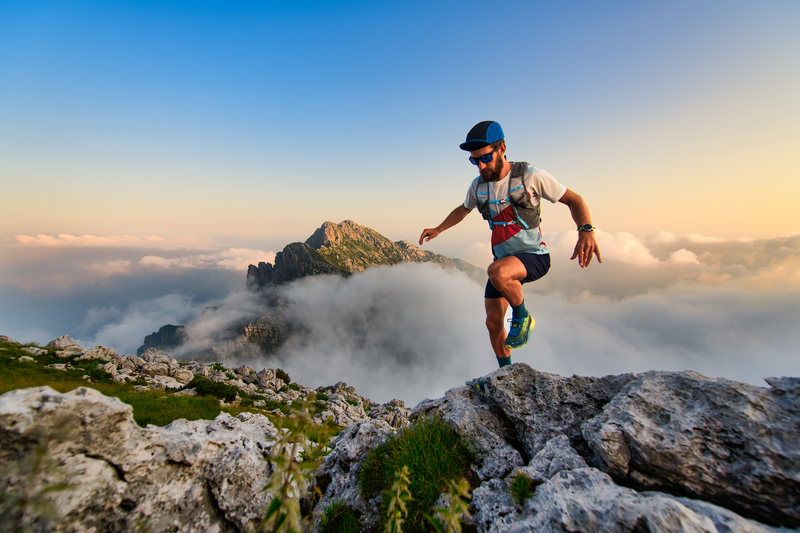
94% of researchers rate our articles as excellent or good
Learn more about the work of our research integrity team to safeguard the quality of each article we publish.
Find out more
ORIGINAL RESEARCH article
Front. Microbiol. , 23 August 2023
Sec. Microorganisms in Vertebrate Digestive Systems
Volume 14 - 2023 | https://doi.org/10.3389/fmicb.2023.1193725
This article is part of the Research Topic Gut Microbiota in Health and Disease View all 19 articles
Background: Observational epidemiological studies suggested an association between the gut microbiota and breast cancer, but it remains unclear whether the gut microbiota causally influences the risk of breast cancer. We employed two-sample Mendelian randomization (MR) analysis to investigate this association.
Methods: We used summary statistics of the gut microbiome from a genome-wide association study (GWAS) of 18,340 individuals in the MiBioGen study. GWAS summary statistics for overall breast cancer risk and hormone receptor subtype-specific analyses were obtained from the UK Biobank and FinnGen databases, totaling 400,000 individuals. The inverse variance-weighted (IVW) MR method was used to examine the causal relationship between the gut microbiome and breast cancer and its subtypes. Sensitivity analyses were conducted using maximum likelihood, MR-Egger, and MR pleiotropic residual sums and outliers methods.
Results: The IVW estimates indicated that an increased abundance of Genus_Sellimonas is causally associated with an increased risk of ER+ breast cancer [odds ratio (OR) = 1.09, p = 1.72E−04, false discovery rate (FDR) = 0.02], whereas an increased abundance of Genus_Adlercreutzia was protective against ER+ breast cancer (OR = 0.88, p = 6.62E−04, FDR = 0.04). For Her2+ breast cancer, an increased abundance of Genus_Ruminococcus2 was associated with a decreased risk (OR = 0.77, p = 4.91E−04, FDR = 0.04), whereas an increased abundance of Genus_Erysipelatoclostridium was associated with an increased risk (OR = 1.25, p = 6.58E−04, FDR = 0.04). No evidence of heterogeneity or horizontal pleiotropy was found.
Conclusion: Our study revealed a gut microbiota–mammary axis, providing important data supporting the potential use of the gut microbiome as a candidate target for breast cancer prevention, diagnosis, and treatment.
Breast cancer is a common malignancy affecting women worldwide, being responsible for an estimated 2 million new cases annually (Sung et al., 2021). Incidence rates are higher in developed countries, which might be attributable to lifestyle and genetic factors (Mubarik et al., 2022). Approximately 5%–10% of breast cancers are related to genetic factors, such as mutations in genes including BRCA1 and BRCA2. Other factors, including diet, exercise, and body weight, have also been linked to the incidence of breast cancer (Brédart et al., 2021).
Although the exact mechanisms of breast cancer development are not fully understood, studies have suggested that the gut microbiota plays a role (Zhu et al., 2018; Teng et al., 2021; Zhang et al., 2021). In particular, fecal transfer experiments and studies of antibiotic use suggest that gut microbiota may be a factor in carcinogenesis (Kovács et al., 2021). Gut bacteria, comprising a community of microbes that reside in the human gut, are closely related to human health (Adak and Khan, 2019; Schoeler and Caesar, 2019). They degrade indigestible food components, releasing nutrients such as vitamins, amino acids, and short-chain fatty acids, and help maintain the balance of the intestinal microbial flora (Mei et al., 2022). However, an imbalance in the gut microbiota, characterized by an overabundance of harmful bacteria and a lack of beneficial bacteria, might contribute to breast cancer development. Certain harmful bacteria can promote inflammation, which is believed to be an important mechanism of tumor formation (Chen et al., 2019; Esposito et al., 2022). Additionally, the gut–mammary pathway, characterized by the transfer of gut bacteria by immune cells to lymph nodes and then to the breasts via blood or lymphatic circulation, has been suggested as a possible mechanism by which gut bacteria influence the development of breast cancer (Rodríguez et al., 2021).
Although the causal relationship between the gut microbiota and breast cancer is not fully understood, observational epidemiological studies have revealed an association (Okubo et al., 2020; Yoon et al., 2021). However, randomized controlled trials investigating the effects of changes in the abundance of intestinal microbes on breast cancer risk have not been conducted. To address this gap, we employed Mendelian randomization (MR), a research method that uses genetic variation as an instrumental variable to assess causality between exposure and outcome (Davey Smith and Ebrahim, 2005), to investigate the causal influence of the gut microbiota on breast cancer development.
In this study, we conducted a rigorous MR analysis that strictly adhered to the three major assumptions of MR analysis (Davies et al., 2018). First, we ensured that the selected genetic variants were associated with the exposure, serving as a predictor of the exposure. Second, we ensured that the genetic variation was independent of any confounding factors, was assigned randomly, and was unaffected by any other factors that could influence the exposure or outcome. Lastly, we ensured that genetic variation did not influence the outcome except through the exposure. A concise summary of the overall study design is presented in Figure 1.
Figure 1. The study design of the present Mendelian randomization study of the associations of the gut microbiota and breast cancer risk.
We obtained summary statistics of gut bacteria from the genome-wide association meta-analysis of the MiBioGen study, which is currently the largest study of transgenic genetics in the human microbiome (Kurilshikov et al., 2021). The study included 18,340 samples of 16S rRNA gene sequencing data from 24 cohorts of European, African, Asian, Middle Eastern, and Hispanic ancestry. In this study, we used seven fecal DNA extraction methods to obtain transgenic taxa data, and we analyzed the microbial composition of samples by targeting three different variable regions of the 16S RNA gene (V1–V2, V3–V4, and V4). All datasets were condensed to 10,000 reads per sample, and we classified the 211 intestinal bacterial taxa into five levels (phylum, class, order, family, and genus) by the direct taxonomic box method. After excluding 15 unnamed bacterial taxa and one duplicate bacterial taxon (Zhang et al., 2022), we selected 195 gut bacterial taxa as exposures for subsequent MR analysis. We selected instrumental variables (IVs) with all-site significance p < 1 × 10−5 and performed clump on all IVs of each gut flora (threshold R2 < 0.01, distance = 500 kb) to reduce the gap between SNPs (Sanna et al., 2019). Linkage disequilibrium (LD) among the IVs was performed to obtain more IVs. LD analysis was performed according to the European 1,000 Genomes Project (Clarke et al., 2012). Subsequently, we harmonized the exposure and outcome data. First, we removed SNPs with inconsistent directions of exposure and outcome alleles. Second, we excluded palindromic A/T or C/G alleles to avoid ambiguous or erroneous results when performing MR analysis. We used F statistic > 10 to ensure that causality was not affected by weak instrumental bias (Burgess and Thompson, 2011). The calculation formula of F statistic is as follows: F = R2 (n − k − 1)/ k (1 − R2), where R2 represents the variance explained by each IV of the gut microbiota, R2 = 2 MAF (1 − MAF) β2, n represents the sample size of the exposure data, k represents the number of IVs, and MAF represents the minor allele frequency.
We obtained breast cancer genome-wide association study (GWAS) data as outcomes from two databases. The genetic influence of cancer risk for overall breast cancer and the estrogen receptor status in the UK Biobank database was obtained from a large GWAS of the Breast Cancer Association Consortium involving 228,951 participants of European ancestry, including 122,977 patients with breast cancer (69,501 ER+ breast cancers, 21,468 ER− breast cancers) and 105,974 controls (Michailidou et al., 2017). The FinnGen database included 14,000 patients with breast cancer and 149,394 controls. The number of patients with HER2− breast cancer was 12,783, and the control group comprised 149,394 subjects. The number of patients with HER2+ breast cancer was 7,729, and the control group comprised 149,279 subjects. A detailed description of the data is available on this website (data available at: https://finngen.gitbook.io/documentation/v/r8/).
In this study, we utilized the inverse variance-weighted (IVW) method as the primary analysis tool to assess the impact of the gut microbiota on breast cancer risk (Burgess et al., 2016). We also conducted sensitivity analyses using the weighted median (WM; Hartwig et al., 2017), MR-Egger regression (Bowden et al., 2015), and MR pleiotropic residual sums and outliers (MR-PRESSO; Verbanck et al., 2018) methods. The WM models yield reliable estimates provided that at least 50% of the weights were derived from valid IVs (Hartwig et al., 2017). Although the MR-Egger method can account for pleiotropic effects, the obtained associations are often imprecise (Bowden et al., 2015). The MR-PRESSO approach can detect pleiotropic outliers for SNPs, and in such instances, MR analysis is repeated after eliminating these SNPs (Verbanck et al., 2018). Cochran’s Q-value was used to evaluate the heterogeneity of causal inference. The intercept test of MR-Egger regression was employed to identify horizontal pleiotropic effects (Bowden et al., 2015). A value of p less than 0.05 suggested the presence of horizontal pleiotropic effects, and thus, we discarded the causal inference. To address multiple hypothesis testing, we used the Benjamini–Hochberg method and controlled for the false discovery rate (FDR; Benjamini and Yekutieli, 2001). A Benjamini–Hochberg-adjusted value of p of <0.05 was considered statistically significant. All MR analyses were conducted using the TwoSampleMR package (Hemani et al., 2018) in R software (version 4.2.1), and the circlize package was used to create circos circle diagrams (Gu et al., 2014).
In total, 195 intestinal flora were identified from the MiBioGen study and categorized into 9 phyla, 15 classes, 20 orders, 32 families, and 119 genera. In this study, we performed MR analysis of three breast cancer datasets from the UK biobank (UKB) database (total breast cancer, ER+ breast cancer, and ER− breast cancer; Supplementary Tables 1–3). Figure 2A presents the impact of changes in the abundance of 195 bacterial taxa on the risk of ER+ breast cancer based on the UKB database. Our findings suggested that an increase in the abundance of Genus_Sellimonas is associated with an elevated risk of ER+ breast cancer (ORIVW = 1.09, PIVW = 1.72E−04, FDRIVW = 0.02; ORWM = 1.08, PWM = 1.01E−02, FDRWM = 0.82; ORMR-Egger = 0.97, PMR-Egger = 0.84, FDRMR-Egger = 1.00). Conversely, an increase in the abundance of Genus_Adlercreutzia was associated with a reduced risk of ER+ breast cancer (ORIVW = 0.88, PIVW = 6.62E−04, FDRIVW = 0.04; ORWM = 0.90, PWM = 4.18E−02, FDRWM = 0.82; ORMR-Egger = 0.98, PMR-Egger = 0.92, FDRMR-Egger = 1.00; Table 1; Figure 3). Although the WM values of the causal inferences for the two gut genera and the risk of ER+ breast cancer were not statistically significant based on our strict FDR threshold control, the findings are consistent with the inferred direction of IVW, indicating our results are highly reliable.
Figure 2. Based on the ER+ breast cancer cohort of the (A) UKB database and the Her2+ breast cancer cohort of the (B) FinnGen database, the causal relationship between intestinal bacteria and these two subtypes of breast cancer was summarized via MR analysis. The three heatmaps from the outermost circle to the inner circle represent the MR methods IVW, WM, and MR–Egger, respectively. The innermost circle represents the OR calculated by the IVW method. MR, Mendelian randomization; IVW, inverse variance-weighted; UKB, UK Biobank; OR, odds ratio; WM, weighted median; BC, breast cancer.
Figure 3. Scatterplot of three Mendelian randomization analysis methods. Causal effects of (A) Genus_Adlercreutzia and (B) Genus_Sellimonas on the risk of ER+ breast cancer.
To deepen our understanding of the potential associations between the gut microbiota and breast cancer, we expanded our analysis to include additional subtypes of breast cancer. Specifically, we examined three distinct subtypes of breast cancer, namely total breast cancer, Her2+ breast cancer, and Her2− breast cancer, using the comprehensive FinnGen database (Supplementary Tables 4–6). Figure 2B presents the effects of changes in the abundance of 195 bacterial taxa on the risk of Her2+ breast cancer using the FinnGen database. Our analysis of this dataset from a diverse population revealed that two specific gut bacteria, Genus_Ruminococcus2 and Genus_Erysipelatoclostridium, were significantly associated with breast cancer risk. We found that an increased abundance of Genus_Ruminococcus2 was linked to a reduced risk of Her2+ breast cancer (ORIVW = 0.77, PIVW = 4.91E−04, FDRIVW = 0.04; ORWM = 0.73, PWM = 1.82E−03, FDRWM = 0.22; ORMR-Egger = 0.70, PMR-Egger = 0.06, FDRMR-Egger = 0.99). Conversely, an increased abundance of Genus_Erysipelatoclostridium was associated with an increased risk of Her2+ breast cancer (ORIVW = 1.25, PIVW = 6.58E−04, FDRIVW = 0.04; ORWM = 1.28, PWM = 6.20E−03, FDRWM = 0.37; ORMR-Egger = 1.12, PMR-Egger = 0.67, FDRMR-Egger = 0.91; Table 2; Figure 4). These observations highlight the potential role of specific gut microbes in the development and progression of certain subtypes of breast cancer.
Figure 4. Scatterplot of three Mendelian randomization analysis methods. Causal effects of (A) Genus_Ruminococcus2 and (B) Genus_Erysipelatoclostridium on the risk of Her2+ breast cancer.
All included variants had an F-statistic greater than 10, indicating the absence of weak instruments (min = 13.38, max = 166.56; Supplementary Table 7). To assess the robustness of the four identified causal estimates that met the FDR control, we performed a series of sensitivity analyses to test the heterogeneity of exposure to outcome. Neither Cochran’s Q test nor MR-Egger revealed heterogeneity, indicating the robustness of our findings. Furthermore, the significance (p < 0.05) of the MR-PRESSO global test indicated the absence of horizontal pleiotropy, with no IVs identified as potential outliers. The intercepts of the MR-Egger regression did not deviate significantly from 0, and all P-values were greater than 0.05, indicating the absence of pleiotropy (Table 3). Leave-one-out sensitivity analysis and funnel plots confirmed the reliability and bias of the causal effects of the four identified associations (Supplementary Figures 1, 2). These results suggest a strong causal link between the identified flora and the corresponding risk of breast cancer, providing further evidence that our findings are reliable.
A recent study found no significant difference in the gut microbiota between Ghanaian women with and without breast cancer (Byrd et al., 2021), and another study revealed that the gut microbial composition of postmenopausal women with breast cancer and benign controls was similar (Aarnoutse et al., 2021). However, Goedert et al. reported inconsistencies in the diversity and specificity of the microbiota in patients with untreated breast cancer and healthy controls, with the former being characterized by elevated counts of Clostridiaceae, Faecalibacterium, and Ruminococcaceae (Goedert et al., 2015). Additionally, Terrisse et al. found that seven bacteria, including Bacteroides uniformis, Clostridium bolteae, and Bilophila wadsworthia, were associated with a worse breast cancer prognosis after comparing healthy human samples (Terrisse et al., 2021). Although the existing data primarily focus on the relationship between the gut microbiome and breast cancer, the causality remains unclear.
To explore the causal relationship between the gut microbiome and breast cancer, we conducted a study using the largest sample size to date, namely the MiBioGen study, which included 195 intestinal flora samples. We performed MR analysis by setting the gut flora as the exposure and the GWAS data of three breast cancers from the UKB and three breast cancers from the FinnGen database as the outcomes. We found that the abundance of two intestinal flora, specifically Genus_Sellimonas and Genus_Erysipelatoclostridium, increased the risk of ER+ breast cancer by 9% and that of Her2+ breast cancer by 25%. Conversely, the abundance of two other flora, Genus_Adlercreutzia and Genus_Ruminococcus2, reduced the risk of ER+ breast cancer by 12% and that of Her2+ breast cancer by 23%. Although the specific effects of the Sellimonas, Erysipelatoclostridium, and Ruminococcus2 flora on breast cancer development are unknown, Adlercreutzia appears to play a role in degrading isoflavones into genistein, which has been revealed to exert tumor-suppressive effects in vivo (Constantinou et al., 1998). Our findings are consistent with those of an animal study in which dietary modification affected the abundance of Adlercreutzia in feces, potentially serving as a biomarker for the efficacy of anticancer dietary supplements (Sharma et al., 2020).
Furthermore, the gut flora can affect hormone levels in the body, particularly estrogen levels, which are closely related to breast cancer development. Certain gut bacteria can boost estrogen synthesis, thereby increasing the risk of breast cancer (Papakonstantinou et al., 2022). The gut flora can also affect the immune system, and an imbalance in the microbiota can weaken the immune system, resulting in an increased risk of breast cancer (Erdman and Poutahidis, 2015). The polymorphic microbiome is recognized as an emerging cancer hallmark, and investigating the interplay between breast tumor tissue and the gut microbiome is particularly interesting and important (Hanahan, 2022). Gut microbiome pathways can further refine breast cancer pathogenesis or complement existing risk stratification algorithms to improve their accuracy. Identifying the characteristics of gut microbes can provide valuable insights for predicting the efficacy and safety of chemotherapy in patients with breast cancer (Guan et al., 2020).
Despite our significant findings, this study had multiple limitations. Our IV selection threshold control was not sufficiently strict to achieve genome-wide statistical significance, which could lead to false-positive results. To address this, we used multiple testing correction via FDR estimation. Additionally, the number of Her2+ breast cancer cases was small, which could limit the statistical power of causal inferences for specific intestinal flora. We also did not differentiate between breast cancers according to molecular types, such as luminal A, luminal B, HER2+/−, and triple-negative breast cancer. Further research is needed to confirm these findings.
In summary, our study adds to the growing body of evidence supporting the existence of a gut microbiome–mammary axis by revealing a causal relationship between four gut microbes and the risk of breast cancer. Our study provides important scientific evidence for the potential use of the gut microbiome as a preventive, diagnostic, and therapeutic tool for breast cancer. However, further research is needed to confirm these findings and investigate the complex interplay between the gut microbiome and breast cancer. The identification of specific gut microbes and pathways involved in breast cancer pathogenesis could lead to the development of novel therapeutic interventions and refinement of existing risk stratification algorithms to improve their accuracy. Additionally, our study highlights the importance of considering the gut microbiome as a modifiable risk factor for breast cancer and underscores the need for further research in this area.
Publicly available datasets were analyzed in this study. This data can be found at: 211 gut bacteria from MiBioGen (data available at: https://mibiogen.gcc.rug.nl/); FinnGen database (https://finngen.gitbook.io/documentation/v/r8/).
All studies for which data were disclosed were approved by the respective ethical review boards and written informed consent was provided by the participants. As this study used published data, no new ethics approval was required.
ZhW designed the study. SZ, WZ, HR, ZiW, and RX collected and analyzed the data. SZ and WZ drew the figures and drafted the early version of the manuscript. QL and WZ supervised the study. All authors contributed to the article and approved the submitted version.
This work was supported by the National Natural Science Foundation of China (No. 82072095), the Technology Research from the Department of Education of Liaoning Province (No. JCZR2020013) and 345 Talent Project of Shengjing hospital of China Medical University (No. M0367).
The authors wish to express our sincere gratitude to the esteemed MiBioGen consortium for their invaluable contribution in publishing the summary statistics of the gut microbiota genome-wide association study. We extend our appreciation to UK Biobank researchers for their generous sharing of these data. Furthermore, we would like to acknowledge the unwavering dedication and efforts of the participants and investigators of the distinguished FinnGen study, whose invaluable contributions have been essential to the advancement of this field of research. We thank Figdraw (www.fgdraw.com) for assistance in making Figure 1. We also thank Joe Barber Jr. from Liwen Bianji (Edanz; www.liwenbianji.cn) for editing the English text of a draft of this manuscript.
The authors declare that the research was conducted in the absence of any commercial or financial relationships that could be construed as a potential conflict of interest.
All claims expressed in this article are solely those of the authors and do not necessarily represent those of their affiliated organizations, or those of the publisher, the editors and the reviewers. Any product that may be evaluated in this article, or claim that may be made by its manufacturer, is not guaranteed or endorsed by the publisher.
The Supplementary material for this article can be found online at: https://www.frontiersin.org/articles/10.3389/fmicb.2023.1193725/full#supplementary-material
Aarnoutse, R., Hillege, L. E., Ziemons, J., De Vos-Geelen, J., de Boer, M., Aerts, E., et al. (2021). Intestinal microbiota in postmenopausal breast Cancer patients and controls. Cancers 13:200. doi: 10.3390/cancers13246200
Adak, A., and Khan, M. R. (2019). An insight into gut microbiota and its functionalities. Cell. Mol. Life Sci. 76, 473–493. doi: 10.1007/s00018-018-2943-4
Benjamini, Y., and Yekutieli, D. (2001). The control of the false discovery rate in multiple testing under dependency. Ann. Stat. 29, 1165–1188. doi: 10.1214/aos/1013699998
Bowden, J., Davey Smith, G., and Burgess, S. (2015). Mendelian randomization with invalid instruments: effect estimation and bias detection through egger regression. Int. J. Epidemiol. 44, 512–525. doi: 10.1093/ije/dyv080
Brédart, A., De Pauw, A., Anota, A., Tüchler, A., Dick, J., Müller, A., et al. (2021). Information needs on breast cancer genetic and non-genetic risk factors in relatives of women with a BRCA1/2 or PALB2 pathogenic variant. Breast 60, 38–44. doi: 10.1016/j.breast.2021.08.011
Burgess, S., Dudbridge, F., and Thompson, S. G. (2016). Combining information on multiple instrumental variables in mendelian randomization: comparison of allele score and summarized data methods. Stat. Med. 35, 1880–1906. doi: 10.1002/sim.6835
Burgess, S., and Thompson, S. G. (2011). Avoiding bias from weak instruments in mendelian randomization studies. Int. J. Epidemiol. 40, 755–764. doi: 10.1093/ije/dyr036
Byrd, D. A., Vogtmann, E., Wu, Z., Han, Y., Wan, Y., Clegg-Lamptey, J. N., et al. (2021). Associations of fecal microbial profiles with breast cancer and nonmalignant breast disease in the Ghana breast health study. Int. J. Cancer 148, 2712–2723. doi: 10.1002/ijc.33473
Chen, J., Douglass, J., Prasath, V., Neace, M., Atrchian, S., Manjili, M. H., et al. (2019). The microbiome and breast cancer: a review. Breast Cancer Res. Treat. 178, 493–496. doi: 10.1007/s10549-019-05407-5
Clarke, L., Zheng-Bradley, X., Smith, R., Kulesha, E., Xiao, C., Toneva, I., et al. (2012). The 1000 genomes project: data management and community access. Nat. Methods 9, 459–462. doi: 10.1038/nmeth.1974
Constantinou, A. I., Krygier, A. E., and Mehta, R. R. (1998). Genistein induces maturation of cultured human breast cancer cells and prevents tumor growth in nude mice. Am. J. Clin. Nutr. 68, 1426s–1430s. doi: 10.1093/ajcn/68.6.1426S
Davey Smith, G., and Ebrahim, S. (2005). What can mendelian randomisation tell us about modifiable behavioural and environmental exposures? BMJ 330, 1076–1079. doi: 10.1136/bmj.330.7499.1076
Davies, N. M., Holmes, M. V., and Davey Smith, G. (2018). Reading mendelian randomisation studies: a guide, glossary, and checklist for clinicians. BMJ 362:k601. doi: 10.1136/bmj.k601
Erdman, S. E., and Poutahidis, T. (2015). Gut bacteria and cancer. Biochim. Biophys. Acta 1856, 86–90. doi: 10.1016/j.bbcan.2015.05.007
Esposito, M. V., Fosso, B., Nunziato, M., Casaburi, G., D'Argenio, V., Calabrese, A., et al. (2022). Microbiome composition indicate dysbiosis and lower richness in tumor breast tissues compared to healthy adjacent paired tissue, within the same women. BMC Cancer 22:30. doi: 10.1186/s12885-021-09074-y
Goedert, J. J., Jones, G., Hua, X., Xu, X., Yu, G., Flores, R., et al. (2015). Investigation of the association between the fecal microbiota and breast cancer in postmenopausal women: a population-based case-control pilot study. J. Natl. Cancer Inst. 107:djv147. doi: 10.1093/jnci/djv147
Gu, Z., Gu, L., Eils, R., Schlesner, M., and Brors, B. (2014). Circlize implements and enhances circular visualization in R. Bioinformatics 30, 2811–2812. doi: 10.1093/bioinformatics/btu393
Guan, X., Ma, F., Sun, X., Li, C., Li, L., Liang, F., et al. (2020). Gut microbiota profiling in patients with HER2-negative metastatic breast Cancer receiving metronomic chemotherapy of capecitabine compared to those under conventional dosage. Front. Oncol. 10:902. doi: 10.3389/fonc.2020.00902
Hanahan, D. (2022). Hallmarks of Cancer: new dimensions. Cancer Discov. 12, 31–46. doi: 10.1158/2159-8290.CD-21-1059
Hartwig, F. P., Davey Smith, G., and Bowden, J. (2017). Robust inference in summary data mendelian randomization via the zero modal pleiotropy assumption. Int. J. Epidemiol. 46, 1985–1998. doi: 10.1093/ije/dyx102
Hemani, G., Zheng, J., Elsworth, B., Wade, K. H., Haberland, V., Baird, D., et al. (2018). The MR-base platform supports systematic causal inference across the human phenome. Elife 7:e34408. doi: 10.7554/eLife.34408
Kovács, T., Mikó, E., Ujlaki, G., Yousef, H., Csontos, V., Uray, K., et al. (2021). The involvement of oncobiosis and bacterial metabolite signaling in metastasis formation in breast cancer. Cancer Metastasis Rev. 40, 1223–1249. doi: 10.1007/s10555-021-10013-3
Kurilshikov, A., Medina-Gomez, C., Bacigalupe, R., Radjabzadeh, D., Wang, J., Demirkan, A., et al. (2021). Large-scale association analyses identify host factors influencing human gut microbiome composition. Nat. Genet. 53, 156–165. doi: 10.1038/s41588-020-00763-1
Mei, Z., Yuan, J., and Li, D. (2022). Biological activity of galacto-oligosaccharides: a review. Front. Microbiol. 13:993052. doi: 10.3389/fmicb.2022.993052
Michailidou, K., Lindström, S., Dennis, J., Beesley, J., Hui, S., Kar, S., et al. (2017). Association analysis identifies 65 new breast cancer risk loci. Nature 551, 92–94. doi: 10.1038/nature24284
Mubarik, S., Yu, Y., Wang, F., Malik, S. S., Liu, X., Fawad, M., et al. (2022). Epidemiological and sociodemographic transitions of female breast cancer incidence, death, case fatality and DALYs in 21 world regions and globally, from 1990 to 2017: an age-period-cohort analysis. J. Adv. Res. 37, 185–196. doi: 10.1016/j.jare.2021.07.012
Okubo, R., Kinoshita, T., Katsumata, N., Uezono, Y., Xiao, J., and Matsuoka, Y. J. (2020). Impact of chemotherapy on the association between fear of cancer recurrence and the gut microbiota in breast cancer survivors. Brain Behav. Immun. 85, 186–191. doi: 10.1016/j.bbi.2019.02.025
Papakonstantinou, A., Nuciforo, P., Borrell, M., Zamora, E., Pimentel, I., Saura, C., et al. (2022). The conundrum of breast cancer and microbiome—a comprehensive review of the current evidence. Cancer Treat. Rev. 111:102470. doi: 10.1016/j.ctrv.2022.102470
Rodríguez, J. M., Fernández, L., and Verhasselt, V. (2021). The gut–breast Axis: programming health for life. Nutrients 13:606. doi: 10.3390/nu13020606
Sanna, S., van Zuydam, N. R., Mahajan, A., Kurilshikov, A., Vich Vila, A., Võsa, U., et al. (2019). Causal relationships among the gut microbiome, short-chain fatty acids and metabolic diseases. Nat. Genet. 51, 600–605. doi: 10.1038/s41588-019-0350-x
Schoeler, M., and Caesar, R. (2019). Dietary lipids, gut microbiota and lipid metabolism. Rev. Endocr. Metab. Disord. 20, 461–472. doi: 10.1007/s11154-019-09512-0
Sharma, M., Arora, I., Stoll, M. L., Li, Y., Morrow, C. D., Barnes, S., et al. (2020). Nutritional combinatorial impact on the gut microbiota and plasma short-chain fatty acids levels in the prevention of mammary cancer in Her2/neu estrogen receptor-negative transgenic mice. PLoS One 15:e0234893. doi: 10.1371/journal.pone.0234893
Sung, H., Ferlay, J., Siegel, R. L., Laversanne, M., Soerjomataram, I., Jemal, A., et al. (2021). Global Cancer statistics 2020: GLOBOCAN estimates of incidence and mortality worldwide for 36 cancers in 185 countries. CA Cancer J. Clin. 71, 209–249. doi: 10.3322/caac.21660
Teng, N. M. Y., Price, C. A., McKee, A. M., Hall, L. J., and Robinson, S. D. (2021). Exploring the impact of gut microbiota and diet on breast cancer risk and progression. Int. J. Cancer 149, 494–504. doi: 10.1002/ijc.33496
Terrisse, S., Derosa, L., Iebba, V., Ghiringhelli, F., Vaz-Luis, I., Kroemer, G., et al. (2021). Intestinal microbiota influences clinical outcome and side effects of early breast cancer treatment. Cell Death Differ. 28, 2778–2796. doi: 10.1038/s41418-021-00784-1
Verbanck, M., Chen, C. Y., Neale, B., and Do, R. (2018). Detection of widespread horizontal pleiotropy in causal relationships inferred from mendelian randomization between complex traits and diseases. Nat. Genet. 50, 693–698. doi: 10.1038/s41588-018-0099-7
Yoon, L. S., Jacobs, J. P., Hoehner, J., Pereira, A., Gana, J. C., Corvalán, C., et al. (2021). The association between breast density and gut microbiota composition at 2 years post-menarche: a cross-sectional study of adolescents in Santiago, Chile. Front. Cell. Infect. Microbiol. 11:794610. doi: 10.3389/fcimb.2021.794610
Zhang, J., Xia, Y., and Sun, J. (2021). Breast and gut microbiome in health and cancer. Genes Dis 8, 581–589. doi: 10.1016/j.gendis.2020.08.002
Zhang, W., Zhang, S., Zhao, F., Du, J., and Wang, Z. (2022). Causal relationship between gut microbes and cardiovascular protein expression. Front. Cell. Infect. Microbiol. 12:1048519. doi: 10.3389/fcimb.2022.1048519
Keywords: breast cancer, gut microbiota, genus, mendelian randomization, pathogenesis
Citation: Zhang S, Zhang W, Ren H, Xue R, Wang Z, Wang Z and Lv Q (2023) Mendelian randomization analysis revealed a gut microbiota–mammary axis in breast cancer. Front. Microbiol. 14:1193725. doi: 10.3389/fmicb.2023.1193725
Received: 04 April 2023; Accepted: 07 August 2023;
Published: 23 August 2023.
Edited by:
Diogo Alpuim Costa, CUF Oncologia, PortugalReviewed by:
Peter Bai, University of Debrecen, HungaryCopyright © 2023 Zhang, Zhang, Ren, Xue, Wang, Wang and Lv. This is an open-access article distributed under the terms of the Creative Commons Attribution License (CC BY). The use, distribution or reproduction in other forums is permitted, provided the original author(s) and the copyright owner(s) are credited and that the original publication in this journal is cited, in accordance with accepted academic practice. No use, distribution or reproduction is permitted which does not comply with these terms.
*Correspondence: Zhe Wang, d3pfY211QDEyNi5jb20=; Qingjie Lv, bHZxaW5namllMjAyM0AxNjMuY29t
†These authors have contributed equally to this work
Disclaimer: All claims expressed in this article are solely those of the authors and do not necessarily represent those of their affiliated organizations, or those of the publisher, the editors and the reviewers. Any product that may be evaluated in this article or claim that may be made by its manufacturer is not guaranteed or endorsed by the publisher.
Research integrity at Frontiers
Learn more about the work of our research integrity team to safeguard the quality of each article we publish.