- 1Organ Transplantation Center, The Third Xiangya Hospital of Central South University, Changsha, Hunan, China
- 2Engineering and Technology Research Center for Transplantation Medicine of the National Ministry of Health, The Third Xiangya Hospital of Central South University, Changsha, Hunan, China
- 3Hunan Institute of Schistosomiasis Control, Yueyang, Hunan, China
Background: The intestinal microbiota is known to play a role in the development of liver disease, there is a limited understanding of the intestinal microbiota associated with chronic schistosomiasis japonica. This study sought to explore the characteristics of the intestinal microbiota in patients with chronic schistosomiasis japonica and identify potential biomarkers that could aid diagnosis.
Methods: A total of 40 residents of Qingshan Island in Yueyang (Hunan, China) were enrolled in this cross-sectional study. These individuals were divided into two groups for analysis of the intestinal microbiota: patients with chronic schistosomiasis japonica-induced liver fibrosis group (CSJ group, n = 10) and a healthy control group (HC group, n = 30). Feces were collected from each participant and analyzed by 16S rRNA gene sequencing, which included species composition analysis at the phylum and family levels, α and β diversity analysis, LEfSe, Kyoto Encyclopedia of Genes and Genome (KEGG) and Clusters of Orthologous Groups of proteins (COG) analysis.
Results: Our results indicated that Schistosoma japonicum infection changed the composition and abundance of intestinal microbiota at the phylum and family levels. Compared with the HC group, the α and β diversity results showed that CSJ group had low diversity of species of the intestinal microbiome. LEfSe and relative abundance analysis found that the Prevotella 7, Alloprevotella, and Holdemanella genera were significantly higher in the CSJ group than in the HC group. Meanwhile, the ROC analysis showed that the area under the curve (AUC) of Prevotella 7, Alloprevotella, and Holdemanella genera was 0.779, 0.769, and 0.840, respectively. KEGG and COG analysis showed that the Replication and Repair, and Defense Mechanism pathways correlated strongly with chronic schistosomiasis japonica infection.
Conclusion: The current study was the first to explore differences in the intestinal microbiota of patients with chronic schistosomiasis japonica-induced liver fibrosis and healthy people from Qingshan Island, which indicated that Prevotella 7, Alloprevotella, and Holdemanella genera could have a potential value in non-invasive diagnosis of chronic schistosomiasis japonica-induced fibrosis.
1. Introduction
Schistosomiasis is a tropical disease worldwide. An estimated 779 million people are threatened by Schistosoma infection and over 250 million people were infected with Schistosoma spp., resulting in about 1.9 million disability-adjusted life years (DALYs) (Steinmann et al., 2006; Utzinger et al., 2009; GBD 2016 DALYs and HALE Collaborators, 2017). Schistosoma japonicum is endemic in China, especially in the Dongting Lake District (Li et al., 2020; Wang et al., 2021). Qingshan Island, previously called Qingtan Town, is an isolated island in the South Dongting Lake District that has an S. japonicum infection rate of 15.8% in 1998, which is detected by Kato–Katz method and miracidia hatching test (Yan et al., 2003), providing a large patient cohort for the study of this disease.
Chronic schistosomiasis japonica is primarily caused by the host’s reaction to eggs produced by the female Schistosoma, not by the worms themselves. Female S. japonicum can produce up to 3,000 eggs per day, some of which are deposited in the portal area of the liver, where they elicit a Th2-mediated immune response by the host; over time, the chronic local immune response leads to the formation of granulomas, irreversible tissue fibrosis generation, and cirrhosis, which can threaten the life of the patient (Colley et al., 2014; McManus et al., 2018, 2020; Llanwarne and Helmby, 2021). Schistosomiasis japonica includes acute, chronic, and advanced stages. Patients with chronic infection may be slightly symptomatic or symptomless. If not treated in time, chronic infection may develop to advanced schistosomiasis, which has a high mortality (Chen, 2014). Thus, inhibiting the transition from chronic to advanced schistosomiasis japonica is essential to effectively reduce disease-associated mortality. However, the lack of a reliable diagnostic method of patients with chronic infection has delayed the onset of treatment and resulted in poorer outcomes (Mao, 1990; LoVerde, 2019). Currently, both parasitology- and serology-based methods are used to diagnose S. japonicum infection. Those methods had several disadvantages, including a high rate of cross-reactivity with other parasitic diseases, false-positive results, and difficulty in distinguishing current from previous infections (Bergquist et al., 2017; Chen et al., 2021). Thus, there is a clear need to explore and develop a non-invasive and highly sensitive diagnostic method to identify chronic schistosomiasis japonica-induced liver fibrosis.
The intestinal microbiota plays a major role in the regulation of the host immune functions and maintaining immune homeostasis (Belkaid and Harrison, 2017; Pacaud et al., 2021). The intestinal microbiome dysbiosis has been associated with several immune diseases, including type II diabetes, inflammatory bowel disease, and multiple sclerosis (Lavelle and Sokol, 2020; Ghezzi et al., 2021; Yang et al., 2021). Liver diseases are also closely related to an imbalance in the intestinal microbiome since the liver secretes bile and other substances that interact directly with intestinal microbiota (Jones and Neish, 2021). Recent studies have shown that the intestinal microbiota becomes altered during acute and advanced schistosomiasis, indicating that the microbiome could serve as a marker for this disease (Gui et al., 2021; Jiang et al., 2021). Moreover, an intervention using Bacillus subtilis was shown to reduce liver fibrosis and other related symptoms in S. japonicum-infected mice (Lin et al., 2021). These findings suggest that intestinal microbiota may aid the diagnosis and treatment of schistosomiasis. However, knowledge about the characteristics of the intestinal microbiota in patients with chronic schistosomiasis japonica-induced liver fibrosis remains limited. The current study conducted an intestinal microbiota analysis of patients with chronic schistosomiasis japonica-induced liver fibrosis and healthy individuals from Qingshan Island. This study explored the characteristics of the intestinal microbiota associated with chronic schistosomiasis japonica-induced liver fibrosis and provided data that could be used to inform more effective diagnostic methods and treatments for this disease.
2. Materials and methods
2.1. Participants and samples collection
This study was conducted in May 2022. A total of 56 inhabitants living on Qingshan Island were engaged into the cross-sectional study, of whom 40 were finally enrolled for intestinal microbiota analysis and divided into two groups: patients with chronic schistosomiasis japonica-induced liver fibrosis group (CSJ group, n = 10) and a healthy control group (HC group, n = 30). CSJ participants were eligible for inclusion in the study if they met the following criteria: (a) with a clear history of S. japonicum infection; (b) history of treatment for S. japonicum; (c) have no prior history anti-helminthic or antibiotic treatment within 1 month (Schneeberger et al., 2018; Ramirez et al., 2020); (d) with liver fibrosis by ultrasound diagnosis, without ascites, portal hypertension and splenomegaly, etc., which referred to the previous studies (Abdel Wahab et al., 1992; Richter et al., 2003; Wu et al., 2015). Participants were excluded if they presented with cognitive or mental disabilities, gastrointestinal diseases, and/or other liver diseases, such as hepatitis, fatty liver and hepatocellular carcinoma. HC individuals were referred to reside on Qingshan Island, have no prior history of S. japonicum and test negative for S. japonicum. Fresh feces and blood were collected from all participants for analysis. Blood routine examination, including hemoglobin (HGB), neutrophils (NEU), eosinophils (EOS), lymphocyte (MON) and platelet (PLT), were tested by an automated hematologic analyzer (Sysmex XN-9000); Blood biochemical examination, including total triglycerides (TG), total cholesterol (TC), alanine aminotransferase (ALT), aspartate aminotransferase (AST), alkaline phosphatase (ALP), albumin (ALB), globulin (GLOB), and total protein (TP) were tested by an automatic biochemical analyzer (Hitachi 7600).
2.2. Extraction of genome DNA
Total genomic DNA from fecal samples were extracted using OMEGA Soil DNA Kit (M5635-02, Omega Bio-Tek). DNA concentration and purity were monitored on 1% agarose gels. According to the concentration, DNA was diluted to 1 ng/μl using sterile water.
2.3. Amplicon generation
The V3–4 hypervariable region of the 16S rRNA genes was amplified using the specific primers with the barcode: 341F (5′-CCTAYGGGRBGCASCAG-3′) and 806R (5′-GGACTACNNGGGTATCTAAT-3′). All PCR reactions were carried out in 30 μl reactions with 15 μl of the Phusion® High-Fidelity PCR Master Mix (New England Biolabs), 0.2 μM of the forward and reverse primers, and about 10 ng template DNA. Thermal cycling included an initial denaturation of 98°C for 1 min, followed by 30 cycles of denaturation at 98°C for 10 s, annealing at 50°C for 30 s, elongation at 72°C for 60 s, and finally heat preservation for 5 min at 72°C.
2.4. PCR product quantification and qualification
The same volume of 1× loading buffer (containing SYB green) was mixed with the PCR products and subjected to electrophoresis on a 2% agarose gel. Samples with a bright strip between 400 and 450 bp were chosen for further experiments. PCR products were mixed in equidensity ratios. Then, mixture PCR products was purified with Qiagen Gel Extraction Kit (Qiagen).
2.5. Library preparation and sequencing
Sequencing libraries were generated using TruSeq® DNA PCR-Free Sample Preparation Kit (Illumina) following manufacturer’s recommendations and index codes were added. The library quality was assessed on the Qubit® 2.0 Fluorometer (Thermo Scientific) and Agilent Bioanalyzer 2100 system. At last, the library was sequenced on an Illumina NovaSeq6000 platform and 250 bp paired-end reads were generated. Quality control on the raw reads was performed using Quantitative Insights into Microbial Ecology (QIIME 1.8.0) as previously described (Caporaso et al., 2010).
2.6. Sequencing data analysis
Paired-end reads from the original DNA fragments were merged using FLASH (Magoè and Salzberg, 2011), a very fast and accurate analysis tool designed to merge paired-end reads. When at least some of the reads overlap the read was generated from the opposite end of the same DNA fragment. Paired-end reads were assigned to each sample according to the unique barcodes. Sequence analysis was performed using the UPARSE software package with the UPARSE-Operational Taxonomic Units (OTU) and UPARSE-OTUref algorithms (Edgar, 2010, 2013). Sequences with ≥97% similarity were assigned to the same OTUs. A representative sequence was chosen for each OTU and the RDP classifier was used to annotate taxonomic information for each representative sequence (Silva 132 for 16S, UNITE for ITS). α (within sample) and β (among sample) diversities were estimated using MOTHUR software (v1.31.2) (Schloss et al., 2009) and the QIIME pipeline (v1.8.0), respectively. The Observed Species, Good’s coverage, Chao1, ACE, Shannon, and Simpson indexes were used to evaluate α-diversity. Shannon curves were generated based on these indexes. β-diversity analysis was calculated using the weighted UniFrac (Lozupone et al., 2011) and visualized by principal coordinates analysis (PCoA) downscaling. To measure differences in individual taxonomic abundance, linear discriminant analysis (LDA) effect size (LEfSe) was used to count species with significant differences in community structure between the samples. A LDA score >2 was considered a significant difference and used to screen for characteristic biomarkers of the intestinal microbiota between groups (Segata et al., 2011). Microbial functions were predicted by PICRUSt (phylogenetic investigation of communities by reconstruction of unobserved states) (Langille et al., 2013) in the Kyoto Encyclopedia of Genes and Genomes (KEGG) and Clusters of Orthologous Groups of proteins (COG) databases based on 16S rRNA gene sequence data. KEGG and COG analysis between two groups were conducted using LEfSe (Segata et al., 2011).
2.7. Statistical analysis
Statistical analyses were performed using SPSS software (v 26.0; SPSS, IL, USA). Continuous variables were expressed as the mean ± standard deviation (SD) or median [interquartile range (IQR)]. The Student’s t-test or Wilcoxon Rank-Sum and the χ2 tests were used to evaluate differences between two groups for continuous and categorical variables, respectively. Receiver operation characteristic (ROC) analysis to predict the diagnostic efficiency of the different intestinal microbiota. The false discovery rate (FDR) was used to correct the calculated Q-values. A P-value < 0.05 was considered statistically significant.
3. Results
3.1. Participant characteristics
A participant flow chart was shown in Figure 1. A total of 56 inhabitants of Qingshan Island participated in the cross-sectional study, of whom 40 were finally enrolled in the intestinal microbiota analysis based on the inclusion and exclusion criteria. These participants were divided into a CSJ group (n = 10) and a HC group (n = 30). The general characteristics of all participants were shown in Table 1, no significant differences were observed between the two groups.
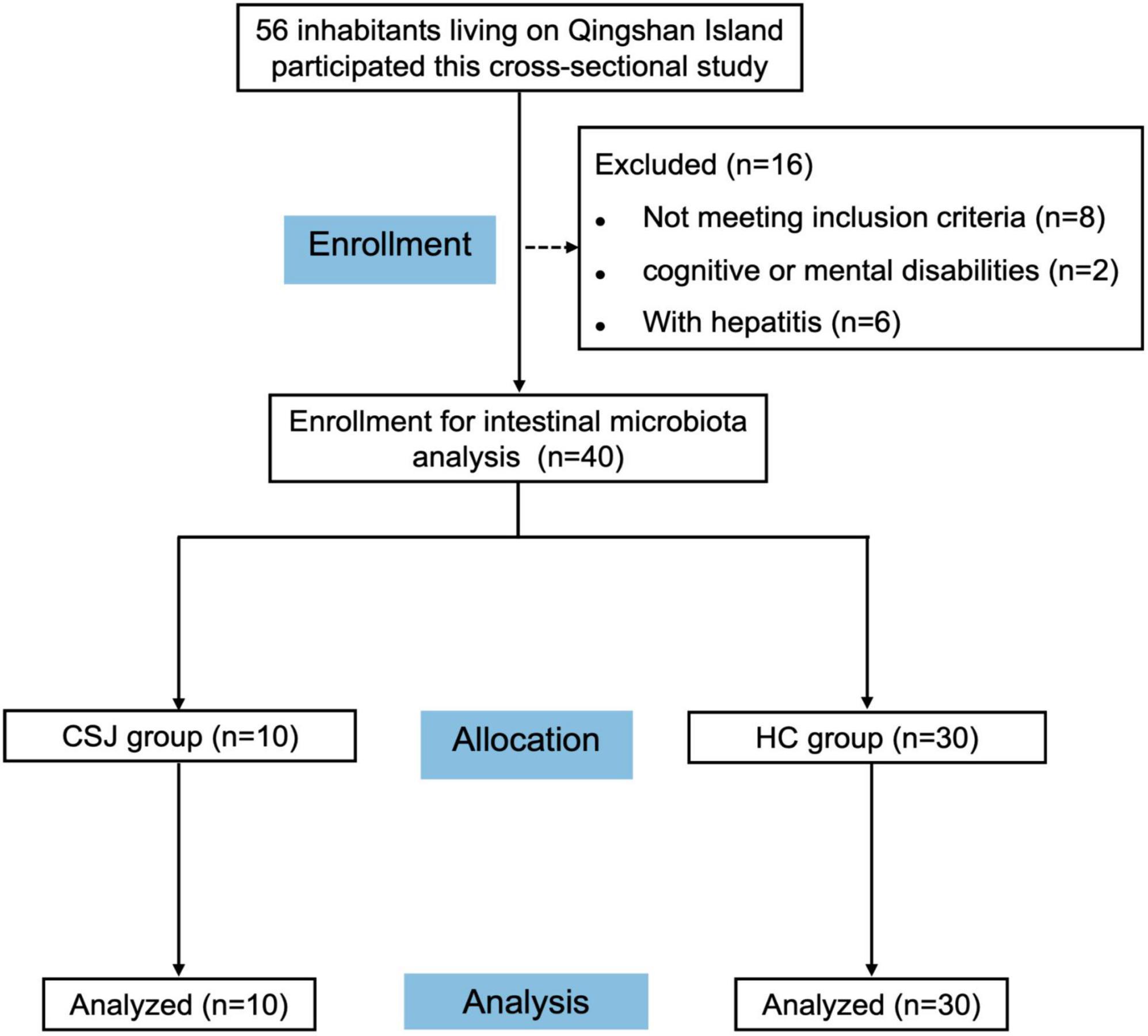
Figure 1. Flow diagram depicting participant recruitment and retention. A total of 56 inhabitants were engaged into the cross-sectional study, of whom 16 were excluded, and 40 were finally enrolled for intestinal microbiota analysis and divided into chronic schistosomiasis japonica-induced liver fibrosis group (CSJ group) and a healthy control group (HC group).
3.2. Species composition and abundance of the intestinal microbiota in the two groups
In our study, 250 bp paired-end reads were generated by Illumina NovaSeq6000 platform. There were 3,060,101 sequences in total after demultiplexing and filtering for read quality, with a median of 77,322 sequences per experimental sample. The species accumulation curve of the samples reached a plateau, indicating that the study sample size was sufficient (Figure 2A). The Shannon curve indicated that the sequencing depth detected the most microbial information for each fecal sample (Figure 2B). To remove potential signal from contaminants, we removed from analysis taxa with >10% relative abundance in the blank swab samples and OTUs with <2 total occurrences among the samples. All reads clustered into 5,051 OTUs. Among them, 1,309 and 3,742 OTUs were detected in the CSJ and HC groups, respectively, and a Venn diagram indicated that 1,207 of these OTUs were found in both groups (Figure 2C). ANOSIM analysis was also performed and the between-group difference was greater than the within-group difference (R = 0.153, P = 0.001), indicating that the study groups were valid (Figure 2D).
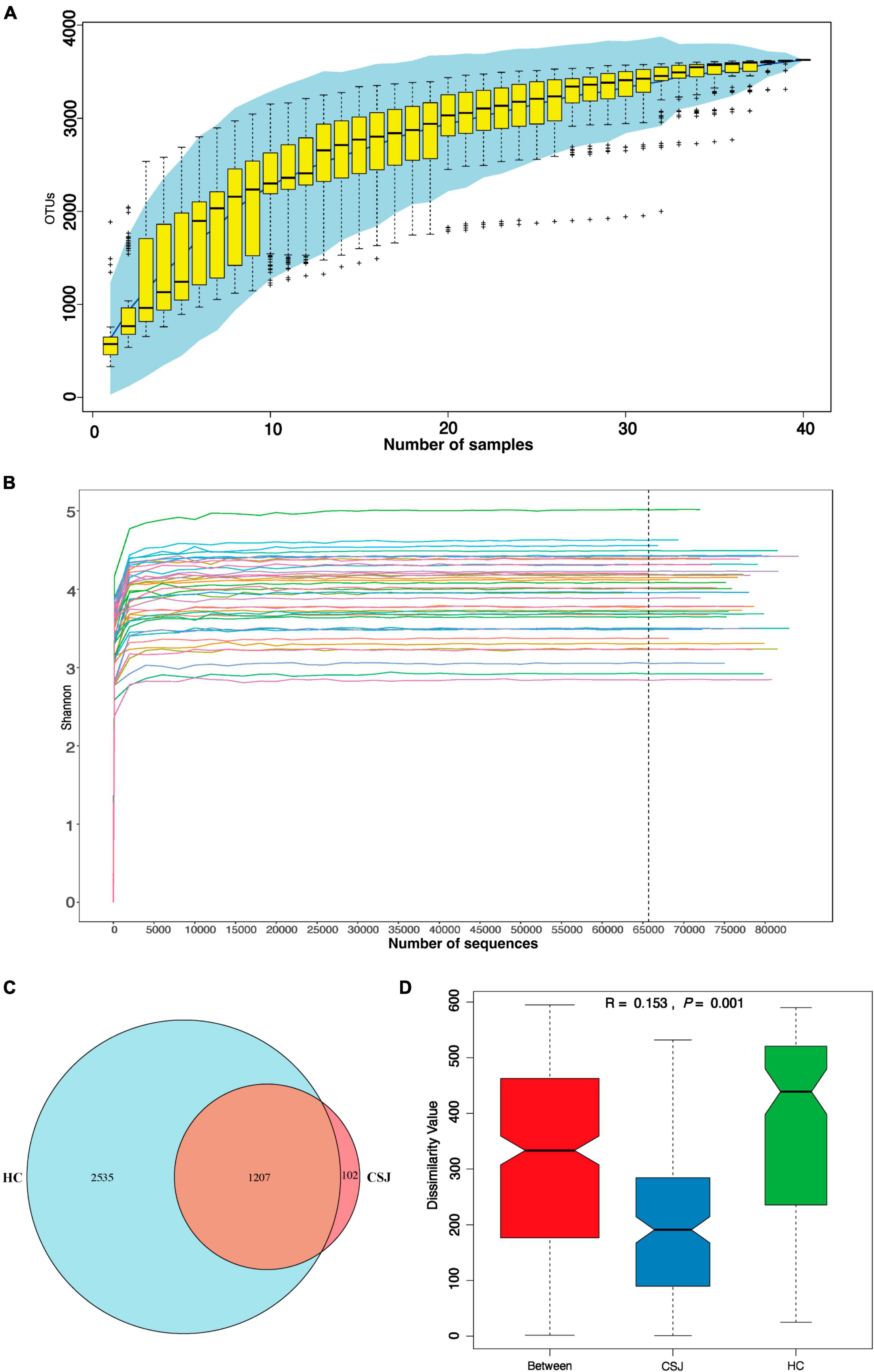
Figure 2. Quality evaluation of 16S rRNA gene sequence data. (A) Accumulation curve was given by cumulative data of operational taxonomic units (OTUs) per sample. (B) Shannon curve was constructed according to the microbial diversity index of the sequencing quantity of each sample at different sequencing depths. (C) Venn diagram represented the OTUs detected each sample between two groups and indicated that 1,207 of these OTUs were found in both groups. (D) Analysis of similarities (ANOSIM) was based on Bray–Curtis algorithm, R > 0 was considered a significant difference between groups rather than within groups, P < 0.05 was considered to be statistically significant.
Relative abundance of the intestinal microbiota at the phylum and family levels was also assessed. At the phylum level, the dominant microbiota of the two groups were similar, indicating that the disease status did not change the “Enterotype” of the patients. Firmicutes, Bacteroidetes, and Proteobacteria were the three most common phyla found in both groups, together accounting for 94.9 and 96.4% of the abundance in the CSJ group and HC groups, respectively. The abundance of Firmicutes and Bacteroidetes was higher in the CSJ group than in the HC group, while the abundance of Proteobacteria was significantly higher in the HC group than in the CSJ group (Figure 3A). At the family level, Lachnospiraceae, Prevotellaceae, and Erysipelotrichaceae were increased in CSJ group, while Enterobacteriaceae and Veillonellaceae were reduced (Figure 3B).
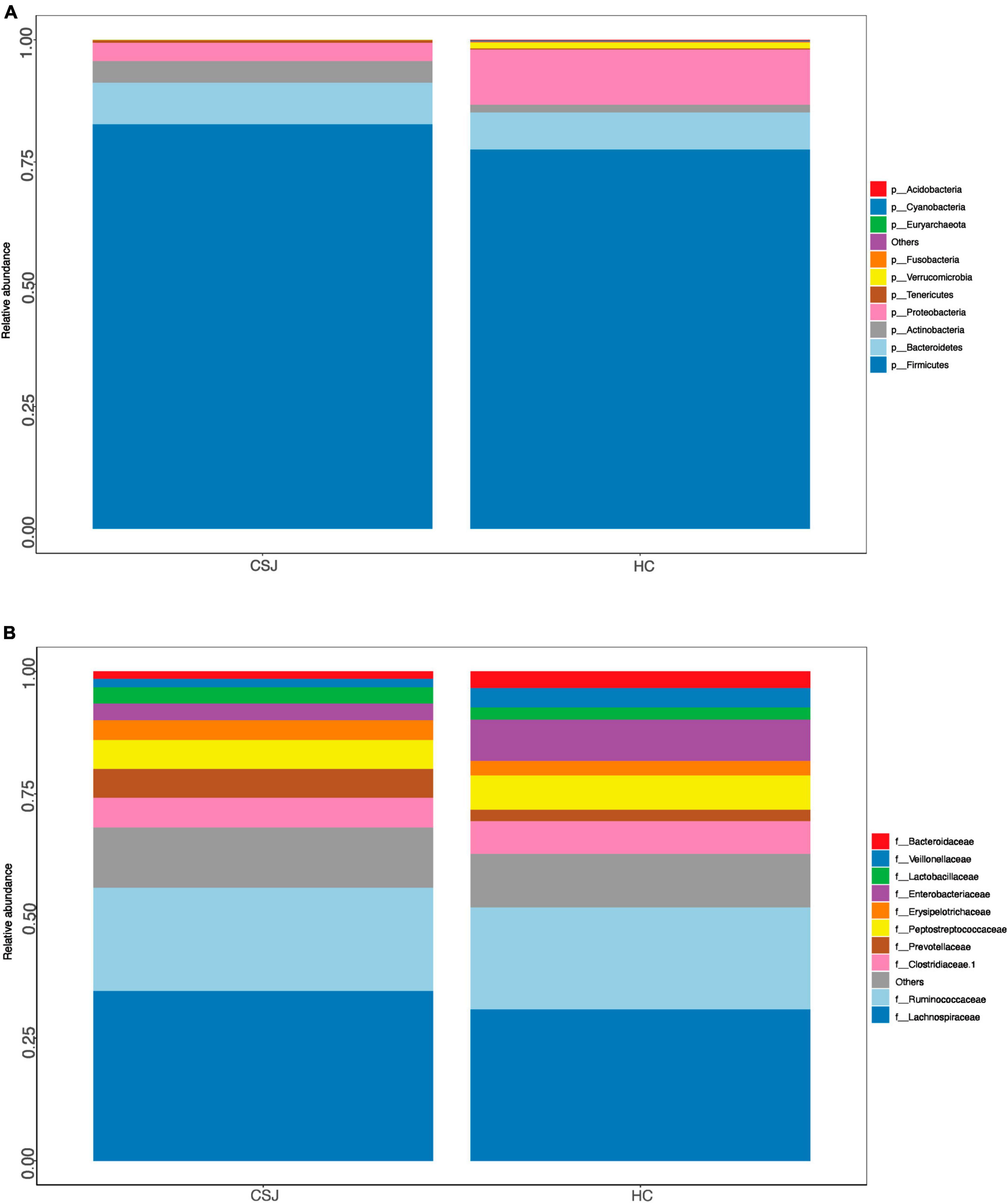
Figure 3. Species distribution of intestinal microbiota between CSJ group and HC group on phylum level (A) and family level (B).
3.3. The diversity analysis of the intestinal microbiota in the two groups
3.3.1. α-Diversity analysis
α-Diversity represented the diversity of species within a given community. The following indexes were used to evaluate α-diversity: Observed Species (Z = −1.55, P = 0.060), Good’s coverage (Z = −1.47, P = 0.072), Chao1 (Z = −1.54, P = 0.062), ACE (Z = −1.54, P = 0.061), Shannon (t(38) = 0.69, P = 0.970), and Simpson (Z = −1.50, P = 0.670). Differences were detected between the two groups in four indexes except Shannon and Simpson indexes, indicating that CSJ group had lower diversity of species (Figure 4).
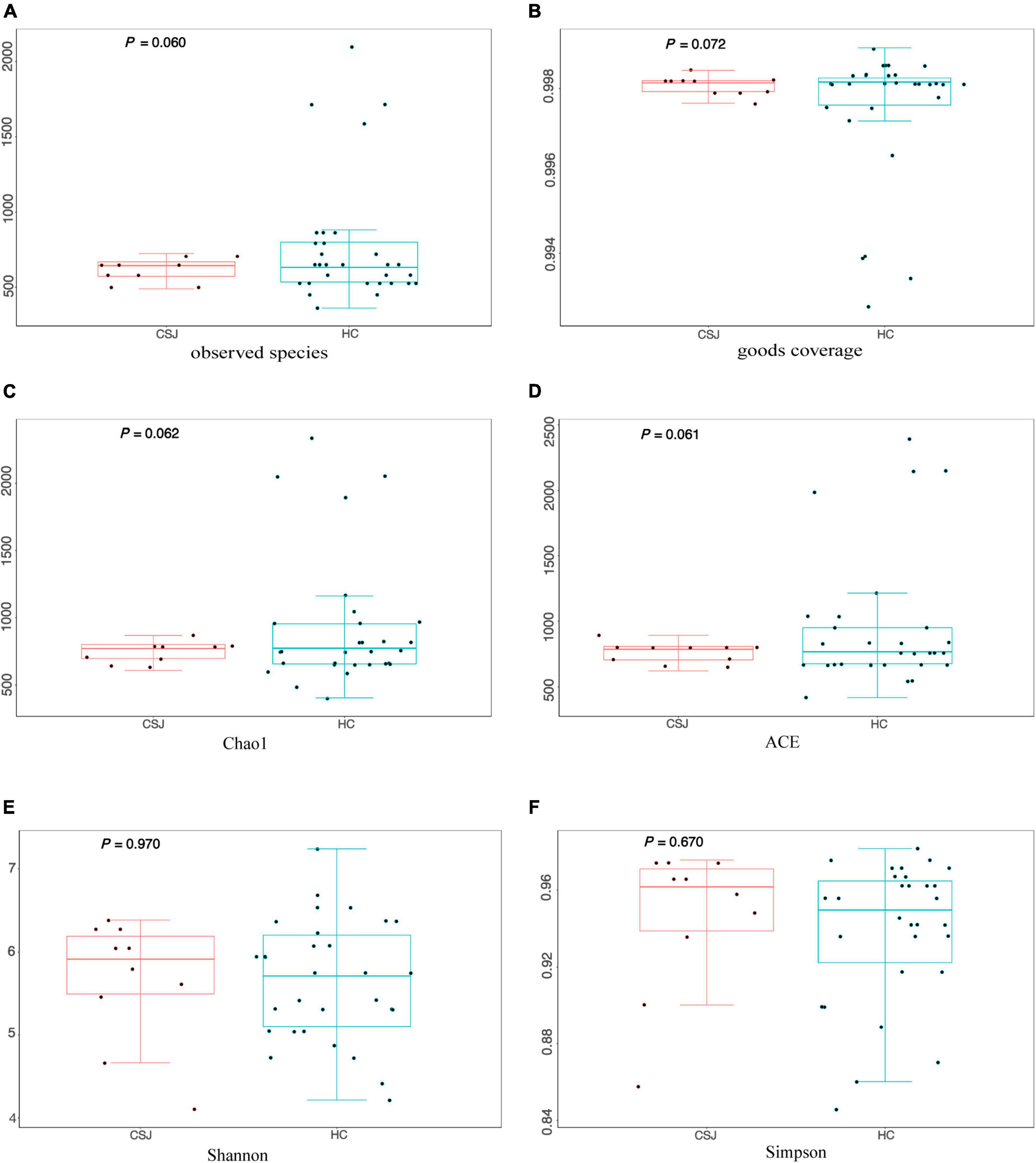
Figure 4. The α-diversity analysis of intestinal microbiota was evaluated by six metrics: (A) Observed Species index (Z = –1.55, P = 0.060); (B) Good’s coverage index (Z = –1.47, P = 0.072); (C) Chao1 index (Z = –1.54, P = 0.062); (D) ACE index (Z = –1.54, P = 0.061); (E) Shannon index (t(38) = 0.69, P = 0.970); (F) Simpson index (Z = –1.50, P = 0.670).
3.3.2. β-Diversity analysis
β-Diversity was used to assess the diversity of intestinal microbiota communities based on weighted UniFrac distances and PCoA analysis. It had trend to reach the significant difference in β-diversity between two groups (Z = −1.30, P = 0.096) (Figure 5A). Meanwhile, the PCoA results indicated that samples from each group had a species-specific heterogeneous distribution, suggesting that the diversity of the intestinal microbiota community was different between the CSJ and HC groups (Figure 5B).
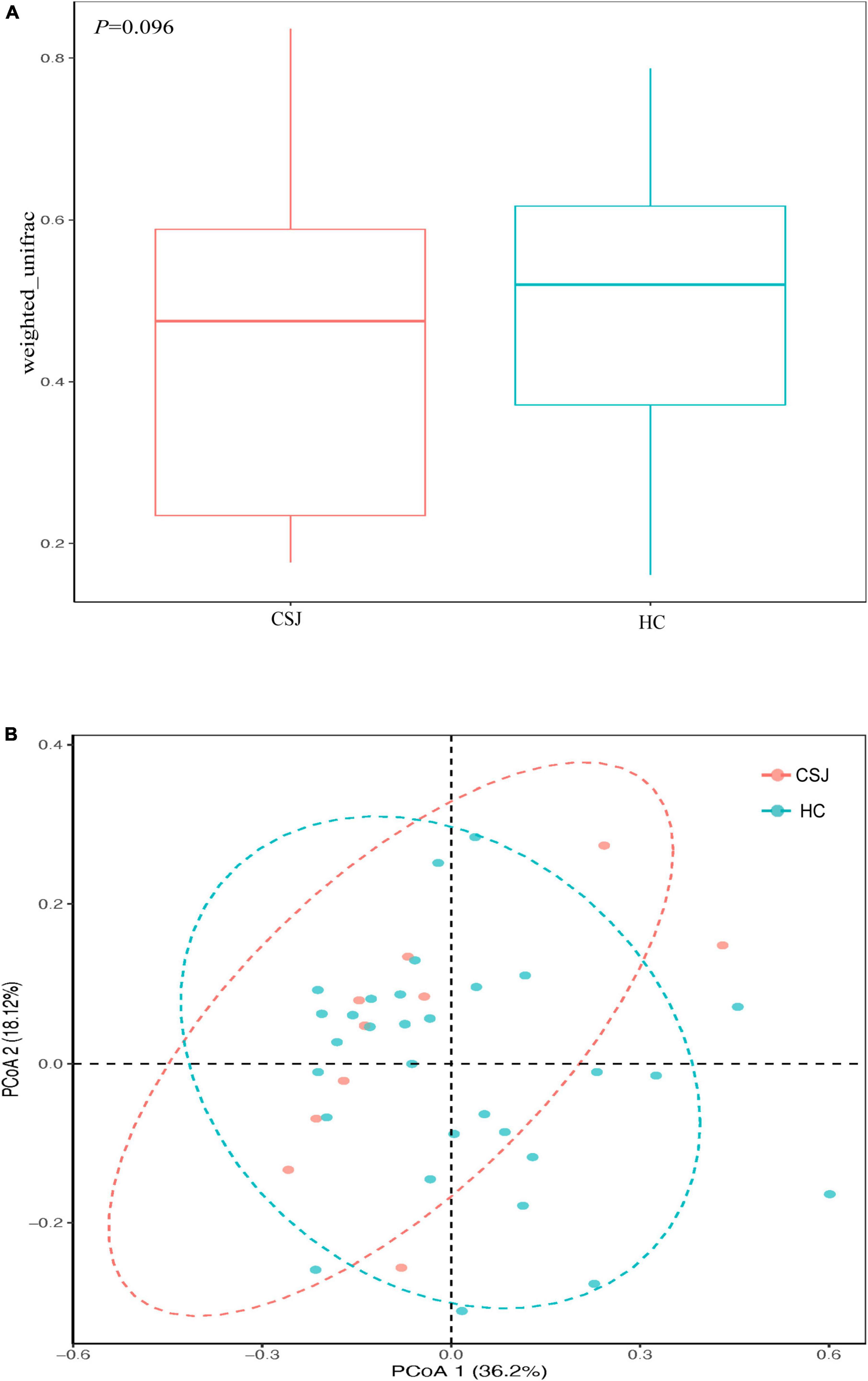
Figure 5. The β-diversity analysis of intestinal microbiota was analyzed based on weighted UniFrac (Z = –1.30, P = 0.096) (A) and PCoA (B) between the CSJ group and HC group.
3.4. Differences of microbiota genera in the CSJ and HC groups
Linear discriminant analysis effect size was used to further explore the microbiota genera that differed significantly between the two groups. The abundance of more than 20 microbiota differed, however, some of these exhibited too low abundance at the family level to perform a clinical function. Combined with their relative abundance, Prevotella 7, Alloprevotella, and Holdemanella genera were significantly higher in the CSJ group than in the HC group (Figure 6A). We also did ROC analysis to explore the diagnostic efficiency of the Prevotella 7, Alloprevotella, and Holdemanella genera by SPSS. The results showed that the area under the curve (AUC) of Prevotella 7, Alloprevotella, and Holdemanella genera was 0.779, 0.769, and 0.840, respectively (Figures 6B–D), which indicated a potential value in non-invasive diagnosis of chronic schistosomiasis japonica-induced fibrosis.
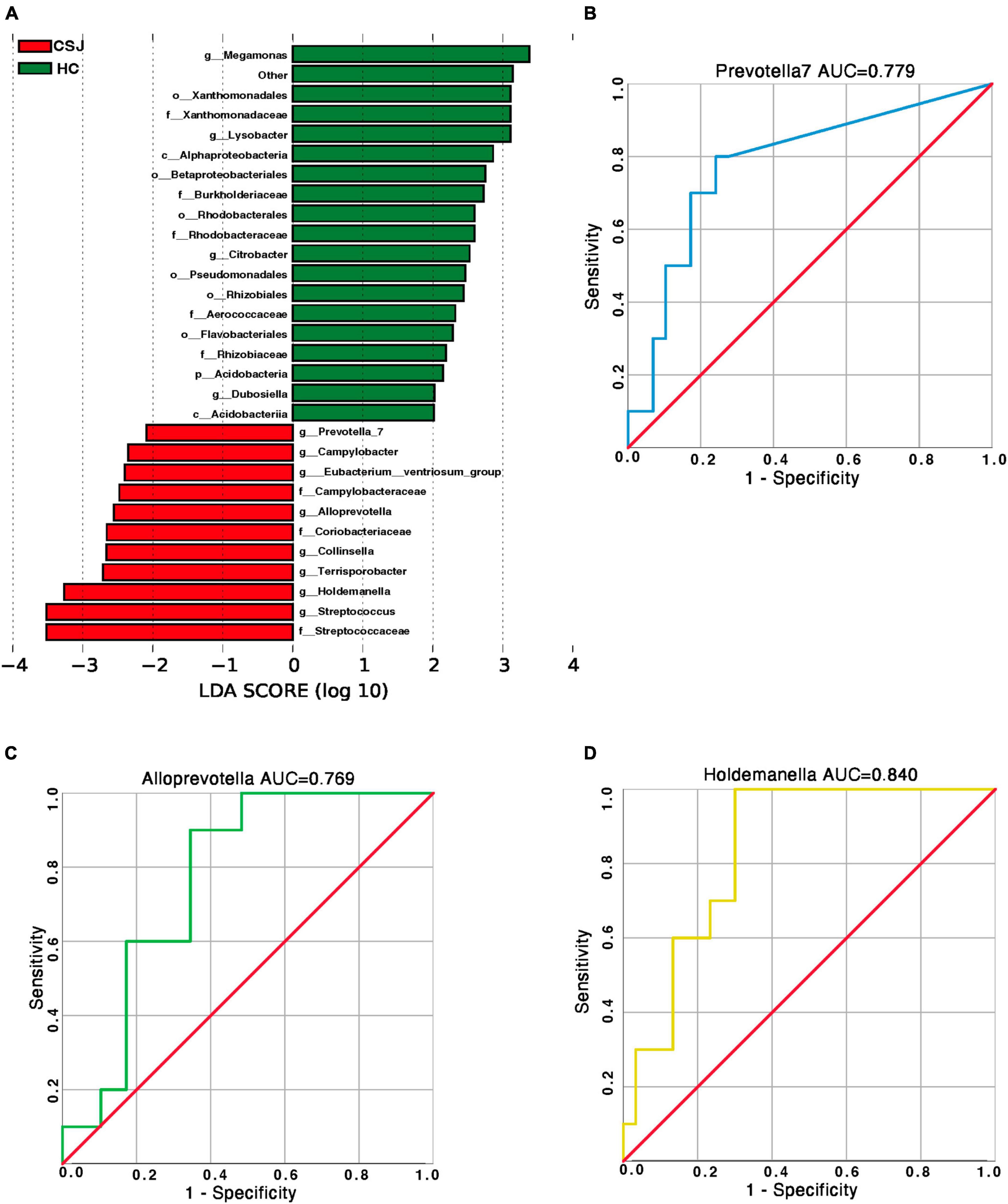
Figure 6. Species-specific differences in the intestinal microbiota between CSJ group and HC group. (A) The histogram of LDA scores showed the significantly different abundance of intestinal microbiota between the CSJ group and HC group. (B–D) The ROC analysis was done to predict the diagnostic efficiency of Prevotella 7, Alloprevotella, and Holdemanella genera for CSJ, and the AUC of Prevotella 7, Alloprevotella, and Holdemanella genera was 0.779, 0.769, and 0.840, respectively.
3.5. The functional prediction of different microbiota genera
Kyoto Encyclopedia of Genes and Genome and COG analyses were used to predict the function of the different intestinal microbiota in patients with chronic schistosomiasis japonica. KEGG analysis revealed that the Replication and Repair, and Translation pathways were significantly higher in the CSJ than in the HC groups (Figure 7A). COG analysis identified enrichment in the translation ribosomal structure and biogenesis and defense mechanisms after S. japonicum infection (Figure 7B).
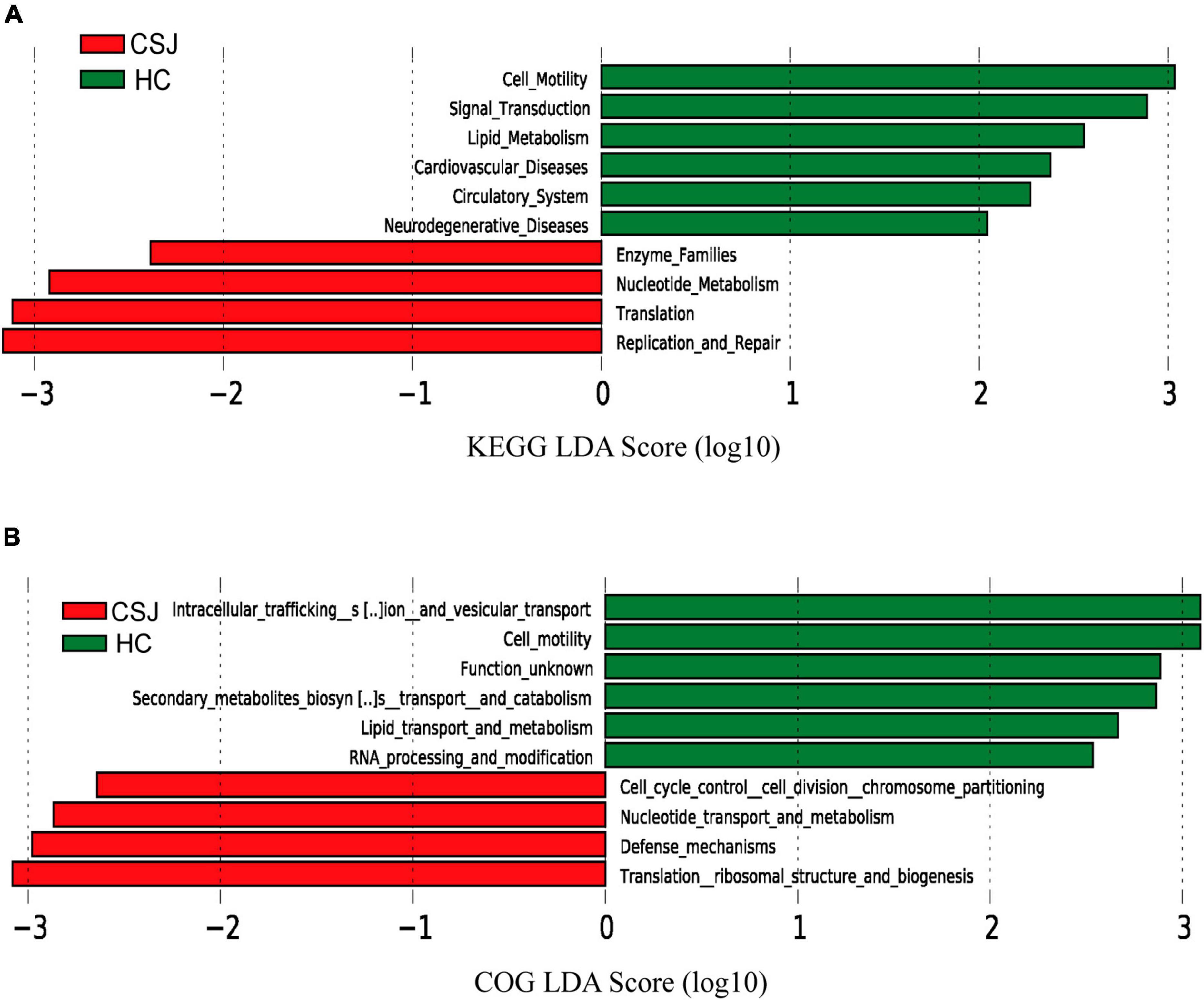
Figure 7. The functional prediction of different intestinal microbiota between CSJ group and HC group. The histogram of LDA scores showed the function of different intestinal microbiota between the two groups based on KEGG database (A) and COG database (B).
4. Discussion
Schistosomiasis is a global disease that remains a significant threat to public health. S. japonicum is largely endemic in China, especially in the Dongting Lake District, negatively impacting human health and causing economic loss (Li et al., 2020; Wang et al., 2021). The current study analyzed the intestinal microbiota of the residents of Qingshan Island and is the first to identify the characteristics of intestinal microbiota among patients with chronic schistosomiasis japonica.
In our study, composition and abundance of intestinal microbiota at the phylum and family levels were different between two groups. The α and β diversity results showed that CSJ group had low diversity of species of the intestinal microbiome. Prevotella 7, Alloprevotella, and Holdemanella genera were markedly increased during the development of chronic schistosomiasis japonica. Moreover, the AUC of Prevotella 7, Alloprevotella, and Holdemanella genera was 0.779, 0.769, and 0.840, respectively, which indicated that the three intestinal genera had a potential value in diagnosis of chronic schistosomiasis japonica-induced fibrosis.
Both Prevotella 7 and Alloprevotella genera belong to the Prevotellaceae family, a Gram-negative obligate anaerobe that is a common component of intestinal microbiota. While these bacteria help digest protein and carbohydrate foods and are often defined as probiotics (Yeoh et al., 2022), they are also associated with chronic inflammatory conditions and intestinal diseases, such as ulcerative colitis (Ley, 2016). A recent study found that Prevotellaceae abundance was reduced in hepatitis B patients who developed cirrhosis (Li et al., 2022). Another study reported that Prevotellaceae family and Prevotella genus were significantly increased and considered as biomarkers in antituberculosis drugs-induced hepatotoxicity (Wu et al., 2022). Chen et al. (2022) verified that mosapride could reduce intestinal bacterial translocation by regulating the intestinal microbiota (main Alloprevotella) in rats with hepatic cirrhosis. Holdemanella genera may exhibit anti-inflammatory activity (Pujo et al., 2021). A recent study indicated that liver fibrosis was strongly correlated with the enrichment of Prevotella and Holdemanella genera (Kwan et al., 2022). Another study about severe acute kidney injury showed that amoxicillin treatment accelerated functional recovery and decreased kidney fibrosis after acute kidney injury by depleting Holdemanella genera (Gharaie et al., 2023). These findings suggest that the Prevotellaceae family and Holdemanella genera is closely associated with liver disease and may contribute to the development of chronic schistosomiasis japonica-induced fibrosis.
Kyoto Encyclopedia of Genes and Genome functional prediction analysis is an effective tool to study functional metabolic changes that help community samples to adapt to environmental changes, while COG is a common protein function classification database for prokaryotes that complements KEGG to reveal the functional composition of microbiota (Kanehisa and Goto, 2000; Galperin et al., 2019). Using these analyses, the Replication and Repair, and Defense Mechanism pathways were identified as the most prominent. S. japonicum-associated liver injury occurs as a result of repeated immune damage from the host granulomatous response to Schistosome eggs (Colley et al., 2014; Llanwarne and Helmby, 2021), which was consistent with the KEGG and COG results. These findings suggest that the intestinal microbiota plays an important role in liver damage repair.
The current study has some limitations. While Qingshan Island was chosen due to its high prevalence of S. japonicum infection, the collection of samples from this district alone may have introduced selection bias. In addition, the implementation of effective schistosomiasis control measures in China and low S. japonicum-associated morbidity rates limited the availability of large samples.
5. Conclusion
This study was the first to explore differences in the intestinal microbiota of patients with chronic schistosomiasis japonica-induced fibrosis and healthy people from Qingshan Island. Prevotella 7, Alloprevotella, and Holdemanella genera were significantly increased during the development of chronic schistosomiasis japonica-induced fibrosis, indicating a potential value in non-invasive diagnosis of chronic schistosomiasis japonica-induced fibrosis. Further studies using larger and more heterogeneous populations were needed to evaluate the value of clinical application.
Data availability statement
The data presented in the study are deposited in the NCBI repository, https://www.ncbi.nlm.nih.gov/bioproject/PRJNA1018653, accession number PRJNA1018653.
Ethics statement
The studies involving humans were approved by the Ethics Committee of The Third Xiangya Hospital of Central South University. The studies were conducted in accordance with the local legislation and institutional requirements. The participants provided their written informed consent to participate in this study. Written informed consent was obtained from the individual(s) for the publication of any potentially identifiable images or data included in this article.
Author contributions
CG: Data curation, Formal analysis, Investigation, Methodology, Writing—original draft, Writing—review and editing. PZ: Funding acquisition, Writing—original draft, Writing—review and editing. JL: Data curation, Investigation, Writing—review and editing. CZ: Data curation, Investigation, Writing—review and editing. ZY: Data curation, Investigation, Writing—review and editing. YZ: Data curation, Investigation. YL: Data curation, Investigation, Writing—review and editing. JZ: Resources, Writing—review and editing. YC: Resources, Writing—review and editing. YM: Conceptualization, Funding acquisition, Project administration, Supervision, Writing—review and editing.
Funding
The author(s) declare financial support was received for the research, authorship, and/or publication of this article. This work was supported by National Natural Science Foundation of China (grant number: 82100695 to PZ), the Key Research and Development Plan of Hunan Province (grant number: 2021SK2032 to YM), and Hunan Provincial Natural Science Foundation (grant number: 2022JJ40759 to PZ).
Acknowledgments
We would like to thank APTBIO for genome-sequencing services.
Conflict of interest
The authors declare that the research was conducted in the absence of any commercial or financial relationships that could be construed as a potential conflict of interest.
Publisher’s note
All claims expressed in this article are solely those of the authors and do not necessarily represent those of their affiliated organizations, or those of the publisher, the editors and the reviewers. Any product that may be evaluated in this article, or claim that may be made by its manufacturer, is not guaranteed or endorsed by the publisher.
References
Abdel Wahab, M. F., Ali, Q. M., Bergquist, N. R., Wei-Men, C., and Dong-Chuan, Q. (1992). The use of diagnostic ultrasound in schistosomiasis–attempts at standardization of methodology. Cairo Working Group. Acta Trop. 51, 45–63.
Belkaid, Y., and Harrison, O. J. (2017). Homeostatic immunity and the microbiota. Immunity 46, 562–576.
Bergquist, R., Zhou, X.-N., Rollinson, D., Reinhard-Rupp, J., and Klohe, K. (2017). Elimination of schistosomiasis: The tools required. Infect Dis Poverty 6:158.
Caporaso, J. G., Kuczynski, J., Stombaugh, J., Bittinger, K., Bushman, F. D., Costello, E. K., et al. (2010). QIIME allows analysis of high-throughput community sequencing data. Nat Methods 7, 335–336.
Chen, C., Guo, Q., Fu, Z., Liu, J., Lin, J., Xiao, K., et al. (2021). Reviews and advances in diagnostic research on Schistosoma japonicum. Acta Trop. 213:105743.
Chen, D., Xiong, J., Feng, H., Liu, Y., Xu, J., and Xu, H. (2022). The influence of mosapride on gut microbiota of carbon tetrachloride-induced cirrhosis rats based on 16S rRNA gene sequencing. Adv. Clin. Exp. Med. 31, 623–633. doi: 10.17219/acem/146320
Chen, M. G. (2014). Assessment of morbidity due to Schistosoma japonicum infection in China. Infect. Dis. Poverty 3:6.
Colley, D. G., Bustinduy, A. L., Secor, W. E., and King, C. H. (2014). Human schistosomiasis. Lancet 383, 2253–2264.
Edgar, R. C. (2010). Search and clustering orders of magnitude faster than BLAST. Bioinformatics 26, 2460–2461.
Edgar, R. C. (2013). UPARSE: highly accurate OTU sequences from microbial amplicon reads. Nat. Methods 10, 996–998. doi: 10.1038/nmeth.2604
Galperin, M. Y., Kristensen, D. M., Makarova, K. S., Wolf, Y. I., and Koonin, E. V. (2019). Microbial genome analysis: the COG approach. Brief Bioinform. 20, 1063–1070.
GBD 2016 DALYs and HALE Collaborators (2017). Global, regional, and national disability-adjusted life-years (DALYs) for 333 diseases and injuries and healthy life expectancy (HALE) for 195 countries and territories, 1990-2016: A systematic analysis for the Global Burden of Disease Study 2016. Lancet 390, 1260–1344. doi: 10.1016/S0140-6736(17)32130-X
Gharaie, S., Lee, K., Newman-Rivera, A. M., Xu, J., Patel, S. K., Gooya, M., et al. (2023). Microbiome modulation after severe acute kidney injury accelerates functional recovery and decreases kidney fibrosis. Kidney Int. 104, 470–491. doi: 10.1016/j.kint.2023.03.024
Ghezzi, L., Cantoni, C., Pinget, G. V., Zhou, Y., and Piccio, L. (2021). Targeting the gut to treat multiple sclerosis. J. Clin. Invest. 131, e143774.
Gui, Q.-F., Jin, H.-L., Zhu, F., Lu, H.-F., Zhang, Q., Xu, J., et al. (2021). Gut microbiota signatures in Schistosoma japonicum infection-induced liver cirrhosis patients: a case-control study. Infect. Dis. Poverty 10:43. doi: 10.1186/s40249-021-00821-8
Jiang, Y., Yuan, Z., Shen, Y., Rosa, B. A., Martin, J., Cao, S., et al. (2021). Alteration of the fecal microbiota in Chinese patients with Schistosoma japonicum infection. Parasite 28:1. doi: 10.1051/parasite/2020074
Jones, R. M., and Neish, A. S. (2021). Gut Microbiota in Intestinal and Liver Disease. Annu. Rev. Pathol. 16, 251–275.
Kanehisa, M., and Goto, S. (2000). KEGG: kyoto encyclopedia of genes and genomes. Nucleic Acids Res. 28, 27–30.
Kwan, S. Y., Jiao, J., Joon, A., Wei, P., Petty, L. E., Below, J. E., et al. (2022). Gut microbiome features associated with liver fibrosis in Hispanics, a population at high risk for fatty liver disease. Hepatology 75, 955–967. doi: 10.1002/hep.32197
Langille, M. G., Zaneveld, J., Caporaso, J. G., McDonald, D., Knights, D., Reyes, J. A., et al. (2013). Predictive functional profiling of microbial communities using 16S rRNA marker gene sequences. Nat. Biotechnol. 31, 814–821.
Lavelle, A., and Sokol, H. (2020). Gut microbiota-derived metabolites as key actors in inflammatory bowel disease. Nat. Rev. Gastroenterol. Hepatol. 17, 223–237. doi: 10.1038/s41575-019-0258-z
Ley, R. E. (2016). Gut microbiota in 2015: Prevotella in the gut: choose carefully. Nat. Rev. Gastroenterol. Hepatol. 13, 69–70. doi: 10.1038/nrgastro.2016.4
Li, F.-Y., Hou, X.-Y., Tan, H.-Z., Williams, G. M., Gray, D. J., Gordon, C. A., et al. (2020). Current status of schistosomiasis control and prospects for elimination in the dongting lake region of the People’s Republic of China. Front. Immunol. 11:574136. doi: 10.3389/fimmu.2020.574136
Li, R., Yi, X., Yang, J., Zhu, Z., Wang, Y., Liu, X., et al. (2022). Gut microbiome signatures in the progression of hepatitis B virus-induced liver disease. Front. Microbiol. 13:916061. doi: 10.3389/fmicb.2022.916061
Lin, D., Song, Q., Zhang, Y., Liu, J., Chen, F., Du, S., et al. (2021). Attenuates Hepatic and Intestinal Injuries and Modulates Gut Microbiota and Gene Expression Profiles in Mice Infected with. Front. Cell Dev. Biol. 9:766205. doi: 10.3389/fcell.2021.766205
Llanwarne, F., and Helmby, H. (2021). Granuloma formation and tissue pathology in Schistosoma japonicum versus Schistosoma mansoni infections. Parasite Immunol. 43:e12778. doi: 10.1111/pim.12778
Lozupone, C., Lladser, M. E., Knights, D., Stombaugh, J., and Knight, R. (2011). UniFrac: an effective distance metric for microbial community comparison. ISME J. 5, 169–172.
Magoè, T., and Salzberg, S. L. (2011). FLASH: fast length adjustment of short reads to improve genome assemblies. Bioinformatics 27, 2957–2963. doi: 10.1093/bioinformatics/btr507
Mao, C. (1990). Biology of schistosome and control of schistosomiasis. Beijing: People’s Health Press.
McManus, D. P., Bergquist, R., Cai, P., Ranasinghe, S., Tebeje, B. M., and You, H. (2020). Schistosomiasis-from immunopathology to vaccines. Semin. Immunopathol. 42, 355–371.
McManus, D. P., Dunne, D. W., Sacko, M., Utzinger, J., Vennervald, B. J., and Zhou, X.-N. (2018). Schistosomiasis. Nat. Rev. Dis. Primers 4:13.
Pacaud, M., Colas, L., and Brouard, S. (2021). Microbiota and immunoregulation: A focus on regulatory B lymphocytes and transplantation. Am. J. Transplant. 21, 2341–2347. doi: 10.1111/ajt.16522
Pujo, J., Petitfils, C., Le Faouder, P., Eeckhaut, V., Payros, G., Maurel, S., et al. (2021). Bacteria-derived long chain fatty acid exhibits anti-inflammatory properties in colitis. Gut 70, 1088–1097. doi: 10.1136/gutjnl-2020-321173
Ramirez, J., Guarner, F., Bustos Fernandez, L., Maruy, A., Sdepanian, V. L., and Cohen, H. (2020). Antibiotics as major disruptors of gut microbiota. Front. Cell Infect. Microbiol. 10:572912. doi: 10.3389/fcimb.2020.572912
Richter, J., Hatz, C., and Häussinger, D. (2003). Ultrasound in tropical and parasitic diseases. Lancet 362, 900–902.
Schloss, P. D., Westcott, S. L., Ryabin, T., Hall, J. R., Hartmann, M., Hollister, E. B., et al. (2009). Introducing mothur: open-source, platform-independent, community-supported software for describing and comparing microbial communities. Appl. Environ. Microbiol. 75, 7537–7541. doi: 10.1128/AEM.01541-09
Schneeberger, P. H. H., Coulibaly, J. T., Panic, G., Daubenberger, C., Gueuning, M., Frey, J. E., et al. (2018). Investigations on the interplays between Schistosoma mansoni, praziquantel and the gut microbiome. Parasit. Vectors 11:168. doi: 10.1186/s13071-018-2739-2
Segata, N., Izard, J., Waldron, L., Gevers, D., Miropolsky, L., Garrett, W. S., et al. (2011). Metagenomic biomarker discovery and explanation. Genome Biol. 12:R60.
Steinmann, P., Keiser, J., Bos, R., Tanner, M., and Utzinger, J. (2006). Schistosomiasis and water resources development: systematic review, meta-analysis, and estimates of people at risk. Lancet Infect. Dis. 6, 411–425. doi: 10.1016/S1473-3099(06)70521-7
Utzinger, J., Raso, G., Brooker, S., De Savigny, D., Tanner, M., Ornbjerg, N., et al. (2009). Schistosomiasis and neglected tropical diseases: towards integrated and sustainable control and a word of caution. Parasitology 136, 1859–1874. doi: 10.1017/S0031182009991600
Wang, W., Bergquist, R., King, C. H., and Yang, K. (2021). Elimination of schistosomiasis in China: Current status and future prospects. PLoS Negl. Trop. Dis. 15:e0009578. doi: 10.1371/journal.pntd.0009578
Wu, S., Wang, M., Zhang, M., and He, J. Q. (2022). Metabolomics and microbiomes for discovering biomarkers of antituberculosis drugs-induced hepatotoxicity. Arch. Biochem. Biophys. 716:109118. doi: 10.1016/j.abb.2022.109118
Wu, W., Feng, A., and Huang, Y. (2015). Research and control of advanced schistosomiasis japonica in China. Parasitol. Res. 114, 17–27. doi: 10.1007/s00436-014-4225-x
Yan, L., Hai, C., Jia, C., and Yue, H. (2003). Schistosomiasis situation changes after migration for 3 years in Qingtan town. Chin. J. Schistosomiasis Control 15: 477.
Yang, G., Wei, J., Liu, P., Zhang, Q., Tian, Y., Hou, G., et al. (2021). Role of the gut microbiota in type 2 diabetes and related diseases. Metabolism 117:154712.
Keywords: chronic schistosomiasis japonica, liver fibrosis, intestinal microbiota, 16S rRNA gene sequence, Prevotella 7, Alloprevotella, Holdemanella
Citation: Guo C, Zhang P, Li J, Zhou C, Yang Z, Zhang Y, Luo Y, Zhou J, Cai Y and Ming Y (2023) The characteristics of intestinal microbiota in patients with chronic schistosomiasis japonica-induced liver fibrosis by 16S rRNA gene sequence. Front. Microbiol. 14:1276404. doi: 10.3389/fmicb.2023.1276404
Received: 11 August 2023; Accepted: 19 September 2023;
Published: 03 October 2023.
Edited by:
Maurizio Sanguinetti, Catholic University of the Sacred Heart, ItalyReviewed by:
Carlos Pérez-Monter, National Institute of Medical Sciences and Nutrition Salvador Zubirán, MexicoXiaofeng Shan, Jilin Agricultural University, China
Copyright © 2023 Guo, Zhang, Li, Zhou, Yang, Zhang, Luo, Zhou, Cai and Ming. This is an open-access article distributed under the terms of the Creative Commons Attribution License (CC BY). The use, distribution or reproduction in other forums is permitted, provided the original author(s) and the copyright owner(s) are credited and that the original publication in this journal is cited, in accordance with accepted academic practice. No use, distribution or reproduction is permitted which does not comply with these terms.
*Correspondence: Yingzi Ming, myz_china@aliyun.com
†These authors have contributed equally to this work