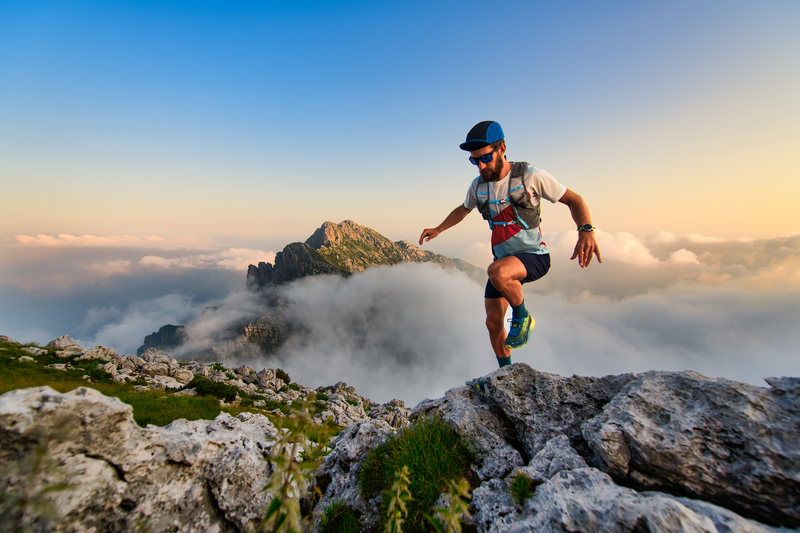
95% of researchers rate our articles as excellent or good
Learn more about the work of our research integrity team to safeguard the quality of each article we publish.
Find out more
ORIGINAL RESEARCH article
Front. Microbiol. , 03 January 2024
Sec. Microorganisms in Vertebrate Digestive Systems
Volume 14 - 2023 | https://doi.org/10.3389/fmicb.2023.1281361
Background: Patients with DN (diabetic nephropathy) show remarkable variations in their gut microbiota composition. However, to date, no study has shown whether a causal relationship exists between gut microbiota composition and DN.
Methods: Here, we performed a two-sample Mendelian randomization (MR) investigation for identifying causal associations of gut microbiota with DN. Gut microbiota genetic data were gathered from the recent genome-wide association study pooled data of the MiBioGen consortium, which included 24 cohorts and 18,340 individuals.
Results: IVW(Inverse variance weighting) revealed that Verrucomicrobia [odds ratio (OR) = 1.390; 95% confidence interval (CI) = 1.10–1.75; p = 0.005], Peptostreptococcaceae (OR = 1.284; 95% CI = 1.03–1.59; p = 0.012), Verrucomicrobiaceae (OR = 1.390; 95% CI = 1.10–1.75; p = 0.005), Akkermansia (OR = 1.390; 95% CI = 1.10–1.75; p = 0.005), Butyricimonas (OR = 1.261; 95% CI = 1.02–1.55; p = 0.031), Catenibacterium (OR = 1.278; 95% CI = 1.02–1.59; p = 0.030).
Conclusion: Two-sample MR analysis identified 12 microbial taxa in gut microbiota (one of which is yet to be officially named) that showed significant causal associations with DN; 8 of these taxa significantly increased the risk of DN, while the remaining 4 taxa (including the one without an official name) reduced the risk of DN. The precise mechanisms influencing the interactions of gut microbiota with DN occurrence remain unclear; hence, additional investigations should be conducted to clarify these mechanisms.
Diabetic nephropathy (DN), a frequently occurring diabetic complication, leads to high mortality in patients with diabetes (Alicic et al., 2017). DN is mainly characterized by glomerulosclerosis caused by microangiopathy due to poor blood glucose control over a long term. DN shows clinical manifestations as proteinuria, hypertension, and incremental decline in renal function (Oshima et al., 2021). Currently, the four main areas of treatment for DN include blood pressure control, glycemic control, reducing cardiovascular disease risk, and suppressing the renin-angiotensin-aldosterone system (Yang et al., 2021). Because DN presents very limited treatment scope currently, it is essential to constantly explore and discover new therapeutic targets.
Recent studies have described many risk factors for DN (Alicic et al., 2017), among which, hyperglycemia and hypertension are the main risk factors (Samsu, 2021). A close link between gut microbiota and DN has been noted. Patients with DN show remarkable differences in their gut microbiota composition; moreover, gut microbiota could influence DN occurrence and development via the gut-kidney axis. Therefore, gut microbiota disruption is considered a risk factor for DN occurrence (Burgess et al., 2019; Cai et al., 2020; Wu et al., 2020; Iatcu et al., 2021; Cai et al., 2022; Deng et al., 2022; Guo et al., 2022; Luo et al., 2022; Lv et al., 2022; Nagase et al., 2022; Ni et al., 2022; Zhang et al., 2022). However, these observational studies did not show a causal relationship of gut microbiota with DN.
Mendelian randomization (MR) is used as an inference analysis for determining causal relationships. It functions on the principle of Mendelian inheritance, and it infers the causal relationship of exposure factors with outcomes through instrumental variables (IVs) as single nucleotide polymorphisms (SNPs) or genetic variants (Davey Smith and Hemani, 2014). MR analysis can determine the underlying biological mechanisms, avoid confounding factor interference, and ensure causal inference accuracy (Birney, 2022). Here, we implemented the MR approach for investigating the causal relationship of gut microbiota composition with DN. We noted that various gut bacterial taxa show a causal relationship with DN.
The exposure factor was 211 bacterial taxa of gut microbiota, and the outcome was DN. We preliminarily screened gut microbiota significantly associated with DN. The detailed MR analysis was performed with the following assumptions: (1) an association between IVs and exposure factors, (2) no relationship between IVs and confounding factors, and (3) influence of IVs on outcomes only through exposure factors. F-statistic of SNPs was calculated to investigate bias existence due to a weak IV (Burgess et al., 2011).
Gut microbiota genetic data were collected using the pooled data of the recent genome-wide association study (GWAS) from the MiBioGen consortium, which included 24 cohorts and 18,340 individuals (URL for data download: https://mibiogen.gcc.rug.nl/). Gut microbiota composition was analyzed according to three variable regions (V1–V2, V3–V4, and V4) of the 16S rRNA gene. Microbiota quantitative trait locus (mbQTL) mapping was applied for identifying genetic variations affecting the relative abundance of microbial taxa (Kurilshikov et al., 2021).
The FinnGen Biobank, which includes data from 213,746 European individuals (210,463 controls and 3,283 cases), was used to retrieve genetic data on diabetic kidney disease. The Integrative Epidemiology Unit OpenGWAS database was searched using “diabetic nephropathy” as the keyword, and two related GWASs were retrieved. Of these, one GWAS [GWAS ID: Finn-b-DM_NEPHROPATHY (more information available at https://gwas.mrcieu.ac.uk)] was selected as it was relatively recent and had a large number of SNPs, with the phenotype as “Diabetic nephropathy.”
The used pooled GWAS data are publicly available. Informed consent as well as institutional ethical approval were received for the original trial. Hence, additional ethical approval was waived off for this study.
Six taxonomic levels were used to classify the 211 bacterial taxa. Among these levels, the genus was the most specific and smallest taxonomic level. IVs were selected according to four criteria: (1) SNPs linked with the gut bacterial taxa were chosen as potential IVs according to p < 5.0 × 10−5 as the significance threshold; (2) to detect independent SNPs, the European genotype of 1,000 genomes was used as the reference genome, and the clumping threshold of linkage disequilibrium was r2 < 0.001, with 10,000 kb as clumping window size; (3) palindromic SNPs were deleted from the curated data; and (4) MR-PRESSO (Mendelian Randomization Pleiotropy RESidual Sum and Outlier) as well as MR-Egger regression were applied for detecting potential pleiotropy and for removing pleiotropy effect by excluding outliers (Bowden et al., 2019). To evaluate the strength with each SNP shows an association with the exposure factor, the F-statistic was determined for each bacterial taxon with the below-mentioned formula, and the IVs’ strength was estimated with the F-statistic.
F-statistic values of ≥10 and < 10 indicated no bias of Ivs (Bowden et al., 2019) and a weak IV that should be eliminated, respectively.
Statistical analysis software used for the data analysis included MR-Presso version 1.0, RStudio version 4.2.1, and TwoSampleMR version 0.5.6. Inverse variance weighting (IVW) was applied as the main statistical test. Weighted median, MR-Egger regression, MR-PRESSO, and simple mode as well as weighted mode methods were additionally used. IVW was chosen as the preferred statistical method because of its higher detection efficiency than the other four MR methods. We selected these statistical tests based on the following logical reasons: (1) IVW is a weighted linear regression model that is mainly used in Mendelian studies of multiple IVs. It assumes that genetic variants are relevant IVs and has strong detection power for causal relationships (Bowden et al., 2017); (2) MR-Egger regression summarizes data and assumes genetic variants to have horizontal gene pleiotropy; in this method, the pleiotropy of IVs is estimated through the intercept generated by weighted linear regression while considering the existence of the intercept (Burgess and Thompson, 2017a). MR-Egger considers some degree of pleiotropy of the IVs; this implies retention of the outcome effect at the exposure effect value of zero (intercept). The MR-Egger intercept term in the MR-Egger intercept test is compared to zero, with a larger variation indicating greater horizontal pleiotropy (Burgess and Thompson, 2017b); (3) MR-PRESSO adds each SNP’s residuals to estimate the magnitude of horizontal pleiotropy, and after adjusting for horizontal pleiotropy, IVW results are derived. The MR-PRESSO global test evaluates IVs’ overall pleiotropy level, while the MR-PRESSO outlier test evaluates aberrant SNPs responsible for the overall pleiotropy level; and (4) the weighted median method adjusts for ineffective IVs’ effects and produces robust estimates even in the presence of 50% of ineffective IVs. We performed leave-one-out analyses (in which the impact of remaining SNPs is calculated after excluding one SNP at a time), which assess how an outlier affects the outcomes. Furthermore, causality direction was determined with a reverse MR analysis.
We initially chose 2,561 SNPs as IVs (all IVs) for the 211 taxa, and finally, 12 taxa were isolated according to p < 0.05 by IVW. Considering the influence of confounding factors, we used PhenoScanner to query the SNPs with the abovementioned positive results. No SNPs were related to the confounding factors.
According to IVW, the presence of class Verrucomicrobia [odds ratio (OR) = 1.390; 95% confidence interval (CI) = 1.10–1.75; p = 0.005], family Peptostreptococcaceae (OR = 1.284; 95% CI = 1.03–1.59; p = 0.012), family Verrucomicrobiaceae (OR = 1.390; 95% CI = 1.10–1.75; p = 0.005), genus Akkermansia (OR = 1.390; 95% CI = 1.10–1.75; p = 0.005), genus Butyricimonas (OR = 1.261; 95% CI = 1.02–1.55; p = 0.031), genus Catenibacterium (OR = 1.278; 95% CI = 1.02–1.59; p = 0.030), genus Marvinbryantia (OR = 1.369; 95% CI = 1.04–1.79; p = 0.022), and order Verrucomicrobiales (OR = 1.390; 95% CI = 1.10–1.75; p = 0.005) in gut microbiota increased risk for DN. However, genus Eubacterium ventriosum group (OR = 0.767; 95% CI = 0.60–0.97; p = 0.030), genus Ruminococcus gauvreauii group (OR = 0.734; 95% CI = 0.56–0.95; p = 0.020), phylum Proteobacteria (OR = 0.750; 95% CI = 0.58–0.97; p = 0.028), and the unknown genus ID 2071 (currently unnamed; OR = 0.805; 95% CI = 0.65–0.99; p = 0.040) in gut microbiota reduced DN risk. As shown in the forest plot (Figure 1).
Figure 1. Forest plot of the effect of gut microbiota on diabetic nephropathy; OR, odds ratio; CI, confidence interval.
As shown in the scatterplot, except Butyricimonas and Catenibacterium, the remaining gut microbiota were all in the same direction. Because the IVW method has the strongest statistical power, our results predominantly relied on the IVW method. Horizontal pleiotropy was estimated primarily with MR-Egger. The MR-PRESSO global and MR-Egger intercept tests exhibited a high likelihood of horizontal pleiotropy (sensitivity analysis; p > 0.05); moreover, in heterogeneity test, all I2 values were < 50%. However, because p value was >0.05, the results were unaffected by bias due to heterogeneity (see Table 1).
Scatterplots (Figure 2) and leave-one-out plots (Figure 3) revealed Verrucomicrobia, Verrucomicrobiaceae, Akkermansia, and Verrucomicrobiales as outliers. No outliers were noted in the MR-PRESSO outlier test. The leave-one-out plots clarified that none of the SNPs largely influenced the effect estimate; this finding indicated that the causality was relatively stable. The funnel plot (Figure 4) showed that the distribution of each IV was symmetric and unbiased.
By conducting a two-sample MR analysis, we evaluated the causal relationship of gut microbiota composition with risk for DN. We observed that Verrucomicrobia, Peptostreptococcaceae, Verrucomicrobiaceae, Akkermansia, Butyricimonas, Catenibacterium, Marvinbryantia, and Verrucomicrobiales increased the risk of diabetic kidney disease, while E. ventriosum group, R. gauvreauii group, Proteobacteria, and unknown genus ID 2071 exhibited a protective effect against DN. This suggests that the gut microbiota significantly influences the development and occurrence of DN.
Interestingly, this study revealed that Akkermansia increased the risk of DN. Numerous studies have demonstrated that Akkermansia is a crucial intestinal probiotic. The lack or decreased abundance Akkermansia was associated with obesity, diabetes, hepatic steatosis, inflammation and tumors (Cani et al., 2022). The mechanism affecting these metabolic diseases was the short-chain fatty acids (SCFAs) produced by Akkermansia bind to GPR43 and PR41, thereby stimulating GLP1 and GLP2 secretion and mucus production and secretion, consequently regulating the glucose metabolism and intestinal function (Cani et al., 2022). In animal experiments, Akkermansia stimulates host cells to produce specific bioactive lipids, stimulate GLP1 and GLP2 secretion, and activates inflammatory pathway and fatty acid oxidation (Cekanaviciute et al., 2017); However, a recent human study revealed that the gut microbiota of patients with chronic kidney disease (CKD) exhibited significantly high levels of five bacterial genera, including Akkermansia, indicating that Akkermansia is an important pathogen associated with CKD progression and may be the key gut microbe causing CKD progression. Other studies have reported that the abundance of Akkermansia is positively correlated with SCr and BUN levels and negatively correlated with eGFR and hemoglobin levels (Ren et al., 2020). which suggests that Akkermansia plays a crucial role in the progression of CKD. Production of uremic toxins may be the mechanism by which Akkermansia affects renal function, which concurs with our findings. Akkermansia is highly associated with metabolic disease significantly as it reduces the risk of diabetes. However, our research showed that Akkermansia increased the risk of DN. We deduced that Akkermansia could reduce the risk of diabetes before the development of diabetes, and if diabetes is already developed, Akkermansia could increase the risk of DN by affecting renal function. However, more clinical studies are needed to confirm this. Our results show that Verrucomicrobia, Verrucomicrobiaceae, and Verrucomicrobiales increased the risk of DN; however, Verrucomicrobia, Verrucomicrobiaceae, and Verrucomicrobiales are in the same bacterial group as Akkermansia and exhibit similar biological functions, suggesting that they probably increase the risk of DN by the same mechanism as Akkermansia. However, further studies are needed to confirm the specific mechanism.
Our study also discovered that Peptostreptococcaceae, Butyricimonas, Catenibacterium, and Marvinbryantia increased the risk of DN. Previous studies have shown that Peptostreptococcaceae and Marvinbryantia aggravate insulin resistance and are risk factors for type 2 diabetes (Chen et al., 2021). Moreover, it has been speculated that Peptostreptococcaceae and Marvinbryantia increase the risk of DN by increasing insulin resistance. The mechanism behind increasing insulin resistance may be that the SCFAs produced by Peptostreptococcaceae and Marvinbryantia affect glucose metabolism. Butyricimonas and Catenibacterium are anaerobic bacteria, and no related studies have been reported; However, it has been speculated that the increased risk of DN may be due to the production of the metabolite trimethylamine (TMA) (Ren et al., 2022); However, further studies are needed to confirm the exact mechanism.
Our study also showed that the E. ventriosum group, R. gauvreauii group, Proteobacteria, and unknown genus ID 2071 reduced the risk of DN. Other studies have shown that the R. gauvreauii group reduces insulin resistance (Chen et al., 2021), and it has been speculated that the R. gauvreauii group reduces the risk of DN by reducing insulin resistance. E. ventriosum group are important gut microbes in the healthy population. Although there has been no concrete report regarding this so far, it has been speculated that this group of microbes produces butyrate hydrochloride, thereby reducing insulin resistance. Additionally, it has been speculated that Proteobacteria may inhibit the growth of other harmful bacteria, reducing the risk of DN (Iatcu et al., 2021); However, the specific mechanism needs to be studied further. Furthermore, the unknown genus ID 2071 is currently unnamed and requires further investigation.
DN stands out as the most prevalent form of chronic kidney disease, primarily marked by damage to kidney function. In clinical practice, several disease, including rare genetic diseases like Fabry disease, Alport syndrome, and Bartter syndrome, can also result in kidney function damage. Fabry disease, an X-linked genetic disorder, arises mainly from mutations in the GLA gene on the chromosome, presenting with symptoms like proteinuria, reduced glomerular filtration rate, and hematuria. Diagnosis hinges on factors like family history, clinical presentation, laboratory tests, histopathological examination, and genetic testing (Chan and Adam, 2018). Alport syndrome exhibits key clinical features such as hematuria, proteinuria, and a progressive decline in kidney function, attributed to genetic mutations in the COL4A3, COL4A4, and COL4A5 genes (Kashtan, 2021). Bartter syndrome, a rare genetic tubulopathy, lacks a standardized diagnostic criterion. Clinical manifestations vary and often lack specificity, with hypokalemia and metabolic alkalosis being fundamental (Mrad et al., 2021). The diagnosis of these rare genetic diseases mainly relies on genetic testing.
The treatment landscape for DN covers a variety of medications, with sodium-glucose cotransporter 2(SGLT-2) inhibitors being prevalent. SGLT-2 inhibitors, a relatively recent class of antidiabetic drugs, have gained widespread clinical use due to their effective glucose-lowering properties. Acting primarily on renal tubules, SGLT-2 inhibitors facilitate the reabsorption of glucose in the primary urine. Extensive studies have indicated the renal protective effects of SGLT-2 inhibitors, although the exact mechanism remains unclear (Fitchett et al., 2019). The findings of this study offer new insights and potential targets for the treatment of DN.
Gut microbiota is an intricate microbial ecosystem existing mainly in the gastrointestinal tract of humans; its composition varies among individuals. It is a key component of gastrointestinal mucosal permeability and regulates the absorption and fermentation of dietary polysaccharides as well as lipid accumulation (Wu et al., 2023). Different microbial communities present in gut microbiota produce different metabolites, including choline, bile acids, neurotransmitters, short-chain fatty acids, small molecules, toxic substances, and inflammatory factors (Schoeler and Caesar, 2019). Based on metabolite production, gut microbiota participates in several physiological processes in various organs, such as signal transduction and energy metabolism (Nishida et al., 2018). These interacting pathways between the gut and the organ, termed the “gut-organ axis,” for example, the axis of gut-kidney, gut-liver, gut-bone, and gut-brain (Ahlawat and Asha, 2021), are highly important in sustaining the functions of several organs. Among these interacting pathways, gut microbiota significantly affects the gut-kidney axis. Intestinal flora affects renal function by synthesizing SCFAs, p-cresyl sulfate, trimethylamine N-oxide (TMAO), indoxyl sulfate, and other compounds (Zhang et al., 2021). SCFAs are essential for the integrity of intestinal epithelial cells and energy balance. They may alleviate hypoxic damage to renal epithelial cells by promoting mitochondrial biogenesis. Indoxyl sulfate and p-cresyl sulfate bind to blood albumin and are secreted by renal tubules. If uremic residual solutes accumulate in the body, they can accelerate glomerulosclerosis and kidney disease progression (Vanholder et al., 2014). As shown by previous studies, TMAO concentrations are 20-fold higher in end-stage renal disease patients than in healthy controls (Zeng et al., 2021). Elevated TMAO levels can lead to renal tubulointerstitial fibrosis and participate in the pathophysiological process of atherosclerosis.
In summary, a two-sample MR analysis revealed 12 taxa of gut microbiota (including one with no official name) to be causally associated with DN. The specific mechanisms responsible for this association, however, remain unclear. Elucidation of these mechanisms responsible for gut microbiota effect on DN will require additional studies.
The datasets presented in this study can be found in online repositories. The names of the repository/repositories and accession number(s) can be found in the article/Supplementary material.
Ethical approval was not required for the study involving humans in accordance with the local legislation and institutional requirements. Written informed consent to participate in this study was not required from the participants or the participants' legal guardians/next of kin in accordance with the national legislation and the institutional requirements.
WY: Data curation, Formal analysis, Funding acquisition, Methodology, Software, Writing – original draft. YG: Software, Writing – original draft. LW: Software, Writing – review & editing. YW: Software, Writing – review & editing. DH: Writing – review & editing.
The author(s) declare financial support was received for the research, authorship, and/or publication of this article. The study was funded by the Youth Research Foundation of Jinshan Hospital of Fudan University (JYQN-LC-202212).
The authors declare that the research was conducted in the absence of any commercial or financial relationships that could be construed as a potential conflict of interest.
All claims expressed in this article are solely those of the authors and do not necessarily represent those of their affiliated organizations, or those of the publisher, the editors and the reviewers. Any product that may be evaluated in this article, or claim that may be made by its manufacturer, is not guaranteed or endorsed by the publisher.
The Supplementary material for this article can be found online at: https://www.frontiersin.org/articles/10.3389/fmicb.2023.1281361/full#supplementary-material
Ahlawat, S., and Asha, S. K. K. (2021). Gut-organ axis: a microbial outreach and networking. Lett. Appl. Microbiol. 72, 636–668. doi: 10.1111/lam.13333
Alicic, R. Z., Rooney, M. T., and Tuttle, K. R. (2017). Diabetic kidney disease: challenges, Progress, and possibilities. Clin. J. Am. Soc. Nephrol. 12, 2032–2045. doi: 10.2215/CJN.11491116
Birney, E. (2022). Mendelian randomization. Cold Spring Harb. Perspect. Med. 12:a041302. doi: 10.1101/cshperspect.a041302
Bowden, J., Del Greco, M. F., Minelli, C., Davey Smith, G., Sheehan, N., and Thompson, J. (2017). A framework for the investigation of pleiotropy in two-sample summary data Mendelian randomization. Stat. Med. 36, 1783–1802. doi: 10.1002/sim.7221
Bowden, J., Del Greco, M. F., Minelli, C., Zhao, Q., Lawlor, D. A., Sheehan, N. A., et al. (2019). Improving the accuracy of two-sample summary-data Mendelian randomization: moving beyond the NOME assumption. Int. J. Epidemiol. 48, 728–742. doi: 10.1093/ije/dyy258
Burgess, S., Davey Smith, G., Davies, N. M., Dudbridge, F., Gill, D., Glymour, M. M., et al. (2019). Guidelines for performing Mendelian randomization investigations. Wellcome Open Res. 4:186. doi: 10.12688/wellcomeopenres.15555.2
Burgess, S., and Thompson, S. G. (2017a). Erratum to: interpreting findings from Mendelian randomization using the MR-egger method. Eur. J. Epidemiol. 32, 391–392. doi: 10.1007/s10654-017-0276-5
Burgess, S., and Thompson, S. G. (2017b). Interpreting findings from Mendelian randomization using the MR-egger method. Eur. J. Epidemiol. 32, 377–389. doi: 10.1007/s10654-017-0255-x
Burgess, S., and Thompson, S. G., Collaboration CCG (2011). Avoiding bias from weak instruments in Mendelian randomization studies. Int. J. Epidemiol. 40, 755–764. doi: 10.1093/ije/dyr036
Cai, K., Ma, Y., Cai, F., Huang, X., Xiao, L., Zhong, C., et al. (2022). Changes of gut microbiota in diabetic nephropathy and its effect on the progression of kidney injury. Endocrine 76, 294–303. doi: 10.1007/s12020-022-03002-1
Cai, T. T., Ye, X. L., Li, R. R., Chen, H., Wang, Y. Y., Yong, H. J., et al. (2020). Resveratrol modulates the gut microbiota and inflammation to protect against diabetic nephropathy in mice. Front. Pharmacol. 11:1249. doi: 10.3389/fphar.2020.01249
Cani, P. D., Depommier, C., Derrien, M., Everard, A., and de Vos, W. M. (2022). Akkermansia muciniphila: paradigm for next-generation beneficial microorganisms. Nat. Rev. Gastroenterol. Hepatol. 19, 625–637. doi: 10.1038/s41575-022-00631-9
Cekanaviciute, E., Yoo, B. B., Runia, T. F., Debelius, J. W., Singh, S., Nelson, C. A., et al. (2017). Gut bacteria from multiple sclerosis patients modulate human T cells and exacerbate symptoms in mouse models. Proc. Natl. Acad. Sci. U. S. A. 114, 10713–10718. doi: 10.1073/pnas.1711235114
Chen, Z., Radjabzadeh, D., Chen, L., Kurilshikov, A., Kavousi, M., Ahmadizar, F., et al. (2021). Association of Insulin Resistance and Type 2 diabetes with gut microbial diversity: a microbiome-wide analysis from population studies. JAMA Netw. Open 4:e2118811. doi: 10.1001/jamanetworkopen.2021.18811
Davey Smith, G., and Hemani, G. (2014). Mendelian randomization: genetic anchors for causal inference in epidemiological studies. Hum. Mol. Genet. 23, R89–R98. doi: 10.1093/hmg/ddu328
Deng, L., Yang, Y., and Xu, G. (2022). Empagliflozin ameliorates type 2 diabetes mellitus-related diabetic nephropathy via altering the gut microbiota. Biochim. Biophys. Acta Mol. Cell Biol. Lipids 1867:159234. doi: 10.1016/j.bbalip.2022.159234
Fitchett, D., Inzucchi, S. E., Cannon, C. P., McGuire, D. K., Scirica, B. M., Johansen, O. E., et al. (2019). Empagliflozin reduced mortality and hospitalization for heart failure across the Spectrum of cardiovascular risk in the EMPA-REG OUTCOME trial. Circulation 139, 1384–1395. doi: 10.1161/CIRCULATIONAHA.118.037778
Guo, Z., Pan, J., Zhu, H., and Chen, Z. Y. (2022). Metabolites of gut microbiota and possible implication in development of diabetes mellitus. J. Agric. Food Chem. 70, 5945–5960. doi: 10.1021/acs.jafc.1c07851
Iatcu, C. O., Steen, A., and Covasa, M. (2021). Gut microbiota and complications of Type-2 diabetes. Nutrients 14:166. doi: 10.3390/nu14010166
Kashtan, C. E. (2021). Alport syndrome: achieving early diagnosis and treatment. Am. J. Kidney Dis. 77, 272–279. doi: 10.1053/j.ajkd.2020.03.026
Kurilshikov, A., Medina-Gomez, C., Bacigalupe, R., Radjabzadeh, D., Wang, J., Demirkan, A., et al. (2021). Large-scale association analyses identify host factors influencing human gut microbiome composition. Nat. Genet. 53, 156–165. doi: 10.1038/s41588-020-00763-1
Luo, L., Luo, J., Cai, Y., Fu, M., Li, W., Shi, L., et al. (2022). Inulin-type fructans change the gut microbiota and prevent the development of diabetic nephropathy. Pharmacol. Res. 183:106367. doi: 10.1016/j.phrs.2022.106367
Lv, Q., Li, Z., Sui, A., Yang, X., Han, Y., and Yao, R. (2022). The role and mechanisms of gut microbiota in diabetic nephropathy, diabetic retinopathy and cardiovascular diseases. Front. Microbiol. 13:977187. doi: 10.3389/fmicb.2022.977187
Mrad, F. C. C., Soares, S. B. M., de Menezes Silva, L. A. W., Dos Anjos Menezes, P. V., and Simões, E. S. A. C. (2021). Bartter's syndrome: clinical findings, genetic causes and therapeutic approach. World J. Pediatr. 17, 31–39. doi: 10.1007/s12519-020-00370-4
Nagase, N., Ikeda, Y., Tsuji, A., Kitagishi, Y., and Matsuda, S. (2022). Efficacy of probiotics on the modulation of gut microbiota in the treatment of diabetic nephropathy. World J. Diabetes 13, 150–160. doi: 10.4239/wjd.v13.i3.150
Ni, Y., Zheng, L., Nan, S., Ke, L., Fu, Z., and Jin, J. (2022). Enterorenal crosstalks in diabetic nephropathy and novel therapeutics targeting the gut microbiota. Acta Biochim. Biophys. Sin. Shanghai 54, 1406–1420. doi: 10.3724/abbs.2022140
Nishida, A., Inoue, R., Inatomi, O., Bamba, S., Naito, Y., and Andoh, A. (2018). Gut microbiota in the pathogenesis of inflammatory bowel disease. Clin. J. Gastroenterol. 11, 1–10. doi: 10.1007/s12328-017-0813-5
Oshima, M., Shimizu, M., Yamanouchi, M., Toyama, T., Hara, A., Furuichi, K., et al. (2021). Trajectories of kidney function in diabetes: a clinicopathological update. Nat. Rev. Nephrol. 17, 740–750. doi: 10.1038/s41581-021-00462-y
Ren, Z., Fan, Y., Li, A., Shen, Q., Wu, J., Ren, L., et al. (2020). Alterations of the human gut microbiome in chronic kidney disease. Adv. Sci. 7:2001936. doi: 10.1002/advs.202001936
Ren, Y., Wang, Z., and Xue, J. (2022). Gut-derived uremic toxin trimethylamine-N-oxide in cardiovascular disease under end-stage renal disease: an injury mechanism and therapeutic target. Sheng Wu Yi Xue Gong Cheng Xue Za Zhi 39, 848–852. doi: 10.7507/1001-5515.202110017
Samsu, N. (2021). Diabetic nephropathy: challenges in pathogenesis, diagnosis, and treatment. Biomed. Res. Int. 2021, 1497449–1497417. doi: 10.1155/2021/1497449
Schoeler, M., and Caesar, R. (2019). Dietary lipids, gut microbiota and lipid metabolism. Rev. Endocr. Metab. Disord. 20, 461–472. doi: 10.1007/s11154-019-09512-0
Vanholder, R., Schepers, E., Pletinck, A., Nagler, E. V., and Glorieux, G. (2014). The uremic toxicity of indoxyl sulfate and p-cresyl sulfate: a systematic review. J. Am. Soc. Nephrol. 25, 1897–1907. doi: 10.1681/ASN.2013101062
Wu, C., Hu, Q., Peng, X., Luo, J., and Zhang, G. (2023). Marine fish protein peptide regulating potassium Oxonate-induced intestinal dysfunction in hyperuricemia rats helps alleviate kidney inflammation. J. Agric. Food Chem. 71, 320–330. doi: 10.1021/acs.jafc.2c04017
Wu, H., Tremaroli, V., Schmidt, C., Lundqvist, A., Olsson, L. M., Kramer, M., et al. (2020). The gut microbiota in prediabetes and diabetes: a population-based cross-sectional study. Cell Metab. 32, 379–390.e3. doi: 10.1016/j.cmet.2020.06.011
Yang, G., Wei, J., Liu, P., Zhang, Q., Tian, Y., Hou, G., et al. (2021). Role of the gut microbiota in type 2 diabetes and related diseases. Metabolism 117:154712. doi: 10.1016/j.metabol.2021.154712
Zeng, Y., Guo, M., Fang, X., Teng, F., Tan, X., Li, X., et al. (2021). Gut microbiota-derived trimethylamine N-oxide and kidney function: a systematic review and Meta-analysis. Adv. Nutr. 12, 1286–1304. doi: 10.1093/advances/nmab010
Zhang, L., Wang, Z., Zhang, X., Zhao, L., Chu, J., Li, H., et al. (2022). Alterations of the gut microbiota in patients with diabetic nephropathy. Microbiol. Spectr. 10:e0032422. doi: 10.1128/spectrum.00324-22
Keywords: diabetic nephropathy, gut microbiota, Mendelian randomization analysis, causal relationship, kidney damage
Citation: Yan W, Ge Y, Wang L, Wang Y and He D (2024) Causal relationship of gut microbiota with diabetic nephropathy: a Mendelian randomization analysis. Front. Microbiol. 14:1281361. doi: 10.3389/fmicb.2023.1281361
Received: 22 August 2023; Accepted: 12 December 2023;
Published: 03 January 2024.
Edited by:
Junling Shi, Northwestern Polytechnical University, ChinaReviewed by:
Cosmin Mihai Vesa, University of Oradea, RomaniaCopyright © 2024 Yan, Ge, Wang, Wang and He. This is an open-access article distributed under the terms of the Creative Commons Attribution License (CC BY). The use, distribution or reproduction in other forums is permitted, provided the original author(s) and the copyright owner(s) are credited and that the original publication in this journal is cited, in accordance with accepted academic practice. No use, distribution or reproduction is permitted which does not comply with these terms.
*Correspondence: Daikun He, ZGFpa3VuX2hlQDEyNi5jb20=
Disclaimer: All claims expressed in this article are solely those of the authors and do not necessarily represent those of their affiliated organizations, or those of the publisher, the editors and the reviewers. Any product that may be evaluated in this article or claim that may be made by its manufacturer is not guaranteed or endorsed by the publisher.
Research integrity at Frontiers
Learn more about the work of our research integrity team to safeguard the quality of each article we publish.