- 1Division of Clinical and Translational Therapeutics, School of Pharmacy and Pharmaceutical Sciences, University at Buffalo, Buffalo, New York, NY, United States
- 2Department of Biology, San Diego State University, San Diego, CA, United States
Bacteriophage (phage) therapy is being explored as a possible response to the antimicrobial resistance public health emergency. Administering a mixture of different phage types as a cocktail is one proposed strategy for therapeutic applications, but the optimal method for formulating phage cocktails remains a major challenge. Each phage strain has complex pharmacokinetic/pharmacodynamic (PK/PD) properties which depend on the nano-scale size, target-mediated, self-dosing nature of each phage strain, and rapid selection of resistant subpopulations. The objective of this study was to explore the pharmacodynamics (PD) of three unique and clinically relevant anti-Pseudomonas phages after simulation of dynamic dosing strategies. The Hollow Fiber Infection Model (HFIM) is an in vitro system that mimics in vivo pharmacokinetics (PK) with high fidelity, providing an opportunity to quantify phage and bacteria concentration profiles over clinical time scales with rich sampling. Exogenous monotherapy-bolus (producing max concentrations of Cmax = 7 log10 PFU/mL) regimens of phages LUZ19, PYO2, and E215 produced Pseudomonas aeruginosa nadirs of 0, 2.14, or 2.99 log10 CFU/mL after 6 h of treatment, respectively. Exogenous combination therapy bolus regimens (LUZ19 + PYO2 or LUZ19 + E215) resulted in bacterial reduction to <2 log10 CFU/mL. In contrast, monotherapy as a continuous infusion (producing a steady-state concentration of Css,avg = 2 log10PFU/mL) was less effective at reducing bacterial densities. Specifically, PYO2 failed to reduce bacterial density. Next, a mechanism-based mathematical model was developed to describe phage pharmacodynamics, phage–phage competition, and phage-dependent adaptive phage resistance. Monte Carlo simulations supported bolus dose regimens, predicting lower bacterial counts with bolus dosing as compared to prolonged phage infusions. Together, in vitro and in silico evaluation of the time course of phage pharmacodynamics will better guide optimal patterns of administration of individual phages as a cocktail.
Introduction
Phage therapy is the treatment of infectious disease using infusions of bacterial viruses called bacteriophages (phages). The origins of phage therapy can be traced back to the early 1920s with the work of d’Herelle (1917), Summers (2012). However, the development of phages as antibacterial agents waned as highly active small molecule antibiotics became commonplace (Stennett et al., 2022). There are many barriers to the success of phage therapy including drug delivery strategies, manufacturing, clinical dosing strategies, and rapid development of resistance (Oechslin, 2018; Suh et al., 2022; Champagne-Jorgensen et al., 2023; Petrovic Fabijan et al., 2023). Regarding the clinical pharmacology of phages, dosing strategies are unclear given the complexities of phage pharmacodynamics in vivo and the effects of phage resistance on efficacy and longevity of any developed phage therapeutic (Abedon, 2019; Hatfull et al., 2022; Nang et al., 2023). With the emergence of extensively and pan drug resistant bacteria, phage therapies are being revitalized as one of the few alternative strategies to combat bacterial diseases (Magiorakos et al., 2012; Gottig et al., 2014; Chen et al., 2017; Tacconelli et al., 2018; CDC, 2019).
To maximize bacterial killing and minimize proliferation of resistant subpopulations, often two or more phage strains with different viral properties are used in combination as a cocktail, here referred to as an “N-phage cocktail.” An optimal N-phage cocktail can both increase spectrum of activity and potentially prevent the selection of resistant mutants by, for example, optimizing selection of unique bacterial cell surface receptors (Abedon et al., 2021; Li et al., 2022; Naknaen et al., 2023). Phages however have multiple infective properties that may also affect the time-course of their pharmacology, for example adsorption rate, burst size, latent period, genome content/size (surrogate for viral complexity), and mutation frequency (Kannoly et al., 2022; Abedon, 2023). These properties vary by phage type and target host bacteria (Abedon et al., 2003; Forti et al., 2018; Nabergoj et al., 2018). As a result, phages represent a class of anti-bacterials where the traditional pharmacokinetic/pharmacodynamic (PK/PD) indices of action are no longer applicable (e.g., percent time above MIC, area under the concentration-time curve, or maximum concentration). Thus, in the evaluation of conventional antibiotics, drug exposure is independent from the exposure-response relationship. Whereas the self-replication of phages complicates the characterization of phage-specific pharmacodynamics. With phage therapy, exposure is no longer an independent variable and lysis outcomes of phage-bacteria interactions are directly correlated to gross anti-bacterial activity (Venturini et al., 2022; Nang et al., 2023). Therefore, developing an efficient N-phage cocktail requires optimal selection and administration of multiple phage strains, leveraging their individual viral properties. In addition, strain–strain interactions can also influence cocktail performance.
The complexity of phage dosing is largely related to their nano-scale sizes, which influences drug disposition. Target-mediated drug disposition (TMDD) is defined as the ability of a drug binding to its receptor to alter its own disposition, elimination, or a combination of both (Levy, 1994). The concept was further refined with the emergence and proliferation of biologics, such as monoclonal antibodies (Dua et al., 2015; An, 2020). However, the unmodified TMDD framework does not accurately characterize the unique aspects of phage therapy. Indeed, TMDD already accounts for an initial rapid, target-dependent decrease in phage concentration due to viral adsorption. However, phage lytic replication (i.e., auto-dosing) is also target-dependent, which results in an increase in phage concentration over time. Therefore, employing a target-mediated phage disposition (TMPD) framework captures the self-replicating mode of phage action and can account for phages’ nonlinear PK.
Moreover, quantifying phage parameters of infectivity can also be accomplished using a mechanism-based mathematical model of phage PD using in vitro phage activity patterns. For instance, PK/PD optimization of an N-phage cocktail should consider both the properties of each phage strain and measured phage strain–strain interaction. Previous models of individual phage activities have largely implemented a susceptible, infected, and recovered subpopulations of bacteria (SIR) model structure (Cairns et al., 2009; Landersdorfer et al., 2013; Styles et al., 2021). This foundation model can be adapted with modern concepts of drug–drug interactions to facilitate description of individual phage activity alongside phage–phage interaction (e.g., additive, synergistic, or antagonistic).
Preclinical PK/PD models play a critical role in designing human dosage regimens and are essential tools for drug development. For antibacterial PK/PD, the in vitro hollow fiber infection model (HFIM) can provide valuable and complementary information for dose selection and translation of antimicrobials from the laboratory to humans (Lodise et al., 2020, 2022). The HFIM is an ideally suited in vitro model for evaluating phage PK/PD and identify target concentrations that best predict bacterial killing and resistance prevention. That is, the HFIM can be leveraged to develop recommendations, identify common pitfalls, and describe the applications, strengths, and limitations of translational approaches over clinically relevant timeframes (Lodise et al., 2020, 2022). This system can simulate virtually any time course of phage concentrations for one or multiple phages with the same or different half-lives. The hollow fiber cartridge has a large surface-to-volume ratio providing optimized growth conditions for bacteria and waste products are continually removed. Ultimately, combining HFIM data of individual phage activity and phage–phage pairs can be leveraged with mechanism-based mathematical modeling to devise optimal phage cocktails both in terms of constituent phage types and phage type administration pattern.
Because the widespread implementation of phage therapy in routine clinical practice is impeded by the scarcity of clinical data, such as randomized controlled trials (RCTs), characterizing preclinical PK/PD relationships of phages in needed to adapt future research and therapy development. In this study, we used a mechanism-based PK/PD evaluation of three clinically relevant virulent phages (LUZ19, PYO2, and E215) that all infect the opportunistic pathogen Pseudomonas aeruginosa. This bacterium can cause several types of human infections and is often resistant to many classes of antibiotics and therapeutic agents, because of its problematic nature during infection it has become a common treatment target for phage therapy (Lin et al., 2017; Luong et al., 2020; Uyttebroek et al., 2022). Here, we utilized a preclinical PK/PD infection model to elucidate N-phage cocktail exposure-response relationships and to subsequently identify optimal administration methods.
Material and methods
Bacteria, phage, and media
Pseudomonas aeruginosa laboratory strain PAO1 was used for all experiments. All culturing was conducted in Mueller–Hinton Broth (Mg2+ 25 mg/L, Ca2+ 12.5 mg/L) or agar (Becton Dicksinson, Sparks, MD) at 37°C. Agar (1.5%) was added to MHB for solid growth medium. Previously characterized virulent podovirus LUZ19 (Lavigne et al., 2013), podovirus PYO2 (Forti et al., 2018), virulent myovirus E215 (Forti et al., 2018) were freshly cultured and purified before treatment, using a methodology previously described (Luong et al., 2020). Briefly, phage lysates were sterilized by 2× high-speed centrifugation and 0.2 μm dead-end filtration. Fresh phage stocks were prepared every 48 h and stored, protected from light, at 4°C.
Hollow fiber infection model
PAO1 was studied over 7 d using polyvinylidene fibers (C2025, FiberCell, New Market, MD) with 0.1 μm pore size. The system half-life was fixed to 2 h based on assessment of limited available literature concerning in vivo PK (Bichet et al., 2021; Suh et al., 2022; Nang et al., 2023). The total system volume of distribution was 125 mL with a clearance rate of 0.7 mL/min. For bolus strategies with a target Cmax = 7 log10 (PFU/mL), a stock concentration of 11.6 log10 PFU/mL was used. For continuous infusion strategies with a target Css,avg = 2 log10 PFU/mL, a stock concentration of 6.58 log10 (PFU/mL) was used.
Serial samples were collected for enumeration of bacteria and phages during treatment. Bacteria were quantified from samples obtained from the extracapillary space of the HFIM cartridge. Bacteria were quantified by serially diluting samples and plating 50 μL on MHA then incubating for 24 h prior to automated enumeration (Protos, Synbiosis). By comparison, phages were quantified in both the central reservoir and the extracapillary space of the HFIM cartridge to fully account for full disposition of phage in the system. Individual phage strains were distinguished through quantification on PAO1-resistant mutant lawns (i.e., PAO1LUZ19R, PAO1E215R, and PAO1PYO2R). Phages were quantified by double aliquot 48-spot serial titration, as previously described. Briefly, phage-resistant PAO1 mutant cultured at OD600 0.2 was lawned and dried over agar medium before spotting two identical 8-well columns of tenfold serial diluted phage 4 μL samples. Agar plates were incubated overnight at 37°C before plaque enumeration. Data were graphed using R (version 4.3.0) with no observable counts (i.e., 0 PFU) plotted as 0 log10 PFU/mL for visualization purposes.
In vitro pharmacokinetics and treatment regimens
Regimens were designed to discriminate between phages administered and those produced endogenously during treatment. This controlled for the dynamic administration of doses over time to the HFIM, as well as support parameter identifiability in the mathematical modeling. Choice of regimen was also made to ensure that eventual mathematical modeling would be able to identify PK parameters related to disposition to the cartridge along with PD parameters. To accomplish these goals, single bolus dosing and continuous infusion strategies were implemented. Single bolus dosing provides a clear peak concentration (Cmax), followed by exponentially declining counts following the system’s 2 h half-life. The dose utilized for bolus dosing was designed to empirically achieve a peak concentration of 7 log10 (PFU/mL), which corresponds to a multiplicity of infection of 1:10. By comparison, keeping the phage concentration constant with continuous infusion can ensure that the phage concentration more readily reaches a steady state. Dosing was selected to achieve a steady-state concentration of 102 PFU/mL to maximize the dynamic range that we can quantify endogenous production of phage. Using this method, concentrations observed >102 PFU/mL were attributed to endogenous production. The 11 regimens are outlined in Table 1. Combination regimens were tested in duplicate and reported as the mean value.
Mechanism-based mathematical modeling
A mechanism-based model was developed with ordinary differential equations (ODE) to quantify the PD of phage monotherapy and 2-phage cocktails using Monolix version 2023R1 (Lixoft, Antony, FR). Model estimates were determined via the stochastic approximation expectation maximization algorithm with standard errors calculated through linearization of the Fisher Information Matrix. Inter-experimental variability was handled by empirically fixing random effects for parameters dependent on experimental set-up or growth conditions to 5% CV (LGCFUMX, LGINOC, MGT, τ, N). For all other parameters, the random effects were fixed to 0.
Given the pharmacodynamic complexity of the phage infection and replication, we next developed a mechanism-based Susceptible-Infected-Recovered (SIR) mathematical model. Importantly, we updated the SIR model to include adaptive phage resistance, which we believe is enviable to occur during phage therapy (Cairns et al., 2009; Jacobs et al., 2016). To produce the most parsimonious model, resistance to PYO2 or E215 was modeled as a single process given both phages target the O-antigen region of the LPS, which was dependent on the concentration of phage-infected host cells, namely virocells. Therefore, only a single resistant sub-population was used to describe LUZ19-susceptible, PYO2/E215-resistant cells.
We describe PAO1 as a single initial subpopulation of cells susceptible to all three phage strains and the cells growth rate was modeled as mean generation time (MGT) (Smith et al., 2022). In addition, a logistic growth model with a shape parameter was used to predict the bacterial population’s saturable growth to its carrying capacity. As mentioned, phage resistance was modeled as an adaptive process, where exposure to a given phage strain selects for resistant mutants and a unique growth rate was estimated MGT of a triple-phage resistant subpopulation to account for mutation fitness loss.
Phage action characterized by adsorption and bacterial conversion to virocells were both described by a second order rate constant. Virocells were modeled as maturing through a series of transit compartments by a first order rate constant, ktr, which, by Eqs. (1)–(3), was fit in terms of the mean transit time through all compartments (Supplemental Material). The initial estimates of mean transit time were set to the previously measured one-step latent periods for each phage strain (Lavigne et al., 2013; Forti et al., 2018).
Phage–phage interactions were characterized as collateral effects of virocells presence of one phage on the adsorption rate of the other phage. This was accomplished by utilizing the virocell concentration in the fourth transit compartment to drive a Hill-type function. The EC50 is thus described as the concentration of virocells for 50% of maximum interaction. The shape parameter was fixed to 5 as it was found to greatly increase the model stability and produced an ‘on/off’-effect related to adaptive resistance and phage–phage interactions. Initially, all possible interactions were tested (i.e., PYO2 on LUZ19, E215 on LUZ19, LUZ19 on PYO2, or LUZ19 on E215). Interaction parameters were backward eliminated based on their respective Wald statistic.
Models were compared based changes in the objective function (−2•Log-likelihood) and diagnostic plots. Data below the limit of quantification (102 CFU/mL or 102 PFU/mL) were modeled as censored data, per the Monolix documentation. Data visualization was performed using ggplot2 and R version 4.2.2 (Fidler et al., 2018). Parameters were backwards eliminated, based on parameters that had the largest Wald-statistic.
Monte Carlo simulation of different regimen structures
The final model was then leveraged to perform simulation-based studies testing different clinical administration strategies to identify the influence of dose and dose fractionation on the pharmacodynamic response. To test the ability to extend small molecule antibiotic dosing practices to the dosing of phages, Monte Carlo simulations were performed utilizing the mechanism-based model. First, dose escalation strategies were explored by simulating monotherapy with each phage with a dose of 3, 4, 5, 6, 7, 8, or 9 log10 (PFU)/day given as a single daily bolus over 48 h. Second, we simulated the effects of dose fractionation by utilizing a constant 7 log10 (PFU)/day dose divided across 1, 2, 3, 4, or 6 doses per day. Simulations were performed using RxODE in R (Fidler et al., 2018).
Results
Phage therapy in the hollow fiber infection model
To verify that the experimental set-up produced the targeted pharmacokinetics, phage concentrations in the central reservoir were analyzed by linear regression and found to decline with a 3.25, 2.54, and 3.08 h half-life for PYO2, E215, and LUZ19 (Figure 1; Supplementary Figure S1). However, the endogenous production of phage resulted in persistently elevated phage concentrations throughout 168 h. In contrast, phage concentrations from the continuous infusion regimens exhibited increased stochasticity for the first 4 h given the quantification limit of 2 log10 (CFU/mL), until endogenously produced phage could distribute from the cartridge back to the central reservoir.
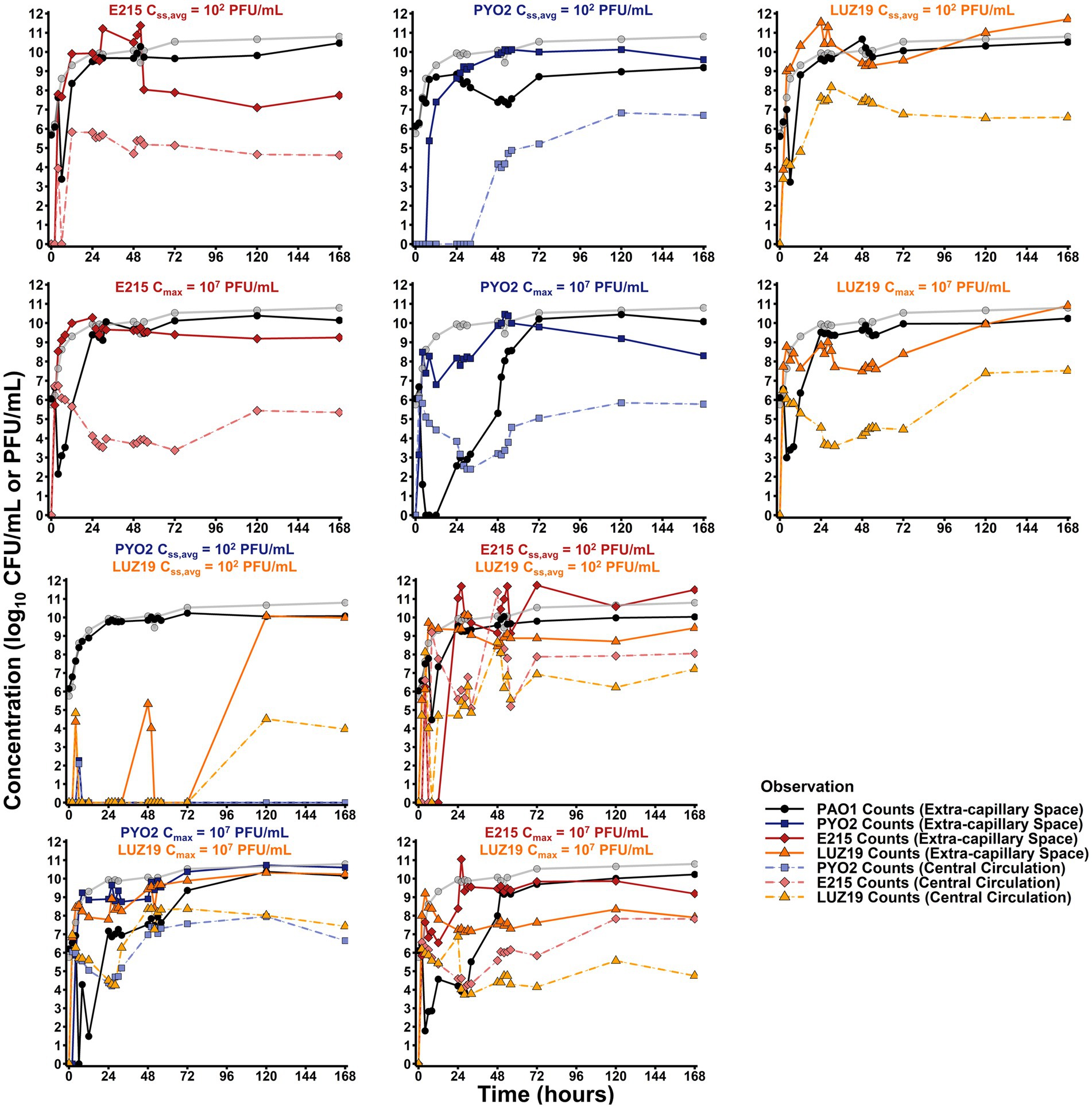
Figure 1. Hollow fiber infection model results. Total observed counts for PAO1 (black) for each regimen tested are overlayed with the growth control (gray). Total counts in the extra-capillary space/central circulation for E215 (dark red/light red), PYO2 (dark blue/light blue), or LUZ19 (dark orange/light orange) are reported with relevant experiments. Bolus regimens display clear first order elimination, as expected, from 0 to 24, when counts either plateau or increase due to endogenous production of phage.
The monotherapy control arms for all three phages were given as either bolus dosing or continuous infusion. Bolus dosing emulated a therapeutic strategy where selected phage concentrations would rapidly achieve a target MOI of 10. Using backward extrapolation from the 2 h sample, PYO2, E215, and LUZ19 were estimated as having peak concentrations of 6.64, 6.88, and 6.67 log10 (PFU/mL) in the central reservoir after bolus administration at 0 h, resulting in a measured MOI of 19. Monotherapy bolus regimens with PYO2, E215, or LUZ19 resulted in significant bactericidal activity as defined by >99.9% or 3 log10 (CFU/mL) reduction and resulted in minimum counts of 0, 2.14, or 2.99 log10 (CFU/mL), respectively. By comparison, continuous infusion regimens were designed to reach a steady-state concentration of 2 log10 (PFU/mL), which would take 10 h given the 2 h half-life of the system. In doing so, antibacterial effects from the continuous infusion regimens could be largely attributed to endogenous production of phages. The continuous infusion strategy resulted in less bacterial killing, for all three phage strains. That is, PYO2 monotherapy failed to produce any bacterial killing as continuous infusion, whereas E215 or LUZ19 monotherapy producing nadirs of 3.38 or 3.23 log10 (CFU/mL), respectively. Across all bactericidal regimens, peak bacterial killing was observed at 6 h, except for monotherapy bolus with LUZ19 which achieved peak killing effect at 4 h.
To test for phage–phage interactions, combination therapy was explored with either LPS-binding phage (i.e., PYO2 or E215) with the pili-binding phage (LUZ19). Duplicate runs of both combination regimens (i.e., PYO2 + LUZ19 and E215 + LUZ19) resulted in bactericidal activity (≥3 log10 CFU/mL reduction) an increased duration of bacterial suppression when utilizing bolus dosing, but both combination regimens exhibited nominal activity under continuous infusion. Single bolus dosing of PYO2 + LUZ19 achieved undetectable counts by 6 h then grew to the system’s carrying capacity after 24 h. By comparison, single bolus dosing of E215 + LUZ19 reached a nadir of 4.47 CFU/mL by 8 h, indicating potential antagonism in the case of simultaneous administration.
Mechanism-based susceptible-infected-adaptively resistant mathematical model
The data were well described by the modified SIR model (see Figure 2) that incorporated susceptible, infected, and adaptively resistant cell subpopulations as indicated by objective model fitting criteria (Table 2; Supplementary Figure S2). Post Hoc fits (Figure 3) show bacterial counts for all regimens to be well characterized. For both all phage susceptible and single-phage resistant subpopulations, the MGT of cells were estimated at 23.1 min (3.73% RSE) and MGT of double phage-resistant subpopulation were estimated at 56.6 min (4.36% RSE) to account for growth fitness loss.
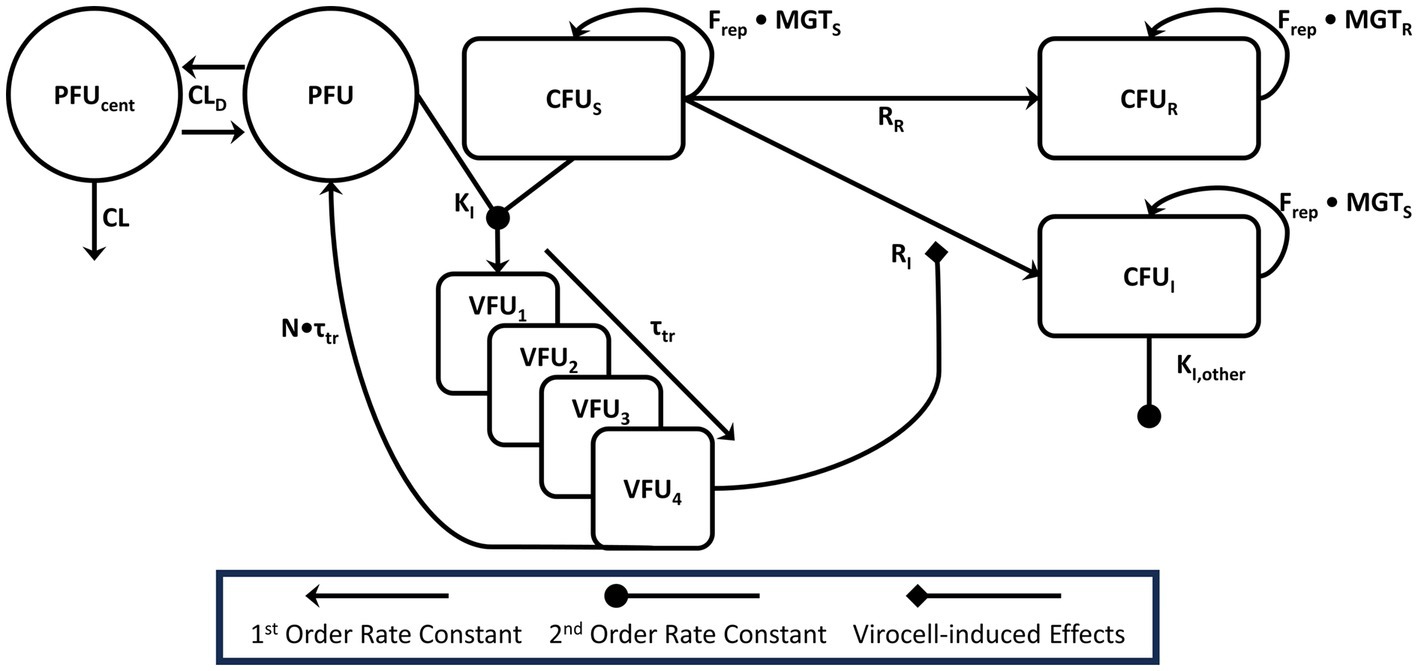
Figure 2. Model diagram this figure is representative for a single phage that infects a given bacterial sub-population. Our platform mechanism-based mathematical model of phage action accounts for the observed phage pharmacokinetics, including the distributional clearance (CLD) between the central reservoir and extracapillary space. Phage (PFU) and bacteria (CFUS or other sensitive sub-populations) were expected to intermingle in the extracapillary space and bind with rate constant KI, indicated by the merging lines connecting the PFU and CFUS compartments. Virocells mature through 4 transit compartments with a total transit time of τtr. Virocells in the final compartment (VFU4) were modeled as inducing the adaptive resistance process, which would convert pan-sensitive cells into either single-phage resistant mutants (CFUI) or double phage resistant mutants (CFUR). For combination therapy, single-phage resistant PAO1 mutants could be acted on by the other phage in an identical manner. Phage–phage interactions were tested empirically on the adsorption rate constant and included based on likelihood ratio testing. For model parsimony, phage–phage interactions were driven by the same virocell-induced effect process that governs adaptive resistance. Bacteria were all modeled as growing logistically (Frep) with a mean generation time (MGTS) for phage-sensitive or single-resistant phages subpopulations or MGTR for 3-phage resistant subpopulations.
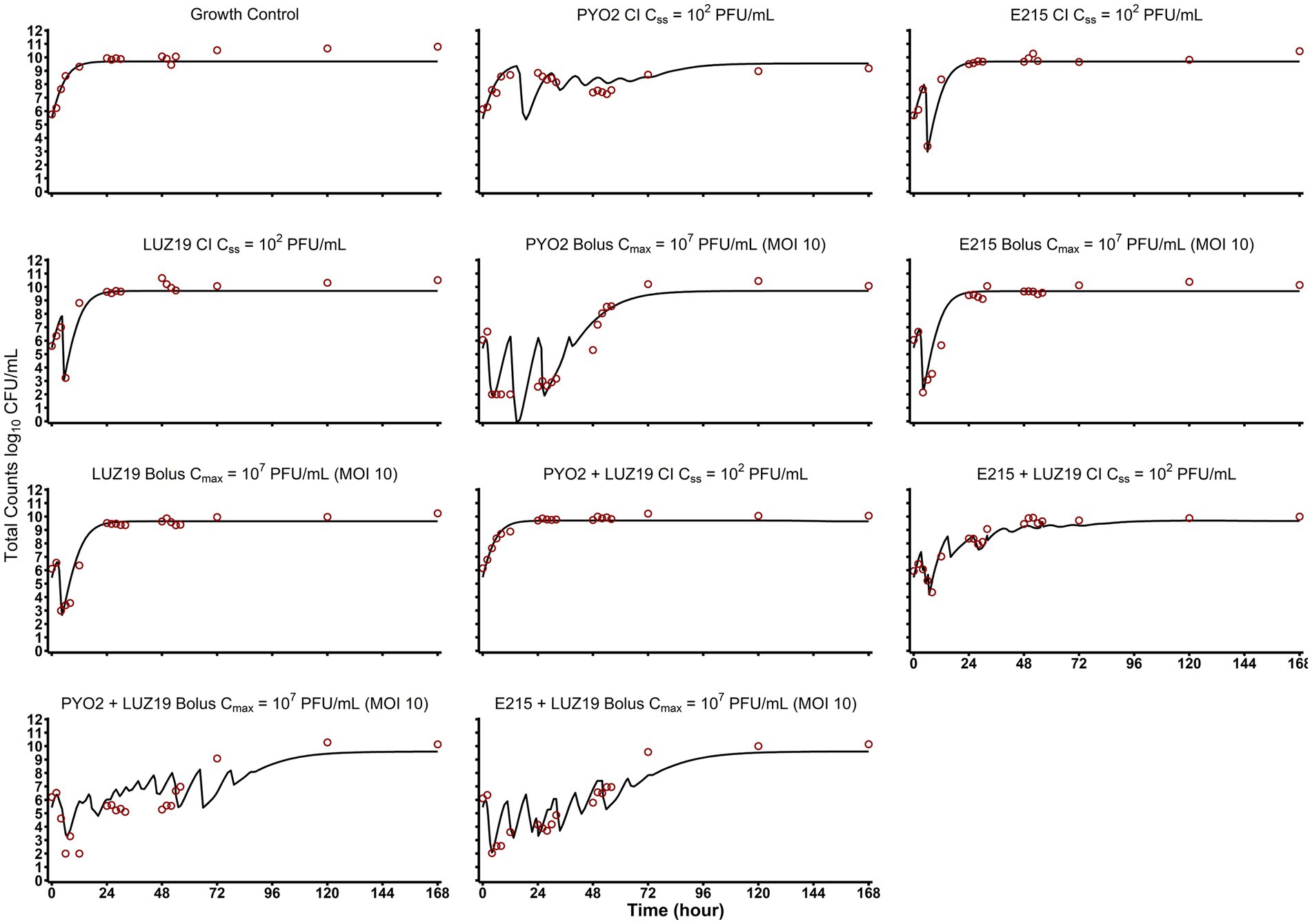
Figure 3. Post Hoc model fits and observed versus predicted plots. Here the individual model fits are depicted for each of the HFIM experimental arms. Combination therapies are graphed as averaged values of the log-transformed concentrations (n = 2). Quantification limits were plotted at 102 CFU/mL and treated as censored data in the mechanism-based mathematical modeling.
Cell adsorption rates for PYO2, E215, and LUZ19 were estimated using the SIR model as −9.64 (0.582% RSE), −9.48 (0.0968% RSE), and −8.43 (0.0973% RSE) log10 (mL•PFU−1•h−1), respectively. E215 was estimated as having a burst size of 168 PFU/CFU, which was significantly larger than the estimated PYO2 burst size of 73.8 PFU/CFU or estimated LUZ19 burst size of 83.0 PFU/CFU. These extrapolated phage properties were in accordance with previously values for these phages (Ceyssens et al., 2011; Forti et al., 2018).
Monte Carlo simulation of phage dosing strategies
Simulations of dose escalation strategies from 3 to 10 log10 (PFU) per day showed varying effects that were phage strain-dependent (Figure 4). PYO2 treatment was predicted to produce a more significant reduction in bacterial counts, but after a longer latent period of activity. By comparison, both E215 and LUZ19 showed similar extents to bacterial killing despite increasing the phage dose by 7 orders of magnitude. Outside to dose effects on extent of bacterial killing, dose also influenced the rate of predator–prey cycling observed for PYO2, with larger doses predicted to produce an increased frequency of the bacterial killing-regrowth cycle. Utilizing typical dose fractionation studies, dividing a daily dose of 7 log10 (PFU)/d across multiple individual doses resulted in a negligible impact on the extent of bacterial killing or the rate of predator–prey cycling (Supplementary Figure S3). Concentration of phages in simulations of dose fractionation were consistently higher than the expected Cmax from administration alone, indicating that the endogenous phage production is expected to obfuscate the exogenous administration for all dose fractionation strategies.
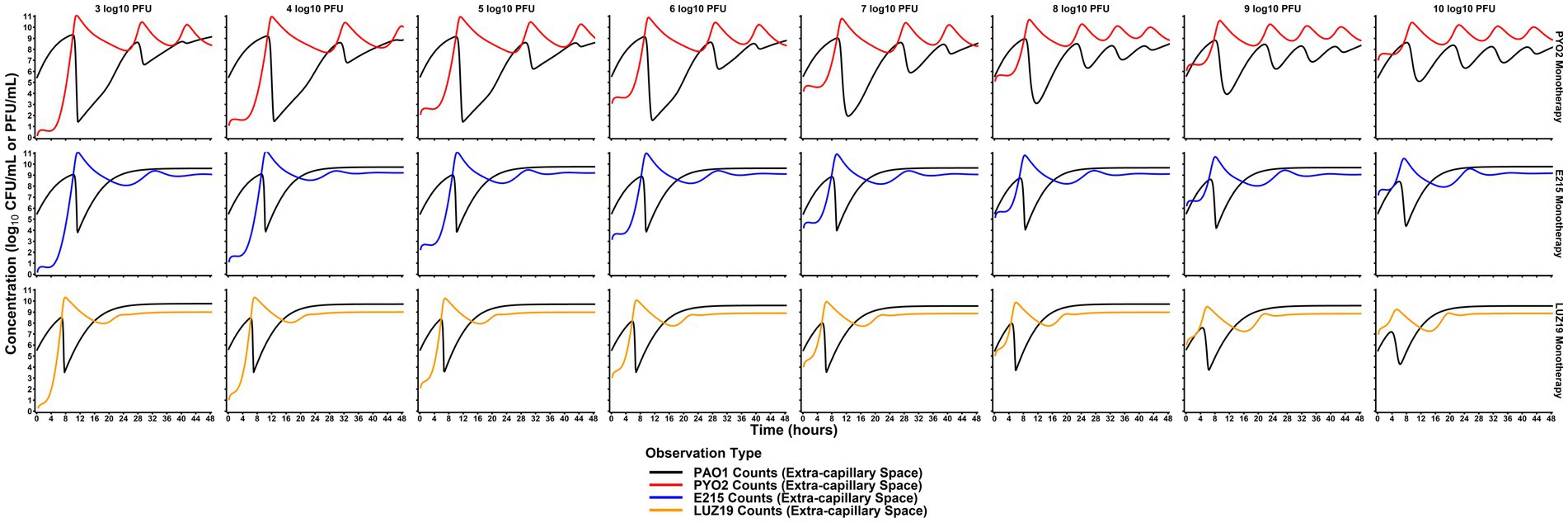
Figure 4. Comparison of dose effects on PAO killing. Monte Carlo simulations were performed at each of the listed doses (columns) for each phage monotherapy (rows) based on the median PD profiles predicted by the mechanism-based model. Simulations of monotherapy regimens predicted that phages could provide therapeutic benefit even at doses significantly lower than the bacterial burden. This is indicated by the significant decrease in concentration even with low doses (3–5 log10 PFU). Compared to dose-fractionation simulations (Supplemental material), initial dose level was more influential on bacterial killing. Paradoxically, the extent of bacterial killing is predicted to decrease as PYO2 dose increases with more frequent predator–prey cycling. Ultimately, these simulation-based studies are critical for translating phages for human use and designing future studies efficiently.
Discussion
This study is the first to quantify the full time-course of phage PD in the HFIM, which was then used to identify optimal target concentrations for a three-phage cocktail using a hybrid ML-PK/PD approach. Current best practice recommendations acknowledge many gaps in the foundational knowledge of phage PK/PD for clinical use in humans (Suh et al., 2022). This study investigated three clinically relevant Pseudomonas virulent phages in vitro under dynamic conditions to quantitatively assess the PD of mono- and 2-phage cocktail treatments. We used the HFIM to explore expected phage TMPD over clinically relevant time periods. Altogether, the most relevant aspects of TMPD relate to self-dosing at the site of infection, second-order phage-bacteria binding processes, maturation of virocells, and phage–phage interactions, which can be quantified in vitro for pre-clinical assessment. Through mathematical modeling, we found that adsorption rate, latent period, and burst size were significant factors affecting TMPD of an individual phage, which themselves are dependent on complex biochemical processes. Further refinement of model-based approaches to quantify and assess TMPD will be critical to the establishment, testing, and implementation of N-phage cocktails.
The decision to utilize bolus or continuous infusions was principally driven by the need to utilize dosage regimens that could discriminate between exogenous administration and endogenous production of phages. Specifically, absent endogenous production of phage, bolus dosing is expected to produce a single maximum concentration, whereas continuous infusion regimens are expected to produce a steady-state concentration after about 10 h. However, both bolus and continuous infusion regimens will eventually lead to phage concentrations that are entirely driven by endogenous production on susceptible cells in situ. To that end, the HFIM successfully was able to replicate target pharmacokinetic profiles based on previous in vivo studies, as confirmed by PK profile of phage bolus doses within the first hours of treatment. For 2-phage cocktails (e.g., E215 + LUZ19 and PYO2 + LUZ19), duplicate continuous infusion experiments showed reduced bacterial killing as compared to the bolus regimens. Though the true mechanism of antagonism is likely to be complex, assessing this interaction as a single parameter on adsorption creates a foundational model structure that more readily compares different phage strains. Previous studies have shown that bacteria can undergo expressional changes to adaptively resist phage action, which was found to improve description of predator–prey cycling throughout (Jacobs et al., 2016; Jurado et al., 2022).
In vitro PYO2, E215, and LUZ19 parameters of infectivity including burst sizes and latent periods agreed with the model estimates trained using bacteria and phage density patterns in the HFIM (Ceyssens et al., 2011; Forti et al., 2018). Given expected differences in growth conditions between the HFIM and the in vitro methods to quantify phage parameters of infectivity (e.g., burst size, latent period, adsorption rate, etc.), being able to accurately assess burst size and latent time with HFIM data may facilitate development of future phage dosing strategies and target concentrations. Modeling approaches with delayed differential equations (DDE) are an alternative analytical strategy to describe phage activity (Cairns et al., 2009). Initial versions of predator–prey models were explored using the DDE solver within Monolix. However, model run times were significantly longer with increased rates of premature termination (data not shown). Therefore, instability and computational cost made an ODE-based model preferable for this study. Furthermore, underlying stochasticity in the timing and extent of killing and re-growth make optimizing phage therapy challenging. Using nonlinear mixed effects modeling can assess and quantify inter-experimental variability through estimation of random effects on select phage parameters, which can then be used hypothesize future studies through Monte Carlo simulations.
Simulations utilizing the mechanism-based model showed significant influence of daily dose on antibacterial PD. Dose effects were phage strain-specific, with PYO2 paradoxically reducing bacterial counts more significantly with lower daily doses. Given the phage strain-specific nature to dosing, this may indicate that cocktail-based strategies may require optimization of the dose for each phage in the cocktail rather than utilizing a uniform dose. Separately, dose fractionation of identical daily doses across multiple daily dosing showed negligible influence on the extent of bacterial killing. Altogether, these results would support dosing strategies that obtain a phage-specific Cmax after the first dose for an N-phage cocktail, with subsequent doses having a less impactful role in overall treatment efficacy. In the context of TMPD, dosing strategies that achieve a target Cmax after the first dose will be predominately dictated by phage PK from exogenous administration rather than endogenous production, initially.
This study is principally limited by not developing models based on MDR P. aeruginosa isolates, which would be more clinically relevant to the types of isolates phage therapy is typically employed. However, given limited availability of data on the correlation between resistance to small molecule antibiotics and phage infectivity, it is unclear whether MDR-status impacts N-phage cocktail efficacy. Given that most phage action on clinical isolates is typically quantified relative to the phages’ isolation host for efficiency of plating studies, future studies could seek to incorporate these metrics as PD covariates to improve model extrapolation (Smith et al., 2021). Variability in phage-bacterial interactions will influence the performance of a given regimen. Future studies should include assessment of multiple, clinical bacterial isolates to better characterize random effects on phage-specific parameters of infectivity. This will allow better assessment of regimens that incorporate observed variability. Characterization of human PK will ultimately be required to identify dosage regimens. The mathematical model of phage action developed in this study addressed bacterial resistance as being driven by number of virocells; this was done for model parsimony as it minimized the number of parameters needed to address resistance development. Bacterial resistance to phage is largely driven by pre-existing mutations which are selected for after phage exposure, though adaptive resistance is still a relevant concern. Nonetheless, these preliminary data aid in developing a strategy to identify target concentrations. Advancing in vitro, animal-sparing studies, to characterize the PK/PD properties of phages will be critically important to maximizing the therapeutic potential of phages as a class of antimicrobials.
Data availability statement
The raw data supporting the conclusions of this article will be made available by the authors, without undue reservation and after execution of a Data Use Agreement.
Author contributions
NS: Conceptualization, Data curation, Formal analysis, Funding acquisition, Investigation, Methodology, Project administration, Resources, Software, Supervision, Validation, Visualization, Writing – original draft, Writing – review & editing. TN: Conceptualization, Data curation, Investigation, Methodology, Writing – review & editing. WC: Conceptualization, Data curation, Investigation, Methodology, Writing – review & editing. JS: Conceptualization, Data curation, Investigation, Methodology, Writing – review & editing. HS: Data curation, Investigation, Methodology, Writing – review & editing. BH: Data curation, Investigation, Methodology, Writing – review & editing. TL: Conceptualization, Investigation, Methodology, Writing – review & editing. DR: Conceptualization, Data curation, Investigation, Methodology, Writing – original draft, Writing – review & editing.
Funding
The author(s) declare financial support was received for the research, authorship, and/or publication of this article. NS was funded under NIH/NIAID award L30AI164450 during this project.
Conflict of interest
The authors declare that the research was conducted in the absence of any commercial or financial relationships that could be construed as a potential conflict of interest.
The author(s) declared that they were an editorial board member of Frontiers, at the time of submission. This had no impact on the peer review process and the final decision.
Publisher’s note
All claims expressed in this article are solely those of the authors and do not necessarily represent those of their affiliated organizations, or those of the publisher, the editors and the reviewers. Any product that may be evaluated in this article, or claim that may be made by its manufacturer, is not guaranteed or endorsed by the publisher.
Supplementary material
The Supplementary material for this article can be found online at: https://www.frontiersin.org/articles/10.3389/fmicb.2023.1292618/full#supplementary-material
References
Abedon, S. T. (2019). Use of phage therapy to treat long-standing, persistent, or chronic bacterial infections. Adv. Drug Deliv. Rev. 145, 18–39. doi: 10.1016/j.addr.2018.06.018
Abedon, S. T. (2023). Bacteriophage adsorption: likelihood of Virion encounter with Bacteria and other factors affecting rates. Antibiotics 12:40723. doi: 10.3390/antibiotics12040723
Abedon, S. T., Danis-Wlodarczyk, K. M., and Wozniak, D. J. (2021). Phage cocktail development for bacteriophage therapy: toward improving Spectrum of activity breadth and depth. Pharmaceuticals 14:101019. doi: 10.3390/ph14101019
Abedon, S. T., Hyman, P., and Thomas, C. (2003). Experimental examination of bacteriophage latent-period evolution as a response to bacterial availability. Appl. Environ. Microbiol. 69, 7499–7506. doi: 10.1128/aem.69.12.7499-7506.2003
An, G. (2020). Concept of pharmacologic target-mediated drug disposition in large-molecule and small-molecule compounds. J. Clin. Pharmacol. 60, 149–163. doi: 10.1002/jcph.1545
Bichet, M. C., Chin, W. H., Richards, W., Lin, Y.-W., Avellaneda-Franco, L., Hernandez, C. A., et al. (2021). Bacteriophage uptake by mammalian cell layers represents a potential sink that may impact phage therapy. iScience 24:102287. doi: 10.1016/j.isci.2021.102287
Cairns, B. J., Timms, A. R., Jansen, V. A. A., Connerton, I. F., and Payne, R. J. H. (2009). Quantitative models of in vitro bacteriophage–host dynamics and their application to phage therapy. PLoS Pathog. 5:e1000253. doi: 10.1371/journal.ppat.1000253
CDC (2019). Antibiotic resistance threats in the United States 2019, (Ed.) US Department of Health and Human Services (Atlanta: CDC)
Ceyssens, P.-J., Glonti, T., Kropinski, N. M., Lavigne, R., Chanishvili, N., Kulakov, L., et al. (2011). Phenotypic and genotypic variations within a single bacteriophage species. Virol. J. 8:134. doi: 10.1186/1743-422X-8-134
Champagne-Jorgensen, K., Luong, T., Darby, T., and Roach, D. R. (2023). Immunogenicity of bacteriophages. Trends Microbiol. 31, 1058–1071. doi: 10.1016/j.tim.2023.04.008
Chen, L., Todd, R., Kiehlbauch, J., Walters, M., and Kallen, A. (2017). Notes from the field: Pan-resistant New Delhi Metallo-Beta-lactamase-producing Klebsiella pneumoniae - Washoe County, Nevada, 2016. MMWR Morb. Mortal. Wkly Rep. 66:33. doi: 10.15585/mmwr.mm6601a7
d’Herelle, F. (1917). Sur un microbe invisible antagoniste des bacilles dysentériques. C. R. Acad. Sci. 165, 173–175.
Dua, P., Hawkins, E., and van der Graaf, P. H. (2015). A tutorial on target-mediated drug disposition (TMDD) models. CPT Pharmacometrics Syst. Pharmacol. 4, 324–337. doi: 10.1002/psp4.41
Fidler, M., Hallow, M., Wilkins, J., and Wang, W. (2018). RxODE: facilities for simulating from ODE-based models. R package version 0.8.0-9 Ed.
Forti, F., Roach, D. R., Cafora, M., Pasini, M. E., Horner, D. S., Fiscarelli, E. V., et al. (2018). Design of a broad-range bacteriophage cocktail that reduces Pseudomonas aeruginosa biofilms and treats acute infections in two animal models. Antimicrob. Agents Chemother. 62:e02573. doi: 10.1128/AAC.02573-17
Gottig, S., Gruber, T. M., Higgins, P. G., Wachsmuth, M., Seifert, H., and Kempf, V. A. (2014). Detection of pan drug-resistant Acinetobacter baumannii in Germany. J. Antimicrob. Chemother. 69, 2578–2579. doi: 10.1093/jac/dku170
Hatfull, G. F., Dedrick, R. M., and Schooley, R. T. (2022). Phage therapy for antibiotic-resistant bacterial infections. Annu. Rev. Med. 73, 197–211. doi: 10.1146/annurev-med-080219-122208
Jacobs, M., Gregoire, N., Couet, W., and Bulitta, J. B. (2016). Distinguishing antimicrobial models with different resistance mechanisms via population pharmacodynamic modeling. PLoS Comput. Biol. 12:e1004782. doi: 10.1371/journal.pcbi.1004782
Jurado, A., Fernández, L., Rodríguez, A., and García, P. (2022). Understanding the mechanisms that drive phage resistance in staphylococci to prevent phage therapy failure. Viruses 14:51061. doi: 10.3390/v14051061
Kannoly, S., Oken, G., Shadan, J., Musheyev, D., Singh, K., Singh, A., et al. (2022). Single-cell approach reveals intercellular heterogeneity in phage-producing capacities. Microbiol. Spectrum 11:e0266321. doi: 10.1128/spectrum.02663-21
Landersdorfer, C. B., Ly, N. S., Xu, H., Tsuji, B. T., and Bulitta, J. B. (2013). Quantifying subpopulation synergy for antibiotic combinations via mechanism-based modeling and a sequential dosing design. Antimicrob. Agents Chemother. 57, 2343–2351. doi: 10.1128/AAC.00092-13
Lavigne, R., Lecoutere, E., Wagemans, J., Cenens, W., Aertsen, A., Schoofs, L., et al. (2013). A multifaceted study of Pseudomonas aeruginosa shutdown by virulent Podovirus LUZ19. MBio 4:e00061. doi: 10.1128/mbio.00061-00013
Levy, G. (1994). Pharmacologic target-mediated drug disposition. Clin. Pharmacol. Ther. 56, 248–252. doi: 10.1038/clpt.1994.134
Li, C., Shi, T., Sun, Y., and Zhang, Y. (2022). A novel method to create efficient phage cocktails via use of phage-resistant bacteria. Appl. Environ. Microbiol. 88:e0232321. doi: 10.1128/aem.02323-21
Lin, D. M., Koskella, B., and Lin, H. C. (2017). Phage therapy: An alternative to antibiotics in the age of multi-drug resistance. World J. Gastrointest. Pharmacol. Ther. 8, 162–173. doi: 10.4292/wjgpt.v8.i3.162
Lodise, T. P., O’Donnell, J. N., Balevic, S., Liu, X., Gu, K., George, J., et al. (2022). Pharmacokinetics of ceftazidime-avibactam in combination with Aztreonam (COMBINE) in a phase 1, open-label study of healthy adults. Antimicrob. Agents Chemother. 66:e0093622. doi: 10.1128/aac.00936-22
Lodise, T. P., Smith, N. M., O’Donnell, N., Eakin, A. E., Holden, P. N., Boissonneault, K. R., et al. (2020). Determining the optimal dosing of a novel combination regimen of ceftazidime/avibactam with aztreonam against NDM-1-producing enterobacteriaceae using a hollow-fibre infection model. J. Antimicrob. Chemother. 75, 2622–2632. doi: 10.1093/jac/dkaa197
Luong, T., Salabarria, A.-C., Edwards, R. A., and Roach, D. R. (2020). Standardized bacteriophage purification for personalized phage therapy. Nat. Protoc. 15, 2867–2890. doi: 10.1038/s41596-020-0346-0
Magiorakos, A. P., Srinivasan, A., Carey, R. B., Carmeli, Y., Falagas, M. E., Giske, C. G., et al. (2012). Multidrug-resistant, extensively drug-resistant and pandrug-resistant bacteria: an international expert proposal for interim standard definitions for acquired resistance. Clin. Microbiol. Infect. 18, 268–281. doi: 10.1111/j.1469-0691.2011.03570.x
Nabergoj, D., Modic, P., and Podgornik, A. (2018). Effect of bacterial growth rate on bacteriophage population growth rate. Microbiology 7:e00558. doi: 10.1002/mbo3.558
Naknaen, A., Samernate, T., Wannasrichan, W., Surachat, K., Nonejuie, P., and Chaikeeratisak, V. (2023). Combination of genetically diverse pseudomonas phages enhances the cocktail efficiency against bacteria. Sci. Rep. 13:8921. doi: 10.1038/s41598-023-36034-2
Nang, S. C., Lin, Y. W., Petrovic Fabijan, A., Chang, R. Y. K., Rao, G. G., Iredell, J., et al. (2023). Pharmacokinetics/pharmacodynamics of phage therapy: a major hurdle to clinical translation. Clin. Microbiol. Infect. 29, 702–709. doi: 10.1016/j.cmi.2023.01.021
Oechslin, F. (2018). Resistance development to bacteriophages occurring during bacteriophage therapy. Viruses 10:351. doi: 10.3390/v10070351
Petrovic Fabijan, A., Iredell, J., Danis-Wlodarczyk, K., Kebriaei, R., and Abedon, S. T. (2023). Translating phage therapy into the clinic: recent accomplishments but continuing challenges. PLoS Biol. 21:e3002119. doi: 10.1371/journal.pbio.3002119
Smith, N. M., Ang, A., Tan, F., Macias, K., James, S., Sidhu, J., et al. (2021). Interaction of Staphylococcus aureus and Acinetobacter baumannii during in vitro β-lactam exposure. Antimicrob. Agents Chemother. 65:20. doi: 10.1128/aac.02414-20
Smith, N. M., Boissonneault, K. R., Chen, L., Petraitis, V., Petraitiene, R., Tao, X., et al. (2022). Mechanistic insights to combating NDM- and CTX-M-coproducing Klebsiella pneumoniae by targeting Cell Wall synthesis and outer membrane integrity. Antimicrob. Agents Chemother. 66:e0052722. doi: 10.1128/aac.00527-22
Stennett, H. L., Back, C. R., and Race, P. R. (2022). Derivation of a precise and consistent timeline for antibiotic development. Antibiotics 11:91237. doi: 10.3390/antibiotics11091237
Styles, K. M., Brown, A. T., and Sagona, A. P. (2021). A review of using mathematical modeling to improve our understanding of bacteriophage, Bacteria, and eukaryotic interactions. Front. Microbiol. 12:724767. doi: 10.3389/fmicb.2021.724767
Suh, G. A., Lodise, T. P., Tamma, P. D., Knisely, J. M., Alexander, J., Aslam, S., et al. (2022). Considerations for the use of phage therapy in clinical practice. Antimicrob. Agents Chemother. 66:e0207121. doi: 10.1128/aac.02071-21
Summers, W. C. (2012). The strange history of phage therapy. Bacteriophage 2, 130–133. doi: 10.4161/bact.20757
Tacconelli, E., Carrara, E., Savoldi, A., Harbarth, S., Mendelson, M., Monnet, D. L., et al. (2018). Discovery, research, and development of new antibiotics: the WHO priority list of antibiotic-resistant bacteria and tuberculosis. Lancet Infect. Dis. 18, 318–327. doi: 10.1016/s1473-3099(17)30753-3
Uyttebroek, S., Chen, B., Onsea, J., Ruythooren, F., Debaveye, Y., Devolder, D., et al. (2022). Safety and efficacy of phage therapy in difficult-to-treat infections: a systematic review. Lancet Infect. Dis. 22, e208–e220. doi: 10.1016/S1473-3099(21)00612-5
Keywords: Pseudomonas aeruginosa, phage therapy, hollow fiber infection model, pharmacokinetics, pharmacodynamics, mathematical modeling, phage cocktails, treatment optimization
Citation: Smith NM, Nguyen TD, Chin WH, Sanborn JT, de Souza H, Ho BM, Luong T and Roach DR (2023) A mechanism-based pathway toward administering highly active N-phage cocktails. Front. Microbiol. 14:1292618. doi: 10.3389/fmicb.2023.1292618
Edited by:
Heejoon Myung, Hankuk University of Foreign Studies, Republic of KoreaReviewed by:
Stephen Tobias Abedon, The Ohio State University, United StatesShinwon Lee, Pusan National University, Republic of Korea
Copyright © 2023 Smith, Nguyen, Chin, Sanborn, de Souza, Ho, Luong and Roach. This is an open-access article distributed under the terms of the Creative Commons Attribution License (CC BY). The use, distribution or reproduction in other forums is permitted, provided the original author(s) and the copyright owner(s) are credited and that the original publication in this journal is cited, in accordance with accepted academic practice. No use, distribution or reproduction is permitted which does not comply with these terms.
*Correspondence: Nicholas M. Smith, bm1zbWl0aDJAYnVmZmFsby5lZHU=