- 1School of Information Engineering, Xuzhou University of Technology, Xuzhou, China
- 2The Affiliated Xuzhou Municipal Hospital of Xuzhou Medical University, Xuzhou, China
- 3Department of Stomatology, Xuzhou First People’s Hospital, Xuzhou, China
Introduction: The oral microbial group typically represents the human body’s highly complex microbial group ecosystem. Oral microorganisms take part in human diseases, including Oral cavity inflammation, mucosal disease, periodontal disease, tooth decay, and oral cancer. On the other hand, oral microbes can also cause endocrine disorders, digestive function, and nerve function disorders, such as diabetes, digestive system diseases, and Alzheimer’s disease. It was noted that the proteins of oral microbes play significant roles in these serious diseases. Having a good knowledge of oral microbes can be helpful in analyzing the procession of related diseases. Moreover, the high-dimensional features and imbalanced data lead to the complexity of oral microbial issues, which can hardly be solved with traditional experimental methods.
Methods: To deal with these challenges, we proposed a novel method, which is oral_voting_transfer, to deal with such classification issues in the field of oral microorganisms. Such a method employed three features to classify the five oral microorganisms, including Streptococcus mutans, Staphylococcus aureus, abiotrophy adjacent, bifidobacterial, and Capnocytophaga. Firstly, we utilized the highly effective model, which successfully classifies the organelle’s proteins and transfers to deal with the oral microorganisms. And then, some classification methods can be treated as the local classifiers in this work. Finally, the results are voting from the transfer classifiers and the voting ones.
Results and discussion: The proposed method achieved the well performances in the five oral microorganisms. The oral_voting_transfer is a standalone tool, and all its source codes are publicly available at https://github.com/baowz12345/voting_transfer.
1 Introduction
Microorganisms are a prominent type of biological group that are small and closely related to humans, including bacteria, viruses, fungi, small native creatures, and microalga (Xia et al., 2010; Ngounou Wetie et al., 2014). The efforts of biology have a history of more than a century. At the end of the 19th and early 20th century, Pasteur, Mechnikov, and Corch founded microbiology and micro-ecology (Zou et al., 2016). Since the discovery of Microorganisms, scholars have been committed to constructing and researching microbiological disciplines (Shen et al., 2007). The concept of Microbiota has become clearer with the in-depth study of microorganisms (Zhang et al., 2012). Microbial groups refer to the general name of all microorganisms in a specific time and specific growth environments, and the sum of the gene sequence contained in the microbial group contained in a specific time and specific growth environment is the microbiome group (Brohee and van Helden, 2006). EDERBERG first proposed the concept of the Human Microbiome in 2001, referring to all microorganisms living on the human body, including symbiotic and pathogenic bacteria, and the genetic sum of the corresponding microbial groups planted by the human body was called the human microbial group (Wu et al., 2009; Shen et al., 2019; Zhang and Zou, 2020).
The oral cavity is a complex micro-ecological environment. There are many types of oral microorganisms (De Las Rivas and Fontanillo, 2010; Wang et al., 2023). The oral microbial group is a typical representative of the human body’s highly complex microbial group ecosystem (Ngo et al., 1994; Whisstock and Lesk, 2003). Most of the oral microorganisms can be related to each other to form a complex community in the form of biofilm. It has strong physical resistance and biological resistance. When the host is balanced, the oral microorganisms can prevent the invasion of exogenous pathogenic bacteria. When changes in environmental changes or other oral conditions occur, such as personal oral hygiene care, They may become pathogenic microorganisms, which induce a variety of chronic infectious diseases of the oral cavity, including dental dentalism, pulp root disease, periodontal disease, wisdom tooth crown weekly Inflammation, mandibular osteomyelitis, etc., seriously endanger oral health (Godzik et al., 1995; Whisstock and Lesk, 2003; Peng et al., 2017; Awais et al., 2019). Studies have shown that the changes in oral microbial groups and the imbalance of oral micro-ecology are closely related to the occurrence and development of oral diseases such as oral cancer and diabetes (Chatterjee et al., 2011; You et al., 2013; Meng et al., 2023).
The protein function site prediction methods have been developed utilizing artificial intelligence techniques based on available data (Sato et al., 1994; Wei et al., 2017). It was noted that the traditional machine learning ones extract effective features manually to represent function site information, such as Shannon entropy, relative entropy, information gain, protein disordered property, and the average cumulative hydrophobicity (Schwikowski et al., 2000). With the development of artificial intelligence, deep-learning approaches with convolutional neural networks (CNNs) have been proposed to process raw sequence data without the need for manual feature extraction (Wenya et al., 2022). TAGPPI extracts multi-dimensional features by employing 1D convolution operation on protein sequences and graph learning method on contact maps constructed from AlphaFold (Song et al., 2022). Nguyen-Vo et al. (2022) proposed iPromoter-Seqvec, which is an efficient computational model to predict TATA and non-TATA promoters in human and mouse genomes using bidirectional long short-term memory neural networks in combination with sequence-embedded features extracted from input sequences. Robson et al. (2022) utilized ProSE to collect the custom heterogeneous systolic arrays and special functions. Several efforts have proposed various approaches for classification function sites in the field of bioinformatics and computational biology (Coates and Hall, 2003; Vazquez et al., 2003; Kim and Subramaniam, 2006; Free et al., 2009; Zhang et al., 2012). Wang et al. (2017) proposed a two-dimensional attention mechanism to classify the protein phosphorylation sites (Yang et al., 2020). In the same year, Wang et al. (2017) proposed a densely connected convolutional neuron network to identify the same PTM sites (Gao et al., 2023). Ahmed et al. employed a stacked long short-term memory recurrent network to deal with the function sites’ classification issue (You et al., 2017). Despite the immense potential of deep learning, a major challenge in developing classification methods based on this technology is the need for huge scale of computational resources. Therefore, the transfer learning has the ability to save the computing resources and improve computational reusability.
In this work, we proposed a novel method, which is oral_voting_transfer, to deal with such classification issues in the field of oral microorganisms. In detail, we utilized the highly effective model, which successfully classifies the organelles proteins and transfer to deal with five microorganisms, including streptococcus mutans, staphylococcus aureus, abiotrophia adjacens, bifidobacterial and capnocytophaga. It was noted that the transfer classifiers can hardly meet the need. On the other hand, some classification methods can be treated as the local classifiers in this work. And then, the final results are voting from the transfer classifiers and the voting ones. It was pointed out that the voting method employed three deep learning features, including TAGPPI, SeqVec, and ProSE. The detailed steps demonstrated in the following sections.
2 Materials and methods
2.1 Dataset
The identified datasets for this experiment was obtained from a protein-specific database website, which provides corresponding sequences and feature information for oral microorganisms (Guo et al., 2008; Torrey and Shavlik, 2010; Niu et al., 2020). In this experiment, we conducted qualitative analysis on the active sites and binding sites of five oral microorganisms. The details of each species are shown in Table 1.
The active proteins are the identified proteins with active sites in their sequences. And the non-active proteins are the proteins without any active sites. The terms, including binding and non-binding proteins, follow a similar procession in this work. Meanwhile, it is noted that there are three employed features in this work, including SeqVec, ProSE, and TAGPPI. Therefore, there are 30 identified datasets have been constructed.
2.2 Feature extraction based on deep learning datasets
2.2.1 TAGPPI
This feature can be treated as a neural network algorithm called TAGPPI (TextCNN-based Approach for Gene-Protein Interaction Prediction), an end-to-end deep learning approach in computational proteomics. The feature utilizes a one-dimensional convolutional neural network (CNN) known as TextCNN, commonly used in natural language processing (NLP) tasks to capture the relationships between adjacent words in a sentence for classification purposes (Koike and Takagi, 2004).
The SCNN (Stacked Convolutional Neural Network) model in TAGPPI consists of six alternately stacked CNN and max-pooling layers (You et al., 2015b). The three combination layers are then stacked together. The architecture diagram of the sequence feature extraction and learning module.
The first step of this feature is to represent the entire amino acid sequence as a matrix, denoted as X ∈ RL*M, where M represents the number of selected feature dimensions (set to 1024), and L represents the maximum length of the given protein sequence (set to 1200) to ensure a fixed size of the output vector from the TextCNN module. For sequences shorter than 1200, zero padding is applied to the matrix before input. Therefore, the input matrix is denoted as X ∈ R1200*1024.
The SCNN model consists of a stack of six-layers CNN and max-pooling layers. In the first convolutional layer, 128 filters of length 3 are applied to the input matrix 1191×128, resulting in feature maps of 399×128. This is followed by a max-pooling layer with a pooling stride of 3, resulting in feature maps of the output matrix. These steps are repeated twice, resulting in a feature map of size 1×128. We have modified it to have six layers stacked, so the final obtained dataset is denoted as ℝn×768, with a fixed dimension of 768 for all data.
With the above-mentioned processions, the input protein sequences are transformed into a matrix representation, which is then processed by the stacked CNN and max-pooling layers to extract sequential features. The output feature maps undergo further stacking and dimension reduction, resulting in a final dataset ℝn×768 with a fixed dimensionality of 768.
2.2.2 SeqVec
This feature can be regarded as a self-attentive deep learning algorithm based on ELMO for protein sequence generation. Since the ordered arrangement of amino acids in proteins follows a specific pattern, combining CharCNN and two LSTM layers is used to capture the surrounding words and context. The CharCNN converts all characters in a word into a vector space through an embedding layer and runs multiple CNNs to fix the vector dimensions (Dudoit et al., 2002; Lee et al., 2005). The first bidirectional LSTM takes the output of CharCNN as input, and the second bidirectional LSTM takes the output of the first LSTM as input.
The second layer, CharCNN, maps each amino acid to a fixed-length latent space of 1024 dimensions. The third layer, LSTM_1, introduces context-specific information by sequentially processing the protein sequence. The fourth layer, LSTM_2, directly operates on the output of LSTM_1 and attempts to predict all previous words in the protein sequence. During training, the forward and backward channels are independently optimized to reduce information leakage. Each layer ultimately generates a 1024-dimensional vector. The method add these vectors together to form a 1024-dimensional feature vector. Finally, the results of each protein sequence are combined to form the dataset ℝn×1024, where the dimension of each data point is fixed at 1024. The variable n represents the number of identified datasets for the five oral microorganisms in this work.
2.2.3 ProSE (protein sequence embeddings)
This feature is based on language modeling. Due to the influence of evolutionary pressures, protein functional properties and other characteristics are often maintained or enhanced in specific functions. These pressures are reflected in the distribution of amino acids in natural protein sequences (Bradford and Westhead, 2005; Díaz-Uriarte and de Andrés, 2006; Pashaei et al., 2016; Cui et al., 2012). The ProSE feature has been successfully utilized in several bioinformatics issues. Therefore, the ProSE feature effectively represents protein sequences as continuous vectors, combining the advantages of self-supervised learning on large-scale sequence corpora and structure-supervised learning on smaller sequence sets.
2.3 Transfer learning
With the development of bioinformatics and computational biology, several artificial intelligence have been utilized in this field. Transfer learning is a novel machine learning approach. The field of data mining and machine learning has been successfully utilized in several areas (Chen and Liu, 2005; Li et al., 2012; Wang et al., 2018; Romero-Molina et al., 2019). First of all, the model utilized to classify the golgi proteins. Then, these ten classification models are transferred to classify the oral microorganisms’ proteins. Lastly, these models construct the voting transfer model to classify the Oral microorganisms’ proteins. The model structure is demonstrated in Figure 1.
2.4 Voting transfer learning model
In this work, we proposed oral_voting_transfer, which is the voting transfer learning method to deal with the classification of oral microbiology protein function sites. During this model, we employed several basic classifiers, including Gaussian Process Classification, Gaussian Naive Bayes, Bernoulli Naive Bayes, Decision Trees, Bagging meta-estimator, Random Forest, AdaBoost, Gradient Tree Boosting and Multilayer Perceptron (You et al., 2015a). In the following section, some typical methods can be shown.
2.4.1 Random forest
The random forest algorithm was proposed by Arlot and Genuer (2016), and it is a very efficient general classification and regression technique (Sun et al., 2017). The method combines multiple probabilistic decision trees, aggregates average predictions, and works well in environments where the number of variables greatly exceeds the number of observations. Furthermore, it is general enough to be applied to large-scale problems, can be easily adapted to different specialized learning tasks, and provides multiple correlation metrics.
The algorithm works by growing M random trees as follows. Cases in the original dataset are randomly selected before building each tree. In each cell of each tree, splitting is performed by maximizing the basket criterion (see below) in m directions chosen uniformly and randomly from the original p directions. When the points of each cell are smaller than the node size, the construction of the tree ends. For any query point x∈X, each regression tree predicts the average of the Yi for which the corresponding Xi.
2.4.2 AdaBoost
Boosting is an important method in blended learning. It provides new methods and ideas for designing learning algorithms. The idea behind it is to combine several weak learners that are slightly more accurate than random guessing to create arbitrarily accurate strong predictors (Zhang et al., 2019). This has important implications for situations where directly developing powerful learning algorithms is very difficult. As a meta-learning framework, Boosting can be applied to almost all popular machine learning algorithms to further improve prediction accuracy. Because of this, it is widely used and has had a major impact on the field of machine learning. Especially among all boosting algorithms, AdaBoost is the most successful representative, and is rated as one of the top ten algorithms in the field of data mining (Saha et al., 2014). Since AdaBoost was proposed, many excellent researchers have conducted research on various theoretical topics, laid a solid theoretical foundation for AdaBoost, and made contributions to the successful implementation of AdaBoost. The success of AdaBoost is not only because it is an effective learning algorithm, but also related to the following points.
2.5 Performances evaluation
Accuracy (Acc), sensitivity (Sn), specificity (Sp), and F1-score were used to evaluate the performance of the prediction system. were obtained using the formulae in Eqs 1–4, provided hereafter (Liu et al., 2018).
The performance of the classifier is evaluated using various metrics such as True Positives (TP), False Positives (FP), True Negatives (TN) and False Negatives (FN). Sensitivity (Sn) and specificity (Sp) measure the proportion of correct predictions for positive and negative samples, respectively. The F1 score reflects the strength of the model. The higher the score, the greater the resistance (Eq. 5). MCC is used to measure the strength of the linear relationship between two variables. The area under the ROC curve (AUC) was also used as an evaluation metric, with higher values indicating better model performance.
3 Results and discussions
3.1 The performances of transfer model
This is a machine learning-based voting ensemble strategy aimed at addressing the sub-classification task of Golgi apparatus proteins. This model can be employed as the transfer classification model. The Table 2 show performances the different ratios between positive and negative samples.
The results show that the proposed machine learning-based voting ensemble model for Golgi apparatus sub-classification achieves a stable accuracy of over 95% on the test set across different ratios of positive and negative samples in the training set. This indicates that the model has a high accuracy and generalizability. Overall, based on the results, the MVVM (Multi-Voting Machine Model) performs exceptionally well for Golgi apparatus sub-classification.
3.2 The performances of voting transfer learning
From Figures 2, 3 and Table 3, it can be observed that for Streptococcus mutans, the choice of feature extraction method has a limited impact on the accuracy of the active site prediction. However, the three different feature extraction methods still influence the model. The ProSE tends to provide better balance in terms of accuracy and positive sample coverage. SeqVec tends to overfit the positive samples and has a poorer balance. TAGPPI performs similarly to ProSE and has better coverage of positive samples but sacrifices some balance. Therefore, depending on the specific objective, ProSE is better when the balance is emphasized, while TAGPPI is preferable when emphasizing positive sample coverage.
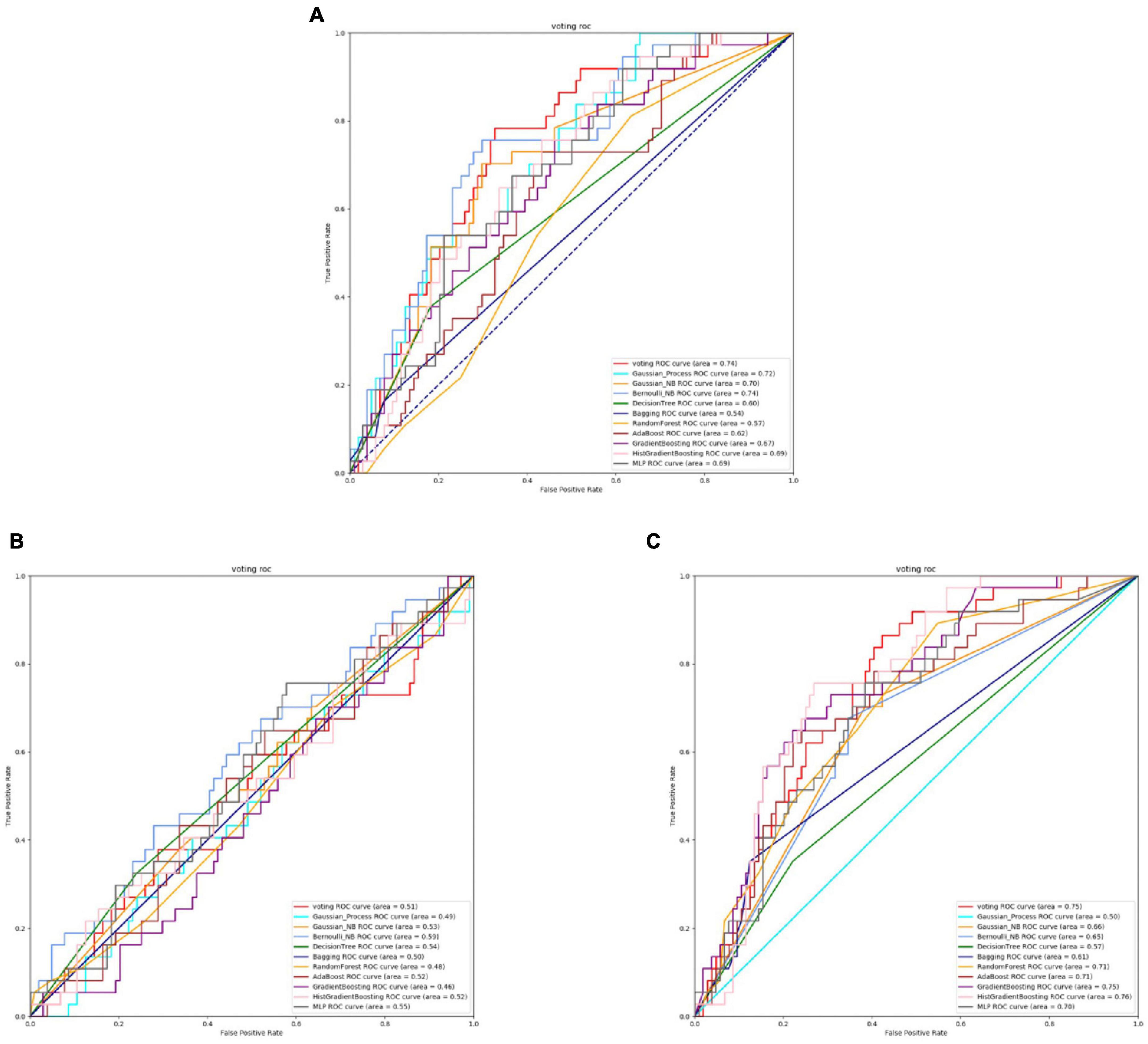
Figure 2. The ROC of Streptococcus mutans active sites. (A) Is the ROC curve of 10-fold cross validation with TAGPPI. (B) Is the ROC curve of 10-fold cross validation with SeqVec. (C) Is the ROC curve of 10-fold cross validation with ProSE.
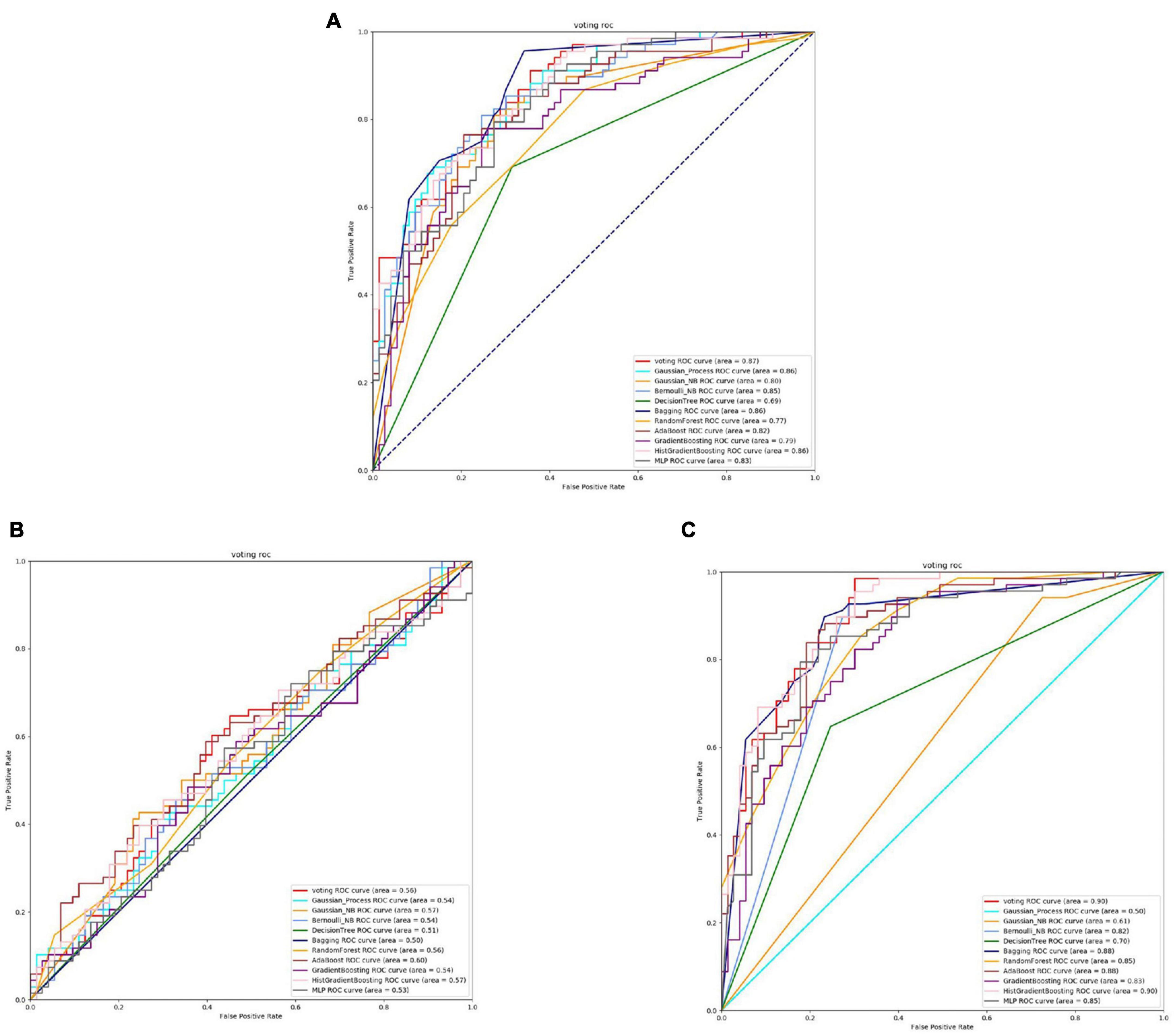
Figure 3. The ROC of Streptococcus mutans binding sites. (A) Is the ROC curve of 10-fold cross validation with TAGPPI. (B) Is the ROC curve of 10-fold cross validation with SeqVec. (C) Is the ROC curve of 10-fold cross validation with ProSE.
Transfer learning offers notable advantages in terms of stability and coverage when it comes to identifying the active site and binding site of Streptococcus mutans. Moreover, the coverage of positive samples mostly exceeds 80%. According to the model accuracy for the binding site, it can be observed that the ProSE feature extraction method outperforms the other two methods. It has higher recall and F1 scores compared to the other methods. Therefore, ProSE is a better choice for identifying the binding site in Streptococcus mutans.
It is necessary to evaluate the specific feature selection for each microorganism using performance metrics and evaluate and optimize the overall transferred model. Below is the performance table for the Voting transfer learning models with three feature extraction methods for the active site.
According to the analysis of the table and ROC curves, it can be observed that the overall accuracy of the Gaussian Process and Bernoulli NB classification models is relatively low. This indicates that Bayesian classification models may not perform well for protein data where features are correlated. To further optimize the transfer learning, we can consider transforming the models or reducing the weight of the Bayesian model in the voting strategy.
By comparing the effect data of the classifier model in the voting and the effect of the transfer learning voting model, the accuracy and stability of the model have been optimized to a certain extent through the voting strategy. The table shows that TAGPPI, SeqVec, and ProSE have accuracy rates of 73.05, 73.76, and 73.05% respectively for the three features. The accuracy has been improved compared to the classification model in the voting process, indicating better stability and generalization than the trained model. On the other hand, the bagging algorithm and Decision Tree classification model consistently maintain high accuracy. We can consider increasing their weights appropriately to leverage their strong performance in the voting ensemble. The following is the scoring table for each model in the Voting transfer learning model of the Binding site in three feature extractions.
Based on the ROC curves, it can be observed that the green curve representing the Bernoulli NB classification model performs relatively poorly compared to the other models, and the difference is quite significant. The SeqVec feature extraction method, compared to the other two, shows overall lower training performance and is not suitable for feature extraction in binding sites.
In summary, the ProSE feature extraction method is more suitable for Streptococcus pneumoniae as it can extract sufficient features to ensure high accuracy in model training and prediction.
From the Figures 4, 5 and Table 4, it can be seen that for Staphylococcus aureus, overall, the three feature extraction methods show excellent training results. However, SeqVec has the lowest accuracy, indicating that it is not suitable for Staphylococcus aureus. ProSE and TAGPPI, feature extraction methods, perform equally well, with accuracy above 90%, and exhibit good balance and coverage. Both methods can be applied to feature extraction in Staphylococcus aureus.
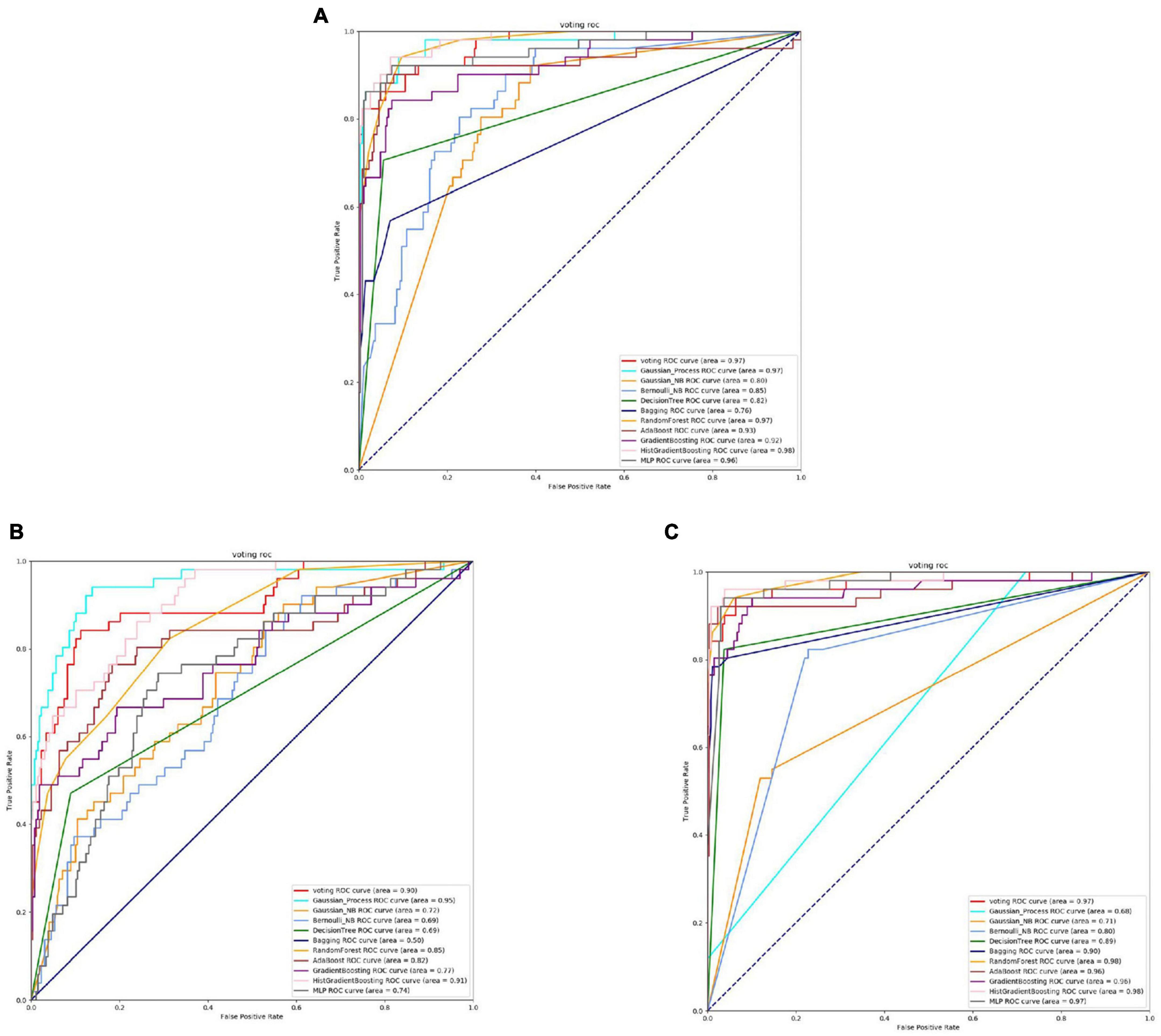
Figure 4. The ROC of Staphylococcus aureus active sites. (A) Is the ROC curve of 10-fold cross validation with TAGPPI. (B) Is the ROC curve of 10-fold cross validation with SeqVec. (C) Is the ROC curve of 10-fold cross validation with ProSE.
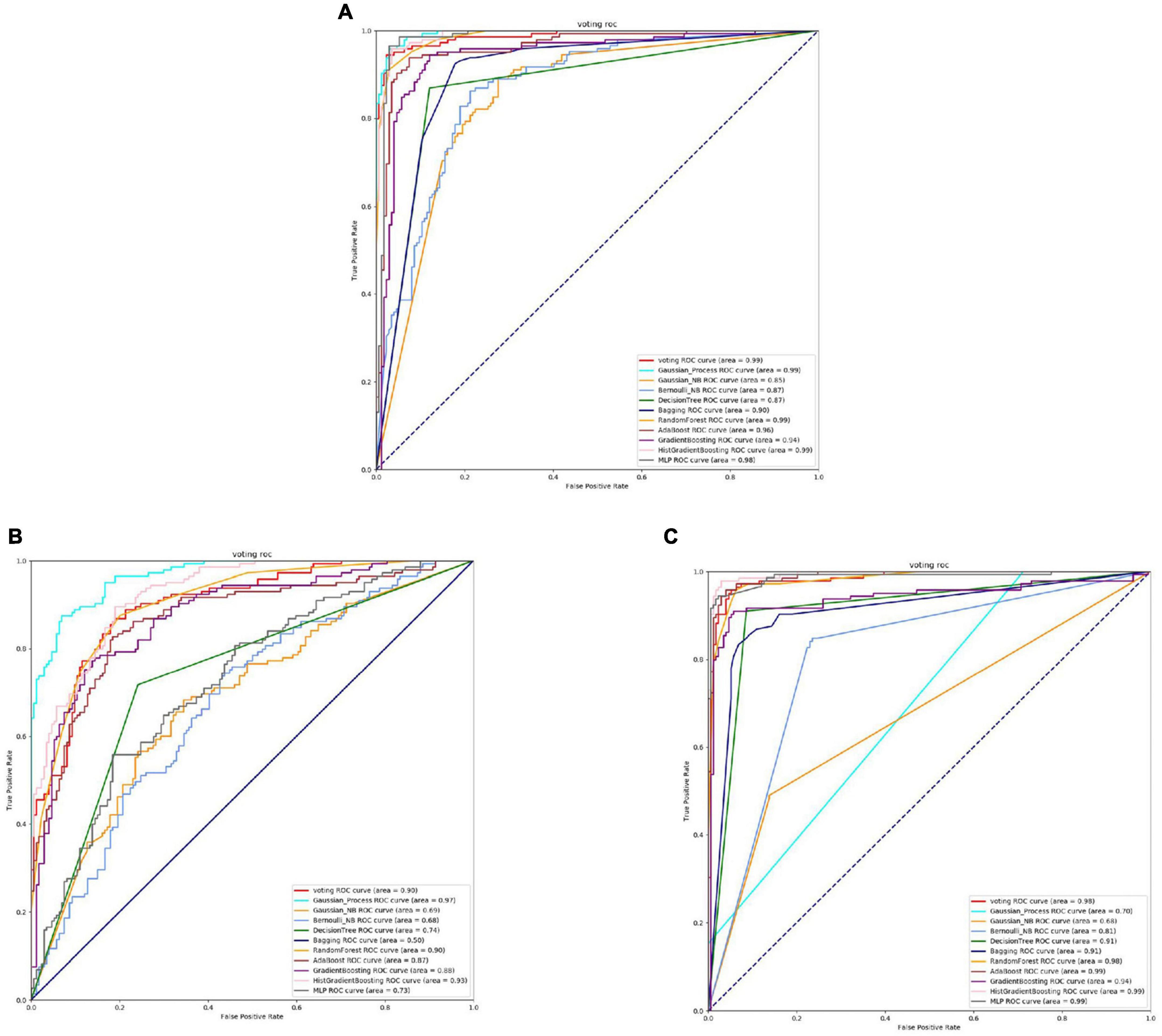
Figure 5. The ROC of Staphylococcus aureus binding sites. (A) Is the ROC curve of 10-fold cross validation with TAGPPI. (B) Is the ROC curve of 10-fold cross validation with SeqVec. (C) Is the ROC curve of 10-fold cross validation with ProSE.
The following is the score table of each model in the Voting transfer learning model of the active site in three feature extractions.
It can be observed that Gaussian Process and Neighbors have lower performance, while the models trained on the datasets extracted by TAGPPI consistently achieve high accuracy. This indicates that TAGPPI feature extraction is superior. Among the ProSE models, three of them show lower performance, and the ROC curve of SeqVec is more scattered. This suggests that the training of the models using these feature extraction methods is mostly based on weak learners. However, after applying the voting strategy, the accuracy is significantly improved, which validates the effectiveness of the transfer learning models. Through the evaluation indicators of each model, it can be observed that the recognition accuracy of Staphylococcus aureus’s active sites is relatively high. However, the indicators for model generalization and stability are inconsistent. After applying Voting Transfer Learning, the final model not only maintains an accuracy rate of more than 88% but also improves its stability. The following is the scoring table for each model in the Voting transfer learning model of the Binding site in three feature extractions.
The table data reveals that the accuracy of the model has improved after applying Voting Transfer Learning. Both the SN and SP index values are above 90%, indicating enhanced stability of the model through transfer learning.
The models trained on datasets extracted by TAGPPI consistently maintain high accuracy, indicating that TAGPPI feature extraction is superior. Among the ProSE models, three of them show lower performance, and the ROC curve of SeqVec is more scattered. This suggests that the training of these feature extraction models mostly relies on weak learners. However, after applying the voting strategy, the accuracy is significantly improved. This demonstrates that the transfer learning model is appropriate and effective.
Based on the Figures 6, 7 and Table 5, we can observe that for Abiotrophia adjacens, overall, the three feature extraction methods yield good training results. However, there are some differences among them. SeqVec has the poorest balance, but it achieves 100% coverage of positive samples, indicating overfitting issues. Based on the data, we can conclude that TAGPPI is the optimal choice for both active site and binding site prediction in Abiotrophia adjacens, considering the model’s generalization, balance, and coverage.
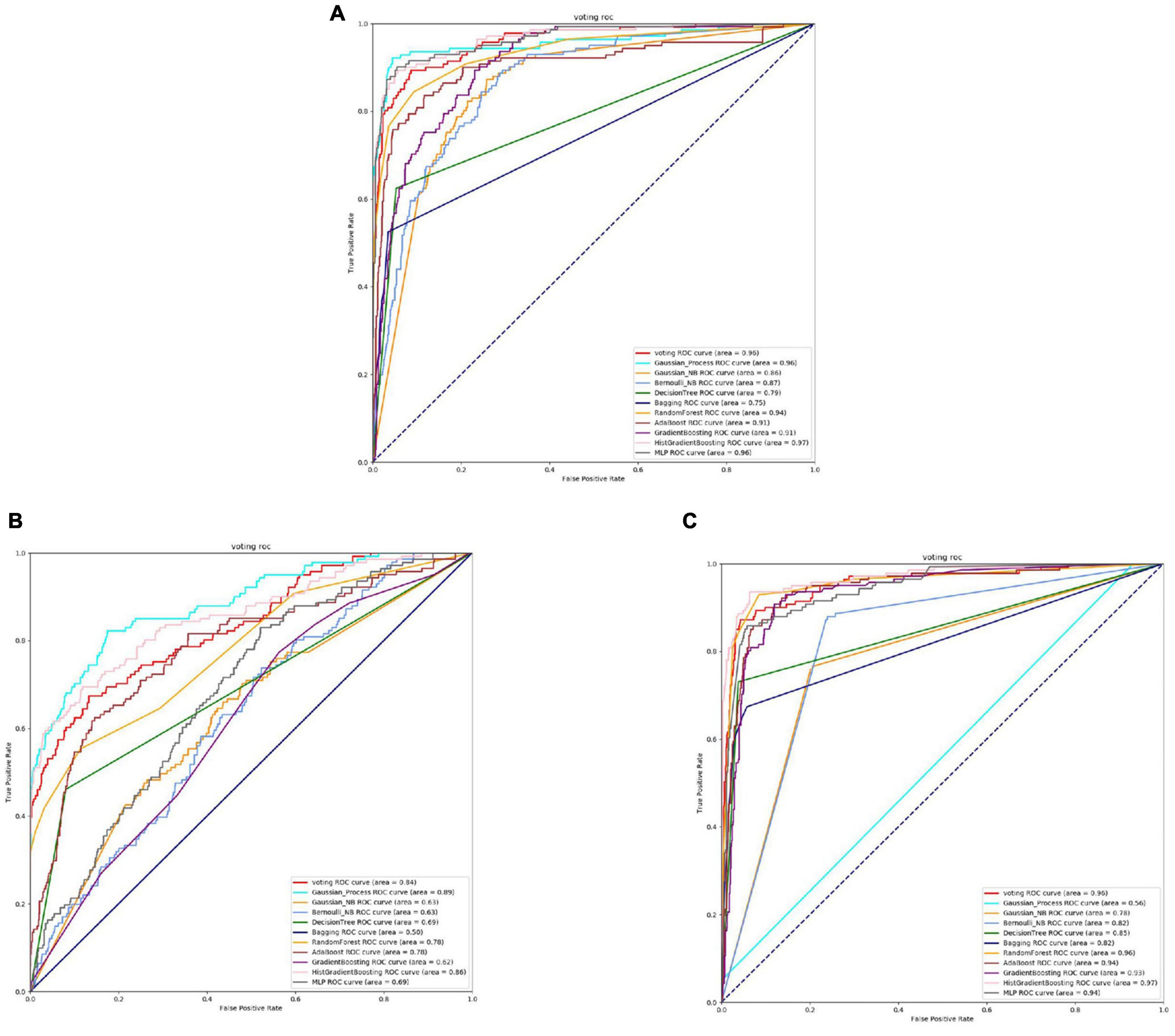
Figure 6. The ROC of Abiotrophia adjacens active sites. (A) Is the ROC curve of 10-fold cross validation with TAGPPI. (B) Is the ROC curve of 10-fold cross validation with SeqVec. (C) Is the ROC curve of 10-fold cross validation with ProSE.
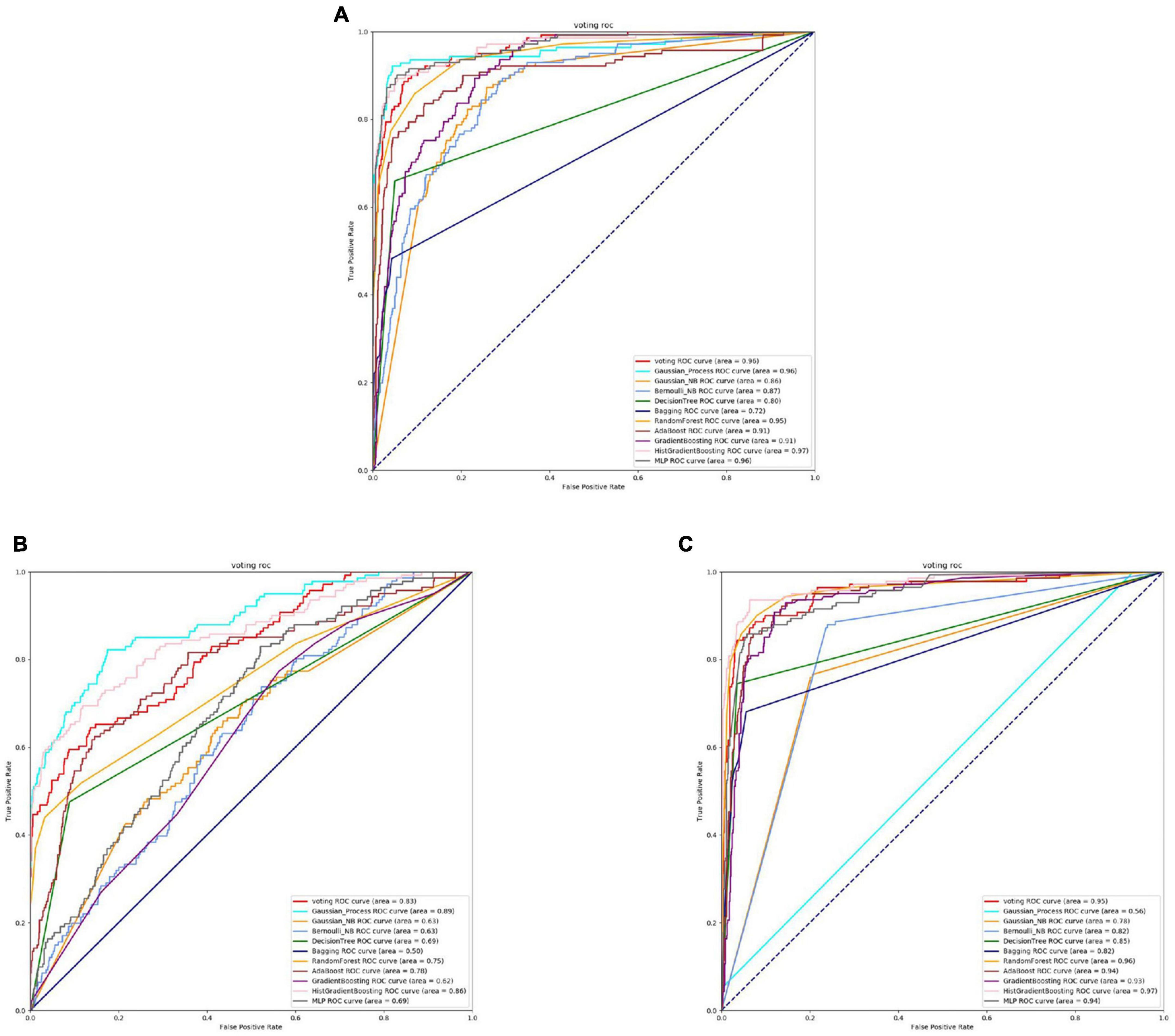
Figure 7. The ROC of Abiotrophia adiacens binding sites. (A) Is the ROC curve of 10-fold cross validation with TAGPPI. (B) Is the ROC curve of 10-fold cross validation with SeqVec. (C) Is the ROC curve of 10-fold cross validation with ProSE.
The following is the score table of each model in the Voting transfer learning model of the active site in three feature extractions.
It can be observed that the Radius Neighbors Classifier performs significantly worse than the other models, and Bernoulli Naive Bayes shows poor performance across all three feature extraction methods, indicating that it is a weak classifier. The ROC curve for SeqVec is more scattered, suggesting that SeqVec does not provide comprehensive feature extraction for the active site of Abiotrophia adjacens.
The following is the scoring table for each model in the Voting transfer learning model of the Binding site in three feature extractions.
Based on the ROC curves and the model performance tables, it can be observed that the active site and binding site exhibit high similarity. This suggests that Abiotrophia adjacens likely possesses both the active site and binding site in most cases, leading to the similarity in the dataset. This hypothesis needs to be verified, and a correlation analysis can be conducted between the active site and binding site of Abiotrophia adjacens to confirm this assumption. If the hypothesis is confirmed, the model can use the known sequence of one site to predict the presence of the other site with a high probability, thus improving the efficiency and accuracy of the model training.
Based on Figures 8, 9 and Table 6, it can be observed that for Bifidobacterium, overall, the three feature extraction methods yield good results in model training, but there are differences among them. SeqVec has the poorest balance and the lowest accuracy, sacrificing generalization to achieve a high positive sample coverage rate, which makes it relatively inferior compared to the other two methods.
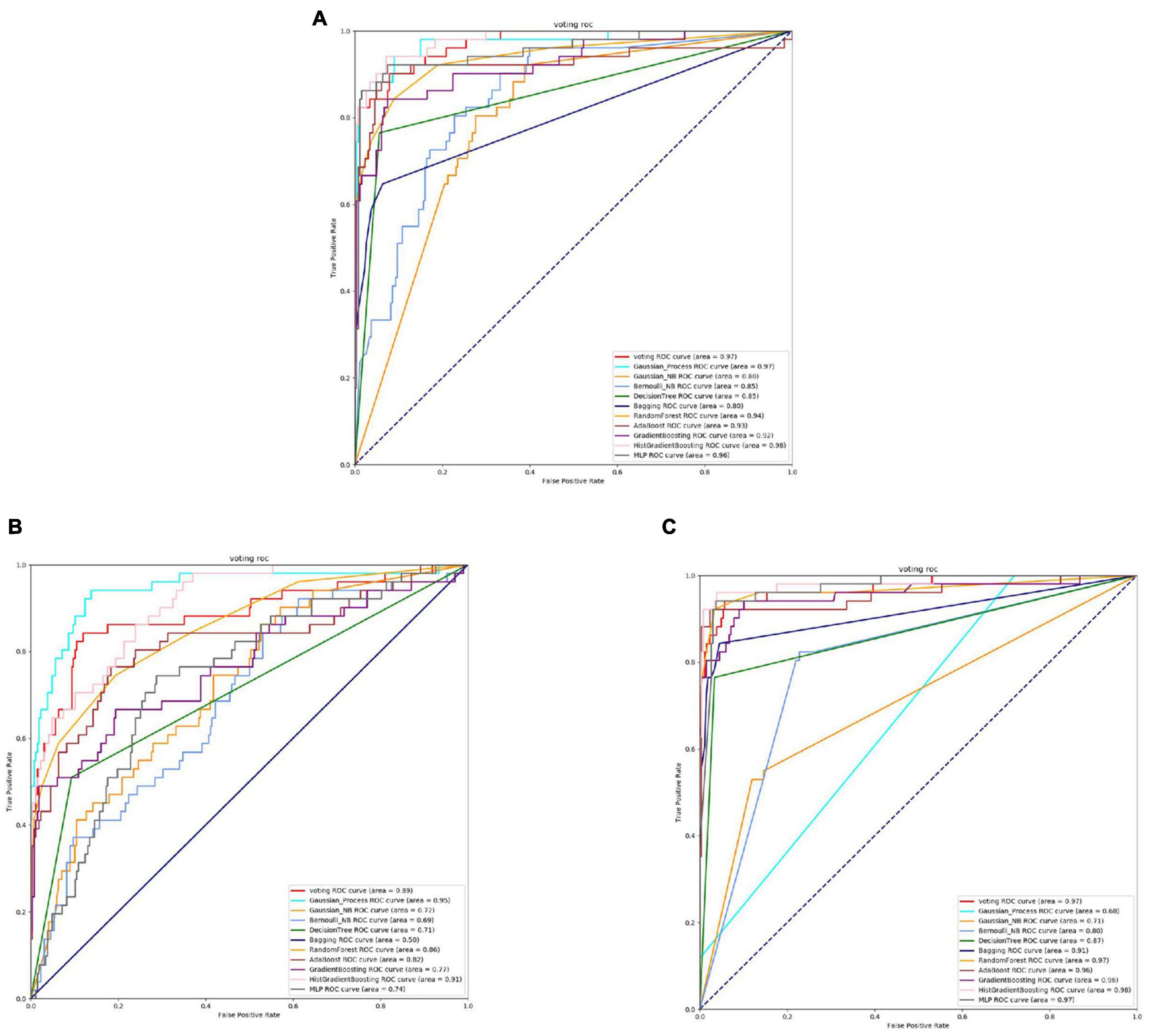
Figure 8. The ROC of Bifidobacterial active sites. (A) Is the ROC curve of 10-fold cross validation with TAGPPI. (B) Is the ROC curve of 10-fold cross validation with SeqVec. (C) Is the ROC curve of 10-fold cross validation with ProSE.
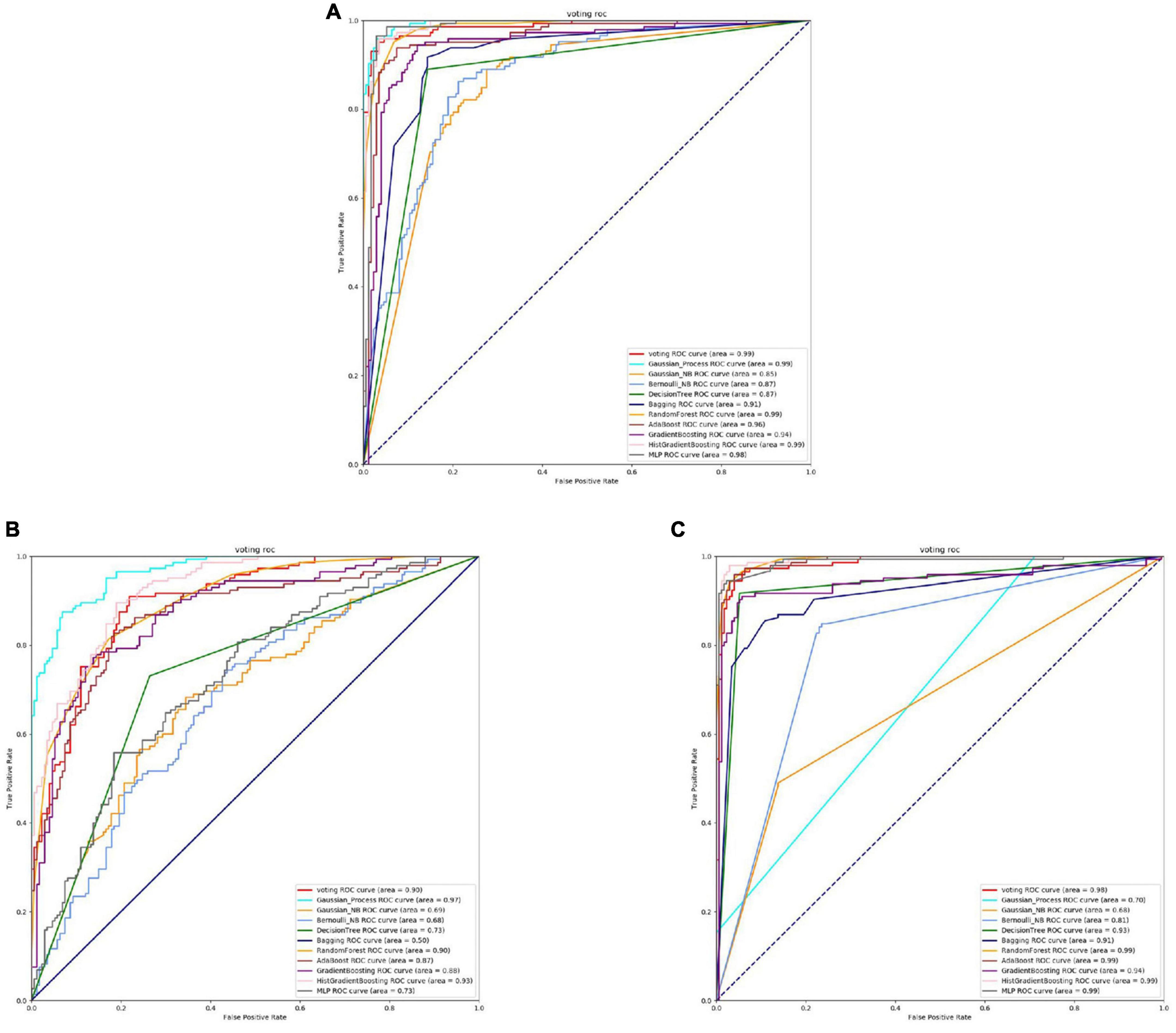
Figure 9. The ROC of Bifidobacterial binding sites. (A) Is the ROC curve of 10-fold cross validation with TAGPPI. (B) Is the ROC curve of 10-fold cross validation with SeqVec. (C) Is the ROC curve of 10-fold cross validation with ProSE.
According to the data, it can be concluded that both ProSE and TAGPPI exhibit good coverage and balance for the active site and binding site of Bifidobacterium. Both feature extraction methods are viable options, but considering model accuracy, ProSE is the optimal choice. However, if factors such as training cost and large-scale application are taken into account, TAGPPI, which has lower dimensionality, is more suitable.
The following is the score table of each model in the Voting transfer learning model of the active site in three feature extractions.
It can be observed that the Gaussian Process consistently performs poorly, and the ROC curve for the SeqVec feature extraction method is scattered. This clearly indicates that SeqVec does not provide comprehensive feature extraction for the active site of Bifidobacterium. This issue was also evident in the previous analysis of neighboring poor-nutrition bacteria, suggesting that SeqVec feature extraction method is not suitable for some microorganisms and yields subpar results. To improve experimental efficiency, one approach could involve identifying protein sequences with poor performance and dividing them into categories of weakly effective proteins.
The following is the scoring table for each model in the Voting transfer learning model of the Binding site in three feature extractions.
Based on the ROC curves and model evaluation scores, it can be observed that the active site and binding site exhibit high similarity, and Gaussian Process consistently performs poorly. The ROC curve for SeqVec feature extraction method is scattered.
In summary, for Bifidobacterium, using TAGPPI for feature extraction is the optimal choice. It yields high accuracy for both the transfer learning model and the training model. SeqVec feature extraction method is not suitable for some microorganisms and produces inferior results. To improve experimental efficiency, one approach could involve categorizing proteins with poor performance and identifying weakly effective protein sequences.
The Figures 10, 11 and Table 7 shows that, overall, the three feature extraction methods perform well for Capnocytophaga. However, SeqVec exhibits relatively poorer performance and even has a case where sp = 0, indicating that this feature extraction method predominantly captures positive site-specific features while weakening the negative site-specific features. On the other hand, the other two feature extraction methods not only preserve positive site-specific features but also maintain a coverage rate of around 60% for negative site-specific features. Therefore, ProSE and TAGPPI are more suitable for Capnocytophaga.
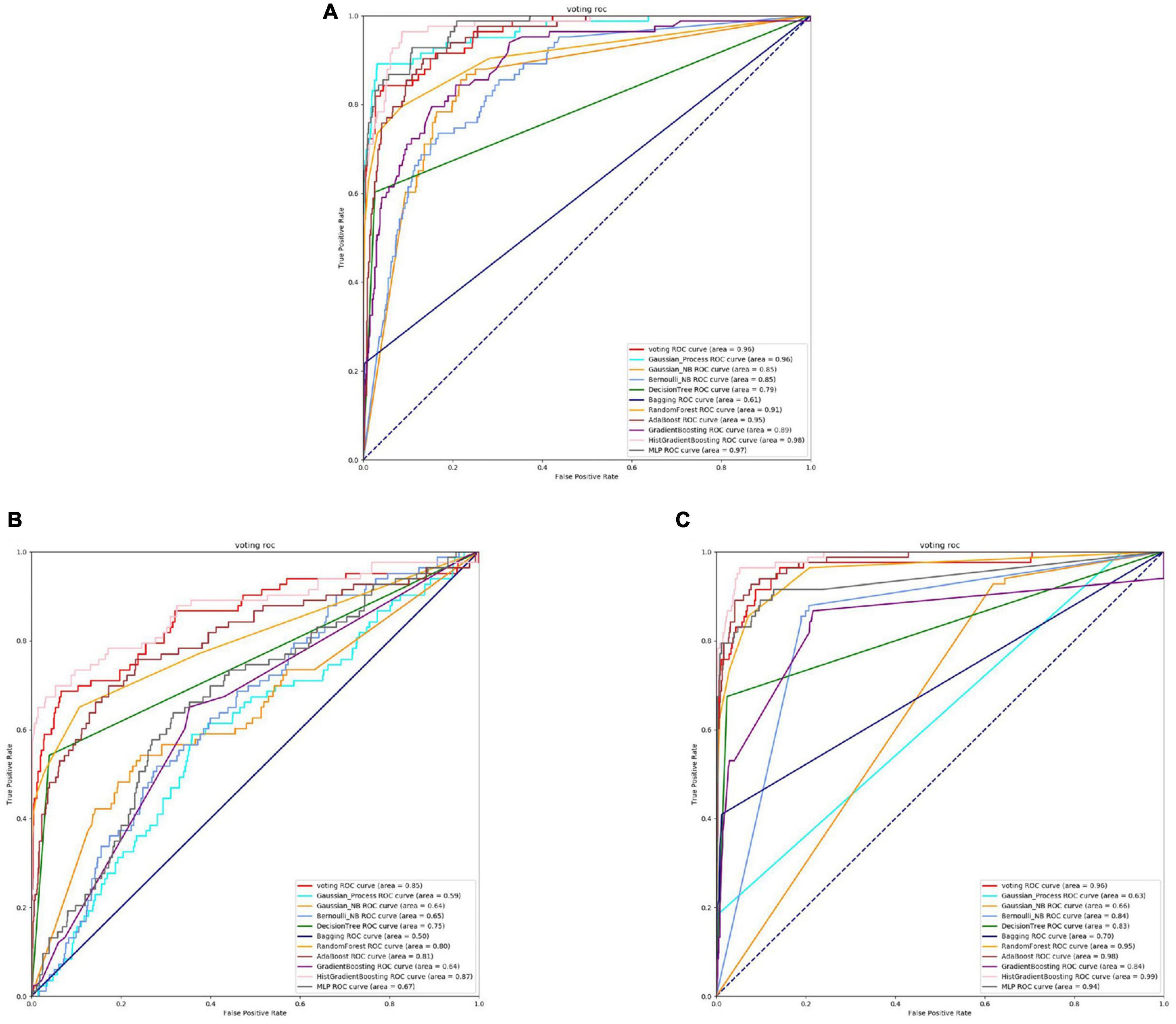
Figure 10. The ROC of Capnocytophaga active sites. (A) Is the ROC curve of 10-fold cross validation with TAGPPI. (B) Is the ROC curve of 10-fold cross validation with SeqVec. (C) Is the ROC curve of 10-fold cross validation with ProSE.
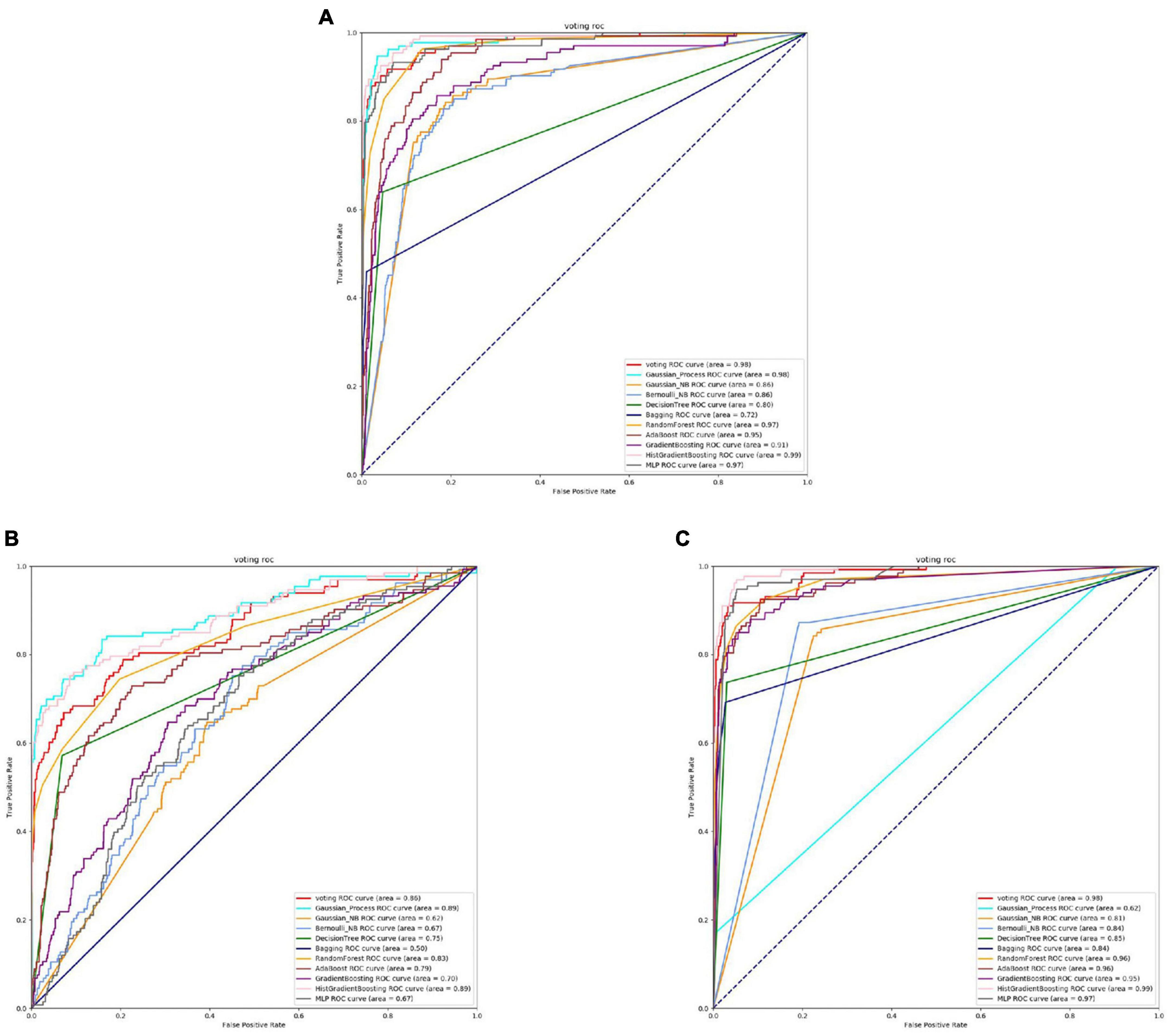
Figure 11. The ROC of Capnocytophaga binding sites. (A) Is the ROC curve of 10-fold cross validation with TAGPPI. (B) Is the ROC curve of 10-fold cross validation with SeqVec. (C) Is the ROC curve of 10-fold cross validation with ProSE.
The following is the score table of each model in the Voting transfer learning model of the active site in three feature extractions.
It can be observed that the Decision Tree model performs relatively poorly, while both ProSE and TAGPPI show relatively higher performance compared to the previous cases, although they both exhibit weak classifiers. In this case, weak classifiers are present in both methods, but ProSE has a slightly higher number of weak classifiers. However, the final accuracy of the transfer learning models is comparable. SeqVec ROC curve is more scattered, indicating that the majority of the models trained using this feature extraction method are weak learners, resulting in significantly worse performance compared to the other two methods. However, after applying the voting strategy, the accuracy is only slightly lower than the other two methods, by around 5%. Overall, this demonstrates the excellent generalization ability of the models.
The following is the scoring table for each model in the Voting transfer learning model of the Binding site in three feature extractions.
The models trained using the TAGPPI feature extraction method consistently exhibit high accuracy, indicating that TAGPPI is a superior feature extraction method. Compared to the active site, it can be observed that there is a significant similarity in both the overall dataset and model results for the binding site. This suggests that the active site and binding site in the Capnocytophaga may have similar feature labels or exhibit some degree of overlap. The SeqVec feature extraction method still shows scattered ROC curves, indicating its ongoing issues with training models effectively.
Based on the above analysis, the following feature extraction methods are recommended for the discrimination of the five oral microorganisms: Streptococcus mutans: ProSE; Staphylococcus aureus: ProSE or TAGPPI; Abiotrophia adjacens: ProSE or TAGPPI; Bifidobacterial: TAGPPI; Capnocytophaga: TAGPPI. The five oral microorganisms will be judged as a whole.
According to Table 8, it can be observed that TAGPPI performs poorly for the Streptococcus mutans model. However, for the remaining four species, the models trained using TAGPPI achieve excellent results with an accuracy of around 95%. Upon observing the F1 scores, it is evident that most of them are above 0.85, indicating that the models trained on TAGPPI’s dataset are stable and comparable to ProSE. The recall values are also generally around or above 0.85. This suggests that TAGPPI is the most effective feature extraction method among the three for this particular transfer learning model.
According to the Supplementary Tables 2, 3, it can be observed that SeqVec performs poorly for the Streptococcus mutans model. For the remaining four species, the models achieve good results with accuracy above 80%, which is considered excellent. However, upon observing the F1 scores, it is found that most of them are below 0.5, indicating a lack of stability in the models. This is something that should be avoided in practical applications. Considering the recall values, only Staphylococcus aureus and Bifidobacterium show relatively good performance. It is also noted that SeqVec exhibits high coverage for positive instances, indicating an emphasis on extracting features related to positive class labels. This can be considered the optimal solution for specific requirements. Therefore, overall, SeqVec is not suitable for all oral microbiota and has its limitations.
4 Conclusion
In this work, we proposed a novel method, which is oral_voting_transfer, to deal with such classification issues in the field of oral microorganisms. In detail, we utilized the highly effective model, which successfully classifies the organelles proteins and transfer to deal with five microorganisms, including streptococcus mutans, staphylococcus aureus, abiotrophia adjacens, bifidobacterial and capnocytophaga. The oral_voting_transfer method employed three deep learning features, including TAGPPI, SeqVec and ProSE.
The performance of the models trained on the Streptococcus mutans dataset was poor for all three feature extraction methods. Among the three methods, ProSE showed the best stability and accuracy, making it the optimal choice. SeqVec, on the other hand, exhibited limitations and its model performance was generally less stable. However, it can be used for feature extraction in specific microbial protein data, as it excels in capturing positive sample labels with high coverage. TAGPPI performed comparably to ProSE, but considering the case of Streptococcus mutans, ProSE is the preferred choice. However, TAGPPI offers the advantage of being simpler and more efficient in terms of feature extraction and training. If large-scale services and training are required, TAGPPI would be the more favorable option.
Data availability statement
The original contributions presented in the study are included in the article/Supplementary material, further inquiries can be directed to the corresponding author.
Author contributions
WB: Data curation, Formal Analysis, Investigation, Resources, Software, Writing – original draft, Writing – review and editing. YL: Data curation, Formal Analysis, Resources, Writing – original draft, Writing – review and editing. BC: Conceptualization, Data curation, Investigation, Writing – review and editing, Writing – original draft.
Funding
The author(s) declare financial support was received for the research, authorship, and/or publication of this article. This work was supported by the National Natural Science Foundation of China (Grant No. 61902337), Xuzhou Science and Technology Plan Project (KC21047), Jiangsu Provincial Natural Science Foundation (No. SBK2019040953), Natural Science Fund for Colleges and Universities in Jiangsu Province (No. 19KJB520016), and Young Talents of Science and Technology in Jiangsu and ghfund202302026465.
Conflict of interest
The authors declare that the research was conducted in the absence of any commercial or financial relationships that could be construed as a potential conflict of interest.
Publisher’s note
All claims expressed in this article are solely those of the authors and do not necessarily represent those of their affiliated organizations, or those of the publisher, the editors and the reviewers. Any product that may be evaluated in this article, or claim that may be made by its manufacturer, is not guaranteed or endorsed by the publisher.
Supplementary material
The Supplementary Material for this article can be found online at: https://www.frontiersin.org/articles/10.3389/fmicb.2023.1277121/full#supplementary-material
References
Arlot, S., and Genuer, R. (2016). Comments on: A random forest guided tour. Test 25, 228–238. doi: 10.1007/s11749-016-0484-4
Awais, M., Hussain, W., Khan, Y., Rasool, N., Khan, S., and Chou, K. (2019). iPhosH-PseAAC: identify phosphohistidine sites in proteins by blending statistical moments and position relative features according to the Chou’s 5-step rule and general pseudo amino acid composition. IEEE/ACM Trans. Comput. Biol. Bioinform. 18, 596–610. doi: 10.1109/TCBB.2019.2919025
Bradford, J. R., and Westhead, D. R. (2005). Improved prediction of protein–protein binding sites using a support vector machines approach. Bioinformatics 21, 1487–1494. doi: 10.1093/bioinformatics/bti242
Brohee, S., and van Helden, J. (2006). Evaluation of clustering algorithms for protein-protein interaction networks. BMC Bioinformatics 7:488. doi: 10.1186/1471-2105-7-488
Chatterjee, P., Basu, S., Kundu, M., Nasipuri, M., and Plewczynski, D. (2011). PPI_SVM: prediction of protein-protein interactions using machine learning, domain-domain affinities and frequency tables. Cell. Mol. Biol. Lett. 16, 264–278. doi: 10.2478/s11658-011-0008-x
Chen, X., and Liu, M. (2005). Prediction of protein–protein interactions using random decision forest framework. Bioinformatics 21, 4394–4400. doi: 10.1093/bioinformatics/bti721
Coates, P. J., and Hall, P. A. (2003). The yeast two-hybrid system for identifying protein–protein interactions. J. Pathol. 199, 4–7. doi: 10.1002/path.1267
Cui, G., Fang, C., and Han, K. (2012). Prediction of protein-protein interactions between viruses and human by an SVM model. BMC Bioinformatics 13:S5. doi: 10.1186/1471-2105-13-S7-S5
De Las Rivas, J., and Fontanillo, C. (2010). Protein–protein interactions essentials: key concepts to building and analyzing interactome networks. PLoS Comput. Biol. 6:e1000807. doi: 10.1371/journal.pcbi.1000807
Díaz-Uriarte, R., and de Andrés, S. (2006). Gene selection and classification of microarray data using random forest. BMC Bioinformatics 7:3. doi: 10.1186/1471-2105-7-3
Dudoit, S., Fridlyand, J., and Speed, T. P. (2002). Comparison of discrimination methods for the classification of tumors using gene expression data. J. Am. Stat. Assoc. 97, 77–87. doi: 10.1198/016214502753479248
Free, R. B., Hazelwood, L. A., and Sibley, D. A. (2009). Identifying novel protein-protein interactions using co-immunoprecipitation and mass spectroscopy. Curr. Protoc. Neurosci. 46, 5–28. doi: 10.1002/0471142301.ns0528s46
Gao, H., Sun, J., Wang, Y., Lu, Y., Liu, L., Zhao, Q., et al. (2023). Predicting metabolite-disease associations based on auto-encoder and non-negative matrix factorization. Brief. Bioinformatics 24:bbad259. doi: 10.1093/bib/bbad259
Godzik, A., Skolnick, J., and Koliński, A. (1995). Are proteins ideal mixtures of amino acids? Analysis of energy parameter sets. Protein Sci. 4, 2107–2117. doi: 10.1002/pro.5560041016
Guo, Y., Yu, L., Wen, Z., and Li, M. (2008). Using support vector machine combined with auto covariance to predict protein–protein interactions from protein sequences. Nucleic Acids Res. 36, 3025–3030. doi: 10.1093/nar/gkn159
Kim, Y., and Subramaniam, S. (2006). Locally defined protein phylogenetic profiles reveal previously missed protein interactions and functional relationships. Proteins Struct. Funct. Bioinformatics 62, 1115–1124. doi: 10.1002/prot.20830
Koike, A., and Takagi, T. (2004). Prediction of protein–protein interaction sites using support vector machines. Protein Eng. Design Selection 17, 165–173. doi: 10.1093/protein/gzh020
Lee, J., Lee, J., Park, M., and Song, S. H. (2005). An extensive evaluation of recent classification tools applied to microarray data. Comput. Stat. Data Anal. 48, 869–885. doi: 10.1016/j.csda.2004.03.017
Li, B., Feng, K., Chen, L., Huang, T., and Cai, Y. (2012). Prediction of protein-protein interaction sites by random forest algorithm with mRMR and IFS. PLoS One 7. 8:e43927. doi: 10.1371/journal.pone.0043927
Liu, Q., Chen, P., Wang, B., Zhang, J., and Li, J. (2018). Hot spot prediction in protein-protein interactions by an ensemble system. BMC Syst. Biol. 12:132. doi: 10.1186/s12918-018-0665-8
Meng, R., Yin, S., Sun, J., Hu, H., and Zhao, Q. (2023). scAAGA: single cell data analysis framework using asymmetric autoencoder with gene attention. Comput. Biol. Med. 165:107417. doi: 10.1016/j.compbiomed.2023.107414
Ngo, J. T., Marks, J., and Karplus, M. (1994). “Computational complexity, protein structure prediction, and the Levinthal paradox,” in The Protein Folding Problem and Tertiary Structure Prediction, eds K. M. Merz and S. M. Grand (Berlin: Springer). doi: 10.1007/978-1-4684-6831-1_14
Ngounou Wetie, A., Sokolowska, I., Woods, A., Roy, U., Deinhardt, K., and Darie, C. (2014). Protein–protein interactions: switch from classical methods to proteomics and bioinformatics-based approaches. Cell. Mol. Life Sci. 71, 205–228. doi: 10.1007/s00018-013-1333-1
Nguyen-Vo, T., Trinh, Q., Nguyen, L., Nguyen-Hoang, P., Rahardja, S., and Nguyen, B. (2022). iPromoter-Seqvec: identifying promoters using bidirectional long short-term memory and sequence-embedded features. BMC Genomics 23:681. doi: 10.1186/s12864-022-08829-6
Niu, S., Liu, Y., Wang, J., and Song, H. (2020). A Decade Survey of Transfer Learning (2010–2020). Piscataway, NJ: IEEE. doi: 10.1109/TAI.2021.3054609
Pashaei, E., Ozen, M., and Aydin, N. (2016). “Splice sites prediction of human genome using AdaBoost,” in Proceedings of the 2016 IEEE-EMBS International Conference on Biomedical and Health Informatics (BHI), (Las Vegas, NV). doi: 10.1109/BHI.2016.7455894
Peng, X., Wang, J., Peng, W., Wu, F., and Pan, Y. (2017). Protein–protein interactions: detection, reliability assessment and applications. Brief. Bioinformatics 18, 798–819. doi: 10.1093/bib/bbw066
Robson, E., Xu, C., and Wills, L. W. (2022). “ProSE: the architecture and design of a protein discovery engine,” in Proceedings of the 27th ACM International Conference on Architectural Support for Programming Languages and Operating Systems, (New York, NY: ACM). doi: 10.1145/3503222.3507722
Romero-Molina, S., Ruiz-Blanco, Y., Harms, M., Münch, J., and Sanchez-Garcia, E. (2019). PPI-Detect: a support vector machine model for sequence-based prediction of protein–protein interactions. J. Comput. Chem. 40, 1233–1242. doi: 10.1002/jcc.25780
Saha, I., Zubek, J., Klingström, T., Forsberg, S., Wikander, J., and Kierczak, M. (2014). Ensemble learning prediction of protein–protein interactions using proteins functional annotations. Mol. BioSyst. 10, 820–830. doi: 10.1039/c3mb70486f
Sato, T., Hanada, M., Bodrug, S., Irie, S., Iwama, N., Boise, L., et al. (1994). Interactions among members of the Bcl-2 protein family analyzed with a yeast two-hybrid system. Proc. Natl. Acad. Sci. 91, 9238–9242. doi: 10.1073/pnas.91.20.9238
Schwikowski, B., Uetz, P., and Fields, S. (2000). A network of protein–protein interactions in yeast. Nat. Biotechnol. 18, 1257–1261. doi: 10.1038/82360
Shen, J., Zhang, J., Luo, X., Zhu, W., Yu, K., and Chen, K. (2007). Predicting protein–protein interactions based only on sequences information. Proc. Natl. Acad. Sci. 104, 4337–4341. doi: 10.1073/pnas.0607879104
Shen, Z., Lin, Y., and Zou, Q. (2019). Transcription factors–DNA interactions in rice: identification and verification. Brief. Bioinformatics 21, 946–956. doi: 10.1093/bib/bbz045
Song, B., Luo, X., Luo, X., Liu, Y., Niu, Z., and Zeng, X. (2022). Learning spatial structures of proteins improves protein–protein interaction prediction. Brief. Bioinformatics 23:bbab558. doi: 10.1093/bib/bbab558
Sun, T., Zhou, B., Lai, L., and Pei, J. (2017). Sequence-based prediction of protein protein interaction using a deep-learning algorithm. BMC Bioinformatics 18:277. doi: 10.1186/s12859-017-1700-2
Torrey, L., and Shavlik, J. (2010). “Transfer learning,” in Handbook of Research on Machine Learning Applications and Trends: Algorithms, Methods, and Techniques, eds E. Soria, G. J. D. Martin, and M.-S. Marcelino (Pennsylvania: IGI global). doi: 10.4018/978-1-60566-766-9.ch011
Vazquez, A., Flammini, A., Maritan, A., and Vespignani, A. (2003). Global protein function prediction from protein-protein interaction networks. Nat. Biotechnol. 21, 697–700. doi: 10.1038/nbt825
Wang, D., Zeng, S., and Xu, C. (2017). MusiteDeep: a deep-learning framework for general and kinase-specific phosphorylation site prediction. J. Bioinform. 33, 3909–3916.
Wang, L., You, Z., Yan, X., Xia, S., Liu, F., and Li, L. (2018). Using two-dimensional principal component analysis and rotation forest for prediction of protein-protein interactions. Sci. Rep. 8:12874. doi: 10.1038/s41598-018-30694-1
Wang, T., Sun, J., and Zhao, Q. (2023). Investigating cardiotoxicity related with hERG channel blockers using molecular fingerprints and graph attention mechanism. Comput. Biol. Med. 153:106464. doi: 10.1016/j.compbiomed.2022.106464
Wei, L., Xing, P., Zeng, J., Chen, J., Su, R., and Guo, F. (2017). Improved prediction of protein–protein interactions using novel negative samples, features, and an ensemble classifier. Artif. Intell. Med. 83, 67–74. doi: 10.1016/j.artmed.2017.03.001
Wenya, W., Zhang, L., Sun, J., Zhao, Q., and Shuai, J. (2022). Predicting the potential human lncRNA–miRNA interactions based on graph convolution network with conditional random field. Brief. Bioinformatics 23:bbac463. doi: 10.1093/bib/bbac463
Whisstock, J. C., and Lesk, A. M. (2003). Prediction of protein function from protein sequence and structure. Q. Rev. Biophys. 36, 307–340. doi: 10.1017/S0033583503003901
Wu, J., Vallenius, T., Ovaska, K., Westermarck, J., Mäkelä, T., Hautaniemi, S., et al. (2009). Integrated network analysis platform for protein-protein interactions. Nat. Methods 6, 75–77. doi: 10.1038/nmeth.1282
Xia, J. F., Han, K., and Huang, D. S. (2010). Sequence-based prediction of protein-protein interactions by means of rotation forest and autocorrelation descriptor. Protein Pept. Lett. 17, 137–145. doi: 10.2174/092986610789909403
Yang, X., Yang, S., Li, Q., Wuchty, S., and Zhang, Z. (2020). Prediction of human-virus protein-protein interactions through a sequence embedding-based machine learning method. Comput. Struct. Biotechnol. J. 18, 153–161. doi: 10.1016/j.csbj.2019.12.005
You, Z., Chan, K. C. C., and Hu, P. (2015a). Predicting protein-protein interactions from primary protein sequences using a novel multi-scale local feature representation scheme and the random forest. PLoS One 10:e0125811. doi: 10.1371/journal.pone.0125811
You, Z., Li, J., Gao, X., He, Z., Zhu, L., Lei, Y., et al. (2015b). Detecting protein-protein interactions with a novel matrix-based protein sequence representation and support vector machines. BioMed. Res. Int. 2015:867516. doi: 10.1155/2015/867516
You, Z., Lei, Y., Zhu, L., Xia, J., and Wang, B. (2013). Prediction of protein-protein interactions from amino acid sequences with ensemble extreme learning machines and principal component analysis. BMC Bioinformatics 14:S10. doi: 10.1186/1471-2105-14-S8-S10
You, Z., Li, X., and Chan, K. C. C. (2017). An improved sequence-based prediction protocol for protein-protein interactions using amino acids substitution matrix and rotation forest ensemble classifiers. Neurocomputing 228, 277–282. doi: 10.1016/j.neucom.2016.10.042
Zhang, L., Yu, G., Xia, D., and Wang, J. (2019). Protein–protein interactions prediction based on ensemble deep neural networks. Neurocomputing 324, 10–19. doi: 10.1016/j.neucom.2018.02.097
Zhang, Q., Petrey, D., Deng, L., Qiang, L., Shi, Y., and Thu, C. (2012). Structure-based prediction of protein–protein interactions on a genome-wide scale. Nature 490, 556–560. doi: 10.1038/nature11503
Zhang, Y. P., and Zou, Q. (2020). PPTPP: a novel therapeutic peptide prediction method using physicochemical property encoding and adaptive feature representation learning. Bioinformatics 36, 3982–3987. doi: 10.1093/bioinformatics/btaa275
Keywords: oral microorganisms proteins, voting transfer model, bioinformatics, machine learning, classification
Citation: Bao W, Liu Y and Chen B (2024) Oral_voting_transfer: classification of oral microorganisms’ function proteins with voting transfer model. Front. Microbiol. 14:1277121. doi: 10.3389/fmicb.2023.1277121
Received: 14 August 2023; Accepted: 19 December 2023;
Published: 07 February 2024.
Edited by:
Qi Zhao, University of Science and Technology Liaoning, ChinaReviewed by:
Yanjing Wang, Shanghai Jiao Tong University, ChinaJunfeng Xia, Anhui University, China
Copyright © 2024 Bao, Liu and Chen. This is an open-access article distributed under the terms of the Creative Commons Attribution License (CC BY). The use, distribution or reproduction in other forums is permitted, provided the original author(s) and the copyright owner(s) are credited and that the original publication in this journal is cited, in accordance with accepted academic practice. No use, distribution or reproduction is permitted which does not comply with these terms.
*Correspondence: Baitong Chen, 591426198@qq.com
†These authors have contributed equally to this work