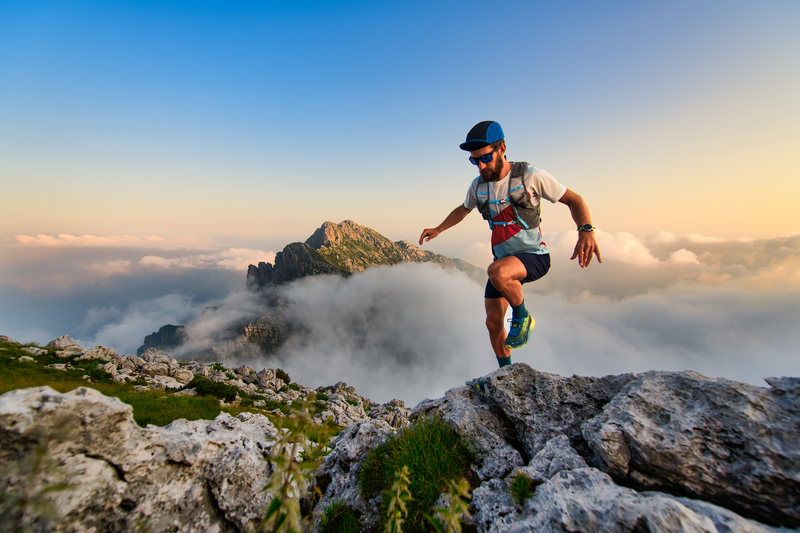
94% of researchers rate our articles as excellent or good
Learn more about the work of our research integrity team to safeguard the quality of each article we publish.
Find out more
ORIGINAL RESEARCH article
Front. Microbiol. , 05 January 2024
Sec. Microbial Symbioses
Volume 14 - 2023 | https://doi.org/10.3389/fmicb.2023.1257903
Background: Non-alcoholic fatty liver disease (NAFLD) is the most prevalent cause of chronic liver disease worldwide, and gut microbes are associated with the development and progression of NAFLD. Despite numerous studies exploring the changes in gut microbes associated with NAFLD, there was no consistent pattern of changes.
Method: We retrieved studies on the human fecal microbiota sequenced by 16S rRNA gene amplification associated with NAFLD from the NCBI database up to April 2023, and re-analyzed them using bioinformatic methods.
Results: We finally screened 12 relevant studies related to NAFLD, which included a total of 1,189 study subjects (NAFLD, n = 654; healthy control, n = 398; obesity, n = 137). Our results revealed a significant decrease in gut microbial diversity with the occurrence and progression of NAFLD (SMD = −0.32; 95% CI −0.42 to −0.21; p < 0.001). Alpha diversity and the increased abundance of several crucial genera, including Desulfovibrio, Negativibacillus, and Prevotella, can serve as an indication of their predictive risk ability for the occurrence and progression of NAFLD (all AUC > 0.7). The occurrence and progression of NAFLD are significantly associated with higher levels of LPS biosynthesis, tryptophan metabolism, glutathione metabolism, and lipid metabolism.
Conclusion: This study elucidated gut microbes relevance to disease development and identified potential risk-associated microbes and functional pathways associated with NAFLD occurrence and progression.
Non-alcoholic fatty liver disease (NAFLD) is metabolic stress–induced liver damage characterized by excess triglyceride accumulation in the liver without consumption of excessive alcohol (Iizuka et al., 2018; Jiang et al., 2022). It is the dominant cause of chronic liver disease worldwide, with a prevalence of approximately 25% (Lu et al., 2021). NAFLD encompasses a spectrum ranging from non-alcoholic simple fatty liver (NAFL) to non-alcoholic steatohepatitis (NASH), then progress to liver cirrhosis, fibrosis, and hepatocellular carcinoma (HCC) (Li et al., 2023). Although NAFLD carries a relatively benign prognosis and is mostly asymptomatic, up to 50% of affected individuals will develop NASH, cirrhosis, and liver fibrosis (Sunny et al., 2011; Zhang T. et al., 2019). Patients may potentially develop liver cancer and bladder cancer, due to the mediation of insulin resistance (Tarantino et al., 2021), which could ultimately increase liver-related morbidity and mortality (Abdul-Hai et al., 2015). However, the pathogenesis of NAFLD, specifically the disease progression to NASH, remains incompletely understood. This information is crucial to reverse and prevent the occurrence and development of the disease (Navarro et al., 2015). To date, liver biopsy is the gold standard for the diagnosis of NAFLD, but it cannot be applied at a large scale due to its associated risk (Zhang et al., 2023). Hence, accurate and precise non-invasive biomarkers are needed to detect NAFLD and its progression.
Accumulating evidence has shown that the gut microbiome is involved in the pathogenesis and progression of NAFLD (Boursier et al., 2016; Ponziani et al., 2019; Saeedi et al., 2020; Zhang et al., 2021). Consistently, gut microbial dysbiosis appears to modify susceptibility to NAFLD. Lee et al. (2020) found a significant decrease in the diversity of microbiota in patients with biopsy-proven NAFLD compared with non-NAFLD controls. In contrast, Jiang et al. (2015) reported no significant difference in the microbiota biodiversity between patients with NAFLD and healthy controls in their study. Lang et al. (2021) found that the degree of inflammation and stages of fibrosis are associated with low abundances of Faecalibacterium, Bacteroides, and Prevotella. And Boursier et al. (2016) reported that the abundance of Bacteroides is significantly increased in patients with NASH, whereas the abundance of Prevotella is decreased. The results imply that gut flora characteristics may vary across different stages of NAFLD. However, it is worth noting that these studies were conducted exclusively in European regions, and due to the strong correlation between ethnicity and the gut microbiota (Human Microbiome Project Consortium, 2012), the composition of the intestinal flora in non-European populations may differ significantly. The exact composition of the gut microbiota in patients with NAFLD, as well as the alterations in the gut microbiota composition across early and advanced stages of the disease, are currently unknown.
In the present study, we obtained 16S ribosomal RNA (rRNA) gene amplicon sequencing data associated with the gut microbiota from publicly available databases for all patients with NAFLD who met our screening criteria. We submitted the data to a rigorous biostatistical analysis to explore changes in the diversity and composition of the gut microbiota in patients with NAFLD as the disease progresses, and to identify the key microbial and functional pathways associated with NAFLD.We aimed to elucidate the relationship between alterations in the intestinal microbiome and the occurrence and development of NAFLD and to provide references for future screening of non-invasive biomarkers for the NAFLD diagnosis.
We identified studies of human fecal flora associated with NAFLD by 16S rRNA gene sequencing up to April 2023 from the National Center for Biotechnology Information (NCBI) database (PubMed1 and BioProject.2) We developed out search strategy based on keywords, medical subject headings (MeSH) terms, and synonyms (Supplementary Table S1). We selected studies based on the following criteria: (1) The study groups contained patients with NAFLD and controls. The controls included healthy controls and patients with obesity but not NAFLD. The diagnostic methods and criteria for each study regarding the NAFLD status and the control group are shown in Supplementary Table S2. “Pre-progression” indicates the pre-progressive state of NAFLD-related diseases, and “post-progression” indicates the post-progressive state of NAFLD-related diseases. (2) The sample type was fecal samples or rectal swabs. (3) We excluded randomized controlled trials. (4) The sample sequencing method was 16S rRNA gene amplicon sequencing and the sequencing data were publicly available. We obtained raw 16S rRNA gene sequences and associated metadata from publicly available databases or directly from the authors. We excluded studies involving animal experiments or in vitro investigations, as well as reviews, meta-analyses, comments, letters, poster abstracts, and those with a sample size of fewer than three individuals in the case or control groups.
We download the Sequence Read Archive (SRA) files from NCBI and converted them to raw FASTQ files by using fastq-dump in the SRA Toolkit.3 We imported these FASTQ files into Quantitative Insights in Microbial Ecology (QIIME) version 2–2021.2 for processing and bioinformatics analysis.4 We processed the raw sequences to remove primers by using “q2-cutadapt.”5 We trimmed primer sequences, chimeras, and low-quality read ends with a quality score below 35 by using the Divisive Amplicon Denoising Algorithm 2 (DADA2) plugin for QIIME 2. The reads were trimmed using parameters in the DADA2 plugin carried out for each study, which can be found in Supplementary Table S3. We used DADA2 (Callahan et al., 2016), implemented in QIIME2, to model errors, to filter the raw FASTQ files, and to remove chimeras. After DADA2 denoising to generate a table of amplicon sequences variants (ASVs) and representative sequences, we filtered samples with a frequency of less than 10 in the feature table by using the “q2-feature-table filter-features” so that low-quality samples did not affect the results of downstream analysis. We annotated the species for each representative ASV by using a pre-trained plain Bayesian classifier (Bokulich et al., 2018) based on the latest version of the SILVA 138 reference database (clustered at 99% similarity). To minimize the effects of different sequencing platforms, sequencing regions, and primer amplifications, we combined the feature tables and representative sequences generated from each separate research by using the “qiime feature-table merge” and “qiime feature-table merge-seqs” commands in QIIME 2. We constructed a reliable phylogenetic tree by inserting the sequences into the SILVA 128 reference tree using the q2-fragment-insertion plugin, through the SATé-enabled phylogenetic placement (SEPP) algorithm, which is commonly used for meta-analyses of microbiome data (Janssen et al., 2018). For the merged feature table, we employed normalization methods to process the large disparity in the sequencing depths of different studies: We rarefied samples to 2,000 and converted them to a centered log-ratio (CLR) with the R package mecodev (Liu et al., 2021).
We imported files of the filtered ASV feature table, phylogenetic tree, metadata, representative sequences, and taxonomic classifications into RStudio 4.2.1 (RStudio, Inc., Boston, MA, USA). Using the R package microeco (Liu et al., 2021), we eliminated ASVs with taxonomic assignments labeled as “mitochondria” or “chloroplast” and created an object of microtable class for subsequent analysis. To mitigate the impact of sequencing depth on diversity measurements, we performed random re-sampling of all samples in the ASV abundance matrix based on the lowest sequencing depth andnormalized the ASV feature table. We employed a sampling-based ASV analysis and used the R package vegan to calculate the alpha diversity indices, including richness (observed species, Chao1, and abundance-based coverage estimator [ACE]), diversity (Shannon, Simpson, Invsimpson, and Fisher), and Faith’s phylogenetic diversity (PD) to evaluate the overall structure of the gut microbiota.6 We determined beta diversity within and between studies with principal coordinates analysis (PCoA) based on the unweighted UniFrac distance and the weighted UniFrac distance, and we employed permutational multivariate analysis of variance (PERMANOVA) with 999 replications on each distance metric. Meanwhile, we calculated bacterial beta diversity with PCoA based on the Bray–Curtis distance to show the species diversity for each study. Besides, based on the distribution of ASVs, we constructed a Venn diagram to show the ASV intersections among different groups through the R package VennDiagram.7
We performed bacterial taxonomic analyses and comparisons between groups based on the level of bacterial phylum, class, order, family, and genus with the Wilcoxon rank-sum test. We applied the linear discriminant analysis effect size (LEfSe) method (Segata et al., 2011) to analyze fecal microbial characterization between cases and controls, and we evaluated the effect size of each feature by linear discriminant analysis (LDA, with an LDA score [log10] = 3 as the cut-off value).
We used the R package Tax4Fun2 (Wemheuer et al., 2020) to predict the Kyoto Encyclopedia of Genes and Genomes (KEGG) functional pathways related to the microbial community based on 16S rRNA gene sequencing data classified on the SILVA 99Ref database. We compared between groups based on with functional abundance of bacterial communities to obtain functional pathways that differed significantly between the groups and visualized enrichment through the R package ggplot2.8 Ultimately, we used the area under the curve (AUC) of the receiver operating characteristic (ROC) curve to evaluate the predictive effectiveness of the alpha diversity indices and differential microbial taxonomy.
We could divide patients with NAFLD into the pre-progressive period or the post-progressive period. The pre-progressive period of NAFLD includes NAFL and NAFLD (assuming the study is a subgroup of NAFLD progress). The post-progressive period of NAFLD includes NASH, NAFLD fibrosis, and NAFLD cirrhosis. We compared gut microbial diversity, the abundance of bacterial taxonomy, and KEGG functional pathways between patients with NAFLD and healthy controls, patients with NAFLD and patients with obesity, or between the pre-progressive and post-progressive periods of NAFLD. We used the R package meta to generate the forest plots of the comparisons of the alpha diversity.9 We explored heterogeneity by calculating I2 and interpreted it as follows: < 25% indicates low heterogeneity, 25–45% indicates moderate heterogeneity, and > 45% indicates significant heterogeneity. If there was significant heterogeneity between studies (I2 > 45%), we applied the random-effect model; otherwise, we applied the fixed-effects model. We used the standardized mean difference (SMD) and the corresponding 95% credible interval (CI) evaluate the results. We conducted ROC curve analysis with GraphPad Prism 9.4 (GraphPad Software, Inc., San Diego, CA, USA). We considered a model with an AUC of >0.7 to be acceptable or better. All tests were two-sided with a value of p of 0.05 set as the threshold for significance.
We found a total of 1,590 items from PubMed and 152 items from BioProject based on the search criteria, among which we included 12 NAFLD-related studies for subsequent analysis (Jiang et al., 2015; Wang et al., 2017; Caussy et al., 2019; Zhang Y. et al., 2019; Dong et al., 2020; Monga Kravetz et al., 2020; Ahmed et al., 2021; Baumann et al., 2021; Kordy et al., 2021; Lang et al., 2021; Pan et al., 2021; Liang et al., 2022) (Figure 1). Most participants included in the studies were diagnosed with NAFLD, while four of the included studies (Caussy et al., 2019; Dong et al., 2020; Kordy et al., 2021; Pan et al., 2021) included participants diagnosed with NAFL, NASH, and liver cirrhosis or fibrosis associated with NAFLD. These 12 studies were conducted in five countries, including five from China, four from the United States, two from Europe (one from Germany and one from Austria), and one from Canada. There were containing a total of 1,189 individual samples (NAFLD, n = 654; healthy control, n = 398; obesity, n = 137). Table 1 provides comprehensive details of the characteristics of included studies.
Figure 1. Flow chart of the selection procedure. A comprehensive search of the NCBI databases (PubMed and Bioproject) up to April 2023 yielded 2029 records related to NAFLD. After eliminating non-intestinal flora studies, non-NAFLD-related studies, animal or in vitro experiments, meta-analyses, letters, reviews, or comments, a total of 161 studies or projects were assessed for eligibility. Subsequently, further screening was conducted to exclude non-fecal samples or non-rectal swabs, non-16S rRNA gene sequencing, lacking controls, raw data not available, not distinguishing between NAFLD and control, and duplicates. Ultimately, the inclusion of 12 studies for analysis.
As estimated by the Chao1, Shannon, and PD indexes, the gut microbial alpha diversity was reduced significantly in patients with NAFLD compared with healthy controls (all p < 0.01; Figure 2). The pooled estimate of all alpha diversity indices showed a significant decrease among patients with NAFLD (SMD = −0.32; 95% CI −0.42 to −0.21; p < 0.001; Figure 2). Furthermore, the pooled estimate showed a significant decrease in alpha diversity among patients with NAFLD compared with patients with obesity (p < 0.001; Supplementary Figure S1). As NAFLD progressed, there was a significant down regulation of alpha diversity (SMD = −0.39; 95% CI, −0.53 to −0.24; p < 0.001; Figure 3). When compared with healthy controls, the pooled estimates showed a significant decline in alpha diversity regardless of whether NAFLD was in the pre-progressive period (SMD = −0.27; 95% CI −0.39 to−0.16; p < 0.001; Figure 4) or the post-progressive period (SMD = −0.57; 95% CI −0.73 to−0.41; p < 0.001; Figure 5).
Figure 2. The forest plot shows the outcomes of a meta-analysis performed on the alpha-diversity of each parameter in both the NAFLD and healthy control groups. As estimated by the Chao1, Shannon, and PD indexes, the gut microbial alpha diversity was reduced significantly in NAFLD patients, compared with healthy controls. The pooled results showed a significant decrease in alpha diversity in NAFLD compared to the healthy control group.
Figure 3. The forest plot shows the outcomes of a meta-analysis performed on the alpha-diversity of each parameter in both the post-NAFLD and pre-NAFLD groups. The pooled results showed a significant decrease in alpha diversity in post-progressive period of NAFLD compared to the post-progressive period of NAFLD.
Figure 4. The forest plot shows the outcomes of a meta-analysis performed on the alpha-diversity of each parameter in both the post-NAFLD and healthy control groups. The pooled results showed a significant decrease in alpha diversity in post-progressive period of NAFLD compared to the healthy control groups.
Figure 5. The forest plot shows the outcomes of a meta-analysis performed on the alpha-diversity of each parameter in both the pre-NAFLD and healthy control groups. The pooled results showed a significant decrease in alpha diversity in pre-progressive period of NAFLD compared to the healthy control groups.
We aggregated the beta diversity analyses of the included studies according to the unweighted and weighted UniFrac distances. There were significant differences between samples when they were grouped by study or cases of NAFLD (PERMANOVA p < 0.001; Figures 6, 7). Furthermore, the PERMANOVA results based on unweighted and weighted UniFrac distances showed that the pseudo-F values in the different study groups (pseudo-F = 8.111, 7.781; p < 0.001, p < 0.001) were higher than in the different groups (Healthy Control and NAFLD pseudo-F = 1.984, 2.398; p < 0.001, p = 0.002; pre-progressive and post-progressive NAFLD pseudo-F = 2.131, 1.985; p = 0.002, p = 0.017). The interstudy variation outweighed the effect of NAFLD. We tested the effect of NAFLD for each study separately on the community composition by employing PCoA of multiple distance metrics and PERMANOVA for statistical testing. Except for the study by Zhang Y. et al. (2019), there was no significant influence of NAFLD on microbial community composition across all distance metrics in the remaining studies (all p > 0.05). However, in all other studies, NAFLD exhibited a significant effect on microbial composition in specific distance metrics (p < 0.05, PERMANOVA; Table 2; Supplementary Figure S2). In addition, we conducted PCoA based on the Bray–Curtis distance to evaluate the similarities in the gut microbiome composition among the groups in all included studies. Based on PERMANOVA, the results reported by Caussy et al. (2019), Zhang Y. et al. (2019), Dong et al. (2020), Kordy et al. (2021), and Lang et al. (2021) showed that the gut microbiome composition differed significantly between patients with NAFLD and healthy controls (p < 0.05). Of note, there were also significant differences in the gut microbial composition between pre-progressive and post-progressive NAFLD according to the results of Caussy et al. (2019), Dong et al. (2020), and Kordy et al. (2021) (p < 0.05).
Figure 6. The PCoA plots showing unweighted UniFrac and weighted UniFrac distance as samples colored by study. Results of PERMANOVA test for significance between studies are shown on each plot.
Figure 7. The PCoA plots showing unweighted UniFrac and weighted UniFrac distance as samples colored by group. Results of PERMANOVA test for significance between groups are shown on each plot. (A) Based on unweighted and weighted UniFrac distances, the PERMANOVA results reveal significant differences in beta diversity between the NAFLD group and the healthy control group (pseudo-F = 1.984, 2.398; P < 0.001, P = 0.002). (B) Based on unweighted and weighted UniFrac distances, the PERMANOVA results demonstrate significant differences in beta diversity between the pre-progressive and post-progressive stages of NAFLD (pseudo-F = 2.131, 1.985; P = 0.002, P = 0.017). (C) Based on unweighted and weighted UniFrac distances, the PERMANOVA results reveal significant differences in beta diversity between the obesity group and the NAFLD group (pseudo-F = 10.222, 17.770; P < 0.001, P < 0.001).
The Venn diagrams showing ASV intersections between groups indicated that there were differences in the gut microbial composition between groups based on the ASV levels (Supplementary Figure S3).
We analyzed the phylogenetic profiles of intestinal microorganisms between the NAFLD and healthy control groups. The average compositions and the top 10 highest relative abundances of the bacterial community in all groups at the phylum and genus levels are shown in Supplementary Figures S4, S5. The results from the studies of by Caussy et al. (2019), Dong et al. (2020), and Lang et al. (2021) showed a trend toward an increased abundance of Firmicutes and a decreased abundance of Bacteroidetes in patients with NAFLD; however, the results from the studies by Zhang Y. et al. (2019), and Kordy et al. (2021) showed a declining trend in Firmicutes in patients with NAFLD, while Bacteroidetes showed an increasing trend (Supplementary Figure S4). According to the results reported by Caussy et al. (2019), Zhang Y. et al. (2019), Dong et al. (2020), Kordy et al. (2021), and Lang et al. (2021), 18 genera including Lactobacillus, Desulfovibrio, Escherichia-Shigella, and Veillonella were significantly enriched, whereas 24 genera including Christensenellaceae_R-7_group, Lachnospiraceae_UCG-001, Lachnospira, and Ruminococcaceae_UCG-002 were significantly reduced in patients with NAFLD compared with healthy controls (all p < 0.05; Supplementary Figure S6).
Furthermore, we compared the phylogenetic profiles of the gut microbiota between NAFLD and NAFLD-related fibrosis or liver cirrhosis at the class (Supplementary Figure S7), family (Supplementary Figure S8), and genus (Supplementary Figure S9) levels. At the class level, six bacterial populations including Actinobacteria, Erysipelotrichia, and Methanobacteria, were significantly enriched, whereas only Bacilli was significantly reduced in patients with NAFLD compared with patients with fibrosis or liver cirrhosis (all p < 0.05; Supplementary Figure S7). At the family level, nine bacterial populations including Campylobacteraceae, Enterobacteriaceae, and Prevotellaceae were significantly enriched, whereas two bacterial populations, namely Bacteroidaceae and Peptostreptococcaceae, were significantly reduced in patients with NAFLD (all p < 0.05; Supplementary Figure S8). At the genus level, 18 bacterial populations including Alloprevotella, Escherichia-Shigella, and Prevotella_2 were significantly increased, whereas seven bacterial populations including Colidextribacter, Bacteroides, and Faecalibacterium were significantly decreased in the pre-progressive period of NAFLD compared with the post-progressive period of NAFLD (all p < 0.05; Supplementary Figure S9).
We used LEfSe to determine the maximum difference in the microbial structures in patients with NAFLD, versus those in healthy controls or patients with obesity. Then random forest methods to validate the LEfSe results. We screened the 12 included studies separately for dominant biomarkers by employing LEfSe. Based on an LDA score ≥ 3 and p < 0.05 and random forest selection, we found 22 genera including Desulfovibrio, Prevotella_9, Dorea, Escherichia-Shigella, and Negativibacillus that were significantly enriched (p < 0.05; Figure 8), while 46 genera including Faecalibacterium, Lachnospira, Catenibacterium, Lactobacillus, and UCG-002 were significantly reduced in patients with NAFLD (p < 0.05; Figure 9), compared with healthy controls or patients with obesity. More details of the LEfSe and random forest analyses are shown in Supplementary Figures S10, S11.
Figure 8. The integration of LEFSE-based and random forestation methods identifies crucial genera that significantly increase in the occurrence or development of NAFLD. Based on the linear discriminant analysis (LDA score ≥ 3 and p < 0.05) and Random Forests selection, 22 genera including Desulfovibrio, Prevotella_9, Dorea, Escherichia-Shigella, and Negativibacillus were significantly enriched in NAFLD.
Figure 9. The integration of LEFSE-based and Random Forest methods identifies crucial genera that significantly decreased in the occurrence or development of NAFLD. Through LDA score ≥ 3 and p < 0.05, in combination with Random Forests selection, and by requiring at least three studies to appear the findings, the results were obtained: 46 genera including Faecalibacterium, Lachnospira, Catenibacterium, and UCG-002 were significantly reduced in NAFLD compared with those in the controls.
We constructed the KEGG pathway profiles by using Tax4fun2 version 1.1.5 and used the 16S rRNA gene sequences to predict the microbial community function. The gut microbial community function profiles and the predominant microbial functions in patients with NAFLD and healthy controls are shown with a heatmap (Figure 10A). Thirty-eight predicted microbial functions including lipopolysaccharide (LPS) biosynthesis, tryptophan metabolism, ether lipid metabolism, alpha-linolenic acid metabolism, and linoleic acid metabolism were remarkably increased, while 56 predicted microbial functions including linoleic acid metabolism, glyoxylate, and dicarboxylate metabolism, biosynthesis of amino acids, fatty acid metabolism, and cholesterol metabolism were remarkably decreased in patients with NAFLD compared with healthy controls (p < 0.05; Figure 10A). Subsequently, we analyzed the predicted differential functional KEGG pathways related to NAFLD progression. As NAFLD progresses, 11 predicted microbial functions including arachidonic acid metabolism, ether lipid metabolism, and glutathione metabolism were remarkably increased, while seven predicted microbial functions including histidine metabolism, primary bile acid biosynthesis, cyanoamino acid metabolism, and fatty acid biosynthesis were remarkably decreased in the pre-progressive period of NAFLD, compared with the post-progressive period of NAFLD (p < 0.05; Figure 10B).
Figure 10. Crucial microbial functional pathways associated with the (A) occurrence and (B) progression of NAFLD and required to be present in at least two studies. The results showed that 56 predicted microbial functions including lipopolysaccharide biosynthesis, tryptophan metabolism, ether lipid metabolism, alpha-Linolenic acid metabolism, and linoleic acid metabolism were remarkably increased compared with those in the healthy controls. As the NAFLD progresses, we observed a significant increase in 11 predicted microbial functions, such as arachidonic acid metabolism, ether lipid metabolism, and glutathione metabolism, compared to post-NAFLD. (C) Five diversity indexes reached AUC values over 0.7 between NAFLD (no fibrosis) patients (n = 16) versus healthy controls (n = 25) based on the dataset of PRJNA542724 (Dong et al., 2020). (D) Seven diversity indexes reached AUC values over 0.7 between NAFLD patients (n = 29) versus healthy controls (n = 30) based on the dataset of PRJNA682382 (Ahmed et al., 2021). (E) Five diversity indexes reached AUC values over 0.7 between NAFLD patients (n = 51) versus healthy controls (n = 117) based on the dataset of PRJEB28350 (Caussy et al., 2019). (F) Five diversity indexes reached AUC values over 0.7 between NAFLD-CIR patients (n = 26) versus healthy controls (n = 117) based on the dataset of PRJEB28350 (Caussy et al., 2019).
We conducted ROC curve analysis to evaluate the potential of the gut microbiota as a non-invasive predictive indicator to assess the risk of occurrence and progression of NAFLD. The predictive risk capacity is considered accurate for an AUC > 0.7 and p < 0.05. In the model predicting NAFLD occurrence and NALFD progression, all alpha diversity metrics reached an AUC > 0.7 (p < 0.05; Figures 10C–F). The observed, Chao1, and ACE indexes reached an AUC of 0.760, 0.756, and 0.767, respectively, in the model predicting the occurrence of NAFLD (Figures 10C,D). The observed, Chao1, and ACE indexes reached an AUC of 0.701, 0.700, and 0.701, respectively, in the model predicting the progression of NAFLD (Figure 10E). Besides, the observed, Chao1, and ACE indexes reached an AUC of 0.732, 0.735, and 0.735, respectively, in the model comparing healthy controls and the NALFD progression groups (Figure 10F).
At the genus level, eight significantly different gut microbes, including Negativibacillus, Prevotella_9, Blautia, and Desulfovibrio, based on LefSe, had a highly accurate predictive risk capacity to predict NAFLD occurrence (AUC > 0.7, p < 0.05, Figure 11A). Negativibacillus, Blautia, Dorea, and gauvreauii_group had an AUC of 0.907, 0.822, 0.817, and 0.800, respectively, in the model predicting NAFLD occurrence. Seven genera including Negativibacillus, Prevotella_9, Desulfovibrio, and Veillonella had a highly accurate predictive risk capacity to predict NAFLD progression (Figure 11B). Among them, Romboutsia, Prevotella_9, Desulfovibrio, and Negativibacillus had an AUC of 0.814, 0.802, 0.766, and 0.762, respectively, in the model predicting NAFLD progression. It is worth mentioning that the AUC was >0.7 for Negativibacillus, Prevotella_9, and Desulfovibrio in the models predicting NAFLD occurrence and NAFLD progression.
Figure 11. Predictive potential of bacteria at the genus level in NAFLD occurrence and NAFLD progress. (A) ROC curve for NAFLD compared to healthy controls. (B) ROC curve comparing the post-progressive period of NAFLD to the pre-progressive period of NAFLD. AUC (0.5–0.7), low accuracy; AUC (0.7–0.9), moderate accuracy; AUC (> 0.9), high accuracy.
NAFLD is a growing global phenomenon and is considered to be a complex disease associated with the dysregulation of multiple interconnected biological pathways (Li et al., 2021). In recent years, there has been increased research attention regarding the relationship between gut dysbiosis and the occurrence and progression of NAFLD. Many studies have been conducted to demonstrate gut microbiota dysregulation in patients with NAFLD compared with healthy controls (Boursier et al., 2016; Zhou et al., 2017; Nishiyama et al., 2020; Long et al., 2021; Shi et al., 2021). However, previous studies have focused on Europe and the Americas (particularly North America), and there are few studies from Asia. Regional differences in diet and culture impact the composition and alterations of the gut microbiota (Dai et al., 2020), resulting in inconsistent findings. There is a lack of consensus among the existing studies on gut microbiota changes in patients with NAFLD. Our study provides a comprehensive assessment of the gut microbiota composition in the context of the occurrence and progression of NAFLD. We aimed to identify specific gut microbiota biomarkers and to clarify the intricate relationship between the gut microbiota and NAFLD pathogenesis.
In our overall evaluation of alpha diversity across the 10 datasets, we found a significant reduction in intestinal alpha microbial diversity in patients with NAFLD compared with healthy controls. This finding indicates that alterations in the abundance and diversity of the gut microbiome are strongly related to NAFLD, consistent with the previous research results (Mouzaki et al., 2013; Zhu et al., 2013; Kim et al., 2019). When restricting the control groups to patients with obesity, there were still significant reductions in alpha diversity among patients with NAFLD compared with controls. This phenomenon indicates that although obesity may affect the diversity of the gut microbiome (Sarmiento et al., 2022), NALFD could play a more crucial role in decreasing the gut microbial diversity.
The Shannon index, an indicator of the diversity of bacterial populations, declined significantly after the progression of NAFLD, indicating that intestinal diversity also decreased as NAFLD progressed. Alpha diversity serves as a crucial indicator to evaluate the health of the intestinal microbiota. It reflects the microbiome richness and community diversity of the intestine. A decrease in microbial alpha diversity can potentially contribute to the pathogenesis and progression of diseases (Wenhui et al., 2022).
Our ROC analysis using alpha diversity as a predictor yielded a high AUC scores were observed for the pre-progressive and post-progressive periods of NAFLD. These findings suggest that alpha diversity exhibits a promising predictive ability and may serve as a potential risk predictor for NAFLD. Our analysis of beta diversity revealed significant technical and experimental variations among the studies. Differences in sequencing methods can impact taxonomic resolution and potentially alter compositional features. These “study effects” have a substantial impact on microbial community composition, but estimating individual effects is challenging due to the potential issue of multicollinearity among variables.
Subsequently, we conducted PCoA using Bray–Curtis distances to examine beta diversity and observed significant variations only in some datasets. Notably, there were significant changes in the composition of the gut microbiota as NAFLD progressed, while there were no significant differences during the pre-progressive period of NAFLD. These findings are consistent with those reported by Dong et al. (2020) and Caussy et al. (2019); these samples were obtained from Europe and the United States. Although samples from Asia also included patients with post-progressive NAFLD, we did not observe significant differences in our study.
At the phylum level, the human gut microbiota is mainly composed of Bacteroidetes and Firmicutes (Mo et al., 2022). In the European and American cohort studies, we observed a trend toward an increased abundance of Firmicutes and a decreased abundance of Bacteroidetes in patients with NAFLD, but the differences did not reach statistical significance. In contrast, the Asian studies showed a significant decrease in Firmicutes and a significant increase in Bacteroidetes in patients with NAFLD. This result is consistent with the study by Tsai et al. (2020), The authors observed that individuals with a body mass index (BMI) greater than 25 kg/m2 had significantly higher proportions of Bacteroidetes after controlling for BMI factors (Tsai et al., 2020). Notably, non-obese individuals with NAFLD exhibited a reduction in the Firmicutes-to-Bacteroidetes ratio (Wang et al., 2019). This observation challenges the conventional view that a high Firmicutes-to-Bacteroidetes ratio is a crucial characteristic of NAFLD (Xing et al., 2019). The Western diet is characterized by high levels of fat, protein, and sugar, while the Asian diet is typically low in fat and protein, but rich in dietary fiber. Similarly, the Latino diet mainly consists of vegetables, nuts, grains, and low-fat red or processed meat (Wang et al., 2021). This difference in diet composition has a significant impact on the growth of the phylum Bacteroidetes; these organisms are associated with the production of branched-chain fatty acids through amino acid fermentation, which can lead to insulin resistance and promote the development of NAFLD (Newgard, 2012; Tsai et al., 2020). Expanding on this comparison revealed varying trends in Bacteroidetes and Firmicutes abundance in patients with NAFLD across geographical regions, highlighting the potential influence of dietary habits on the gut microbiota composition. Interestingly, we observed an enrichment of lactic acid-producing bacteria (such as Lactobacillus) in patients with NAFLD, consistent with the microbiome results reported by Min et al. (2022) in patients with bladder cancer. Although there is an association between NAFLD and bladder cancer (Bjorkstrom et al., 2022), the complex mechanisms through which NAFLD promotes the occurrence of bladder cancer remain to be fully elucidated. Given the pivotal role of the microbiome in the development and progression of cancer (Wu et al., 2018), the relationship between NAFLD and bladder cancer might be clarified by further analysis of the microbiome. Subsequently, we identified 22 dominant genera in NAFLD using LEfSe and random forest analyses. Among them, Escherichia-Shigella could evade host immune surveillance and induce inflammation in the intestine by inhibiting autophagy in epithelial and inflammatory cells (Liang et al., 2022). Desulfovibrio, a bacterial genus purported to promote inflammation, synthesizes hydrogen sulfide that exhibits cytotoxicity toward intestinal cells (Zheng et al., 2018; He et al., 2020). Notably, Desulfovibrio is associated with LPS biosynthesis, and an increase in LPS levels triggers a systemic inflammatory response. Elevated levels of LPS are crucial for both the occurrence and progression of NAFLD (Diling et al., 2017). Prevotella can ferment carbohydrates, resulting in the production of short-chain fatty acids that reduce hepatic lipogenesis, potentially providing relief from the development of NAFLD (Gaike et al., 2020; Luo et al., 2021). This implies that gut microbiome disturbances may trigger compensatory mechanisms both before and after NAFLD progression. Prevotella, as a common probiotic, plays a crucial role in regulating gut health (Liu et al., 2019). Focusing on Prevotella could become a promising direction for NAFLD treatments, perhaps through the development of specific probiotic formulations, the implementation of targeted dietary interventions, or the exploration of alternative approaches to modulate gut microbiota balance. However, caution is warranted because this cannot be regarded as conclusive evidence of compensatory mechanisms. Additional validation experiments are necessary to elucidate the compensatory mechanisms of Prevotella in the development and progression of NAFLD. Moreover, ROC analysis identified 13 genera, including Desulfovibrio, Prevotella, Negativibacillus, and Escherichia-Shigella, with preferable diagnostic potential to distinguish patients with NAFLD from healthy controls and to predict NAFLD progression. The diagnostic power of a single genus in predicting the occurrence and progression of NAFLD is limited (Fenn et al., 2022). Therefore, machine learning could be utilized to build a potential risk prediction model based on the combination of many critical genera or metabolites for assessing the risk of NAFLD.
Based on the predicted function analysis, LPS, tryptophan metabolism, and various fatty acid metabolism pathways were significantly enriched in patients with NAFLD. Additionally, glutathione metabolism was enriched in patients with post-progressive NAFLD. Interestingly, in a cohort study examining NAFLD in adolescents, glutathione metabolism declined significantly with the development of NAFLD (Xanthakos et al., 2015), suggesting that the dysregulation of glutathione metabolism is closely associated with the pathological conditions of NAFLD. However, the majority of studies have focused on glutathione synthesis rather than glutathione redox balance (Gansemer et al., 2020). Glutathione, the primary antioxidant molecule involved in oxidative defense mechanisms (Kang et al., 2018), exists in the body primarily in reduced glutathione (GSH) and oxidized glutathione (GSSG) forms. GSH can be converted to GSSG by glutathione reductase. The progression of NAFLD is significantly influenced by oxidative stress caused by an imbalance between the production of reactive oxygen species (ROS) and antioxidant defenses (Hong et al., 2021). Due to the accumulation of hepatic lipids in patients with NAFLD, liver ROS levels are elevated (Oh and Chun, 2022). Excess ROS disrupts lipid metabolism and inhibits the activity of antioxidant enzymes (Hong et al., 2021), leading to the accumulation of intracellular GSSG. Elevated levels of GSSG promote the progression of NAFLD to an inflammatory state by inducing the formation of glutathionylated IKK-β (IKK-β-SSG), which inhibits nuclear factor κB and increases the expression of the pro-inflammatory cytokine tumor necrosis factor α (Dou et al., 2018). Therefore, we postulate that alterations in these crucial gut microbes impact the production of diverse metabolites within the body, including LPS and glutathione metabolism, thereby promoting the onset and progression of NAFLD.
We conducted a comprehensive investigation of studies from diverse regions, including healthy controls, patients with obesity, and, and patients with biopsy-confirmed NAFLD, to provide more meaningful findings. However, this study has a few limitations that need to be acknowledged. First, due to insufficient information on detailed sample characteristics such as age, BMI, and diet, we could not conduct further stratified analysis. Additionally, it should be noted that each study used different diagnostic methods and criteria for NAFLD, leading to some heterogeneity among the studies. Moreover, we could not include some articles in the integrated analysis due to the unavailability of the original data. This constitutes a major limitation of our study because we could discuss the findings from these articles but not include them in our comprehensive analysis. Second, the decision to use 16S rRNA gene sequencing data instead of macro-genomic sequencing data limited the accuracy of species-level and functional analyses. Additionally, differences in the choice of sequencing instruments and variable regions sequenced across studies may have introduced confounding factors that could have biased the results. we urge caution regarding the interpretation of our findings. Finally, this study is cross-sectional and only explored the correlation between the gut microbiota and NAFLD. We cannot draw conclusions about causality, which will require the use of in vitro experiments to illustrate the cause-effect relationship between crucial bacteria and NAFLD.
In summary, our results demonstrate that gut microbial diversity declines significantly with the progression of NAFLD, while alpha diversity exhibits promise as a predictive indicator for NAFLD risk. The increased abundance of Desulfovibrio, Negativibacillus, Prevotella, Escherichia-Shigella, and other genera may serve as an indication of their predictive risk ability for NAFLD progression. With the occurrence and progression of NAFLD, changes in functional pathways such as LPS metabolism, glutathione metabolism, and lipid metabolism are significantly upregulated. These findings are important for elucidating the relationship between the gut microbiota and NAFLD, and are crucial for elucidating the pathogenesis, prevention, and treatment of this disease.
The original contributions presented in the study are included in the article/Supplementary material, further inquiries can be directed to the corresponding authors.
Ethical approval was not required for the study involving humans in accordance with the local legislation and institutional requirements. Written informed consent to participate in this study was not required from the participants or the participants' legal guardians/next of kin in accordance with the national legislation and the institutional requirements.
HM: Writing – original draft. XY: Writing – original draft, YX: Data curation, Formal analysis, Visualization, Writing – review & editing. JZ: Visualization, Writing – review & editing. QW: Writing – review & editing. YW: Writing – review & editing. YY: Data curation, Writing – review & editing. DL: Data curation, Writing – review & editing. LY: Supervision, Writing – review & editing. PC: Supervision, Writing – review & editing. HL: Conceptualization, Writing – review & editing. JH: Conceptualization, Funding acquisition, Writing – review & editing.
The author(s) declare financial support was received for the research, authorship, and/or publication of this article. This work was supported by funds from the National Natural Science Foundation of China (NSFC, grant nos. 82060366, 82273694, and 82160385) and the Natural Science Foundation of Guangxi Province (grant no. 2023GXNSFDA026036).
The authors declare that the research was conducted in the absence of any commercial or financial relationships that could be construed as a potential conflict of interest.
All claims expressed in this article are solely those of the authors and do not necessarily represent those of their affiliated organizations, or those of the publisher, the editors and the reviewers. Any product that may be evaluated in this article, or claim that may be made by its manufacturer, is not guaranteed or endorsed by the publisher.
The Supplementary material for this article can be found online at: https://www.frontiersin.org/articles/10.3389/fmicb.2023.1257903/full#supplementary-material
1. ^https://pubmed.ncbi.nlm.nih.gov/
2. ^https://www.ncbi.nlm.nih.gov/bioproject
3. ^http://ncbi.github.io/sra-tools/
5. ^https://github.com/qiime2/q2-cutadapt
6. ^https://github.com/vegandevs/vegan
7. ^https://CRAN.R-project.org/package=VennDiagram
Abdul-Hai, A., Abdallah, A., and Malnick, S. D. (2015). Influence of gut bacteria on development and progression of non-alcoholic fatty liver disease. World J. Hepatol. 7, 1679–1684. doi: 10.4254/wjh.v7.i12.1679
Ahmed, B. A., Ong, F. J., Barra, N. G., Blondin, D. P., Gunn, E., Oreskovich, S. M., et al. (2021). Lower brown adipose tissue activity is associated with non-alcoholic fatty liver disease but not changes in the gut microbiota. Cell. Rep. Med. 2:100397. doi: 10.1016/j.xcrm.2021.100397
Baumann, A., Nier, A., Hernandez-Arriaga, A., Brandt, A., Lorenzo Pisarello, M. J., Jin, C. J., et al. (2021). Toll-like receptor 1 as a possible target in non-alcoholic fatty liver disease. Sci. Rep. 11:17815. doi: 10.1038/s41598-021-97346-9
Bjorkstrom, K., Widman, L., and Hagstrom, H. (2022). Risk of hepatic and extrahepatic cancer in NAFLD: a population-based cohort study. Liver Int. 42, 820–828. doi: 10.1111/liv.15195
Bokulich, N. A., Kaehler, B. D., Rideout, J. R., Dillon, M., Bolyen, E., Knight, R., et al. (2018). Optimizing taxonomic classification of marker-gene amplicon sequences with QIIME 2's q2-feature-classifier plugin. Microbiome 6:90. doi: 10.1186/s40168-018-0470-z
Boursier, J., Mueller, O., Barret, M., Machado, M., Fizanne, L., Araujo-Perez, F., et al. (2016). The severity of nonalcoholic fatty liver disease is associated with gut dysbiosis and shift in the metabolic function of the gut microbiota. Hepatology 63, 764–775. doi: 10.1002/hep.28356
Callahan, B. J., McMurdie, P. J., Rosen, M. J., Han, A. W., Johnson, A. J., and Holmes, S. P. (2016). DADA2: high-resolution sample inference from Illumina amplicon data. Nat. Methods 13, 581–583. doi: 10.1038/nmeth.3869
Caussy, C., Tripathi, A., Humphrey, G., Bassirian, S., Singh, S., Faulkner, C., et al. (2019). A gut microbiome signature for cirrhosis due to nonalcoholic fatty liver disease. Nat. Commun. 10:1406. doi: 10.1038/s41467-019-09455-9
Dai, X., Guo, Z., Chen, D., Li, L., Song, X., Liu, T., et al. (2020). Maternal sucralose intake alters gut microbiota of offspring and exacerbates hepatic steatosis in adulthood. Gut Microbes 11, 1043–1063. doi: 10.1080/19490976.2020.1738187
Diling, C., Chaoqun, Z., Jian, Y., Jian, L., Jiyan, S., Yizhen, X., et al. (2017). Immunomodulatory activities of a fungal protein extracted from Hericium erinaceus through regulating the gut microbiota. Front. Immunol. 8:666. doi: 10.3389/fimmu.2017.00666
Dong, T. S., Katzka, W., Lagishetty, V., Luu, K., Hauer, M., Pisegna, J., et al. (2020). A microbial signature identifies advanced fibrosis in patients with chronic liver disease mainly due to NAFLD. Sci. Rep. 10:2771. doi: 10.1038/s41598-020-59535-w
Dou, X., Li, S., Hu, L., Ding, L., Ma, Y., Ma, W., et al. (2018). Glutathione disulfide sensitizes hepatocytes to TNFalpha-mediated cytotoxicity via IKK-beta S-glutathionylation: a potential mechanism underlying non-alcoholic fatty liver disease. Exp. Mol. Med. 50, 1–16. doi: 10.1038/s12276-017-0013-x
Fenn, D., Abdel-Aziz, M. I., van Oort, P. M. P., Brinkman, P., Ahmed, W. M., Felton, T., et al. (2022). Composition and diversity analysis of the lung microbiome in patients with suspected ventilator-associated pneumonia. Crit. Care 26:203. doi: 10.1186/s13054-022-04068-z
Gaike, A. H., Paul, D., Bhute, S., Dhotre, D. P., Pande, P., Upadhyaya, S., et al. (2020). The gut microbial diversity of newly diagnosed diabetics but not of Prediabetics is significantly different from that of healthy nondiabetics. mSystems 5:e00578–19. doi: 10.1128/mSystems.00578-19
Gansemer, E. R., McCommis, K. S., Martino, M., King-McAlpin, A. Q., Potthoff, M. J., Finck, B. N., et al. (2020). NADPH and glutathione redox link TCA cycle activity to endoplasmic reticulum homeostasis. iScience 23:101116. doi: 10.1016/j.isci.2020.101116
He, L. X., Abdolmaleky, H. M., Yin, S., Wang, Y., and Zhou, J. R. (2020). Dietary fermented soy extract and oligo-lactic acid alleviate chronic kidney disease in mice via inhibition of inflammation and modulation of gut microbiota. Nutrients 12:2376. doi: 10.3390/nu12082376
Hong, T., Chen, Y., Li, X., and Lu, Y. (2021). The role and mechanism of oxidative stress and nuclear receptors in the development of NAFLD. Oxidative Med. Cell. Longev. 2021, 6889533–6889525. doi: 10.1155/2021/6889533
Human Microbiome Project Consortium (2012). A framework for human microbiome research. Nature 486, 215–221. doi: 10.1038/nature11209
Iizuka, K., Takao, K., Kato, T., Horikawa, Y., and Takeda, J. (2018). ChREBP reciprocally regulates liver and plasma triacylglycerol levels in different manners. Nutrients 10:1699. doi: 10.3390/nu10111699
Janssen, S., McDonald, D., Gonzalez, A., Navas-Molina, J. A., Jiang, L., Xu, Z. Z., et al. (2018). Phylogenetic placement of exact amplicon sequences improves associations with clinical information. mSystems 3, e00021–e00018. doi: 10.1128/mSystems.00021-18
Jiang, G., Sun, C., Wang, X., Mei, J., Li, C., Zhan, H., et al. (2022). Hepatoprotective mechanism of Silybum marianum on nonalcoholic fatty liver disease based on network pharmacology and experimental verification. Bioengineered 13, 5216–5235. doi: 10.1080/21655979.2022.2037374
Jiang, W., Wu, N., Wang, X., Chi, Y., Zhang, Y., Qiu, X., et al. (2015). Dysbiosis gut microbiota associated with inflammation and impaired mucosal immune function in intestine of humans with non-alcoholic fatty liver disease. Sci. Rep. 5:8096. doi: 10.1038/srep08096
Kang, R., Zeng, L., Zhu, S., Xie, Y., Liu, J., Wen, Q., et al. (2018). Lipid peroxidation drives Gasdermin D-mediated Pyroptosis in lethal Polymicrobial Sepsis. Cell Host Microbe 24, 97–108.e4. doi: 10.1016/j.chom.2018.05.009
Kim, H. N., Joo, E. J., Cheong, H. S., Kim, Y., Kim, H. L., Shin, H., et al. (2019). Gut microbiota and risk of persistent nonalcoholic fatty liver diseases. J. Clin. Med. 8:1089. doi: 10.3390/jcm8081089
Kordy, K., Li, F., Lee, D. J., Kinchen, J. M., Jew, M. H., La Rocque, M. E., et al. (2021). Metabolomic predictors of non-alcoholic steatohepatitis and advanced fibrosis in children. Front. Microbiol. 12:713234. doi: 10.3389/fmicb.2021.713234
Lang, S., Martin, A., Zhang, X., Farowski, F., Wisplinghoff, H., Vehreschild, M., et al. (2021). Combined analysis of gut microbiota, diet and PNPLA3 polymorphism in biopsy-proven non-alcoholic fatty liver disease. Liver Int. 41, 1576–1591. doi: 10.1111/liv.14899
Lee, G., You, H. J., Bajaj, J. S., Joo, S. K., Yu, J., Park, S., et al. (2020). Distinct signatures of gut microbiome and metabolites associated with significant fibrosis in non-obese NAFLD. Nat. Commun. 11:4982. doi: 10.1038/s41467-020-18754-5
Li, X., Ge, J., Li, Y., Cai, Y., Zheng, Q., Huang, N., et al. (2021). Integrative lipidomic and transcriptomic study unravels the therapeutic effects of saikosaponins a and D on non-alcoholic fatty liver disease. Acta Pharm. Sin. B 11, 3527–3541. doi: 10.1016/j.apsb.2021.03.018
Li, H., Guo, L., Su, K., Li, C., Jiang, Y., Wang, P., et al. (2023). Construction and validation of TACE therapeutic efficacy by ALR score and nomogram: a large, multicenter study. J. Hepatocell. Carcinoma 10, 1009–1017. doi: 10.2147/JHC.S414926
Liang, T., Li, D., Zunong, J., Li, M., Amaerjiang, N., Xiao, H., et al. (2022). Interplay of lymphocytes with the intestinal microbiota in children with nonalcoholic fatty liver disease. Nutrients 14:4641. doi: 10.3390/nu14214641
Liu, C., Cui, Y., Li, X., and Yao, M. (2021). Microeco: an R package for data mining in microbial community ecology. FEMS Microbiol. Ecol. 97:fiaa255. doi: 10.1093/femsec/fiaa255
Liu, Q., Li, F., Zhuang, Y., Xu, J., Wang, J., Mao, X., et al. (2019). Alteration in gut microbiota associated with hepatitis B and non-hepatitis virus related hepatocellular carcinoma. Gut Pathog. 11:1. doi: 10.1186/s13099-018-0281-6
Long, X., Liu, D., Gao, Q., Ni, J., Qian, L., Ni, Y., et al. (2021). Bifidobacterium adolescentis alleviates liver steatosis and steatohepatitis by increasing fibroblast growth factor 21 sensitivity. Front. Endocrinol. 12:773340. doi: 10.3389/fendo.2021.773340
Lu, C. W., Lee, Y. C., Chiang, C. H., Chang, H. H., Yang, W. S., and Huang, K. C. (2021). Independent dose-response associations between Fetuin-a and lean nonalcoholic fatty liver disease. Nutrients 13:2928. doi: 10.3390/nu13092928
Luo, H., Wu, H., Wang, L., Xiao, S., Lu, Y., Liu, C., et al. (2021). Hepatoprotective effects of Cassiae semen on mice with non-alcoholic fatty liver disease based on gut microbiota. Commun. Biol. 4:1357. doi: 10.1038/s42003-021-02883-8
Min, K., Kim, H. T., Lee, E. H., Park, H., and Ha, Y. S. (2022). Bacteria for treatment: microbiome in bladder Cancer. Biomedicine 10:1783. doi: 10.3390/biomedicines10081783
Mo, J., Ni, J., Zhang, M., Xu, Y., Li, Y., Karim, N., et al. (2022). Mulberry anthocyanins ameliorate DSS-induced ulcerative colitis by improving intestinal barrier function and modulating gut microbiota. Antioxidants 11:1674. doi: 10.3390/antiox11091674
Monga Kravetz, A., Testerman, T., Galuppo, B., Graf, J., Pierpont, B., Siebel, S., et al. (2020). Effect of gut microbiota and PNPLA3 rs738409 variant on nonalcoholic fatty liver disease (NAFLD) in obese youth. J. Clin. Endocrinol. Metab. 105, e3575–e3585. doi: 10.1210/clinem/dgaa382
Mouzaki, M., Comelli, E. M., Arendt, B. M., Bonengel, J., Fung, S. K., Fischer, S. E., et al. (2013). Intestinal microbiota in patients with nonalcoholic fatty liver disease. Hepatology 58, 120–127. doi: 10.1002/hep.26319
Navarro, L. A., Wree, A., Povero, D., Berk, M. P., Eguchi, A., Ghosh, S., et al. (2015). Arginase 2 deficiency results in spontaneous steatohepatitis: a novel link between innate immune activation and hepatic de novo lipogenesis. J. Hepatol. 62, 412–420. doi: 10.1016/j.jhep.2014.09.015
Newgard, C. B. (2012). Interplay between lipids and branched-chain amino acids in development of insulin resistance. Cell Metab. 15, 606–614. doi: 10.1016/j.cmet.2012.01.024
Nishiyama, M., Ohtake, N., Kaneko, A., Tsuchiya, N., Imamura, S., Iizuka, S., et al. (2020). Increase of Akkermansia muciniphila by a diet containing Japanese traditional medicine Bofutsushosan in a mouse model of non-alcoholic fatty liver disease. Nutrients 12:839. doi: 10.3390/nu12030839
Oh, J. M., and Chun, S. (2022). Ginsenoside CK inhibits the early stage of Adipogenesis via the AMPK, MAPK, and AKT signaling pathways. Antioxidants 11:1890. doi: 10.3390/antiox11101890
Pan, X., Kaminga, A. C., Liu, A., Wen, S. W., Luo, M., and Luo, J. (2021). Gut microbiota, glucose, lipid, and water-electrolyte metabolism in children with nonalcoholic fatty liver disease. Front. Cell. Infect. Microbiol. 11:683743. doi: 10.3389/fcimb.2021.683743
Ponziani, F. R., Bhoori, S., Castelli, C., Putignani, L., Rivoltini, L., Del Chierico, F., et al. (2019). Hepatocellular carcinoma is associated with gut microbiota profile and inflammation in nonalcoholic fatty liver disease. Hepatology 69, 107–120. doi: 10.1002/hep.30036
Saeedi, B. J., Liu, K. H., Owens, J. A., Hunter-Chang, S., Camacho, M. C., Eboka, R. U., et al. (2020). Gut-resident lactobacilli activate hepatic Nrf2 and protect against oxidative liver injury. Cell Metab. 31, 956–968.e5. doi: 10.1016/j.cmet.2020.03.006
Sarmiento, J., Pulgar, R., Mandakovic, D., Porras, O., Flores, C. A., Luco, D., et al. (2022). Nocturnal light pollution induces weight gain in mice and reshapes the structure, functions, and interactions of their colonic microbiota. Int. J. Mol. Sci. 23:1673. doi: 10.3390/ijms23031673
Segata, N., Izard, J., Waldron, L., Gevers, D., Miropolsky, L., Garrett, W. S., et al. (2011). Metagenomic biomarker discovery and explanation. Genome Biol. 12:R60. doi: 10.1186/gb-2011-12-6-r60
Shi, A., Li, T., Zheng, Y., Song, Y., Wang, H., Wang, N., et al. (2021). Chlorogenic acid improves NAFLD by regulating gut microbiota and GLP-1. Front. Pharmacol. 12:693048. doi: 10.3389/fphar.2021.693048
Sunny, N. E., Parks, E. J., Browning, J. D., and Burgess, S. C. (2011). Excessive hepatic mitochondrial TCA cycle and gluconeogenesis in humans with nonalcoholic fatty liver disease. Cell Metab. 14, 804–810. doi: 10.1016/j.cmet.2011.11.004
Tarantino, G., Crocetto, F., Di Vito, C., Creta, M., Martino, R., Pandolfo, S. D., et al. (2021). Association of NAFLD and insulin resistance with non metastatic bladder Cancer patients: a cross-sectional retrospective study. J. Clin. Med. 10:346. doi: 10.3390/jcm10020346
Tsai, M. C., Liu, Y. Y., Lin, C. C., Wang, C. C., Wu, Y. J., Yong, C. C., et al. (2020). Gut microbiota Dysbiosis in patients with biopsy-proven nonalcoholic fatty liver disease: a cross-sectional study in Taiwan. Nutrients 12:820. doi: 10.3390/nu12030820
Wang, Z., Usyk, M., Vazquez-Baeza, Y., Chen, G. C., Isasi, C. R., Williams-Nguyen, J. S., et al. (2021). Microbial co-occurrence complicates associations of gut microbiome with US immigration, dietary intake and obesity. Genome Biol. 22:336. doi: 10.1186/s13059-021-02559-w
Wang, J., Wang, Y., Zhang, X., Liu, J., Zhang, Q., Zhao, Y., et al. (2017). Gut microbial Dysbiosis is associated with altered hepatic functions and serum metabolites in chronic hepatitis B patients. Front. Microbiol. 8:2222. doi: 10.3389/fmicb.2017.02222
Wang, H., Yan, Y., Yi, X., Duan, Y., Wang, J., Li, S., et al. (2019). Histopathological features and composition of gut microbiota in Rhesus monkey of alcoholic liver disease. Front. Microbiol. 10:165. doi: 10.3389/fmicb.2019.00165
Wemheuer, F., Taylor, J. A., Daniel, R., Johnston, E., Meinicke, P., Thomas, T., et al. (2020). Tax4Fun2: prediction of habitat-specific functional profiles and functional redundancy based on 16S rRNA gene sequences. Environ. Microbiome 15:11. doi: 10.1186/s40793-020-00358-7
Wenhui, Y., Zhongyu, X., Kai, C., Zhaopeng, C., Jinteng, L., Mengjun, M., et al. (2022). Variations in the gut microbiota in breast Cancer occurrence and bone metastasis. Front. Microbiol. 13:894283. doi: 10.3389/fmicb.2022.894283
Wu, P., Zhang, G., Zhao, J., Chen, J., Chen, Y., Huang, W., et al. (2018). Profiling the urinary microbiota in male patients with bladder Cancer in China. Front. Cell. Infect. Microbiol. 8:167. doi: 10.3389/fcimb.2018.00167
Xanthakos, S. A., Jenkins, T. M., Kleiner, D. E., Boyce, T. W., Mourya, R., Karns, R., et al. (2015). High prevalence of nonalcoholic fatty liver disease in adolescents undergoing bariatric surgery. Gastroenterology 149, 623–634.e8. doi: 10.1053/j.gastro.2015.05.039
Xing, Y. W., Lei, G. T., Wu, Q. H., Jiang, Y., and Huang, M. X. (2019). Procyanidin B2 protects against diet-induced obesity and non-alcoholic fatty liver disease via the modulation of the gut microbiota in rabbits. World J. Gastroenterol. 25, 955–966. doi: 10.3748/wjg.v25.i8.955
Zhang, X., Coker, O. O., Chu, E. S., Fu, K., Lau, H. C. H., Wang, Y. X., et al. (2021). Dietary cholesterol drives fatty liver-associated liver cancer by modulating gut microbiota and metabolites. Gut 70, 761–774. doi: 10.1136/gutjnl-2019-319664
Zhang, T., Hu, J., Wang, X., Zhao, X., Li, Z., Niu, J., et al. (2019). MicroRNA-378 promotes hepatic inflammation and fibrosis via modulation of the NF-kappaB-TNFalpha pathway. J. Hepatol. 70, 87–96. doi: 10.1016/j.jhep.2018.08.026
Zhang, S., Jiang, C., Jiang, L., Chen, H., Huang, J., Zhang, J., et al. (2023). Uncovering the immune microenvironment and molecular subtypes of hepatitis B-related liver cirrhosis and developing stable a diagnostic differential model by machine learning and artificial neural networks. Front. Mol. Biosci. 10:1275897. doi: 10.3389/fmolb.2023.1275897
Zhang, Y., Xu, J., Wang, X., Ren, X., and Liu, Y. (2019). Changes of intestinal bacterial microbiota in coronary heart disease complicated with nonalcoholic fatty liver disease. BMC Genomics 20:862. doi: 10.1186/s12864-019-6251-7
Zheng, J., Cheng, G., Li, Q., Jiao, S., Feng, C., Zhao, X., et al. (2018). Chitin oligosaccharide modulates gut microbiota and attenuates high-fat-diet-induced metabolic syndrome in mice. Mar. Drugs 16:66. doi: 10.3390/md16020066
Zhou, D., Pan, Q., Xin, F. Z., Zhang, R. N., He, C. X., Chen, G. Y., et al. (2017). Sodium butyrate attenuates high-fat diet-induced steatohepatitis in mice by improving gut microbiota and gastrointestinal barrier. World J. Gastroenterol. 23, 60–75. doi: 10.3748/wjg.v23.i1.60
Keywords: gut microbiota, 16S ribosomal RNA gene amplicon sequencing, nonalcoholic fatty liver disease, liver disease, microbial markers
Citation: Mai H, Yang X, Xie Y, Zhou J, Wang Q, Wei Y, Yang Y, Lu D, Ye L, Cui P, Liang H and Huang J (2024) The role of gut microbiota in the occurrence and progression of non-alcoholic fatty liver disease. Front. Microbiol. 14:1257903. doi: 10.3389/fmicb.2023.1257903
Received: 13 July 2023; Accepted: 12 December 2023;
Published: 05 January 2024.
Edited by:
Steven Gill, University of Rochester, United StatesReviewed by:
Giovanni Tarantino, University of Naples Federico II, ItalyCopyright © 2024 Mai, Yang, Xie, Zhou, Wang, Wei, Yang, Lu, Ye, Cui, Liang and Huang. This is an open-access article distributed under the terms of the Creative Commons Attribution License (CC BY). The use, distribution or reproduction in other forums is permitted, provided the original author(s) and the copyright owner(s) are credited and that the original publication in this journal is cited, in accordance with accepted academic practice. No use, distribution or reproduction is permitted which does not comply with these terms.
*Correspondence: Jiegang Huang, amllZ2FuZ2h1YW5nQGd4bXUuZWR1LmNu; Hao Liang, bGlhbmdoYW9AZ3htdS5lZHUuY24=
†These authors have contributed equally to this work and share first authorship
Disclaimer: All claims expressed in this article are solely those of the authors and do not necessarily represent those of their affiliated organizations, or those of the publisher, the editors and the reviewers. Any product that may be evaluated in this article or claim that may be made by its manufacturer is not guaranteed or endorsed by the publisher.
Research integrity at Frontiers
Learn more about the work of our research integrity team to safeguard the quality of each article we publish.