pheatmap, corrplot, piecewiseSEM, and ggplot2. Finally, a microbial co-occurrence network diagram (ρ > 0.7; p < 0.05) was constructed and mapped using BioinCloud.1
GE altered the CH4 flux, vegetation characteristics, and soil properties of the study site (Table 1). Although alpine meadow soil is a CH4 sink, compared with grazing, GE increased the CH4 flux of the 0–10-cm deep soil from −34.10 to −22.82 μg‧m−2‧h−1. In total, 73 grass species belonging to 46 genera and 22 families were recorded in the experimental plots (Figure 2A; Supplementary Table S2). The top five families in terms of species richness were Compositae, Gramineae, Cyperaceae, Gentianaceae, and Leguminosae. All species were categorized into four functional groups (Gramineae, Cyperaceae, Leguminosae, and forbs). GE decreased the importance value of Gramineae by 46.31% and increased the importance value of Cyperaceae by 388.03% (Figure 2B). Notably, a high proportion of forbs, particularly Bistorta vivipara, Equisetum arvense, and Thalictrum alpinum, was detected at the experimental site (Supplementary Table S2). GAB and GRB were higher in NPs than in GPs (p < 0.01), and grass species number (GSN) decreased by 40.30% after fencing. LB was 2.34 times higher in NPs than in GPs. Furthermore, compared with GPs, soil aggregates (SAs; >1.0 mm) and SM increased in NPs by 17.12 and 49.62%, respectively. However, SBD and pH were lower in NPs than in GPs. Regarding soil nutrition, the SOM, AN, AP, AK, TP, and TK contents detected in the soil were higher after GE than before GE.
Figure 2. Plant community composition at the family level and its importance values at the experimental site. (A) Grass species number at the family level. (B) Response of the importance values of the plant functional group to GE. **p < 0.01.
Table 2 presents the composition of MOB and MPA at family and genus levels as well as their relative abundances in response to GE. ANME was not detected in the soil samples. The relative abundance of MOB accounted for 0.34 and 0.30% of the total microorganisms in GPs and NPs, respectively. And the relative abundance of MPA accounted for 0.012 and 0.015% of the total microorganisms in GPs and NPs, respectively. Regarding CH4 uptake, type I MOB comprised 23 species from 12 genera and type II MOB comprised 10 species from 4 genera (Table 2 and Figure 3A). Conversely, Verrucomicrobia or the NC10 phylum was not detected in the analyzed samples. The level of species diversity in type I MOB was higher than that in type II MOB. However, their relative abundances exhibited the opposite trend. The relative abundances of type I MOB were 32.14% in GPs and 21.59% in NPs and those of type II MOB were 64.64% in GPs and 76.34% in NPs. Furthermore, GE decreased the relative abundances of type II MOB (Figure 3B). Among all MOB, the top five genera in terms of relative abundance were Methylocystis, Methylocapsa, Methylosinus, Methylocella, and Methylobacter, four of which belonged to type II MOB (Table 2). The top five species in terms of relative abundance were Methylocystis rosea, Methylosinus trichosporium, Methylocystis parvus, Methylocystis bryophila, and Methylocystis sp. SC2, all of which belonged to type II MOB (Figure 3A). These results revealed that type II MOB was the dominant taxa associated with CH4 uptake, indicating that the serine pathway plays a dominant role in CH4 uptake. Regarding CH4 production, MPA exhibited lower diversity and relative abundance than MOB. MPA comprised 3 genera (Methanobrevibacter, Methanosarcina, and Methanosphaera) and 12 species (Table 2, Figure 3A). Methanobrevibacter and Methanosarcina constituted the dominant genera, whereas Methanobrevibacter millerae was the absolute dominant species. GE increased the relative abundance of Methanobrevibacter, and Methanosarcina was not found in NPs. Additionally, GE reduced the relative abundance of MPA species by 35.71%, leading to the disappearance of nine species. However, the relative abundance of M. millerae increased significantly from 1.47 to 4.69% (p < 0.01).
Table 2. CH4-metabolizing microbial taxa and their relative abundances at the family and genus levels.
Figure 3. Response of microbial communities associated with CH4 metabolism to GE. (A) Relative abundance of type I MOB, type II MOB, and MPA at the species level (six repetitions). (B) Effect of GE on the relative abundance of type I MOB, type II MOB, and MPA. (C) A ternary diagram illustrating the compositions of type I MOB, type II MOB, and MPA. The size of the bubbles indicates the sum of the relative abundance of type I MOB, type II MOB, and MPA taxa. (D) Microbial biomarkers for CH4 metabolism at different taxonomic levels according to the linear discriminant analysis (LDA) effect size method (LDA score of >2). Uppercase letters indicate biomarkers, whereas lowercase letters indicate microbial taxa at different levels: c, class; o, order; f, family; g, genus; s, species. (E) Co-occurrence networks of soil microbial species. Visualization of the connectivity in type I MOB, type II MOB, and MPA taxa. Nodes indicate individual microbial species, and edges represent significant Spearman correlations (ρ > 0.7; p < 0.05). The size of the nodes indicates the relative abundance of species. Dark cyan edges indicate positive correlations, and light cyan edges indicate negative correlations. *p < 0.05, **p < 0.01.
As shown in the ternary diagram in Figure 3C, type II MOB was the most abundant among all CH4-metabolizing microbial communities. The LEfSe results revealed the presence of 12 and 8 biomarkers in GPs and NPs, respectively (Figure 3D). Methanmicrobia, Methanosarcinales, Methanosarcinaceae, and Methylocystaceae were the biomarkers in GPs, whereas Beijerinckiaceae was the biomarker in NPs. At the species level, Methylocapsa aurea, Methylocella silvestris, M. bryophila, and M. trichosporium were the biomarkers in NPs, whereas M. rosea, Methanosarcina mazei, M. sp. SC2, Methylobacter luteus, and Methylobacter tundripaludum were the biomarkers in GPs. The positive correlation among the species exceeded the negative correlation, and the top five species in terms of positive correlation were Methanosarcina barkeri, M. aurea, Methylocapsa palsarum, M. trichosporium, and M. silvestris. Among all species, M. barkeri was at the center of the network, with a maximum value of 28.75 for connectivity (Figure 3E).
In total, 25 and 22 functional genes associated with methanotrophic and methanogenic processes, respectively, were analyzed (Figure 4; Supplementary Figure S1). Among CH4 uptake-related genes, the top five functional genes in terms of relative abundance were ENO, glyA, mdh, fbaA, and pfk. Among them, three functional genes (ENO, glyA, and mdh) belonged to the serine pathway. The relative abundance of functional genes related to the serine pathway was higher than that of functional genes related to xylulose monophosphate (XuMP) and RuMP pathways (Figure 4C). Furthermore, GE decreased significantly the relative abundance of functional genes related to the serine pathway (p < 0.01). Among CH4 production-related genes, the top five functional genes in terms of relative abundance were ACSS, metH, pta, comD, and fdhA. The relative abundances of ACSS and metH were higher than those of other functional genes related to the CH4 production pathway. The order of the three pathways in terms of relative abundance was acetate pathway > CO2 pathway > methanol pathway. GPs exhibited a lower relative abundance of functional genes related to the CH4 production pathway than NPs (Figure 4C). One biomarker gene (mtrD) was detected in GPs and five biomarker genes (metH, pta, comA, fbaA, and hxlA) were detected in NPs (Figure 4D).
Figure 4. Response of the relative abundance of functional genes related to CH4 uptake (A) and production (B) to GE in GPs and NPs (six repetitions). (C) Relative abundance of genes involved in the different pathways of CH4 uptake and production. (D) Biomarker genes for CH4 metabolism according to the linear discriminant analysis effect size method (LDA score of >2). *p < 0.05, **p < 0.01, ***p < 0.001.
The responses of MOB and MPA communities and functional genes to vegetation and soil factors were analyzed. The top five influential factors were SM, AP, GSN, GAB, and TP for microbial taxa at the genus level (Figure 5A) and AP, SM, GSN, TP, and SAs for microbial taxa at the species level (Figure 5B). Overall, 10 genera were significantly affected by vegetation and soil factors (p < 0.05); 8 of these genera were type I MOB, 1 genus was a type II MOB, and 1 genus was a type MPA (Supplementary Figure S2). Overall, 19 species were significantly affected by vegetation and soil factors, and 68.42, 21.05, and 10.53% of them were type I MOB, type II MOB, and MPA, respectively (Figure 6). Thus, type I MOB were more likely to be influenced by vegetation and soil factors than type II MOB and MPA. The influence of environmental factors varied in different species. Among the top five genera in terms of relative abundance, Methylobacter and Methylocistis were primarily negatively correlated with environmental factors. Conversely, Methylocapsa, Methylosinus, and Methylocella were primarily positively correlated with environmental factors. M. parvus and M. bryophila, exhibiting the highest relative abundance, were not significantly correlated with the 16 environmental factors and showed strong stability. Conversely, M. rosea was significantly positively correlated with GSN and SBD and significantly negatively correlated with SAs, LB, SOM, AP, AN, SM, and GAB. The trend observed for M. tundripaludum and Methylococcus capsulatus was similar to that observed for M. rosea, i.e., they were insensitive to the effects of environmental factors (Figures 5B, 6). Type I MOB were negatively correlated with 4 environmental factors and positively correlated with 12 environmental factors, with 72.31% of the correlations being significant. Type II MOB were negatively correlated with 5 environmental factors and positively correlated with 11 environmental factors, with 24.37% of the correlations being significant. MPA were negatively correlated with eight environmental factors and positively correlated with eight environmental factors, with all correlations being nonsignificant (Figure 5C).
Figure 5. Redundancy analysis of vegetation features, soil properties, and CH4 metabolism-related taxa at the genus (A) and species (B) levels; type I MOB, type II MOB, and MPA (C); CH4 uptake and production pathways (D); and functional genes (E). Red dots represent the top five genera in (A), species in (B), and functional genes in (D) in terms of relative abundance. The size of the points reflects the relative abundance. GSN, grass species number; GAB, grass aboveground biomass; GRB, grass root biomass; LB, litter biomass; SBD, soil bulk density; SAs, soil aggregates; ST, soil temperature; SM, soil moisture; SOM, soil organic matter; AN, available nitrogen; AP, available phosphorus; AK, available potassium; TN, total nitrogen; TP, total phosphorus; TK, total potassium. The same as below.
Figure 6. Correlation analysis including MOB and MPA at the species level and environmental factors. Red indicates a positive relationship and blue indicates a negative relationship. Features with no significant correlation were removed. *p < 0.05, **p < 0.01, ***p < 0.001.
Environmental factors were found to affect CH4 metabolic pathways, which explained 49.81% (RDA1) and 28.54% (RDA2) of the variation (Figure 5D). The CH4 uptake pathway was primarily negatively correlated with environmental factors, whereas the CH4 production pathway was primarily positively correlated with environmental factors. Specifically, compared with XuMP and RuMP pathways, the serine pathway was extremely sensitive to alterations in vegetation and environmental factors. The low proportion (12.5–18.7%) of significant correlations between the 16 environmental factors and CH4 production pathways is shown in Supplementary Table S3. Therefore, the CH4 production pathway was found to be more stable than the CH4 uptake pathway. Among the top five genes in terms of relative abundance, mdh, ENO, ACSS, and glyA were positively correlated with SBD, GSN, TN, and ST (Figure 5E; Supplementary Table S4).
Finally, soil composite variables were established based on soil indicators (AP, SM, SBD, and SAs) using random forest analysis (Supplementary Figure S3), and the SEMs of vegetation, soil, microbial taxa, and CH4 metabolic pathways were established (Figure 7). Overall, the influence of soil on CH4 flux was more significant than that of vegetation. The type II MOB community was primarily affected by differences in vegetation, with a value of 0.41. Conversely, type I MOB and MPA communities were primarily affected by alterations in soil properties, with values of 0.73 and 0.31, respectively. CH4 flux was mainly regulated by serine and acetate pathways, with values of −0.85 and 0.79, respectively. The serine pathway was primarily driven by soil factors, whereas the acetate pathway was jointly driven by vegetation and soil factors. The SEMs revealed that high plant diversity and biomass were conducive for CH4 uptake. Conversely, compact and water-filled soil was conducive to CH4 production.
Figure 7. Response of CH4 flux to vegetation and soil factors. GE affects CH4 flux by altering different soil microbial taxa (A) and pathways (B). The solid line indicates a significant level (p < 0.05), whereas the dotted line indicates a nonsignificant level. The size of the line indicates the value of the influence coefficient. *p < 0.05, **p < 0.01, ***p < 0.001. The line with an arrow indicates that the response of the indicator to grazing is increasing or decreasing in (B). Global goodness of fit: Fisher’s C = 5.02, p = 0.081, AIC = 33.02, BIC = 72.05 in (A); Fisher’s C = 11.37, p = 0.078, AIC = 49.37, BIC = 102.33 in (B).
The effect of livestock on alpine meadows was determined via three mechanisms: trampling, defoliation (removal of plant shoot tissue), and returning of dung and urine (Liu et al., 2015). After removing grazers, higher contents of SOM, AN, AP, and AK were detected, possibly owing to the input of plant residues. The composition and transport of soil nitrogen alter methanotrophic activities (Jeremy et al., 2010). For example, ammonia nitrogen typically reduces CH4 uptake via product toxicity or competitive binding in CH4 monooxygenase (Nold et al., 1999; Jeremy et al., 2010). The physical properties of soil improved in terms of SBD and SAs (>1.0 mm; Table 1), which was conducive to vegetation growth (Li et al., 2018). In grassland ecosystems, herbage is an essential factor affecting CH4 metabolism, and CH4 fluxes in soil are altered by plant species and vegetation types (Praeg et al., 2017). Large herbivores promote the expansion of Gramineae forage by reducing the coverage of moss and shrubs via trampling and ingestion (Kitti et al., 2009; Falk et al., 2015). A high proportion of Leguminosae forage in a community increases CH4 emissions from shallow soil, whereas a high proportion of Gramineae forage decreases CH4 emissions (Khalsa et al., 2014). In the present study, GE significantly decreased the proportion of Gramineae forage but had no significant effect on Leguminosae forage (Figure 2). A high proportion of forbs, such as Bistorta vivipara, Equisetum arvense, and Thalictrum alpinum, was observed in GPs and NPs. Continuous high-intensity grazing increases the coverage of species that can adapt to drought habitats, such as Plantago depressa and Leontopodium nanum. However, it reduces the yield of high-quality forages, such as Elymus dahuricus, E. nutans, and Elymus sibiricus. GE can effectively reduce grassland degradation and accelerate ecological restoration. Reportedly, short-term GE can enhance plant diversity, whereas long-term GE can reduce species richness (Li et al., 2017), plant productivity, and soil organic carbon content (Cao et al., 2022). Fencing for 6 years may be adequate for vegetation and soil restoration (Li et al., 2018). Therefore, a 6-year GE period was selected in this study. However, fencing for 6 years reduced the potential of the CH4 sink in this study, and the optimal GE duration remains unclear. Short-term GE can enhance vegetation biomass, increase the proportion of large SAs, and reduce SBD. However, the effect of GE on vegetation characteristics tends to vary, and it is necessary to further distinguish the heterogeneous effects of GE on the vegetation of different patches in alpine regions.
The amount of CH4 emissions from the soil to the atmosphere depends on the balance between methanotrophic and methanogenic communities. Our analyses of relative abundance and functional genes indicated that type II MOB were the dominant taxa involved in CH4 uptake in alpine meadow soil (Figures 3, 4). Conversely, type I MOB were the dominant taxa in wetlands, where Methylomonas, Methylobacter, and Methylovulum dominated the community (Liu et al., 2020). The MOB in sediment samples comprised Methylococcus, Methylobacter, and Methylosinus (type II MOB); type I MOB species are usually more abundant than type II MOB species (Yang et al., 2016). Our knowledge regarding metabolic versatility of type II MOB is expanding. For example, type II MOB were initially considered to have dedicated growth requirements. However, studies have shown that numerous species of type II MOB are parthenogenic and capable of growing in the presence of multicarbon compounds. For example, the strain SC2 can grow with mixed nutrients in the presence of H2 and CH4 (Myung et al., 2016; Wen et al., 2016; Hakobyan and Liesack, 2020). Further, M. silvestris can use various organic acids (succinate, pyruvate, malate, and acetate) and ethanol as the only growth substrates (Dedysh et al., 2005). Type II MOB exhibits distinct advantages over type I MOB, including a high acetyl coenzyme flux and the combination of two important greenhouse gasses (CH4 and CO2), and has been considered for industrial application (Nguyen et al., 2021). The findings of this study revealed that type I MOB can grow rapidly in a heterogeneous environment. However, type II MOB can adapt to more significant environmental fluctuations, especially in soils with high CH4 concentration (Macalady et al., 2002; Jeremy et al., 2010). Some studies have classified Types I and II MOB as R- and K-strategists, respectively (Steenbergh et al., 2009; Siljanen et al., 2011). ANME can proliferate in anoxic environments and drive the CH4 cycle in coastal sediments (Wallenius et al., 2021); however, they were not detected in this study. This may be related to the soil texture; SAs (>1.0 mm) accounted for 55.96–65.54% of the total particles, and the loose structure of soils facilitated oxygen flow. In addition, the ANME sequence library should be further expanded to ensure the accuracy of species annotation.
The dominant microbial taxa drive soil microbial functions (Jiao et al., 2022). Thus, understanding the composition of dominant species can help determine grassland CH4 sink and source transition. In the present study, the MOB genus Methylocystis exhibited the highest relative abundance. Some species were classified as facultative MOB because they used various carbon substrates, such as acetate and methanol. They grew in the temperature range of 15°C–37°C and pH range of 6.5–10. Their genome comprised two particulate CH4 monooxygenases (pMMOs), one for low-affinity CH4 oxidation and the other for high-affinity CH4 oxidation. Additionally, several genes are involved in nitrogen fixation, hydrogenase production, heavy metal resistance, and polyhydroxybutyrate synthesis (Jung et al., 2020). The diverse energy sources and metabolic versatility of type II MOB may explain their dominant CH4 uptake in alpine meadow soil. In grazing systems, MOB is a food source rich in K and contains certain trace elements, such as Mg and Fe (Kuzniar et al., 2019). From a thermodynamic perspective, the RuMP pathway is a more efficient carbon assimilation pathway than the serine pathway (Wang, 2020). Notably, almost all Gammaproteobacteria of MOB belong to the Methylococacaceae family. However, the Crenothrix genus belongs to the Crenotrichaceae family (Knief, 2015). Some cultivation-independent species remain poorly delineated, such as jasper ridge clusters (JR1, JR2, and JR3), upland soil clusters (USCα and USCγ), Moore house peat clusters, and rice paddy clusters. Thus, it is challenging to determine the exact number of known MOB at the species level because the taxonomic status of some species, such as Methylococcus thermophilus, Methylococcus mobilis, and Methylococcus chroococcus, remain unclear (Knief, 2015). Limited information on these uncultured methanotrophic and methanogenic bacteria restricts data interpretation.
GE increased the relative abundance of Methanobrevibacter, the dominant genus of MPA in alpine meadow soil. Methanomethylovorans is a widespread methanogenic archaeal genus that exists under freshwater conditions with a broad niche (Tsola et al., 2021). GE increased the GAB and SM, which decreased ST, consequently leading to the decline of Methanosarcina because the species in this genus are sensitive to temperature changes. A study reported that the relative abundance of Methanosarcina increased when the temperature increased from 10°C to 30°C (Chen et al., 2022). This increase may also be caused by alterations in substrates, such as acetate, CO2, and methanol, in the soil because Methanosarcina species are multitrophic methanogenic archaea that can use various simple compounds as substrates (Chen et al., 2022). Additionally, species such as M. mazei, M. barkeri, and M. flavescens were found only in NPs (Figure 4). The disappearance of archaeal species may be due to the removal of livestock excreta input, and methanogens are highly abundant in the intestines and feces of livestock (Vechi et al., 2022). However, the abundance of M. millerae (the absolute dominant species) increased by 219.05% in NPs compared to NPs. Thus, GE reduced the diversity of MPA but increased the relative abundance of the dominant methanogenic species.
This study assessed multiple functional genes together to analyze CH4 metabolic pathways. Previous studies have primarily used pmoA and mcrA to analyze microbial CH4 oxidation and production processes (Bhardwaj and Dubey, 2020; Zhang et al., 2022). pmoA is a common functional gene in MOB and is often used to assess the diversity and phylogeny of MOB in different environments (Pandit et al., 2016; Wen et al., 2016). The final step of methanogenesis is catalyzed by the protein encoded by mcrA, which is abundant in MPA and is considered a functional gene used to detect methanogenic community diversity (Harirchi et al., 2022; Shen et al., 2022). However, MOB detection using pmoA as a functional marker can reveal thousands of sequences representing unknown methanotrophic bacteria. This limits data interpretation as information regarding these uncultured MOB is limited. Some uncultured methanotrophs are considered vital for CH4 oxidation, although the biological mechanisms underlying the effects of other sequenced groups remain unclear (Knief, 2015). pmoA must be used cautiously when interpreting data regarding unknown MOB. In the present study, the acetate pathway was the dominant CH4 production pathway in terms of the relative abundance of related genes. ACSS alone catalyzes the conversion of acetate to acetyl-CoA, whereas ackA and pta together complete this process, thus increasing the extent of functional redundancy and improving the stability of the acetate pathway. fwdA, which catalyzes the production of formyl-MFR from CO2, a critical step in the acetate pathway, was found only in NPs.
CH4 metabolism is regulated by various environmental factors (Wei et al., 2015; Liu et al., 2021), of which SM content and temperature are considered the dominant factors that control the CH4 cycle in grasslands (Wang et al., 2014). In the present study, AP, SAs, TP, GSN, and SM were the top five factors. The microbial community showed a positive response to soil phosphorus, especially under phosphorus-deficient soil conditions (Chen and Xiao, 2023); this is because soil phosphorus deficiency limits MOB growth (Sheng et al., 2016). Furthermore, GE increases AP content, thereby favoring the development of bacterial communities. The effect of SAs was second only to that of AP. Substantial interactions occur between microbial communities and soil properties during CH4 flux regulation (Sengupta and Dick, 2017; Zhang et al., 2022). Unlike MOB, MPA are specialized, strict anaerobes sensitive to oxygen. Thus, they are inhibited and sometimes killed under oxygen-rich conditions. MPA may grow gradually as they have limited available substrates and can only use simple substances, such as CO2, H2, formic acid, acetate, and methylamine. Such simple substances must be transformed by other fermentative bacteria, which break down complex organic matter and make it available to MPA (Chen et al., 2022). GE can indeed improve the physical properties of soil (SBD, SAs, and SM), which impact microbial communities and CH4 flux diffusivity. In addition, this study examined the response of three methanogenic pathways in the KEGG database to grazing exclusion. Previous studies have shown that methanogens are classified into three groups, including hydrogenotrophic, aceticlastic and methylotrophic, based on their substrate utilization (Lyu et al., 2018). Different classification categories may lead to different results, but the response of dominant species to environmental factors is certain, and focusing on changes in dominant species may be crucial to explaining CH4-oxidation.
Notably, MOB and MPA exhibited heterogeneous responses to environmental factors in this study. Type I MOB were primarily affected by SM and SBD, whereas type II methanotrophs were primarily affected by TP, SBD, and SM. Microvirga ossetica, M. extorquens, M. rosea, M. populi, and P. koreensis belong to MOB. M. ossetica and M. rosea were positively and negatively affected by AP, respectively. Therefore, changes in AP cannot be interpreted only as a decrease or increase in the CH4 uptake potential. The insensitivity of M. populi and P. koreensis to environmental factors increases the stability of the MOB community under different environmental conditions. A high proportion of species in type I MOB varied synergistically with the environment, whereas an even higher proportion of species in type II MOB and MPA varied antagonistically with the environment. Thus, type I MOB were more susceptible to environmental impacts than type II MOB and MPA. When the feedback of species to environmental factors was diverse, the redundancy of community functions increased.
Large herbivores are additional factors that may substantially alter high-latitude landscape characteristics. However, their potential impact is rarely considered in the alpine ecosystem (Fischer et al., 2022). The aboveground biomass in fenced meadows was higher than that in grazing meadows, i.e., the abundance of Leguminosae and Poaceae forage increased and that of Cyperaceae forage decreased due to grazing (Li et al., 2019). Regarding belowground ecosystem properties, studies have shown that changes in ST, moisture, and texture are associated with disturbances caused by large animals (Olofsson et al., 2001; Beer et al., 2020). However, forbs, including B. vivipara, E. arvense, and T. alpinum, were the dominant species (78.9% in GPs and 72.1% in NPs) in the experimental area in this study. Nevertheless, there is a lack of reference data regarding CH4 uptake and production from forb patches and their responses to GE. In the present study, alpine meadow soil was a CH4 sink (including GPs and NPs in the growing season) whose potential was reduced by GE. However, SEMs revealed that the influence of soil (especially soil physical factors) on CH4 flux was greater than that of vegetation. Moreover, an increase in SM negatively affects aerobic MOB, and a decrease in plant diversity may promote this trend. CH4 can be produced not only by microorganisms (microbial CH4) but also by natural gas sealed under or inside the permafrost that is emitted during thawing (thermogenic CH4) (Froitzheim et al., 2021). During autumn and winter, the primary source of CH4 is thermogenic CH4, and increased CH4 concentration associated with microbial processes is observed during spring and summer (Li et al., 2022). The CH4 produced by abiotic factors and different lifestyles of plants should be investigated in studies on CH4 flux, and their changes should be monitored in different seasons to refine CH4 studies in alpine meadow ecosystems.
GE significantly altered the vegetation structure, increasing the importance value of Cyperaceae while decreasing the importance value of Graminaea, and improved the physical properties and nutrient content of the soil. Alpine meadow soil is a CH4 sink; type II MOB were the dominant taxa involved in CH4 uptake, with the representative group of Methylocistis. The diversity and abundance of MPA were significantly lower than those of MOB, with the representative group of Methanobrevibacter. M. aurea, M. silvestris, M. bryophila, and M. trichosporium were the biomarkers in NPs, whereas M. rosea, M. mazei, M. sp. SC2, M. luteus, and M. tundripaludum were the biomarkers in GPs. CH4 flux was mainly regulated by serine and acetate pathways, and the serine pathway was primarily driven by soil factors, whereas the acetate pathway was mainly driven by soil and vegetation factors. The influence of soil on methanotrophic and methanogenic microbial taxa was greater than that of vegetation. Type II MOB and MPA taxa were more stable during the response to environmental changes than type I MOB. Regarding CH4 production, GE reduced the diversity of MPA. However, the relative abundance of M. millerae (the absolute dominant species in the methanogenic community) increased substantially. Our study shows that GE increases soil CH4 flux and alters methanotrophic and methanogenic communities during the growing season in alpine meadows. However, the functions of soil microbial communities are usually determined by dominant taxa, and further studies are needed to characterize the biology of Methylocystis and Methanobrevibacter, the dominant genus in alpine meadow soils, and their responses to environmental factors.
The datasets presented in this study can be found in online repositories. The names of the repository/repositories and accession number(s) can be found at: https://www.ncbi.nlm.nih.gov/, PRJNA837727.
SW: Conceptualization, Data curation, Funding acquisition, Methodology, Software, Writing – original draft. XC: Investigation, Software, Writing – review & editing. WL: Investigation, Writing – review & editing. WG: Investigation, Methodology, Writing – review & editing. ZW: Software, Writing – review & editing. WC: Data curation, Funding acquisition, Methodology, Software, Writing – review & editing.
The author(s) declare financial support was received for the research, authorship, and/or publication of this article. This work was supported by the Fostering Foundation for the Excellent Ph.D. Dissertation of Gansu Agricultural University [grant number YB2020004] and the National Natural Science Foundation of China [grant number 32060269].
We are grateful to Tianzhu Scientific Observation and Research Station for Alpine Grassland Ecosystem for providing access to the experimental field and facilities, and Wekemo Tech Co., Ltd., Shenzhen, China, for soil microbiomes sequencing.
The authors declare that the research was conducted in the absence of any commercial or financial relationships that could be construed as a potential conflict of interest.
All claims expressed in this article are solely those of the authors and do not necessarily represent those of their affiliated organizations, or those of the publisher, the editors and the reviewers. Any product that may be evaluated in this article, or claim that may be made by its manufacturer, is not guaranteed or endorsed by the publisher.
The Supplementary material for this article can be found online at: https://www.frontiersin.org/articles/10.3389/fmicb.2023.1293720/full#supplementary-material
Beer, C., Zimov, N., Olofsson, J., Porada, P., and Zimov, S. (2020). Protection of permafrost soils from thawing by increasing herbivore density. Sci. Rep. 10:4170. doi: 10.1038/s41598-020-60938-y
Bhardwaj, Y., and Dubey, S. K. (2020). Changes in pmoA gene containing methanotrophic population and methane oxidation potential of dry deciduous tropical forest soils. Curr. Sci. 118, 750–758. doi: 10.18520/cs/v118/i5/750-758
Cao, J., Jiao, Y., Che, R., Holden, N. M., Zhang, X., Biswas, A., et al. (2022). The effects of grazer exclosure duration on soil microbial communities on the Qinghai-Tibetan Plateau. Sci. Total Environ. 839:156238. doi: 10.1016/j.scitotenv.2022.156238
Chen, X., Genxu, W., Zhang, T., Mao, T., Wei, D., Hu, Z., et al. (2017). Effects of warming and nitrogen fertilization on GHG flux in the permafrost region of an alpine meadow. Atmos. Environ. 157, 111–124. doi: 10.1016/j.atmosenv.2017.03.024
Chen, Y., Wu, N. Y., Liu, C. L., Mi, T. Z., Li, J., He, X. L., et al. (2022). Methanogenesis pathways of methanogens and their responses to substrates and temperature in sediments from the South Yellow Sea. Sci. Total Environ. 815:152645. doi: 10.1016/j.scitotenv.2021.152645
Chen, C., and Xiao, W. (2023). The global positive effect of phosphorus addition on soil microbial biomass. Soil Biol. Biochem. 176:108882. doi: 10.1016/j.soilbio.2022.108882
Dang, Z., Guo, N., Li, S., Degen, A., Cao, J., Deng, B., et al. (2023). Effect of grazing exclusion on emission of greenhouse gases and soil organic carbon turnover in alpine shrub meadow. Sci. Total Environ. 858:159758. doi: 10.1016/j.scitotenv.2022.159758
De Bernardi, M., Priano, M. E., Fernández, M. E., Gyenge, J., and Juliarena, M. P. (2022). Impact of land use change on soil methane fluxes and diffusivity in Pampean plains, Argentina. Agric. Ecosyst. Environ. 329:107866. doi: 10.1016/j.agee.2022.107866
Dedysh, S. N., Knief, C., and Dunfield, P. F. (2005). Methylocella species are facultatively methanotrophic. J. Bacteriol. 187, 4665–4670. doi: 10.1128/JB.187.13.4665-4670.2005
Dutaur, L., and Verchot, L. V. (2007). A global inventory of the soil CH4 sink. Global Biogeochem. Cycles 21:GB4013. doi: 10.1029/2006GB002734
Falk, J. M., Schmidt, N. M., Christensen, T. R., and Ström, L. (2015). Large herbivore grazing affects the vegetation structure and greenhouse gas balance in a high arctic mire. Environ. Res. Lett. 10:045001. doi: 10.1088/1748-9326/10/4/045001
Fischer, W., Thomas, C. K., Zimov, N., and Gockede, M. (2022). Grazing enhances carbon cycling but reduces methane emission during peak growing season in the Siberian Pleistocene Park tundra site. Biogeosciences 19, 1611–1633. doi: 10.5194/bg-19-1611-2022
Froitzheim, N., Majka, J., and Zastrozhnov, D. (2021). Methane release from carbonate rock formations in the Siberian permafrost area during and after the 2020 heat wave. Proc. Natl. Acad. Sci. 118:e2107632118. doi: 10.1073/pnas.2107632118
Hakobyan, A., and Liesack, W. (2020). Unexpected metabolic versatility among type II methanotrophs in the Alphaproteobacteria. Biol. Chem. 401, 1469–1477. doi: 10.1515/hsz-2020-0200
Hanson, R. S., and Hanson, T. E. (1996). Methanotrophic bacteria. Microbiol. Rev. 60, 439–471. doi: 10.1128/MMBR.60.2.439-471.1996
Harirchi, S., Wainaina, S., Sar, T., Nojoumi, S. A., Parchami, M., Parchami, M., et al. (2022). Microbiological insights into anaerobic digestion for biogas, hydrogen or volatile fatty acids (VFAs): a review. Bioengineered 13, 6521–6557. doi: 10.1080/21655979.2022.2035986
Hultman, J., Waldrop, M. P., Mackelprang, R., David, M. M., McFarland, J., Blazewicz, S. J., et al. (2015). Multi-omics of permafrost, active layer and thermokarst bog soil microbiomes. Nature 521, 208–212. doi: 10.1038/nature14238
Jeremy, D. S., Alan, A. D., and Sukhwan, Y. (2010). Methanotrophs and copper. FEMS Microbiol. Rev. 34, 496–531. doi: 10.1111/j.1574-6976.2010.00212.x
Jiao, S., Chen, W. M., and Wei, G. H. (2022). Core microbiota drive functional stability of soil microbiome in reforestation ecosystems. Glob. Chang. Biol. 28, 1038–1047. doi: 10.1111/gcb.16024
Jung, G. Y., Rhee, S. K., Han, Y. S., and Kim, S. J. (2020). Genomic and physiological properties of a facultative methane-oxidizing bacterial strain of methylocystis sp. from a Wetland. Microorganisms 8:1719. doi: 10.3390/microorganisms8111719
KEGG (2022). Methane metabolism [Online]. Available at: https://www.kegg.jp/pathway/map00680.
Khalsa, J., Fricke, T., Weigelt, A., and Wachendorf, M. (2014). Effects of species richness and functional groups on chemical constituents relevant for methane yields from anaerobic digestion: results from a grassland diversity experiment. Grass Forage Sci. 69, 49–63. doi: 10.1111/gfs.12028
Kim, J. W., Kim, M. S., Koh, A. Y., Xie, Y., and Zhan, X. W. (2016). FMAP: Functional Mapping and Analysis Pipeline for metagenomics and metatranscriptomics studies. BMC Bioinformatics 17:420. doi: 10.1186/s12859-016-1278-0
Kitti, H., Forbes, B. C., and Oksanen, J. (2009). Long- and short-term effects of reindeer grazing on tundra wetland vegetation. Polar Biol. 32, 253–261. doi: 10.1007/s00300-008-0526-9
Knief, C. (2015). Diversity and habitat preferences of cultivated and uncultivated aerobic methanotrophic bacteria evaluated based on pmoA as molecular marker. Front. Microbiol. 6:1346. doi: 10.3389/fmicb.2015.01346
Kuzniar, A., Furtak, K., Wlodarczyk, K., Stepniewska, Z., and Wolinska, A. (2019). Methanotrophic bacterial biomass as potential mineral feed ingredients for animals. Int. J. Environ. Res. Public Health 16:2674. doi: 10.3390/ijerph16152674
Li, W., Cao, W., Wang, J., Li, X., Xu, C., and Shi, S. (2017). Effects of grazing regime on vegetation structure, productivity, soil quality, carbon and nitrogen storage of alpine meadow on the Qinghai-Tibetan Plateau. Ecol. Eng. 98, 123–133. doi: 10.1016/j.ecoleng.2016.10.026
Li, W. J., Liu, S. S., Li, J. H., Zhang, R. L., Rang, K. Z. C., Zhou, H. K., et al. (2019). Plant traits response to grazing exclusion by fencing assessed via multiple classification approach: a case from a subalpine meadow. Pol. J. Ecol. 67, 33–52. doi: 10.3161/15052249pje2019.67.1.003
Li, W., Liu, Y. Z., Wang, J. L., Shi, S. L., and Cao, W. X. (2018). Six years of grazing exclusion is the optimum duration in the alpine meadow-steppe of the north-eastern Qinghai-Tibetan Plateau. Sci. Rep. 8:17269. doi: 10.1038/s41598-018-35273-y
Li, X. Q., Xing, J. W., Pang, S. J., Zhu, Y. H., Zhang, S., Xiao, R., et al. (2022). Carbon isotopic evidence for gas hydrate release and its significance on seasonal wetland methane emission in the muli permafrost of the Qinghai-Tibet Plateau. Int. J. Environ. Res. Public Health 19:2437. doi: 10.3390/ijerph19042437
Liu, J. M., Bao, Z. H., Cao, W. W., Han, J. J., Zhao, J., Kang, Z. Z., et al. (2020). Enrichment of type I methanotrophs with nirS genes of three emergent macrophytes in a eutrophic wetland in China. Microbes Environ. 35:n/a. doi: 10.1264/jsme2.ME19098
Liu, N., Kan, H. M., Yang, G. W., and Zhang, Y. J. (2015). Changes in plant, soil, and microbes in a typical steppe from simulated grazing: explaining potential change in soil C. Ecol. Monogr. 85, 269–286. doi: 10.1890/14-1368.1
Liu, Z. Y., Li, H. Z., Wang, T. Q., Huang, N., Huang, Z. X., Luo, Y. Q., et al. (2021). Grassland restoration measures alter soil methane uptake by changing community phylogenetic structure and soil properties. Ecol. Indic. 133:108368. doi: 10.1016/j.ecolind.2021.108368
Lu, J., Breitwieser, F. P., Thielen, P., and Salzberg, S. L. (2017). Bracken: estimating species abundance in metagenomics data. Peerj Comput. Sci. 3:e104. doi: 10.7717/peerj-cs.104
Lyu, Z., Shao, N., Akinyemi, T., and Whitman, W. B. (2018). Methanogenesis. Curr. Biol. 28, R727–R732. doi: 10.1016/j.cub.2018.05.021
Ma, Y., Lang, B., Li, Q., Shi, J., and Dong, Q. (2002). Study on rehabilitating and rebuilding technologies for degenerated alpine meadow in the Changjiang and Yellow river source region. Pratacult. Sci. 19, 1–5.
Macalady, J. L., McMillan, A. M. S., Dickens, A. F., Tyler, S. C., and Scow, K. M. (2002). Population dynamics of type I and II methanotrophic bacteria in rice soils. Environ. Microbiol. 4, 148–157. doi: 10.1046/j.1462-2920.2002.00278.x
Mackelprang, R., Waldrop, M. P., DeAngelis, K. M., David, M. M., Chavarria, K. L., Blazewicz, S. J., et al. (2011). Metagenomic analysis of a permafrost microbial community reveals a rapid response to thaw. Nature 480, 368–371. doi: 10.1038/nature10576
Myung, J., Flanagan, J. C. A., Waymouth, R. M., and Criddle, C. S. (2016). Methane or methanol-oxidation dependent synthesis of poly (3-hydroxybutyrate-co-3-hydroxyvalerate) by obligate type II methanotrophs. Process Biochem. 51, 561–567. doi: 10.1016/j.procbio.2016.02.005
Nguyen, D. T. N., Lee, O. K., Nguyen, T. T., and Lee, E. Y. (2021). Type II methanotrophs: A promising microbial cell-factory platform for bioconversion of methane to chemicals. Biotechnol. Adv. 47:107700. doi: 10.1016/j.biotechadv.2021.107700
Nold, S. C., Boschker, H. T. S., Pel, R., and Laanbroek, H. J. (1999). Ammonium addition inhibits 13C-methane incorporation into methanotroph membrane lipids in a freshwater sediment. FEMS Microbiol. Ecol. 29, 81–89. doi: 10.1111/j.1574-6941.1999.tb00600.x
Olofsson, J., Kitti, H., Rautiainen, P., Stark, S., and Oksanen, L. (2001). Effects of summer grazing by reindeer on composition of vegetation, productivity and nitrogen cycling. Ecography 24, 13–24. doi: 10.1034/j.1600-0587.2001.240103.x
Padilla, C. C., Bristow, L. A., Sarode, N., Garcia-Robledo, E., Ramirez, E. G., Benson, C. R., et al. (2016). NC10 bacteria in marine oxygen minimum zones. ISME J. 10, 2067–2071. doi: 10.1038/ismej.2015.262
Pandit, P. S., Ranade, D. R., Dhakephalkar, P. K., and Rahalkar, M. C. (2016). A pmoA-based study reveals dominance of yet uncultured Type I methanotrophs in rhizospheres of an organically fertilized rice field in India. 3 Biotech 6:135. doi: 10.1007/s13205-016-0453-3
Praeg, N., Wagner, A. O., and Illmer, P. (2017). Plant species, temperature, and bedrock affect net methane flux out of grassland and forest soils. Plant Soil 410, 193–206. doi: 10.1007/s11104-016-2993-z
Priano, M. E., Fuse, V. S., Mestelan, S., Berkovic, A. M., Guzman, S. A., Gratton, R., et al. (2018). Afforested sites in a temperate grassland region: influence on soil properties and methane uptake. Agrofor. Syst. 92, 311–320. doi: 10.1007/s10457-017-0104-7
Ren, J., Zhang, Z., Fu, Y., Wang, Y., Sun, J., Zhu, X., et al. (1998). Grassland Science Research Methods. Beijing: Chinese Agriculture Press.
Schmieder, R., and Edwards, R. (2011). Quality control and preprocessing of metagenomic datasets. Bioinformatics 27, 863–864. doi: 10.1093/bioinformatics/btr026
Schmitz, R. A., Peeters, S. H., Versantvoort, W., Picone, N., Pol, A., Jetten, M. S. M., et al. (2021). Verrucomicrobial methanotrophs: ecophysiology of metabolically versatile acidophiles. FEMS Microbiol. Rev. 45:fuab007. doi: 10.1093/femsre/fuab007
Sengupta, A., and Dick, W. A. (2017). Methanotrophic bacterial diversity in two diverse soils under varying land-use practices as determined by high-throughput sequencing of the pmoA gene. Appl. Soil Ecol. 119, 35–45. doi: 10.1016/j.apsoil.2017.05.031
Shen, L. D., Geng, C. Y., Ren, B. J., Jin, J. H., Huang, H. C., Liu, X., et al. (2022). Detection and quantification of candidatus methanoperedens-like archaea in freshwater wetland soils. Microb. Ecol. 85, 441–453. doi: 10.1007/s00248-022-01968-z
Sheng, R., Chen, A. L., Zhang, M. M., Whiteley, A. S., Kumaresan, D., and Wei, W. X. (2016). Transcriptional activities of methanogens and methanotrophs vary with methane emission flux in rice soils under chronic nutrient constraints of phosphorus and potassium. Biogeosciences 13, 6507–6518. doi: 10.5194/bg-13-6507-2016
Siljanen, H. M. P., Saari, A., Krause, S., Lensu, A., Abell, G. C. J., Bodrossy, L., et al. (2011). Hydrology is reflected in the functioning and community composition of methanotrophs in the littoral wetland of a boreal lake. FEMS Microbiol. Ecol. 75, 430–445. doi: 10.1111/j.1574-6941.2010.01015.x
Steenbergh, A. K., Meima, M. M., Kamst, M., and Bodelier, P. L. E. (2009). Biphasic kinetics of a methanotrophic community is a combination of growth and increased activity per cell. FEMS Microbiol. Ecol. 71, 12–22. doi: 10.1111/j.1574-6941.2009.00782.x
Stocker, T., Qin, D., Plattner, G., Tignor, M., Allen, S., Boschung, J., et al. (2013). Climate change 2013: the physical science basis. In: Contribution of working group i to the fifth assessment report of the intergovernmental panel on climate change. Cambridge: Cambridge University Press.
Tang, S. M., Ma, L., Wei, X. T., Tian, D. S., Wang, B. J., Li, Z. L., et al. (2019). Methane emissions in grazing systems in grassland regions of China: A synthesis. Sci. Total Environ. 654, 662–670. doi: 10.1016/j.scitotenv.2018.11.102
Tsola, S. L., Zhu, Y. Z., Ghurnee, O., Economou, C. K., Trimmer, M., and Eyice, O. (2021). Diversity of dimethylsulfide-degrading methanogens and sulfate-reducing bacteria in anoxic sediments along the Medway Estuary, UK. Environ. Microbiol. 23, 4434–4449. doi: 10.1111/1462-2920.15637
Upton, R. N., Checinska Sielaff, A., Hofmockel, K. S., Xu, X., Polley, H. W., and Wilsey, B. J. (2020). Soil depth and grassland origin cooperatively shape microbial community co-occurrence and function. Ecosphere 11:e02973. doi: 10.1002/ecs2.2973
Vechi, N. T., Mellqvist, J., and Scheutz, C. (2022). Quantification of methane emissions from cattle farms, using the tracer gas dispersion method. Agric. Ecosyst. Environ. 330:107885. doi: 10.1016/j.agee.2022.107885
Wallenius, A. J., Martins, P. D., Slomp, C. P., and Jetten, M. S. M. (2021). Anthropogenic and Environmental Constraints on the Microbial Methane Cycle in Coastal Sediments. Front. Microbiol. 12:631621. doi: 10.3389/fmicb.2021.631621
Wang, J. (2020). Methanotrophs’ niche differentiation and its driving mechanism, Doctor Doctor. Hangzhou: Zhejiang University.
Wang, S., Abalori, T. A., Wang, W., Deng, X., Liu, W., Wang, J., et al. (2022). Response of soil microbial compositional and functional heterogeneity to grazing exclusion in alpine shrub and meadows in the Qinghai–Tibet Plateau. Front. Microbiol. 13:1038805. doi: 10.3389/fmicb.2022.1038805
Wang, Y. F., Chen, H., Zhu, Q., Peng, C. H., Wu, N., Yang, G., et al. (2014). Soil methane uptake by grasslands and forests in China. Soil Biol. Biochem. 74, 70–81. doi: 10.1016/j.soilbio.2014.02.023
Wang, J. L., Liu, Y. Z., Cao, W. X., Li, W., Wang, X. J., Zhang, D. G., et al. (2020). Effects of grazing exclusion on soil respiration components in an alpine meadow on the north-eastern Qinghai-Tibet Plateau. Catena 194:104750. doi: 10.1016/j.catena.2020.104750
Wei, D., Xu-Ri, T.-T., Wang, Y. S., and Wang, Y. H. (2015). Considerable methane uptake by alpine grasslands despite the cold climate: in situ measurements on the central Tibetan Plateau, 2008-2013. Glob. Chang. Biol. 21, 777–788. doi: 10.1111/gcb.12690
Wen, X., Yang, S. Z., and Liebner, S. (2016). Evaluation and update of cutoff values for methanotrophic pmoA gene sequences. Arch. Microbiol. 198, 629–636. doi: 10.1007/s00203-016-1222-8
Wood, D. E., and Salzberg, S. L. (2014). Kraken: ultrafast metagenomic sequence classification using exact alignments. Genome Biol. 15:R46. doi: 10.1186/gb-2014-15-3-r46
Xing, T., Liu, P., Ji, M., Deng, Y., Liu, K., Wang, W., et al. (2022). Sink or source: alternative roles of glacier foreland meadow soils in methane emission is regulated by glacier melting on the Tibetan Plateau. Front. Microbiol. 13:862242. doi: 10.3389/fmicb.2022.862242
Yang, Y. Y., Zhao, Q., Cui, Y. H., Wang, Y. L., Xie, S. G., and Liu, Y. (2016). Spatio-temporal variation of sediment methanotrophic microorganisms in a large eutrophic lake. Microb. Ecol. 71, 9–17. doi: 10.1007/s00248-015-0667-7
Yu, L. J., Huang, Y., Zhang, W., Li, T. T., and Sun, W. J. (2017). Methane uptake in global forest and grassland soils from 1981 to 2010. Sci. Total Environ. 607-608, 1163–1172. doi: 10.1016/j.scitotenv.2017.07.082
Yue, P., Li, K. H., Gong, Y. M., Hu, Y. K., Mohammat, A., Christie, P., et al. (2016). A five-year study of the impact of nitrogen addition on methane uptake in alpine grassland. Sci. Rep. 6:32064. doi: 10.1038/srep32064
Zhang, P. L., Fang, H. J., Cheng, S. L., Xu, M. J., Li, L. S., and Dang, X. S. (2013). The early effects of nitrogen addition on CH4 uptake in an alpine meadow soil on the Eastern Qinghai-Tibetan Plateau. Acta Ecol. Sin. 33, 4101–4110. doi: 10.5846/stxb201208281217
Keywords: grazing management, greenhouse gas, alpine meadow, methane flux, methane sink, methane-oxidizing bacteria
Citation: Wang S, Chen X, Li W, Gong W, Wang Z and Cao W (2023) Grazing exclusion alters soil methane flux and methanotrophic and methanogenic communities in alpine meadows on the Qinghai–Tibet Plateau. Front. Microbiol. 14:1293720. doi: 10.3389/fmicb.2023.1293720
Received: 13 September 2023; Accepted: 04 December 2023;
Published: 18 December 2023.
Edited by:
Marika Pellegrini, University of L’Aquila, ItalyReviewed by:
Cuijing Zhang, Institute for Advanced Study, Shenzhen University, ChinaCopyright © 2023 Wang, Chen, Li, Gong, Wang and Cao. This is an open-access article distributed under the terms of the Creative Commons Attribution License (CC BY). The use, distribution or reproduction in other forums is permitted, provided the original author(s) and the copyright owner(s) are credited and that the original publication in this journal is cited, in accordance with accepted academic practice. No use, distribution or reproduction is permitted which does not comply with these terms.
*Correspondence: Wenxia Cao, Y2Fvd3hAZ3NhdS5lZHUuY24=
†ORCID: Wenxia Cao, https://orcid.org/0000-0001-5255-0934
Disclaimer: All claims expressed in this article are solely those of the authors and do not necessarily represent those of their affiliated organizations, or those of the publisher, the editors and the reviewers. Any product that may be evaluated in this article or claim that may be made by its manufacturer is not guaranteed or endorsed by the publisher.
Research integrity at Frontiers
Learn more about the work of our research integrity team to safeguard the quality of each article we publish.