- 1Department of Orthopaedics, The Second Affiliated Hospital of Nanjing University of Chinese Medicine, Nanjing, China
- 2Department of Oncology, Jiangsu Province Hospital of Chinese Medicine, Affiliated Hospital of Nanjing University of Chinese Medicine, Nanjing, China
- 3Department of General Surgery, Jiangsu Province Hospital of Chinese Medicine, Affiliated Hospital of Nanjing University of Chinese Medicine, Nanjing, China
- 4Department of Ophthalmology, Children’s Hospital of Nanjing Medical University, Nanjing, China
Background: Recent observational studies and clinical trials demonstrated an association between gut microbiota and musculoskeletal (MSK) diseases. Nonetheless, whether the gut microbiota composition has a causal effect on the risk of MSK diseases remains unclear.
Methods: Based on large-scale genome-wide association studies (GWAS), we performed a two-sample Mendelian randomization (MR) analysis to investigate the causal relationship between gut microbiota and six MSK diseases, namely osteoporosis (OP), fracture, sarcopenia, low back pain (LBP), rheumatoid arthritis (RA), and ankylosing spondylitis (AS). Instrumental variables for 211 gut microbiota taxa were obtained from the largest available GWAS meta-analysis (n = 18,340) conducted by the MiBioGen consortium. And the summary-level data for six MSK diseases were derived from published GWAS. The inverse-variance weighted (IVW) method was conducted as a primary analysis to estimate the causal effect, and the robustness of the results was tested via sensitivity analyses using multiple methods. The Bonferroni-corrected test was used to determine the strength of the causal relationship between gut microbiota and various MSK diseases. Finally, a reverse MR analysis was applied to evaluate reverse causality.
Results: According to the IVW method, we found 57 suggestive causal relationships and 3 significant causal relationships between gut microbiota and MSK diseases. Among them, Genus Bifidobacterium (β: 0.035, 95% CI: 0.013–0.058, p = 0.0002) was associated with increased left handgrip strength, Genus Oxalobacter (OR: 1.151, 95% CI: 1.065–1.245, p = 0.0003) was correlated with an increased risk of LBP, and Family Oxalobacteraceae (OR: 0.792, 95% CI: 0.698–0.899, p = 0.0003) was linked with a decreased risk of RA. Subsequently, sensitivity analyses revealed no heterogeneity, directional pleiotropy, or outliers for the causal effect of specific gut microbiota on MSK diseases (p > 0.05). Reverse MR analysis showed fracture may result in a higher abundance of Family Bacteroidales (p = 0.030) and sarcopenia may lead to a higher abundance of Genus Sellimonas (p = 0.032).
Conclusion: Genetic evidence suggested a causal relationship between specific bacteria taxa and six MSK diseases, which highlights the association of the “gut-bone/muscle” axis. Further exploration of the potential microbiota-related mechanisms of bone and muscle metabolism might provide novel insights into the prevention and treatment of MSK diseases.
1. Introduction
Musculoskeletal (MSK) diseases encompass a spectrum of pathologies that affect muscles, soft tissues, joints and the spine (Murray et al., 2012). Globally, all MSK diseases combined accounted for 21.3% of the total years lived with disability (YLDs), second only to mental and behavioral disorders (23.2%) (March et al., 2014; Smith et al., 2014). Disability due to MSK diseases has increased by 45% from 1990 to 2010, in particular sarcopenia, osteoporosis (OP) and osteoarthritis (OA), and is expected to continue to rise with an increasingly obese, sedentary, and ageing population (Vos et al., 2012). In addition, MSK diseases also impose a significant economic burden on both patients and the health care system (Sebbag et al., 2019). Recent epidemiological studies have revealed that MSK diseases-related lost productivity accounts for 2% of the gross domestic product (GDP) in the European Union (Bevan, 2015). According to data from the 2012 National Health Interview Survey (NHIS), the annual cost of direct treatment and lost wages for MSK diseases in the United States was estimated at $213 billion (Rosenfeld et al., 2018). Therefore, the prevention and management of MSK diseases has been globally recognized as a crucial public health issue that needs to be solved urgently.
The gut microbiota is a large and complex community of microbial species inhabiting the human gastrointestinal tract, which plays a critical role in human health and diseases (Li et al., 2022). Human gut microbiota can influence host physiology by regulating multiple processes, including inflammation, oxidative stress, immune function, and anabolic balance (Ticinesi et al., 2019). Growing evidence suggests that many MSK diseases, such as OP, OA, sarcopenia, and low back pain (LBP) are closely correlated with altered gut microbiota (Biver et al., 2019). According to a recent study by Xu et al., the gut microbiota compositions of patients with osteoporosis were significantly different from those of healthy controls, especially the enriched Dialister and Faecalibacterium genera (Xu et al., 2020). Another observational study indicated that the abundance of Lactobacilli, Bacteroides, and Prevotella is higher and the abundance of Enterobacteriaceae is lower in normal individuals than in those with sarcopenia (Castro-Mejia et al., 2020). In addition, the gut microbiota can also be involved in the development of rheumatoid arthritis (RA) and ankylosing spondylitis (AS) by altering intestinal barrier permeability, regulating expression levels of host hormones, and mediating immune responses (Asquith et al., 2019). Although the correlations between gut microbiota and Musculoskeletal (MSK) diseases have been reported by previous researches, these findings are mainly based on observational and cross-sectional analyses, and it is still unclear whether there is a causal link between the gut microbiome and MSK diseases.
Mendelian randomization (MR) is a groundbreaking analytical method that provides an unbiased estimation of the causal link between phenotypes (Smith and Ebrahim, 2003). Compared with traditional observational studies, the MR study uses genetic variation as instrumental variables (IVs) to avoid the influence of traditional confounding factors and reverse causality, which provides robust evidence on the mechanisms of disease pathogenesis and the efficacy of treatments (Davey and Hemani, 2014). Given the aforementioned findings and the MR method, our objective was to conduct a bidirectional two-sample MR analysis using extensive genome-wide association study (GWAS) data to explore the causality between specific gut microbiota and six MSK diseases, including OP, fracture, sarcopenia, LBP, RA, and AS.
2. Materials and methods
2.1. Study design
GWAS summary data focusing on the relationships between genetics and disease have been used to detect a causal relationship between the gut microbiome and six MSK diseases (OP, fracture, sarcopenia, LBP, RA, and AS) through bidirectional two-sample MR analysis (Visscher et al., 2012; Jia et al., 2019). Our first step was to determine whether the gut microbiome contributes to the prevention or promotion of six MSK diseases, by selecting the gut microbiome as the exposure and the six MSK diseases as the outcome. Furthermore, we examined changes in gut microbiota following the development of six MSK diseases. According to Bownden et al., the two-sample MR needs to satisfy the following three assumptions (Bowden et al., 2015): (Murray et al., 2012) The instruments of genetic variations should be robustly associated with gut microbiota; (b) The genetic variations should not be associated with any confounders of gut microbiota and six MSK diseases; (c) The genetic variations should affect six MSK diseases solely through gut microbiota, not via other pathways (Sanderson et al., 2019) (Figure 1A). All studies included in the cited GWASs were approved by relevant review committees and all participants provided informed consent. The flow chart of the MR analysis was presented in Figure 1B.
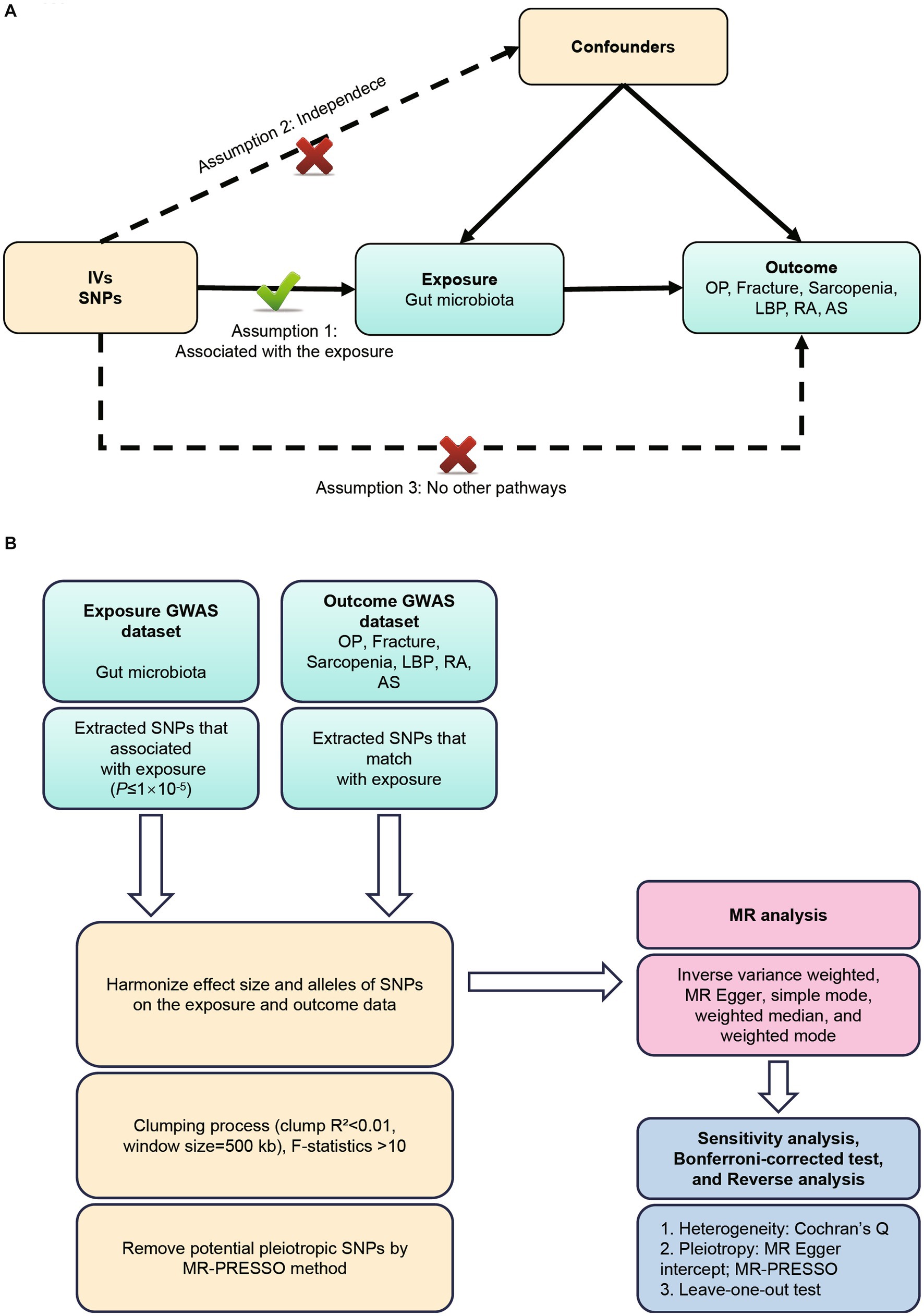
Figure 1. (A) Three assumptions of Mendelian randomization. (B) Flowchart of this Mendelian randomization study. MR, Mendelian randomization; MSK, musculoskeletal; OP, osteoporosis; LBP, low back pain; RA, rheumatoid arthritis; AS, ankylosing spondylitis.
2.2. Genome-wide association studies sources
Our gut microbiota summary data came from a large multi-ethnic GWAS meta-analysis that included 18,340 European individuals and individuals from 24 cohorts (MiBioGen Consortium). Three different variable regions of the 16S rRNA gene were targeted in order to profile the microbial composition. And only the taxa found in >10% of the samples were included in the quantitative microbiome trait loci (mbQTL) mapping study for each cohort. Furthermore, after adjustment for age, sex, technical covariates, and genetic principal components, Spearman’s correlation analysis was conducted to identify genetic loci that affected the covariate-adjusted abundance of bacterial taxa (Kurilshikov et al., 2021).
In the study, we extracted GWAS summary statistics for six MSK diseases from publicly GWAS analyses. The GWAS summary data on OP were mainly extracted from UK Biobank, including 7,547 cases and 455,386 controls of European ancestry (Sudlow et al., 2015). Summary statistics for fracture were derived from a GWAS meta-analysis, including 44,502 cases and 415,887 controls of European ancestry (Morris et al., 2019). Summary data for ALM and handgrip strength were obtained from the UK Biobank study. An analysis of 450,243 UK Biobank cohort participants was conducted to quantify ALM-related values by summing fat-free mass (Pei et al., 2020). For handgrip strength, UK Biobank provided GWAS summary statistics on right and left handgrip strength based on 461,089 and 461,026 United Kingdom people, respectively (Sudlow et al., 2015). The data on LBP came from a meta-analysis including 13,178 cases and 164,682 controls of European ancestry (https://gwas.mrcieu.ac.uk/datasets/ukb-d-M13_LOWBACKPAIN). For RA, we collected summary statistics from a GWAS meta-analysis that includes 14,361 RA cases and 43,923 controls of European ancestry (Okada et al., 2014). The genetic data of AS risk were derived from a large GWAS involving 9,069 cases and 1,550 controls of European (Cortes et al., 2013). Detailed information on the demographic characteristics of selected summary-level GWASs applied in this study was shown in Supplementary Table S1.
2.3. Selection of genetic instrumental variables
During the selection of the optimal IVs, the following quality control steps were taken to ensure the validity and accuracy of the conclusions on the causal relationship between the gut microbiome and the six MSK diseases (Murray et al., 2012) The instrumental variables selected for analysis need to be highly related to the corresponding exposures (We choose significant SNPs based on a loose cutoff of p < 1 × 10−5 to ensure sufficient instrumental variables for screening). (March et al., 2014) The instrumental variables are mutually independent and avoid the offset caused by linkage disequilibrium (LD) between the SNPs (r2 < 0.01, LD distance = 500 kb). (Smith et al., 2014) We eliminated instrumental variables with an F-statistic less than 10 to minimize potential weak instrument bias F = R2(n-k-1)/k(1-R2) (n is the sample size, k is the number of included instrumental variables, and R2 is the exposure variance explained by the selected SNPs).
2.4. Statistical analysis
The inverse variance weighted (IVW) method was employed as the main analysis, to obtain an unbiased estimate of the causal relationship between gut microbiota and MSK diseases. Furthermore, the weighted median, MR Egger, simple mode, and weighted mode methods were applied as additional methods to estimate causal effects under different conditions. The weighted median method could combine data on multiple genetic variants into a single causal estimate and provide a consistent estimate if at least half of the weight is derived from valid IVs (Bowden et al., 2016). The MR-Egger method could evaluate whether genetic variants have directional pleiotropy and provide a consistent estimate of the causal effect (Bowden et al., 2015). The intercept of MR-Egger regression was calculated to assess horizontal pleiotropy, and p value >0.05 indicated that the possibility of pleiotropy effect in the causal analysis is weak (Verbanck et al., 2018). Cochran’s Q test was derived from IVW estimation and used to detect heterogeneity among instrumental variables (Pierce and Burgess, 2013). In addition, we applied the Mendelian randomization pleiotropy residual sum and outlier (MR-PRESSO) method to determine horizontal pleiotropy and correct potential outliers. The leave-one-out method was used for sensitivity analysis, which sequentially removed one of the SNPs and used the remaining SNPs as instrumental variables for two-sample MR analysis to judge the degree of influence of causal association effect by a single SNP. In addition, we also performed a reverse-direction MR analysis to determine whether there was a reverse-direction causal relationship.
To obtain a more accurate assessment of the causal relationship, we applied Bonferroni correction to determine the significance of multiple-testing at each feature level (p < 0.05/n, where n is the number of bacterial taxa included in each feature level). Hence, the multiple-testing significance was 5.56 × 10−3, 3.13 × 10−3, 2.50 × 10−3, 1.56 × 10−3, and 4.20 × 10−4, respectively, for phylum, class, order, family, and genus. The ‘TwoSampleMR’ package and the ‘MRPRESSO’ package in R software (version 4.1.3) were used for all MR analyses.
3. Results
3.1. Selection of instrumental variables
To begin with, 14,587 SNPs correlated with the gut microbiota were identified as IVs from the MiBioGen Consortium at a relatively loose significance level (p < 1 × 10−5). It contained 211 bacterial traits, including 131 genera, 35 families, 20 orders, 16 classes, and 9 phyla. After a series of quality control steps, 2,236 SNPs were finally included in the analysis. In addition, the F-statistics of all IVs were >10, indicating no evidence of weak instrument bias.
3.2. Causal effects of gut microbiota on musculoskeletal diseases
3.2.1. Causal effect of gut microbiota on osteoporosis risk
According to the results of the IVW method, the higher genetically predicted abundance of Order NB1n (OR: 0.998, 95% CI: 0.997–0.999, p = 0.038), Genus Lachnospiraceae NK4A136 group (OR: 0.996, 95% CI: 0.994–0.999, p = 0.003), Genus Christensenellaceae R.7 group (OR: 0.996, 95% CI: 0.991–1.000, p = 0.028) was associated with a reduced risk of OP (Table 1). In contrast, genetically predicted abundance of Genus Howardella (OR: 1.002, 95% CI: 1.000–1.003, p = 0.033), and Genus Eubacterium oxidoreducens group (OR: 1.003, 95% CI: 1.000–1.005, p = 0.046) was positively related to OP risk (Figure 2).
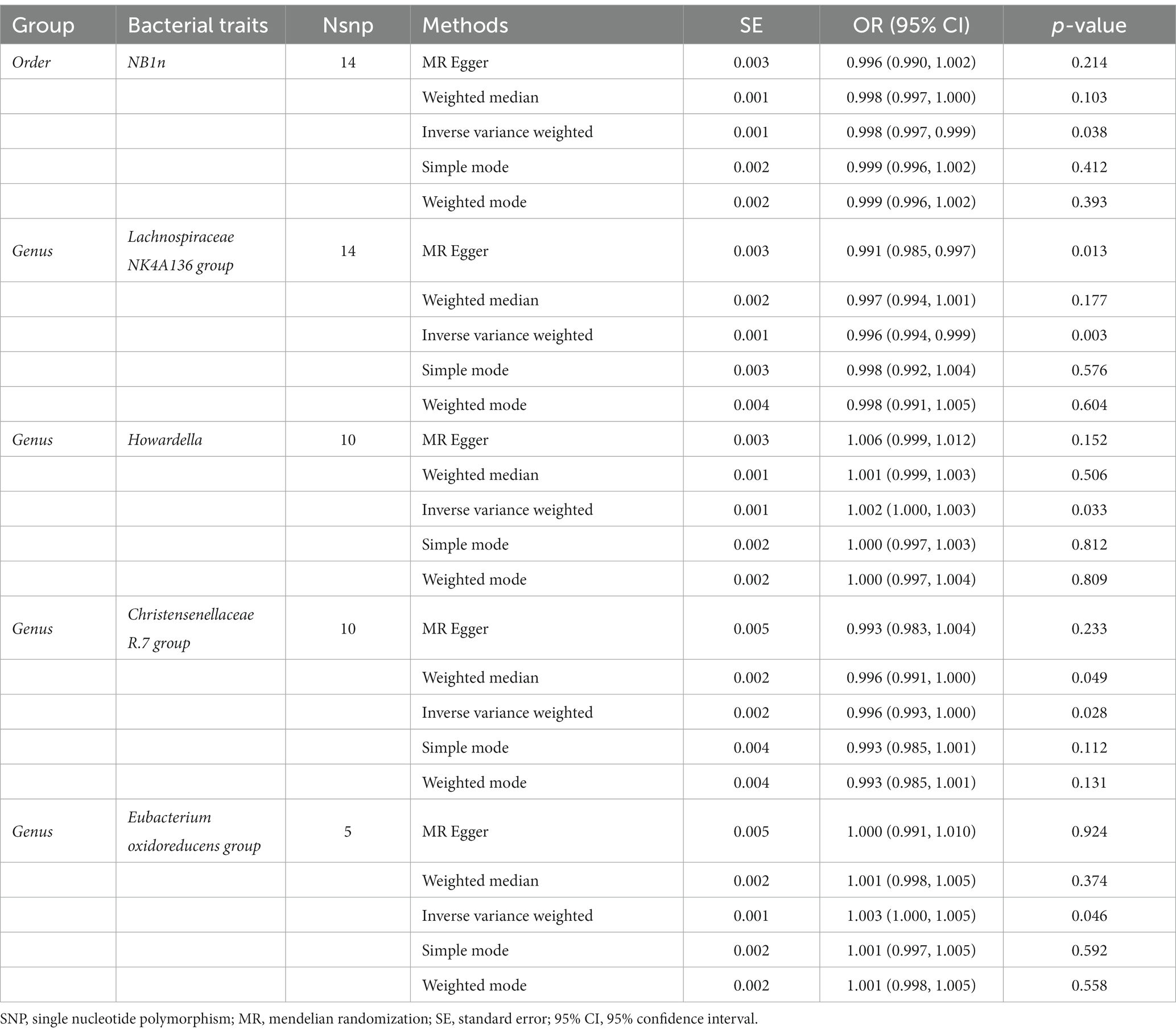
Table 1. Mendelian randomisation (MR) results of causal effects between gut microbiome and the risk of osteoporosis.
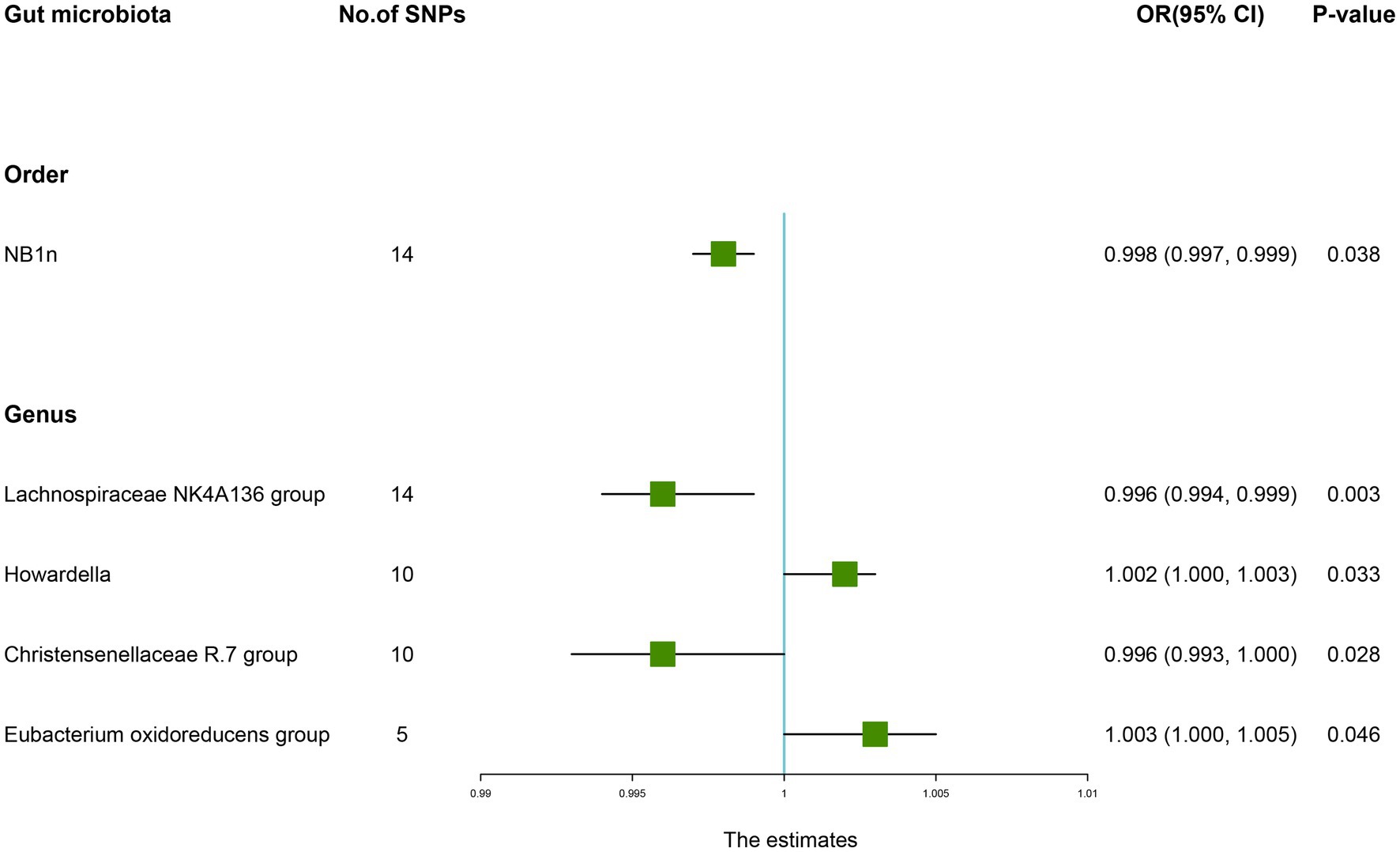
Figure 2. Forest plot of the causality between gut microbiota with the risk of osteoporosis. The estimates: Inverse variance weighted (IVW) results of gut microbiota and osteoporosis risk; p-value: p-value of the estimate. OR, odds ratio; SNP, single-nucleotide polymorphism.
3.2.2. Causal effect of gut microbiota on fracture risk
The estimates of the IVW test showed that the genetically predicted four genera, namely, Family Defluviitaleaceae (OR: 1.005, 95% CI: 1.001–1.010, p = 0.022), Family Bacteroidales S24.7 group (OR: 1.007, 95% CI: 1.000–1.013, p = 0.049), Genus Allisonella (OR: 1.005, 95% CI: 1.001–1.009, p = 0.015), Genus Defluviitaleaceae UCG011 (OR: 1.006, 95% CI: 1.001–1.011, p = 0.019) were positively associated with the risk of fracture (Table 2). And higher genetically predicted Class Mollicutes (OR: 0.994, 95% CI: 0.988–0.999, p = 0.022), Genus Collinsella (OR: 0.993, 95% CI: 0.986–1.000, p = 0.047), and Phylum Tenericutes (OR: 0.994, 95% CI: 0.988–0.999, p = 0.022) were linked with a decreased fracture risk (Figure 3).
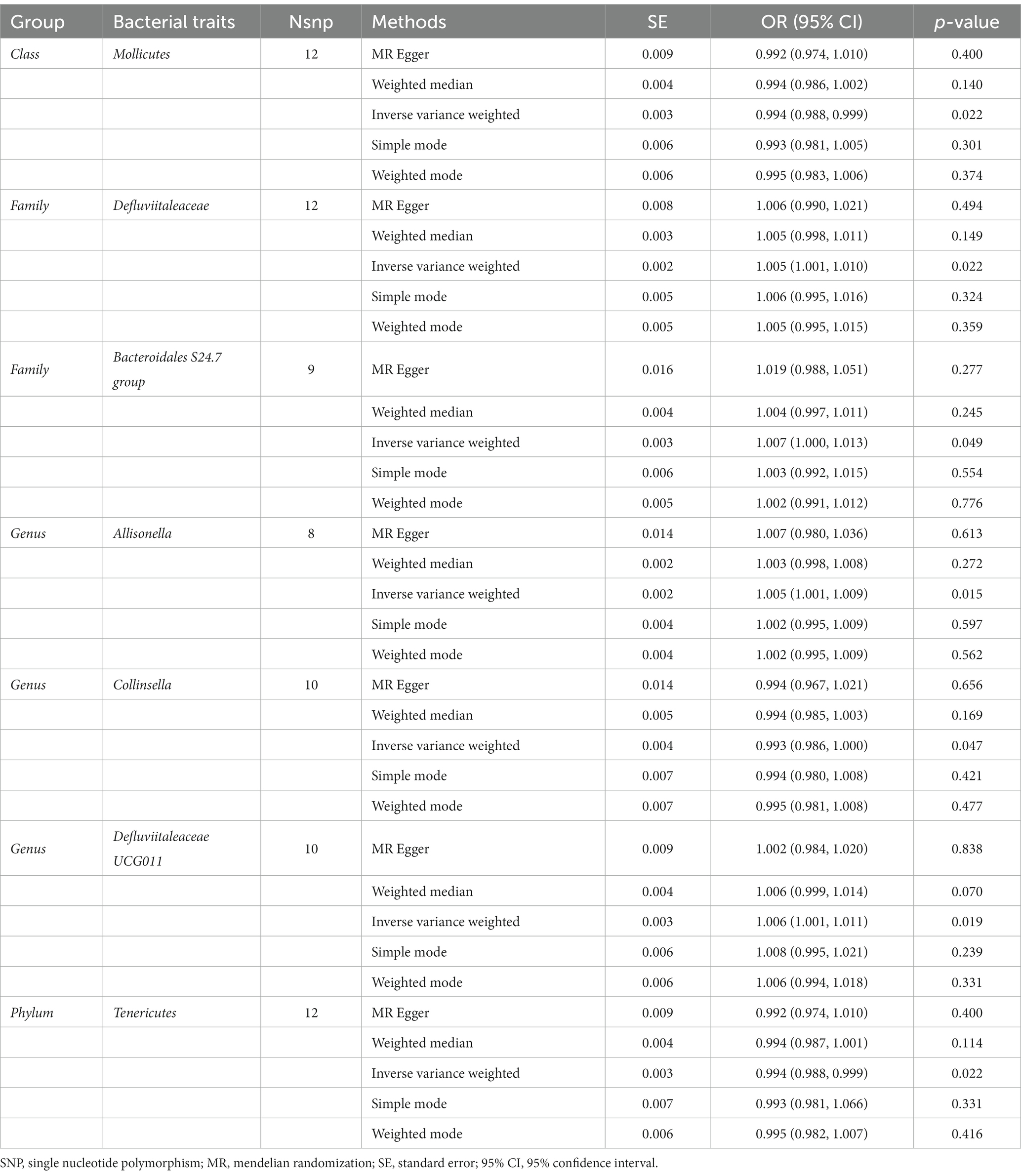
Table 2. Mendelian randomisation (MR) results of causal effects between gut microbiome and the risk of fracture.
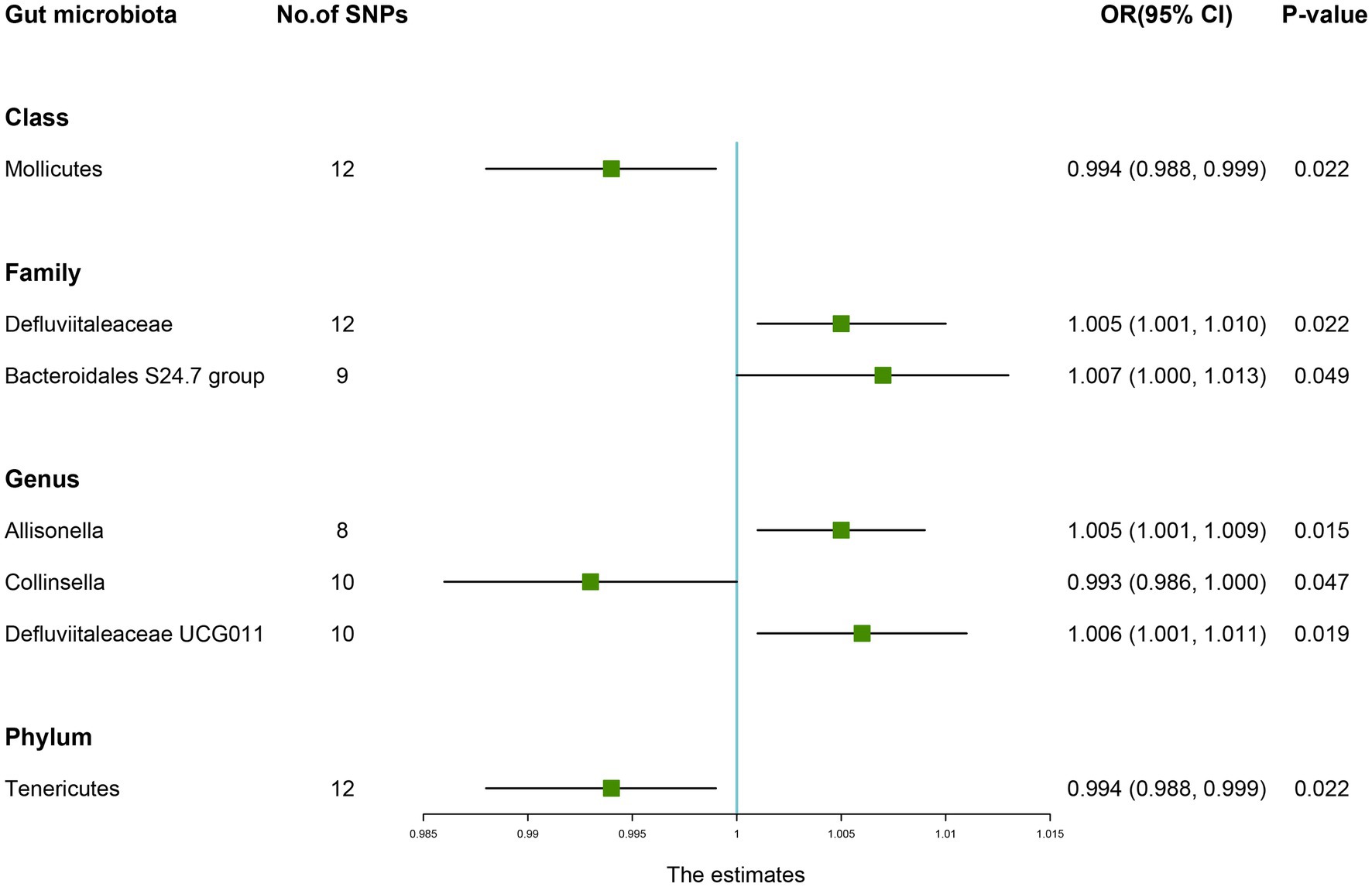
Figure 3. Forest plot of the causality between gut microbiota with the risk of fracture. The estimates: Inverse variance weighted (IVW) results of gut microbiota and fracture risk; p-value: p-value of the estimate. OR, odds ratio; SNP, single-nucleotide polymorphism.
3.2.3. Causal effect of gut microbiota on sarcopenia-related traits
The IVW results suggested that genetically predicted Class Actinobacteria (β: 0.026, 95% CI: 0.003–0.050, p = 0.029), Family Bifidobacteriaceae (β: 0.028, 95% CI: 0.004–0.052, p = 0.024), Genus Alloprevotella (β: 0.012, 95% CI: 0.002–0.022, p = 0.021), Genus Bifidobacterium (β: 0.028, 95% CI: 0.006–0.050, p = 0.012), Genus Eisenbergiella (β: 0.012, 95% CI: 0.000–0.025, p = 0.049), Genus Parabacteroides (β: 0.030, 95% CI: 0.008–0.051, p = 0.006), Genus Sellimonas (β: 0.013, 95% CI: 0.004–0.022, p = 0.003), Order Bifidobacteriales (β: 0.028, 95% CI: 0.004–0.052, p = 0.024), Phylum Actinobacteria (β: 0.030, 95% CI: 0.003–0.056, p = 0.027) were linked to an increase in right handgrip strength, while Genus Paraprevotella (β: -0.014, 95% CI: −0.023–-0.004, p = 0.007) and Genus Prevotella9 (β: -0.014, 95% CI: −0.027–0.000, p = 0.042) were associated with an decrease in right handgrip strength (Table 3). Similarly, we found that genetically predicted Family Bifidobacteriaceae (β: 0.035, 95% CI: 0.011–0.060, p = 0.005), Genus Eubacterium nodatum group (β: 0.010, 95% CI: 0.002–0.017, p = 0.013), Genus Bifidobacterium (β: 0.035, 95% CI: 0.013–0.058, P <0.001), Genus Parabacteroides (β: 0.029, 95% CI: 0.007–0.051, p = 0.011), Genus Sellimonas (β: 0.013, 95% CI: 0.005–0.021, p = 0.002), Order Bifidobacteriales (β: 0.035, 95% CI: 0.011–0.060, p = 0.005) were also positively associated with left grip (Figures 4A,B). And the MR estimates of MR Egger and weighted median indicated that genetically predicted Family Bifidobacteriaceae, Order Bifidobacteriales were positively related to right and left handgrip strength (P <0.05), suggesting Family Bifidobacteriaceae, Order Bifidobacteriales were protective factors for sarcopenia (Table 4).
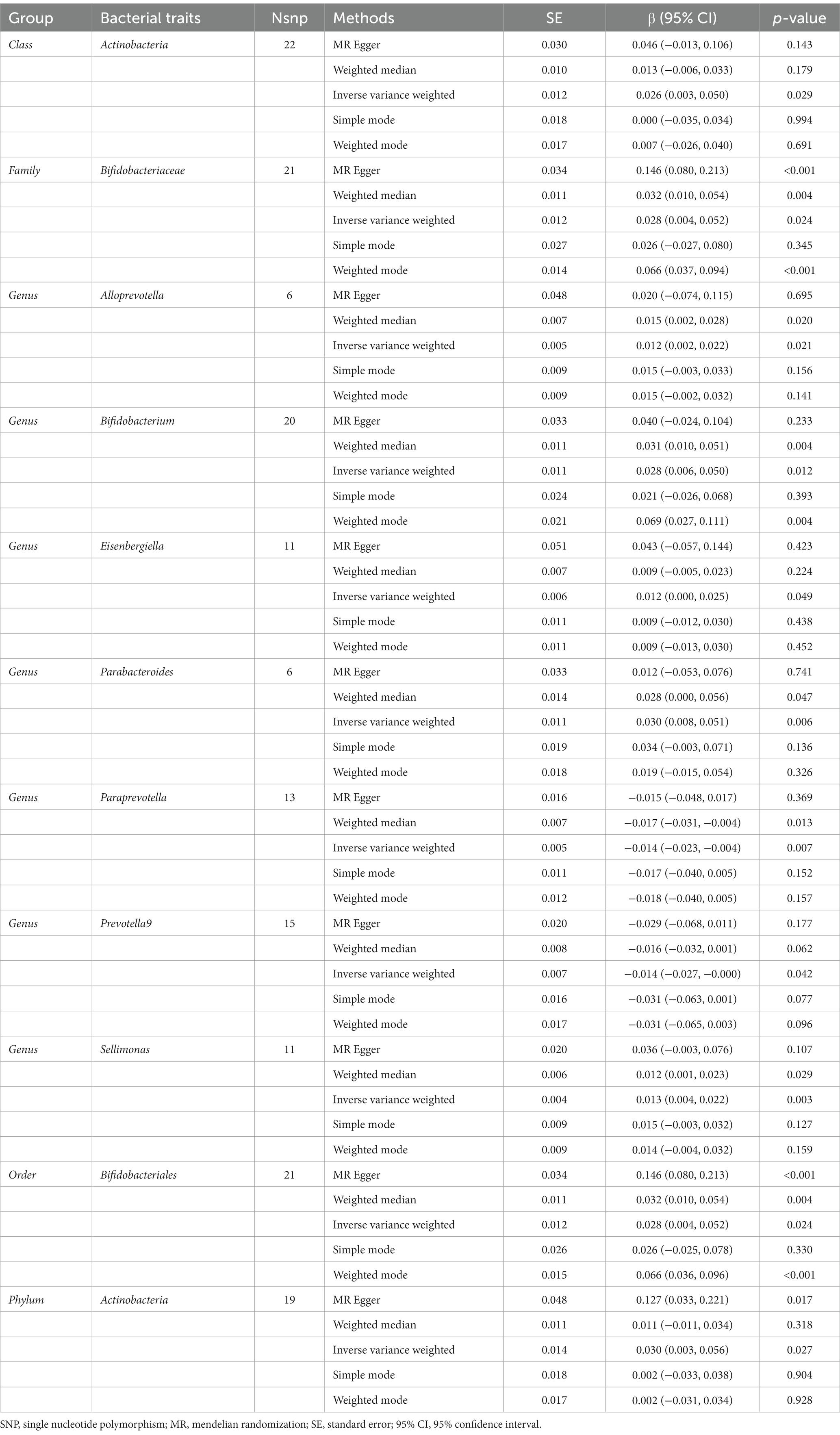
Table 3. Mendelian randomisation (MR) results of causal effects between gut microbiome and hand grip strength (Right).
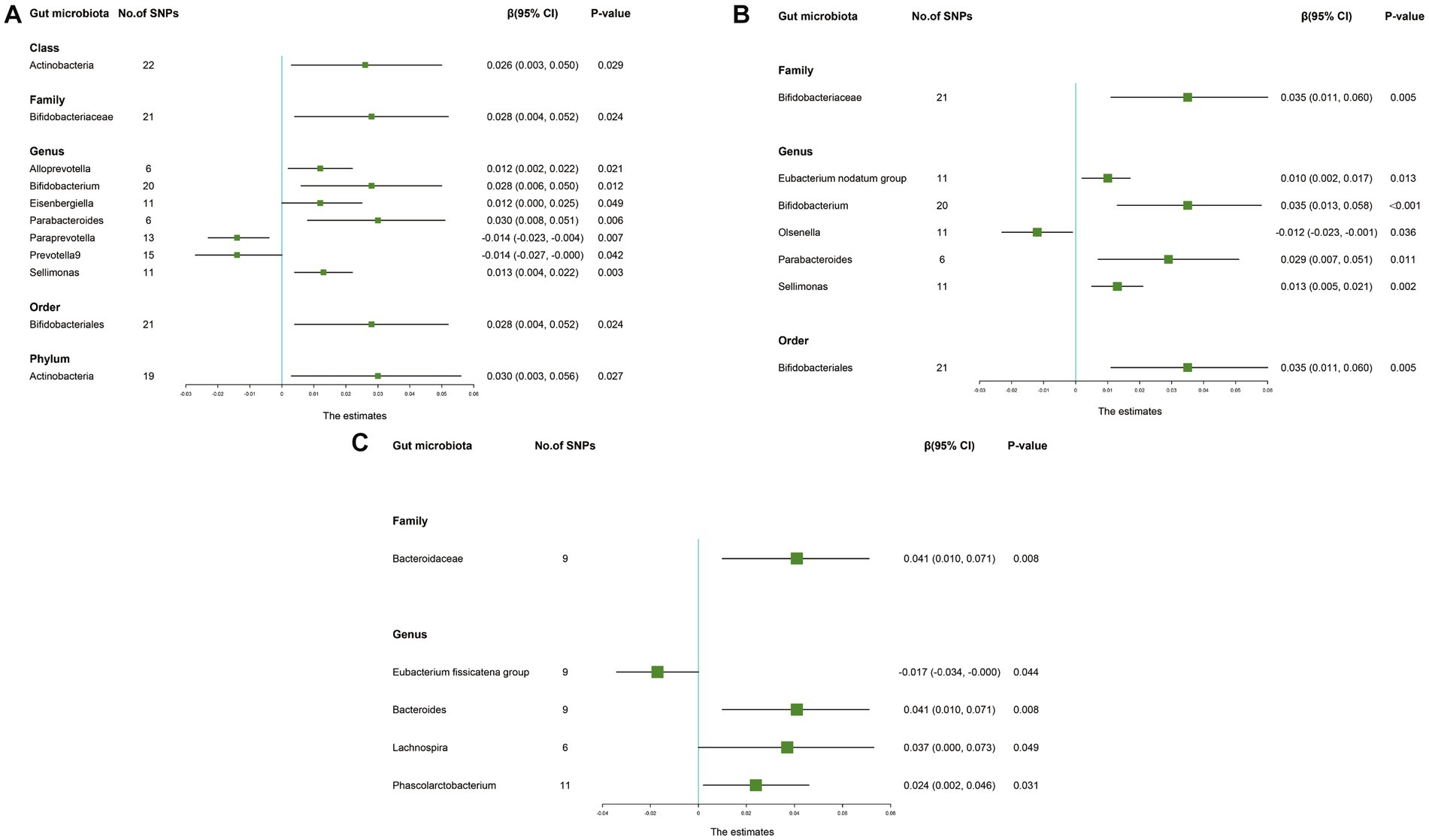
Figure 4. Forest plot of the causality between gut microbiota with sarcopenia-related traits. The estimates: Inverse variance weighted (IVW) results of gut microbiota and sarcopenia-related traits; p-value: p-value of the estimate. (A) Hand grip strength (Right); (B) Hand grip strength (Left); (C) Appendicular lean mass. SNP, single-nucleotide polymorphism.
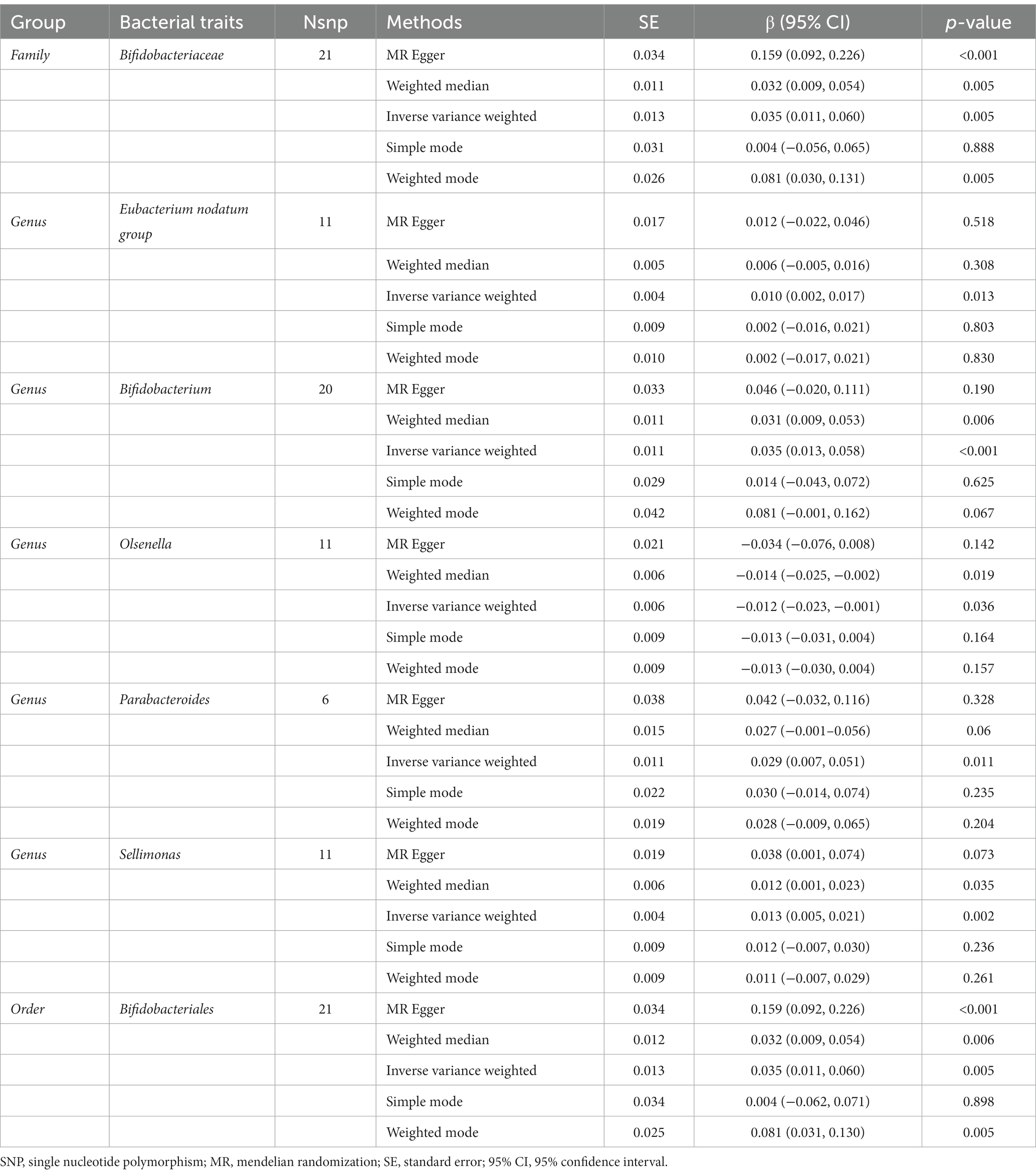
Table 4. Mendelian randomisation (MR) results of causal effects between gut microbiome and hand grip strength (Left).
In addition, the estimates of IVW indicated that genetically predicted Family Bacteroidaceae (β: 0.041, 95% CI: 0.010–0.071, p = 0.008), Genus Bacteroides (β: 0.041, 95% CI: 0.010–0.071, p = 0.008), Genus Lachnospira (β: 0.037, 95% CI: 0.000–0.073, p = 0.049), Genus Phascolarctobacterium (β: 0.024, 95% CI: 0.002–0.046, p = 0.031) were positively correlated with ALM (Table 5). And genetically predicted Genus Eubacterium fissicatena group (β: -0.017, 95% CI: −0.034–-0.000, p = 0.044), which suggested that a 1-SD increase in genetically determined Genus Eubacterium fissicatena group was correlated with an increase in ALM by 0.017 g (Figure 4C).
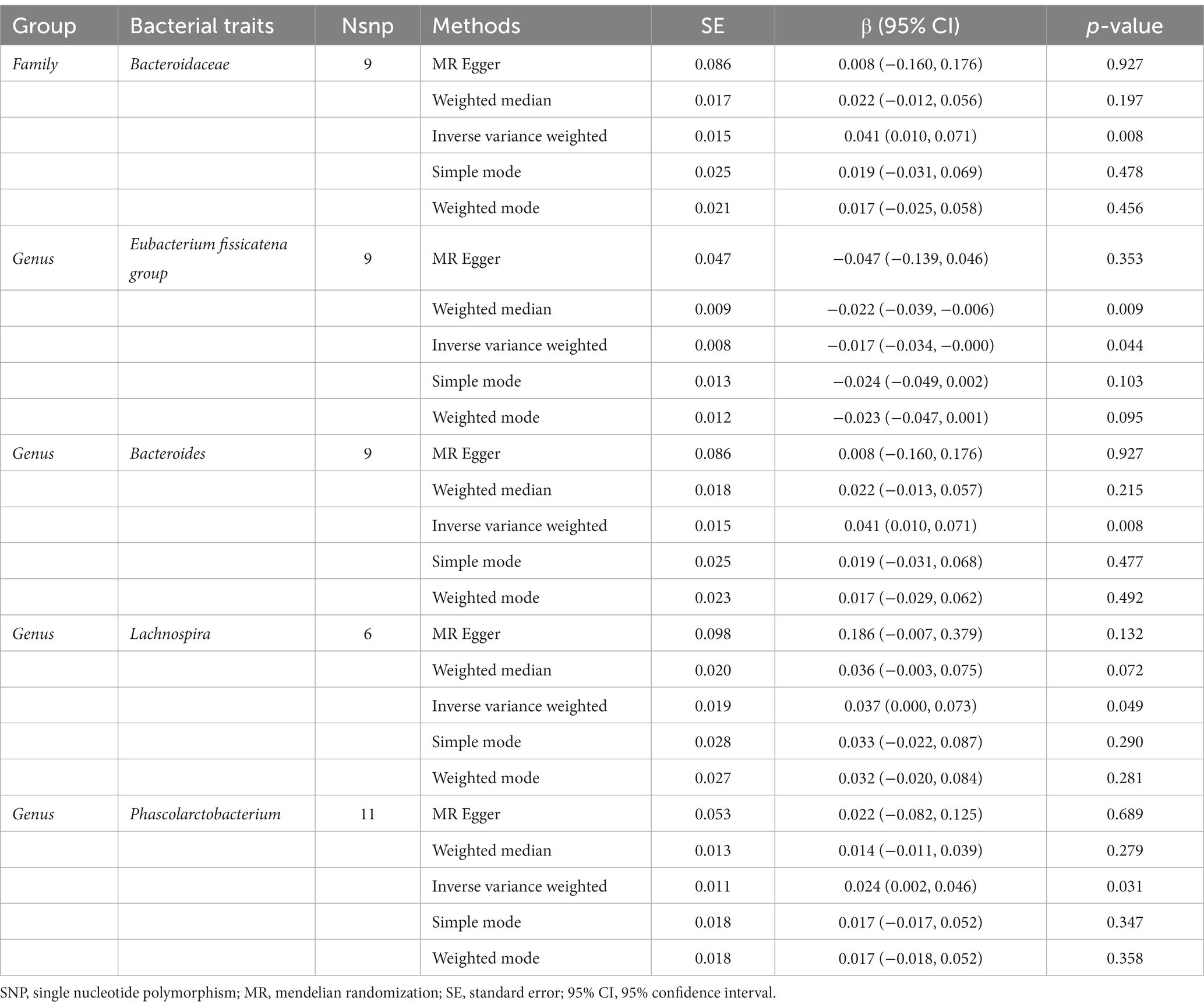
Table 5. Mendelian randomisation (MR) results of causal effects between gut microbiome and appendicular lean mass.
3.2.4. Causal effect of gut microbiota on low back pain risk
As presented in Table 6, there was a causal correlation between four microbiotas and LBP. According to the results of the IVW method, higher genetically predicted Family Prevotellaceae (OR: 1.182, 95% CI: 1.038–1.346, p = 0.012), Genus Oxalobacter (OR: 1.151, 95% CI: 1.065–1.245, P <0.001), Genus Tyzzerella3 (OR: 1.121, 95% CI: 1.032–1.217, p = 0.007) were correlated with a higher risk of LBP. However, Class Melainabacteria (OR: 0.891, 95% CI: 0.808–0.984, p = 0.022) was linked with a lower risk of LBP, which indicated that a 1-SD increase in Class Melainabacteria was correlated with a 10.9% decrease in LBP risk (Figure 5).
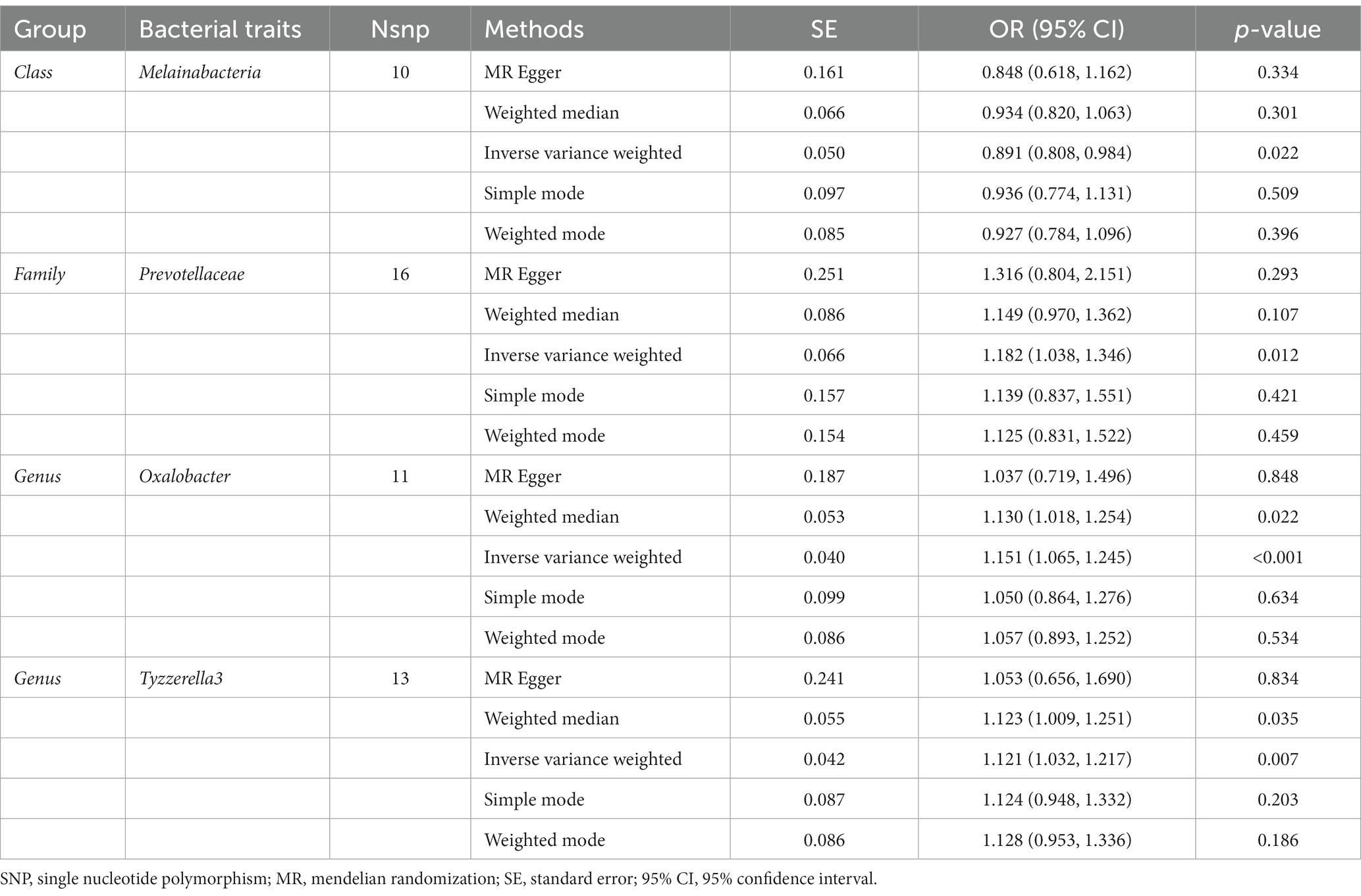
Table 6. Mendelian randomisation (MR) results of causal effects between gut microbiome and the risk of low back pain.
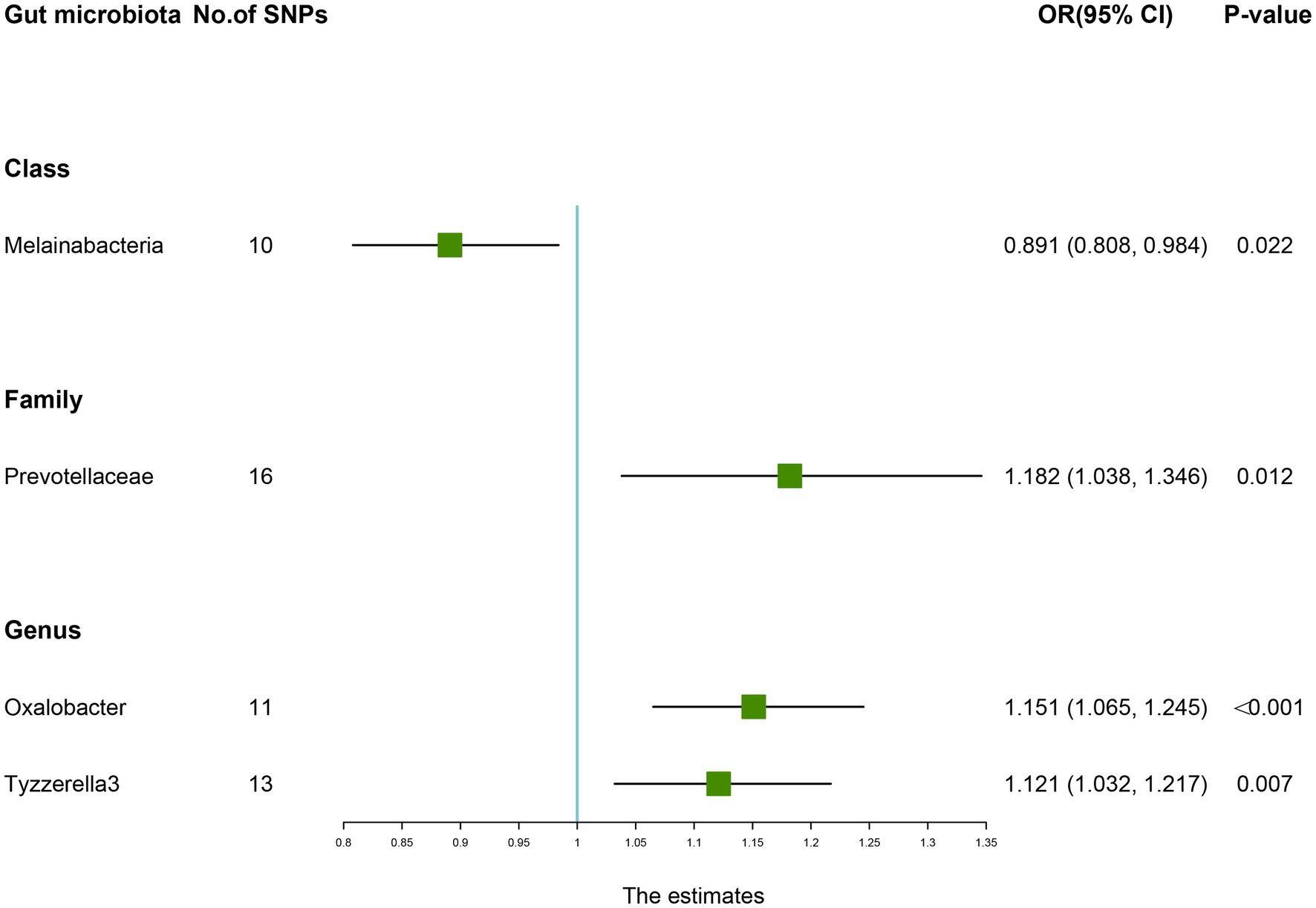
Figure 5. Forest plot of the causality between gut microbiota with the risk of low back pain. The estimates: Inverse variance weighted (IVW) results of gut microbiota and low back pain risk; p-value: p-value of the estimate. OR, odds ratio; SNP, single-nucleotide polymorphism.
3.2.5. Causal effect of gut microbiota on rheumatoid arthritis risk
As shown in Table 7, the IVW results suggested that genetically predicted Class Clostridia (OR: 1.368, 95% CI: 1.033–1.812, p = 0.029), Family Clostridialesvadin BB60 group (OR: 1.200, 95% CI: 1.018–1.416, p = 0.032), Family Desulfovibrionaceae (OR: 1.253, 95% CI: 1.008–1.558, p = 0.042), Family Streptococcaceae (OR: 1.296, 95% CI: 1.038–1.620, p = 0.022), Genus Desulfovibrio (OR: 1.255, 95% CI: 1.020–1.544, p = 0.032), Genus Ruminococcaceae UCG013 (OR: 1.365, 95% CI: 1.057–1.763, p = 0.017), Genus Turicibacter (OR: 1.218, 95% CI: 1.005–1.476, p = 0.044), and Order Clostridiales (OR: 1.349, 95% CI: 1.042–1.748, p = 0.023) were positively associated with the risk of RA. By contrast, there was evidence that increasing abundance of Family Christensenellaceae (OR: 0.750, 95% CI: 0.578–0.973, p = 0.031), Family Oxalobacteraceae (OR: 0.792, 95% CI: 0.698–0.899, P <0.001), Genus Oxalobacter (OR: 0.825, 95% CI: 0.722–0.943, p = 0.005), Genus Ruminococcaceae UCG002 (OR: 0.805, 95% CI: 0.667–0.971, p = 0.023), Order Bacillales (OR: 0.850, 95% CI: 0.743–0.972, p = 0.018), and Phylum Cyanobacteria (OR: 0.783, 95% CI: 0.635–0.965, p = 0.022) have a protective effect on RA risk (Figure 6).
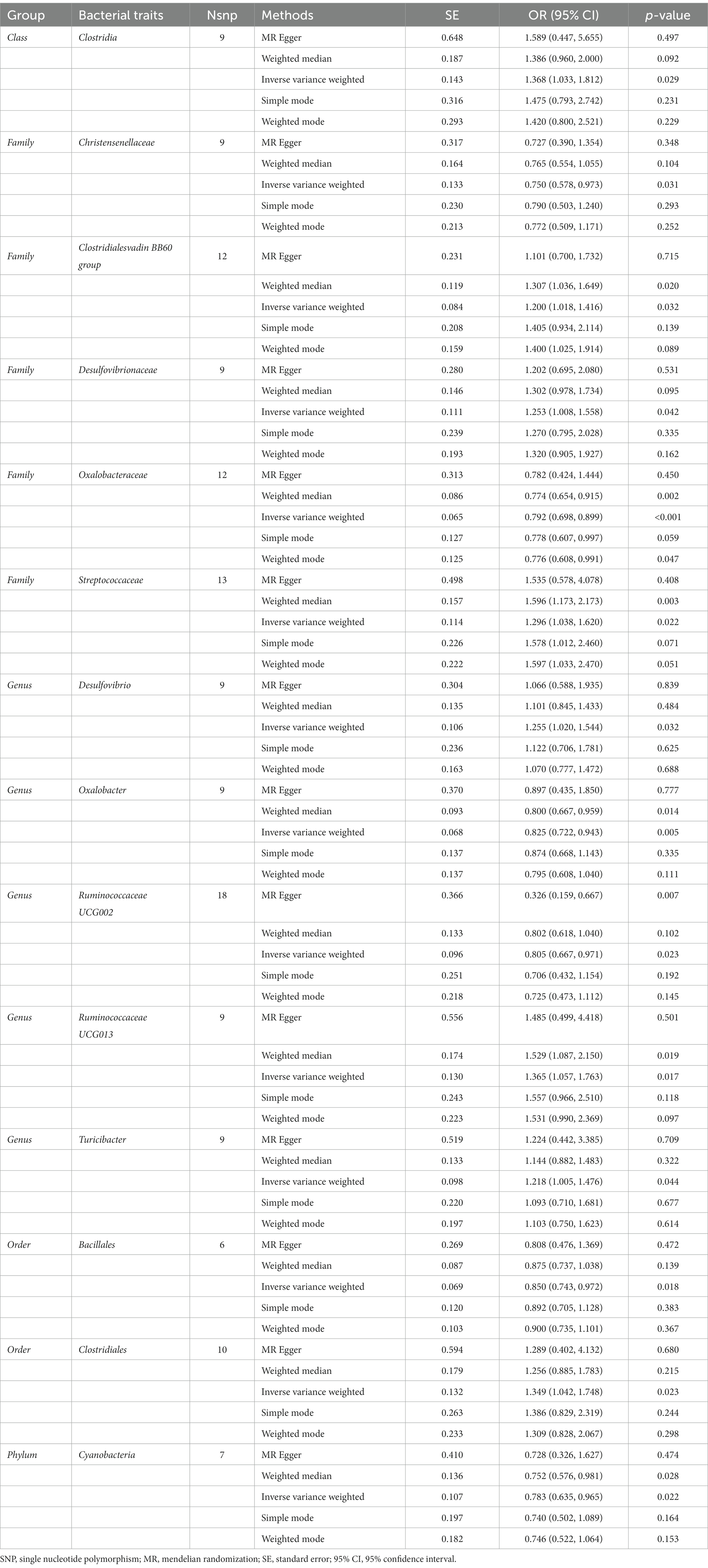
Table 7. Mendelian randomisation (MR) results of causal effects between gut microbiome and the risk of rheumatoid arthritis.
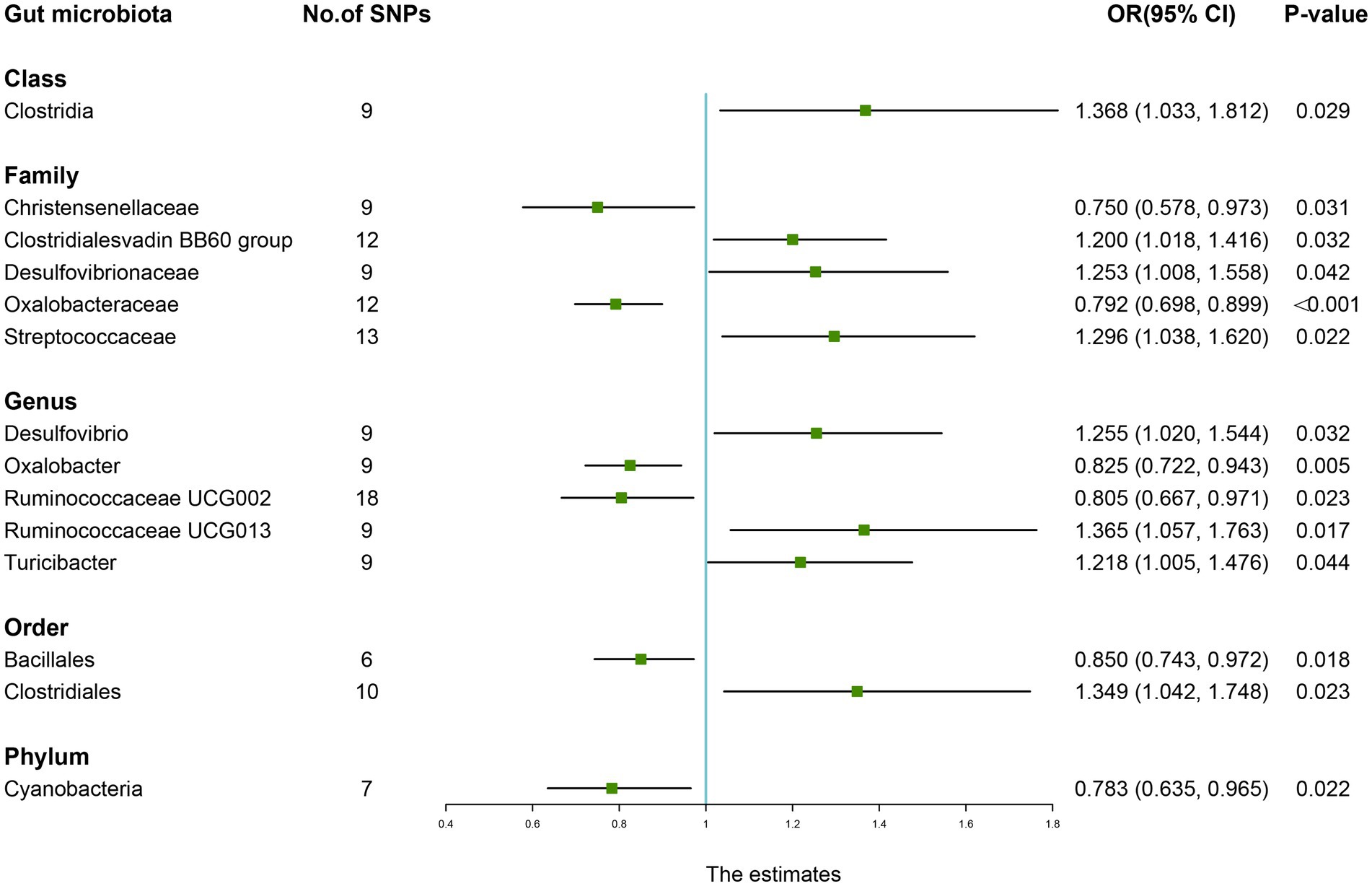
Figure 6. Forest plot of the causality between gut microbiota with the risk of rheumatoid arthritis. The estimates: Inverse variance weighted (IVW) results of gut microbiota and rheumatoid arthritis risk; p-value: p-value of the estimate. OR, odds ratio; SNP, single-nucleotide polymorphism.
3.2.6. Causal effect of gut microbiota on ankylosing spondylitis risk
According to the IVW method, genetically predicted Family Lactobacillaceae (OR: 0.696, 95% CI: 0.527–0.919, p = 0.011), Family Rikenellaceae (OR: 0.680, 95% CI: 0.491–0.942, p = 0.020), Genus Howardella (OR: 0.802, 95% CI: 0.650–0.991, p = 0.041), Genus Anaerotruncus (OR: 0.671, 95% CI: 0.454–0.991, p = 0.045) were negatively associated with the risk of AS (Table 8), while Genus Ruminococcaceae NK4A214 group (OR: 1.707, 95% CI: 1.190–2.449, p = 0.045) was positively linked with the AS risk (Figure 7).
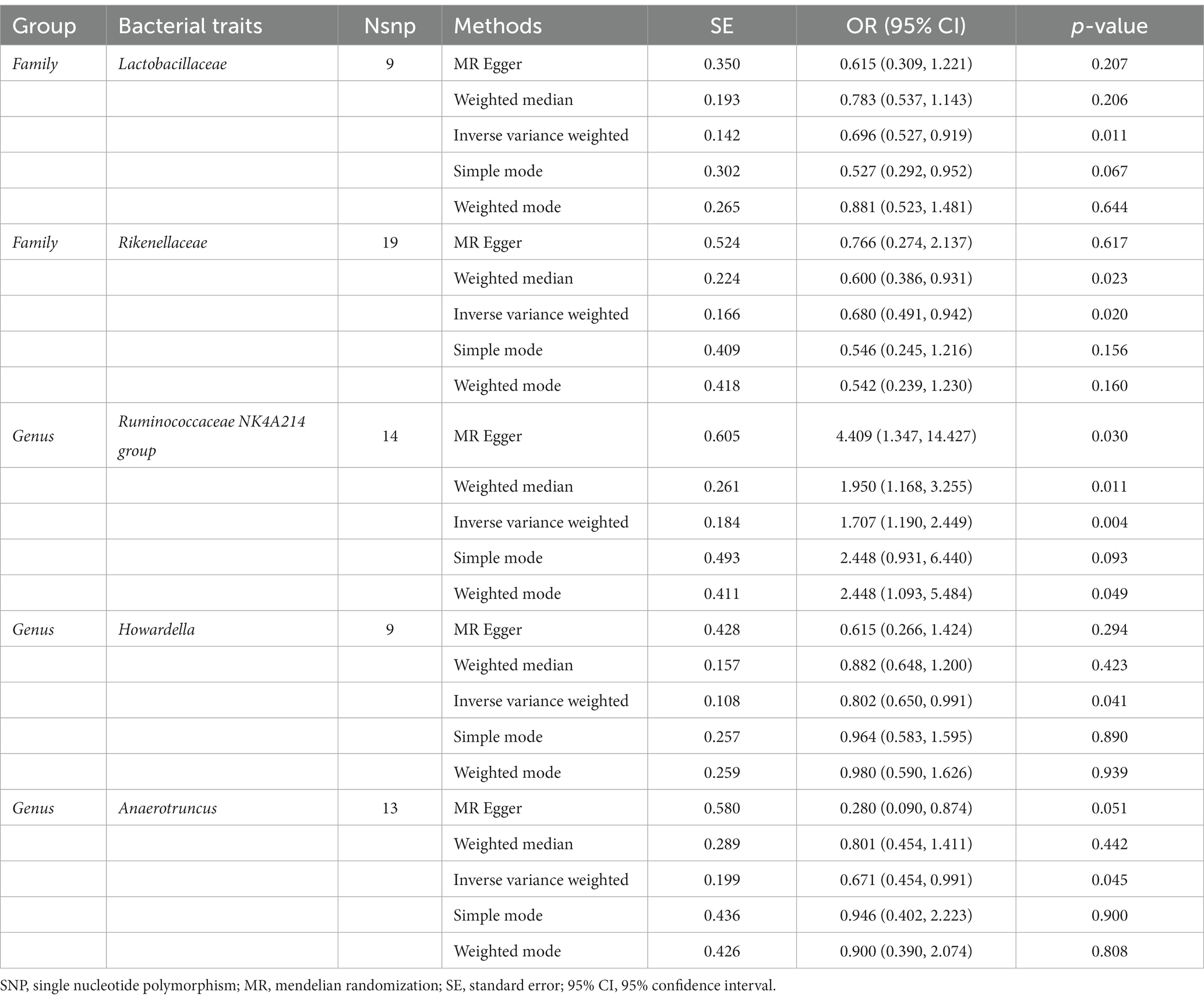
Table 8. Mendelian randomisation (MR) results of causal effects between gut microbiome and the risk of ankylosing spondylitis.
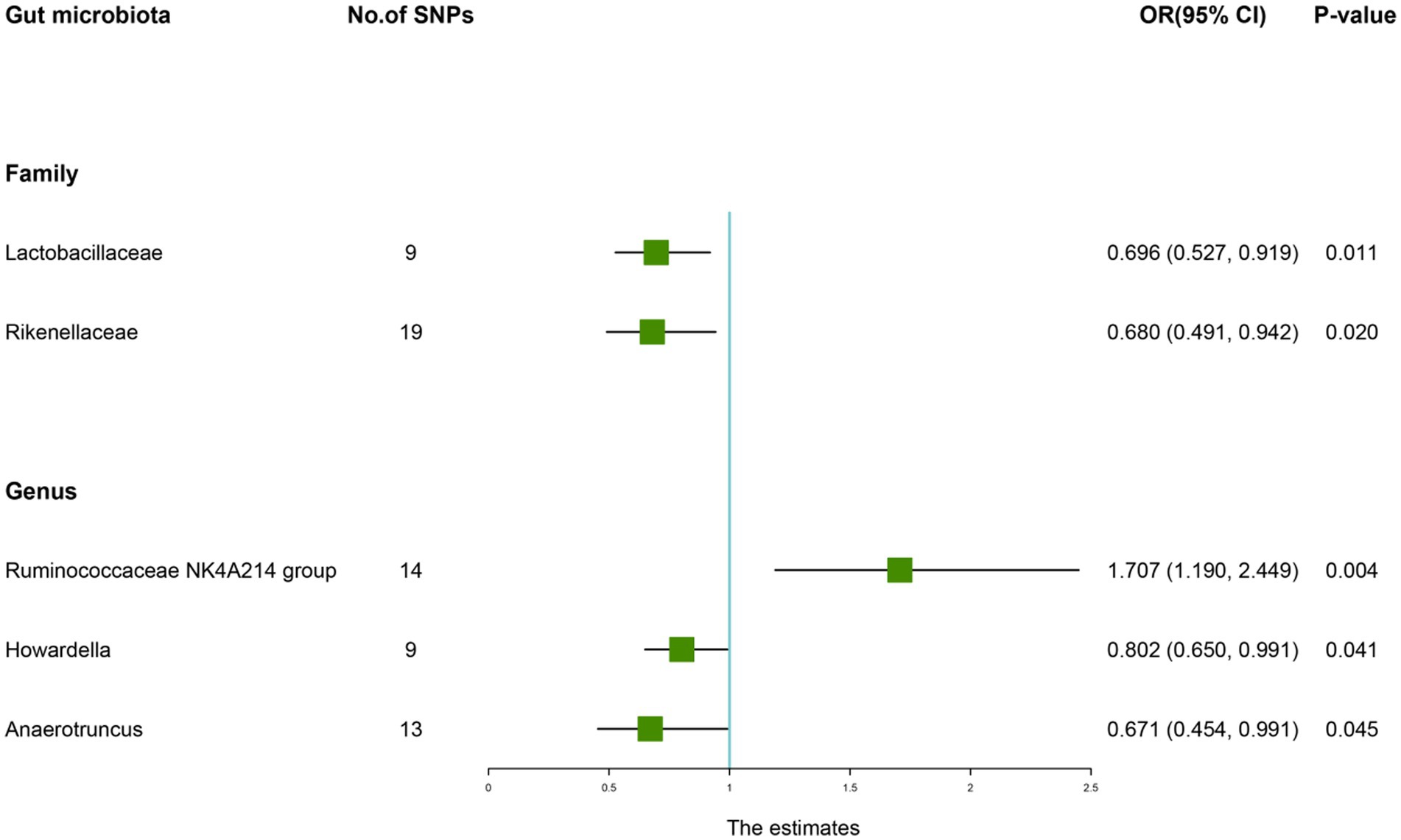
Figure 7. Forest plot of the causality between gut microbiota with the risk of ankylosing spondylitis. The estimates: Inverse variance weighted (IVW) results of gut microbiota and ankylosing spondylitis risk; p-value: p-value of the estimate. OR, odds ratio; SNP, single-nucleotide polymorphism.
3.2.7. Sensitivity analysis, Bonferroni-corrected test, and reverse analysis
We performed a series of sensitivity analyses to test the heterogeneity and horizontal pleiotropy of the selected IVs. Based on Cochran’s Q test, we observed no significant heterogeneity (p > 0.05) (Table 9). All p values of the MR-Egger intercept tests were > 0.05, which indicated no horizontal pleiotropy. Furthermore, we also did not discover any outliers through the MR-PRESSO global test (Table 9). Detailed scatter plots for each MR method analysis were shown in Supplementary Figures S2–S9. And results from a leave-one-out analysis demonstrated that no SNP was an influential outlier (Supplementary Figures S9–S16).
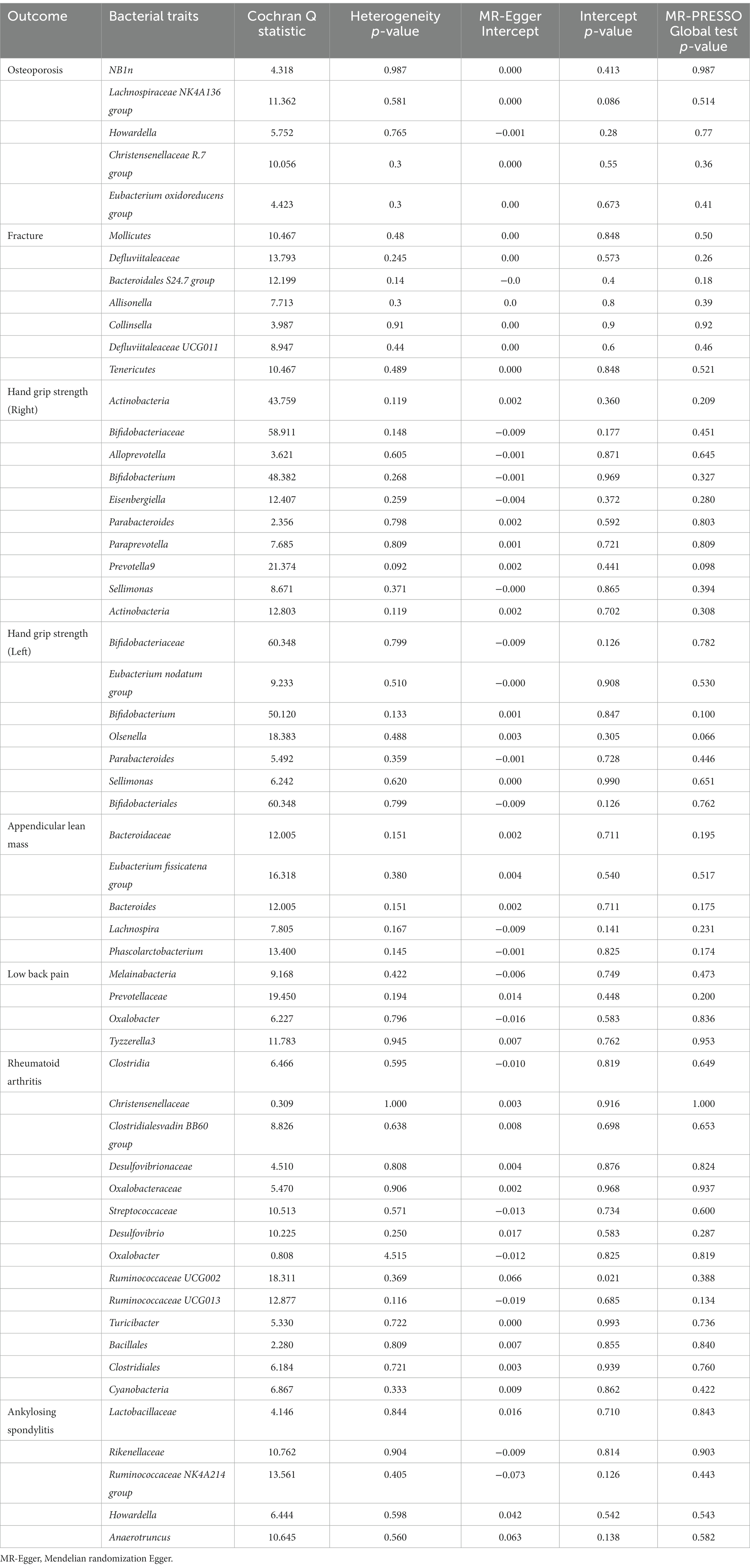
Table 9. Sensitivity analysis of the Mendelian randomization (MR) analysis results of gut microbiota and musculoskeletal diseases.
According to the results of the Bonferroni-corrected test, Genus Bifidobacterium (β: 0.035, 95% CI: 0.013–0.058, p = 0.0002) was significantly correlated with left handgrip strength. In addition, higher level of Genus Oxalobacter retains a strong causal association with an increased risk of LBP (OR: 1.151, 95% CI: 1.065–1.245, p = 0.0003) and higher level of Family Oxalobacteraceae (OR: 0.792, 95% CI: 0.698–0.899, p = 0.0003) retains a strong causal association with a decreased risk of RA. In addition, reverse analysis demonstrates that fracture may result in a higher abundance of Family Bacteroidales (p = 0.030) and sarcopenia may lead to a higher abundance of Genus Sellimonas (p = 0.032) (Supplementary Table S10).
4. Discussion
To our knowledge, this was the first comprehensive and in-depth investigation of causal associations between gut microbiota and six common MSK diseases (OP, fracture, sarcopenia, LBP, RA, and AS) based on publicly available GWAS data. According to the findings of our study, a total of 57 gut microbiota were potentially causally associated with the progression of MSK diseases. After multiple testing corrections, we observed a significant causal relationship between Genus Bifidobacterium, Genus Oxalobacter, and Family Oxalobacteraceae with the risk of sarcopenia, LBP and RA, respectively. These findings might provide new ideas for the future treatment of MSK diseases by targeting the specific gut microbiota.
The gut microbiota consists of trillions of bacteria in the gastrointestinal tract, which have functions such as improving intestinal permeability, attenuating the inflammatory response, and participating in the immune regulation of the skeletal system (Rizzoli, 2019). In recent years, the “gut-bone/muscle” axis has received increasing attention in the field of bone health and orthopedic diseases and multiple studies indicated that gut microbiota composition is involved in the MSK disease pathogenesis through multiple pathways (Tu et al., 2021; Zhang et al., 2021). In our study, we discovered that genetically proxied higher abundance of Genus Bifidobacterium was significantly correlated with increased grip strength. And consistent directional effects for all analyses were observed in both MR Egger and weighted median methods, which suggests that Bifidobacterium might be a promising target for sarcopenia prevention. According to recent studies, Bifidobacterium has been identified as a critical taxon for frailty and sarcopenia in the elderly (Ticinesi et al., 2019). In a case–control study of elderly Chinese women, metagenomic sequencing of the gut microbiota revealed that the abundance of Prevotella and Bifidobacterium in the healthy control group was higher than those in the sarcopenia group (Wang et al., 2023). An animal experiment reported that Bifidobacterium longum could improve exercise-associated peripheral fatigue indicators (forelimb grip strength) and oxidative stress-related damage indicators in mice, which indicated Bifidobacterium may be involved in the occurrence and progression of age-related physical frailty and sarcopenia (Huang et al., 2020). In addition, a double-blind randomized clinical trial of older adults 65 aged 65 and over demonstrated that long-term intake of a prebiotic supplement containing Bifidobacterium significantly improved grip strength and reduced the level of fatigue (Buigues et al., 2016). Bifidobacterium might affect sarcopenia through its metabolites, such as short-chain fatty acids (acetate, propionate, and butyrate), which are important for immune regulation and metabolic homeostasis (Wang et al., 2015). Bifidobacterium also contributed to the absorption and utilization of vitamin D and minerals such as calcium, phosphorus, and iron, which are essential for muscle metabolism (Montazeri-Najafabady et al., 2019). Furthermore, Bifidobacterium was rich in genes encoding proteins related to the carbohydrate and amino acid transport and metabolism, influencing protein synthesis and nutrient utilization, which might also be one of the potential mechanisms by which Bifidobacterium prevents sarcopenia (Tu et al., 2023).
Our MR analysis also identified a causal relationship between Genus Oxalobacter, Family Oxalobacteraceae and the prevalence of LBP and RA, respectively. Oxalobacter, is a unique anaerobic bacterium that plays an important role in degrading dietary oxalate and stimulating oxalate secretion by the gut mucosa (Liu et al., 2017; Daniel et al., 2021). Recently, intensive studies have reported that Oxalobacter is closely related to the pathogenesis of kidney stones, hyperoxaluria, and chronic kidney disease (CKD) (Sidhu et al., 1999; Turkmen and Erdur, 2015; Falony, 2018). In addition, Li et al. observed that the diversity and relative abundance of gut microbiota in individuals with RA were significantly different from those in healthy controls. Specifically, the relative abundance of Pelagibacterium was higher and Oxalobacter and Blautia were lower in patients with RA (Li et al., 2021), which was consistent with our findings. Numerous studies emphasized the key role of the gut microbiota in RA pathogenesis, through a variety of mechanisms, including the production of proinflammatory metabolites, impairment of the intestinal mucosal barrier, and regulation of hormones throughout the body (Zhao et al., 2022). However, studies on the role of Oxalobacter in LBP are currently limited. Thus, it is necessary to further study the possible role of Oxalobacter in LBP and RA development.
In addition, we also observed some promising gut microbiota were associated with the risk of OP and AS. Several mechanisms seem to offer preliminary explanation for the relationship between gut flora and OP. On the one hand, the gut microbiota could regulate the Treg/Th17 cells balance or relevant cytokines through the immune system, affecting the intestinal and systemic immune states, thereby establishing a dynamic balance between osteoblasts (OB) and osteoclasts (OC), which is important for normal bone mass maintenance (Locantore et al., 2020). On the other hand, microbial metabolites such as SFAs, secondary bile acid (SBA), and indole derivatives participate in the reconstruction of bone resorption, metabolism and fracture healing by providing energy to gut epithelium cells and promoting calcium and phosphorus absorption (Tu et al., 2021). In addition, intestinal flora imbalance could promote the systemic chronic inflammatory response, which is closely related to the occurrence of AS (Guggino et al., 2021). Stebbings et al. demonstrated that the abundance of Lachnospiraceae, Prevotellaceae, and Bacteroidaceae was higher and that of Ruminococcaceae and Rikenellaceae was lower in AS patients compared with the healthy controls (Stebbings et al., 2002). According to an animal study in mice, regulating the gut microbiota and improving gut barrier function could delay AS progression (Liu et al., 2019). On the basis of the above evidence, we can further investigate how specific gut microbiota affect OP and AS.
Reverse MR analysis showed that fracture may lead to a higher abundance of Family Bacteroidales and sarcopenia may result in a lower abundance of Genus Sellimonas. According to a case–control study of 50 elderly patients, in patients with fracture, the relative abundance of Bacteroidales was higher and Lachnospirales was lower compared to healthy controls, suggesting that altering the microbiome may be an effective way to reduce fracture risk (Rosello-Anon et al., 2023). Another study of postmenopausal Japanese women reported that Bacteroidels and Rikenellaceae may be involved in bone metabolism and fracture risk (Ozaki et al., 2021). Furthermore, an animal study has also revealed that the proportion of Firmicutes and Bacteroidetes increased significantly by 16S RNA sequencing in postmenopausal osteoporosis mice (Wen et al., 2020). In addition, Sellimonas has been shown to be involved in restoring the balance of the intestinal flora (Munoz et al., 2020). Despite this, there has been a very limited amount of literature about Sellimonas. Thus, the underlying mechanism warrants future investigation.
Our study had several strengths. First of all, we used MR analysis to infer that the relationship between the gut microbiome and MSK diseases should be less susceptible to confounding and reverse causation than traditional observational analyses. Additionally, we analyzed the causal effect of each taxon on MSK diseases from the genus to the phylum level, which provides guidance for the prevention and treatment of MSK diseases by targeting specific gut microbiota in clinical practice. Third, the combination of stringent quality control procedures and multiple sensitivity analysis approaches enhances the credibility and robustness in the causal relationships inferred from the MR study. However, the present study also had some limitations that should be noted. Firstly, due to the lack of demographic data in the original study (e.g., gender, age, and race), we could not perform further subgroup analyses to obtain more specific effect relationships. Secondly, the GWAS data on gut microbiota used in this study are based on the population cohort from the largest macro-genome sequencing study to date. In the future, summary data of other gut microbiota needs to be obtained to provide a more comprehensive assessment of the causal relationship between gut microbes and the risk of MSK diseases. Last but not least, this study was confined to individuals of European origin and other populations require further MR studies since causal relationships may vary from race to race.
Overall, our study comprehensively evaluated the potential causal association between gut microbiota and six common MSK diseases. These findings provided new insights into the prevention, progression, and treatment of MSK diseases through targeting specific gut microbiota. In the future, more clinical trials and mechanism studies are needed to explore the exact mechanisms underlying the interactions between the gut microbiota and the prevalence of MSK diseases.
5. Conclusion
In summary, our study provided evidence of a potential causal relationship between specific gut microbiota and MSK diseases through the bi-directional MR analysis. These findings opened up new avenues for exploring the underlying mechanisms by which gut microbiota influence MSK diseases and contributed to the development of targeted interventions and personalized treatments.
Data availability statement
The original contributions presented in the study are included in the article/Supplementary material, further inquiries can be directed to the corresponding authors.
Author contributions
SC collected data. HH, XS, and GZ organized the study and performed the statistical analysis. QZ and ZL drafted the manuscript. All authors contributed to the article and approved the submitted version.
Funding
This work was supported by the Elderly Health Research Project of Jiangsu Commission of Health (LKZ2022008), the Natural Science Foundation of Nanjing University of Chinese Medicine (XZR2021060), and the Foundation of The Second Affiliated Hospital of Nanjing University of Chinese Medicine (SEZ202003).
Acknowledgments
The authors are very grateful to Genetic Factors for Osteoporosis Consortium (GEFOS) and MiBioGen Consortium for their selfless public sharing of GWAS summary data, which provides us with great convenience to carry out this research.
Conflict of interest
The authors declare that the research was conducted in the absence of any commercial or financial relationships that could be construed as a potential conflict of interest.
Publisher’s note
All claims expressed in this article are solely those of the authors and do not necessarily represent those of their affiliated organizations, or those of the publisher, the editors and the reviewers. Any product that may be evaluated in this article, or claim that may be made by its manufacturer, is not guaranteed or endorsed by the publisher.
Supplementary material
The Supplementary material for this article can be found online at: https://www.frontiersin.org/articles/10.3389/fmicb.2023.1238800/full#supplementary-material
References
Asquith, M., Sternes, P. R., Costello, M. E., Karstens, L., Diamond, S., Martin, T. M., et al. (2019). HLA alleles associated with risk of ankylosing spondylitis and rheumatoid arthritis influence the Gut microbiome. Arthritis Rheumatol. 71, 1642–1650. doi: 10.1002/art.40917
Bevan, S. (2015). Economic impact of musculoskeletal disorders (MSDs) on work in Europe. Best Pract. Res. Clin. Rheumatol. 29, 356–373. doi: 10.1016/j.berh.2015.08.002
Biver, E., Berenbaum, F., Valdes, A. M., Araujo, D. C. I., Bindels, L. B., Brandi, M. L., et al. (2019). Gut microbiota and osteoarthritis management: an expert consensus of the European society for clinical and economic aspects of osteoporosis, osteoarthritis and musculoskeletal diseases (ESCEO). Ageing Res. Rev. 55:100946. doi: 10.1016/j.arr.2019.100946
Bowden, J., Davey, S. G., and Burgess, S. (2015). Mendelian randomization with invalid instruments: effect estimation and bias detection through egger regression. Int. J. Epidemiol. 44, 512–525. doi: 10.1093/ije/dyv080
Bowden, J., Davey, S. G., Haycock, P. C., and Burgess, S. (2016). Consistent estimation in Mendelian randomization with some invalid instruments using a weighted median estimator. Genet. Epidemiol. 40, 304–314. doi: 10.1002/gepi.21965
Buigues, C., Fernandez-Garrido, J., Pruimboom, L., Hoogland, A. J., Navarro-Martinez, R., Martinez-Martinez, M., et al. (2016). Effect of a prebiotic formulation on frailty syndrome: a randomized, double-blind clinical trial. Int. J. Mol. Sci. 17:17. doi: 10.3390/ijms17060932
Castro-Mejia, J. L., Khakimov, B., Krych, L., Bulow, J., Bechshoft, R. L., Hojfeldt, G., et al. (2020). Physical fitness in community-dwelling older adults is linked to dietary intake, gut microbiota, and metabolomic signatures. Aging Cell 19:e13105. doi: 10.1111/acel.13105
Cortes, A., Hadler, J., Pointon, J. P., Robinson, P. C., Karaderi, T., Leo, P., et al. (2013). Identification of multiple risk variants for ankylosing spondylitis through high-density genotyping of immune-related loci. Nat. Genet. 45, 730–738. doi: 10.1038/ng.2667
Daniel, S. L., Moradi, L., Paiste, H., Wood, K. D., Assimos, D. G., Holmes, R. P., et al. (2021). Forty years of Oxalobacter formigenes, a gutsy oxalate-degrading specialist. Appl. Environ. Microbiol. 87:e54421. doi: 10.1128/AEM.00544-21
Davey, S. G., and Hemani, G. (2014). Mendelian randomization: genetic anchors for causal inference in epidemiological studies. Hum. Mol. Genet. 23, R89–R98. doi: 10.1093/hmg/ddu328
Falony, G. (2018). Beyond Oxalobacter: the gut microbiota and kidney stone formation. Gut 67, 2078–2079. doi: 10.1136/gutjnl-2018-316639
Guggino, G., Mauro, D., Rizzo, A., Alessandro, R., Raimondo, S., Bergot, A. S., et al. (2021). Inflammasome activation in ankylosing spondylitis is associated with Gut Dysbiosis. Arthritis Rheumatol. 73, 1189–1199. doi: 10.1002/art.41644
Huang, W. C., Hsu, Y. J., Huang, C. C., Liu, H. C., and Lee, M. C. (2020). Exercise training combined with Bifidobacterium longum OLP-01 supplementation improves exercise physiological adaption and performance. Nutrients 12:12. doi: 10.3390/nu12041145
Jia, J., Dou, P., Gao, M., Kong, X., Li, C., Liu, Z., et al. (2019). Assessment of causal direction between Gut Microbiota-dependent metabolites and Cardiometabolic health: a bidirectional Mendelian randomization analysis. Diabetes 68, 1747–1755. doi: 10.2337/db19-0153
Kurilshikov, A., Medina-Gomez, C., Bacigalupe, R., Radjabzadeh, D., Wang, J., Demirkan, A., et al. (2021). Large-scale association analyses identify host factors influencing human gut microbiome composition. Nat. Genet. 53, 156–165. doi: 10.1038/s41588-020-00763-1
Li, C., Liu, C., and Li, N. (2022). Causal associations between gut microbiota and adverse pregnancy outcomes: a two-sample Mendelian randomization study. Front. Microbiol. 13:1059281. doi: 10.3389/fmicb.2022.1059281
Li, Y., Zhang, S. X., Yin, X. F., Zhang, M. X., Qiao, J., Xin, X. H., et al. (2021). The Gut Microbiota and its relevance to peripheral lymphocyte subpopulations and cytokines in patients with rheumatoid arthritis. J Immunol Res 2021, 6665563–6665569. doi: 10.1155/2021/6665563
Liu, M., Koh, H., Kurtz, Z. D., Battaglia, T., PeBenito, A., Li, H., et al. (2017). Oxalobacter formigenes-associated host features and microbial community structures examined using the American Gut project. Microbiome 5:108. doi: 10.1186/s40168-017-0316-0
Liu, B., Yang, L., Cui, Z., Zheng, J., Huang, J., Zhao, Q., et al. (2019). Anti-TNF-alpha therapy alters the gut microbiota in proteoglycan-induced ankylosing spondylitis in mice. Microbiology 8:e927. doi: 10.1002/mbo3.927
Locantore, P., Del, G. V., Gelli, S., Paragliola, R. M., and Pontecorvi, A. (2020). The interplay between immune system and Microbiota in osteoporosis. Mediat. Inflamm. 2020:3686748. doi: 10.1155/2020/3686749
March, L., Smith, E. U., Hoy, D. G., Cross, M. J., Sanchez-Riera, L., Blyth, F., et al. (2014). Burden of disability due to musculoskeletal (MSK) disorders. Best Pract. Res. Clin. Rheumatol. 28, 353–366. doi: 10.1016/j.berh.2014.08.002
Montazeri-Najafabady, N., Ghasemi, Y., Dabbaghmanesh, M. H., Talezadeh, P., Koohpeyma, F., and Gholami, A. (2019). Supportive role of probiotic strains in protecting rats from Ovariectomy-induced cortical bone loss. Probiotics Antimicrob. Proteins 11, 1145–1154. doi: 10.1007/s12602-018-9443-6
Morris, J. A., Kemp, J. P., Youlten, S. E., Laurent, L., Logan, J. G., Chai, R. C., et al. (2019). An atlas of genetic influences on osteoporosis in humans and mice. Nat. Genet. 51, 258–266. doi: 10.1038/s41588-018-0302-x
Munoz, M., Guerrero-Araya, E., Cortes-Tapia, C., Plaza-Garrido, A., Lawley, T. D., and Paredes-Sabja, D. (2020). Comprehensive genome analyses of Sellimonas intestinalis, a potential biomarker of homeostasis gut recovery. Microb. Genom. 6:6. doi: 10.1099/mgen.0.000476
Murray, C. J., Vos, T., Lozano, R., Naghavi, M., Flaxman, A. D., Michaud, C., et al. (2012). Disability-adjusted life years (DALYs) for 291 diseases and injuries in 21 regions, 1990-2010: a systematic analysis for the global burden of disease study 2010. Lancet 380, 2197–2223. doi: 10.1016/S0140-6736(12)61689-4
Okada, Y., Wu, D., Trynka, G., Raj, T., Terao, C., Ikari, K., et al. (2014). Genetics of rheumatoid arthritis contributes to biology and drug discovery. Nature 506, 376–381. doi: 10.1038/nature12873
Ozaki, D., Kubota, R., Maeno, T., Abdelhakim, M., and Hitosugi, N. (2021). Association between gut microbiota, bone metabolism, and fracture risk in postmenopausal Japanese women. Osteoporos. Int. 32, 145–156. doi: 10.1007/s00198-020-05728-y
Pei, Y. F., Liu, Y. Z., Yang, X. L., Zhang, H., Feng, G. J., Wei, X. T., et al. (2020). The genetic architecture of appendicular lean mass characterized by association analysis in the UK biobank study. Commun. Biol. 3:608. doi: 10.1038/s42003-020-01334-0
Pierce, B. L., and Burgess, S. (2013). Efficient design for Mendelian randomization studies: subsample and 2-sample instrumental variable estimators. Am. J. Epidemiol. 178, 1177–1184. doi: 10.1093/aje/kwt084
Rizzoli, R. (2019). Nutritional influence on bone: role of gut microbiota. Aging Clin. Exp. Res. 31, 743–751. doi: 10.1007/s40520-019-01131-8
Rosello-Anon, A., Chiappe, C., Valverde-Vazquez, M. R., Sanguesa-Nebot, M. J., Gomez-Cabrera, M. C., Perez-Martinez, G., et al. (2023). [translated article] pilot study to determine the association between gut microbiota and fragility hip fracture. Rev. Esp. Cir. Ortop. Traumatol. 67, T279–T289. doi: 10.1016/j.recot.2023.03.002
Rosenfeld, S. B., Schroeder, K., and Watkins-Castillo, S. I. (2018). The economic burden of musculoskeletal disease in children and adolescents in the United States. J. Pediatr. Orthop. 38, e230–e236. doi: 10.1097/BPO.0000000000001131
Sanderson, E., Davey, S. G., Windmeijer, F., and Bowden, J. (2019). An examination of multivariable Mendelian randomization in the single-sample and two-sample summary data settings. Int. J. Epidemiol. 48, 713–727. doi: 10.1093/ije/dyy262
Sebbag, E., Felten, R., Sagez, F., Sibilia, J., Devilliers, H., and Arnaud, L. (2019). The world-wide burden of musculoskeletal diseases: a systematic analysis of the World Health Organization burden of diseases database. Ann. Rheum. Dis. 78, 844–848. doi: 10.1136/annrheumdis-2019-215142
Sidhu, H., Schmidt, M. E., Cornelius, J. G., Thamilselvan, S., Khan, S. R., Hesse, A., et al. (1999). Direct correlation between hyperoxaluria/oxalate stone disease and the absence of the gastrointestinal tract-dwelling bacterium Oxalobacter formigenes: possible prevention by gut recolonization or enzyme replacement therapy. J. Am. Soc. Nephrol. 10, S334–S340.
Smith, G. D., and Ebrahim, S. (2003). 'Mendelian randomization': can genetic epidemiology contribute to understanding environmental determinants of disease? Int. J. Epidemiol. 32, 1–22. doi: 10.1093/ije/dyg070
Smith, E., Hoy, D. G., Cross, M., Vos, T., Naghavi, M., Buchbinder, R., et al. (2014). The global burden of other musculoskeletal disorders: estimates from the global burden of disease 2010 study. Ann. Rheum. Dis. 73, 1462–1469. doi: 10.1136/annrheumdis-2013-204680
Stebbings, S., Munro, K., Simon, M. A., Tannock, G., Highton, J., Harmsen, H., et al. (2002). Comparison of the faecal microflora of patients with ankylosing spondylitis and controls using molecular methods of analysis. Rheumatology (Oxford) 41, 1395–1401. doi: 10.1093/rheumatology/41.12.1395
Sudlow, C., Gallacher, J., Allen, N., Beral, V., Burton, P., Danesh, J., et al. (2015). UK biobank: an open access resource for identifying the causes of a wide range of complex diseases of middle and old age. PLoS Med. 12:e1001779. doi: 10.1371/journal.pmed.1001779
Ticinesi, A., Nouvenne, A., Cerundolo, N., Catania, P., Prati, B., Tana, C., et al. (2019). Muscle mass and function in aging: a focus on physical frailty and sarcopenia. Nutrients 11:11. doi: 10.3390/nu11071633
Tu, Y., Kuang, X., Zhang, L., and Xu, X. (2023). The associations of gut microbiota, endocrine system and bone metabolism. Front. Microbiol. 14:1124945. doi: 10.3389/fmicb.2023.1124945
Tu, Y., Yang, R., Xu, X., and Zhou, X. (2021). The microbiota-gut-bone axis and bone health. J. Leukoc. Biol. 110, 525–537. doi: 10.1002/JLB.3MR0321-755R
Turkmen, K., and Erdur, F. M. (2015). The relationship between colonization of Oxalobacter formigenes serum oxalic acid and endothelial dysfunction in hemodialysis patients: from impaired colon to impaired endothelium. Med. Hypotheses 84, 273–275. doi: 10.1016/j.mehy.2015.01.010
Verbanck, M., Chen, C. Y., Neale, B., and Do, R. (2018). Detection of widespread horizontal pleiotropy in causal relationships inferred from Mendelian randomization between complex traits and diseases. Nat. Genet. 50, 693–698. doi: 10.1038/s41588-018-0099-7
Visscher, P. M., Brown, M. A., McCarthy, M. I., and Yang, J. (2012). Five years of GWAS discovery. Am. J. Hum. Genet. 90, 7–24. doi: 10.1016/j.ajhg.2011.11.029
Vos, T., Flaxman, A. D., Naghavi, M., Lozano, R., Michaud, C., Ezzati, M., et al. (2012). Years lived with disability (YLDs) for 1160 sequelae of 289 diseases and injuries 1990-2010: a systematic analysis for the global burden of disease study 2010. Lancet 380, 2163–2196. doi: 10.1016/S0140-6736(12)61729-2
Wang, I. K., Wu, Y. Y., Yang, Y. F., Ting, I. W., Lin, C. C., Yen, T. H., et al. (2015). The effect of probiotics on serum levels of cytokine and endotoxin in peritoneal dialysis patients: a randomised, double-blind, placebo-controlled trial. Benef. Microbes 6, 423–430. doi: 10.3920/BM2014.0088
Wang, Z., Xu, X., Deji, Y., Gao, S., Wu, C., Song, Q., et al. (2023). Bifidobacterium as a potential biomarker of sarcopenia in elderly women. Nutrients 15:15. doi: 10.3390/nu15051266
Wen, K., Tao, L., Tao, Z., Meng, Y., Zhou, S., Chen, J., et al. (2020). Fecal and serum Metabolomic signatures and microbial community profiling of postmenopausal osteoporosis mice model. Front. Cell. Infect. Microbiol. 10:535310. doi: 10.3389/fcimb.2020.535310
Xu, Z., Xie, Z., Sun, J., Huang, S., Chen, Y., Li, C., et al. (2020). Gut microbiome reveals specific Dysbiosis in primary osteoporosis. Front. Cell. Infect. Microbiol. 10:160. doi: 10.3389/fcimb.2020.00160
Zhang, Y. W., Li, Y. J., Lu, P. P., Dai, G. C., Chen, X. X., and Rui, Y. F. (2021). The modulatory effect and implication of gut microbiota on osteoporosis: from the perspective of "brain-gut-bone" axis. Food Funct. 12, 5703–5718. doi: 10.1039/d0fo03468a
Keywords: musculoskeletal diseases, gut microbiota, Mendelian randomization, sarcopenia, causality
Citation: Chen S, Han H, Sun X, Zhou G, Zhou Q and Li Z (2023) Causal effects of specific gut microbiota on musculoskeletal diseases: a bidirectional two-sample Mendelian randomization study. Front. Microbiol. 14:1238800. doi: 10.3389/fmicb.2023.1238800
Edited by:
Karolina Skonieczna-Żydecka, Pomeranian Medical University, PolandReviewed by:
Joseph Atia Ayariga, Alabama State University, United StatesDiego Fernández Lázaro, University of Valladolid, Spain
Copyright © 2023 Chen, Han, Sun, Zhou, Zhou and Li. This is an open-access article distributed under the terms of the Creative Commons Attribution License (CC BY). The use, distribution or reproduction in other forums is permitted, provided the original author(s) and the copyright owner(s) are credited and that the original publication in this journal is cited, in accordance with accepted academic practice. No use, distribution or reproduction is permitted which does not comply with these terms.
*Correspondence: Qing Zhou, qingzhou112@sina.com; Zhiwei Li, 039216235@njucm.edu.cn
†These authors have contributed equally to this work