- 1School of the First Clinical Medical Sciences (School of Information and Engineering), Wenzhou Medical University, Wenzhou, China
- 2Department of Gastrointestinal Surgery, The First Affiliated Hospital of Wenzhou Medical University, Wenzhou, China
Background: The correlation between gut microbiota and infections has garnered significant attention in previous studies; nevertheless, our understanding of the causal relationships and mechanisms between specific microbial species and infections remains limited.
Methods: This study aimed to employ Mendelian randomization (MR) using single-nucleotide polymorphisms (SNPs) and genome-wide association study (GWAS) data of European ancestry to explore the genetic-level relationships between distinct types of gut microbiota and susceptibility to infections. Our analysis encompassed three prevalent infections: intestinal infections, pneumonia, and urinary tract infections, while concurrently examining various types of gut microbiota.
Results: We identified 18 protective gut microbiotas alongside 13 associated with increased infection risk. Particularly noteworthy are certain microbial communities capable of producing butyrate, such as the Ruminococcaceae and Lachnospiraceae families, which exhibited both favorable and unfavorable effects. Additionally, we observed a few certain communities linked to infection susceptibility, including ErysipelotrichaceaeUCG003 (OR = 0.13, 95% CI: 0.054–0.33, p = 1.24E-05), Collinsella (OR = 3.25, 95% CI: 2.00–5.27, p = 1.87E-06), and NB1n (OR = 1.24, 95% CI: 1.09–1.40, p = 1.12E-03).
Conclusion: This study reveals complex relationships between gut microbiota and various infections. Our findings could potentially offer new avenues for exploring prevention and treatment strategies for infectious diseases.
1. Introduction
Infections, such as intestinal infections, pneumonia, and urinary tract infections, pose significant risks to human health, often leading to immune system disorders and even fatal outcomes (Naghavi et al., 2017; National Vital Statistics Reports, 2020). The gut, acknowledged as a complex environment, engages in intricate interactions that substantially contribute to host homeostasis (Clark and Coopersmith, 2007). Concurrently, gut microbiotas are recognized for their pivotal role in governing physiological functions, with numerous studies illustrating their impact on host metabolism and disease progression (Clemente et al., 2012; Gao et al., 2023).
Previous researches have demonstrated that various infectious diseases can arise from disturbances or deficiencies in gut microbiota, while the gut microbiota itself can act as a protective mediator during infections (Schuijt et al., 2016; El-Sayed et al., 2021; Gagnon et al., 2023). However, the results have yet to provide a definitive picture due to the complexity of identifying specific microbiota types and their underlying mechanisms. Randomized controlled trials (RCTs) are widely acknowledged as robust study designs for establishing causal relationships. Nonetheless, logistical challenges and high costs can impede their implementation, and RCTs often offer qualitative rather than quantitative assessments of effects. Mendelian randomization (MR), employing single-nucleotide polymorphisms (SNPs) and genome-wide association studies (GWAS), presents a compelling method for elucidating causality and effect measurement (Emdin et al., 2017). By utilizing genetic variants as substitutes for adjustable elements, MR allows us to infer potential causative links between exposures and outcomes. Through leveraging natural genetic variations, this approach offers a quasi-randomized framework to tackle confounding issues in observational studies. MR has emerged as a valuable tool in epidemiology and genetics, facilitating the exploration of causality.
The aim of this study was to explore the genetic-level correlation between distinct categories of gut microbiota and susceptibility to infectious diseases. Our investigation encompassed three prevalent bacterial infections: intestinal infections, pneumonia, and urinary tract infections. In addition, we conducted a comprehensive analysis of various types of gut microbiotas. Simultaneously, multiple kinds of gut microbiotas were included in the assessment.
2. Materials and methods
2.1. Study design
We adhered to the STROBE-MR guidelines for our study methodology (Additional File 4. Supplementary Table S1) (Skrivankova et al., 2021). Our approach involved a two-sample MR design using summary-level statistics derived from three distinct consortia. To minimize potential bias, both exposure and outcome data were limited to individuals of European ancestry (Sanderson et al., 2022). It’s important to note that all statistics utilized in this study are sourced from publicly available databases with participant consent and ethical approvals, rendering any further permission unnecessary for our study (Figure 1).
2.2. Instrumental variables (IVs)
The criteria for selecting IVs were as follows: (1) Only independent SNPs of gut microbiota were included in the analysis, with a clumping window size of 10,000 kb and an r2 threshold of less than 0.01. (2) To ensure an adequate number of SNPs for exposures, a threshold p-value of 1e-05 was applied (Li et al., 2022). (3) SNPs with F-statistics power below 10, indicative of potential weak instrumental bias, were excluded to mitigate this risk. (4) Additionally, palindromic SNPs were also excluded from consideration.
2.3. Data sources
Instrumental variables (IVs) for a total of 211 intestinal microflora (131genera, 35 families, 20 orders, 16 classes and 9 phyla) involved in this study were accessed from a genome-wide meta-analysis published on MibioGen consortium (Wang et al., 2018; Kurilshikov et al., 2021). There are 18,340 participants from 24 cohorts, mostly have European ancestry (n = 13,266), and 16S rRNA gene sequencing profiles were coordinated (Kurilshikov et al., 2021). Summary statistics from GWAS on infectious diseases were acquired from independent cohorts of European ancestry: UK biobank (Gagliano Taliun et al., 2020) and FinnGen Release (Kurki et al., 2023). The GWAS statistics from UK Biobank were modified for genetic relatedness, sex, birth year, and the first four principal components as covariates using SAIGE (Gagliano Taliun et al., 2020). The GWAS statistics from FinnGen (R9) were also adjusted via SAIGE, using sex, age, genotyping batch and ten PCs as covariates (Kurki et al., 2023). In these two database, cases and controls were defined based on ICD10-codes. For each infection, MR analysis were performed on data from two consortium respectively, mainly based on data from FinnGen.
2.4. Statistical analysis
The F-statistic power was estimated by using the formula: F= / (Wang S. et al., 2022). F-statistic power calculations were performed for all genetic variants related to exposures. We employed various methods, including Inverse Variance Weighted (IVW), MR Egger, Weighted Median, Simple Mode, and Weighted Mode, to analyze the association between gut microbiota and infectious diseases. The primary method was IVW, while MR Egger assessed the presence of pleiotropy with the intercept term. A zero-intercept term indicates horizontal pleiotropy. Cochran’s Q tests were conducted to quantify IV heterogeneity and leave-one-out analyses were employed to identify potential IV heterogeneity.
For exposures displaying significant associations with outcomes, PhenoScanner was utilized to examine each SNP and determine whether the association could be attributed to pleiotropy. To establish a causal association between gut microbiota and infectious diseases, inverse MR analyses were conducted. We applied Bonferroni correction for multiple testing, setting the nominal significance threshold at p < 0.05. Corrected thresholds were defined as follows: genera: 0.05 / 131 (3.81E-04), families: 0.05 / 35 (1.4E-03), orders: 0.05 / 20 (2.5E-03), classes: 0.05 / 16 (3.1E-03), and phyla: 0.05 / 9 (5.56E-03).
All the analyses were conducted in R Studio environment (R version 4.3.1) by using TwoSampleMR package (version 0.5.7). PhenoScanner were available at http://www.phenoscanner.medschl.cam.ac.uk/.
2.5. Post hoc analysis
To further verify the association between gut microbiota and intestinal infections, we performed MR analysis on statistics from UK Biobank. To access the possibility of reverse cause, bi-directional MR analyses were also performed.
3. Results
3.1. Main analysis
After multiple testing correction, there are several statistically significant associations between gut microbiota and infectious diseases (Additional File 1. Supplementary Table S2, Additional File 2. Supplementary Table S2, Additional File 3. Supplementary Table S2).
Our analysis revealed a total of 14 gut microbiotas associations with intestinal infection, encompassing 1 class, 2 families, 8 genera, 2 orders, and 1 phylum. Employing IVW evaluation, we identified significant associations (Figure 2). Notably, class Deltaproteobacteria (OR = 0.026, 95% CI: 0.0046–0.14, p = 2.69E-05), family Enterobacteriaceae (OR = 0.018, 95% CI: 0.0018–0.17, p = 5.37E-04), genus Alloprevotella (OR = 0.17, 95% CI: 0.063–0.43, p = 1.87E-06), genus ErysipelotrichaceaeUCG003 (OR = 0.13, 95% CI: 0.054–0.33, p = 1.24E-05), genus Marvinbryantia (OR = 0.019, 95% CI: 0.027–0.13, p = 6.18E-05), genus Ruminococcaceae NK4A214 group (OR = 0.025, 95% CI: 0.004–0.14, p = 1.89E-06), genus Subdoligranulum (OR = 0.10, 95% CI: 0.036–0.30, p = 3.11E-05), order Desulfovibrionales (OR = 0.024, 95% CI: 0.0042–0.14, p = 2.55E-05), and order Enterobacteriales (OR = 0.024, 95% CI: 0.0042–0.14, p = 2.55E-05) exhibited protective effects against intestinal infection. Conversely, family Victivallaceae (OR = 1.49, 95% CI: 1.17–1.90, p = 1.21E-03), genus Eubacteriumventriosumgroup (OR = 10.40, 95% CI: 3.18–34.06, p = 1.09E-04), genus Gordonibacter (OR = 2.92, 95% CI: 1.79–4.74, p = 1.57E-05), genus Collinsella (OR = 3.25, 95% CI: 2.00–5.27, p = 1.87E-06), and phylum Firmicutes (OR = 3.73, 95% CI: 1.65–8.45, p = 1.60E-03) were associated with an increased risk of intestinal infection.
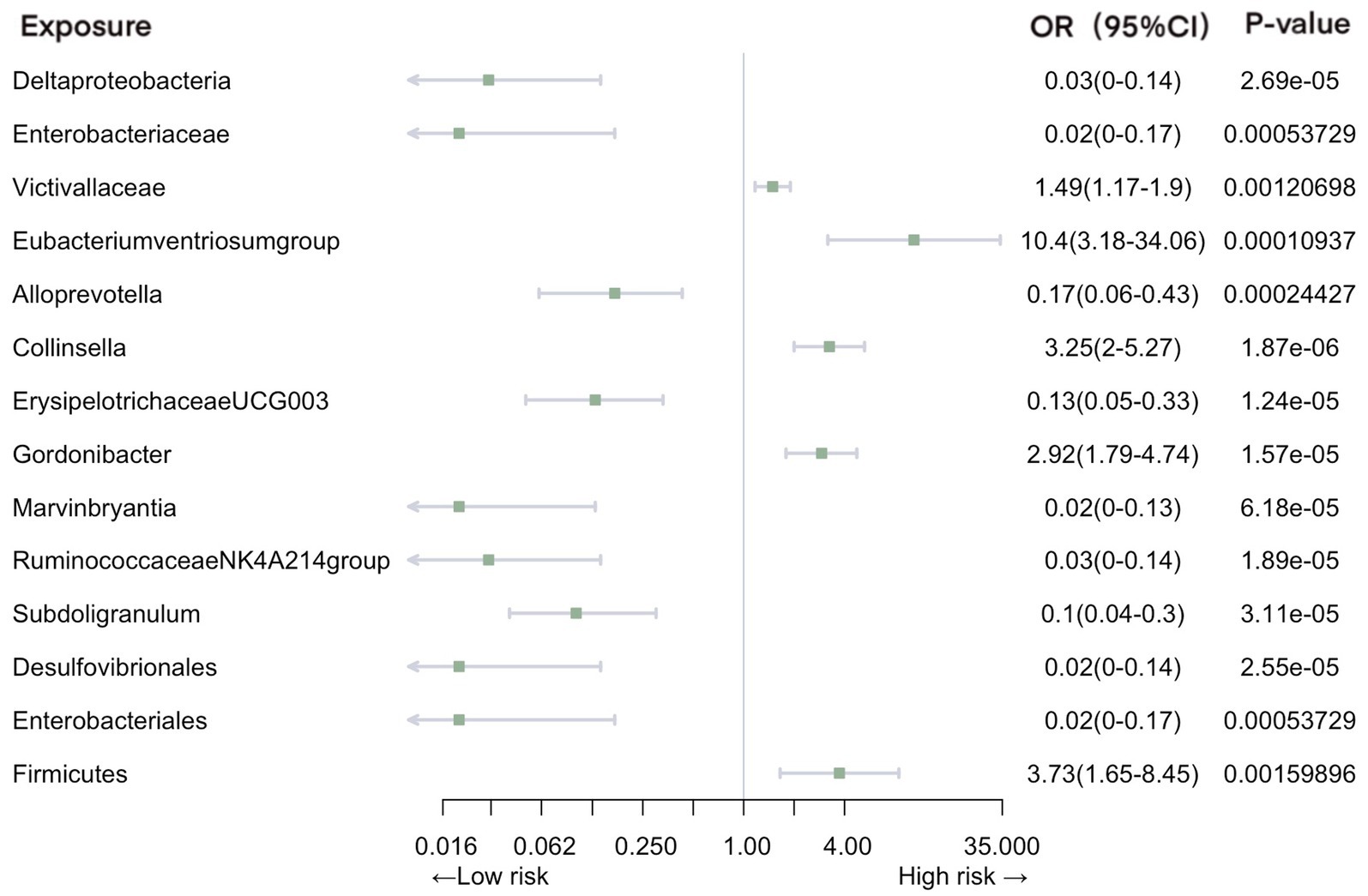
Figure 2. Mendelian randomization analyses of gut microbiota on the risk of intestinal infection. Legend: Forest plot of IVW analyses.
Significant associations with pneumonia were observed in gut microbiota from 2 families, 4 genera, and 2 phyla (Figure 3). Our analysis revealed positive causal effects, from phylum Euryarchaeota (OR = 0.063, 95% CI: 0.010–0.40, p = 3.38E-03), phylum Verrucomicrobia (OR = 0.099, 95% CI: 0.026–0.38, p = 7.85E-04), family FamilyXI (OR = 0.39, 95% CI: 0.23–0.67, p = 5.77E-04), and genus Marvinbryantia (OR = 0.34, 95% CI: 0.19–0.60, p = 2.22E-04). In contrast, family Defluviitaleaceae (OR = 1.41, 95% CI: 1.14–1.74, p = 1.38E-03), genus Blautia (OR = 1.98, 95% CI: 1.41–2.77, p = 8.19E-05), and genus Romboutsia (OR = 7.37, 95% CI: 2.98–18.23, p = 1.56E-05) were associated with negative effects.
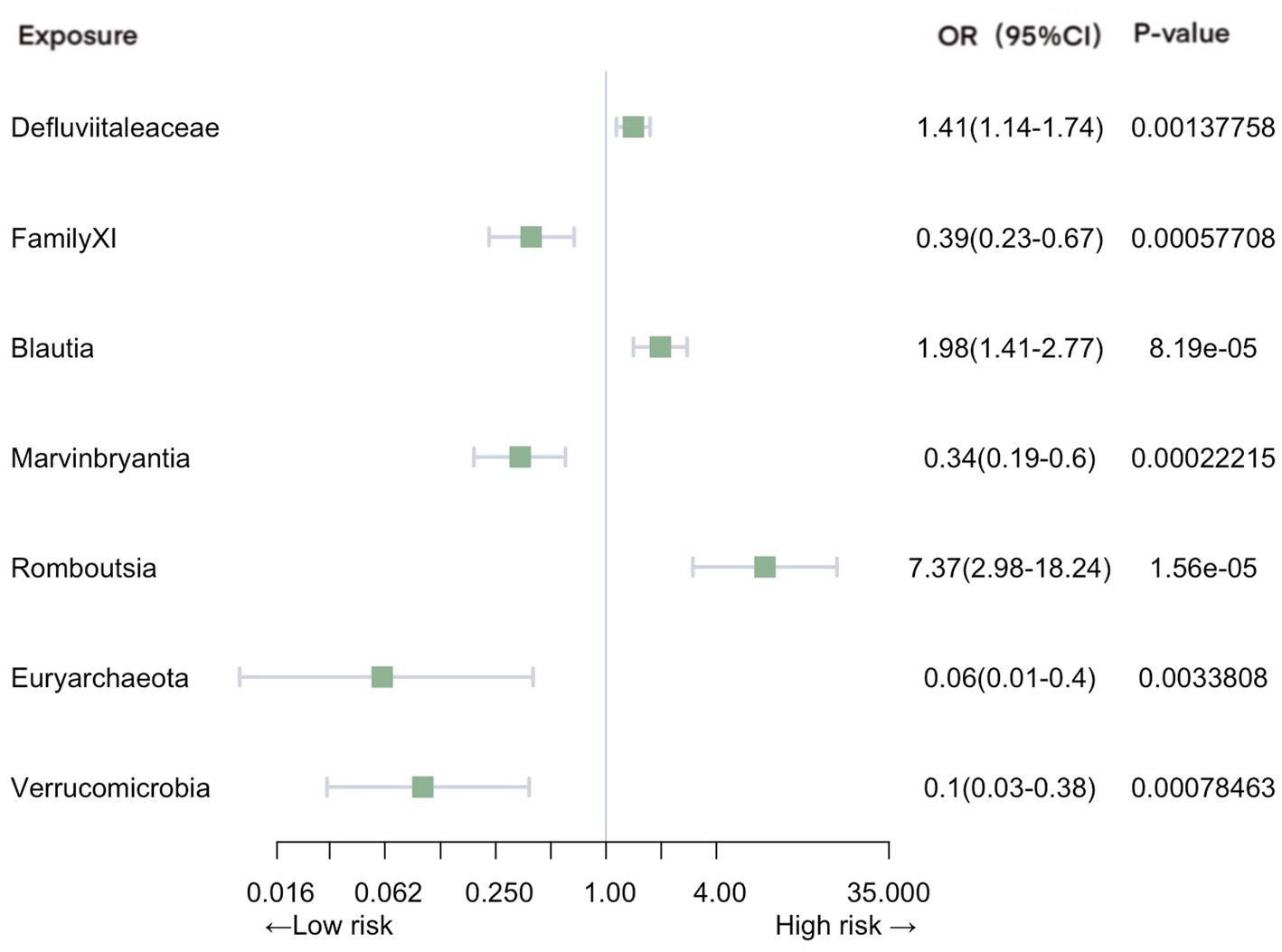
Figure 3. Mendelian randomization analyses of gut microbiota on the risk of pneumonia. Legend: Forest plot of IVW analyses.
In the context of urinary tract infections, our analysis identified several significant associations (Figure 4). Family unknownfamily1000005471 (OR = 0.73, 95% CI: 0.60–0.88, p = 7.80E-04), genus Ruminococcustorquesgroup (OR = 0.64, 95% CI: 0.51–0.79, p = 6.25E-05), genus Ruminococcaceae NK4A214 group (OR = 0.64, 95% CI: 0.54–0.77, p = 8.91E-07), order MollicutesRF9 (OR = 0.73, 95% CI: 0.60–0.88, p = 7.80E-04), and phylum Actinobacteria (OR = 0.70, 95% CI: 0.56–0.88, p = 2.22E-03) were associated with a reduced risk of urinary tract infections. In contrast, family unknownfamily1000006161 (OR = 1.24, 95% CI: 1.09–1.40, p = 1.12E-03), genus RuminococcaceaeUCG003 (OR = 1.43, 95% CI: 1.18–1.75, p = 3.64E-04), genus unknowngenus (OR = 1.31, 95% CI: 1.14–1.50, p = 1.39E-04), order NB1n (OR = 1.24, 95% CI: 1.09–1.40, p = 1.12E-03), and phylum Cyanobacteria (OR = 1.23, 95% CI: 1.07–1.42, p = 4.14E-03) exhibited an increased association with the risk of urinary tract infections.
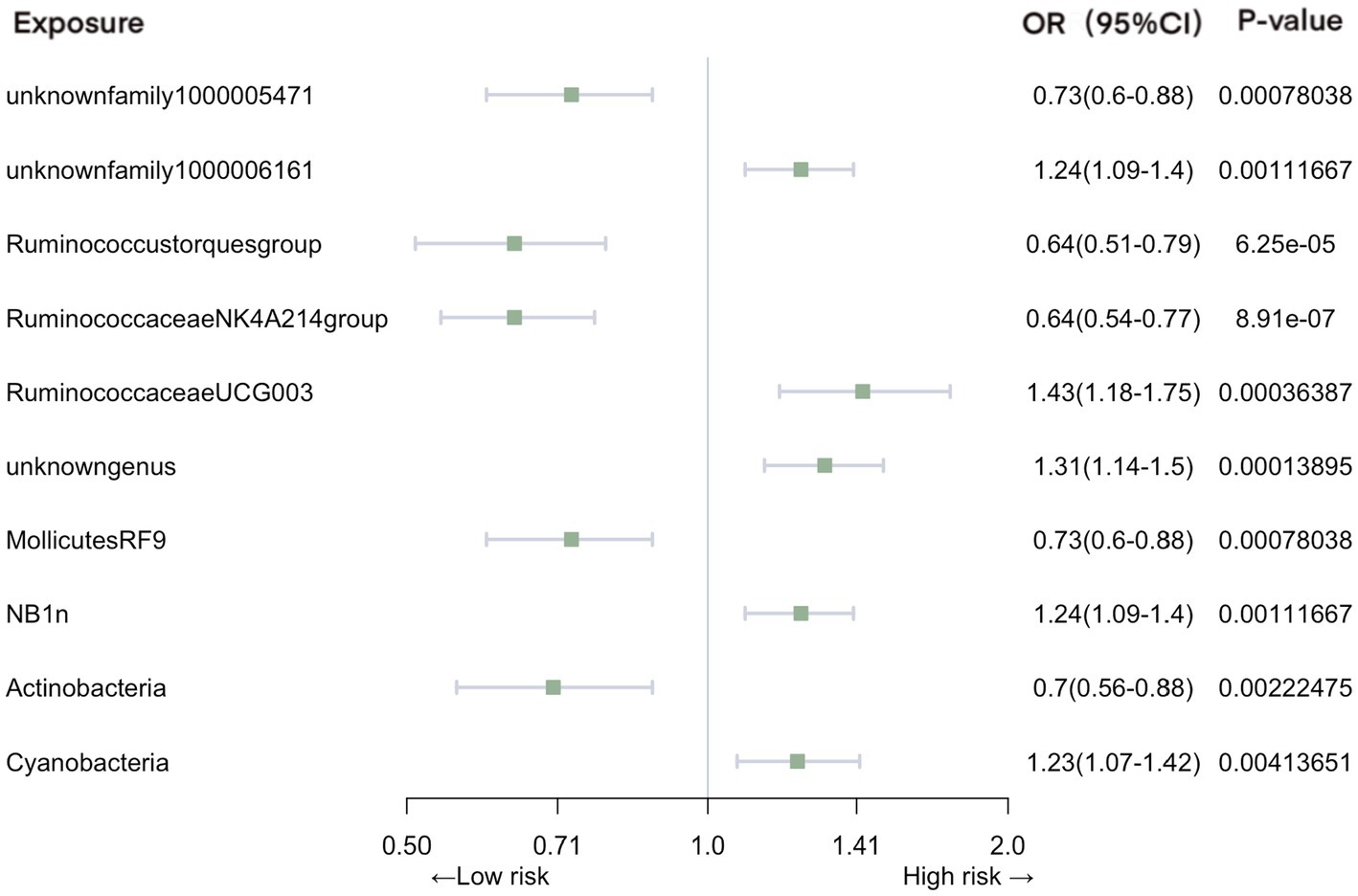
Figure 4. Mendelian randomization analyses of gut microbiota on the risk of urinary tract infection. Legend: Forest plot of IVW analyses.
3.2. Sensitivity analysis
The MR-Egger, weighted median, simple mode, and weighted mode methods were selectively applied exclusively to gut microbiota instances where the number of SNPs were more than 3. This approach was informed by F-statistic power exceeding 10, effectively eliminating weak instrumental bias (Additional File 1. Supplementary Table S1, Additional File 2. Supplementary Table S1, Additional File 3. Supplementary Table S1). All analyses consistently yielded congruent beta directions, substantially reinforcing the robustness of our primary analyses (Additional File 1. Supplementary Table S3, Additional File 2. Supplementary Table S3, Additional File 3. Supplementary Table S3). Conspicuously, no instances of heterogeneity or pleiotropy emerged in our main analyses across the three infectious diseases (Additional File1. Supplementary Table S5, Additional File 2. Supplementary Tables S5, S6, Additional File 3. Supplementary Tables S5, S6). Upon employing PhenoScanner, we identified associations between rs11597285 and rs12251396 and eosinophil percentage in blood. Remarkably, leave-one-out analyses indicated the absence of any individual SNP significantly associated with all three infectious diseases, thereby signifying a dearth of pleiotropic effects (Additional File 1. Supplementary Table S4, Additional File 2. Supplementary Table S4, Additional File 3. Supplementary Table S4).
3.3. Post hoc analysis
Our comprehensive bi-directional MR analyses ruled out any reversed causal relationships between infectious diseases and gut microbiota. To uncover shared effects within specific gut microbiota, we leveraged the robust GWAS statistics from the UK Biobank. Remarkably, our findings highlighted nominal associations: order NB1n exhibited a connection with urinary tract infection, while genus ErysipelotrichaceaeUCG003 displayed a distinctive tie to intestinal infection.
Additionally, our investigation delved into the implications of two distinct SNPs associated with eosinophil counts. The MR analysis covering eosinophilic diseases and intestinal infections yielded results that did not support the presence of any causal association (p = 0.908).
4. Discussion
In this TwoSample MR analysis, we integrated 211 diverse microbiota types from the extensive MiBioGen consortium’s meta-analysis GWAS, along with summary statistics from FinnGen R9 release data for three infectious diseases, aiming to assess their causal relationship. Our findings unveiled a range of protective gut microbiotas alongside a few associated with increased risk. Specific microbial communities proficient in butyrate production, including the families Ruminococcaceae and Lachnospiraceae, manifested a duality of advantageous and adverse effects. The impact of particularly microbial families, including Erysipelotrichaceae and Defluviitaleaceae, was also identified. These results could potentially introduce a novel approach for addressing infectious diseases in human populations.
An increasing number of observational studies has highlighted the link between gut microbiota and infections (Schuijt et al., 2016; Ducarmon et al., 2019; Wang et al., 2019). The gut microbiota plays a pivotal role in bolstering resistance against bacterial infections (Ducarmon et al., 2019), while it can also contribute to the disruption of the gut barrier, potentially leading to infections originating from the gut (Wang et al., 2019). For instance, Erysipelotrichaceae has shown a strong correlation with host lipid metabolism, thus potentially influencing inflammation (Kaakoush, 2015).
A prior investigation revealed that Desulfocibrio desulfuricans could contribute to aneurysm infections (Fujihara et al., 2023). Additionally, another study noted that the order Desulfovibrionales and the family Desulfovibrionaceae were linked to an increased risk of chronic obstructive pulmonary disease (Wei et al., 2023). In the study we conducted, the results indicate that the order Desulfovibrionales and the class Deltaproteobacteria served as safeguards in preventing intestinal infections. One potential explanation is their influence on RNA transcription (Wang W. et al., 2022), subsequently impacting gut protein levels (Langwig et al., 2022; Hu et al., 2023a). However, the exact mechanisms through which these microorganisms influence the development of gut infections require more in-depth investigation. Further research is needed to better understand how these specific bacterial groups contribute to safeguarding the gut against infections and to uncover the underlying molecular and biological processes involved.
Prevotella, recognized as the second most abundant family in the human oral cavity, frequently maintains a high prevalence within gut microbiota (Tett et al., 2021). Some observations suggest that specific Prevotella strains might function as clinical pathobionts, contributing to human diseases by elevating inflammation risk and promoting Th1 immune responses (Larsen, 2017). Conversely, a distinct study found a decrease in Prevotella abundance in pediatric Crohn’s disease (Lewis et al., 2015). Parallelly, our study highlights that the genus Alloprevotella within the Prevotella family exhibits a protective effect. Genus ErysipelotrichaceaeUCG003 as another defensive factor, aligns with an observation that noted lower family Erysipelotrichaceae abundance in patients experiencing Crohn’s recurrence (Dey et al., 2013). Furthermore, the family Enterobacteriaceae within the order Enterobacteriales, known for antibiotic resistance (Nordmann et al., 2012), was found to confer a protective effect. This contrasts with previous research that implicated it in acute knee prosthetic joint infections (Martínez-Pastor et al., 2010).
Members of Lachnospiraceae exhibit anaerobic, fermentative, and chemoorganotrophic characteristics, primarily generating butyrate—an ending product of bacterial metabolism known to regulate gut inflammatory processes. Despite the repeated identification of this family’s taxa for their ability to produce beneficial metabolites for humans, their abundance paradoxically increases in the intestinal lumen of a subset of patients (Vacca et al., 2020). Within this context, our study demonstrates noteworthy correlations: genus Marvinbryantia exhibited a positive effect on both intestinal infections and pneumonia, while genus Blautia—previously reported to associated with beneficial anti-inflammatory effects—displayed a negative influence pneumonia (Vacca et al., 2020; Liu et al., 2021).
A study previously discovered a higher abundance of the Victivallaceae family in patients who developed AIDS (Chen et al., 2021). Correspondingly, our findings paralleled this observation, with Victivallaceae emerging as a risk factor for intestinal infections. Similarly, the Firmicutes phylum also stood out as a risk factor. Despite an earlier study indicating a positive influence of the Eubacterium ventriosum group genus on Inflammatory Bowel Disease (Liu et al., 2022), our research contradicts this by identifying it as a risk factor for intestinal infections. The intricate mechanism through which the Eubacterium ventriosum group affects infections necessitates further investigation. Moreover, the genus Gordonibacter, associated with inflammatory responses in both birds and mammals (Nair et al., 2021), was isolated from a Crohn’s disease patient (Wurdemann et al., 2009) and emerged as a risk factor for intestinal infections in our study. The presence of the genus Collinsella also exerted a negative influence.
The phyla Euryarchaeota and Verrucomicrobia were identified as protective elements against pneumonia. Nonetheless, due to their extensive diversity and limited prior observations, substantial research is warranted to unveil their underlying mechanisms and specific species involved. We identified the Family Defluviitaleaceae as a potential risk factor for pneumonia within our study. Significantly, a recent observation linked the genus Romboutsia to bone marrow necrosis induction, and our research further identifies it as a risk factor for pneumonia (Seviar et al., 2022).
The order MollicutesRF9 and class Actinobacteria served as factors contributing to urinary tract infection protection. Cyanobacteria, known for their significant impact on water quality and ecosystems, have been previously suggested to produce highly neurotoxic cyanotoxins that exert their effects through diverse molecular mechanisms. In our study, we identified Cyanobacteria as a risk factor for urinary tract infections (Sini et al., 2021). Additionally, the lack of order NB1n was also associated with an elevated risk.
Throughout our comprehensive study, we observed a distinct group of gut microbiotas that exhibited a strong correlation with both intestinal and urinary tract infections—namely, the Ruminococcaceae family. As a typical butyrate-producing bacteria in mammals, its influence extends to bacterial metabolism, and a decrease in its abundance has been linked to infection susceptibility (He et al., 2022). RuminococcaceaeUCG003, a key genus, was implicated in chronic insomnia and cardiometabolic diseases, while the Ruminococcustorques group has been found to be markedly prevalent in chronic infections (Jiang et al., 2022; Tran et al., 2023). Additionally, the Ruminococcaceae NK4A214 group has been associated with diarrhea (Gu et al., 2022). Intriguingly, our findings indicated a protective role for the Ruminococcaceae NK4A214 group against both intestinal and urinary tract infections, while Subdoligranulum exhibited a negative impact on intestinal infection. The Ruminococcustorques group demonstrated a protective effect against urinary tract infections, whereas Ruminococcaceae UCG003 increased the risk. We postulate that this family plays a crucial role in mediating the overall gastrointestinal environment through its butyrate production. Nonetheless, the innate mechanism behind this phenomenon remains contentious, necessitating further trials to unravel its complexities.
This significant revelation of two previously unidentified SNPs in our analysis adds a new layer of complexity to our understanding. These unique genetic variations may hold the key to unlocking insights into the gene’s involvement in the innate pathway, shedding light on its potential role in infectious disease susceptibility (Hu et al., 2023b). As we embark on future investigations, delving deeper into the intricate molecular mechanisms behind these associations promises to unveil novel therapeutic targets and advance our knowledge of genetic contributions to disease risk (Wang et al., 2023).
Our study boasts several strengths and limitations. Employing MR analysis, we mitigated confounding risks commonly encountered in observational trials. Furthermore, our study encompassed a larger sample size compared to conventional RCTs. However, certain limitations are present. The GWAS data used for analysis predominantly originated from individuals of European ancestry, potentially limiting the generalizability of our results to other racial groups. Additionally, our microbiota data from MibioGen was categorized into 211 groups, ranging from phylum to genus level, which precluded the identification of specific gut microbiota species. While the causal relationships established in FinnGen data did not replicate in the UK Biobank data, our post hoc analysis revealed just two overlapping microbiotas. We posit that regional differences between the UK and Finland, coupled with distinct phenotype definitions across the two cohorts, might have contributed to this discrepancy. Furthermore, due to our utilization of summary-level data, we were unable to ascertain potential overlap in participants between the exposure and outcome data, introducing the possibility of bias.
5. Conclusion
In this comprehensive exploration of the connection between gut microbiota and infectious diseases, our research has unveiled a diverse landscape of microbial influences on infection susceptibility. Utilizing the power of TwoSample Mendelian randomization, we identified several microbiota types that play pivotal roles in either enhancing or mitigating the risk of infections. Of particular interest is the Ruminococcaceae family, whose subgroups demonstrated both protective and risk-related impacts on infections. These findings underscore the intricate nature of microbiota-infection interactions and hold the promise of informing novel strategies for disease prevention and management. Moving forward, continued investigations are imperative to unravel the underlying mechanisms and clinical implications of these complex relationships.
Data availability statement
The original contributions presented in the study are included in the article/Supplementary material, further inquiries can be directed to the corresponding author.
Ethics statement
Written informed consent was obtained from the individual(s) for the publication of any potentially identifiable images or data included in this article.
Author contributions
BL: Conceptualization, Formal analysis, Methodology, Software, Validation, Visualization, Writing – original draft. JM: Data curation, Validation, Writing – review & editing, Formal analysis. YB: Resources, Validation, Writing – original draft. ZF: Conceptualization, Investigation, Methodology, Project administration, Supervision, Writing – review & editing.
Funding
The author(s) declare that no financial support was received for the research, authorship, and/or publication of this article.
Acknowledgments
The authors thank Jianwei Shuai and Jie Lyu in Wenzhou Institute, UCAS for helpful discussions. Earnest acknowledgement for the participants and investigations of MiBioGen, FinnGen and the UK Biobank study. Thanks to Xu Zhang of Youhe AI and Medicine.
Conflict of interest
The authors declare that the research was conducted in the absence of any commercial or financial relationships that could be construed as a potential conflict of interest.
Publisher’s note
All claims expressed in this article are solely those of the authors and do not necessarily represent those of their affiliated organizations, or those of the publisher, the editors and the reviewers. Any product that may be evaluated in this article, or claim that may be made by its manufacturer, is not guaranteed or endorsed by the publisher.
Supplementary material
The Supplementary material for this article can be found online at: https://www.frontiersin.org/articles/10.3389/fmicb.2023.1284723/full#supplementary-material
References
Chen, Y., Lin, H., Cole, M., Morris, A., Martinson, J., Mckay, H., et al. (2021). Signature changes in gut microbiome are associated with increased susceptibility to HIV-1 infection in MSM. Microbiome 9:237. doi: 10.1186/s40168-021-01168-w
Clark, J. A., and Coopersmith, C. M. (2007). Intestinal crosstalk: a new paradigm for understanding the gut as the “motor” of critical illness. Shock 28, 384–393. doi: 10.1097/shk.0b013e31805569df
Clemente, J. C., Ursell, L. K., Parfrey, L. W., and Knight, R. (2012). The impact of the gut microbiota on human health: an integrative view. Cells 148, 1258–1270. doi: 10.1016/j.cell.2012.01.035
Dey, N., Soergel, D. A., Repo, S., and Brenner, S. E. (2013). Association of gut microbiota with post-operative clinical course in Crohn’s disease. BMC Gastroenterol. 13:131. doi: 10.1186/1471-230X-13-131
Ducarmon, Q. R., Zwittink, R. D., Hornung, B. V. H., Van Schaik, W., Young, V. B., and Kuijper, E. J. (2019). Gut microbiota and colonization resistance against bacterial enteric infection. Microbiol. Mol. Biol. Rev. 83, e00007–e00019. doi: 10.1128/MMBR.00007-19
El-Sayed, A., Aleya, L., and Kamel, M. (2021). Microbiota’s role in health and diseases. Environ. Sci. Pollut. Res. 28, 36967–36983. doi: 10.1007/s11356-021-14593-z
Emdin, C. A., Khera, A. V., and Kathiresan, S. (2017). Mendelian randomization. Jama 318:1925. doi: 10.1001/jama.2017.17219
Fujihara, T., Kimura, K., Matsuo, H., Sada, R. M., Hamaguchi, S., Yamamoto, G., et al. (2023). Infected aneurysm caused by Desulfovibrio desulfuricans infection. Emerg. Infect. Dis. 29, 1680–1681. doi: 10.3201/eid2908.230403
Gagliano Taliun, S. A., Vande Haar, P., Boughton, A. P., Welch, R. P., Taliun, D., Schmidt, E. M., et al. (2020). Exploring and visualizing large-scale genetic associations by using PheWeb. Nat. Genet. 52, 550–552. doi: 10.1038/s41588-020-0622-5
Gagnon, E., Mitchell, P. L., Manikpurage, H. D., Abner, E., Taba, N., Esko, T., et al. (2023). Impact of the gut microbiota and associated metabolites on cardiometabolic traits, chronic diseases and human longevity: a Mendelian randomization study. J. Transl. Med. 21:60. doi: 10.1186/s12967-022-03799-5
Gao, H., Sun, J., Wang, Y., Lu, Y., Liu, L., Zhao, Q., et al. (2023). Predicting metabolite–disease associations based on auto-encoder and non-negative matrix factorization. Brief. Bioinform 24:bbad259. doi: 10.1093/bib/bbad259
Gu, X., Sim, J. X. Y., Lee, W. L., Cui, L., Chan, Y. F. Z., Chang, E. D., et al. (2022). Gut Ruminococcaceae levels at baseline correlate with risk of antibiotic-associated diarrhea. iScience 25:103644. doi: 10.1016/j.isci.2021.103644
He, Z., Ma, Y., Yang, S., Zhang, S., Liu, S., Xiao, J., et al. (2022). Gut microbiota-derived ursodeoxycholic acid from neonatal dairy calves improves intestinal homeostasis and colitis to attenuate extended-spectrum β-lactamase-producing enteroaggregative Escherichia coli infection. Microbiome 10:79. doi: 10.1186/s40168-022-01269-0
Hu, H., Feng, Z., Lin, H., Cheng, J., Lyu, J., Zhang, Y., et al. (2023a). Gene function and cell surface protein association analysis based on single-cell multiomics data. Comput. Biol. Med. 157:106733. doi: 10.1016/j.compbiomed.2023.106733
Hu, H., Feng, Z., Lin, H., Zhao, J., Zhang, Y., Xu, F., et al. (2023b). Modeling and analyzing single-cell multimodal data with deep parametric inference. Brief. Bioinform. 24:bbad005. doi: 10.1093/bib/bbad005
Jiang, Z., Zhuo, L., He, Y., Fu, Y., Shen, L., Xu, F., et al. (2022). The gut microbiota-bile acid axis links the positive association between chronic insomnia and cardiometabolic diseases. Nat. Commun. 13:3002. doi: 10.1038/s41467-022-30712-x
Kaakoush, N. O. (2015). Insights into the role of Erysipelotrichaceae in the human host. Front. Cell. Infect. Microbiol. 5:84. doi: 10.3389/fcimb.2015.00084
Kurilshikov, A., Medina-Gomez, C., Bacigalupe, R., Radjabzadeh, D., Wang, J., Demirkan, A., et al. (2021). Large-scale association analyses identify host factors influencing human gut microbiome composition. Nat. Genet. 53, 156–165. doi: 10.1038/s41588-020-00763-1
Kurki, M. I., Karjalainen, J., Palta, P., Sipilä, T. P., Kristiansson, K., Donner, K. M., et al. (2023). FinnGen provides genetic insights from a well-phenotyped isolated population. Nature 613, 508–518. doi: 10.1038/s41586-022-05473-8
Langwig, M. V., De Anda, V., Dombrowski, N., Seitz, K. W., Rambo, I. M., Greening, C., et al. (2022). Large-scale protein level comparison of Deltaproteobacteria reveals cohesive metabolic groups. ISME J. 16, 307–320. doi: 10.1038/s41396-021-01057-y
Larsen, J. M. (2017). The immune response to Prevotella bacteria in chronic inflammatory disease. Immunology 151, 363–374. doi: 10.1111/imm.12760
Lewis, J. D., Chen, E. Z., Baldassano, R. N., Otley, A. R., Griffiths, A. M., Lee, D., et al. (2015). Inflammation, antibiotics, and diet as environmental stressors of the gut microbiome in pediatric Crohn’s disease. Cell Host Microbe 18, 489–500. doi: 10.1016/j.chom.2015.09.008
Li, P., Wang, H., Guo, L., Gou, X., Chen, G., Lin, D., et al. (2022). Association between gut microbiota and preeclampsia-eclampsia: a two-sample Mendelian randomization study. BMC Med. 20:443. doi: 10.1186/s12916-022-02657-x
Liu, X., Mao, B., Gu, J., Wu, J., Cui, S., Wang, G., et al. (2021). Blautia —a new functional genus with potential probiotic properties? Gut Microbes 13, 1–21. doi: 10.1080/19490976.2021.1875796
Liu, B., Ye, D., Yang, H., Song, J., Sun, X., Mao, Y., et al. (2022). Two-sample Mendelian randomization analysis investigates causal associations between gut microbial genera and inflammatory bowel disease, and specificity causal associations in ulcerative colitis or Crohn’s disease. Front. Immunol. 13:921546. doi: 10.3389/fimmu.2022.921546
Martínez-Pastor, J. C., Vilchez, F., Pitart, C., Sierra, J. M., and Soriano, A. (2010). Antibiotic resistance in orthopaedic surgery: acute knee prosthetic joint infections due to extended-spectrum beta-lactamase (ESBL)-producing Enterobacteriaceae. Eur. J. Clin. Microbiol. Infect. Dis. 29, 1039–1041. doi: 10.1007/s10096-010-0950-y
Naghavi, M., Abajobir, A. A., Abbafati, C., Abbas, K. M., Abd-Allah, F., Abera, S. F., et al. (2017). Global, regional, and national age-sex specific mortality for 264 causes of death, 1980–2016: a systematic analysis for the global burden of disease study 2016. Lancet 390, 1151–1210. doi: 10.1016/S0140-6736(17)32152-9
Nair, D. V. T., Johnson, T. J., Noll, S. L., and Kollanoor Johny, A. (2021). Effect of supplementation of a dairy-originated probiotic bacterium, Propionibacterium freudenreichii subsp. freudenreichii, on the cecal microbiome of turkeys challenged with multidrug-resistant Salmonella Heidelberg. Poult. Sci. 100, 283–295. doi: 10.1016/j.psj.2020.09.091
National Vital Statistics Reports . (2020). Cause-of-death Data From the Fetal Death File, 2015–2017. 69:4.
Nordmann, P., Dortet, L., and Poirel, L. (2012). Carbapenem resistance in Enterobacteriaceae: here is the storm! Trends Mol. Med. 18, 263–272. doi: 10.1016/j.molmed.2012.03.003
Sanderson, E., Glymour, M. M., Holmes, M. V., Kang, H., Morrison, J., Munafò, M. R., et al. (2022). Mendelian randomization. Nat Rev Methods Primers 2:6. doi: 10.1038/s43586-021-00092-5
Schuijt, T. J., Lankelma, J. M., Scicluna, B. P., De Sousa, E., Melo, F., Roelofs, J. J. T. H., et al. (2016). The gut microbiota plays a protective role in the host defence against pneumococcal pneumonia. Gut 65, 575–583. doi: 10.1136/gutjnl-2015-309728
Seviar, D., Nyunt, K., Sayer, C., Wright, D., and Rider, T. (2022). Romboutsia-induced bone marrow necrosis at presentation of acute myeloid leukaemia. Br. J. Haematol. 196:262. doi: 10.1111/bjh.17812
Sini, P., Dang, T. B. C., Fais, M., Galioto, M., Padedda, B. M., Lugliè, A., et al. (2021). Cyanobacteria, cyanotoxins, and neurodegenerative diseases: dangerous liaisons. Int. J. Mol. Sci. 22:8726. doi: 10.3390/ijms22168726
Skrivankova, V. W., Richmond, R. C., Woolf, B. A. R., Davies, N. M., Swanson, S. A., VanderWeele, T. J., et al. (2021). Strengthening the reporting of observational studies in epidemiology using mendelian randomisation (STROBE-MR): explanation and elaboration. BMJ 375:n2233. doi: 10.1136/bmj.n2233
Tett, A., Pasolli, E., Masetti, G., Ercolini, D., and Segata, N. (2021). Prevotella diversity, niches and interactions with the human host. Nat. Rev. Microbiol. 19, 585–599. doi: 10.1038/s41579-021-00559-y
Tran, N. T. D., Chaidee, A., Surapinit, A., Yingklang, M., Roytrakul, S., Charoenlappanit, S., et al. (2023). Chronic Strongyloides stercoralis infection increases presence of the Ruminococcus torques group in the gut and alters the microbial proteome. Sci. Rep. 13:4216. doi: 10.1038/s41598-023-31118-5
Vacca, M., Celano, G., Calabrese, F. M., Portincasa, P., Gobbetti, M., and De Angelis, M. (2020). The controversial role of human gut Lachnospiraceae. Microorganisms 8:573. doi: 10.3390/microorganisms8040573
Wang, J., Kurilshikov, A., Radjabzadeh, D., Turpin, W., Croitoru, K., Bonder, M. J., et al. (2018). Meta-analysis of human genome-microbiome association studies: the MiBioGen consortium initiative. Microbiome 6:101. doi: 10.1186/s40168-018-0479-3
Wang, C., Li, Q., and Ren, J. (2019). Microbiota-immune interaction in the pathogenesis of gut-derived infection. Front. Immunol. 10:1873. doi: 10.3389/fimmu.2019.01873
Wang, S., Qi, L., Wei, H., Jiang, F., and Yan, A. (2022). Smoking behavior might affect allergic rhinitis and vasomotor rhinitis differently: a mendelian randomization appraisal. World Allergy Organ J 15:100630. doi: 10.1016/j.waojou.2022.100630
Wang, T., Sun, J., and Zhao, Q. (2023). Investigating cardiotoxicity related with hERG channel blockers using molecular fingerprints and graph attention mechanism. Comput. Biol. Med. 153:106464. doi: 10.1016/j.compbiomed.2022.106464
Wang, W., Zhang, L., Sun, J., Zhao, Q., and Shuai, J. (2022). Predicting the potential human lncRNA–miRNA interactions based on graph convolution network with conditional random field. Brief. Bioinform. 23:bbac463. doi: 10.1093/bib/bbac463
Wei, Y., Lu, X., and Liu, C. (2023). Gut microbiota and chronic obstructive pulmonary disease: a Mendelian randomization study. Front. Microbiol. 14:1196751. doi: 10.3389/fmicb.2023.1196751
Wurdemann, D., Tindall, B. J., Pukall, R., Lunsdorf, H., Strompl, C., Namuth, T., et al. (2009). Gordonibacter pamelaeae gen. Nov., sp. nov., a new member of the Coriobacteriaceae isolated from a patient with Crohn’s disease, and reclassification of Eggerthella hongkongensis Lau et al. 2006 as Paraeggerthella hongkongensis gen. Nov., comb. nov. Int. J. Syst. Evol. Microbiol. 59, 1405–1415. doi: 10.1099/ijs.0.005900-0
Keywords: Mendelian randomization, gut microbiota, infection susceptibility, genetic relationships, genome-wide association study
Citation: Lyu B, Ma J, Bai Y and Feng Z (2023) Casual effects of gut microbiota on risk of infections: a two-sample Mendelian randomization study. Front. Microbiol. 14:1284723. doi: 10.3389/fmicb.2023.1284723
Edited by:
Qi Zhao, University of Science and Technology Liaoning, ChinaReviewed by:
Shunzhe Song, Dalian Medical University, ChinaSilvia-Giono Cerezo, National Polytechnic Institute (IPN), Mexico
Copyright © 2023 Lyu, Ma, Bai and Feng. This is an open-access article distributed under the terms of the Creative Commons Attribution License (CC BY). The use, distribution or reproduction in other forums is permitted, provided the original author(s) and the copyright owner(s) are credited and that the original publication in this journal is cited, in accordance with accepted academic practice. No use, distribution or reproduction is permitted which does not comply with these terms.
*Correspondence: Zhen Feng, zfeng2019@foxmail.com