- 1School of Biological Sciences, University of Auckland, Auckland, New Zealand
- 2High-Value Nutrition National Science Challenge, Auckland, New Zealand
- 3Human Nutrition Unit, University of Auckland, Auckland, New Zealand
- 4Centro de Investigação em Tecnologias e Serviços de Saúde (CINTESIS), NOVA University of Lisbon, Lisbon, Portugal
- 5Department of Statistics, University of Auckland, Auckland, New Zealand
- 6Department of Food and Nutrition, Faculty of Agriculture and Forestry, University of Helsinki, Helsinki, Finland
- 7Department of Nutrition, Exercise and Sports, Faculty of Science, University of Copenhagen, Copenhagen, Denmark
- 8Clinical Research, Copenhagen University Hospital – Steno Diabetes Center Copenhagen, Herlev, Denmark
- 9Department of Medicine, University of Auckland, Auckland, New Zealand
Obesity-related metabolic diseases such as type 2 diabetes (T2D) are major global health issues, affecting hundreds of millions of people worldwide. The underlying factors are both diverse and complex, incorporating biological as well as cultural considerations. A role for ethnicity – a measure of self-perceived cultural affiliation which encompasses diet, lifestyle and genetic components – in susceptibility to metabolic diseases such as T2D is well established. For example, Asian populations may be disproportionally affected by the adverse ‘TOFI’ (Thin on the Outside, Fat on the Inside) profile, whereby outwardly lean individuals have increased susceptibility due to excess visceral and ectopic organ fat deposition. A potential link between the gut microbiota and metabolic disease has more recently come under consideration, yet our understanding of the interplay between ethnicity, the microbiota and T2D remains incomplete. We present here a 16S rRNA gene-based comparison of the fecal microbiota of European-ancestry and Chinese-ancestry cohorts with overweight and prediabetes, residing in New Zealand. The cohorts were matched for mean fasting plasma glucose (FPG: mean ± SD, European-ancestry: 6.1 ± 0.4; Chinese-ancestry: 6.0 ± 0.4 mmol/L), a consequence of which was a significantly higher mean body mass index in the European group (BMI: European-ancestry: 37.4 ± 6.8; Chinese-ancestry: 27.7 ± 4.0 kg/m2; p < 0.001). Our findings reveal significant microbiota differences between the two ethnicities, though we cannot determine the underpinning factors. In both cohorts Firmicutes was by far the dominant bacterial phylum (European-ancestry: 93.4 ± 5.5%; Chinese-ancestry: 79.6 ± 10.4% of 16S rRNA gene sequences), with Bacteroidetes and Actinobacteria the next most abundant. Among the more abundant (≥1% overall relative sequence abundance) genus-level taxa, four zero-radius operational taxonomic units (zOTUs) were significantly higher in the European-ancestry cohort, namely members of the Subdoligranulum, Blautia, Ruminoclostridium, and Dorea genera. Differential abundance analysis further identified a number of additional zOTUs to be disproportionately overrepresented across the two ethnicities, with the majority of taxa exhibiting a higher abundance in the Chinese-ancestry cohort. Our findings underscore a potential influence of ethnicity on gut microbiota composition in the context of individuals with overweight and prediabetes.
1. Introduction
Type 2 diabetes (T2D) is a significant global health issue and among the fastest growing chronic disease worldwide (International Diabetes Federation, 2021). When considering prediabetes, where individuals develop dysglycaemia indicative of increased risk of later T2D, the situation is even starker. Among the ~5.5 million residents of New Zealand, for example, it is estimated that ~4% have T2D (Te Whatu Ora: Health New Zealand, 2021) and a further ~20% have prediabetes (New Zealand Society for the Study of Diabetes, 2023). Fortunately, there is strong evidence that lifestyle (including dietary) change in those with prediabetes can delay or even prevent progression to frank T2D (Liu et al., 2015). It is therefore of considerable importance to understand the factors which influence prediabetes. Ethnicity, defined here as a measure of self-perceived cultural affiliation, encompassing diet, lifestyle as well as underlying genetics, is one such factor. An association between ethnicity and susceptibility to obesity, T2D and other metabolic disorders is well documented (Spanakis and Golden, 2013; Yip et al., 2017). Certainly, differences in glucose metabolism and insulin resistance have been observed between ethnic groups, but with complex causes (Golden et al., 2012). Another potential contributor to (pre)diabetes is the gut microbiota (Deschasaux et al., 2018). Although the underlying mechanisms remain unclear, a combination of biological, clinical and social factors likely play a role, with metabolic susceptibility and microbiota profile also potentially associated through mechanisms yet to be determined.
Given that T2D progression is a result of both environmental and genetic factors, a higher T2D risk profile observed in Asian ethnicities at low body mass index (BMI) (Haldar et al., 2015) has been suggested to reflect a general inability to adapt to the Western lifestyle and dietary changes popularized with the rise of urbanization and increased globalization over the past two centuries (Akiyama, 2008). As the gut microbiota plays a prominent role in regulating host metabolic homeostasis (Sears, 2005; LeBlanc et al., 2013; Quigley, 2013; Clarke et al., 2014; Duvallet et al., 2017), the utility of microbial community characteristics as non-invasive diagnostic indicators of health has in recent years become widely recognized (Duvallet et al., 2017). However, although associations between disease pathogenicity and the gut microbiota have been established, the taxonomic composition is highly personalized among individuals (Gaulke and Sharpton, 2018) and the noise projected strongly influences the accuracy of microbiota-based investigations. Yet the extent of contribution, if any, to this inter-individual variation by ethnicity remains largely unresolved: indeed, large observational studies examining the gut microbiota of participants of varied ethnic backgrounds but with shared geography have produced findings both in support of ethnicity explaining more of the variation than environmental factors (in 2,084 participants) (Deschasaux et al., 2018), and vice versa (in 1,046 participants) (Rothschild et al., 2018).
The higher T2D risk profile in the absence of high BMI in Asian cohorts is characterized by adverse body fat distribution, including excess abdominal, visceral and ectopic fat deposition compared with, for example, European-ancestry individuals (Lear et al., 2007; Nazare et al., 2012; Jo and Mainous, 2018). An inability to expand subcutaneous fat stores (Misra and Khurana, 2011; Ramachandran et al., 2012) may contribute to this adverse Asian TOFI (Thin on the Outside, Fat on the Inside) phenotype (Thomas et al., 2012), where ectopic lipid infiltration into pancreas and liver (Dickinson et al., 2002; Liew et al., 2003; Cortés and Fernández-Galilea, 2015) may occur even in individuals with low BMI and whole body adiposity (WHO Expert Consultation, 2004). This condition may both inhibit glucose-mediated β-cell insulin secretion (Lee et al., 1994) and decrease insulin sensitivity (Shibata et al., 2007), and could potentially be associated with disparate microbiota.
The primary aim of this study was to describe the fecal microbiota in individuals with prediabetes. Previous research has implicated metabolic characteristics such as BMI and body weight as being of relevance, with ethnicity also potentially playing a role. We thus leveraged a subset of a previously published 16S rRNA gene dataset which focused on adults of European descent living in New Zealand [from the multi-national PREVIEW lifestyle intervention (Raben et al., 2021; Jian et al., 2022)], and an unpublished dataset from a cohort of Chinese-descent adults also residing in New Zealand (Tū Ora dietary intervention). Mean fasting plasma glucose (FPG) was matched between cohorts in this sub-analysis, a consequence of which was a higher mean BMI in the European group. The issue of confounding due to difference in BMI was mitigated through the use of permutational analysis of variance (PERMANOVA). Cohorts were defined and named in accordance with Statistics New Zealand terminology. This allowed us to consider differences which may relate to ethnicity as well as glucoregulatory markers and body composition.
2. Materials and methods
2.1. Study design and participants
This study utilized cross-sectional baseline data from two separate clinical trials, each focusing on prevention of T2D in individuals with overweight and prediabetes. The dataset from the Chinese-ancestry cohort was obtained from the Tū Ora dietary intervention, and the European-ancestry cohort from the PREVIEW lifestyle intervention (Raben et al., 2021; Jian et al., 2022). Cohorts were defined and named in accordance with Statistics New Zealand terminology. Both clinical trials were conducted at the University of Auckland Human Nutrition Unit, New Zealand, with study participants resident predominantly in Auckland (~85%), or in Wellington (~15%), New Zealand. Participants for the current analysis were selected from their respective larger cohorts based on confirmed prediabetes, defined by raised FPG of 5.6–6.9 mmol/L (American Diabetes Association, 2018) measured in clinic. The two cohorts were matched for mean FPG. Both cohorts were also required to meet the following inclusion criteria, as named in accordance with the original publications: self-reported ‘Chinese’ (Tū Ora) or ‘Caucasian’ (PREVIEW) descent, currently resident in New Zealand, 25–70 years of age, overweight or obese defined as BMI ≥23 kg/m2 for Chinese-ancestry adults and ≥25 kg/m2 for European-ancestry adults, Finnish Diabetes Risk Score (FINDRISC) ≥9 for Chinese-ancestry adults and ≥12 for European-ancestry adults indicative of increased future risk of T2D (Lindström and Tuomilehto, 2003; Silvestre et al., 2017), and stable body weight for 2 months prior to the trial (<5% change, self-reported). Exclusion criteria for both cohorts were: smoking, pregnancy, diagnosis of any significant co-morbidities such as diabetes or cancer, medications or supplements that may influence glycaemia (self-reported). None of the participants from either cohort reported antibiotic use within 1 month prior to the trial. All participants who met all inclusion, but none of the exclusion, criteria were selected from each cohort for this current study. Human ethics approval was granted by the Health and Disabilities Ethics Committee (HDEC), New Zealand, and participants provided written, informed consent before enrolment. Tū Ora was registered with the Australian New Zealand Clinical Trial Registry (Trial ID: ACTRN12618000476235) and PREVIEW with ClinicalTrials.gov (Trial ID: NCT01777893). Thirty-two Chinese-ancestry and 39 European-ancestry participants with overweight and prediabetes were selected for the purpose of this analysis.
2.2. Clinical assessments
Participants attended their clinic visit in the morning, following an overnight fast, with procedures conducted as previously described for the PREVIEW study (Fogelholm et al., 2017). Body weight, height, waist and hip circumference, and body composition were all measured in the clinic. Body weight was measured using digital scales (Mettler Toledo Spider 2, Zurich, Switzerland) and height using a wall-mounted stadiometer (Seca 222, Hamburg, Germany), both while lightly clad and without shoes. Waist and hip circumference were measured using a non-stretch tape (Abbott Laboratories, Illinois, USA). Waist was measured at the midpoint of the lowest palpable rib and the hip joint bone, around the belly and not under, and hip at the widest point over the greater trochanter. Body composition was assessed using dual energy x-ray absorptiometry (iDXA, GE Healthcare, Madison, WI, USA). Total body fat % and abdominal fat % estimates were calculated according to the formulae:
A fasted venous blood sample was also collected for analysis of glucose (FPG) and insulin. Demographic information for participants is presented in Table 1.
2.3. Blood analyses
FPG was measured using the hexokinase method (Cobas C311 analyzer, Roche Diagnostics, Indianapolis, USA) from plasma samples in Chinese-ancestry participants, and whole blood with manual conversion to plasma glucose in European-ancestry participants (Reflotron Plus Desk Top Analyser, Mannheim, Germany) (Kim, 2016). Insulin was analyzed using Elecsys immunoassay with electrochemiluminescence technology (Cobas e411 Analyser, Roche Diagnostics, Indianapolis, USA).
2.4. Fecal sample collection
Fecal sample collection, processing and microbiological analyses were performed for both studies in the same laboratory using identical methodologies. Fecal samples were collected at home by participants into a sterile collection tube, frozen at −18°C in their home freezers and then delivered to the research clinic in a chilly bin without sample thawing (sample tubes were encased in an “ice jacket” to prevent rapid thawing). All fecal samples were then stored in the clinic at −80°C prior to DNA extraction.
2.5. DNA extraction
Genomic DNA was extracted from 0.25 g fecal sample using International Human Microbiome Standards (IHMS) Protocol #9 (Costea et al., 2017), which is a repeated bead-beating method utilizing 0.1 mm silica and 3 mm glass beads. Cell lysis was performed using a non-commercial lysis buffer recipe (500 mM NaCl, 50 mM Tris–HCl at pH 8.0, 50 mM EDTA and 4% SDS) as per the protocol, however a Qiagen Tissuelyser II (Retch) was used (frequency of 30 Hz, for two cycles of 1.5 min) to break cells instead of the FastPrep® -24 Instrument advised by the protocol. A QIAamp DNA Minikit (Qiagen, 51306) was utilized in the final steps of the protocol for removal of RNA, protein and for DNA purification, as recommended by the protocol. Negative control extractions containing 250 μL of sterile water instead of 0.25 g fecal sample were also carried out to test for potential contamination. All extracts were subsequently analyzed on a Nanodrop 3,300 fluorospectrometer (Nanodrop Technologies Inc., Wilmington, USA) to determine DNA quality and concentration.
2.6. Bacterial 16S rRNA gene amplicon sequencing
Bacterial community structure was analyzed by polymerase chain reaction (PCR) amplification, then sequencing of the highly variable V3-V4 region of the 16S rRNA gene. The KAPA High Fidelity HotStart Readymix PCR Kit (Kapa Biosystems®) was utilized, with ~50 ng of template genomic DNA used per reaction. Labeled with Illumina MiSeq-compatible adaptors, the widely used primer pair 341F (5’-CCTACGGGNGGCWGCAG-3’) and 785R (5’-GACTACHVGGGTATCTAATCC-3’) (Klindworth et al., 2013) was used with the following thermocycling conditions: initial denaturation and activation of enzymes at 95°C for 3 min, followed by 25 cycles of denaturation (95°C for 30 s), annealing (55°C for 30 s) and elongation (72°C for 30 s), with a final extension of 72°C for 10 min. PCR products were electrophoresed on 1% (w/v) agarose gels with SYBR Safe nucleic acid stain (Invitrogen Co., USA) to ensure correct amplicon size. Negative PCR controls, in which nuclease-free H2O was used instead of template DNA, as well as amplifications of eluates from the negative DNA extractions, did not produce any visible products. Randomly selected negative controls were nonetheless submitted for sequencing even if no product was visible on an agarose gel. PCR amplicons were purified using AMPure magnetic beads (Beckman-Coulter Inc., USA) in accordance with the manufacturer’s instructions, and quantified using a Qubit dsDNA high-sensitivity kit (Invitrogen Co., USA). Standardized concentrations of the purified samples were submitted to Auckland Genomics Ltd. for Illumina MiSeq sequencing (2 × 300 bp chemistry).
2.7. Bioinformatics
A bioinformatics pipeline previously described by Hoggard et al. (2019), and compatible with the USEARCH (Edgar, 2010) software package, was utilized to join the raw pairs of 16S rRNA gene sequence reads, remove primer-binding regions, quality filter and study the merged sequences (Edgar et al., 2011). Merged sequence reads were further error-corrected and zero-radius operational taxonomic units (zOTUs) of 100% similarity were generated (Edgar, 2016a). Non-target (e.g., human-derived) sequences were removed and the SILVA v123 database used to assign taxonomy to each zOTU (Quast et al., 2013; Edgar, 2016b). All unassigned sequences were manually checked via BLAST nucleotide search and any sequences producing non-target (i.e., not bacteria) hits were removed (Johnson et al., 2008). The sequence depth of each sample was rarefied to 2,405 reads (range: 3–69,963), which was the lowest practical number of reads obtained across samples, to ensure an even number of sequences would be compared for each sample during downstream statistical analysis. The 16S rRNA gene sequence data from the Chinese-ancestry cohort were deposited in Genbank under SRA Bioproject PRJNA900794, and European-ancestry cohort data in the European Nucleotide Archive under accession number PRJEB43667.
2.8. Statistical analysis
The statistical environment R was used for all analyses (R Core Team, 2022), with a value of p <0.05 considered to be statistically significant. Statistical analysis was performed using all available data from all participants. Fisher test was utilized for testing participant distribution by categorical parameters (i.e., clinical site and sex), while distribution by all other continuous constraints was tested with a two-sample t-test. A two-sample t-test was also used to compare gut microbiota diversity between cohorts and Bray–Curtis dissimilarity was utilized for the non-metric multidimensional scaling (nMDS) analysis of bacterial community structure. Significance of variance was determined with PERMANOVA, performing 9,999 unrestricted permutations of the raw data. Covariate analyses with PERMANOVA included testing for associations between ethnicity and key demographic variables (i.e., age and sex) and metabolic variables (i.e., FPG and BMI). Further details pertaining to the PERMANOVA analysis, including the R script used, are provided in the Supplementary material. Differential zOTU abundances were calculated using the log2 fold-change of counts data (based on European-ancestry cohort as the reference factor, representing a value of 0). Standard error (SEM) estimates were used for the log2 fold-change values and the Wald test was performed for the generation of p-values (subsequently adjusted for false discovery rate using the Benjamini–Hochberg procedure).
3. Results
The European-ancestry and Chinese-ancestry cohorts both demonstrated dysglycaemia characteristic of prediabetes (mean ± SD: European-ancestry 6.1 ± 0.4; Chinese-ancestry 6.0 ± 0.4 mmol/L). They were also of similar age and height but with differences in mean body weight (104.0 ± 17.4; 77.6 ± 14.2 kg), BMI (37.4 ± 6.8; 27.7 ± 4.0 kg/m2), waist and hip circumference, total body fat (44.9 ± 8; 31.6 ± 6%) and abdominal fat (52.4 ± 8.7, 40.3 ± 6.7%), all of which were significantly lower in Chinese-ancestry participants (p < 0.001). Whilst the two cohorts had similar FPG, fasting insulin was significantly higher in Chinese-ancestry participants (European-ancestry 12 ± 6.9 μU/mL; Chinese-ancestry 16.7 ± 9.9 μU/mL; p = 0.04). Sex distribution also differed between ethnicities (European-ancestry 11 M:28F; Chinese-ancestry 21 M:11F; p = 0.002). Demographic, anthropometric, metabolic and body composition data for the two cohorts are presented in Table 1.
In both cohorts, Firmicutes was by far the most abundant bacterial phylum (Figure 1), though its average relative sequence abundance was significantly higher among European-ancestry individuals (mean ± SD: 93.4 ± 5.5%) than Chinese-ancestry individuals (79.6 ± 10.4%; p < 0.001). The bacterial phyla Bacteroidetes (p = 0.001) and Actinobacteria (p = 0.006) also differed significantly between the cohorts, with both occurring at similar relative abundance in European-ancestry participants (Bacteroidetes = 2.7 ± 4.5%; Actinobacteria = 2.7 ± 2.7%), but Bacteroidetes (11 ± 11.2%) being more abundant than Actinobacteria (6.2 ± 5.8%) in Chinese-ancestry participants (p > 0.05). Members of the phyla Verrucomicrobia and Proteobacteria were also present in both cohorts, though their respective populations did not differ significantly between the cohorts. At a finer taxonomic level (i.e., zOTU), there was considerable inter-individual variation, however among those zOTUs with ≥1% overall relative sequence abundance, four taxa were significantly more abundant in European-ancestry participants, namely OTU1_Subdoligranulum (p = 0.01), OTU25_Blautia (p = 0.002), OTU45_Ruminoclostridium_5 (p = 0.004) and OTU26_Dorea (p = 0.007).
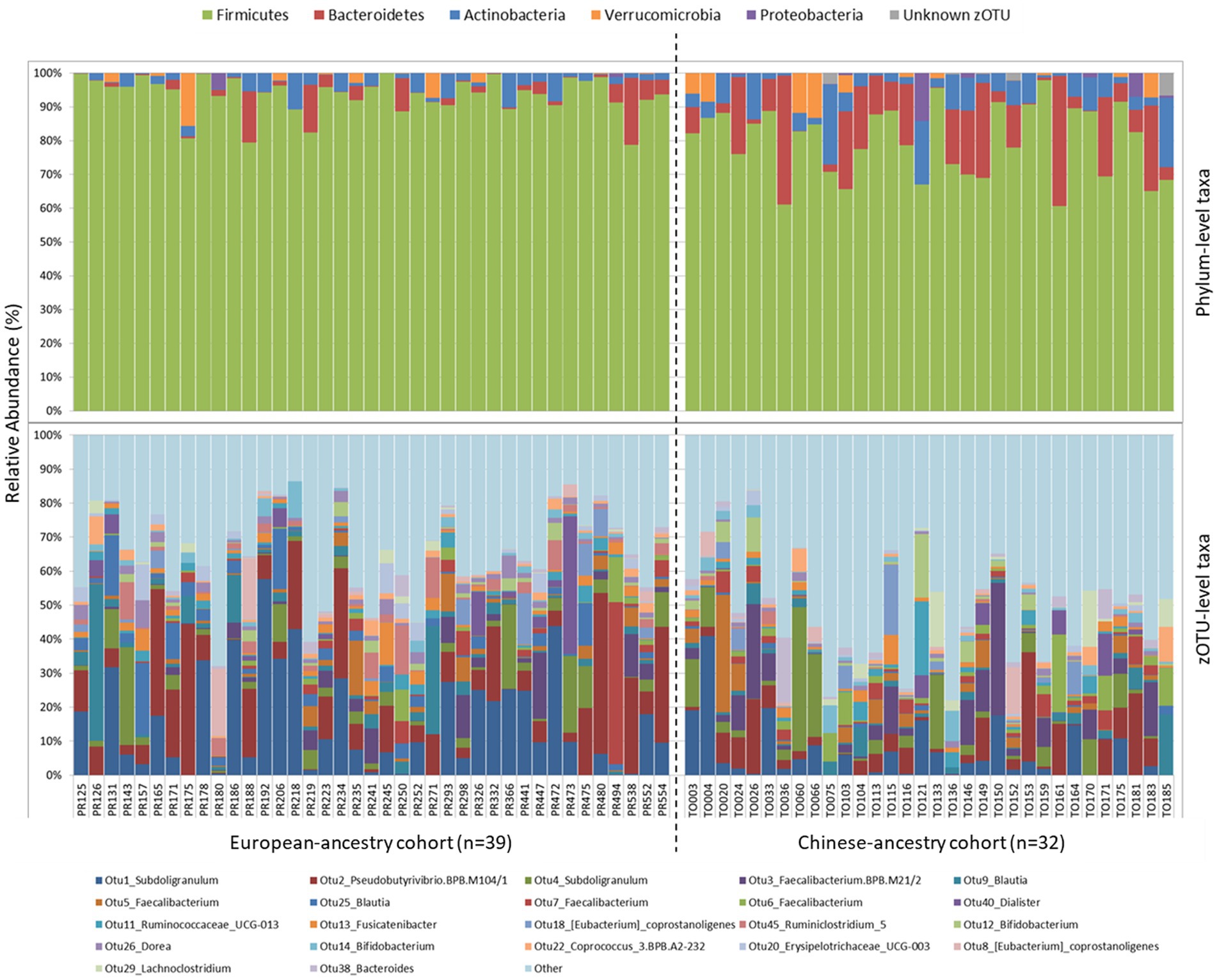
Figure 1. Phylum-level (top) and zOTU-level (bottom) gut bacterial community composition for European-ancestry and Chinese-ancestry cohorts. zOTUs with ≥1% overall 16S rRNA gene relative sequence abundance are shown, with all remaining zOTUs grouped together in “Other”.
Concerning alpha diversity (Figure 2), bacterial richness (number of observed zOTUs) did not differ significantly between the two cohorts (p = 0.526), but zOTUs were more evenly distributed in the Chinese-ancestry cohort (Shannon diversity p = 0.018). PERMANOVA revealed a highly significant difference in bacterial community composition between the two cohorts (p < 0.001; r2 = 0.051), with the outcome that ethnicity but not age, sex, BMI or FPG was identified as being significantly associated with microbiota composition when these key factors were tested against each other. This ethnicity-correlated difference in beta diversity was evident in the observed clustering of samples (albeit with some overlap) in the nMDS plot (Figure 3).
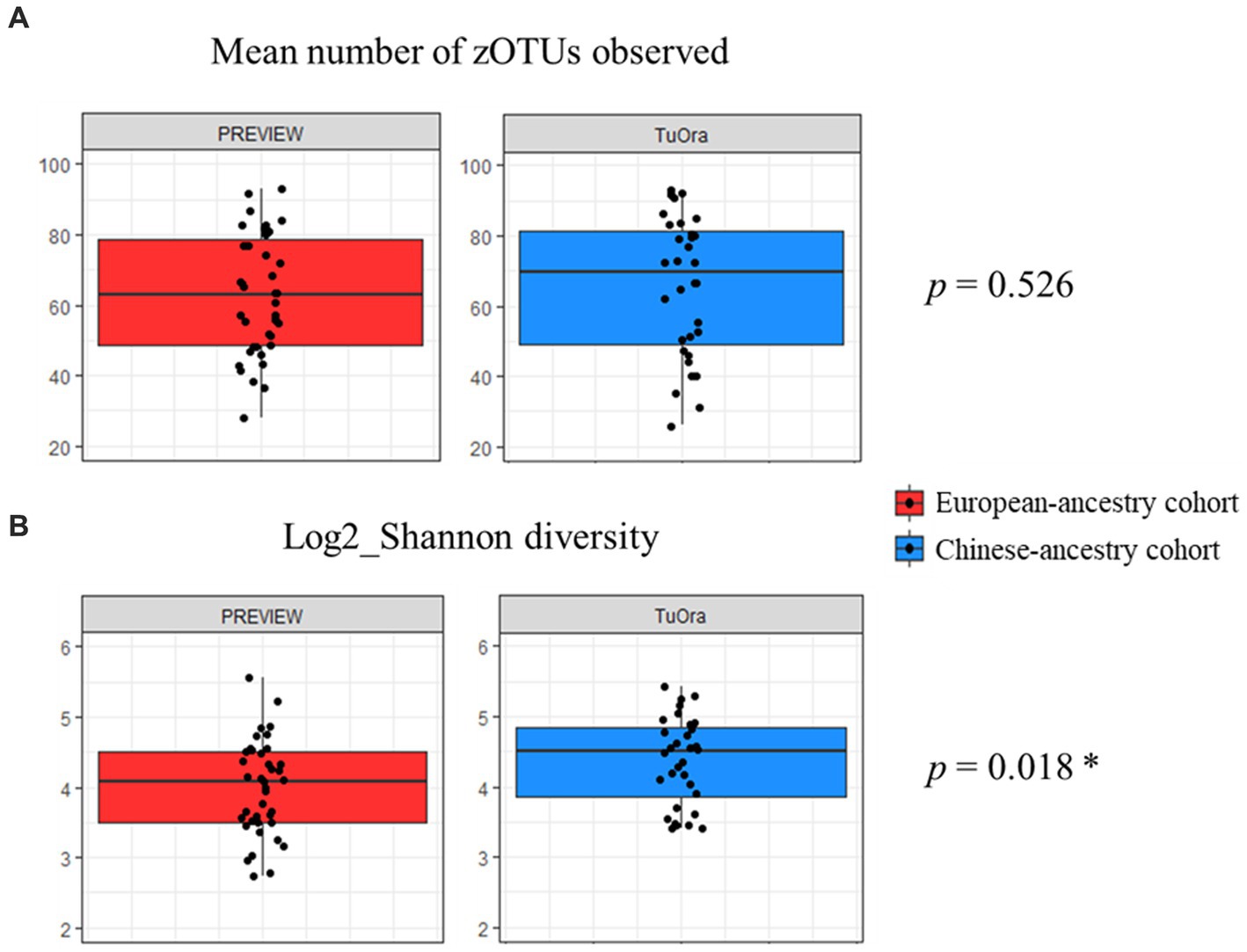
Figure 2. Mean alpha diversity parameters compared between the two ethnicity cohorts. P-values determined using unpaired t-test. (A) Mean number of observed zOTUs for each cohort. (B) Mean log2_Shannon diversity index of observed zOTUs for each cohort.
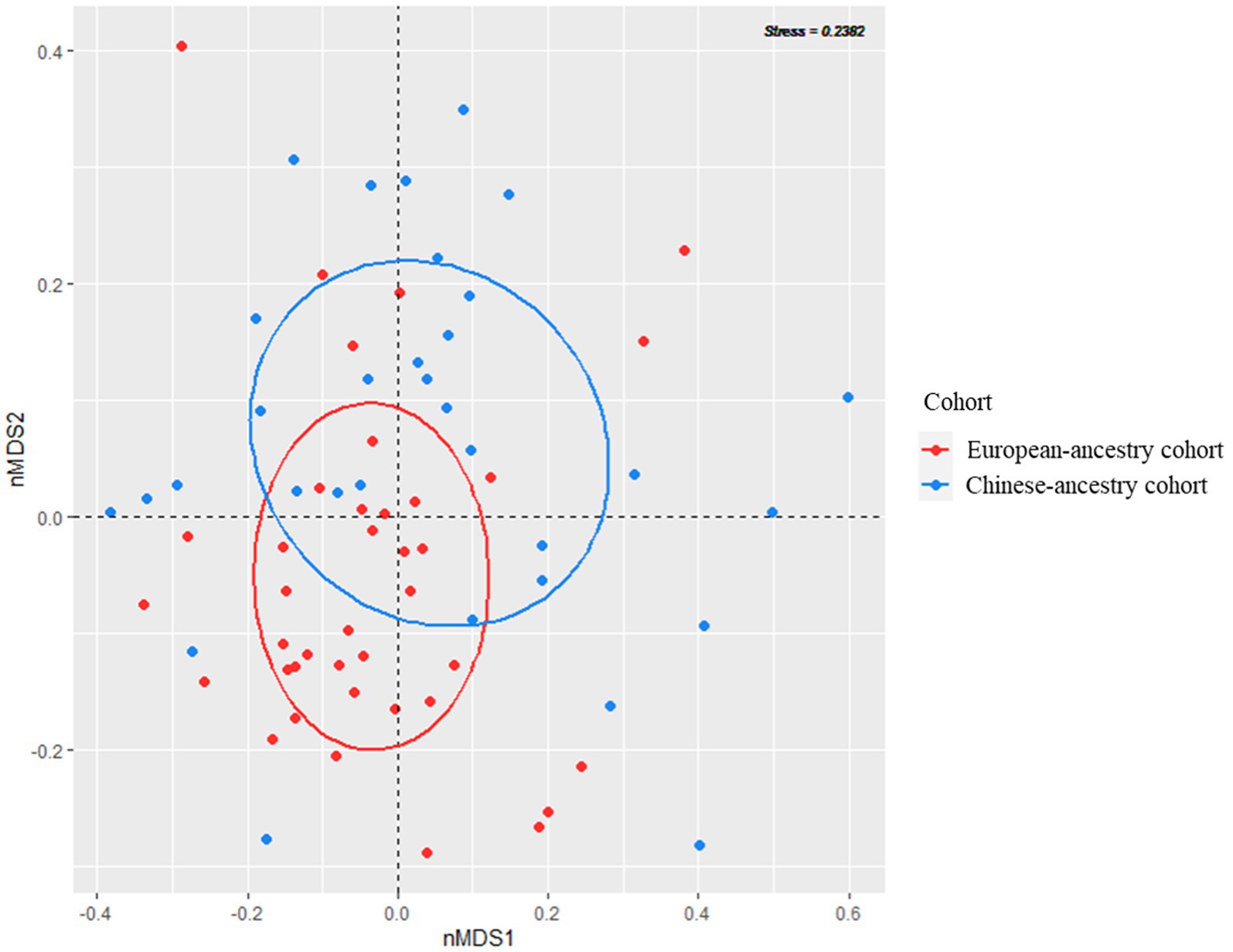
Figure 3. Visualization of bacterial community beta diversity in the two ethnicity cohorts, using non-metric multidimensional scaling (nMDS) based on Bray–Curtis dissimilarity.
Differential abundances of zOTUs across the cohorts were explored using European-ancestry participants as the reference factor (representing an abundance of 0), with the majority of differentially abundant zOTUs being more abundant among the Chinese-ancestry participants (Figure 4). No zOTUs from phyla other than Firmicutes were over-represented among European-ancestry individuals, but different members of the same genus were often observed to be differentially enriched across either ethnic cohort.
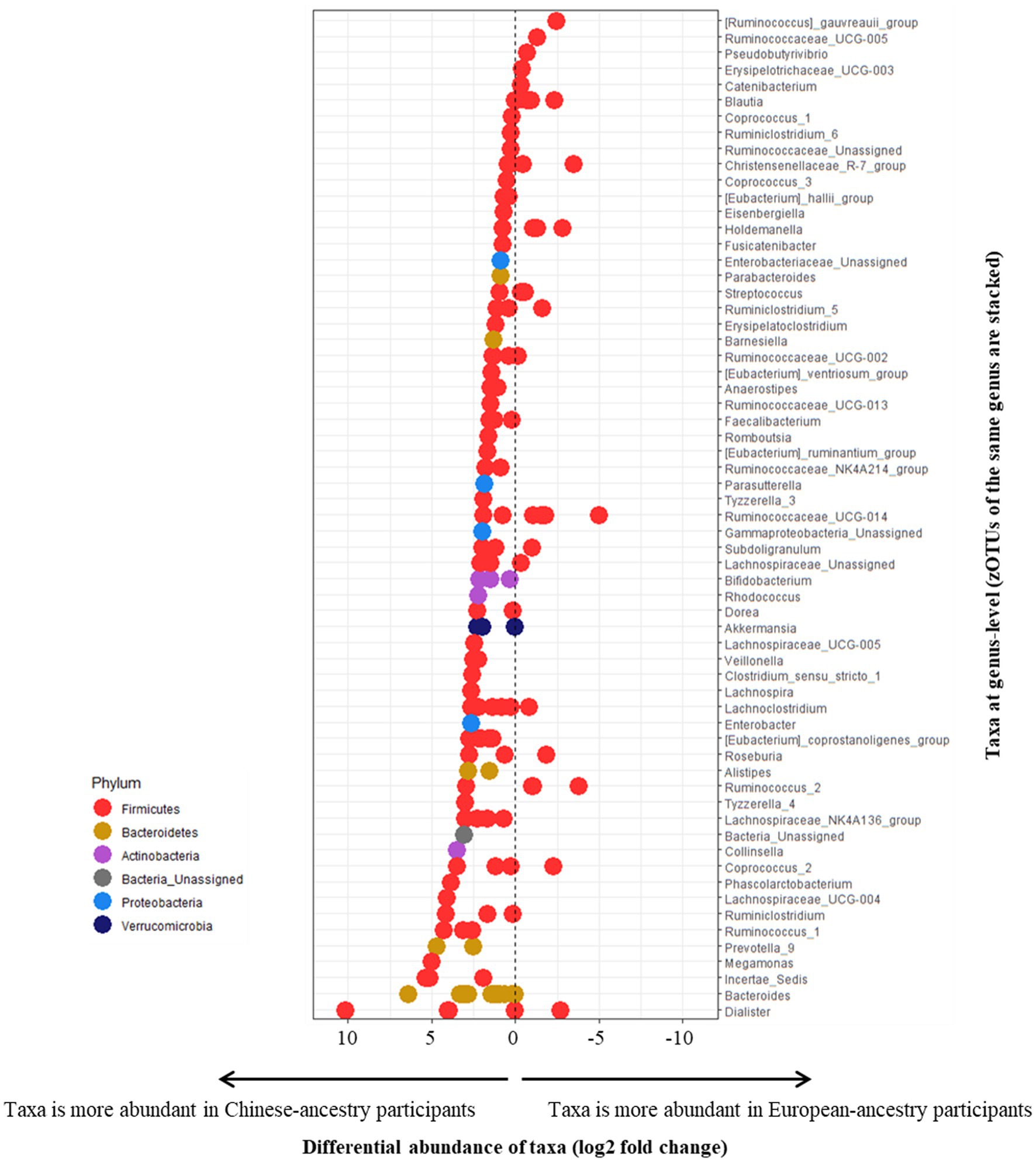
Figure 4. Differential abundance of zOTUs (based on European-ancestry participants as the reference factor, representing an abundance of 0). All positively measuring zOTUs represent taxa more abundant in Chinese-ancestry participants, and negatively measuring zOTUs represent taxa more abundant in European-ancestry participants. zOTUs measuring 0 indicate a balanced distribution of taxa across both cohorts. All zOTUs from the same genus are stacked horizontally (based on their respective differential abundance). zOTUs are color-coded by their respective phyla.
4. Discussion
The underlying hypothesis for this study was that two ethnically distinct communities, both in the same country of residence and with increased risk of T2D-onset based on fasting dysglycaemia, would differ in their gut (fecal) microbiota profiles. To address this point, we conducted a direct comparison of fecal-derived 16S rRNA gene sequence data from two separate clinical trials investigating prediabetes in Chinese-ancestry and European-ancestry communities living in New Zealand. The two cohorts were matched for mean FPG during selection of participants for this sub-analysis. Coincidentally, Chinese-ancestry participants were enrolled at a lower BMI than European-ancestry participants, likely due to their higher propensity for T2D onset at lower BMI as is consistent with the aforementioned TOFI profile. Microbiota analyses were performed separately for samples from each clinical trial, but in the same laboratory at the University of Auckland, and the methodologies utilized for fecal sample collection and processing (DNA extraction, PCR and sequencing) were identical. While sample type for FPG measurement differed between the cohorts, both are internationally accredited methods and all participants included in the current analysis were identified with prediabetes.
Although not directly assessing the TOFI phenotype, which requires measurement of ectopic fat infiltration, our findings show this Asian cohort with prediabetes to be characterized by similar age and FPG but significantly lower body weight, BMI, total and abdominal body fat, and higher hyperinsulinaemia compared to the European-ancestry cohort. This aligns with the hypothesis that Chinese-ancestry participants may have increased T2D susceptibility at lower BMI with accompanying lower total and upper body (abdominal) fat. In a previous larger study using MRI techniques to assess body composition in a different Chinese-ancestry cohort also residing in Auckland, we have shown % pancreas and visceral fat to have the strongest positive correlation with FPG, independent of age and % total body fat (Sequeira et al., 2022). In the context of gut microbiota structure, we observed significant differences between the two ethnic cohorts, with key differences in relative abundances of the Firmicutes, Bacteroidetes, and Actinobacteria bacterial phyla. While our microbiota findings could in principle reflect the substantial difference in, for example, BMI between the two cohorts, we note that ethnicity but not BMI was statistically significant in our analyses. The greater relative abundance of Firmicutes observed in the European-ancestry cohort may have contributed to this cohort’s lower overall Shannon diversity. These findings are consistent with those from recent large-scale studies in the USA (Brooks et al., 2018) and Netherlands (Deschasaux et al., 2018), in ethnically diverse participants from common urban environments. Here in our current analysis, as per PERMANOVA analyses, ethnicity also had a strong influence on gut bacterial composition in participants with fasting dysglycaemia consistent with prediabetes. It is unlikely that genetic factors are the only important characteristics that may be driving microbiota diversity, but we propose that they are likely to contribute. Interestingly, there is some evidence that such differences mitigate over time as immigrants adapt to a Western lifestyle (Vangay et al., 2018), however this could not be assessed in our current cohorts as data on years of residence in New Zealand were not collected in the original studies. Conversely, no significant effect of ethnicity on gut microbiota composition was observed in a large Middle Eastern cohort (Rothschild et al., 2018), though this could potentially be due to more homogenous local dietary and lifestyle factors in comparison to the social diversity observed in Western countries.
In a recent multi-omics study, Ang et al. (2021) investigated the gut microbiome profiles of 46 East Asian and White individuals resident in the San Francisco Bay area, across a range of body composition profiles from lean to those with obesity, and showed a strong ethnically-associated distinction between the two cohorts. Consistent with our findings, they reported a high proportion of Firmicutes in individuals with obesity and also noted the East Asian cohort to have a higher relative abundance of Bacteroidetes. Unexpectedly, although they were able to explain some of the observed gut microbiota differences by ethnicity and geography, they did not identify any associations with host diet. Methodological differences in dietary data collection between the two origin studies in our current analysis prevented a quantitative comparison of background diet, but this would be of interest to determine. Moreover, the observed ethnicity-associated differences in the study of Ang and colleagues were stronger in lean individuals, indicating a potential capacity for obesity-related influences to “overwrite” or diminish ethnicity-associated microbial signatures. Although they suggest the possibility of a shared ethnicity-independent microbiome pattern that increases host susceptibility to obesity onset (Ang et al., 2021), such outcomes elevate the importance of controlling for ethnicity (in addition to demographic and metabolic parameters) in studies exploring associations between the gut microbiota and diseases of interest.
All samples analyzed in this study were obtained from participants with overweight or obesity and prediabetes. Perhaps unsurprisingly, among the highly abundant zOTUs (≥1% overall 16S rRNA gene relative sequence abundance) observed, several bacterial genera were previously associated with prediabetes as well as frank T2D. Bacteria negatively correlated with diabetes such as Faecalibacterium (4 zOTUs) (Furet et al., 2010; Graessler et al., 2013; Karlsson et al., 2013; Zhang et al., 2013; Remely et al., 2014; Murphy et al., 2017; Gao et al., 2018; Salamon et al., 2018; Ghaemi et al., 2020; Molinaro et al., 2020), Bifidobacterium (Wu et al., 2010, 2017; Xu et al., 2015; Candela et al., 2016; Pedersen et al., 2016; Sedighi et al., 2017; Barengolts et al., 2018; Gao et al., 2018; Ghaemi et al., 2020) (2 zOTUs in Chinese-ancestry participants) and Bacteroides (Munukka et al., 2012; Zhang et al., 2013, 2020; Candela et al., 2016; Yamaguchi et al., 2016; Lippert et al., 2017; Ghaemi et al., 2020) were found in both cohorts, with the further addition of Akkermansia (Everard et al., 2013; Zhang et al., 2013, 2020; Greer et al., 2016; Allin et al., 2018; Ghaemi et al., 2020) in Chinese-ancestry participants. Both cohorts also harbored two bacteria previously shown to be positively associated with T2D, Blautia (Zhang et al., 2013, 2020; Egshatyan et al., 2016; Inoue et al., 2017; Lippert et al., 2017; He et al., 2018) (3 zOTUs in European-ancestry participants) and Ruminococcus (Zhang et al., 2013; Candela et al., 2016; Patrone et al., 2016; Allin et al., 2018; Salamon et al., 2018; Molinaro et al., 2020) (2 zOTUs in European-ancestry participants and 3 zOTUs in Chinese-ancestry participants). While butyrate-producing bacteria are generally considered to be negatively associated with T2D co-morbidities (Vital et al., 2017) such as adipocyte inflammation (Wang et al., 2015) and insulin resistance (Gao et al., 2009, 2019), butyrate producers in the genera Pseudobutyrivibrio (Xu et al., 2015; Nie et al., 2019; Zhao et al., 2019), Coprococcus (Chávez-Carbajal et al., 2019) and Anaerostipes (Org et al., 2017; Zhao et al., 2019) have previously been linked with T2D and metabolic syndrome, hence their precise role remains unclear. In this study, both cohorts contained a high abundance of Pseudobutyrivibrio and Coprococcus, as well as Anaerostipes in the Asian cohort. Nevertheless, and as evident from our differential abundance analyses (Figure 4), there can exist considerable variation at the zOTU level within a given genus (such as Dialister, Ruminococcaceae_UCG-014), with different members often differentially enriched in either ethnic cohort despite their close genetic distances.
5. Conclusion
Our findings have revealed a clear distinction between the gut microbiota profile of two disparate communities from the same country of residence, predominantly from the city of Auckland. These were a European-ancestry cohort with prediabetes and a Chinese-ancestry cohort with prediabetes plus phenotype traits consistent with TOFI (i.e., dysglycaemia and possible early pancreatic β-cell dysfunction despite a lean external appearance). While sex distribution did differ between the two cohorts, our findings support the notion that ethnicity, defined as self-perceived cultural affiliation encompassing diet, lifestyle and genetic components, may at least partially explain some of the commonly observed high variation in the gut microbiota among individuals. However, even after accounting for ethnicity we observed substantial inter-individual variation in zOTU-level assignments within each cohort. Human diet, lifestyle and genetics are broad characteristics that have been proposed to shape gut microbiota composition, and are likely all contributing in our current study together with other factors that may affect these highly host-specific variables. A notable recent gut microbiome study conveyed the importance of controlling for frequency of alcohol consumption and bowel movement quality (based on the Bristol stool scale), among other factors (Vujkovic-Cvijin et al., 2020). Social factors such as family size, living conditions, income, religion, education and employment in turn may all have an impact on diet and lifestyle and therefore should also be considered. Our findings underscore the potential influence of ethnicity on gut microbiota composition in the context of individuals with overweight and prediabetes.
Data availability statement
The datasets presented in this study can be found in online repositories. The names of the repository/repositories and accession number(s) can be found at: https://www.ncbi.nlm.nih.gov/genbank/, SRA Bioproject PRJNA900794 for the Chinese-ancestry cohort. The data for the European-ancestry cohort is held in the European Nucleotide Archive under accession number PRJEB43667.
Ethics statement
The studies involving humans were approved by Health and Disabilities Ethics Committee (HDEC), New Zealand. The studies were conducted in accordance with the local legislation and institutional requirements. The participants provided their written informed consent to participate in this study.
Author contributions
AM: metadata collection and database entry, performed lab work (DNA extraction, amplification, purification), processed sequence data, statistical analyses, data interpretation, and primary manuscript preparation. LL: conducted the Tū Ora clinical trial. IS-B: study design and clinical trial oversight. MS: conducted the PREVIEW clinical trial. MH: performed lab work (DNA extraction, amplification, purification). DB: statistical analyses. MF: PREVIEW clinical trial PI and study design. AR: PREVIEW project and clinical trial fund raiser, study design. SP: fund raiser, study design, trial supervision, data interpretation, and manuscript preparation. MT: fund raiser, data interpretation, and manuscript preparation. All authors reviewed and edited the manuscript.
Funding
This study was supported by the New Zealand National Science Challenge High Value Nutrition Program, Ministry for Business, Innovation and Employment (MBIE grant #3710040), EU 7th Framework Programme (FP7/2007–2013, grant #312057), New Zealand Health Research Council (grant #14/191), University of Auckland Faculty Research Development Fund (grants #3702182 and #3706738), and Maurice Wilkins Centre Flexible Research Programme (grant #3713851).
Acknowledgments
We thank research nurses Madhavi Bolleneni and Clarence Vivar, plus our team of research students for assistance at the Human Nutrition Unit; also Lindsay Plank, Director of the Body Composition Unit. We are also grateful to the participants in the PREVIEW and Tū Ora intervention studies.
Conflict of interest
AR received honoraria from Unilever, Nestlé, and the International Sweeteners Association.
The remaining authors declare that the research was conducted in the absence of any commercial or financial relationships that could be construed as a potential conflict of interest.
Publisher’s note
All claims expressed in this article are solely those of the authors and do not necessarily represent those of their affiliated organizations, or those of the publisher, the editors and the reviewers. Any product that may be evaluated in this article, or claim that may be made by its manufacturer, is not guaranteed or endorsed by the publisher.
Supplementary material
The Supplementary material for this article can be found online at: https://www.frontiersin.org/articles/10.3389/fmicb.2023.1244179/full#supplementary-material
References
Akiyama, C. (2008). Bridging the gap between two cultures: an analysis on identity attitudes and attachment of Asian Americans. Br Treat Cris Interv 8, 251–263. doi: 10.1093/brief-treatment/mhn015
Allin, K. H., Tremaroli, V., Caesar, R., Jensen, B. A. H., Damgaard, M. T. F., Bahl, M. I., et al. (2018). Aberrant intestinal microbiota in individuals with prediabetes. Diabetologia 61, 810–820. doi: 10.1007/s00125-018-4550-1
American Diabetes Association (2018). Classification and diagnosis of diabetes: standards of medical care in diabetes 2018. Diabetes Care 41, S13–S27. doi: 10.2337/dc18-S002
Ang, Q. Y., Alba, D. L., Upadhyay, V., Bisanz, J. E., Cai, J., Lee, H. L., et al. (2021). The east Asian gut microbiome is distinct from colocalized white subjects and connected to metabolic health. eLife 10:E70349. doi: 10.7554/elife.70349
Barengolts, E., Green, S. J., Eisenberg, Y., Akbar, A., Reddivari, B., Layden, B. T., et al. (2018). Gut microbiota varies by opioid use, circulating leptin and oxytocin in African American men with diabetes and high burden of chronic disease. PLoS One 13:E0194171. doi: 10.1371/journal.pone.0194171
Brooks, A. W., Priya, S., Blekhman, R., and Bordenstein, S. R. (2018). Gut microbiota diversity across ethnicities in the United States. PLoS Biol. 16, E2006842–e2006842. doi: 10.1371/journal.pbio.2006842
Candela, M., Biagi, E., Soverini, M., Consolandi, C., Quercia, S., Severgnini, M., et al. (2016). Modulation of gut microbiota dysbioses in type 2 diabetic patients by macrobiotic Ma-Pi 2 diet. Br. J. Nutr. 116, 80–93. doi: 10.1017/S0007114516001045
Chávez-Carbajal, A., Nirmalkar, K., Pérez-Lizaur, A., Hernández-Quiroz, F., Ramírez-del-Alto, S., García-Mena, J., et al. (2019). Gut microbiota and predicted metabolic pathways in a sample of Mexican women affected by obesity and obesity plus metabolic syndrome. Int. J. Mol. Sci. 20:438. doi: 10.3390/ijms20020438
Clarke, G., Stilling, R. M., Kennedy, P. J., Stanton, C., Cryan, J. F., and Dinan, T. G. (2014). Minireview: gut microbiota: the neglected endocrine organ. Mol. Endocrinol. 28, 1221–1238. doi: 10.1210/me.2014-1108
Cortés, V. A., and Fernández-Galilea, M. (2015). Lipodystrophies: adipose tissue disorders with severe metabolic implications. J. Physiol. Biochem. 71, 471–478. doi: 10.1007/s13105-015-0404-1
Costea, P. I., Zeller, G., Sunagawa, S., Pelletier, E., Alberti, A., Levenez, F., et al. (2017). Towards standards for human fecal sample processing in metagenomic studies. Nat. Biotechnol. 35, 1069–1076. doi: 10.1038/nbt.3960
Deschasaux, M., Bouter, K. E., Prodan, A., Levin, E., Groen, A. K., Herrema, H., et al. (2018). Depicting the composition of gut microbiota in a population with varied ethnic origins but shared geography. Nat. Med. 24, 1526–1531. doi: 10.1038/s41591-018-0160-1
Dickinson, S., Colagiuri, S., Petocz, P., Brand-Miller, J. C., and Faramus, E. (2002). Postprandial hyperglycemia and insulin sensitivity differ among lean young adults of different ethnicities. J. Nutr. 132, 2574–2579. doi: 10.1093/jn/132.9.2574
Duvallet, C., Gibbons, S. M., Gurry, T., Irizarry, R. A., and Alm, E. J. (2017). Meta-analysis of gut microbiome studies identifies disease-specific and shared responses. Nat. Commun. 8:1784. doi: 10.1038/s41467-017-01973-8
Edgar, R. C. (2010). Search and clustering orders of magnitude faster than BLAST. Bioinformatics 26, 2460–2461. doi: 10.1093/bioinformatics/btq461
Edgar, R. C. (2016a). UNOISE2: improved error-correction for Illumina 16S and ITS amplicon sequencing. bioRxiv :81257. doi: 10.1101/081257
Edgar, R. C. (2016b). SINTAX: a simple non-Bayesian taxonomy classifier for 16S and ITS sequences. bioRxiv :74161. doi: 10.1101/074161
Edgar, R. C., Haas, B. J., Clemente, J. C., Quince, C., and Knight, R. (2011). UCHIME improves sensitivity and speed of chimera detection. Bioinformatics 27, 2194–2200. doi: 10.1093/bioinformatics/btr381
Egshatyan, L., Kashtanova, D., Popenko, A., Tkacheva, O., Tyakht, A., Alexeev, D., et al. (2016). Gut microbiota and diet in patients with different glucose tolerance. Endocr. Connect. 5, 1–9. doi: 10.1530/EC-15-0094
Everard, A., Belzer, C., Geurts, L., Ouwerkerk, J. P., Druart, C., Bindels, L. B., et al. (2013). Cross-talk between Akkermansia muciniphila and intestinal epithelium controls diet-induced obesity. Proc. Natl. Acad. Sci. U. S. A. 110, 9066–9071. doi: 10.1073/pnas.1219451110
Fogelholm, M., Larsen, T. M., Westerterp-Plantenga, M., Macdonald, I., Martinez, J., Boyadjieva, N., et al. (2017). PREVIEW: prevention of diabetes through lifestyle intervention and population studies in Europe and around the world. Design, methods, and baseline participant description of an adult cohort enrolled into a three-year randomised clinical trial. Nutrients 9:e632. doi: 10.3390/nu9060632
Furet, J.-P., Kong, L.-C., Tap, J., Poitou, C., Basdevant, A., Bouillot, J. L., et al. (2010). Differential adaptation of human gut microbiota to bariatric surgery-induced weight loss: links with metabolic and low-grade inflammation markers. Diabetes 59, 3049–3057. doi: 10.2337/db10-0253
Gao, F., Lv, Y.-W., Long, J., Chen, J. M., He, J. M., Ruan, X. Z., et al. (2019). Butyrate improves the metabolic disorder and gut microbiome dysbiosis in mice induced by a high-fat diet. Front. Pharmacol. 10:1040. doi: 10.3389/fphar.2019.01040
Gao, Z., Yin, J., Zhang, J., Ward, R. E., Martin, R. J., Lefevre, M., et al. (2009). Butyrate improves insulin sensitivity and increases energy expenditure in mice. Diabetes 58, 1509–1517. doi: 10.2337/db08-1637
Gao, R., Zhu, C., Li, H., Yin, M., Pan, C., Huang, L., et al. (2018). Dysbiosis signatures of gut microbiota along the sequence from healthy, young patients to those with overweight and obesity. Obesity (Silver Spring) 26, 351–361. doi: 10.1002/oby.22088
Gaulke, C. A., and Sharpton, T. J. (2018). The influence of ethnicity and geography on human gut microbiome composition. Nat. Med. 24, 1495–1496. doi: 10.1038/s41591-018-0210-8
Ghaemi, F., Fateh, A., Sepahy, A. A., Zangeneh, M., Ghanei, M., and Siadat, S. D. (2020). Intestinal microbiota composition in Iranian diabetic, pre-diabetic and healthy individuals. J. Diabetes Metab. Disord. 19, 1199–1203. doi: 10.1007/s40200-020-00625-x
Golden, S. H., Brown, A., Cauley, J. A., Chin, M. H., Gary-Webb, T. L., Kim, C., et al. (2012). Health disparities in endocrine disorders: biological, clinical, and nonclinical factors--an Endocrine Society scientific statement. J. Clin. Endocrinol. Metab. 97, E1579–E1639. doi: 10.1210/jc.2012-2043
Graessler, J., Qin, Y., Zhong, H., Zhang, J., Licinio, J., Wong, M. L., et al. (2013). Metagenomic sequencing of the human gut microbiome before and after bariatric surgery in obese patients with type 2 diabetes: correlation with inflammatory and metabolic parameters. Pharmacogenomics J. 13, 514–522. doi: 10.1038/tpj.2012.43
Greer, R. L., Dong, X., Moraes, A. C. F., Zielke, R. A., Fernandes, G. R., Peremyslova, E., et al. (2016). Akkermansia muciniphila mediates negative effects of IFNγ on glucose metabolism. Nat. Commun. 7:13329. doi: 10.1038/ncomms13329
Haldar, S., Chia, S. C., and Henry, C. J. (2015). Body composition in Asians and Caucasians: comparative analyses and influences on cardiometabolic outcomes. Adv. Food Nutr. Res. 75, 97–154. doi: 10.1016/bs.afnr.2015.07.001
He, Y., Wu, W., Zheng, H.-M., Li, P., McDonald, D., Sheng, H. F., et al. (2018). Regional variation limits applications of healthy gut microbiome reference ranges and disease models. Nat. Med. 24, 1532–1535. doi: 10.1038/s41591-018-0164-x
Hoggard, M., Zoing, M., Biswas, K., Taylor, M. W., and Douglas, R. G. (2019). The sinonasal mycobiota in chronic rhinosinusitis and control patients. Rhinology 190–199. doi: 10.4193/Rhin18.256
Inoue, R., Ohue-Kitano, R., Tsukahara, T., Tanaka, M., Masuda, S., Inoue, T., et al. (2017). Prediction of functional profiles of gut microbiota from 16S rRNA metagenomic data provides a more robust evaluation of gut dysbiosis occurring in Japanese type 2 diabetic patients. J. Clin. Biochem. Nutr. 61, 217–221. doi: 10.3164/jcbn.17-44
Jian, C., Silvestre, M. P., Middleton, D., Korpela, K., Jalo, E., Broderick, D., et al. (2022). Gut microbiota predicts body fat change following a low-energy diet: a PREVIEW intervention study. Genome Med. 14:54. doi: 10.1186/s13073-022-01053-7
Jo, A., and Mainous, A. G. III. (2018). Informational value of percent body fat with body mass index for the risk of abnormal blood glucose: a nationally representative cross-sectional study. BMJ Open 8:E019200. doi: 10.1136/bmjopen-2017-019200
Johnson, M., Zaretskaya, I., Raytselis, Y., Merezhuk, Y., McGinnis, S., and Madden, T. L. (2008). NCBI BLAST: A better web interface. Nucleic Acids Res. 36, W5–W9. doi: 10.1093/nar/gkn201
Karlsson, F. H., Tremaroli, V., Nookaew, I., Bergström, G., Behre, C. J., Fagerberg, B., et al. (2013). Gut metagenome in European women with normal, impaired and diabetic glucose control. Nature 498, 99–103. doi: 10.1038/nature12198
Kim, H. S. (2016). Blood glucose measurement: is serum equal to plasma? Diabetes Metab. J. 40, 365–366. doi: 10.4093/dmj.2016.40.5.365
Klindworth, A., Pruesse, E., Schweer, T., Peplies, J., Quast, C., Horn, M., et al. (2013). Evaluation of general 16S ribosomal RNA gene PCR primers for classical and next-generation sequencing-based diversity studies. Nucleic Acids Res. 41, E1–e1. doi: 10.1093/nar/gks808
Lear, S. A., Humphries, K. H., Kohli, S., Chockalingam, A., Frohlich, J. J., and Birmingham, C. L. (2007). Visceral adipose tissue accumulation differs according to ethnic background: results of the multicultural community health assessment trial (M-CHAT). Am. J. Clin. Nutr. 86, 353–359. doi: 10.1093/ajcn/86.2.353
LeBlanc, J. G., Milani, C., de Giori, G. S., Sesma, F., van Sinderen, D., and Ventura, M. (2013). Bacteria as vitamin suppliers to their host: a gut microbiota perspective. Curr. Opin. Biotechnol. 24, 160–168. doi: 10.1016/j.copbio.2012.08.005
Lee, Y., Hirose, H., Ohneda, M., Johnson, J. H., McGarry, J. D., and Unger, R. H. (1994). Beta-cell lipotoxicity in the pathogenesis of non-insulin-dependent diabetes mellitus of obese rats: impairment in adipocyte-beta-cell relationships. Proc. Natl. Acad. Sci. U. S. A. 91, 10878–10882. doi: 10.1073/pnas.91.23.10878
Liew, C.-F., Seah, E.-S., Yeo, K.-P., Lee, K. O., and Wise, S. D. (2003). Lean, nondiabetic Asian Indians have decreased insulin sensitivity and insulin clearance, and raised leptin compared to Caucasians and Chinese subjects. Int J Obes Relat Metab Disord J Int Assoc Study Obes 27, 784–789. doi: 10.1038/sj.ijo.0802307
Lindström, J., and Tuomilehto, J. (2003). The diabetes risk score: a practical tool to predict type 2 diabetes risk. Diabetes Care 26, 725–731. doi: 10.2337/diacare.26.3.725
Lippert, K., Kedenko, L., Antonielli, L., Kedenko, I., Gemeier, C., Leitner, M., et al. (2017). Gut microbiota dysbiosis associated with glucose metabolism disorders and the metabolic syndrome in older adults. Benef Microbes 8, 545–556. doi: 10.3920/BM2016.0184
Liu, A. Y., Silvestre, M. P., and Poppitt, S. D. (2015). Prevention of type 2 diabetes through lifestyle modification: is there a role for higher-protein diets? Adv. Nutr. 6, 665–673. doi: 10.3945/an.115.008821
Misra, A., and Khurana, L. (2011). Obesity-related non-communicable diseases: south Asians vs white Caucasians. Int. J. Obes. 35, 167–187. doi: 10.1038/ijo.2010.135
Molinaro, A., Bel Lassen, P., Henricsson, M., Wu, H., Adriouch, S., Belda, E., et al. (2020). Imidazole propionate is increased in diabetes and associated with dietary patterns and altered microbial ecology. Nat. Commun. 11:5881. doi: 10.1038/s41467-020-19589-w
Munukka, E., Wiklund, P., Pekkala, S., Völgyi, E., Xu, L., Cheng, S., et al. (2012). Women with and without metabolic disorder differ in their gut microbiota composition. Obesity (Silver Spring) 20, 1082–1087. doi: 10.1038/oby.2012.8
Murphy, R., Tsai, P., Jüllig, M., Liu, A., Plank, L., and Booth, M. (2017). Differential changes in gut microbiota after gastric bypass and sleeve gastrectomy bariatric surgery vary according to diabetes remission. Obes. Surg. 27, 917–925. doi: 10.1007/s11695-016-2399-2
Nazare, J.-A., Smith, J. D., Borel, A.-L., Haffner, S. M., Balkau, B., Ross, R., et al. (2012). Ethnic influences on the relations between abdominal subcutaneous and visceral adiposity, liver fat, and cardiometabolic risk profile: the international study of prediction of intra-abdominal adiposity and its relationship with cardiometabolic risk/intra. Am. J. Clin. Nutr. 96, 714–726. doi: 10.3945/ajcn.112.035758
New Zealand Society for the Study of Diabetes (2023) Type 2 diabetes management guidelines: prediabetes, Ministry of Health Manatū Hauora, Available at: https://t2dm.nzssd.org.nz/Section-98-Prediabetes (Accessed September 30, 2023)
Nie, Q., Chen, H., Hu, J., Fan, S., and Nie, S. (2019). Dietary compounds and traditional Chinese medicine ameliorate type 2 diabetes by modulating gut microbiota. Crit. Rev. Food Sci. Nutr. 59, 848–863. doi: 10.1080/10408398.2018.1536646
Org, E., Blum, Y., Kasela, S., Mehrabian, M., Kuusisto, J., Kangas, A. J., et al. (2017). Relationships between gut microbiota, plasma metabolites, and metabolic syndrome traits in the METSIM cohort. Genome Biol. 18:70. doi: 10.1186/s13059-017-1194-2
Patrone, V., Vajana, E., Minuti, A., Callegari, M. L., Federico, A., Loguercio, C., et al. (2016). Postoperative changes in fecal bacterial communities and fermentation products in obese patients undergoing bilio-intestinal bypass. Front. Microbiol. 7:200. doi: 10.3389/fmicb.2016.00200
Pedersen, C., Gallagher, E., Horton, F., Ellis, R. J., Ijaz, U. Z., Wu, H., et al. (2016). Host-microbiome interactions in human type 2 diabetes following prebiotic fibre (galacto-oligosaccharide) intake. Br. J. Nutr. 116, 1869–1877. doi: 10.1017/S0007114516004086
Quast, C., Pruesse, E., Yilmaz, P., Gerken, J., Schweer, T., Yarza, P., et al. (2013). The SILVA ribosomal RNA gene database project: improved data processing and web-based tools. Nucleic Acids Res. 41, D590–D596. doi: 10.1093/nar/gks1219
Quigley, E. M. M. (2013). Gut bacteria in health and disease. Gastroenterol Hepatol (NY). 9, 560–569.
R Core Team (2022) R: A language and environment for statistical computing. R foundation for statistical computing: Vienna, Austria
Raben, A., Vestentoft, P. S., Brand-Miller, J., Jalo, E., Drummen, M., Simpson, L., et al. (2021). The PREVIEW intervention study: results from a 3-year randomized 2 x 2 factorial multinational trial investigating the role of protein, glycaemic index and physical activity for prevention of type 2 diabetes. Diabetes Obes. Metab. 23, 324–337. doi: 10.1111/dom.14219
Ramachandran, A., Chamukuttan, S., Shetty, S. A., Arun, N., and Susairaj, P. (2012). Obesity in Asia – is it different from rest of the world. Diabetes Metab. Res. Rev. 28, 47–51. doi: 10.1002/dmrr.2353
Remely, M., Aumueller, E., Merold, C., Dworzak, S., Hippe, B., Zanner, J., et al. (2014). Effects of short chain fatty acid producing bacteria on epigenetic regulation of FFAR3 in type 2 diabetes and obesity. Gene 537, 85–92. doi: 10.1016/j.gene.2013.11.081
Rothschild, D., Weissbrod, O., Barkan, E., Kurilshikov, A., Korem, T., Zeevi, D., et al. (2018). Environment dominates over host genetics in shaping human gut microbiota. Nature 555, 210–215. doi: 10.1038/nature25973
Salamon, D., Sroka-Oleksiak, A., Kapusta, P., Szopa, M., Mrozińska, S., Ludwig-Słomczyńska, A. H., et al. (2018). Characteristics of gut microbiota in adult patients with type 1 and type 2 diabetes based on next-generation sequencing of the 16S rRNA gene fragment. Polish. Arch. Intern. Med. 128, 336–343. doi: 10.20452/pamw.4246
Sears, C. L. (2005). A dynamic partnership: celebrating our gut flora. Anaerobe 11, 247–251. doi: 10.1016/j.anaerobe.2005.05.001
Sedighi, M., Razavi, S., Navab-Moghadam, F., Khamseh, M. E., Alaei-Shahmiri, F., Mehrtash, A., et al. (2017). Comparison of gut microbiota in adult patients with type 2 diabetes and healthy individuals. Microb. Pathog. 111, 362–369. doi: 10.1016/j.micpath.2017.08.038
Sequeira, I. R., Yip, W. C., Lu, L. W. W., Jiang, Y., Murphy, R., Plank, L. D., et al. (2022). Pancreas fat, an early marker of metabolic risk? A magnetic resonance study of Chinese and Caucasian women: TOFI_Asia study. Front. Physiol. 13:819606. doi: 10.3389/fphys.2022.819606
Shibata, M., Kihara, Y., Taguchi, M., Tashiro, M., and Otsuki, M. (2007). Nonalcoholic fatty liver disease is a risk factor for type 2 diabetes in middle-aged Japanese men. Diabetes Care 30, 2940–2944. doi: 10.2337/dc07-0792
Silvestre, M. P., Jiang, Y., Volkova, K., Chisholm, H., Lee, W., and Poppitt, S. D. (2017). Evaluating FINDRISC as a screening tool for type 2 diabetes among overweight adults in the PREVIEW:NZ cohort. Prim. Care Diabetes 11, 561–569. doi: 10.1016/j.pcd.2017.07.003
Spanakis, E. K., and Golden, S. H. (2013). Race/ethnic difference in diabetes and diabetic complications. Curr. Diab. Rep. 13, 814–823. doi: 10.1007/s11892-013-0421-9
Te Whatu Ora: Health New Zealand (2021). Virtual diabetes register web tool. Available at: https://www.tewhatuora.govt.nz/our-health-system/data-and-statistics/virtual-diabetes-tool (Accessed September 30, 2023).
Thomas, E. L., Parkinson, J. R., Frost, G. S., Goldstone, A. P., Doré, C. J., McCarthy, J. P., et al. (2012). The missing risk: MRI and MRS phenotyping of abdominal adiposity and ectopic fat. Obesity (Silver Spring) 20, 76–87. doi: 10.1038/oby.2011.142
Vangay, P., Johnson, A. J., Ward, T. L., al-Ghalith, G. A., Shields-Cutler, R. R., Hillmann, B. M., et al. (2018). US immigration westernizes the human gut microbiome. Cells 175, 962–972.e10. doi: 10.1016/j.cell.2018.10.029
Vital, M., Karch, A., and Pieper, D. H. (2017). Colonic butyrate-producing communities in humans: an overview using omics data. mSystems 2, E00130–E00117. doi: 10.1128/msystems.00130-17
Vujkovic-Cvijin, I., Sklar, J., Jiang, L., Natarajan, L., Knight, R., and Belkaid, Y. (2020). Host variables confound gut microbiota studies of human disease. Nature 587, 448–454. doi: 10.1038/s41586-020-2881-9
Wang, X., He, G., Peng, Y., Zhong, W., Wang, Y., and Zhang, B. (2015). Sodium butyrate alleviates adipocyte inflammation by inhibiting NLRP3 pathway. Sci. Rep. 5:12676. doi: 10.1038/srep12676
WHO Expert Consultation (2004). Appropriate body-mass index for Asian populations and its implications for policy and intervention strategies. Lancet 363, 157–163. doi: 10.1016/S0140-6736(03)15268-3
Wu, H., Esteve, E., Tremaroli, V., Khan, M. T., Caesar, R., Mannerås-Holm, L., et al. (2017). Metformin alters the gut microbiome of individuals with treatment-naive type 2 diabetes, contributing to the therapeutic effects of the drug. Nat. Med. 23, 850–858. doi: 10.1038/nm.4345
Wu, X., Ma, C., Han, L., Nawaz, M., Gao, F., Zhang, X., et al. (2010). Molecular characterisation of the faecal microbiota in patients with type II diabetes. Curr. Microbiol. 61, 69–78. doi: 10.1007/s00284-010-9582-9
Xu, J., Lian, F., Zhao, L., Zhao, Y., Chen, X., Zhang, X., et al. (2015). Structural modulation of gut microbiota during alleviation of type 2 diabetes with a Chinese herbal formula. ISME J. 9, 552–562. doi: 10.1038/ismej.2014.177
Yamaguchi, Y., Adachi, K., Sugiyama, T., Shimozato, A., Ebi, M., Ogasawara, N., et al. (2016). Association of intestinal microbiota with metabolic markers and dietary habits in patients with type 2 diabetes. Digestion 94, 66–72. doi: 10.1159/000447690
Yip, W. C. Y., Sequeira, I. R., Plank, L. D., and Poppitt, S. (2017). Prevalence of pre-diabetes across ethnicities: a review of impaired fasting glucose (IFG) and impaired glucose tolerance (IGT) for classification of dysglycaemia. Nutrients 9:1273. doi: 10.3390/nu9111273
Zhang, X., Shen, D., Fang, Z., Jie, Z., Qiu, X., Zhang, C., et al. (2013). Human gut microbiota changes reveal the progression of glucose intolerance. PLoS One 8:E71108. doi: 10.1371/journal.pone.0071108
Zhang, X., Zhao, A., Sandhu, A. K., Edirisinghe, I., and Burton-Freeman, B. M. (2020). Functional deficits in gut microbiome of young and middle-aged adults with prediabetes apparent in metabolizing bioactive (poly)phenols. Nutrients 12:3595. doi: 10.3390/nu12113595
Keywords: bacterial diversity, prediabetes, ethnicity, Firmicutes , Bacteroidetes
Citation: Mathrani A, Lu LW, Sequeira-Bisson IR, Silvestre MP, Hoggard M, Barnett D, Fogelholm M, Raben A, Poppitt SD and Taylor MW (2023) Gut microbiota profiles in two New Zealand cohorts with overweight and prediabetes: a Tū Ora/PREVIEW comparative study. Front. Microbiol. 14:1244179. doi: 10.3389/fmicb.2023.1244179
Edited by:
George Grant, University of Aberdeen, United KingdomReviewed by:
Eugenia Bezirtzoglou, Democritus University of Thrace, GreeceScott Hazelhurst, University of the Witwatersrand, South Africa
Sadequr Rahman, Monash University Malaysia, Malaysia
Copyright © 2023 Mathrani, Lu, Sequeira-Bisson, Silvestre, Hoggard, Barnett, Fogelholm, Raben, Poppitt and Taylor. This is an open-access article distributed under the terms of the Creative Commons Attribution License (CC BY). The use, distribution or reproduction in other forums is permitted, provided the original author(s) and the copyright owner(s) are credited and that the original publication in this journal is cited, in accordance with accepted academic practice. No use, distribution or reproduction is permitted which does not comply with these terms.
*Correspondence: Sally D. Poppitt, s.poppitt@auckland.ac.nz; Michael W. Taylor, mw.taylor@auckland.ac.nz