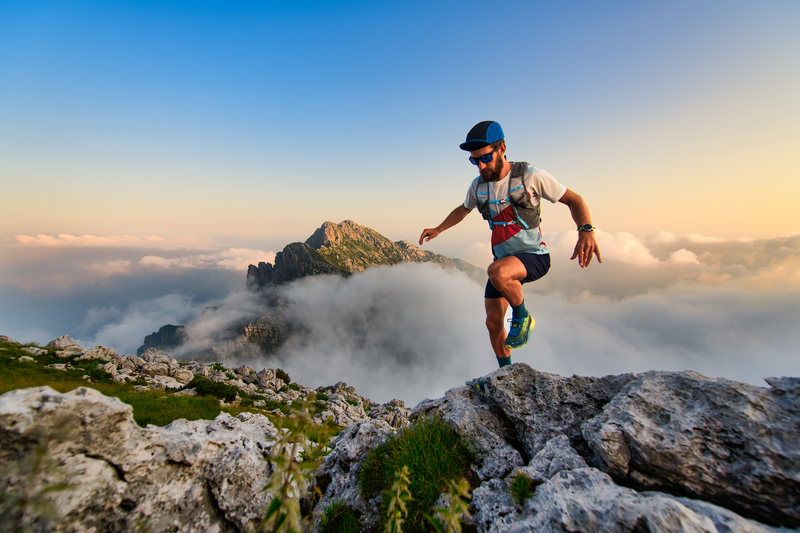
95% of researchers rate our articles as excellent or good
Learn more about the work of our research integrity team to safeguard the quality of each article we publish.
Find out more
ORIGINAL RESEARCH article
Front. Microbiol. , 30 August 2023
Sec. Aquatic Microbiology
Volume 14 - 2023 | https://doi.org/10.3389/fmicb.2023.1219261
This article is part of the Research Topic Ecology and molecular biology of bloom-forming cyanobacteria View all 12 articles
Lake Okeechobee is a large eutrophic, shallow, subtropical lake in south Florida, United States. Due to decades of nutrient loading and phosphorus rich sediments, the lake is eutrophic and frequently experiences cyanobacterial harmful algal blooms (cyanoHABs). In the past, surveys of the phytoplankton community structure in the lake have been conducted by morphological studies, whereas molecular based studies have been seldom employed. With increased frequency of cyanoHABs in Lake Okeechobee (e.g., 2016 and 2018 Microcystis-dominated blooms), it is imperative to determine the diversity of cyanobacterial taxa that exist within the lake and the limnological parameters that drive bloom-forming genera. A spatiotemporal study of the lake was conducted over the course of 1 year to characterize the (cyano)bacterial community structure, using 16S rRNA metabarcoding, with coincident collection of limnological parameters (e.g., nutrients, water temperature, major ions), and cyanotoxins. The objectives of this study were to elucidate spatiotemporal trends of community structure, identify drivers of community structure, and examine cyanobacteria-bacterial relationships within the lake. Results indicated that cyanobacterial communities within the lake were significantly different between the wet and dry season, but not between periods of nitrogen limitation and co-nutrient limitation. Throughout the year, the lake was primarily dominated by the picocyanobacterium Cyanobium. The bloom-forming genera Cuspidothrix, Dolichospermum, Microcystis, and Raphidiopsis were highly abundant throughout the lake and had disparate nutrient requirements and niches within the lake. Anatoxin-a, microcystins, and nodularins were detected throughout the lake across both seasons. There were no correlated (cyano)bacteria shared between the common bloom-forming cyanobacteria Dolichospermum, Microcystis, and Raphidiopsis. This study is the first of its kind to use molecular based methods to assess the cyanobacterial community structure within the lake. These data greatly improve our understanding of the cyanobacterial community structure within the lake and the physiochemical parameters which may drive the bloom-forming taxa within Lake Okeechobee.
Shallow lakes are sensitive to anthropogenic influences (Scheffer, 2004) and cyanobacteria can often dominate the phytoplankton community of eutrophic shallow lakes, especially in warm climates (Reynolds, 1987; Bonilla et al., 2023). Lake Okeechobee is a large shallow subtropical lake in peninsular Florida (United States), that has been undergoing anthropogenic induced eutrophication since the 1970’s (Canfield et al., 2021). The accumulation of nutrients has resulted in an increase in cyanobacterial dominance of the phytoplankton community leading to cyanobacterial harmful algal blooms (cyanoHABs). Lake Okeechobee has a humid subtropical climate and experiences wet (May through November) and dry (November through May) seasons. The lake has a mean depth of ~2.7 m (Havens et al., 1994) and a large drainage basin (12,000 km2), which begins in Orlando running through the Kissimmee Chain of Lakes via the Kissimmee River, flowing south before emptying into the northern region of the lake. This inflow accounts for the majority of the input, with lesser inputs from Lake Istokpoga and Fisheating Creek (Zhang Y. et al., 2020; Canfield et al., 2021). Lake outflow is controlled by the United States Army Corps of Engineers through three main tributaries: south through the Everglades Agricultural Area and ultimately into Florida Bay, west through the Caloosahatchee River into the Gulf of Mexico, and east via the St. Lucie Canal into the St. Lucie Estuary. Land use in the drainage basin is predominantly agricultural (~46%), but urban and suburban areas also exist contributing to the increased nutrient inputs into the lake (Zhang et al., 2011).
Prior to 1974, records show that the phytoplankton community of Lake Okeechobee consisted of <30% cyanobacteria (Marshall, 1977); however, by the 1980’s, the community structure had shifted to a cyanobacteria dominated community (>60%) due to increased eutrophication (Cichra et al., 1995; Havens et al., 1998). This shift to a cyanobacterial dominated community corresponded with an increase in cyanoHABs within Lake Okeechobee. In the past (1970’s–1980’s), cyanoHABs were dominated by diazotrophic cyanobacteria (i.e., Aphanizomenon, Dolichospermum, Raphidiopsis; Joyner, 1974; Marshall, 1977; Jones, 1987), whereas current blooms are often dominated by the non-diazotrophic species Microcystis aeruginosa (Kützing) Kützing; although M. aeruginosa blooms have been reported as early as 1973 (Davis and Marshall, 1975). Despite this, Dolichospermum and Raphidiopsis dominated blooms still occur within the lake, though blooms composed of these genera are less frequent and intense than those composed of Microcystis. These three notorious genera are known to form cyanoHABs globally and have disparate nutrient requirements where Dolichospermum is known to proliferate in low nitrogen conditions, whereas Microcystis prefers high nitrogen, low phosphorus concentrations (Werner and Laughinghouse, 2009; Li and Li, 2012; Chia et al., 2018; Werner et al., 2020). These bloom-forming genera can also produce several cyanotoxins (e.g., anatoxins, cylindrospermopsins, microcystins), resulting in deleterious effects to aquatic systems and human health (O’Neil et al., 2012; Huang and Zimba, 2019).
Environmental drivers of cyanoHABs and bloom-forming genera have been studied in detail (e.g., O’Neil et al., 2012; Paerl et al., 2016). Much of the historical focus was on the role of phosphorus (P) on cyanobacteria productivity, known as the P-only paradigm, although there was a recent shift to focus on the role of both nitrogen (N) and P in bloom proliferation (Paerl et al., 2016). External nutrient loading into Lake Okeechobee, primarily as P, has decreased water quality and total phosphorus (TP) concentrations have nearly doubled since the 1970’s, while total nitrogen (TN) concentrations have remained relatively stable (James and Pollman, 2011). Additionally, much of the P in the lake is legacy phosphorus bound to sediment which, when resuspended, can further increase P concentrations (i.e., internal loading; Moore et al., 1998; Fisher et al., 2005). Because of this increased P loading, primary productivity within Lake Okeechobee has been considered N-limited (Havens, 1995; Havens et al., 2003; Kramer et al., 2018), and periods of increased N loading into the lake have increased cyanoHABs (Havens et al., 2016; Lapointe et al., 2017; Kramer et al., 2018).
In fresh waters, the bacterioplankton community (including cyanobacteria) play critical roles in biogeochemical cycles (e.g., carbon, N, P; Falkowski et al., 2008). Bacteria can form symbiotic relationships with cyanobacteria, either as epibionts on colonies, known as the phycosphere (Bell and Mitchell, 1972) or as co-existing, free living, taxa (Morris et al., 2011). The associated bacteria are capable of filling in missing genomic functions (e.g., vitamin synthesis, nitrogen cycling; Morris et al., 2011; Garcia et al., 2015) and form mutualistic relationships with cyanobacteria (Cook et al., 2020). Thus, associated bacteria have the potential to increase the fitness of cyanobacteria, such as intensifying their growth rate (Jackrel et al., 2021). Despite their close relationships, the role of bacteria in cyanoHABs and relationships with bloom-forming cyanobacteria are often overlooked (Pound et al., 2021). Furthermore, the majority of the focus on bacterial-cyanobacterial interactions have centered on the phycosphere (i.e., epibionts or particle-associated) bacteria, with less focus on the bacterioplankton (i.e., free-living; Louati et al., 2023).
High-throughput sequencing (HTS) facilitates insights into microbial community via sequencing taxonomically informative regions (i.e., metabarcoding), such as the 16S rRNA, or whole genome sequencing (metagenomics). Metabarcoding is used extensively for bacterial communities, including the characterization of the cyanobacterial community (e.g., Pessi et al., 2016; Huang et al., 2020; Khomutovska et al., 2020) as these methods provide valuable information on the cyanobacterial community structure and provide increased taxonomic resolution compared to traditional morphological evaluations alone (MacKeigan et al., 2022).
Extensive research has investigated the global/general drivers of cyanoHABs, with much of the focus on Microcystis and Dolichospermum and intergeneric competition (e.g., O’Neil et al., 2012; Paerl and Otten, 2013; Paerl and Otten, 2016; Almanza et al., 2019; Shan et al., 2019). Within Lake Okeechobee, previous research has studied how various limnological parameters affect shifts within the cyanobacterial community (Havens et al., 1998; Ma et al., 2022), the diversity of phytoplankton including bloom forming genera (Cichra et al., 1995; Ma et al., 2022), and the drivers of increased algal abundance (as chlorophyll; Havens et al., 1994; Xu et al., 2022); However, the specific drivers of bloom forming cyanobacterial genera within Lake Okeechobee remain unexplored. Considering the dominance of cyanobacteria within Lake Okeechobee and the increased frequency and intensity of cyanoHABs (e.g., 2016 and 2018 Microcystis blooms), it is imperative to characterize the cyanobacterial community to identify spatial and temporal trends of common bloom-forming genera, specifically Dolichospermum, Microcystis and Raphidiopsis, and elucidate their respective environmental drivers within this system.
Over the course of 1 year, six sites within Lake Okeechobee were sampled for 16S rRNA metabarcoding analysis and limnological parameters to characterize the cyanobacterial and associated bacterial community. Our objectives were to (1) characterize (temporally and spatially) the cyanobacterial community structure within Lake Okeechobee, (2) elucidate the limnological parameters that potentially drive cyanobacterial abundance, and (3) examine cyanobacterial-bacterial relationships. To our knowledge, a spatiotemporal assessment using molecular methods has yet to be conducted on Lake Okeechobee and this study is the first of its kind.
Sampling on Lake Okeechobee occurred 10 times over the course of 1 year (August 2019–September 2020) at approximately five-week intervals at six locations within the lake, Figure 1. Surface water samples (<0.5 m depth) were collected using acid washed and sterile 1 L Nalgene bottles for environmental DNA extractions and stored on ice until processing. Additional water samples were collected for nutrient analyses (i.e., nitrate, nitrite, ammonium, orthophosphate, and total reactive phosphorus) and major ion analysis (i.e., boron, copper, calcium, potassium, sodium, iron, cobalt, magnesium, manganese, aluminum, and zinc). For orthophosphate and major ion analysis, samples were filtered through a 0.45 μm glass filter (MilliporeSigma, Burlington, MA, United States) in the field and the latter acidified with nitric acid. All samples were kept on ice until processing. Water quality measurements (i.e., dissolved oxygen, water temperature, pH, salinity, conductivity, turbidity, chlorophyll-a abundance, and phycocyanin abundance) were gathered using a YSI EXO3 (Xylem Inc., OH, United States) multiparameter sonde on site. Secchi depth was measured using a Secchi disk and used to estimate water transparency and to calculate photic depth (Zeu). Samples for cyanotoxin analysis were collected in 250 mL HDPE amber bottles. A total of 57 samples were collected during this study.
Immediately upon arrival in the laboratory, water samples for eDNA were filtered through 0.7 μm Whatman glass filters (GF/F MilliporeSigma, Burlington, MA, United States) until clogging and stored at −80°C. Water samples for nutrient composition analysis were frozen and stored, except orthophosphate, which was kept at 4°C until processing. Total reactive phosphorus (TRP; i.e., unfiltered), nitrate, nitrite, and ammonium were analyzed using Standard Methods 4500 (APHA, 2017) on a Seal AutoAnalyzer (Seal AA500; Seal Analytical, WI, United States). Trace elements were quantified using an Avio 200 ICP-OES (Inductively Coupled Plasma Optical Emission Spectrometer) following Standard Method 3,120 (Baird and Bridgewater, 2017). Additional water chemistry parameters were obtained from the South Florida Water Management Districts DBHydro database.1
Mass spectrometry multiple reaction monitoring (MS-MRM) was used to analyze samples from all collection sites for multiple microcystin (MC) congeners as well as nodularin (NOD), saxitoxin (STX), and cylindrospermopsin (CYN). Water samples were frozen and thawed three times, then concentrated using C18 sorbent (Strata-X, Phenomenex Corporation, Torrance, CA, United States, 60 mg sorbent, 3 mL syringe volume). After elution, samples were placed into autosampler vials for high performance liquid chromatography tandem mass spectrometry (HPLC-MS/MS) analysis on an Agilent 1200 series HPLC in-line with an Agilent 6410b triple quadrupole mass spectrometer (Agilent, Santa Clara, CA, United States) fitted with an electrospray ionization source. The autosampler was maintained at 8°C and injected 40 μL of sample. The analytes were passed through a column shield prefilter (MAC-MOD Analytical, Inc., Chadds Ford, PA, United States) and loaded onto a Luna C18(2), 3-μm particle size, 150 × 3 mm column (Phenomenex Corporation, Torrance, CA, United States) heated to 35°C with 100% mobile phase A (90% water, 10% acetonitrile, 0.1% formic acid) at a flow rate of 0.4 mL min−1. Initial conditions were maintained for 2 min, and analytes were eluted over a six-minute gradient from 0% to 90% mobile phase B (100% acetonitrile, 0.1% formic acid) followed by 3 min at 90% mobile phase B, before returning to initial conditions for 3 min. MS/MS analysis used Agilent MassHunter Data Acquisition software (version B.02.01, Agilent, Santa Clara, CA, United States). Samples were run in positive ion mode by MS-MRM and full scan mode (m/z 100–1,200). Data were analyzed using Agilent MassHunter Qualitative Analysis software (version B.03.01, Agilent, Santa Clara, CA, United States). A standard curve (1/y2 weighting) was established for each toxin (except MC-LW, which was quantified using the MC-LR standard curve) by integrating the peak area of the quantifier ion from duplicate standards (6 concentrations ranging from 0 to 10 ng μL−1), with a limit of detection of 0.5 ng on the Phenomenex column. Standards were prepared in methanol and analyzed in the same manner as the samples. To measure the amount of each toxin in the samples, the peak area of the quantifier ion was compared to the appropriate standard curve. The limit of detection of each toxin in water is 0.0003–0.0009 μg L−1 microcystin (varies based on congener), 0.0005 μg L−1 cylindrospermopsin, and 0.0009–0.0013 μg L−1 saxitoxin. Standards for toxin analysis included various sources for microcystins including Enzo Life Sciences (Farmingdale, NY, United States), Cayman Chemical (Ann Arbor, MI, United States), Greenwater Laboratories (Palatka, FL, United States), and CCS purification. Pure saxitoxin standards were purchased from Cayman Chemical (Ann Arbor, MI, United States) and additional material was isolated from a toxic strain of Dolichospermum circinale (obtained from Dr. Brett Neilan). Cylindrospermopsin standards were obtained from Dr. Brett Neilan.
DNA was extracted using a DNeasy Blood and Tissue Kit (Qiagen, Hilden, Germany), modified according to Djurhuus et al. (2017). The V4–V5 hypervariable regions of the 16S rRNA were amplified using 515FY-926R primer pair described in Parada et al. (2016). Samples were amplified in triplicate before pooling. Amplicon libraries were sequenced using paired-end (2 × 250 bp) Illumina Novaseq (Novogene, Beijing, China), sequencing depth varied from 88,722 to 139,935 reads per samples with a mean of 129,647. The V3–V4 variable regions of the 16S rRNA were obtained by using both sets of cyanobacterial specific primer pairs (i.e., CYA359F-781Ra/b) described by Nübel et al. (1997). However, these produced a low number of cyanobacterial ASV’s due to amplification of eukaryotic phytoplankton chloroplast 16S rRNA sequences and were thus excluded from analysis (data not shown).
Amplicon sequences were demultiplexed and assigned to specific sample IDs based on their MIDs at Novogene using an in-house bioinformatic pipeline. DADA2 (Callahan et al., 2016) was used to process raw sequences in R v4.0.0 (R Core Team, 2023). Paired-end reads were filtered, trimmed, and merged. Cleaned and merged reads were dereplicated and subsequently analyzed for detection and removal of potential chimeras using DADA2. Non-chimeric sequences were pooled to define amplicon sequence variants (ASVs) and identical ASVs which only varied in length were collapsed using the “collapseNoMismatch” command in DADA2, ASVs ranged in length from 325 to 393 nt.
Taxonomic assignment of ASVs was based on a naïve Bayesian classifying method (Wang et al., 2007) with CyanoSeq V1.2 (Lefler et al., 2023) and SILVA 138.1 (Quast et al., 2012) as the taxonomic databases. The CyanoSeq database was supplemented with 16S rRNA sequences from unialgal cyanobacterial cultures isolated from Lake Okeechobee and surrounding fresh waters housed in the Berthold Laughinghouse Culture Collection (BLCC) at the University of Florida – IFAS, Fort Lauderdale Research and Education Center (Davie, FL, United States). All non-cyanobacterial ASVs, including chloroplasts, were removed prior to downstream analyses. All archaeal, chloroplast, eukaryotic, and mitochondrial ASVs were removed for network analysis. A maximum likelihood phylogenetic tree of the cyanobacterial ASVs was created using RAxML-NG (Kozlov et al., 2019), by determining the sequence evolutionary model (GTR-I-G4) using ModelTestNG (Darriba et al., 2020). A maximum likelihood phylogenetic tree of the bacterial ASVs was created using IQTree with ultrafast bootstrapping (Minh et al., 2020).
ASV’s which corresponded to the Aphanizomenonaceae and Microcystaceae were extracted, and phylogenetic trees were constructed for each family. Cyanobacterial ASVs that could not be classified to the genus level (except for Prochlorococcaceae) were extracted and placed in the reference tree from CyanoSeq (v1.2) along with their three closest BLAST hits to provide increased resolution. Sequences were added to the alignment using MAFFT (Katoh and Standley, 2013), full length sequences (i.e., >600 bp) were added with—add and—keeplength parameters, while ASVs and short sequences (i.e., <600 bp) were added using—add-fragment and—keeplength parameters. The alignment was trimmed using TrimAl (Capella-Gutiérrez et al., 2009) using -automated1 parameter and the sequence evolutionary model was determined using ModelTestNG (Darriba et al., 2020). The phylogenetic tree was built using RAxML-NG with 1,000 bootstrap replicates (Kozlov et al., 2019).
Sequence read abundances were filtered using the phyloseq package (McMurdie and Holmes, 2013). ASVs that occurred in less than 10% of samples or occurred less than 100 times across all samples were removed from all downstream analyses, except alpha diversity. The vegan package (Oksanen et al., 2019) was used for statistical analyses, calculation of richness and diversity indices, and generation of ordinations in combination with ggplot2 (Wickham, 2016) in R v4.0.0 (R Core Team, 2023). Alpha diversity was calculated using Faith’s Phylogenetic Distance indices, and Wilcoxon tests were used to compare between groups. Data were not rarefied (McMurdie and Holmes, 2014), prior to analyses, the data were log-transformed (1og10) to avoid biases toward rare species and minimize influence of most abundant groups. Indicator species were determined using the indicspecies package (De Cáceres and Legendre, 2009).
Similarities in cyanobacterial communities among sampling sites and seasons (i.e., wet vs. dry season, nitrogen limitation vs. co-limitation) were explored using the Non-Metric Multidimensional Scaling (NMDS) analysis with generalized Unifrac distances (Chen J. et al., 2012; Chen X. et al., 2012). The “adonis2” function of the vegan package was used to conduct a permutational multivariate analysis of variance (PERMANOVA) on generalized Unifrac distances to test the effect of sampling sites, nutrient limitation, and seasonal impact on cyanobacterial community composition. Partial redundancy analysis (pRDA) was employed using the rda() function in the vegan package to find relationships between significant environmental variables (p ≤ 0.05) and Cuspidothrix, Cyanobium, Dolichospermum, Microcystis, Raphidiopsis, and Vulcanococcus were selected as these were the five most abundant described genera in our data. Environmental variables were standardized based on square root transformation prior to analysis.
Generalized additive models (GAMs) were used to model the relationship between cyanobacterial genera (as rarefied read abundance) and limnological parameters (e.g., water temperature, nutrients, etc.) with sampling sites and outing as random effects. GAMs were conducted in R using the mgcv package (v1.8-42; Wood, 2011) and drawn with gratia (Simpson, 2023) and ggplot2.
Cyanobacterial-bacterial relationships were explored using the Sparse Inverse Covariance Estimation for Ecological Association Inference (SpiecEasi; v1.1.0) package in R (Kurtz et al., 2015) using the top 500 most abundant genera. Networks were visualized in Cytoscape v3.9.1 (Shannon et al., 2003).
Dissolved inorganic nitrogen to dissolved inorganic phosphorus ratio (DIN:DIP) was determined, as was the dissolved inorganic nitrogen (DIN), defined here as the sum of nitrate, nitrite, and ammonia, to soluble reactive phosphate. DIN:DIP ratio ranged from 0.02 to 75, while DIN ranged from 0.05–0.634 mg L−1. These data are reported in Supplementary Data S1. Total nitrogen and phosphorus measurements were obtained from the South Florida Water Management Districts DBHydro database; the TN:TP mass ratio ranged from 11 to 71 with a mean of 28. Total nitrogen concentrations ranged from 0.87–3.14 mg L−1and total phosphorus concentrations ranged from 0.056–0.392 mg L−1. Periods of nutrient limitation were determined by collecting TN and TP data from the sites in closest proximity to our sampling sites and plotting the TN:TP ratio as a time series during our sampling events (Supplementary Figure S1). Only one site indicated phosphorus limitation (TN:TP ≥ 23) and was thus excluded from further analyses. Nutrient limitation was based on values provided by Paerl et al. (2016) where N:P ≥ 23 indicates P-limitation, N:P ≤ 9 indicates N-limitation, and 23 > N:P > 9 indicates co-nutrient limitation. Other water quality parameters (e.g., trace elements, conductivity, photic depth) are reported in Supplementary Data S1. Daily mean lake depth was determined from the LZ40 station (lat 26.901815, long −80.789003) and ranged from 3.5–4.7 m.
After filtering, there were an average of 67,826 (min = 44,914, max = 84,259, sd = 8,876) reads across samples assigned to 4,048 ASVs, 274 of which were cyanobacteria with an average of 20,168 (min = 2,429, max = 58,317, sd = 11,403) reads. The cyanobacteria, phylum Cyanobacteriota, frequently made up >25% of the total bacterial community (Supplementary Figure S2A). Based on relative read abundance, the Synechococcales, Nostocales, and Chroococcales were the most abundant cyanobacterial orders (Supplementary Figure S2B), with the Prochlorococcaceae, Aphanizomenonaceae, and Microcystaceae as the most abundant families (Supplementary Figure S2C). The most abundant genus within Lake Okeechobee was Cyanobium, a member of the Prochlorococcaceae, followed by Dolichospermum, and Microcystis. The most abundant toxigenic bloom-forming genera were Dolichospermum and Microcystis (Figure 2), although several other potentially toxic bloom-forming genera were found throughout the lake at lower relative abundances including Aphanizomenon, Cuspidothrix, Raphidiopsis, and Sphaerospermopsis.
Figure 2. Bar plots showing the relative abundance of most abundant cyanobacterial genera found during the study.
Due to the high abundance and bloom potential of the Aphanizomenonaceae and Microcystaceae, phylogenetic inferences of these ASVs were conducted to confirm taxonomic assignment (Supplementary Figures S3, S4). All ASVs assigned to a genus were found to be monophyletic with their respective genus. ASV1987 was assigned as “Aphanizomenonaceae” but phylogenetic inferences revealed this belonged to Amphiheterocytum and was manually reassigned (Supplementary Figure S3). ASV176 was only assigned to the rank “Aphanizomenonaceae” and formed a well-supported clade away from known genera within the Aphanizomenonaceae, thus this was reassigned as “Aphanizomenonaceae Cluster 1” (Supplementary Figure S3). ASV1176 was assigned as “Microcystaceae” but phylogenetic inferences revealed this belonged to Coelosphaerium and was manually reassigned (Supplementary Figure S4). Three ASVs (ASV339, ASV751, ASV2762) were assigned as Microcystaceae and formed a well-supported clade away from known genera within the Microcystaceae, thus reassigned as “Microcystaceae Cluster 1” (Supplementary Figure S4). There were several sequences which were classified as Microcystaceae X, an undescribed genus within the Microcystaceae; this genus was within the top 15 most abundance genera (Figure 2). These ASVs formed a clade with no cultured strains (Supplementary Figure S4), only sequences which were collected in a culture-independent manner from other fresh waterbodies (e.g., Reelfoot Lake, Tennessee, United States). A phylogenetic tree of the picocyanobacteria, order Synechococcales, was constructed with 259 sequences, 176 of which were ASVs (Supplementary Figure S5).
Phylogenetic inferences of ASVs which could not be classified past the order level were also conducted. These were found in several clades across the tree (Supplementary Figure S6). Two ASVs (125 and 2,225) were only classified as Cyanophyceae class and manually reassigned as “Leptolyngbyaceae Cluster 1,” as these ASVs formed a clade within the Leptolyngbyaceae with sequences from other freshwater lakes. AVS’s 378 and 1838 were also only classified at the class level and fell within the Synechococcaceae and reassigned as “Synechococcaceae Cluster 1.” These ASV’s formed a well-supported clade with other uncultured sequences from freshwater bacterioplankton samples. ASV3311 was found to be Pseudanabaena, ASV5289 clustered with Neocylindrospermum, and several ASVs (ASV170, ASV437, ASV669, ASV793, ASV5103) clustered with Nodosilinea; these were all manually reassigned.
A network was created to observe the correlations between most abundant cyanobacterial genera (Figure 3). There were no correlated taxa, bacterial nor cyanobacterial, shared between Dolichospermum, Microcystis, and Raphidiopsis. Dolichospermum was positively correlated with several taxa, and negatively correlated with two bacteria including Acidibacter. Microcystis was correlated with less taxa than Dolichospermum, and negatively correlated with Rheinheimera. Raphidiopsis was also negatively correlated with Rheinheimera, in addition to Legionella. Raphidiopsis was correlated with several cyanobacterial taxa, in comparison to Dolichospermum and Microcystis. Pseudanabaena was correlated to both Microcystis and Cuspidothrix, however Microcystis and Cuspidothrix were not correlated with each other. The Prochlorococcecean taxa (i.e., Cyanobium, Regnicoccus, Lacustricoccus, Vulcanococcus) shared several correlated taxa, distinct from the crown cyanobacteria.
Figure 3. Network analysis of the most abundant cyanobacterial genera and the significant cyanobacterial-(cyano)bacterial relations. Edge thickness represent weight and color represent positive (red) or negative (blue) correlations. Box color represent phylum.
There were no significant differences in taxonomic richness between cyanobacterial communities based on either metric at each sampling site (Figures 4A,B). The NMDS revealed overlap between these communities (Figure 4C), and results from the pairwise PERMANOVA showed that there were no significant differences in cyanobacterial communities between sites (p > 0.05). The southern region of the lake (i.e., Clewiston and South Lake) had higher relative abundances of Dolichospermum, while the northern region near the mouth of the Kissimmee River had a higher relative abundance of Microcystis; Moore Haven had the highest relative abundance of Raphidiopsis (Figure 4D).
Figure 4. Diversity measures of the cyanobacterial communities at the different sampling locations. Faith phylogenetic diversity (A) and Chao1 index (B) between each sampling location. Non-metric Multidimensional Scaling (NMDS) ordination within two dimensions of the cyanobacterial communities based on generalized-Unifrac distances between sampling locations, colors represent (C). Bar plot of the average relative abundance of the most abundant cyanobacterial genera at each location (D).
Similar to the cyanobacterial communities, there were no significant differences in taxonomic richness between bacterial communities based on either metric at each sampling site (Supplementary Figures S7A,B). The NMDS revealed overlap between these communities (Supplementary Figure S7C), and results from the pairwise PERMANOVA showed that there were significant differences between bacterial communities between Clewiston and Kissimmee Mouth (R2 = 0.11, p = 0.03). There were no major differences between relative abundances of bacterial phyla between sites (Supplementary Figure S7D).
There were no significant differences in taxonomic richness in cyanobacterial communities between the wet and dry seasons (Figures 5A,B). The non-metric multidimensional scaling (NMDS) analysis showed an overlap between wet and dry seasons for the cyanobacterial communities (Figure 5C). Results from PERMANOVA showed significant differences between the cyanobacterial communities in the wet and dry seasons, although season accounted for a relatively small proportion of the variation data (R2 = 0.06, p < 0.01). The relative abundance of the Prochlorococcaceae was nearly equal between wet and dry seasons, accounting for ~60% of the cyanobacterial relative abundance (Supplementary Figure S8A). Dolichospermum relative abundance was nearly double in the wet season compared to the dry season, where it was also the dominant non-Prochlorococcaceae taxon. There also appeared to be an increased relative abundance in Amphiheterocytum, Cuspidothrix, and Raphidiopsis in the dry season; Microcystis relative abundance appeared even between the two seasons (Figure 5D). Indicator species, as genera, between seasonal communities were determined and listed in Supplementary Table S1.
Figure 5. Diversity measures of the cyanobacterial communities between wet and dry season. Faith phylogenetic diversity (A) and Chao1 index (B) between each season. Non-metric Multidimensional Scaling (NMDS) ordination within two dimensions of the cyanobacterial communities based on generalized-Unifrac distances between sampling locations, colors represent season (C). Bar plot of the average relative abundance of the most abundant, non-prochlorococcacean, cyanobacterial genera during each season (D).
There were no significant differences in taxonomic richness in bacterial communities between the wet and dry seasons (Supplementary Figures S9A,B). The non-metric multidimensional scaling (NMDS) analysis showed an overlap between wet and dry seasons for the cyanobacterial communities (Figure 5C). Results from PERMANOVA showed significant differences between the bacterial communities in the wet and dry seasons, although season accounted for a relatively small proportion of the variation data (R2 = 0.08, p < 0.001). There was an increased relative abundance of Proteobacteria (=Pseudomonadota) in the wet season, and an increased relative abundance of Actinobacteria (=Actinomycetota) in the dry season (Supplementary Figure S9D).
The relative abundance of the phylum Cyanobacteriota within the lake did not differ between seasons comprising ~30% of the relative abundance (Supplementary Figure S8C). Additionally, there were no significant differences in cyanobacterial communities between seasons based on alpha diversity metrics (Figures 5A,B). Conversely, the cyanobacterial communities differed between seasons based on results from the PERMANOVA.
There were no significant differences in taxonomic richness between cyanobacterial communities during N-limitation and co-limitation (Figures 6A,B). The NMDS analysis showed an overlap between the cyanobacterial communities during N-limitation and co-limitation (Figure 6C). When comparing the N-limited and co-limited cyanobacterial communities, there were no significant differences in community composition (PERMANOVA R2 = 0.02, p = 0.29). The relative abundance of the Prochlorococcaceae was nearly equal between N-limited and co-limited communities and accounted for ~55%–60% of the relative abundance, although their relative abundance was slightly higher in N-limited communities (Supplementary Figure S8B). When observing the non-Prochlorococcaceae taxa, there appears to be a non-significant increased relative abundance in Dolichospermum in the N-limited communities compared to the co-limited communities (PERMANOVA R2 = 0.02, p = 0.5), while Microcystis relative abundance showed the opposite trend (PERMANOVA R2 = 0.05, p = 0.2; Figure 6D). Indicator species, as genera, between N-limited and co-limited communities were determined; only Planktothrix and Synechococcaceae Cluster 1 were determined to be indicator species within N-limited communities. There was an increased relative abundance of cyanobacteria (=Cyanobacteriota) in the co-limited communities compared to the N-limited communities (Supplementary Figure S8D).
Figure 6. Diversity measures of the cyanobacterial communities between nitrogen and co-nutrient limitation. Faith phylogenetic diversity (A) and Chao1 index (B) between nutrient limitations. Non-metric Multidimensional Scaling (NMDS) ordination within two dimensions of the cyanobacterial communities based on generalized-Unifrac distances between nutrient limitations, colors represent limitation (C). Bar plot of the average relative abundance of the most abundant, non-prochlorococcacean, cyanobacterial genera during nutrient limitations (D).
There were no significant differences in taxonomic richness between bacterial communities during N-limitation and co-limitation (Supplementary Figures S10A,B). The NMDS analysis showed an overlap between the bacterial communities in the wet and dry seasons (Supplementary Figure S10C). When comparing the N-limitation and co-limited cyanobacterial communities, there were no significant differences in community composition (PERMANOVA R2 = 0.01, p = 0.42). There were no major differences between relative abundances of any bacterial phyla between seasons (Supplementary Figure S10D). There was an increased relative abundance of Proteobacteria (=Pseudomonadota) and Actinobacteria (=Actinomycetota) in N-limited communities, and an increased relative abundance of Planctomycetota in the co-limited communities (Supplementary Figure S10D).
Due to the high relative abundance of Cuspidothrix, Cyanobium, Dolichospermum, Microcystis, Raphidiopsis, and Vulcanococcus (Figure 2) the environmental drivers of these taxa were subjected to further investigation via a partial redundancy analysis (pRDA). From the model, the conditioned variables (sample sites and outings) explained 11% of the variation, while water chemistry explained 34.4% of the variation, the remaining 54.6% of the variation was unexplained; the value of p for the model was 0.002. The triplot from the pRDA revealed that Cuspidothrix, Cyanobium, Microcystis, Raphidiopsis, and Vulcanococcus were associated with each other, but not Dolichospermum (Figure 7). Cuspidothrix, Cyanobium, Microcystis, and Vulcanococcus were positively associated with increased dissolved oxygen, copper, and zinc concentrations, and inversely associated with orthophosphate and iron concentration. Dolichospermum was positively associated with photic depth, inversely associated with DIN, and not associated with TRP.
Figure 7. Partial redundancy analysis (pRDA) ordination within two dimensions of the cyanobacterial genera Cuspidothrix, Cyanobium, Dolichospermum, Microcystis, Raphidiopsis, and Vulcanococcus. Drivers of taxonomic variation are shown by blue arrows. Significant environmental drivers are shown by red arrows. Circles indicate samples, colors indicate sample site.
Effects of individual limnological parameters (i.e., water temperature, photic depth, lake depth, DIN, TRP, and DIN:DIP) on Cuspidothrix, Cyanobium, Dolichospermum, Microcystis, Raphidiopsis, and Vulcanococcus relative abundances were investigated using GAMs. In this study, GAMs used the negative binomial distribution assumption; sample outing and site were regarded as random effects. Results are listed in Table 1. The effects of water temperature, photic depth, lake depth, and conductivity on bloom-forming genera (i.e., Cuspidothrix, Dolichospermum, Microcystis, and Raphidiopsis) are visualized in Figure 8 and the relationship between nutrients and bloom-forming cyanobacteria are visualized in Figure 9. The diazotrophic bloom-forming genera (i.e., Cuspidothrix, Dolichospermum and Raphidiopsis) were inversely correlated with increases in DIN (Figures 9A,C,D; Table 1), whereas Microcystis was positively correlated with DIN (Figure 9B; Table 1). Dolichospermum was positively, and significantly, correlated with increased TRP concentrations whereas Raphidiopsis relative abundance decreased with increasing TRP concentrations, although this trend was not significant (Figures 9F,H; Table 1). Cuspidothrix relative abundance was positively correlated with TRP concentrations up until ~0.5 mgL−1 after which it began to decrease (Figure 9E; Table 1). There was no noticeable relationship between Microcystis relative abundance and TRP concentrations (Figure 9F; Table 1), Both Cuspidothrix and Dolichospermum relative abundances were negatively correlated with DIN:DIP, whereas Microcystis and Raphidiopsis relative abundances were positively, and linearly, correlated with DIN:DIP (Figures 9I–L; Table 1). Water temperature had a varied response on the bloom-forming genera, with Cuspidothrix and Raphidiopsis having the highest relative abundances in the cooler waters (~25°C) of the dry season, whereas Dolichospermum and Microcystis relative abundances increased with increasing water temperatures (Figures 8A–D). Both Dolichospermum and Microcystis relative abundances peaked around 30°C, in the warmer wet season. Dolichospermum and Microcystis relative abundances were positively correlated with photic depth (Figures 8E–H; Table 1), whereas there were no trends between Cuspidothrix and Raphidiopsis relative abundances and photic depth. Furthermore, lake depth had a significant negative linear relationship with Raphidiopsis relative abundance (Figure 8L; Table 1).
Figure 8. Effects of water temperature (A–D), photic depth (E–H), lake depth (I–L), on bloom forming cyanobacteria as identified with generalized additive models (GAMs). Shaded areas indicate 95% confidence intervals, shapes indicate limitation, and colors indicate season.
Figure 9. Effects of dissolved inorganic nitrogen (A–D), total reactive phosphorus (E–H), and DIN:DIP (I–L) on bloom forming cyanobacteria as identified with generalized additive models (GAMs). Shaded areas indicate 95% confidence intervals, shapes indicate limitation, and colors indicate season.
The effects of water temperature, photic depth, lake depth, and conductivity on Cyanobium and Vulcanococcus are visualized in Supplementary Figure S11 and the effects of nutrients in Supplementary Figure S12. Water temperature had a positive linear relationship on both Cyanobium and Vulcanococcus relative abundance, although this relationship was greater on Vulcanococcus (Supplementary Figures S11A,D; Table 1). Both Cyanobium and Vulcanococcus relative abundance had a negative relationship with both photic and lake depth (Supplementary Figures S11B,C,E,F; Table 1). DIN had a negative, although weak, correlation with Cyanobium and Vulcanococcus relative abundance (Supplementary Figures S12A,D; Table 1). TRP had a strong negative correlation with Cyanobium relative abundance (Supplementary Figure S12B; Table 1). DIN:DIP had no relationship with Cyanobium relative abundance, but had a negative, and linear, relationship with Vulcanococcus relative abundance (Supplementary Figures S12C,F; Table 1).
Cyanotoxins were detected on 16 occasions over the annual cycle at the six sites sampled. Microcystins, nodularins, and anatoxin-a were detected throughout the lake and through time (Supplementary Table S2). Both microcystin-LR (MC-LR) and microcystin-RR (MC-RR) were detected with MC-LR being the most commonly occurring congener; MC-LR was detected 11 times and MC-RR once. Nodularins were detected seven times, and anatoxin-a was detected once. Microcystin-LR co-occurred with nodularins three times. Cyanotoxin concentrations were low, and ranged between 0.04 and 1.4 μg L−1, with nodularin being the toxin with the highest concentration (1.12 μg L−1), while MC-LR reached concentrations of 0.6 μg L−1. Cyanotoxins were detected nine times during the wet season in four out of six sampling events and seven times in the dry season in three out of four sampling events. Anatoxin-a was only detected once, in the wet season. Nodularins occurred more frequently in the dry season than the wet season (five vs. two occurrences) while microcystins were observed six times in the wet season and five times in the dry season. Due to the infrequent occurrence of these toxins, statistical analyses to elucidate drivers of their occurrence proved unsuccessful (data not shown).
Several known toxin producing genera occurred (e.g., Aphanizomenon, Dolichospermum, Microcystis, Raphidiopsis). A correlation analyses was applied to identify which genera were correlated with these cyanotoxins (Supplementary Figure S13). There were several genera correlated with MC-LR, with Microcystis being the only confirmed microcystin producer in Lake Okeechobee. Several genera were correlated to MC-RR, with Chrysosporum, Microcystis, and Planktothricoides being the known toxin producing genera. Aphanizomenon, Lagosinema, Microcystaceae Cluster 1, Parasynechococcus, Planktothricoides, Prochlorococcaceae_XX, and Pseudanabaena were the genera most correlated with nodularins (Supplementary Figure S13).
This study provides a detailed analysis of Lake Okeechobee’s spatiotemporal cyanobacterial and bacterial community structure. Lake Okeechobee has gained notoriety for its Microcystis-dominated cyanoHABs in the past decade. Until now, characterizations of the cyanobacterial community structure in Lake Okeechobee have been carried out via microscopy (e.g., Havens et al., 1994; Cichra et al., 1995; Beaver et al., 2013), apart from Kramer et al. (2018) which focused on full metagenomic sequencing of the cyanoHAB that occurred in 2016. Thus, few data exist on molecular characterizations of the bacterial/cyanobacterial community structure within Lake Okeechobee and this study is the first of its kind.
Many of the cyanobacterial taxa that are well documented in the literature via microscopy (e.g., Aphanizomenon, Dolichospermum [=Anabaena], Microcystis, and Raphidiopsis [=Cylindrospermopsis]) were identified from the molecular methods employed in this study. Surprisingly, the high abundance of Prochlorococcacean cyanobacteria was not expected, as these taxa are not well recorded in Lake Okeechobee, likely due to their small size (< 2 μm). Additionally, the cyanobacterial genus Planktolyngbya, whose presence in Lake Okeechobee is well documented (e.g., Beaver et al., 2013), was not observed in the molecular data. However, Limnolyngbya was observed which was separated from Planktolyngbya (Li and Li, 2016), and may be the correct taxon. Within the Aphanizomenonaceae a single ASV (176) clustered with sequences classified as Anabaena and Dolichospermum but away from these genera (Supplementary Figure S3). Within the Microcystaceae, the ASVs labeled as Microcystaceae Cluster 1, clustered with sequences from the freshwater lakes, Las Cumbres Lake (Panama) and Reelfoot Lake (Tennessee, United States), and may represent a widespread cyanobacterium (Supplementary Figure S4). Phylogenetic inferences of the ASVs that could not be classified past the class level revealed potentially novel cyanobacterial diversity within the lake. There were two clades of ASV’s which clustered within the Leptolyngbyaceae and Synechococcaceae, respectively, which may represent novel diversity (Supplementary Figure S6).
Picocyanobacteria (<2 μm) belonging to the family Prochlorococcaceae dominated the cyanobacterial community throughout the lake, with Cyanobium demonstrating the highest relative abundance followed by Vulcanococcus. The genera Dolichospermum, Microcystis, and Raphidiopsis are known to cause cyanoHABs within Lake Okeechobee (e.g., Jones, 1987; James et al., 2008; Kramer et al., 2018) and were highly abundant throughout the lake during this study (Figure 2). Other bloom-forming, diazotrophic Aphanizomenon-like and Dolichospermum-like genera, such as Cuspidothrix and Sphaerospermopsis, were also observed in the molecular data, although their presence in Lake Okeechobee have not been recorded, likely due to their cryptic morphology (Rajaniemi et al., 2005; Werner et al., 2012).
Notably, Dolichospermum was not correlated to microcystins nor to nodularins (Supplementary Figure S13). Dolichospermum is known to produce several cyanotoxins (Otten and Paerl, 2015), however toxin production by Dolichospermum within Lake Okeechobee remains unknown, although metagenomic analyses suggest it may produce saxitoxin (Kramer et al., 2018). From the correlation analysis, the potential producer of nodularins remains obscure as none of the positively correlated genera are known producers of nodularins (Supplementary Figure S13). Conversely, Iningainema is an established nodularin producer (McGregor and Sendall, 2017; Berthold et al., 2021) which is known to occur in the lake (Laughinghouse lab, unpublished data), however its abundance was negatively correlated to nodularin concentrations (Supplementary Figure S13). Microcystis is a known microcystins producer within Lake Okeechobee (Lefler et al., 2020; Kinley-Baird et al., 2021) and is the likely toxin producer, although other taxa may also be producing these toxins. The correlations between the picocyanobacteria genera and toxins (i.e., Parasynechococcus with MC-LR, and Prochlorococcaceae_XX with nodularin) were unexpectedly high considering the diversity of the known toxigenic taxa (Supplementary Figure S13). While this group are not traditionally considered toxigenic, it has been recently found that picocyanobacteria in tropical freshwaters are capable of cylindrospermopsin production (Gin et al., 2021; Sim et al., 2023). Thus, it imperative to further assess the toxigenic potential of these abundant cyanobacteria. The lack of definitive correlations between a genus (or genera) and toxins highlights the unknown toxigenic potential within the lake.
The community structure was more variable during the wet season, while communities from the dry season were more similar (Figure 5C). Communities within both seasons were dominated by picocyanobacteria (e.g., Cyanobium) with an non-significant increase in Dolichospermum in the wet season (PERMANOVA R2 = 0.02, p = 0.2), and significant increase in Raphidiopsis in the dry season (PERMANOVA R2 = 0.08, p = 0.02; Figure 5D). Raphidiopsis relative abundance was higher in the dry season (Figure 5D) and determined to be an indicator species for dry season communities (Supplementary Table S1). Furthermore, Raphidiopsis relative abundance was higher at Moore Haven, the headwaters of the Caloosahatchee River, a shallow area of the lake within the rim canal. While Raphidiopsis blooms are uncommon in Lake Okeechobee, Raphidiopsis-dominated blooms have recently been observed in the shallow areas of the lake (i.e., transition zone) during dry periods (Laughinghouse and Lefler, pers. observ.). The communities at the mouth of the Kissimmee River had a higher relative abundance of Microcystis in comparison to other locations (Figure 4D). This region of Lake Okeechobee is known to have increased frequency of Microcystis-dominated cyanoHABs, likely due to external nutrient loading from the Kissimmee drainage basin (Havens et al., 1994). Conversely, the southern region of the lake is distant from major inflows and thus external nutrient loadings and was found to possess higher relative abundance of Dolichospermum (Figures 2, 4D).
Increases in both N and P are known to drive the growth of bloom-forming genera, although their concentrations and ratios have disparate effects on these genera (Paerl et al., 2016). Our results highlight the disparate responses in abundances of these bloom-forming genera to N, P, and DIN:DIP. While increasing DIN concentrations had a negative relationship on relative abundance of the bloom-forming diazotrophic genera, as expected, only Dolichospermum relative abundance had a positive, and linear, response to increasing TRP concentrations (Figures 9E,G,H). Cuspidothrix relative abundance was highest with TRP concentrations around ~0.5 mg L−1 (Figure 9E), potentially indicating this genus has lower P requirements, but higher than that of Raphidiopsis. These data suggest that P concentrations do not affect all bloom-forming diazotrophic genera similarly. Furthermore, Raphidiopsis relative abundance had an increased, although weak, response to DIN:DIP, whereas Cuspidothrix and Dolichospermum relative abundances were negatively correlated with increasing DIN:DIP (Figures 9I,K,L), suggesting that DIN:DIP, and potentially TN:TP, does not affect all diazotrophs equally. Dolichospermum and Microcystis relative abundances were positively affected by photic depth (Figures 8F,G; Table 1), supporting previous research on their drivers within Lake Okeechobee (Havens et al., 1998, 2003). Cuspidothrix and Raphidiopsis relative abundances were both generally unaffected by photic depth, indicating these genera are more adapted to low light conditions, an observation supported by previous research on Raphidiopsis within the system (Havens et al., 2003).
Altogether, these genera have overlapping, but distinct niches within Lake Okeechobee. Dolichospermum and Microcystis favor the warmer wet season, in clear waters with increased photic depth, which align with previous research on the lake (Havens et al., 1998). They differ in their nutrient requirements, with Dolichospermum benefitting from waters with low DIN and high TRP concentrations whereas Microcystis is ambivalent to TRP concentrations and prefers waters with a high DIN:DIP (Paerl et al., 2016; Chia et al., 2018). Cuspidothrix and Raphidiopsis were more prevalent during the cooler waters of the dry season with low DIN, where Cuspidothrix relative abundance is correlated with higher photic depths and Raphidiopsis is correlated with shallow waters.
Spatially, Dolichospermum relative abundance was highest in the southern region of the lake (i.e., South Lake and Clewiston), away from sites of significant hydrological, and thus external nutrient, inputs. This genus prefers increased SRP concentrations and likely benefits from the internal legacy P that is continuously resuspended and released from the sediments. Furthermore, the lack of an external N load likely behooves Dolichospermum in so far as reducing competition from non-diazotrophic bloom forming genera, specifically Microcystis. Microcystis relative abundances was highest in the northern part of the lake, at the mouth of the Kissimmee River, which is known to have an increased abundance of Microcystis (Havens et al., 1994). Kramer et al. (2018) indicated that increases in N concentrations in Lake Okeechobee promotes non-diazotrophic cyanobacterial abundance in the lake and data from Zhang et al. (2011) show a significant increase in TN concentrations from several point sources in this region of the watershed. The increase in Microcystis abundance in the northern region is likely due to the N rich inputs from the Kissimmee drainage basin via the Kissimmee River, which accounts for ~70% of the inputs into Lake Okeechobee (Zhang J. et al., 2020), as well as the surrounding agricultural and urban inputs. This area has a large drainage basin that is affected by both agricultural and urban runoff, contributing to nutrient inputs (e.g., N & P). Modern fertilizers are comprised of ammonium and urea as their source of nitrogen and their increased use is hypothesized to drive harmful algal blooms, known as the HAB-HB (Harmful Algal Bloom-Haber Bosch) connection (Glibert et al., 2014); additional sources of urea include sewage/septic and livestock runoff. Urea can represent >50% of the dissolved organic nitrogen pool and can be high in agriculturally impacted lakes (Bogard et al., 2012). An increase in urea may give Microcystis a competitive advantage as it is capable of assimilating urea as a source of carbon and nitrogen (Krausfeldt et al., 2019). However, neither total nitrogen nor organic nitrogen were quantified during this study and, to the authors best knowledge, urea concentrations in Lake Okeechobee are unknown. The effects of urea, and other forms of organic nitrogen, on Microcystis abundance in Lake Okeechobee remain unknown and warrants further investigation to better understand bloom drivers in this system.
Considering the lake is an N, or co-nutrient, limited system, nitrogen inputs also likely lead to an increase in N:P. During this study, the northern region was frequently co-nutrient limited, experiencing N-limitation only briefly (Supplementary Figure S1). Together these results, along with results from Kramer et al. (2018), suggest N inputs, both organic and inorganic, from the Kissimmee River can promote increased Microcystis abundance in the northern region of the lake, potentially effectuating Microcystis blooms throughout Lake Okeechobee. Furthermore, there is a need to understand the role of organic N (e.g., urea) within this system to determine how much is coming into the lake and its effect on Microcystis and other bloom forming taxa.
In comparison to the bloom-forming genera, the drivers of the picocyanobacteria are more elusive. Notably, Vulcanococcus relative abundance was positively correlated with water temperatures, and decreased with increased DIN:DIP (Supplementary Figures S11A, S12C), whereas the relative abundance of Cyanobium decreased with increasing TRP (Supplementary Figure S12B). The picocyanobacteria likely dominate the system due to their large surface-to-volume ratio that facilitates nutrient uptake when nutrients are scarce and reduces their light requirements (Havens et al., 1998).
Since the taxonomic resolution of metabarcoding is limited, the potential drivers are for the genera, and are not species specific. A total of six ASVs corresponded to Microcystis (Supplementary Figure S4), which may be several different species of Microcystis. Microcystis species are known to form microcystin-producing blooms within Lake Okeechobee (Kinley-Baird et al., 2021; Pokrzywinski et al., 2022), however both microcystin and non-microcystin producing Microcystis species are known to occur and bloom in Florida (Lefler et al., 2020, 2022, 2023). Furthermore, recent phylogenomic analyses supported several of the morphologically different species of Microcystis, each with various toxigenic potential (Cai et al., 2023). Similarly, several species of Dolichospermum and Raphidiopsis are known to occur in Lake Okeechobee (Cichra et al., 1995; Havens et al., 2003). Due to the diversity of bloom-forming genera within Lake Okeechobee, it is imperative to characterize these taxa and experimentally test how limnological parameters (e.g., N, P, water temperature, etc.) affect their potential to bloom and synthesize various toxins using in-situ, ex-situ, and strain level approaches (e.g., Kramer et al., 2018; Wagner et al., 2021).
Cyanobacterial-bacterial relationships have garnered large interest in the past years (Morris et al., 2011; Cook et al., 2020; Smith et al., 2021), highlighting the importance of these enigmatic relationships. These relationships can be mutualistic (Woodhouse et al., 2016), cyanoHABs can alter the bacterioplankton communities (Berry et al., 2017), and some cyanobacterial genera (e.g., Microcystis) can be dependent on bacteria, and cyanobacteria, within their mucilage (Cook et al., 2020). Relationships between Microcystis and bacteria have been the focus of much research (e.g., Cook et al., 2020; Smith et al., 2022). However, these studies have concentrated on the relationships between Microcystis and the epibiont bacterial communities (e.g., Cook et al., 2020; Smith et al., 2021) or understanding the distinctions between epibiont and pelagic bacterial communities during bloom conditions (e.g., Parveen et al., 2013; Louati et al., 2023). However, temporal associations between cyanobacterial and co-occurring bacterioplankton during non-bloom conditions are rarely investigated. Previous research on Lake Taihu (China), another shallow subtropical lake, indicated that the bacterial community structure changes with the phytoplankton community (Niu et al., 2011).
Overall, the bacterial and cyanobacterial communities changed concurrently, with significant differences between communities in the wet and dry seasons, with neither cyanobacterial nor bacterial communities differing between N or co-limitation. The majority of the associated bacterial taxa belonged to the Proteobacteria (=Pseudomonadota; Figure 3), which was the dominate bacterial phylum, excluding Cyanobacteriota (Supplementary Figures S8C,D). Of the bloom forming genera Cuspidothrix, Dolichospermum, Microcystis, and Raphidiopsis, only Cuspidothrix and Raphidiopsis were correlated. Furthermore, only a single bacterial taxon, an unknown member of the Firmicutes (=Bacillota) was shared between Dolichospermum and Microcystis, this lack of correlated bacteria is supported by results from Louati et al. (2015) (Figure 3). Cuspidothrix and Raphidiopsis both occupied a similar niche within Lake Okeechobee and were correlated with each other but shared no correlated taxa. However, Cuspidothrix and Microcystis occupied distinct niches within Lake Okeechobee, and were not correlated with each other, but were both positively correlated with the cyanobacterial genus Pseudanabaena (Figure 3). This genus is known to occur within the mucilage of Microcystis colonies and may occupy the sheath of Cuspidothrix. Despite the dominance of Cyanobium, this genus was not correlated to the common bloom-forming genera, Cuspidothrix, Dolichospermum, and Raphidiopsis, but was correlated with Microcystis (Figure 3).
Dolichospermum shared correlations with several bacteria (n = 11) across five phyla, compared to Microcystis’ two (Figure 3). Cuspidothrix was also correlated to several bacteria (n = 4) across three phyla. This may indicate that Cuspidothrix and Dolichospermum have coevolved with these bacteria and/or have a symbiotic relationship. Conversely, Raphidiopsis was only positively correlated to a single bacteria genus, Rubellimicrobium, a member of the Pseudomonadota, and may not be as reliant on bacterial interactions as Dolichospermum. However, more in-depth analyses (e.g., in situ metagenomic and metatranscriptomic studies, laboratory experiments) are needed to better understand these relationships (e.g., Vico et al., 2021; Zuo et al., 2022).
In addition to other cyanobacteria, Microcystis was only positively correlated to Pseudomonadota, with its sole negative correlation with an unknown member of the Desulfobacterota (Figure 3). Proteobacteria are known to co-occur with Microcystis blooms (Parveen et al., 2013; Louati et al., 2023) and are copiotrophic (Simonato et al., 2010). One of the known correlated genera within the Pseudomonadota was Silanimonas. Silanimonas is known to co-exist with Microcystis, and the species S. algicola was isolated from a Microcystis colony (Chun et al., 2017). This species is known to perform nitrate reduction, part of the denitrification process in aerobic systems. However, it remains to be seen if denitrification was occurring, although denitrification is known to occur during cyanoHABs (Chen et al., 2011; Zhang et al., 2017).
In contrast to the bloom-forming genera, the picocyanobacteria (Cyanobium, Lacustricoccus, and Regnicoccus) were correlated with several bacteria and cyanobacteria (Figure 3). Whereas Vulcanococcus possessed few correlated taxa, many of which were negative. Similarly, to the closely related Prochlorococcus, these freshwater picocyanobacteria are likely reliant on co-occurring bacterial taxa (Morris et al., 2012).
Understanding the complex relationships between bacteria and cyanobacteria has the potential to provide valuable insights into functional bacterial traits that may assist cyanobacterial bloom proliferations (Louati et al., 2023). Due to the limitations of these data (i.e., 16S rRNA), those potential functional roles of the co-occurring pelagic bacterial taxa cannot be assessed; however, future efforts should be mindful of these relationships. Additionally, due to the shallow depth and polymictic nature of Lake Okeechobee, we cannot rule out if some of these bacterial taxa are particle-associated, and resuspended sediment particles. Furthermore, this study was limited to the bacterial community, and relationships with protists and other microalgae were not studied and may help further elucidate these dynamics. These data do, however, highlight an important observation that within the same water body, disparate bloom-forming cyanobacteria co-occur with dissimilar associated bacterial taxa.
These data highlight the cyanobacterial diversity within Lake Okeechobee, confirming the presence of many genera found from previous morphological assessments of the cyanobacteria, as well as highlighting potentially novel cyanobacteria. Cyanobium dominates the cyanobacterial communities, with Cuspidothrix, Dolichospermum, Microcystis, Raphidiopsis, and Vulcanococcus as the most abundant described genera. There were no differences between cyanobacterial nor bacterial communities during N or co-nutrient limitation. The cyanobacterial and bacterial communities significantly differ between wet and dry season, with a significant increase in Raphidiopsis in the dry season, although both seasons showed dominance of the picocyanobacteria. Cuspidothrix, Dolichospermum, Microcystis, and Raphidiopsis were the most commonly occurring bloom-forming taxa and possessed contrasting environmental drivers and microbial communities. Overall, these three bloom-forming genera have distinct abiotic drivers within Lake Okeechobee. Both Dolichospermum and Microcystis prefer the warmer wet season, in waters with increased photic depth. Our results, along with others (i.e., Havens et al., 1994; Kramer et al., 2018), suggest N inputs, both organic and inorganic, from the Kissimmee River can promote Microcystis abundance in the northern region of the lake, potentially effectuating Microcystis blooms in Lake Okeechobee. Furthermore, there is a need to understand the effects of organic forms of N (e.g., urea) on Microcystis in this system to elucidate whether it’s a species of nitrogen (e.g., NO3, urea, etc.) or solely the N:P ratio which may drive these proliferations. Dolichospermum increased abundance in the southern region of the lake, away from external nutrient inputs, may indicate that it benefits from resuspension of legacy P from sediments from the increased weather events associated with the wet season. In addition to their disparate abiotic drivers, these two genera were correlated with distinct bacteria, which may facilitate their ability to form blooms and out-compete one another, or other, cyanobacteria when conditions are right. Cuspidothrix and Raphidiopsis relative abundance increases in the cooler waters of the dry season, with low DIN and TRP concentrations. While these genera are correlated (Figure 3), and have similar nutrient requirements (Figure 9), they differ by lake depth, with Raphidiopsis relative abundance higher in shallow waters. Additionally, Cuspidothrix and Raphidiopsis have distinct correlated bacteria, which may promote dominance of one over the other when abiotic conditions are ideal for both.
These data highlight the variable nutrient requirement and niches these bloom-forming genera occupy within Lake Okeechobee, increasing our understanding of who is blooming when and why. They also highlight the need for dual nutrient control, as increases in both N and P can drive different bloom-forming genera.
Sequences were deposited at the Sequence Read Archive of the National Center for Biotechnology Information (NCBI) and made publicly available under accession number PRJNA967631. R code used for data analysis, including a full list of R packages, is on GitHub (github.com/flefler/LakeOkeechobee_16SrRNA).
FL, DB, and HL contributed to the conception and design of the study and finalized the manuscript. FL, MB, DB, and HL collected data. FL, MB, DB, and PZ were responsible for the laboratory data analyses. FL analyzed sequencing data, performed statistical analyses, and wrote the first draft of the manuscript. HL supervised the project and secured funding. FL, MB, DB, PZ, AS, and HL critically reviewed the draft and provided feedback. All authors contributed to the article and approved the submitted version.
The authors acknowledge the University of Florida—IFAS Seed Fund and USDA-NIFA Hatch Project #FLA-FTL-00565697 for financial support.
The authors would like to thank the US Army Corps South Florida Operations Office for logistical support in the field. For PZ, this is contribution #99 from the Rice Rivers Center, VCU.
The authors declare that the research was conducted in the absence of any commercial or financial relationships that could be construed as a potential conflict of interest.
All claims expressed in this article are solely those of the authors and do not necessarily represent those of their affiliated organizations, or those of the publisher, the editors and the reviewers. Any product that may be evaluated in this article, or claim that may be made by its manufacturer, is not guaranteed or endorsed by the publisher.
The Supplementary material for this article can be found online at: https://www.frontiersin.org/articles/10.3389/fmicb.2023.1219261/full#supplementary-material
Almanza, V., Pedreros, P., Laughinghouse, H. D., Félez, J., Parra, O., Azócar, M., et al. (2019). Association between trophic state, watershed use, and blooms of cyanobacteria in south-Central Chile. Limnologica 75, 30–41. doi: 10.1016/j.limno.2018.11.004
APHA (2017). Standard methods for the examination of water and wastewater, 23rd Edn. American Public Health Association; American Water Works Association; Water Environment Federation Washington, DC, USA.
Baird, R., and Bridgewater, L. (2017). Standard methods for the examination of water and wastewater, 23rd Edn. American Water Works Association: Washington, DC, USA.
Beaver, J. R., Casamatta, D. A., East, T. L., Havens, K. E., Rodusky, A. J., James, R. T., et al. (2013). Extreme weather events influence the phytoplankton community structure in a large lowland subtropical lake (Lake Okeechobee, Florida, USA). Hydrobiologia 709, 213–226. doi: 10.1007/s10750-013-1451-7
Bell, W., and Mitchell, R. (1972). Chemotactic and growth responses of marine bacteria to algal extracellular products. Biol. Bull. 143, 265–277. doi: 10.2307/1540052
Berry, M. A., White, J. D., Davis, T. W., Jain, S., Johengen, T. H., Dick, G. J., et al. (2017). Are Oligotypes meaningful ecological and phylogenetic units? A case study of Microcystis in Freshwater Lakes. Front. Microbiol. 8:365. doi: 10.3389/fmicb.2017.00365
Berthold, D. E., Lefler, F. W., Huang, I.-S., Abdulla, H., Zimba, P. V., and Laughinghouse, H. D. (2021). Iningainema tapete sp. nov. (Scytonemataceae, Cyanobacteria) from greenhouses in central Florida (USA) produces two types of nodularin with biosynthetic potential for microcystin-LR and anabaenopeptin production. Harmful Algae 101:101969. doi: 10.1016/j.hal.2020.101969
Bogard, M. J., Donald, D. B., Finlay, K., and Leavitt, P. R. (2012). Distribution and regulation of urea in lakes of Central North America: urea in prairie lakes. Freshw. Biol. 57, 1277–1292. doi: 10.1111/j.1365-2427.2012.02775.x
Bonilla, S., Aguilera, A., Aubriot, L., Huszar, V., Almanza, V., Haakonsson, S., et al. (2023). Nutrients and not temperature are the key drivers for cyanobacterial biomass in the Americas. Harmful Algae 121:102367. doi: 10.1016/j.hal.2022.102367
Cai, H., McLimans, C. J., Beyer, J. E., Krumholz, L. R., and Hambright, K. D. (2023). Microcystis pangenome reveals cryptic diversity within and across morphospecies. Sci. Adv. 9:eadd3783. doi: 10.1126/sciadv.add3783
Callahan, B. J., McMurdie, P. J., Rosen, M. J., Han, A. W., Johnson, A. J. A., and Holmes, S. P. (2016). DADA2: high-resolution sample inference from Illumina amplicon data. Nat. Methods 13, 581–583. doi: 10.1038/nmeth.3869
Canfield, D. E., Bachmann, R. W., and Hoyer, M. V. (2021). Restoration of Lake Okeechobee, Florida: mission impossible? Lake Reserv Manage 37, 95–111. doi: 10.1080/10402381.2020.1839607
Capella-Gutiérrez, S., Silla-Martínez, J. M., and Gabaldón, T. (2009). trimAl: a tool for automated alignment trimming in large-scale phylogenetic analyses. Bioinformatics 25, 1972–1973. doi: 10.1093/bioinformatics/btp348
Chen, J., Bittinger, K., Charlson, E. S., Hoffmann, C., Lewis, J., Wu, G. D., et al. (2012). Associating microbiome composition with environmental covariates using generalized UniFrac distances. Bioinformatics 28, 2106–2113. doi: 10.1093/bioinformatics/bts342
Chen, W., Liu, H., Zhang, Q., and Dai, S. (2011). Effect of nitrite on growth and microcystins production of Microcystis aeruginosa PCC7806. J. Appl. Phycol. 23, 665–671. doi: 10.1007/s10811-010-9558-y
Chen, X., Yang, L., Xiao, L., Miao, A., and Xi, B. (2012). Nitrogen removal by denitrification during cyanobacterial bloom in Lake Taihu. J. Freshwater Ecol. 27, 243–258. doi: 10.1080/02705060.2011.644405
Chia, M. A., Jankowiak, J. G., Kramer, B. J., Goleski, J. A., Huang, I.-S., Zimba, P. V., et al. (2018). Succession and toxicity of Microcystis and anabaena (Dolichospermum) blooms are controlled by nutrient-dependent allelopathic interactions. Harmful Algae 74, 67–77. doi: 10.1016/j.hal.2018.03.002
Chun, S.-J., Cui, Y., Ko, S.-R., Lee, H.-G., Oh, H.-M., and Ahn, C.-Y. (2017). Silanimonas algicola sp. nov., isolated from laboratory culture of a bloom-forming cyanobacterium, Microcystis. Int. J. Syst. Evol. Microbiol. 67, 3274–3278. doi: 10.1099/ijsem.0.002102
Cichra, M. F., Badylak, S., Henderson, N., Rueter, B. H., and Phlips, E. J. (1995). Phytoplankon community structure in the open water zone of a shallow subtropical lake (Lake Okeechobee, Florida, USA). Archiv für Hydrobiologie 45, 157–175.
Cook, K. V., Li, C., Cai, H., Krumholz, L. R., Hambright, K. D., Paerl, H. W., et al. (2020). The global Microcystis interactome. Limnol. Oceanogr. 65:11361. doi: 10.1002/lno.11361
Darriba, D., Posada, D., Kozlov, A. M., Stamatakis, A., Morel, B., and Flouri, T. (2020). ModelTest-NG: A new and scalable tool for the selection of DNA and protein evolutionary models. Mol. Biol. Evol. 37, 291–294. doi: 10.1093/molbev/msz189
Davis, F. E., and Marshall, M. L. (1975). Chemical and biological investigations of Lake Okeechobee January 1973—June 1974 interim Report.
De Cáceres, M., and Legendre, P. (2009). Associations between species and groups of sites: indices and statistical inference. Ecology 90, 3566–3574. doi: 10.1890/08-1823.1
Djurhuus, A., Port, J., Closek, C. J., Yamahara, K. M., Romero-Maraccini, O., Walz, K. R., et al. (2017). Evaluation of filtration and DNA extraction methods for environmental DNA biodiversity assessments across multiple trophic levels. Front. Mar. Sci. 4:314. doi: 10.3389/fmars.2017.00314
Falkowski, P. G., Fenchel, T., and Delong, E. F. (2008). The microbial engines that drive Earth’s biogeochemical cycles. Science 320, 1034–1039. doi: 10.1126/science.1153213
Fisher, M. M., Reddy, K. R., and James, R. T. (2005). Internal nutrient loads from sediments in a shallow, subtropical Lake. Lake Reserv Manage 21, 338–349. doi: 10.1080/07438140509354439
Garcia, S. L., Buck, M., McMahon, K. D., Grossart, H. P., Eiler, A., and Warnecke, F. (2015). Auxotrophy and intrapopulation complementary in the ‘interactome’ of a cultivated freshwater model community. Mol. Ecol. 24, 4449–4459. doi: 10.1111/mec.13319
Gin, K. Y. H., Sim, Z. Y., Goh, K. C., Kok, J. W. K., Te, S. H., Tran, N. H., et al. (2021). Novel cyanotoxin-producing Synechococcus in tropical lakes. Water Res. 192:116828. doi: 10.1016/j.watres.2021.116828
Glibert, P. M., Maranger, R., Sobota, D. J., and Bouwman, L. (2014). The Haber Bosch–harmful algal bloom (HB–HAB) link. Environ. Res. Lett. 9:105001. doi: 10.1088/1748-9326/9/10/105001
Havens, K. E. (1995). Secondary nitrogen limitation in a subtropical lake impacted by non-point source agricultural pollution. Environ. Pollut. 89, 241–246. doi: 10.1016/0269-7491(94)00076-P
Havens, K. E., Hanlon, C., and James, R. T. (1994). Seasonal and spatial variation in algal bloom frequencies in Lake Okeechobee, Florida, U.S.A. Lake Reserv Manage 10, 139–148. doi: 10.1080/07438149409354185
Havens, K. E., James, R. T., East, T. L., and Smith, V. H. (2003). N:P ratios, light limitation, and cyanobacterial dominance in a subtropical lake impacted by non-point source nutrient pollution. Environ. Pollut. 122, 379–390. doi: 10.1016/S0269-7491(02)00304-4
Havens, K. E., Phlips, E. J., Cichra, M. F., and Li, B. (1998). Light availability as a possible regulator of cyanobacteria species composition in a shallow subtropical lake. Freshw. Biol. 39, 547–556. doi: 10.1046/j.1365-2427.1998.00308.x
Havens, K., Paerl, H., Phlips, E., Zhu, M., Beaver, J., and Srifa, A. (2016). Extreme weather events and climate variability provide a lens to how Shallow Lakes may respond to climate change. Water 8:229. doi: 10.3390/w8060229
Huang, I.-S., Pinnell, L. J., Turner, J. W., Abdulla, H., Boyd, L., Linton, E. W., et al. (2020). Preliminary assessment of microbial community structure of wind-tidal flats in the Laguna Madre, Texas, USA. Biology 9:183. doi: 10.3390/biology9080183
Huang, I.-S., and Zimba, P. V. (2019). Cyanobacterial bioactive metabolites—A review of their chemistry and biology. Harmful Algae 86, 139–209. doi: 10.1016/j.hal.2019.05.001
Jackrel, S. L., Yang, J. W., Schmidt, K. C., and Denef, V. J. (2021). Host specificity of microbiome assembly and its fitness effects in phytoplankton. ISME J. 15, 774–788. doi: 10.1038/s41396-020-00812-x
James, R. T., and Pollman, C. D. (2011). Sediment and nutrient management solutions to improve the water quality of Lake Okeechobee. Lake Reserv Manage 27, 28–40. doi: 10.1080/07438141.2010.536618
James, T. R., Chimney, M. J., Sharfstein, B., Engstrom, D. R., Schottler, S. P., East, T., et al. (2008). Hurricane effects on a shallow lake ecosystem, Lake Okeechobee, Florida (USA). Fundam. Appl. Limnol. 172, 273–287. doi: 10.1127/1863-9135/2008/0172-0273
Joyner, B. F. (1974). Chemical and biological conditions of Lake Okeechobee, Florida, 1969-72. Florida Geol Survey. doi: 10.35256/RI71
Katoh, K., and Standley, D. M. (2013). MAFFT multiple sequence alignment software version 7: improvements in performance and usability. Mol. Biol. Evol. 30, 772–780. doi: 10.1093/molbev/mst010
Khomutovska, N., de los Ríos, A., and Jasser, I. (2020). Diversity and colonization strategies of endolithic cyanobacteria in the Cold Mountain desert of Pamir. Microorganisms 9:6. doi: 10.3390/microorganisms9010006
Kinley-Baird, C., Calomeni, A., Berthold, D. E., Lefler, F. W., Barbosa, M., Rodgers, J. H., et al. (2021). Laboratory-scale evaluation of algaecide effectiveness for control of microcystin-producing cyanobacteria from Lake Okeechobee, Florida (USA). Ecotoxicol. Environ. Saf. 207:111233. doi: 10.1016/j.ecoenv.2020.111233
Kozlov, A. M., Darriba, D., Flouri, T., Morel, B., and Stamatakis, A. (2019). RAxML-NG: a fast, scalable and user-friendly tool for maximum likelihood phylogenetic inference. Bioinformatics 35, 4453–4455. doi: 10.1093/bioinformatics/btz305
Kramer, B. J., Davis, T. W., Meyer, K. A., Rosen, B. H., Goleski, J. A., Dick, G. J., et al. (2018). Nitrogen limitation, toxin synthesis potential, and toxicity of cyanobacterial populations in Lake Okeechobee and the St. Lucie River estuary, Florida, during the 2016 state of emergency event. PLoS One 13:e0196278. doi: 10.1371/journal.pone.0196278
Krausfeldt, L. E., Farmer, A. T., Castro Gonzalez, H. F., Zepernick, B. N., Campagna, S. R., and Wilhelm, S. W. (2019). Urea is both a carbon and nitrogen source for Microcystis aeruginosa: tracking 13C incorporation at bloom pH conditions. Front. Microbiol. 10:1064. doi: 10.3389/fmicb.2019.01064
Kurtz, Z. D., Müller, C. L., Miraldi, E. R., Littman, D. R., Blaser, M. J., and Bonneau, R. A. (2015). Sparse and compositionally robust inference of microbial ecological networks. PLoS Comput. Biol. 11:e1004226. doi: 10.1371/journal.pcbi.1004226
Lapointe, B. E., Herren, L. W., and Paule, A. L. (2017). Septic systems contribute to nutrient pollution and harmful algal blooms in the St. Lucie estuary, Southeast Florida, USA. Harmful Algae 70, 1–22. doi: 10.1016/j.hal.2017.09.005
Lefler, F. W., Barbosa, M., Berthold, D. E., and Laughinghouse, H. D. (2020). Genome Sequences of Two Microcystis aeruginosa (Chroococcales, Cyanobacteria) Strains from Florida (United States) with Disparate Toxigenic Potentials. Microbiol Resour Announc 9, e00844–e00820. doi: 10.1128/MRA.00844-20
Lefler, F. W., Berthold, D. E., Barbosa, M., and Laughinghouse, H. D. (2022). The effects of algaecides and herbicides on a nuisance Microcystis wesenbergii-dominated bloom. Water 14:1739. doi: 10.3390/w14111739
Lefler, F. W., Berthold, D. E., and Laughinghouse, H. D. (2023). CyanoSeq: a database of cyanobacterial 16S rRNA sequences with curated taxonomy. J. Phycol. 59, 470–480. doi: 10.1111/jpy.13335
Li, X., and Li, R. (2016). Limnolyngbya circumcreta gen. & comb. nov. (Synechococcales, Cyanobacteria) with three geographical (provincial) genotypes in China. Phycologia 55, 478–491. doi: 10.2216/15-149.1
Li, Y., and Li, D. (2012). Competition between toxic Microcystis aeruginosa and nontoxic Microcystis wesenbergii with anabaena PCC7120. J. Appl. Phycol. 24, 69–78. doi: 10.1007/s10811-010-9648-x
Louati, I., Nunan, N., Tambosco, K., Bernard, C., Humbert, J.-F., and Leloup, J. (2023). The phyto-bacterioplankton couple in a shallow freshwater ecosystem: who leads the dance? Harmful Algae 126:102436. doi: 10.1016/j.hal.2023.102436
Louati, I., Pascault, N., Debroas, D., Bernard, C., Humbert, J.-F., and Leloup, J. (2015). Structural diversity of bacterial communities associated with bloom-forming freshwater cyanobacteria differs according to the cyanobacterial genus. PLoS One 10:e0140614. doi: 10.1371/journal.pone.0140614
MacKeigan, P. W., Garner, R. E., Monchamp, M.-È., Walsh, D. A., Onana, V. E., Kraemer, S. A., et al. (2022). Comparing microscopy and DNA metabarcoding techniques for identifying cyanobacteria assemblages across hundreds of lakes. Harmful Algae 113:102187. doi: 10.1016/j.hal.2022.102187
Ma, C., Li, Z., Mwagona, P. C., Rabbany, A., and Bhadha, J. H. (2022). Spatial and seasonal dynamics of phytoplankton groups and its relationship with environmental variables in Lake Okeechobee, USA. J. Freshwater Ecol. 37, 173–187. doi: 10.1080/02705060.2022.2032852
Marshall, M. L. (1977). Phytoplankton and Primary Productivity Studies in Lake Okeechobee. South florida water management district.
McGregor, G. B., and Sendall, B. C. (2017). Iningainema pulvinus gen nov., sp nov. (Cyanobacteria, Scytonemataceae) a new nodularin producer from Edgbaston Reserve, north-eastern Australia. Harmful Algae 62, 10–19. doi: 10.1016/j.hal.2016.11.021
McMurdie, P. J., and Holmes, S. (2013). Phyloseq: an R package for reproducible interactive analysis and graphics of microbiome census data. PLoS One 8:e61217. doi: 10.1371/journal.pone.0061217
McMurdie, P. J., and Holmes, S. (2014). Waste not, want not: why rarefying microbiome data is inadmissible. PLoS Comput. Biol. 10:e1003531. doi: 10.1371/journal.pcbi.1003531
Minh, B. Q., Schmidt, H. A., Chernomor, O., Schrempf, D., Woodhams, M. D., von Haeseler, A., et al. (2020). IQ-TREE 2: new models and efficient methods for phylogenetic inference in the genomic era. Mol. Biol. Evol. 37, 1530–1534. doi: 10.1093/molbev/msaa015
Moore, P. A., Reddy, K. R., and Fisher, M. M. (1998). Phosphorus flux between sediment and overlying water in Lake Okeechobee, Florida: spatial and temporal variations. J. Environ. Qual. 27, 1428–1439. doi: 10.2134/jeq1998.00472425002700060020x
Morris, J. J., Johnson, Z. I., Szul, M. J., Keller, M., and Zinser, E. R. (2011). Dependence of the cyanobacterium Prochlorococcus on hydrogen peroxide scavenging microbes for growth at the Ocean’s surface. PLoS One 6:e16805. doi: 10.1371/journal.pone.0016805
Morris, J. J., Lenski, R. E., and Zinser, E. R. (2012). The black queen hypothesis: evolution of dependencies through adaptive gene loss. MBio 3, e00036–e00012. doi: 10.1128/mBio.00036-12
Niu, Y., Shen, H., Chen, J., Xie, P., Yang, X., Tao, M., et al. (2011). Phytoplankton community succession shaping bacterioplankton community composition in Lake Taihu, China. Water Res. 45, 4169–4182. doi: 10.1016/j.watres.2011.05.022
Nübel, U., Garcia-Pichel, F., and Muyzer, G. (1997). PCR primers to amplify 16S rRNA genes from cyanobacteria. Appl. Environ. Microbiol. 63, 3327–3332. doi: 10.1128/aem.63.8.3327-3332.1997
O’Neil, J. M., Davis, T. W., Burford, M. A., and Gobler, C. J. (2012). The rise of harmful cyanobacteria blooms: the potential roles of eutrophication and climate change. Harmful Algae 14, 313–334. doi: 10.1016/j.hal.2011.10.027
Oksanen, J., Blanchet, F. G., Friendly, M., Kindt, R., Legendre, P., McGlinn, D., et al. (2019). Vegan: community ecology package. R Package Version 2.5-6.
Otten, T. G., and Paerl, H. W. (2015). Health effects of toxic cyanobacteria in U.S. drinking and recreational waters: our current understanding and proposed direction. Curr Envir Health Rpt 2, 75–84. doi: 10.1007/s40572-014-0041-9
Paerl, H. W., and Otten, T. G. (2013). Harmful cyanobacterial blooms: causes, consequences, and controls. Microb. Ecol. 65, 995–1010. doi: 10.1007/s00248-012-0159-y
Paerl, H. W., and Otten, T. G. (2016). Duelling ‘CyanoHABs’: unravelling the environmental drivers controlling dominance and succession among diazotrophic and non-N 2 -fixing harmful cyanobacteria: environmental drivers of CyanoHABs. Environ. Microbiol. 18, 316–324. doi: 10.1111/1462-2920.13035
Paerl, H. W., Scott, J. T., McCarthy, M. J., Newell, S. E., Gardner, W. S., Havens, K. E., et al. (2016). It takes two to tango: when and where dual nutrient (N & P) reductions are needed to Protect Lakes and downstream ecosystems. Environ. Sci. Technol. 50, 10805–10813. doi: 10.1021/acs.est.6b02575
Parada, A. E., Needham, D. M., and Fuhrman, J. A. (2016). Every base matters: assessing small subunit rRNA primers for marine microbiomes with mock communities, time series and global field samples: primers for marine microbiome studies. Environ. Microbiol. 18, 1403–1414. doi: 10.1111/1462-2920.13023
Parveen, B., Ravet, V., Djediat, C., Mary, I., Quiblier, C., Debroas, D., et al. (2013). Bacterial communities associated with Microcystis colonies differ from free-living communities living in the same ecosystem: bacterial diversity inside Microcystis colonies. Environ. Microbiol. Rep. 5:716. doi: 10.1111/1758-2229.12071
Pessi, I. S., Maalouf, P. D. C., Laughinghouse, H. D., Baurain, D., and Wilmotte, A. (2016). On the use of high-throughput sequencing for the study of cyanobacterial diversity in Antarctic aquatic mats. J. Phycol. 52, 356–368. doi: 10.1111/jpy.12399
Pokrzywinski, K. L., Bishop, W. M., Grasso, C. R., Fernando, B. M., Sperry, B. P., Berthold, D. E., et al. (2022). Evaluation of a peroxide-based algaecide for cyanobacteria control: A mesocosm trial in Lake Okeechobee, FL, USA. Water 14:169. doi: 10.3390/w14020169
Pound, H. L., Martin, R. M., Sheik, C. S., Steffen, M. M., Newell, S. E., Dick, G. J., et al. (2021). Environmental studies of cyanobacterial harmful algal blooms should include interactions with the dynamic microbiome. Environ. Sci. Technol. 55:12776. doi: 10.1021/acs.est.1c04207
Quast, C., Pruesse, E., Yilmaz, P., Gerken, J., Schweer, T., Yarza, P., et al. (2012). The SILVA ribosomal RNA gene database project: improved data processing and web-based tools. Nucleic Acids Res. 41, D590–D596. doi: 10.1093/nar/gks1219
Rajaniemi, P., Komárek, J., Willame, R., Hrouzek, P., Katovská, K., Hoffmann, L., et al. (2005). Taxonomic consequences from the combined molecular and phenotype evaluation of selected anabaena and Aphanizomenon strains. Algol Stud 117, 371–391. doi: 10.1127/1864-1318/2005/0117-0371
R Core Team (2023). R: A language and environment for statistical computing. R Foundation for Statistical Computing, Vienna, Austria.
Reynolds, C. S. (1987). Cyanobacterial water-blooms. Editor, callow J.A. Adv. Bot. Res. 13, 67–143. doi: 10.1016/S0065-2296(08)60341-9
Shan, K., Song, L., Chen, W., Li, L., Liu, L., Wu, Y., et al. (2019). Analysis of environmental drivers influencing interspecific variations and associations among bloom-forming cyanobacteria in large, shallow eutrophic lakes. Harmful Algae 84, 84–94. doi: 10.1016/j.hal.2019.02.002
Shannon, P., Markiel, A., Ozier, O., Baliga, N. S., Wang, J. T., Ramage, D., et al. (2003). Cytoscape: A software environment for integrated models of biomolecular interaction networks. Genome Res. 13, 2498–2504. doi: 10.1101/gr.1239303
Simonato, F., Gómez-Pereira, P. R., Fuchs, B. M., and Amann, R. (2010). Bacterioplankton diversity and community composition in the southern lagoon of Venice. Syst. Appl. Microbiol. 33, 128–138. doi: 10.1016/j.syapm.2009.12.006
Simpson, G. (2023). Gratia: graceful ggplot-based graphics and other functions for GAMs fitted using mgcv. R package version 0.1. Available at: https://gavinsimpson.github.io/gratia/.
Sim, Z. Y., Goh, K. C., He, Y., and Gin, K. Y. H. (2023). Present and future potential role of toxin-producing Synechococcus in the tropical region. Sci. Total Environ. 896:165230. doi: 10.1016/j.scitotenv.2023.165230
Smith, D. J., Berry, M. A., Cory, R. M., Johengen, T. H., Kling, G. W., Davis, T. W., et al. (2022). Heterotrophic bacteria dominate catalase expression during Microcystis blooms. Appl. Environ. Microbiol. 88, e02544–e02521. doi: 10.1128/aem.02544-21
Smith, D. J., Tan, J. Y., Powers, M. A., Lin, X. N., Davis, T. W., and Dick, G. J. (2021). Individual Microcystis colonies harbour distinct bacterial communities that differ by Microcystis oligotype and with time. Environ. Microbiol. 23, 3020–3036. doi: 10.1111/1462-2920.15514
Vico, P., Iriarte, A., Bonilla, S., and Piccini, C. (2021). Metagenomic analysis of Raphidiopsisraciborskii microbiome: beyond the individual. Biodivers Data J. 9:514. doi: 10.3897/BDJ.9.e72514
Wagner, N. D., Quach, E., Buscho, S., Ricciardelli, A., Kannan, A., Naung, S. W., et al. (2021). Nitrogen form, concentration, and micronutrient availability affect microcystin production in cyanobacterial blooms. Harmful Algae 103:102002. doi: 10.1016/j.hal.2021.102002
Wang, Q., Garrity, G. M., Tiedje, J. M., and Cole, J. R. (2007). Naïve Bayesian classifier for rapid assignment of rRNA sequences into the new bacterial taxonomy. Appl. Environ. Microbiol. 73, 5261–5267. doi: 10.1128/AEM.00062-07
Werner, V. R., and Laughinghouse, H. D. (2009). Bloom-forming and other planktonic anabaena (cyanobacteria) morphospecies with twisted trichomes from Rio Grande do Sul state, Brazil. Nova Hedwigia 89, 17–47. doi: 10.1127/0029-5035/2009/0089-0017
Werner, V. R., Laughinghouse, H. D., Fiore, M. F., Santanna, C. L., Hoff, C., de Souza Santos, K. R., et al. (2012). Morphological and molecular studies of Sphaerospermopsis torques-reginae (cyanobacteria, Nostocales) from south American water blooms. Phycologia 51, 228–238. doi: 10.2216/11-32.1
Werner, V. R., Tucci, A., Silva, L. M., Yunes, J. S., Neuhaus, E. B., Berthold, D. E., et al. (2020). Morphological, ecological and toxicological aspects of Raphidiopsis raciborskii (cyanobacteria) in a eutrophic urban subtropical lake in southern Brazil. Iheringia Ser Bot 75, –e2020018. doi: 10.21826/2446-82312020v75e2020018
Woodhouse, J. N., Kinsela, A. S., Collins, R. N., Bowling, L. C., Honeyman, G. L., Holliday, J. K., et al. (2016). Microbial communities reflect temporal changes in cyanobacterial composition in a shallow ephemeral freshwater lake. ISME J. 10, 1337–1351. doi: 10.1038/ismej.2015.218
Wood, S. N. (2011). Fast stable restricted maximum likelihood and marginal likelihood estimation of semiparametric generalized linear models. J. R. Stat. Soc. Series B. Stat. Methodol. 73, 3–36. doi: 10.1111/j.1467-9868.2010.00749.x
Xu, T., Yang, T., Zheng, X., Li, Z., and Qin, Y. (2022). Growth limitation status and its role in interpreting chlorophyll a response in large and shallow lakes: A case study in Lake Okeechobee. J. Environ. Manage. 302:114071. doi: 10.1016/j.jenvman.2021.114071
Zhang, J., Burke, P., Iricanin, N., Hill, S., Gray, S., and Budell, R. (2011). Long-term water quality trends in the Lake Okeechobee watershed, Florida. Crit. Rev. Environ. Sci. Technol. 41, 548–575. doi: 10.1080/10643389.2010.530577
Zhang, J., Welch, Z., and Jones, P. (2020) Chapter 8B: Lake Okeechobee watershed annual Report. 2020 South Florida environmental Report volume I 2020;(8B):1–77.
Zhang, W., Gao, Y., Yi, N., Wang, C., Di, P., and Yan, S. (2017). Variations in abundance and community composition of denitrifying bacteria during a cyanobacterial bloom in a eutrophic shallow lake in China. J. Freshwater Ecol. 32, 467–476. doi: 10.1080/02705060.2017.1323681
Zhang, Y., Zuo, J., Salimova, A., Li, A., Li, L., and Li, D. (2020). Phytoplankton distribution characteristics and its relationship with bacterioplankton in Dianchi Lake. Environ. Sci. Pollut. Res. 27, 40592–40603. doi: 10.1007/s11356-020-10033-6
Keywords: harmful algal blooms, Microcystis, Dolichospermum, eutrophication, picocyanobacteria, metabarcoding, microbiome
Citation: Lefler FW, Barbosa M, Zimba PV, Smyth AR, Berthold DE and Laughinghouse HD (2023) Spatiotemporal diversity and community structure of cyanobacteria and associated bacteria in the large shallow subtropical Lake Okeechobee (Florida, United States). Front. Microbiol. 14:1219261. doi: 10.3389/fmicb.2023.1219261
Received: 08 May 2023; Accepted: 14 August 2023;
Published: 30 August 2023.
Edited by:
Petra M. Visser, University of Amsterdam, NetherlandsReviewed by:
Nico Salmaso, Fondazione Edmund Mach, ItalyCopyright © 2023 Lefler, Barbosa, Zimba, Smyth, Berthold and Laughinghouse. This is an open-access article distributed under the terms of the Creative Commons Attribution License (CC BY). The use, distribution or reproduction in other forums is permitted, provided the original author(s) and the copyright owner(s) are credited and that the original publication in this journal is cited, in accordance with accepted academic practice. No use, distribution or reproduction is permitted which does not comply with these terms.
*Correspondence: H. Dail Laughinghouse, aGxhdWdoaW5naG91c2VAdWZsLmVkdQ==
Disclaimer: All claims expressed in this article are solely those of the authors and do not necessarily represent those of their affiliated organizations, or those of the publisher, the editors and the reviewers. Any product that may be evaluated in this article or claim that may be made by its manufacturer is not guaranteed or endorsed by the publisher.
Research integrity at Frontiers
Learn more about the work of our research integrity team to safeguard the quality of each article we publish.