- 1Department of Chemical Engineering, Soongsil University, Seoul, Republic of Korea
- 2Department of Laboratory Medicine, Kangdong Sacred Heart Hospital, Hallym University College of Medicine, Seoul, Republic of Korea
- 3Department of Biological Engineering, College of Engineering, Konkuk University, Seoul, Republic of Korea
Antibiotic-induced gut microbiota disruption constitutes a major risk factor for Clostridioides difficile infection (CDI). Further, antibiotic therapy, which is the standard treatment option for CDI, exacerbates gut microbiota imbalance, thereby causing high recurrent CDI incidence. Consequently, probiotic-based CDI treatment has emerged as a long-term management and preventive option. However, the mechanisms underlying the therapeutic effects of probiotics for CDI remain uninvestigated, thereby creating a knowledge gap that needs to be addressed. To fill this gap, we used a multiomics approach to holistically investigate the mechanisms underlying the therapeutic effects of probiotics for CDI at a molecular level. We first screened Bifidobacterium longum owing to its inhibitory effect on C. difficile growth, then observed the physiological changes associated with the inhibition of C. difficile growth and toxin production via a multiomics approach. Regarding the mechanism underlying C. difficile growth inhibition, we detected a decrease in intracellular adenosine triphosphate (ATP) synthesis due to B. longum–produced lactate and a subsequent decrease in (deoxy)ribonucleoside triphosphate synthesis. Via the differential regulation of proteins involved in translation and protein quality control, we identified B. longum–induced proteinaceous stress. Finally, we found that B. longum suppressed the toxin production of C. difficile by replenishing proline consumed by it. Overall, the findings of the present study expand our understanding of the mechanisms by which probiotics inhibit C. difficile growth and contribute to the development of live biotherapeutic products based on molecular mechanisms for treating CDI.
1. Introduction
The human gut is a habitat for diverse and numerous microorganisms (∼1013 to 1014). However, an imbalance in the gut microbiota, also known as dysbiosis, can lead to infections caused by various opportunistic pathogens, which can further lead to various diseases as well as severely affect the immune system of the host (Pickard et al., 2017). Clostridioides difficile, a representative enteric pathobiont, is a gram-positive anaerobe that can cause disorders ranging in severity from mild diarrhea to acute colitis and death (Leffler and Lamont, 2015). C. difficile infection (CDI) constitutes a leading cause of nosocomial disease and is responsible for ∼30% of all antibiotic-associated diarrhea incidence, reportedly causing a total of 462,100 cases and > 13,000 deaths in the United States in 2017 alone (McFarland et al., 2016; Guh et al., 2020). In general, antibiotic administration constitutes the main risk factor for CDI as it causes dysbiosis and leads to the loss of colonization resistance against C. difficile (Deshpande et al., 2013; Schubert et al., 2015). However, as a standard treatment option for CDI, antibiotic therapy, including those of metronidazole, vancomycin, and fidaxomicin, is preferentially used (Kelly et al., 2021). Eventually, antibiotic therapy causes gut microbiota perturbation, resulting in a very high CDI recurrence rate (∼25%), and approximately 35∼65% of patients with recurrent CDI (rCDI) experience multiple rCDI (Crowther and Wilcox, 2015; Collins and Auchtung, 2017).
Therapeutic strategies involving live microorganism intervention can overcome the aforementioned problems with antibiotic therapy. Thus, recently, approaches to leverage probiotics, which are beneficial gut microorganisms, for preventing and treating CDI and rCDI have been proposed (Crow et al., 2015; Valdés-Varela et al., 2018; Pal et al., 2022; Zhang et al., 2022). Although several preclinical studies have reported the potential of probiotics for improving CDI outcomes, evidence regarding the preventive and therapeutic efficacies of probiotics for CDI was insufficient in several meta-analyses of clinical trials (Goldenberg et al., 2017; Heil et al., 2021; Kelly et al., 2021; Pal et al., 2022). Consequently, based on these studies, the American College of Gastroenterology recently recommended that probiotics should not be employed to prevent CDI and rCDI in patients receiving antibiotic therapy (Kelly et al., 2021). This recommendation was based on the inconsistent outcomes of several trials involving probiotics for CDI alongside the poor quality of the evidence regarding their efficacy. This problem stems from a lack of understanding and research regarding the mechanisms by which probiotics improve CDI symptoms, thereby leading to inadequate study designs involving inappropriate strain selection and efficacy assessment methods (Pal et al., 2022).
To date, several studies have reported various mechanisms by which traditional probiotics (Lactobacillus spp. and Bifidobacterium spp.) inhibit C. difficile growth and virulence. First, probiotics inhibit C. difficile growth by producing antimicrobial substances such as organic acids, hydrogen peroxide, and bacteriocin. Naaber et al. (2004) compared the antagonistic activity of 50 Lactobacillus spp. strains against 23 C. difficile strains and observed that lactobacilli with C. difficile growth-inhibitory activity produced more lactate and hydrogen peroxide. Furthermore, reportedly, Lactobacillus reuteri inhibits C. difficile growth by producing a bacteriocin known as reuterin (Spinler et al., 2017). Probiotics can also decrease C. difficile virulence by reducing the expression or degrading C. difficile toxins. Valdés-Varela et al. (2016) compared the protective effect of 20 Bifidobacterium spp. and Lactobacillus spp. strains against the cytotoxicity of C. difficile culture supernatants, confirming that Bifidobacterium longum and Bifidobacterium breve exhibit antitoxin effects and that these strains effectively reduce the amount of toxin in the supernatant. In addition, probiotics may prevent C. difficile colonization by inhibiting C. difficile adhesion to intestinal epithelial cells. Reportedly, L. acidophilus La-5 culture supernatants reduce C. difficile adhesion to the human intestinal epithelial cell lines, Caco-2 and HT-29, further alleviating the cytotoxicity of C. difficile supernatants (Najarian et al., 2019). Despite numerous efforts to elucidate the mechanism underlying C. difficile growth inhibition by probiotics, our understanding regarding this mechanism at the molecular level remains very limited. For example, the molecular-level interactions between probiotics and C. difficile and the resulting changes in metabolism associated with the C. difficile growth inhibition and virulence factor regulation remain unexplored. Multiomics study, a holistic qualitative and quantitative analysis of biomolecules in a biological system, can help fill the knowledge gaps regarding the mechanism of action of probiotics against CDI (Kim et al., 2020; Song et al., 2021; Lee et al., 2022; Park et al., 2022). Therefore, this study aimed to investigate the interaction and inhibition mechanisms at the molecular level between Bifidobacterium strains and C. difficile through a multiomics approach.
Herein, we selected Bifidobacterium strains that exhibited the highest growth inhibition on anaerobic coculture with C. difficile and investigated these inhibitory mechanisms via liquid chromatography–tandem mass spectrometry (LC-MS/MS) based multiomics analysis. First, we cocultured five Bifidobacterium strains with C. difficile and selected Bifidobacterium subsp. longum (B. longum), which showed the highest growth efficiency. Next, we assessed lactic acid and acetate production by B. longum and their contribution to its antimicrobial activity against C. difficile using spot-on-lawn assay and organic acid quantification. Finally, we analyzed changes in the quantitative proteomic and metabolomic profiles of C. difficile upon coculture to elucidate the growth inhibition mechanism of B. longum on C. difficile. Through this approach, we were able to observe global changes in the proteome and metabolome of C. difficile due to B. longum and confirm that essential regulated metabolic processes (including lactate reduction via the lactate dehydrogenase complex, proline metabolism, butyrate metabolism, translation, and nucleoside phosphorylation) were involved in C. difficile growth inhibition and reduced toxin production. We think that the results of this study can not only broaden our understanding regarding the action mechanism of probiotics against C. difficile but also contribute to the development of live biotherapeutic products based on molecular mechanisms for CDI treatment in future.
2. Materials and methods
2.1. Bacterial strains and culture conditions
Clostridioides difficile Korean Collection for Type Culture (KCTC) 5009 (i.e., ATCC 9689), Bifidobacterium longum subsp. longum KCTC 3128 (i.e., ATCC 15707), Bifidobacterium longum subsp. infantis KCTC 3249 (i.e., ATCC 15697), Bifidobacterium animalis subsp. animalis KCTC 3219 (i.e., ATCC 25527), Bifidobacterium animalis subsp. lactis KCTC 5854 (i.e., DSM 10140), and Bifidobacterium breve KCTC 3419 (i.e., ATCC 15701) were obtained from the KCTC. All the strains were cultured with reduced Reinforced Clostridium Medium (RCM) in an anaerobic chamber (90% N2, 5% CO2, and 5% H2; Coy Laboratory Products, MI, USA) at 37°C.
2.2. Cocultivation of Bifidobacterium spp. and C. difficile for probiotics screening
Clostridioides difficile and five strains of Bifidobacterium spp. were cultured alone until midlog phase. Then, the cultures were centrifuged at 13,500 rpm for 3 min, and the cell pellets were resuspended in fresh RCM broth at 1 × 108 colony forming units (CFU)/mL for Bifidobacterium spp. and 5 × 106 CFU/mL for C. difficile. 12-mm transwells (3401, Corning, NY, USA), round plastic wells with permeable membrane inserts, were used for the cocultivation of Bifidobacterium spp. and C. difficile. Bifidobacterium spp. (0.5 mL) was inoculated in the insert of the transwell, and 1 mL C. difficile, which is 1/10 of the inoculated CFU of Bifidobacterium spp., was inoculated in the bottom chamber. The bacterial cells were cocultured for 10 h. A C. difficile monoculture control (grown alone) had 0.5 mL fresh RCM in the insert. For the growth assay, the OD600 of the bottom chamber after 10 h coincubation was measured using an UV spectrophotometer (Multiskan Go, Thermo Fisher Scientific, MA, USA). The pH of the culture supernatant was measured using a pH meter (815600, Thermo Fisher Scientific, MA, USA).
2.3. Antimicrobial activity assay
To determine the antibacterial activity of B. longum KCTC 3128 against C. difficile, a spot-on-lawn assay was performed. C. difficile (5 × 106 CFU/mL) was spread on RCM agar plates using a sterile swab. Then, an inoculum was prepared using a 10-fold serial dilution of 5 × 109 CFU/mL B. longum. Each inoculum (2 μL) was spotted onto the C. difficile lawns and incubated in an anaerobic chamber for 48 h. “R” width of the inhibition zone was calculated using the formula where d Inhibit denotes the diameter of the inhibition zone around the d spot and d spot denotes the diameter of the spot formed by B. longum.
2.4. Intracellular and extracellular metabolite sample preparation for the metabolomic analysis
For metabolomics sample preparation, C. difficile and B. longum were cocultured in 75-mm transwells (3419, Corning, NY, USA) for 10 h. The insert of the transwell was inoculated with B. longum at 6.5 × 108 CFU in 9 mL RCM, and the bottom chamber was inoculated with C. difficile at 6.5 × 107 CFU in 13 mL RCM. Following coculture for 10 h, equal cells of C. difficile in the bottom chamber were harvested based on the OD600 and centrifuged (4,000 rpm, 4°C, 15 min) to separate the supernatant and cell pellet. The culture supernatant was stored at −80°C for extracellular metabolite extraction and organic acid. Metabolite sample preparation method was performed based on previous study (Yuan et al., 2012). To reduce changes in the metabolome during metabolite sample preparation, cold solvents were used, and samples were manipulated on ice for all extraction procedures. The cell pellet was washed twice with ice-cold 0.9% NaCl solution to remove any remaining media on the cells. For the intracellular metabolite extraction, 1 mL −80°C 80% methanol spiked with L-[13C9, 15N]phenylalanine (608017, Sigma-Aldrich, MO, USA) at a concentration of 0.5 μM as an internal standard incubated at −80°C for 4 h. The supernatant was subsequently collected via centrifugation (13,800 rpm, 4°C, 3 min). Then, the second and third extractions were performed in the same manner for 30 min to pool the extracted metabolites. The extracted metabolite samples were dried using a centrifugal vacuum concentrator (Modulspin 40, Hanil Science Industrial, Korea) and stored at −80°C until analysis.
For hydrophilic extracellular metabolite extraction, 400 μL methanol with 3.3 μM L-[13C9, 15N]phenylalanine and 800 μL chloroform were added to 400 μL culture supernatant. The mixed sample was vortexed for 3 min and then centrifuged (13,800 rpm, 4°C, 3 min). Then, 650 μL of the upper layer mixture was collected and dried using a centrifugal vacuum concentrator. The dried samples were stored at −80°C until analysis.
2.5. LC-MS/MS based targeted metabolomics
The dried intracellular and extracellular metabolite samples were dissolved in 40 and 100 μL high-performance liquid chromatography grade water, respectively, and subjected to LC–MS/MS analysis. 1260 Infinity Binary LC (Agilent, CA, USA) combined with 6420 Triple Quadrupole MS (Agilent, CA, USA) was used for the LC–MS/MS analysis. The prepared metabolite sample (10 μL) was injected into the XBridge® Amide column (186004868, 4.6 × 250 mm, particle size 3.5 μm, Waters, MA, USA). Solvent A comprised water/acetonitrile (95:5) with 20 mM ammonium acetate and 20 mM ammonium hydroxide, while solvent B comprised 100% acetonitrile. The LC gradient was: 0 min, 85% B; 5 min, 42% B; 16 min, 0% B; 24 min 0% B; 25 min, 85% B; 32 min, 85% B. The flow rate was 0.4 mL/min. The capillary temperature was 300°C. The electrospray ionization voltage was 4 kV. Agilent MassHunter Qualitative Analysis (version B.07.00) software was used to extract the MS peak areas. The peak area was normalized using the peak area of the internal standard. Statistical analysis of the normalized peak area data was performed using MetaboAnalyst 5.0, and significance was determined using the false discovery rate (FDR) adjusted p-value (Pang et al., 2021). The metabolites with fold-change of ≥ 1.5 and a p-value of ≤ 0.05 were defined as quantitatively significant.
2.6. Protein sample preparation for the proteomic analysis
Cell pellets were obtained in the same way as described above. The obtained cell pellets were resuspended in 1 mL of RIPA lysis and extraction buffer (Thermo Fisher Scientific, MA, USA) containing 0.1% protease inhibitor cocktail (P1860, Sigma-Aldrich, MO, USA) and sonicated using a probe sonicator (Sonics & Materials, CT, USA) for cell lysis. The samples were centrifuged (13,800 rpm, 4°C, 5 min), following which the supernatants were collected. The protein samples were subjected to the filter-aided sample preparation method (Wiśniewski et al., 2009). The protein samples were mixed with 1M dithiothreitol (Sigma-Aldrich, MO, USA) to a final concentration of 50 mM and incubated at 95°C for 5 min. Then, 100 μg protein extract and 200 μL UA buffer (aqueous buffer of 8M urea in 0.1 M Tris/HCl pH 8.5) were mixed and loaded on Microcon-30kDa (MRCF0R030, Merck Millipore, Darmstadt, Germany). The filter unit was centrifuged at 10,000 × g for 30 min. Then, 200 μL UA buffer was added to the filter unit and centrifuged at 10,000 × g for 15 min, and the process was repeated twice. Then, 100 μL 0.05 M iodoacetamide (Sigma-Aldrich, MO, USA) in UA buffer was added to the filter unit and incubated in the dark for 20 min. The filter unit was centrifuged at 10,000 × g for 10 min, following which 100 μL UA buffer was loaded onto the filter and centrifuged at 10,000 × g for 15 min. After repeating this process twice, 100 μL 0.05 M Tris/HCl buffer (pH 8.5) was added to the filter unit and centrifuged at 10,000 × g for 10 min. This process was repeated twice. The protein sample was subsequently digested by adding 2 μg trypsin and incubating at 37°C for 20 h. To obtain the peptide sample, 250 μL 0.05 M Tris/HCl buffer (pH 8.5) was loaded into the filter unit and centrifuged at 10,000 × g for 10 min. Then, the collected peptide samples were desalted using the Pierce Peptide Desalting Spin Columns (89851, Thermo Fisher, MO, USA) according to the manufacturer’s instructions. Finally, the protein samples were dried using a vacuum concentrator and stored at −80°C until analysis.
2.7. LC-MS/MS based bottom-up proteomic analysis
Proteomic analysis was performed as described previously (Kwon et al., 2022). The dried peptide samples were reconstituted in solvent A (water/acetonitrile [98:2 v/v] and 0.1% formic acid). For protein analysis, the peptide samples were analyzed using the LC–MS system, which was a combination of Easy-nLC 3000 (Thermo Fisher Scientific, Waltham, MA, USA) coupled with the EASY-spray ion source (Thermo Fisher Scientific, Waltham, MA, USA) on Q-Exactive mass spectrometer (Thermo Fisher Scientific, Waltham, MA, USA). The peptides were separated on the two-column setup using a Acclaim PepMap 100 trap column (100 mm × 2 cm, nanoViper C18, 5 mm, 100 Å, Thermo Scientific, MA, USA) and Acclaim PepMap 100 capillary column (75 mm × 15 cm, nanoViper C18, 3 mm, 100 Å, Thermo Scientific, MA, USA). The peptide sample (2 μg) was first trapped in a trap column and washed with 98% solvent A at a flow rate of 4 μL/min for 6 min. After washing, the sample was separated at a flow rate of 300 nL/min using the capillary column. The LC gradient was run at 2–40% solvent B (100% ACN and 0.1% Formic acid) over 30 min, then from 40 to 95% over 5 min, followed by 95% solvent B (100% ACN and 0.1% Formic acid) for 10 min, and finally 2% solvent B for 20 min. The ion spray voltage was 2,100 eV. Mass data were acquired automatically using Proteome Discoverer 2.5 (Thermo Scientific, USA). Orbitrap analyzer scanned the precursor ions within a mass range of m/z 350–1,800 and a resolution of 70,000 at m/z 200. For collision-induced dissociation, mass spectra were acquired in a data-dependent manner via a top 15 method using Q-Exactive. The normalized collision energy (NCE) was 32.
Protein identification and quantification were performed by MaxQuant (version 1.6.17.0) (Cox and Mann, 2008). MS and MS/MS data spectra were queried against the C. difficile ATCC 9689 UniProt database (2021.10 released) using the Andromeda search engine. Cysteine carbamidomethylation was set as a fixed modification. Methionine oxidation and N-terminal acetylation were set as variable modifications. Trypsin was used for cleavage, and up to two missed cleavages were allowed. The “match between runs” option was enabled. Proteins and peptides were filtered if they had an FDR of < 1% and quantified via label-free quantification (MaxLFQ) (Cox et al., 2014). The statistical analysis of the MaxLFQ data was performed using Perseus software (version 2.0.3.0) (Tyanova et al., 2016), the significance was determined using the adjusted p-value. Proteins with a fold-change of ≥ 1.5 and a p-value ≤ 0.05 were defined as quantitatively significant.
2.8. Organic acid quantification by LC-MS/MS
LC–MS/MS-based organic acid analysis was performed as described previously with some modifications (Kim et al., 2020; Song et al., 2020). The culture supernatant sample was filtered using a polyvinylidene fluoride membrane syringe-driven 0.45-μm pore size filter (SLHVX13NL, Millex-DV, Millipore, MA, USA) to remove cell debris. Next, the filtered sample was transferred to a microtube and diluted 10-fold with water. Then, 50 μl 50% acetonitrile, 40 μl 100 mM N-ethyl-N′-[3-(dimethylamino)propyl]carbodiimide (EDC, 1769, Sigma-Aldrich, MO, USA), 40 μL 100 mM Girard’s reagent T (G900, Sigma-Aldrich, MO, USA), and 10 μL 1 mM sodium [2H7]butyrate (D5372, CDN ISOTOPES, QC, Canada) were added in 20 μl diluted sample and mixed. The mixture was incubated at 40°C for 1 h, and the samples were diluted 20-fold with 50% acetonitrile. The prepared samples were stored at −20°C until further analysis.
For LC–MS/MS analysis, Acquity UPLC H-Class (Waters, MA, USA) combined with an LTQ XL™ linear ion trap mass spectrometer (Thermo Fisher Scientific, MA, USA) was used. The sample (5 μL) was injected into a Zorbax HILIC plus column (4.6 mm, 100 mm, and 3.5 mm; Agilent, CA, USA). Solvent A comprised water, 20 mM ammonium acetate, and 20 mM acetic acid, while solvent B comprised 100% acetonitrile. The LC gradient was 0 min, 70% B; 1 min, 70% B; 10 min, 30% B; 15 min, 30% B; 15.1 min, 70% B; and 20 min, 70% B. The flow rate was 0.3 mL/min. The NCE was 15–30 eV. For the analysis of organic acids, the previously reported MS/MS detection parameters of 5 GT-labeled short-chain fatty acid and lactate detection parameters (m/z of precursor ion: 204.1; m/z of product ion: 100.1; NCE: 30) were used.
2.9. Toxin A quantification using enzyme-linked immunosorbent assay
Toxin A was quantified in culture media using an ELISA kit (TGC-E002-1, tgcBIOMICS, Bingen, Germany). The culture supernatant was obtained via centrifugation (13,000 rpm, 3 min, 4°C) of the bottom chamber culture media following coculturing with B. longum for 48 h in the transwell. The supernatant was concentrated eight-fold via filtration (13,000 rpm, 3 min, 4°C) using a 50 kDa molecular weight cutoff membrane filter (UFC505096, Millipore, MA, USA). Toxin quantification was conducted using the ELISA kit according to the manufacturer’s instructions.
2.10. Statistical analysis
Pearson’s correlation coefficient was calculated using IBM SPSS statistics version 27 software (IBM, NY, USA). One-way analysis of variance and post-hoc was conducted using GraphPad Prism version 7 software. The heatmap visualization and PCA of omics datasets were conducted using R statistical programming. Volcano plot was created using VolcaNoseR webtool (Goedhart and Luijsterburg, 2020). For computation of the protein–protein association network and functional enrichment analysis, the STRING database version 11.5 was used (Szklarczyk et al., 2021).
3. Results and discussion
3.1. Screening of probiotics with growth-inhibitory effects against C. difficile
First, to identify the Bifidobacterium strains with the highest inhibitory activity against C. difficile growth, we cocultured five Bifidobacterium strains (B. longum subsp. longum, B. longum subsp. infantis, B. animalis subsp. animalis, B. animalis subsp. lactis, and B. breve) with C. difficile in transwells for 10 h. Then, we compared the growth of C. difficile with the different strains by measuring optical density at 600 nm (OD600) (Figure 1A). Compared with the C. difficile monoculture (C. difficile alone), all Bifidobacterium spp. caused growth inhibition; however, there were significant differences in the extent of growth inhibition among the strains (29.9% for B. breve to 61.6% for B. longum subsp. longum). Interestingly, two bacteria of the same species but different subspecies, B. longum subsp. longum and B. longum subsp. infantis, demonstrated a significant difference in their inhibitory activity (61.6 vs. 40.6%, respectively). In addition, the pH of the culture supernatant decreased by approximately 0.46–0.84 following cocultivation compared with that of the C. difficile alone group (Table 1). Several previous studies have reported that organic acids produced by probiotics contribute to C. difficile growth inhibition (Naaber et al., 2004; Kolling et al., 2012). This suggests that differences in the production of specific organic acids among Bifidobacterium strains may be responsible for the differential inhibitory activity of Bifidobacterium spp. against C. difficile; however, the underlying mechanisms remain unclear.
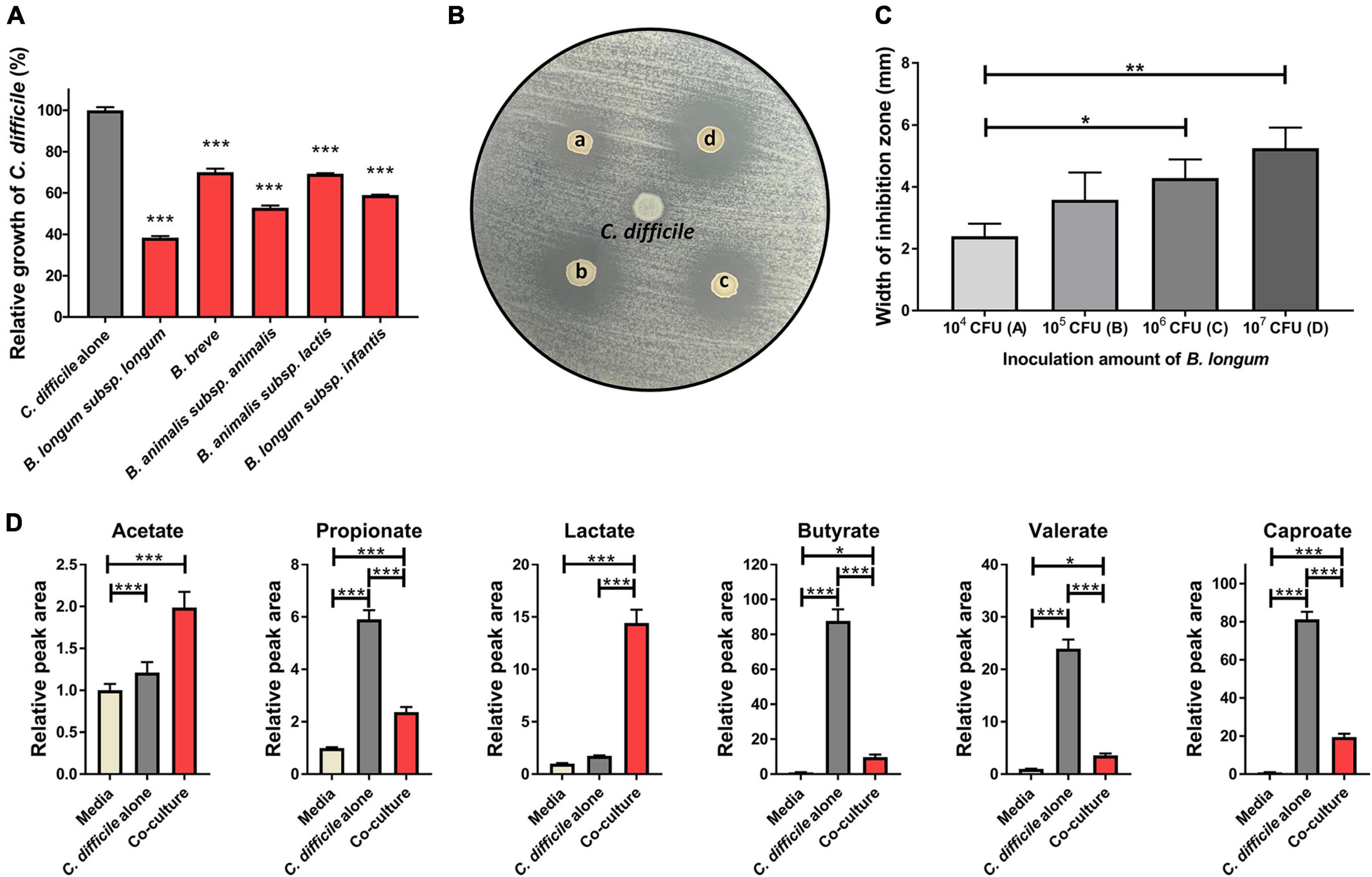
Figure 1. (A) Growth inhibition of C. difficile due to Bifidobacterium strains. Growth inhibition test was performed in triplicate, and the significance was compared with the C. difficile alone group. (B) Antimicrobial activity of B. longum against C. difficile was assessed using the spot-on-lawn method. Representative picture showing inoculum density–dependent antimicrobial activity of B. longum against C. difficile. C. difficile was used as a negative control (center spot of agar plate). Circles indicate inhibition zone. (a–d) Indicate the inoculated CFU of B. longum (104, 105, 106, and 107 CFU, respectively). (C) Width of the inhibition zone (mm) around the B. longum spot on the agar plate depends on the inoculated density of B. longum. These experiments were performed in triplicate. (D) Quantitative comparison of organic acids in culture media (n = 4). The significance was calculated via one-way analysis of variance and post-hoc (Tukey’s Honestly Significant Difference test). Error bar indicates the standard deviation. The symbols (*), (**), and (***) indicate p-value < 0.05, < 0.01, and < 0.001, respectively.
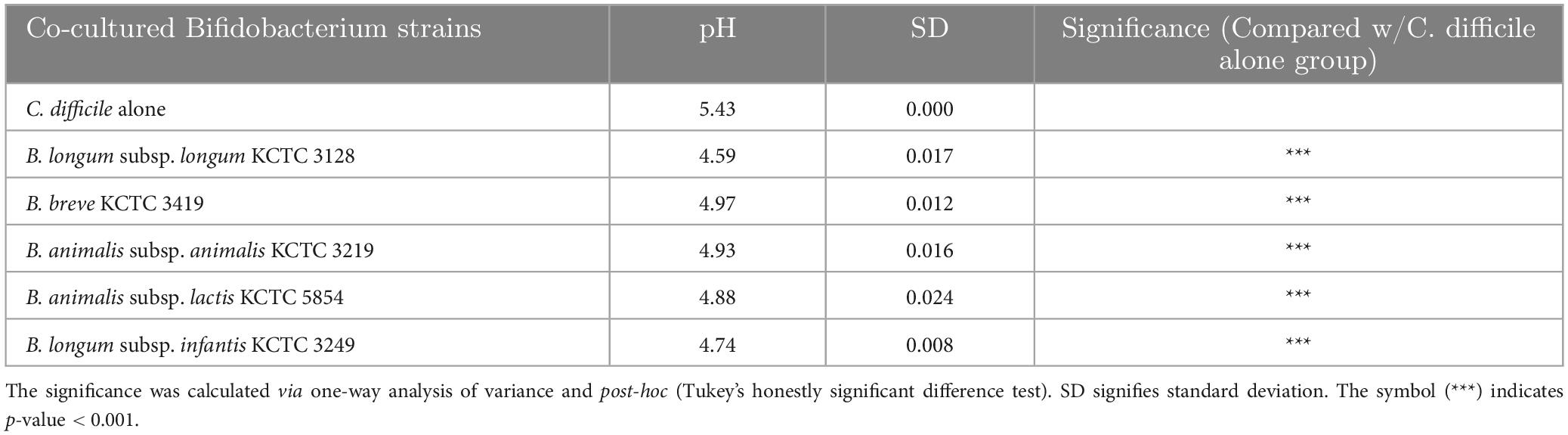
Table 1. pH of the supernatant following coculture of C. difficile with Bifidobacterium strains (n = 3).
If C. difficile growth inhibition is related to the production of specific organic acid, then B. longum subsp. longum, the strain with the highest inhibitory activity in the coculture experiment, should exhibit antibacterial activity against C. difficile. To confirm this hypothesis, a spot-on-lawn assay was performed to determine the antibacterial potential of B. longum against C. difficile. We found that B. longum formed an inhibition zone, whose width was dependent on the inoculum density of B. longum (Figures 1B, C). Next, the organic acids (acetate, propionate, valerate, caproate, butyrate, and lactate) that had accumulated in the cultures following the coculture of B. longum and C. difficile were quantitatively analyzed using LC–MS/MS to identify the organic acids predominantly produced by B. longum. Among the six organic acids, acetate and lactate accumulated significantly more in cocultured with B. longum than in the C. difficile alone group (Figure 1D). Specifically, lactate and acetate increased by 8.3- and 1.6-fold over the C. difficile alone group, respectively (Figure 1D), indicating that lactate was predominantly produced among the organic acids. This result was consistent with those of previous studies involving the same strain of B. longum (Yun et al., 2017). Yun et al. (2017) observed an inhibitory effect of B. longum on C. difficile when the two were cocultured in vitro, and this inhibitory effect was dependent on the inoculum density of B. longum and the low pH of the culture. The decrease in pH was speculated to be caused by the production of organic acids, including lactic acid, by B. longum (Yun et al., 2017). Furthermore, B. longum increased the survival rate of C. difficile–infected mice and reduced histological damage in the gut. Therefore, lactate was predicted to play a major role in the antibacterial potential of B. longum against C. difficile.
Other studies have reported on the same species as the B. longum strains selected in this study, which have demonstrated growth inhibition of C. difficile or alleviation of CDI symptoms. For example, B. longum was confirmed to inhibit C. difficile growth and reduce its cytotoxicity toward intestinal epithelial cells (HT-29) in vitro (Valdés-Varela et al., 2016). Furthermore, B. longum reportedly exhibits a growth-inhibitory effect on C. difficile in vitro in addition to improving the survival rate of C. difficile–infected mice and alleviating intestinal inflammation in a mouse model (Wei et al., 2018). However, despite these studies, the molecular mechanisms underlying the inhibition of C. difficile growth and virulence by probiotics remain unclear. Therefore, this study aimed to analyze the physiological changes in C. difficile caused by B. longum at the molecular level using a multiomics approach to understand these molecular mechanisms.
3.2. Proteomic and metabolomic analysis of C. difficile cocultured with B. longum
For a comprehensive analysis of the physiological changes in C. difficile upon coculture with B. longum, we conducted LC–MS/MS-based quantitative proteomics and metabolomics analyses (Supplementary Informations 1–3). Initially, a total of 1,055 proteins were identified following the intracellular proteome analysis, among which the expression levels of 636 proteins remained almost unchanged, those of 234 proteins were downregulated, and those of 185 proteins were upregulated based on the criteria of differentially expressed proteins (DEP, fold-change > 1.5 and p-value < 0.05) (Figure 2A). Principal component analysis (PCA) of the proteomics dataset revealed distinct quantitative differences in the proteome of C. difficile depending on whether it was cocultured with or without B. longum (Figure 2B).
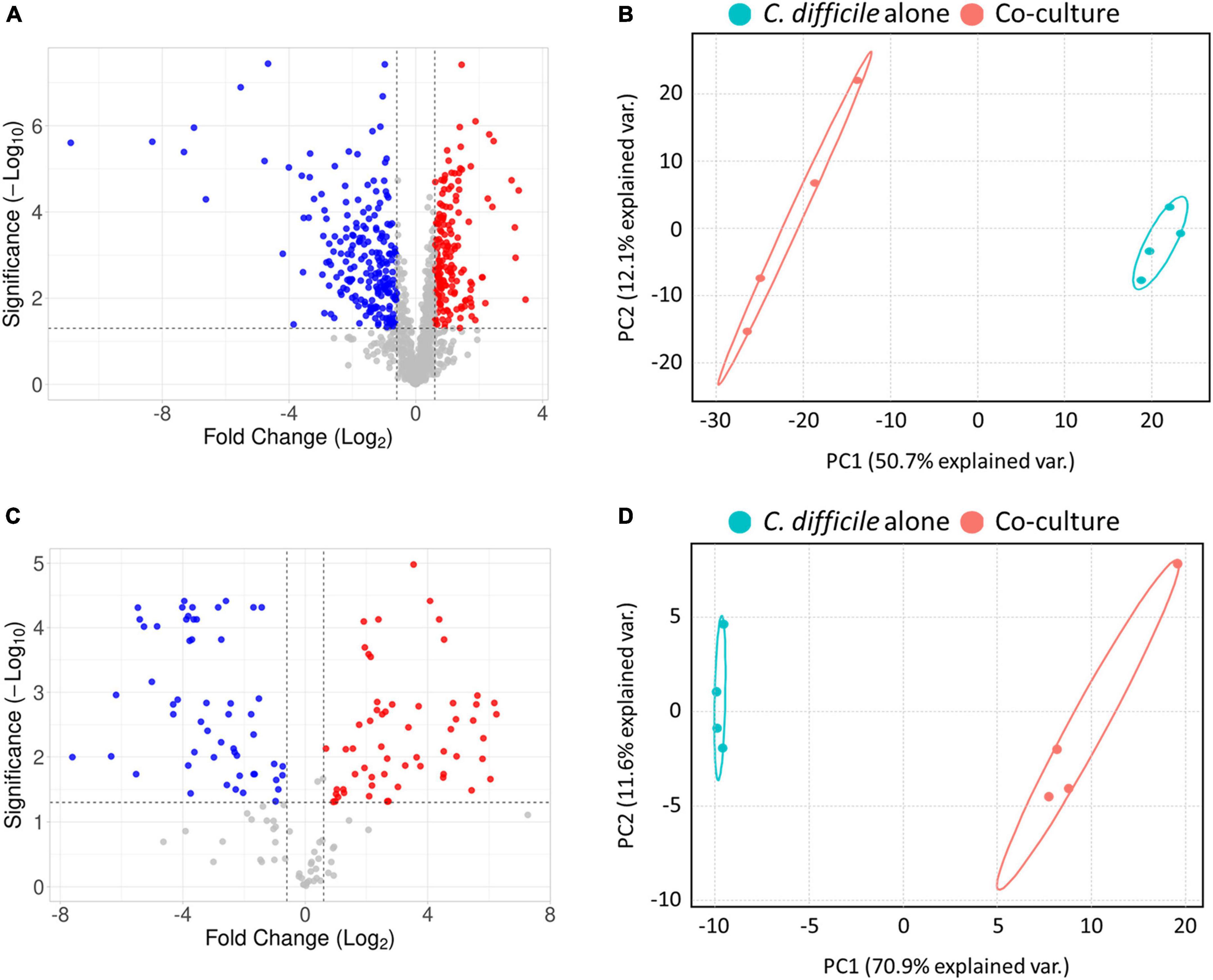
Figure 2. (A) Volcano plot of proteomics analysis. (B) Principal component analysis (PCA) plot of proteomics analysis. (C) Volcano plot of metabolomics analysis. (D) PCA plot of metabolomics analysis. In the volcano plot in panel (A), the red and blue colors indicate significantly upregulated and downregulated proteins, and in panel C the red and blue colors indicate metabolites that are at higher and lower levels, respectively, C. difficile cocultured with B. longum compared to C. difficile cultured alone. In the PCA plot, each experimental group was separately clustered. These results indicate that coculture with B. longum caused significant proteomic and metabolomic changes in C. difficile.
Subsequently, we monitored quantitative changes in intracellular metabolites using an internal multiple reaction monitoring library including 184 metabolites involved in various primary metabolisms. Consequently, we detected 160 intracellular metabolites, with 48 unchanged, 58 upregulated and 54 downregulated metabolites in the B. longum coculture group compared with the C. difficile alone group (Figure 2C). Moreover, the PCA of the metabolomics dataset revealed that B. longum coculture induced quantitative differences in C. difficile metabolites, which is consistent with the results of the proteomics data analysis (Figure 2D). Overall, the proteomics and metabolomics analyses demonstrated that B. longum coculture induces physiological changes at the molecular level, which are implicated in C. difficile growth inhibition.
3.3. B. longum–produced lactate influences C. difficile energy metabolism
In our proteomics data, proteins belonging to the lactate dehydrogenase (LDH) complex of C. difficile (LDH and electron-transferring flavoproteins) were significantly upregulated upon B. longum coculture (Figure 3B). Consistent with this finding, metabolomics data revealed that the intracellular lactate of C. difficile increased upon B. longum coculture (Figure 3C). Hofmann et al. (2021) reported that L-lactate addition to the culture media induced the expression of an LDH complex–containing operon via transcriptome analysis. Therefore, the upregulation of the LDH complex in C. difficile cocultured with B. longum is attributable to the lactate produced by B. longum.
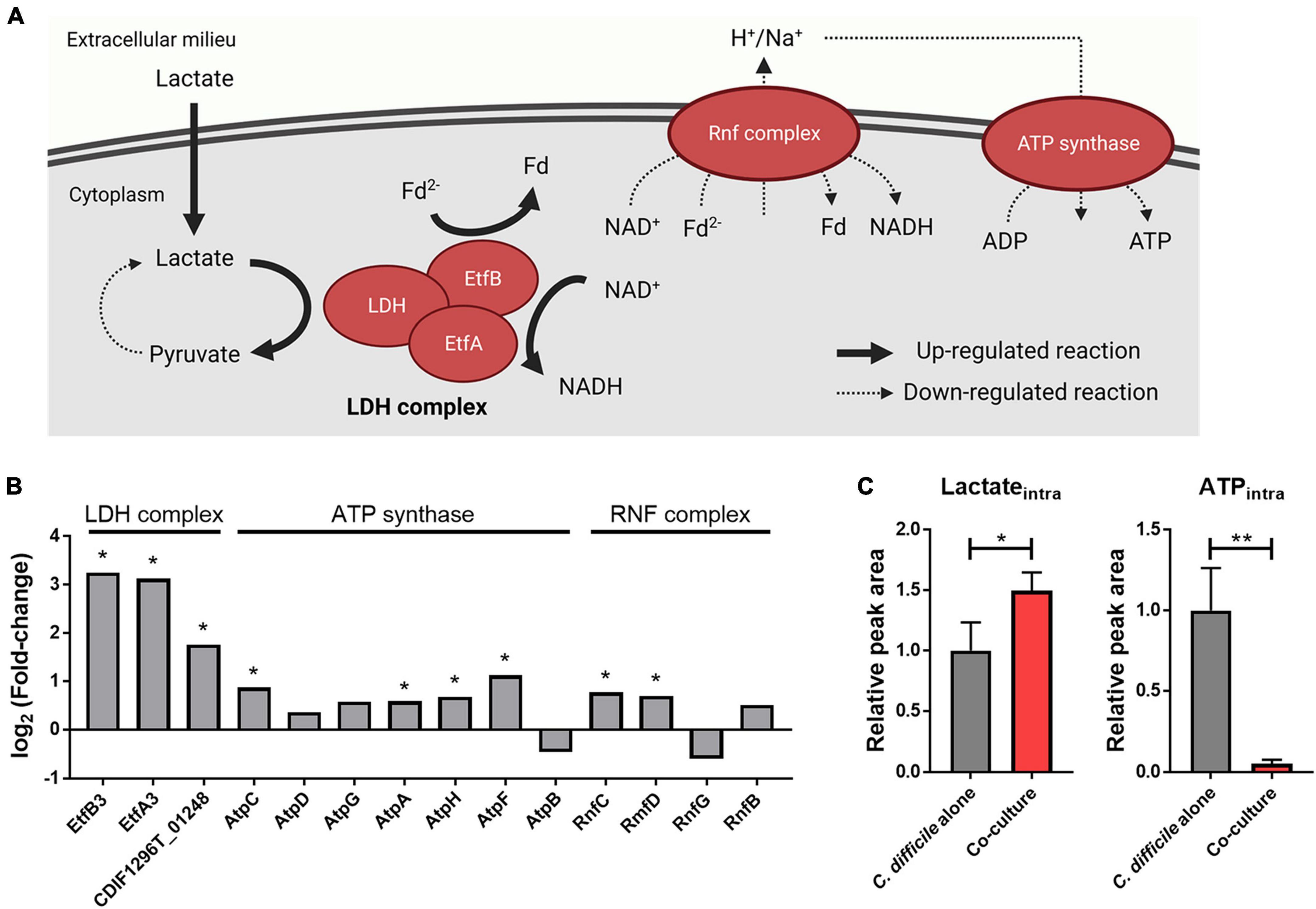
Figure 3. (A) Illustration of the effects of lactate reduction on ATP synthesis. This illustration was created using BioRender.com. (B) Alterations in intracellular protein levels of the LDH complex, ATP synthase, and Rnf complex. The symbol (*) indicates differentially expressed proteins (DEPs). (C) Intracellular protein levels of lactate and ATP. Error bar indicates the standard deviation. The symbols (*) and (**) indicate adjusted p-value < 0.05 and < 0.01, respectively.
The LDH complex reduces NAD+ and metabolizes lactate in the presence of reduced ferredoxin (Fd2–) (Weghoff et al., 2015). In addition, previous studies have confirmed that L-lactate added to the medium is metabolized by a LDH complex in C. difficile (Hofmann et al., 2021). Therefore, we hypothesized that as lactate produced by B. longum increases lactate metabolism in C. difficile, changes associated with Fd2– will occur as well in the cellular metabolism of C. difficile. Owing to the absence of the classical electron transport chain in C. difficile, its alternative, the membrane-spanning Rnf complex, utilizes Fd2– and NADH to generate an ion (i.e., sodium or proton) gradient, which is subsequently utilized by the adenosine triphosphate (ATP) synthase complex for ATP biosynthesis (Neumann-Schaal et al., 2019). In our proteomics and metabolomics analysis, we observed that the Rnf complex and ATP synthase–related proteins either quantitatively remained unchanged or slightly increased in C. difficile cocultured with B. longum in contrast to the significant decrease in intracellular ATP levels (Figures 3B, C). Thus, the elevated intracellular lactate levels promoted the consumption of Fd2–, the Rnf complex substrate during lactate metabolization, by inducing the expression of the LDH complex. Decline in intracellular ATP levels can be attributed to a possible decrease in the intracellular levels of Fd2–, which can limit ion gradient generation by the Rnf complex, ultimately leading to reduced ATP production within the cell (Figure 3A). Thus, (1) the large amount of lactate produced by B. longum enhances the expression of the LDH complex in C. difficile, and (2) the increased lactate oxidation reaction depletes Fd2–, thereby inhibiting ion gradient generation by the Rnf complex. (3) This ultimately inhibits ATP biosynthesis via the ATP synthase complex, and the resulting lower intracellular ATP level may contribute to C. difficile growth inhibition.
3.4. B. longum–produced proline reduces C. difficile toxin production
The Stickland reaction in the energy metabolism of C. difficile produces ATP and NAD+ through the oxidation and reduction of amino acids (Neumann-Schaal et al., 2019). C. difficile utilizes amino acids, such as proline and glycine, as electron acceptors in the reductive Stickland reaction (Bouillaut et al., 2013). In particular, C. difficile converts L-proline to D-proline via proline racemase for proline reduction and produces NAD+ and 5-aminovalerate using this metabolite (Bouillaut et al., 2013). Initially, our proteomics analysis revealed that proteins involved in proline metabolism, such as proline racemase and D-proline reductase, were significantly upregulated in C. difficile upon coculture with B. longum (Figure 4B). Intracellular metabolite analysis revealed that the levels of intracellular proline and 5-aminovalerate, the end products of proline metabolism, were significantly elevated in C. difficile when co cultured with B. longum (Figure 4C). These results demonstrate that the upregulation of proline metabolism in C. difficile during coculture with B. longum promotes the reductive reaction of proline. However, when cocultured with B. longum, the area value of extracellular 5-aminovalerate was 0.54 times lower than that of the C. difficile alone group (Figure 4C). In the C. difficile alone group, the substrate proline was almost completely depleted (0.002-fold), whereas in the coculture with B. longum, the proline levels remained unchanged (Figure 4C). Therefore, the low accumulation of 5-aminovalerate in the culture media despite increased intracellular 5-aminovalerate levels due to elevated proline metabolism may be attributable to a total decreased proline reduction caused by C. difficile growth inhibition during coculture with B. longum. Notably, despite the considerable production of 5-aminovalerate in the coculture of C. difficile and B. longum, the level of extracellular proline remained similar to that in the fresh media. Thus, we hypothesized that C. difficile consumed the proline produced by B. longum while B. longum replenished the proline in the culture medium. To confirm this, we performed a quantitative analysis of proline in the culture supernatant of a B. longum monoculture and found that the area value of proline was 1.27 times higher than that of the fresh media (Figure 4E). Because proline can induce proline metabolism–related protein expression (Bouillaut et al., 2013), these results indicate that proline produced by B. longum is responsible for the upregulation of proline metabolism in C. difficile.
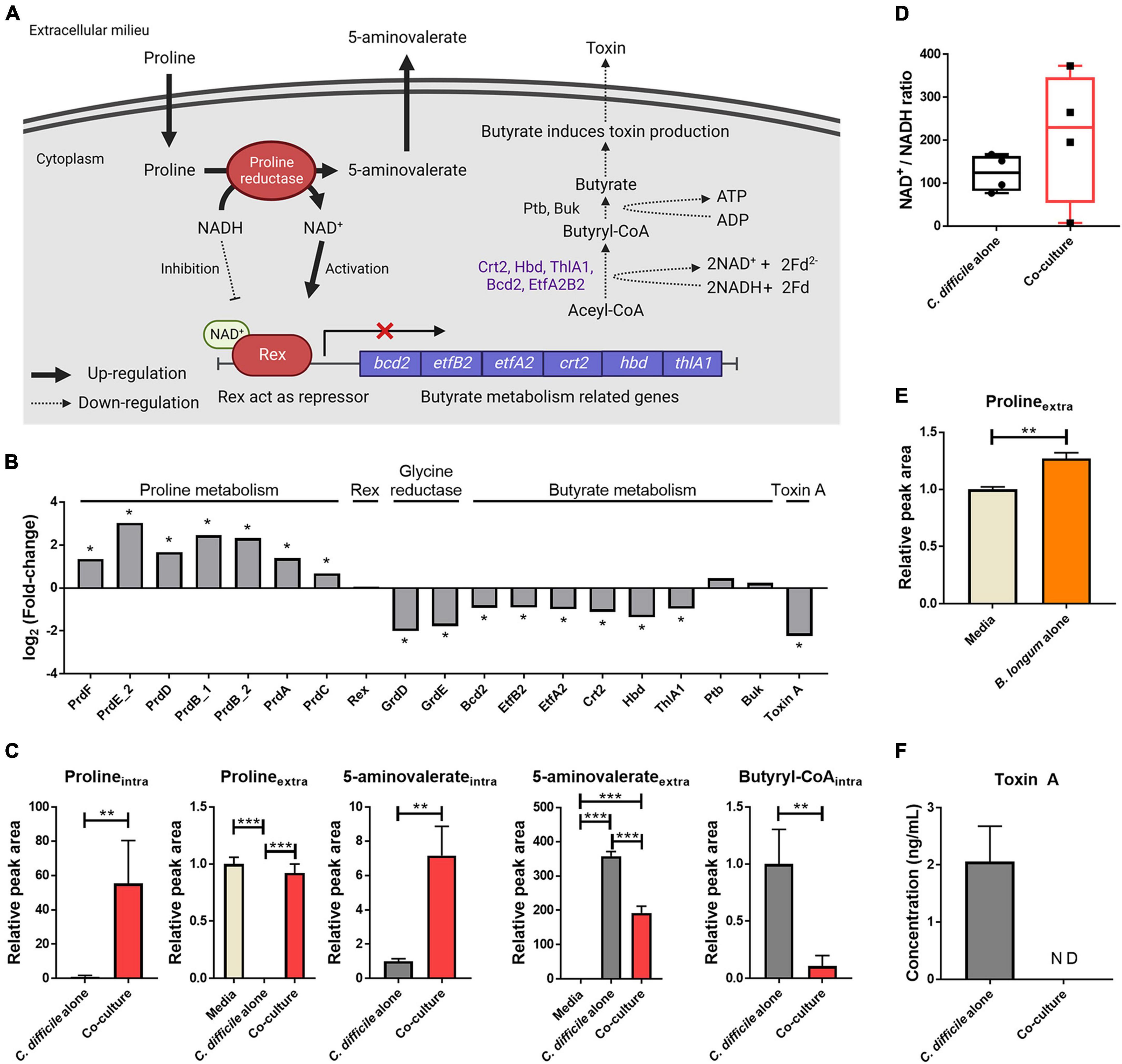
Figure 4. (A) Illustration of the effects of proline metabolism upregulation on butyrate metabolism and toxin production. This illustration was created using BioRender.com. (B) Alterations in the intracellular protein levels of proline-dependent regulation. The symbol (*) indicates differentially expressed proteins. (C) Abundance levels of metabolites belonging to proline reduction and butyrate metabolism. (D) Increased levels of the intracellular NAD+/NADH ratio in C. difficile cocultured with B. longum. To measure the NAD+/NADH ratio, absolute quantification of NAD+ and NADH was performed, and then the NAD+ concentration was divided by the NADH concentration of each sample. (E) Increased extracellular proline level in B. longum monocultured media (n = 3). (F) Toxin A concentration in culture supernatant after 48 h of cultivation (n = 3). Error bar indicates the standard deviation. The symbols (*), (**) and (***) indicate p-value < 0.05, < 0.01, and < 0.001, respectively.
In a previous study, proline supplementation to the C. difficile media downregulated toxin A expression (Bouillaut et al., 2013). Furthermore, Bouillaut et al. (2019) reported that Rex, an NAD+/NADH-responsive regulator, directly binds to DNA and represses the genes involved in alternative NAD+-generating pathways (i.e., glycine reductive reaction and butyrate metabolism). They demonstrated that NAD+ and NADH act as activators and repressors of the DNA binding activity of Rex, respectively, and that NAD+ regeneration by D-proline reductase represses these alternative pathways (Bouillaut et al., 2019). Consistent with the results of these previous studies, the changes in C. difficile physiology due to D-proline reductase upregulation were observed in this study (Figure 4). First, an intracellular metabolomic analysis revealed an increased NAD+/NADH ratio in C. difficile cocultured with B. longum (Figure 4D). These results indicate that in C. difficile cocultured with B. longum, proline metabolism upregulation causes NAD+-regeneration, in turn activating Rex. Notably, while the NAD+/NADH ratio increased, both NAD+ and NADH were significantly reduced in C. difficile cocultured with B. longum by 14.8- and 29.1-fold, respectively. The NAD+ and NADH redox pair is involved as a cofactor in various redox metabolisms, and therefore, the reduction of this metabolite pair in C. difficile cocultured with B. longum may adversely affect C. difficile metabolism and contribute to its growth inhibition. In other words, the total amount of NAD+/NADH pair is reduced in C. difficile due to the presence of B. longum; however, the relative NAD+/NADH ratio is increased through the upregulation of NAD+ regeneration via proline reduction, which activates Rex and inhibits butyrate metabolism.
Second, proteins involved in glycine reductase and butyrate metabolism were downregulated (Figure 4B). Because Rex acts as a repressor that downregulates the expression of these proteins, these results indicate that Rex-dependent regulation occurs due to an increase in the NAD+/NADH ratio. Among these downregulated pathways, butyrate metabolism generates energy in C. difficile (Figure 4A). As butyrate metabolism generates Fd2– and produces ATP through substrate-level phosphorylation, the downregulation of butyrate metabolism contributes to intracellular ATP level reduction due to decreased ATP production via the Rnf complex and ATP synthase and via substrate-level phosphorylation. The downregulation of butyrate metabolism was also confirmed by the aforementioned organic acid quantitative analysis (Figure 1C). The production of butyrate decreased to 9.5-fold in the coculture compared with that in the C. difficile alone group and decreased to 3.4-fold when the area value was normalized by OD to account for the growth inhibition of C. difficile. This result reconfirms the downregulation of butyrate metabolism in C. difficile.
Finally, proteomics analysis revealed the downregulation of C. difficile toxin A (Figure 4B). Because butyrate acts as a stimulator for toxin production in C. difficile, the observed reduction in butyrate production due to the downregulation of its metabolism may contribute to the reduced expression of tcdA in C. difficile (Karlsson et al., 2000). In addition, we aimed to verify whether the accumulation of toxin A in the culture media was reduced in presence of B. longum. Following coculture with B. longum, toxin A was quantified via enzyme-linked immunosorbent assay (ELISA), and the results revealed that ∼2.1 ng/mL toxin A accumulated in the C. difficile alone group, whereas no toxin A was detected in the coculture with B. longum (Figure 4F). Thus, proline produced by B. longum induces inhibition of toxin production in C. difficile, ultimately contributing to the growth and virulence inhibition of C. difficile.
3.5. B. longum–induced proteinaceous stress in C. difficile
As a result of the functional enrichment analysis of DEPs using STRING, the terms “ribosomal protein” and “Regulation of translation” were enriched among the gene ontology (GO) (Figure 5A). In C. difficile cocultured with B. longum, the ribosomal proteins, ribosome maturation factor (RimP), and transcription antitermination protein (NusB) were upregulated (Figure 5B). RimP is a protein required for the maturation of the 30S ribosomal subunit, and NusB is required for the transcription of ribosomal RNA (rRNA) and functions as an antiterminator in rRNA operons. Together, these results consistently indicate that ribosomal proteins are upregulated in C. difficile cocultured with B. longum.
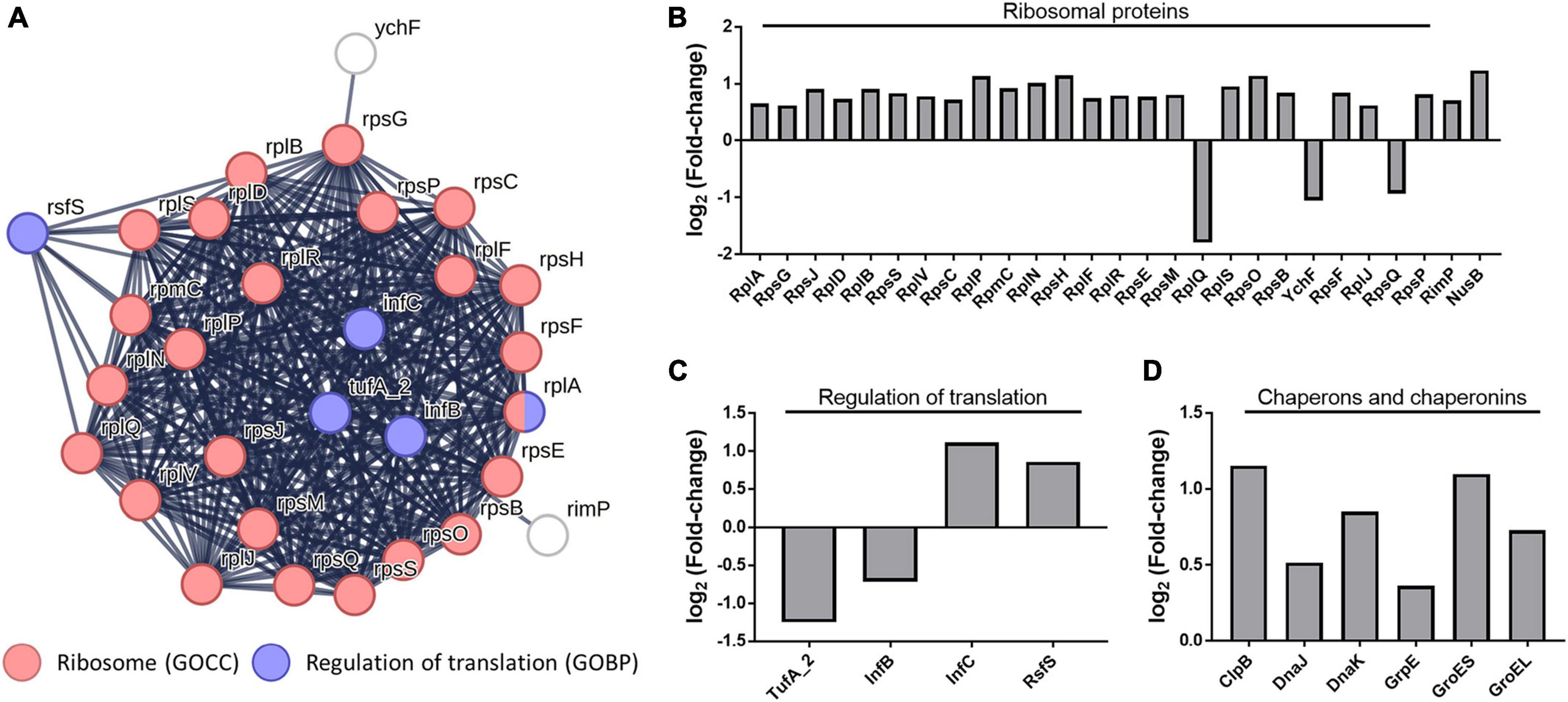
Figure 5. (A) Protein–protein interaction network of the gene ontology (GO) terms “ribosome” and “regulation of translation.” GOCC indicates gene ontology cellular Component and GOBP indicates the gene ontology biological process. Alteration of intracellular protein levels related to (B) ribosome, (C) regulation of translation, (D) molecular chaperones, and chaperonins.
Conversely, changes in the abundance of proteins involved in the regulation of translation indicate protein synthesis inhibition. In our proteomics data, the translation initiation factor IF-2 (InfB) and elongation factor Tu (TufA_2), proteins involved in translation regulation, were downregulated, whereas the translation initiation factor IF-3 (InfC) and ribosomal silencing factor (RsfS) were upregulated (Figure 5C) InfB, a GTPase, promotes the binding of N-formylmethionyl-tRNA to the 30S ribosomal subunit, contributing to the formation of the initiation complex (IC), and catalyzes the hydrolysis of GTP to form the 70S ribosome by joining the 50S and 30S IC (Milon et al., 2010). The InfC protein performs two functions: it constitutes the IC and plays a role in dissociating the 70S ribosome into its constituent 30S and 50S ribosomal subunits (Petrelli et al., 2001). TufA_2 is a G protein that participates in translation elongation by catalyzing GTP hydrolysis, which facilitates the binding of aminoacyl-tRNA to the A-site of the ribosome (Agirrezabala and Frank, 2009). Therefore, InfB and TufA_2 downregulation decreased IC formation and impedes translation elongation, whereas InfC upregulation decreases 70S ribosomal subunit formation. This suggests that translation inhibition decreases protein synthesis in C. difficile cocultured with B. longum. Moreover, RsfS inhibits 70S ribosomal subunit formation in the ribosome (Häuser et al., 2012), indicating that RsfS upregulation inhibits protein synthesis.
Molecular chaperones (ClpB, DnaJ, DnaK, and GrpE) and chaperonins (GroES and GroEL) were observed to be upregulated in C. difficile cocultured with B. longum (Figure 5D). Chaperons and chaperonins are highly expressed in stressful environments, such as high temperatures, where denatured and aggregated proteins are formed (Kedzierska and Matuszewska, 2001). The Heat shock protein 70 (Hsp70) system proteins (i.e., DnaJ and DnaK) and GrpE, play a role in cooperating with ClpB to refold denatured protein aggregates, thereby ensuring protein quality control and promoting cell survival under stressed conditions (Kedzierska and Matuszewska, 2001). The GroES–GroEL chaperonin system facilitates protein folding and prevents aggregation by creating a nanocage environment favorable for protein folding (Hayer-Hartl et al., 2016). The upregulation of these proteins indicates that B. longum induces proteinaceous stress in C. difficile. Thus, proteinaceous stress induction causes the differential regulation of ribosomal proteins, translational regulators, and molecular chaperones and chaperonins.
3.6. B. longum altered the intracellular level of nucleoside phosphates in C. difficile
Deoxyribonucleoside triphosphate (dNTP) and ribonucleoside triphosphate (NTP), which constitute nucleoside triphosphates, are the building blocks of DNA and RNA, respectively. In our metabolomic analysis, nucleoside phosphates [ribonucleoside monophosphate (NMP), ribonucleoside diphosphate (NDP), deoxyribonucleoside monophosphate (dNMP), and deoxyribonucleoside diphosphate (dNDP)], including all NTPs and dNTPs except GMP, were considerably decreased in C. difficile cocultured with B. longum (Figure 6). Conversely, nucleosides were increased in C. difficile cocultured with B. longum (Figure 6). These results suggest that C. difficile growth can be inhibited by depleting NTPs and dNTPs, which are directly required for DNA and RNA syntheses. Furthermore, as nucleoside phosphate synthesis requires sequential phosphorylation with ATP, it is reasonable to speculate that nucleoside accumulation and nucleoside phosphate reduction occurred due to low cellular ATP levels. Moreover, decreased GTP levels are another factor that contribute to translation inhibition in C. difficile, as GTP is used to activate the aforementioned proteins, including InfB and TufA_2. Therefore, NTP and dNTP depletion in C. difficile due to B. longum suppresses C. difficile proliferation by inhibiting DNA replication, transcription, and translation.
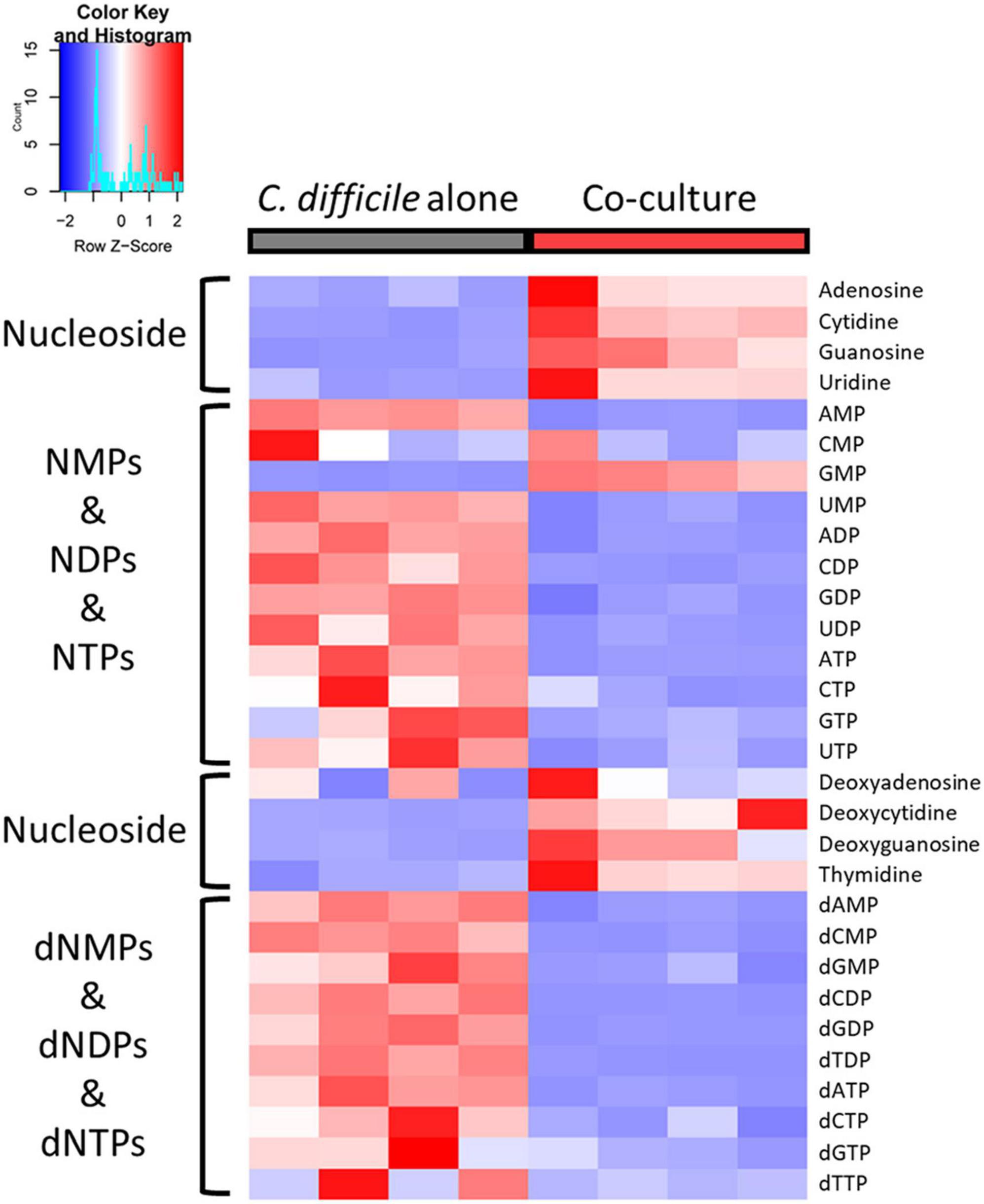
Figure 6. Heatmap of nucleosides and nucleoside phosphates. The red and blue colors indicate relatively high and low abundances, respectively.
4. Conclusion
This study aimed to uncover the molecular mechanisms underlying growth inhibition and reduced toxin production in C. difficile due to B. longum via the screening of C. difficile–inhibiting Bifidobacterium spp. and a multiomics approach combining proteomics and metabolomics. First, B. longum subsp. longum, which exhibited the highest growth inhibition, was screened via coculture of C. difficile with five Bifidobacterium spp. Further, the antimicrobial activity and high lactate production of B. longum were confirmed via spot-on-lawn assay and LC–MS/MS-based organic acid quantification. Subsequently, we identified numerous proteins and metabolites of C. difficile that were significantly altered upon coculture with B. longum using quantitative LC–MS/MS-based multiomics analysis. Among these, we proposed the probable mechanisms of the upregulation of LDH and proline metabolism by B. longum metabolites, namely lactate and proline, which also contribute to the decreased intracellular ATP levels and toxin production, respectively. Furthermore, proteomics analysis revealed increased ribosomal protein levels, decreased translation-related protein levels, and increased molecular chaperone and chaperonin levels, indicating that B. longum induces proteinaceous stress in C. difficile. The metabolomics analysis helped us to deduce the mechanism by which decreased nucleoside phosphate levels, including those of (d)NTPs, due to decreased nucleoside phosphorylation inhibits DNA replication, transcription, and translation, leading to the C. difficile growth inhibition. Thus, we unveiled the mechanisms of growth and toxin production inhibition in C. difficile due to the probiotic bacterium B. longum via a multiomics approach. We look forward to identifying the most critical mechanisms of C. difficile growth and virulence inhibition through further in-depth studies on such mechanisms and hope to contribute to the development of live biotherapeutic products based on molecular mechanisms for CDI treatment.
Data availability statement
The data presented in the study are deposited in the ProteomeXchange Consortium via the PRIDE partner repository (Perez-Riverol et al., 2022), accession number PXD040738. All proteomic and metabolomic datasets used in this paper are available in the Supplementary material.
Author contributions
S-HJ: Conceptualization, Formal analysis, Investigation, Methodology, Project administration, Validation, Visualization, Writing – original draft, Writing – review and editing. H-JJ: Conceptualization, Formal analysis, Investigation, Methodology, Project administration, Validation, Visualization, Writing – original draft. W-SS: Writing – review and editing. J-SL: Writing – review and editing. J-EK; Writing – review and editing. J-HP: Writing – review and editing. Y-RK: Writing – review and editing. M-GK: Writing – review and editing. J-HB: Writing – review and editing. S-YK: Writing – review and editing. J-SK: Writing – review and editing. Y-HY: Writing – review and editing. Y-GK: Conceptualization, Funding acquisition, Project administration, Supervision, Writing – review and editing.
Funding
The authors declare financial support was received for the research, authorship, and/or publication of this article. This work was supported by the Basic Science Research Program through the National Research Foundation of Korea (NRF-2017M3A9E407723531, NRF-2019M2C8A2058418, NRF-2020R1A6A1A03044977, and NRF-2022R1F1A1073595).
Conflict of interest
The authors declare that the research was conducted in the absence of any commercial or financial relationships that could be construed as a potential conflict of interest.
Publisher’s note
All claims expressed in this article are solely those of the authors and do not necessarily represent those of their affiliated organizations, or those of the publisher, the editors and the reviewers. Any product that may be evaluated in this article, or claim that may be made by its manufacturer, is not guaranteed or endorsed by the publisher.
Supplementary material
The Supplementary Material for this article can be found online at: https://www.frontiersin.org/articles/10.3389/fmicb.2023.1293149/full#supplementary-material
References
Agirrezabala, X., and Frank, J. (2009). Elongation in translation as a dynamic interaction among the ribosome, tRNA, and elongation factors EF-G and EF-Tu. Q. Rev. Biophys. 42, 159–200.
Bouillaut, L., Dubois, T., Francis, M. B., Daou, N., Monot, M., Sorg, J. A., et al. (2019). Role of the global regulator Rex in control of NAD+-regeneration in Clostridioides (Clostridium) difficile. Mol. Microbiol. 111, 1671–1688. doi: 10.1111/mmi.14245
Bouillaut, L., Self, W. T., and Sonenshein, A. L. (2013). Proline-dependent regulation of Clostridium difficile Stickland metabolism. J. Bacteriol. 195, 844–854. doi: 10.1128/JB.01492-12
Collins, J., and Auchtung, J. M. (2017). Control of Clostridium difficile infection by defined microbial communities. Microbiol. Spectr. 5:7. doi: 10.1128/microbiolspec.BAD-0009-2016
Cox, J., Hein, M. Y., Luber, C. A., Paron, I., Nagaraj, N., and Mann, M. (2014). Accurate proteome-wide label-free quantification by delayed normalization and maximal peptide ratio extraction, termed MaxLFQ. Mol. Cell. Proteom. 13, 2513–2526. doi: 10.1074/mcp.M113.031591
Cox, J., and Mann, M. (2008). MaxQuant enables high peptide identification rates, individualized ppb-range mass accuracies and proteome-wide protein quantification. Nat. Biotechnol. 26, 1367–1372. doi: 10.1038/nbt.1511
Crow, J. R., Davis, S. L., Chaykosky, D. M., Smith, T. T., and Smith, J. M. (2015). Probiotics and fecal microbiota transplant for primary and secondary prevention of C lostridium difficile infection. Pharmacotherapy 35, 1016–1025. doi: 10.1002/phar.1644
Crowther, G. S., and Wilcox, M. H. (2015). Antibiotic therapy and Clostridium difficile infection–primum non nocere–first do no harm. Infect. Drug Resist. 8:333. doi: 10.2147/IDR.S87224
Deshpande, A., Pasupuleti, V., Thota, P., Pant, C., Rolston, D. D., Sferra, T. J., et al. (2013). Community-associated Clostridium difficile infection and antibiotics: a meta-analysis. J. Antimicrob. Chemother. 68, 1951–1961. doi: 10.1093/jac/dkt129
Goedhart, J., and Luijsterburg, M. S. (2020). VolcaNoseR is a web app for creating, exploring, labeling and sharing volcano plots. Sci. rep. 10, 1–5. doi: 10.1038/s41598-020-76603-3
Goldenberg, J. Z., Yap, C., Lytvyn, L., Lo, C. K. F., Beardsley, J., Mertz, D., et al. (2017). Probiotics for the prevention of Clostridium difficile-associated diarrhea in adults and children. Cochrane Database Syst. Rev. 2017:CD006095.
Guh, A. Y., Mu, Y., Winston, L. G., Johnston, H., Olson, D., Farley, M. M., et al. (2020). Trends in US burden of Clostridioides difficile infection and outcomes. N. Engl. J. Med. 382, 1320–1330. doi: 10.1056/NEJMoa1910215
Häuser, R., Pech, M., Kijek, J., Yamamoto, H., Titz, B., Naeve, F., et al. (2012). RsfA (YbeB) proteins are conserved ribosomal silencing factors. PLoS Genet. 8:e1002815. doi: 10.1371/journal.pgen.1002815
Hayer-Hartl, M., Bracher, A., and Hartl, F. U. (2016). The GroEL–GroES chaperonin machine: a nano-cage for protein folding. Trends Biochem. Sci. 41, 62–76. doi: 10.1016/j.tibs.2015.07.009
Heil, E. L., Harris, A. D., Brown, C., Seung, H., Thom, K. A., Von Rosenvinge, E., et al. (2021). A multicenter evaluation of probiotic use for the primary prevention of Clostridioides difficile infection. Clin. Infect. Dis. 73, 1330–1337. doi: 10.1093/cid/ciab417
Hofmann, J. D., Biedendieck, R., Michel, A.-M., Schomburg, D., Jahn, D., and Neumann-Schaal, M. (2021). Influence of L-lactate and low glucose concentrations on the metabolism and the toxin formation of Clostridioides difficile. PLoS One 16:e0244988. doi: 10.1371/journal.pone.0244988
Karlsson, S., Lindberg, A., Norin, E., Burman, L. G., and Åkerlund, T. (2000). Toxins, butyric acid, and other short-chain fatty acids are coordinately expressed and down-regulated by cysteine in Clostridium difficile. Infect. Immun. 68, 5881–5888. doi: 10.1128/IAI.68.10.5881-5888.2000
Kedzierska, S., and Matuszewska, E. (2001). The effect of co-overproduction of DnaK/DnaJ/GrpE and ClpB proteins on the removal of heat-aggregated proteins from Escherichia coli Δ clpB mutant cells–new insight into the role of Hsp70 in a functional cooperation with Hsp100. FEMS Microbiol. Lett. 204, 355–360. doi: 10.1111/j.1574-6968.2001.tb10910.x
Kelly, C. R., Fischer, M., Allegretti, J. R., Laplante, K., Stewart, D. B., Limketkai, B. N., et al. (2021). ACG clinical guidelines: prevention, diagnosis, and treatment of Clostridioides difficile infections. Am. Coll. Gastroenterol. 116, 1124–1147. doi: 10.14309/ajg.0000000000001278
Kim, S.-M., Park, H.-G., Song, W.-S., Jo, S.-H., Yang, Y.-H., and Kim, Y.-G. (2020). LC–MS/MS based observation of Clostridium difficile inhibition by Lactobacillus rhamnosus GG. J. Ind. Eng. Chem. 85, 161–169.
Kolling, G. L., Wu, M., Warren, C. A., Durmaz, E., Klaenhammer, T. R., and Guerrant, R. L. (2012). Lactic acid production by Streptococcus thermophilus alters Clostridium difficile infection and in vitro Toxin A production. Gut Microbes 3, 523–529. doi: 10.4161/gmic.21757
Kwon, J., Jo, S., Song, W., Lee, J., Jeon, H., Park, J., et al. (2022). Investigation of metabolic crosstalk between host and pathogenic Clostridioides difficile via multiomics approaches. Front. Bioeng. Biotechnol. 10:971739. doi: 10.3389/fbioe.2022.971739
Lee, J. S., Song, W. S., Lim, J. W., Choi, T. R., Jo, S. H., Jeon, H. J., et al. (2022). An integrative multiomics approach to characterize anti-adipogenic and anti-lipogenic effects of Akkermansia muciniphila in adipocytes. Biotechnol. J. 17:2100397. doi: 10.1002/biot.202100397
Leffler, D. A., and Lamont, J. T. (2015). Clostridium difficile infection. N. Engl. J. Med. 372, 1539–1548.
McFarland, L. V., Ozen, M., Dinleyici, E. C., and Goh, S. (2016). Comparison of pediatric and adult antibiotic-associated diarrhea and Clostridium difficile infections. World J. Gastroenterol. 22:3078. doi: 10.3748/wjg.v22.i11.3078
Milon, P., Carotti, M., Konevega, A. L., Wintermeyer, W., Rodnina, M. V., and Gualerzi, C. O. (2010). The ribosome-bound initiation factor 2 recruits initiator tRNA to the 30S initiation complex. EMBO Rep. 11, 312–316. doi: 10.1038/embor.2010.12
Naaber, P., Smidt, I., Štšepetova, J., Brilene, T., Annuk, H., and Mikelsaar, M. (2004). Inhibition of Clostridium difficile strains by intestinal Lactobacillus species. J. Med. Microbiol. 53, 551–554. doi: 10.1099/jmm.0.45595-0
Najarian, A., Sharif, S., and Griffiths, M. (2019). Evaluation of protective effect of Lactobacillus acidophilus La-5 on toxicity and colonization of Clostridium difficile in human epithelial cells in vitro. Anaerobe 55, 142–151. doi: 10.1016/j.anaerobe.2018.12.004
Neumann-Schaal, M., Jahn, D., and Schmidt-Hohagen, K. (2019). Metabolism the difficile way: the key to the success of the pathogen Clostridioides difficile. Front. Microbiol. 10:219. doi: 10.3389/fmicb.2019.00219
Pal, R., Athamneh, A. I., Deshpande, R., Ramirez, J. A., Adu, K. T., Muthuirulan, P., et al. (2022). Probiotics: insights and new opportunities for Clostridioides difficile intervention. Crit. Rev. Microbiol. 49, 414–434. doi: 10.1080/1040841X.2022.2072705
Pang, Z., Chong, J., Zhou, G., De Lima Morais, D. A., Chang, L., Barrette, M., et al. (2021). MetaboAnalyst 5.0: narrowing the gap between raw spectra and functional insights. Nucleic Acids Res. 49, W388–W396. doi: 10.1093/nar/gkab382
Park, J.-H., Song, W.-S., Lee, J., Jo, S.-H., Lee, J.-S., Jeon, H.-J., et al. (2022). An Integrative Multiomics Approach to Characterize Prebiotic Inulin Effects on Faecalibacterium prausnitzii. Front. Bioeng. Biotechnol. 10:825399. doi: 10.3389/fbioe.2022.825399
Perez-Riverol, Y., Bai, J., Bandla, C., García-Seisdedos, D., Hewapathirana, S., Kamatchinathan, S., et al. (2022). The PRIDE database resources in 2022: a hub for mass spectrometry-based proteomics evidences. Nucleic Acids Res. 50, D543–D552. doi: 10.1093/nar/gkab1038
Petrelli, D., La Teana, A., Garofalo, C., Spurio, R., Pon, C. L., and Gualerzi, C. O. (2001). Translation initiation factor IF3: two domains, five functions, one mechanism? EMBO J. 20, 4560–4569.
Pickard, J. M., Zeng, M. Y., Caruso, R., and Núñez, G. (2017). Gut microbiota: role in pathogen colonization, immune responses, and inflammatory disease. Immunol. Rev. 279, 70–89.
Schubert, A. M., Sinani, H., and Schloss, P. D. (2015). Antibiotic-induced alterations of the murine gut microbiota and subsequent effects on colonization resistance against Clostridium difficile. MBio 6, e974–e915. doi: 10.1128/mBio.00974-15
Song, W.-S., Park, H.-G., Kim, S.-M., Jo, S.-H., Kim, B.-G., Theberge, A. B., et al. (2020). Chemical derivatization-based LC–MS/MS method for quantitation of gut microbial short-chain fatty acids. J. Ind. Eng. Chem. 83, 297–302. doi: 10.4155/bio-2018-0241
Song, W. S., Shin, S. G., Jo, S. H., Lee, J. S., Jeon, H. J., Kwon, J. E., et al. (2021). Development of an in vitro coculture device for the investigation of host–microbe interactions via integrative multiomics approaches. Biotechnol. Bioeng. 118, 1593–1604. doi: 10.1002/bit.27676
Spinler, J. K., Auchtung, J., Brown, A., Boonma, P., Oezguen, N., Ross, C. L., et al. (2017). Next-generation probiotics targeting Clostridium difficile through precursor-directed antimicrobial biosynthesis. Infect. Immun. 85, e00303–e00317. doi: 10.1128/IAI.00303-17
Szklarczyk, D., Gable, A. L., Nastou, K. C., Lyon, D., Kirsch, R., Pyysalo, S., et al. (2021). The STRING database in 2021: customizable protein–protein networks, and functional characterization of user-uploaded gene/measurement sets. Nucleic Acids Res. 49, D605–D612.
Tyanova, S., Temu, T., Sinitcyn, P., Carlson, A., Hein, M. Y., Geiger, T., et al. (2016). The Perseus computational platform for comprehensive analysis of (prote) omics data. Nat. Methods 13, 731–740. doi: 10.1038/nmeth.3901
Valdés-Varela, L., Alonso-Guervos, M., García-Suárez, O., Gueimonde, M., and Ruas-Madiedo, P. (2016). Screening of bifidobacteria and lactobacilli able to antagonize the cytotoxic effect of Clostridium difficile upon intestinal epithelial HT29 monolayer. Front. Microbiol. 7:577. doi: 10.3389/fmicb.2016.00577
Valdés-Varela, L., Gueimonde, M., and Ruas-Madiedo, P. (2018). Probiotics for prevention and treatment of Clostridium difficile infection. Adv. Exp. Med. Biol. 1050, 161–176.
Weghoff, M. C., Bertsch, J., and Müller, V. (2015). A novel mode of lactate metabolism in strictly anaerobic bacteria. Environ. Microbiol. 17, 670–677. doi: 10.1111/1462-2920.12493
Wei, Y., Yang, F., Wu, Q., Gao, J., Liu, W., Liu, C., et al. (2018). Protective effects of bifidobacterial strains against toxigenic Clostridium difficile. Front. Microbiol. 9:888. doi: 10.3389/fmicb.2018.00888
Wiśniewski, J. R., Zougman, A., Nagaraj, N., and Mann, M. (2009). Universal sample preparation method for proteome analysis. Nat. Methods 6, 359–362.
Yuan, M., Breitkopf, S. B., Yang, X., and Asara, J. M. (2012). A positive/negative ion–switching, targeted mass spectrometry–based metabolomics platform for bodily fluids, cells, and fresh and fixed tissue. Nat. Protoc. 7:872. doi: 10.1038/nprot.2012.024
Yun, B., Song, M., Park, D.-J., and Oh, S. (2017). Beneficial effect of Bifidobacterium longum ATCC 15707 on survival rate of Clostridium difficile infection in mice. Korean J. Food Sci. Anim. Resourc. 37:368. doi: 10.5851/kosfa.2017.37.3.368
Keywords: Bifidobacterium longum, Clostridioides difficile, microbe-microbe interaction, molecular mechanism, multiomics
Citation: Jo S-H, Jeon H-J, Song W-S, Lee J-S, Kwon J-E, Park J-H, Kim Y-R, Kim M-G, Baek J-H, Kwon S-Y, Kim J-S, Yang Y-H and Kim Y-G (2023) Unveiling the inhibition mechanism of Clostridioides difficile by Bifidobacterium longum via multiomics approach. Front. Microbiol. 14:1293149. doi: 10.3389/fmicb.2023.1293149
Received: 13 September 2023; Accepted: 23 October 2023;
Published: 08 November 2023.
Edited by:
Félix López De Felipe, Spanish National Research Council (CSIC), SpainReviewed by:
Takahiko Nagamine, Sunlight Brain Research Center, JapanMeng Zhang, Inner Mongolia Agricultural University, China
Copyright © 2023 Jo, Jeon, Song, Lee, Kwon, Park, Kim, Kim, Baek, Kwon, Kim, Yang and Kim. This is an open-access article distributed under the terms of the Creative Commons Attribution License (CC BY). The use, distribution or reproduction in other forums is permitted, provided the original author(s) and the copyright owner(s) are credited and that the original publication in this journal is cited, in accordance with accepted academic practice. No use, distribution or reproduction is permitted which does not comply with these terms.
*Correspondence: Yun-Gon Kim, eWdraW1Ac3N1LmFjLmty
†These authors have contributed equally to this work