- 1Evolutionary Biology, Institut für Biologie, Freie Universität Berlin, Berlin, Germany
- 2Health Research Institute, University of Costa Rica, San José, Costa Rica
- 3Division of Biodeterioration and Reference Organisms (4.1), Department of Materials and the Environment, Federal Institute for Materials Research and Testing (BAM), Berlin, Germany
- 4Institute of Chemistry and Biochemistry, Freie Universität Berlin, Berlin, Germany
- 5School of Science, Engineering, and Environment (SEE), University of Salford, Manchester, United Kingdom
- 6Escuela de Biología, University of Costa Rica, San José, Costa Rica
- 7Small Animal Internal Medicine, Clinic for Small Animals, University of Veterinary Medicine (Vetmeduni), Vienna, Austria
Excessive discharge of quaternary ammonium disinfectants such as benzalkonium chloride (BAC) into aquatic systems can trigger several physiological responses in environmental microorganisms. In this study, we isolated a less-susceptible strain of Aeromonas hydrophila to BAC, designated as INISA09, from a wastewater treatment plant in Costa Rica. We characterized its phenotypic response upon exposure to three different concentrations of BAC and characterized mechanisms related to its resistance using genomic and proteomic approaches. The genome of the strain, mapped against 52 different sequenced A. hydrophila strains, consists of approximately 4.6 Mb with 4,273 genes. We found a massive genome rearrangement and thousands of missense mutations compared to the reference strain A. hydrophila ATCC 7966. We identified 15,762 missense mutations mainly associated with transport, antimicrobial resistance, and outer membrane proteins. In addition, a quantitative proteomic analysis revealed a significant upregulation of several efflux pumps and the downregulation of porins when the strain was exposed to three BAC concentrations. Other genes related to membrane fatty acid metabolism and redox metabolic reactions also showed an altered expression. Our findings indicate that the response of A. hydrophila INISA09 to BAC primarily occurs at the envelop level, which is the primary target of BAC. Our study elucidates the mechanisms of antimicrobial susceptibility in aquatic environments against a widely used disinfectant and will help better understand how bacteria can adapt to biocide pollution. To our knowledge, this is the first study addressing the resistance to BAC in an environmental A. hydrophila isolate. We propose that this bacterial species could also serve as a new model to study antimicrobial pollution in aquatic environments.
1. Introduction
Antimicrobial agents used for disinfection in domestic, industrial, and medical scenarios are deposited in wastewater and can be released into the environment after treatment (Tezel and Pavlostathis, 2015). One of the most used disinfectants is the quaternary ammonium compound benzalkonium chloride (BAC), a cationic surfactant with biocidal properties against viruses, bacteria, and some fungi, including yeast. BAC has homologs with varying alkyl chain lengths and is used in large quantities, with over 450,000 kg manufactured or imported to the United States annually. As a result, the American Environmental Protection Agency (EPA) has placed BAC on its High Production Volume List (Barber and Hartmann, 2022). During the COVID-19 pandemic, the consumption of quaternary ammonium compound (QAC) products containing BAC increased significantly due to their widespread use. Remarkably, in the last few years, the mass load of QAC compounds in wastewater increased by 331% compared to pre-pandemic levels (Mohapatra et al., 2023).
BAC can remain in biosolids, such as activated sludge, for extended periods because a significant proportion of it gets integrated into the biomass, with nearly 95% of the compound adsorbed in just a few hours (Zhang et al., 2011). Furthermore, BAC degradation in solids is approximately 20 times slower than in the liquid phase, exacerbating the situation (Zhang et al., 2011; Barber and Hartmann, 2022). Previous studies have demonstrated that BAC is present in digested and sewage sludges used for fertilization purposes as land biosolids (Östman et al., 2017; U.S. Environmental Protection Agency, 2018). The biocidal effect of BAC begins when it interacts with cell membranes, leading to perturbation of the lipid bilayer and a progressive leakage of cytoplasmic materials outside the cell. BAC can also bind to anionic sites on surface membranes and induce osmoregulatory losses. BAC affects bacteria's respiration, solute transport, and cell wall biosynthesis at high concentrations. One common mechanism of microbial death is the solubilization of the cell membrane, which leads to the release of cellular contents into the extracellular space (Gilbert and Moore, 2005). Although QACs are commonly associated with damaging cell membranes, recent research suggests that their effects may extend beyond the surface to intracellular targets (Knauf et al., 2018). To survive under BAC exposure, some intrinsic responses have been described in Gram-negative bacteria, including structural cell membrane modification, increased activity of efflux pumps to extrude biocides, biofilm formation, and formation of persister cells. Some of these adaptations can be related to cross-tolerance to other types of QAC and antibiotics (Mohapatra et al., 2023).
The most common bacterial models used to study the development of resistance to BAC are reference strains of Pseudomonas aeruginosa (Loughlin et al., 2002; Edirsana et al., 2014; El-Banna et al., 2019), isolates from food of Listeria monocytogenes (Romanova et al., 2006; Conficoni et al., 2016; Yu et al., 2018), and other clinically relevant isolates (Mahzounieh et al., 2014; Worthing et al., 2018; Abdelaziz et al., 2019). However, it is recognized that antimicrobial resistance originates in the environment, where waters, soil, and other sites can provide an unmatched gene pool with a great diversity that can exceed that of the animal and human microbiota. Consequently, antimicrobial pollution contributes to the mutation-based evolution of resistance (Larsson and Flach, 2022). For this reason, it is necessary to address the resistance profile and response to antibiotics and biocides to understand their impact on environmentally-relevant bacterial species. Hypothesis-independent OMIC approaches allow the inference of the molecular mechanisms underlying antimicrobial resistance in such environmental bacteria without building these strains into elaborate model systems.
During a microbiological sampling campaign at a wastewater treatment plant near San José, Costa Rica, we isolated and identified a bacterial environmental isolate as A. hydrophila through sequencing. We designated this strain as A. hydrophila INISA09. This bacterial species belongs to the Phylum Proteobacteria, Class Gammaproteobacteria, Order Aeromonadales, and Family Aeromonadaceae. It is a Gram-negative bacillus positive for oxidase and catalase, a glucose fermenter, and it can reduce nitrates to nitrites. Different strains of this species are ubiquitous and typically presents in aquatic environments (Fernández-Bravo and Figueras, 2020). A. hydrophila's ability to colonize different natural and urban aquatic ecosystems and its capacity as an opportunistic human and animal pathogen makes it a desirable candidate for evaluating the dissemination of antimicrobial resistance (Igbinosa and Okoh, 2012; Karkman et al., 2018).
This study aims to characterize a naturally occurring bacterial isolate, a strain of A. hydrophila named INISA09, from a tropical activated sludge and to understand its response to increasing benzalkonium chloride (BAC) concentrations using a quantitative label-free proteomics approach.
2. Materials and methods
2.1. Bacteria and growth conditions
An A. hydrophila INISA09 strain, isolated from activated sludge in Costa Rica, was used as a bacterial model for all experiments with BAC. The isolate was recovered after an activated sludge exposure to BAC (Chacón et al., 2021) in trypticase soy agar (TSA) (Oxoid®, United States) enriched to 25 mg/L BAC (≥95.0% Fluka 12060 Sigma, United States). The 16S rRNA gene was sequenced to preliminarily identify the bacterial species. LB Lennox medium (Carl Roth, Germany) was used for all experiments. The growing temperature in all experiments was 30°C, and when shaking conditions were required, the shaker incubator was set to 200 rpm.
2.2. Genome sequencing
DNA extraction was conducted with DNeasy Blood & Tissue Kit (Qiagen, USA) using the pre-treatment step for Gram-negative bacteria recommended by the manufacturer. The Illumina NovaSeq platform at the company Novogene (Hong Kong) was used for DNA sequencing. Sequence quality was examined using FastQC (Brown et al., 2017). The removal of low-quality reads and adapters from the paired ends for all sequences was filtered by Trim Galore v. 0.6.6 (Krueger, 2020) using a Phred quality cutoff of 30. Then, human reads were discarded by mapping them against the Genome Reference Consortium Human Build 38 (GRCh38; Schneider et al., 2017) using Bowtie2 v. 2.4.2 (Langmead and Salzberg, 2012) with default parameters. The reads were mapped against 52 different sequenced A. hydrophila strains (Supplementary Table S1) using Bowtie2 with default parameters which were used to remove all potential bacterial contamination. After that, for the de novo assembly, Shovill v. 1.1.0 (Seemann, 2020) was executed using SPAdes v. 3.15.0 (Bankevich et al., 2012) as the main assembler and with default parameters. The resulting contigs were scaffolded against A. hydrophila ATCC 7966, the recommended reference strain by the European Committee on Antimicrobial Susceptibility Testing (EUCAST), and the other strains detailed in Supplementary Table S1, using CSAR (Chen et al., 2018). Duplicated entries were removed using SeqKit v. 2.3.1 (Shen et al., 2016). All resulting scaffolds were annotated with Prokka v.1.14.6 (Seemann, 2014) using the bacterial genetic code for gene and tRNA prediction (--gcode 11), allowing the search of non-coding RNA elements (--rfam), predicting the Gram-negative signal peptides in the CDSs (--gram neg), and complying with the GenBank specifications for the file formatting (--compliant). Fast average nucleotide identity (ANI) (Jain et al., 2018) was used to detect the conservation degree among all A. hydrophila strains mentioned in this study, including A. hydrophila INISA09. ANI analysis was visualized using R v. 4.2.2. (R Development Core Team, 2015) with the libraries ape v. 5.7.1 (Paradis and Schliep, 2019) and phangorn v. 2.11.1 (Schliep, 2011; Schliep et al., 2017) altogether with FigTree v. 1.4.4 (http://tree.bio.ed.ac.uk/software/figtree) for the phylogram, and the library ComplexHeatmap v. 2.14.0 (Gu et al., 2016) for the heatmap. Snippy v. 4.6.0 (Seemann, 2015) was used to detect the conservation degree and genomic variation between A. hydrophila INISA09 and A. hydrophila ATCC 7966. Visualization of the genome was performed using the CGView server (Stothard and Wishart, 2005), with CRISPRCasFinder (Couvin et al., 2018) and CARD (Alcock et al., 2019) modules for the visualization of CRISPR-Cas associated elements and antibiotic resistance genes, respectively. This whole-genome shotgun project has been deposited at DDBJ/ENA/GenBank under the accession JAPKVL000000000. The version described in this study is JAPKVL010000000.1.
2.3. BAC minimum inhibitory concentration (MIC) and dose–Response curve determination
A bacterial inoculum of 106 colony-forming units per milliliter (CFU/mL) was used to start the minimum inhibitory concentration assay (MIC). The MIC was determined with the broth microdilution method (Andrews, 2001) with slight modifications. A volume of 200 μL was used as the total volume per well in 96-well polypropylene microtiter plates (Greiner Bio-One, Germany), and the MIC was assessed after 36 h at 30°C of incubation in a microtiter plate reader (Biotek Epoch 2). The MIC was defined as the lowest concentration inhibiting liquid culture growth. The BAC (Fluka® Analytical, Germany) concentrations tested were 0.7 μg/mL, 7.0 μg/mL, 14.0 μg/mL, 19.0 μg/mL, 23.0 μg/mL, 28.0 μg/mL, 33.0 μg/mL, 38.0 μg/mL, 76.0 μg/mL, and 380.0 μg/mL. For each concentration, seven replicates were tested. Additionally, as control, one well was used to blank for BAC + LB medium turbidity, seven wells were used as growing control (without BAC), and seven wells were used as LB medium turbidity control. Optical density measurements were collected in the plate reader using the software Gen5 3.09. A growth curve analysis was performed using the Growthcurver package for R (Sprouffske and Wagner, 2016). For analyzing differences in growing parameters, a Kruskal–Wallis test was conducted with software R version 4.1.1 (2021-08-10) (R Development Core Team, 2015). For the dose–response curve, a growth rate was calculated from OD600 measurement, which was taken every 10-min interval in LB medium (with and without BAC), using an algorithm described by Swain (Swain et al., 2016) and implemented in Python 3.6. Finally, the time–kill curve to BAC was determined by exposing exponential phase bacteria at a density of ~1x109 CFU/mL to 40.0 μg/mL (data not shown).
2.4. Antibiotic susceptibility profile
Approximately 106 CFU/mL were inoculated onto LB Lennox Agar plates (LB medium, Carl Roth, Germany; plus agar 1.5%), following the disk diffusion method previously described (CLSI, 2018). Filter disks were impregnated with 10 μL of the following antibiotics: ciprofloxacin (1 mg/mL), streptomycin (50 μg/mL), ceftazidime (50 μg/mL), trimethoprim (30 μg/mL), fosfomycin (30 μg/mL), colistin (20 μg/mL), daptomycin (4 μg/mL), rifampicin (62 μg/mL), gentamicin (8 μg/mL), chloramphenicol (30 μg/mL), ampicillin (100 μg/mL), doxycycline (100 mg/mL), amoxicillin (2,048 μg/mL), vancomycin (20 mg/mL), and tetracycline (15 mg/mL). In addition, a MIC estimation was established using E-test strips (BioMérieux®, France) for the following antibiotics: ampicillin, ciprofloxacin, fosfomycin, gentamicin, streptomycin, tobramycin, and ceftazidime.
2.5. Swimming test
An overnight culture was inoculated with a sterile toothpick on swimming plates. The swimming motility plates were prepared with 0.3% agar, 1.0% peptone (Acros Organics, Germany), and 0.5% NaCl (≥99.5% Carl Roth, Germany) (Zorzano et al., 2005).
2.6. Biofilm production test
The assay was conducted in 96-well microtiter dishes made of polypropylene (Greiner Bio One, Germany). Bacterial cells of overnight cultures at 30°C were adjusted to 107 CFU/mL in LB Lennox medium (Carl Roth, Germany). Plates were inoculated with the bacterial suspensions (100 μl per well) and incubated at 30°C for 24 h, 48 h, and 72 h. Next, the top of the plate was washed with water and placed in a new plate with 100 μl of crystal violet (1%) (O'Toole and Kolter, 1998). The plates were incubated for 30 min at room temperature and rinsed thoroughly and repeatedly with water. Finally, the dye was solubilized in acetic acid 35% (100 μL per well). The absorbance was determined at 595 nm. Each result represents 40 independent replicates of the experiment. For analyzing differences in biofilm formation, a Kruskal–Wallis test was conducted using software R version 4.1.1 (2021-08-10) (R Development Core Team, 2015).
2.7. Sample preparation and liquid chromatography–label-free quantification mass spectrometry (LC–LFQ-MS)
A. hydrophila proteomic analysis was carried out on exponential growing cultures treated with different BAC concentrations (9.5 μg/mL, 19 μg/mL, 38 μg/mL) for 30 min at 30°C. The main aim of this experiment was to determine the global response of A. hydrophila INISA09 strain to BAC. Non-treated samples were used as control. Each experiment consisted of six replicates. After exposure, the bacterial cells were pelleted by centrifugation at 4°C, 10,000 x g for 2 min, the supernatant was discharged, and the pellet was kept at −80°C. The bacterial pellets were resuspended in 100 μL of urea denaturing buffer (6 M urea, 2 M thiourea, and 10 mM HEPES, pH 8.0) followed by 8 cycles of freezing–unfreezing. All further sample preparation steps were performed as previously published (Rodríguez-Rojas and Rolff, 2020). After the digestion of proteins into peptides, salts and contaminations (e.g., BAC) were removed before LC-MS analysis by SDB-RPS StageTips (Empore™ 2241) (Rappsilber et al., 2007). After elution from the StageTips, peptides were dried under a vacuum.
Peptides trifluoroacetic acid (TFA) were reconstituted in 30 μL of 0.05% trifluoroacetic acid (TFA), 2% acetonitrile in water, and 1 μL and TFA analyzed by a reversed-phase capillary nano liquid chromatography system (Ultimate 3000, Thermo Scientific) connected to a Q Exactive HF mass spectrometer (Thermo Scientific). Samples were injected and concentrated on a trap column (PepMap100 C18, 3 μm, 100 Å, 75 μm i.d.×2 cm, Thermo Scientific) equilibrated with 0.05% TFA in water. After switching the trap column inline, LC separations were performed on a capillary column (Acclaim PepMap100 C18, 2 μm, 100 Å, 75 μm i.d. ×25 cm, Thermo Scientific) at an eluent flow rate of 300 nL/min. Mobile phase A contained 0.1% formic acid in water, and mobile phase B had 0.1% formic acid in 80% acetonitrile / 20% water. The column was pre-equilibrated with 5% mobile phase B, and peptides were separated using a 5–44% mobile phase B gradient within 35 min. Mass spectra were acquired in a data-dependent mode utilizing a single MS survey scan (m/z 300–1650) with a resolution of 60,000 in the Orbitrap and MS/MS scans of the 15 most intense precursor ions with a resolution of 15,000. HCD fragmentation was performed for all peptide ions with charge states of 2+ to 5+ using normalized collision energy of 27 and an isolation window of 1.4 m/z. The dynamic exclusion time was set to 20 s. Automatic gain control (AGC) was set to 3 × 106 for MS scans using a maximum injection time of 20 ms. For MS2 scans, the AGC target was set to 1 × 105 with a maximum injection time of 25 ms.
2.8. LC-MS data processing and protein quantification
MS and MS/MS raw data were analyzed using the MaxQuant software package (version 2.0.3.0) with the implemented Andromeda peptide search engine (Tyanova et al., 2016a). For the database search, a FASTA formatted protein sequence database of A. hydrophila downloaded from Uniprot (Bateman et al., 2023) (4,121 proteins, taxonomy 380,703, 22 March 2022) was used. Filtering and statistical analysis were performed using Perseus software version 1.6.14 (Tyanova et al., 2016b) (Supplementary Table S3). Only proteins that were identified with LFQ intensity values in at least three out of six replicates (within at least one of the four experimental groups) were used for downstream analysis. Missing values were replaced from a normal distribution (imputation) using the default settings (width 0.3, downshift 1.8). Mean log2-fold differences between BAC-treated vs. non-treated control were calculated in Perseus, using Student's t-tests with permutation-based FDR of 0.05. Proteins are defined as significantly regulated if they express a q-value of < 0.05 (corresponds to the adjusted p-value) and at least a 2-fold change in intensity. The increased or decreased degree of overlapping proteins was represented as Venn diagrams using the online tool Venny (Oliveros, 2007–2015). Finally, the ontology and network of the identified proteins were retrieved with the Uniprot database (Bateman et al., 2023) and the STRING database (Szklarczyk et al., 2019) to obtain their functions and relations.
3. Results and discussion
First, from an activated sludge previously exposed to BAC 10 mg/L (Chacón et al., 2021), a bacterial inoculum was transferred to TSA plates enriched to 25 mg/mL of BAC. After 24 h incubation at 28°C, only less-susceptible bacteria formed colonies. DNA extraction and 16S rRNA gene sequencing were conducted by Macrogen® (South Korea). Of the 20 different identified isolates, eight were identified as A. hydrophila with 99% of identity, while the others were Serratia marcescens (12), Burkholderia cepacia (1), and an unidentified bacteria. A preliminary susceptibility test showed that INISA09 had a low susceptibility to BAC (MIC = 38 μg/mL). Thereafter, we sequenced the bacterial genome of A. hydrophila INISA09 (NZ_JAPKVL000000000.1). The draft genome had approximately a size of 4.6 Mbp, 61.3% GC content, 121 tRNA genes, 14 rRNAs, 1 tmRNA, 50 miscRNAs, and 4,087 CDS. No CRISPR sequences or prophages were present in the genome (Figure 1A). These characteristics are in common with the reference strain A. hydrophila ATCC 7966 (Seshadri et al., 2006), which is recommended by European Committee on Antimicrobial Susceptibility Testing (EUCAST) as a reference strain in antibiotic susceptibility testing, and which was previously reported to be susceptible to BAC (Stratev and Vashin, 2014). The draft genome contained 4,273 genes. An analysis of single-nucleotide polymorphisms (SNPs) showed 106,503 mutations (compared to A. hydrophila ATCC 7966), with 16,099 mutations classified as non-synonymous mutations and 15,762 corresponding to missense variants. Some of these mutations were found in genes associated with transport, antimicrobial resistance, gene expression regulation, motility, and redox activity. The strain A. hydrophila INISA09 underwent a profound genome rearrangement and plasticity compared to other strains (Figures 1B, 2, and Supplementary material S1), probably fine-tuned by thousands of mutations (including compensatory mutations) for better survival in the heavily contaminated environment where the isolate was taken. The changes are so numerous that it is not possible to tackle the significance of each genomic using the classical microbial functional genetic approach. One possibility is that exposure to many different antimicrobials could contribute to accelerating the adaptation of the A. hydrophila INISA09. Many antibiotics increase the mutation rate in bacteria (Rodríguez-Rojas et al., 2013), and we recently showed that BAC also increases the mutation rate in some bacteria, such as Escherichia coli (Schmidt et al., 2022). Thus, this increased mutagenesis is a plausible explanation for the high number of SNPs observed in the genome of the A. hydrophila INISA09.
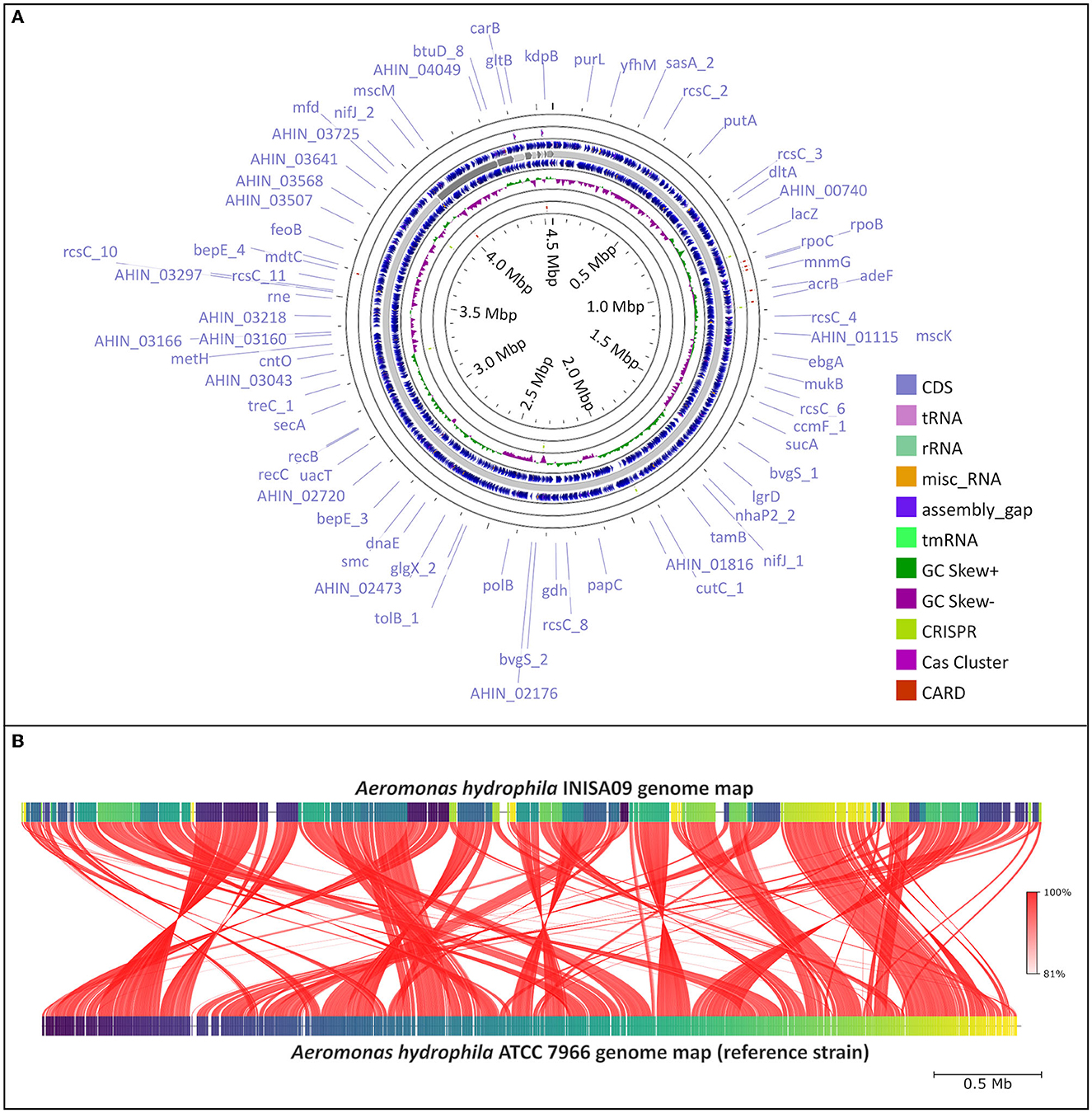
Figure 1. Aeromonas hydrophila INISA09 genome description. (A) Visualization of the genome performed using the CGView server (Stothard and Wishart, 2005), with CRISPRCasFinder (Couvin et al., 2018) and CARD (Alcock et al., 2019) modules for the visualization of CRISPR-Cas associated protein and antibiotic resistance genes, respectively. (B) Average nucleotide identity (ANI) comparison between Aeromonas hydrophila INISA09 and Aeromonas hydrophila ATCC 7966. The red lines represent the reciprocal mapping among both strains indicating the conservation degree.
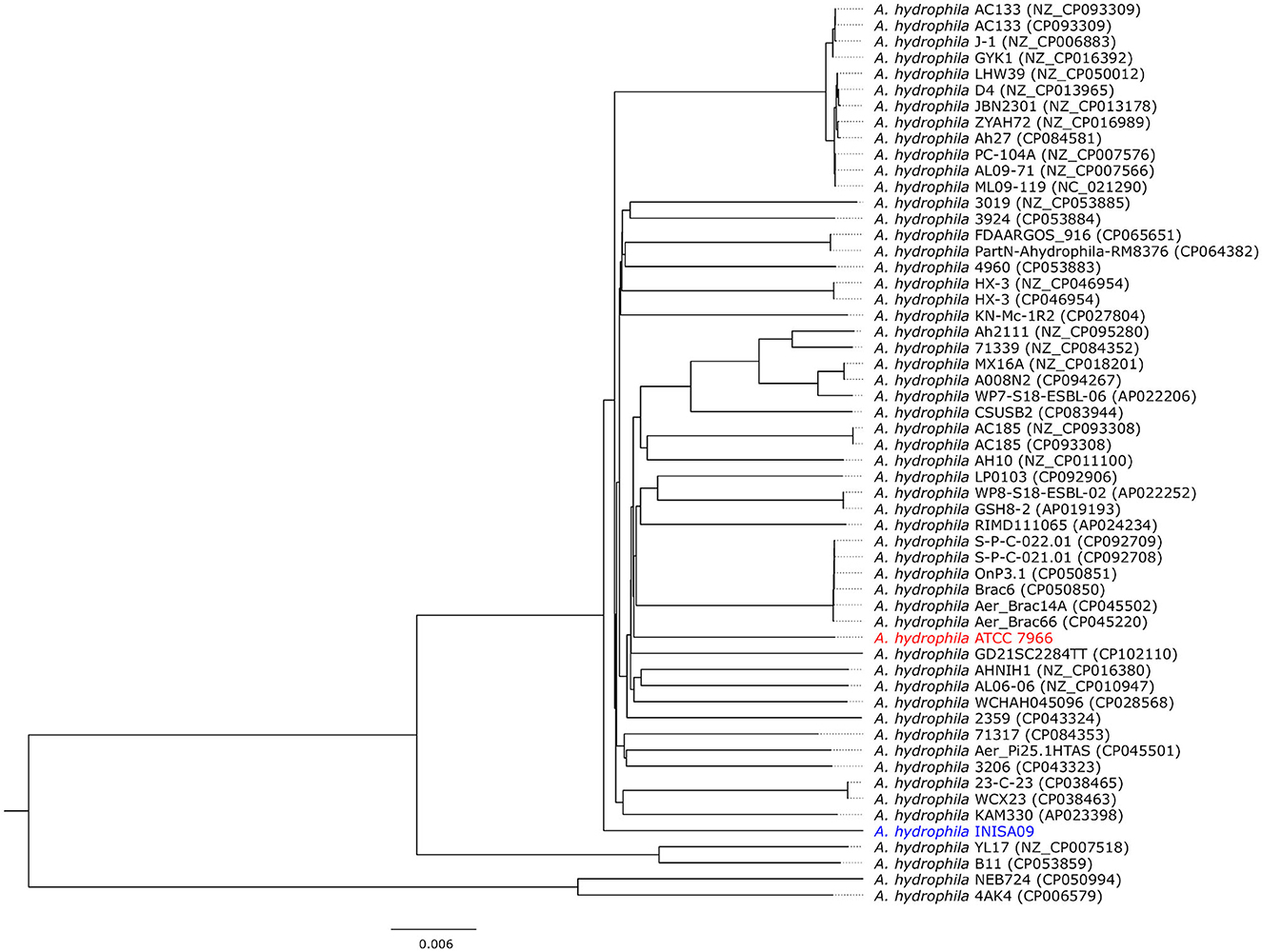
Figure 2. Phylogram representation of the divergence of 54 Aeromonas hydrophila strains. Details about the strains can be found in Supplementary Table S1 and Supplementary Figure S1. The ATCC 7966 (in red) and the INISA09 (in blue) are highlighted in the tree constructed from average nucleotide identity (ANI) comparison value (Jain et al., 2018) and visualized using FigTree v.1.4.4 (http://tree.bio.ed.ac.uk/software/figtree).
Phylogenetic analysis based on the 16S rRNA gene sequences is inappropriate for closely related species or strains. This is because, in closely related species, the variable region of the 16S rRNA gene does not provide sufficient variability to perform phylogenetic analysis accurately (Johnson et al., 2019; Nakano et al., 2022). Therefore, we also used whole-genome phylogeny to compare the divergence of A. hydrophila INISA09 with other 53 A. hydrophila strain genomes. The resulting phylogenetic tree (Figure 2) confirmed that A. hydrophila INISA09 is very dissimilar to at least 90% of the strains, indicating the drastic changes imposed by selective the environmental pressure. In addition, the divergence may include the absence of prophages, which is uncommon for this species (see Supplementary Table S1). During highly stressful conditions, prophages have been observed to be induced at a high frequency (Feiner et al., 2015). This induction can cause the lytic cycle, ultimately resulting in the death of the host. However, in a heavily antimicrobial-polluted environment, genetic variants lacking prophages may be selected for in order to increase the likelihood of survival. In the same vein, it has been observed that during the evolution of E. coli against increasing concentrations of hydrogen peroxide, another biocide, a high number of inactivating mutations were present in the prophage genomes (Rodríguez-Rojas et al., 2020), suggesting a positive selection of prophage-free variants in highly stressful conditions. This wipeout of prophages could have significant consequences for bacterial communities since prophages are essential players in bacterial diversity and ecology (Nadeem and Wahl, 2017).
While exploring the A. hydrophila INISA09 genome, focusing on genes with known roles in antimicrobial resistance, we found mutations in 112 loci associated with transport functions: 33 related to antimicrobial resistance and 33 related to integral membrane composition. In addition, we identified mutations in genes such as bcr (bicyclomycin resistance protein), arnA (bifunctional polymyxin resistance protein ArnA), drrA (daunorubicin/doxorubicin resistance ATP-binding protein DrrA), fsr (fosmidomycin resistance protein), emrB, mdtE, mdtA, mdtH, mdtL, norM, mdlB (all multidrug resistance proteins), and qacC (quaternary ammonium compound-resistance protein QacC). The mutations in genes associated with efflux pumps include acrB, acrA, acrE, and the outer membrane protein tolC (outer membrane protein), which is part of the AcrAB-TolC multidrug efflux pump complex (Tikhonova and Zgurskaya, 2004). These results are consistent with a previous study conducted in Salmonella enterica serovar Typhimurium, showing that the TolC protein connects with the AcrAB efflux pump system to expel BAC to the extracellular space (Guo et al., 2014).
We also found intragenic mutations in genes that code for membrane-related proteins such as the porins ompA (outer membrane protein A) and ompW (outer membrane protein W); both are also related to the adhesion process and biofilm formation (Maiti et al., 2012; Wang et al., 2022). The porins ompD (outer membrane protein D) and ompN (outer membrane protein N) also showed mutations in the INISA09 strain, which might confer low susceptibility to benzalkonium chloride (BAC) or other biocides since cationic surfactants target the outer membrane as the primary site (Gilbert and Moore, 2005). In a previous study in Escherichia coli, some of these genes were differentially expressed under BAC exposure in a transcriptomic assay (Forbes et al., 2019). On the other hand, an in vitro experiment in E. coli showed that BAC could induce tolerance mediated by the genes lpxL and lpxM. These genes code for myristoyl transferases responsible for the last acylation steps in lipid A synthesis (Nordholt et al., 2021). The wild A. hydrophila INISA09 genome presented 18 mutations in this gene; three were non-synonymous mutations: Y21H, M59I, and Y225H. However, these mutations differ from those observed in E. coli: A96E, M148R, L207I, A242E, and W298G.
We analyzed the antibiotic susceptibility of A. hydrophila INISA09 strain by E-test and disk diffusion assays. The MIC estimated by an E-test showed different degrees of susceptibility to seven antibiotics, including ampicillin >256 μg/mL, ciprofloxacin 0.064 μg/mL, fosfomycin 8 μg/mL, gentamycin 1 μg/mL, streptomycin 8 μg/mL, tobramycin 1.5 μg/Ml, and ceftazidime 0.032 μg/mL. A previous study conducted in environmental isolates of Aeromonas sp. (Goñi-Urriza et al., 2000) showed high resistance to penicillins such as ampicillin and increased susceptibility to the third generation of cephalosporins. Our results were similar for the susceptibility of A. hydrophila INISA09 to the third generation of cephalosporins, indicating the absence of extended-spectrum beta-lactamases. The values obtained in comparison with those of Goñi-Urriza et al. (2000) were the same for gentamycin (1 μg/mL) and were lower for streptomycin (16 μg/mL) and third-generation cephalosporins (cefotaxime 8 μg/mL). We highlight that strain INISA09 was highly susceptible to fosfomycin (0.2–8 μg/mL). We have included some antibiotics such as amoxicillin, ampicillin, and vancomycin to investigate whether resistance to BAC caused some collateral sensitivity as observed for resistance to other cationic drugs (Lázár et al., 2018).
The EUCAST (EUCAST, 2022) informed that all clinical isolates of A. hydrophila display intrinsic resistance to ampicillin, amoxicillin, amoxicillin–clavulanic acid, ampicillin–sulbactam, and cefoxitin. A. hydrophila is intrinsically resistant to benzylpenicillin, glycopeptides, lipoglycopeptides, fusidic acid, lincosamides, streptogramins, rifampicin, oxazolidines, and macrolides (except azithromycin). In this case, A. hydrophila INISA09 was only resistant to ampicillin (penicillin) and susceptible to ciprofloxacin (fluoroquinolone), tobramycin, streptomycin, gentamycin (aminoglycosides), ceftazidime (cephalosporin), and fosfomycin (epoxide). It is important to mention that the criteria of the European Center for Disease Prevention and Control (ECDC) define multidrug resistance (MDR) as acquired non-susceptibility to at least one agent in three or more antimicrobial categories (Magiorakos et al., 2012). Table 1 details the antibiotics tested and their classification according to the resistance level recommended by EUCAST for Aeromonas sp. and Vibrio sp. (another phylogenetically related Gammaproteobacterium), and in Supplementary Table S1, we show the tested concentration and inhibition halo diameter.
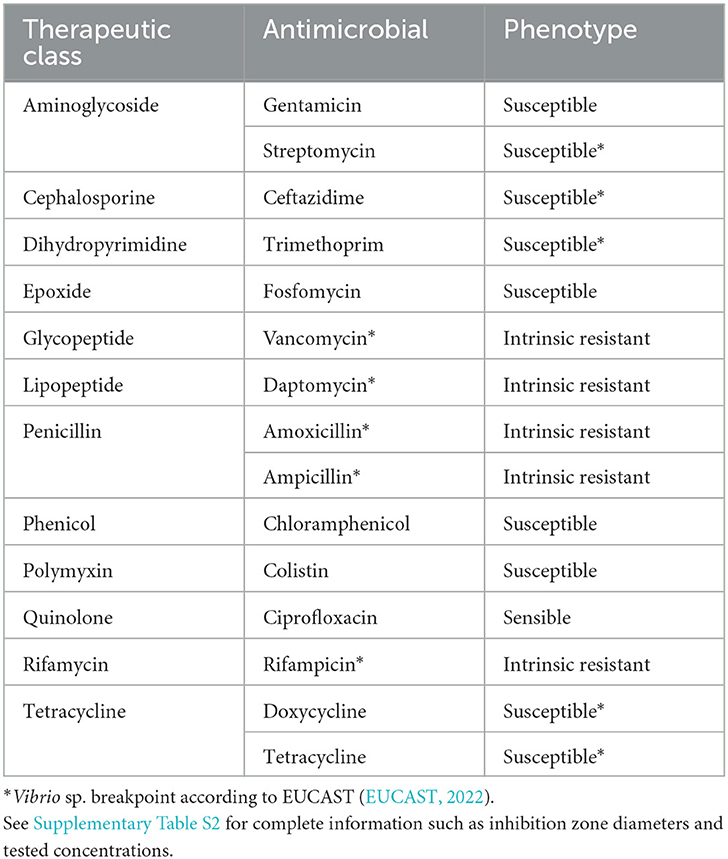
Table 1. Antibiotic susceptibility testing of Aeromonas hydrophila INISA09 determined by the disk diffusion method for different antibiotic families.
We analyzed the effect of different near-MIC BAC concentrations on the growth curve of the INISA09 strain. To this end, we used the Growthcurver R package, which fits growth curve data to the standard form of the logistic equation commonly used in ecology and evolution (Sprouffske and Wagner, 2016), due to its limitations as low fitting in bacteria with very slow or fast doubling time since the first ones can produce high optical densities by extracellular products presence or low optical densities associated with a low growing rate; the parameters estimated are shown in Supplementary Figure S2. To this end, we employed the estimated MIC for BAC (38.0 μg/mL) to evaluate parameters related to growth, such as the growth rate (r-value), the maximum population size or carrying capacity (k-value), the generation time, and the empirical area under the curve (auce) estimated from the obtained optical density (OD600) data. We compared these values with a non-treated control.
In Table 2, we present the results for relevant growth curve parameters (see also Supplementary Figure S2). We found significant statistical differences for the carrying capacity (k, which describes the maximum reachable biomass of the culture, p = 1.5 × 10−7) and the empirical area under the curve parameters (auce, a global parameter that integrates effects on the initial population size, maximum growth rate, and carrying capacity, p = 7.7 × 10−7). In a dose–response curve analysis, the EC50 (half maximal effective concentration) of BAC for INISA09 corresponded to 33.0 μg/mL. Figure 3 shows a typical growth curve behavior of A. hydrophila under three different BAC concentrations: 9.5 μg/mL, 19 μg/mL, and 38 μg/mL, and the average area under the curve obtained. This strain's resistance level is notably higher than that of other bacteria, which were reported to have the following ecological cut-off concentrations based on the MIC-distributions of a total of 3327 microbial isolates: 128 μg/mL for Salmonella spp.; 64 μg/mL for E. coli; 32 μg/mL for K. pneumoniae; 32 μg/mL for Enterobacter spp.; 16 μg/mL for S. aureus; 8 μg/mL for E. faecium; and 8 μg/mL for E. faecalis (Morrissey et al., 2014).
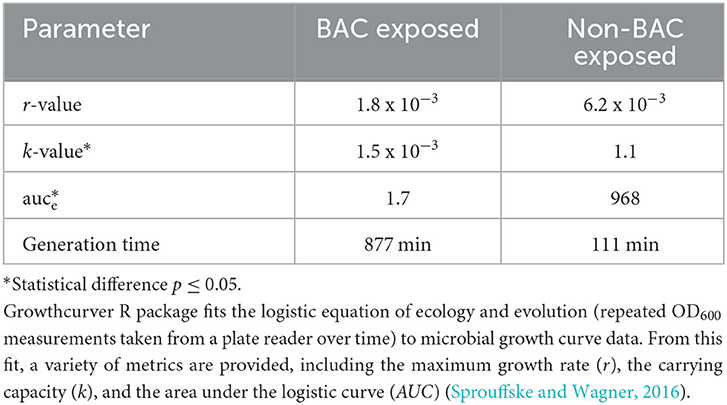
Table 2. Parameters descriptive of growth for Aeromonas hydrophila INISA09 grown in the presence (38 μg/mL) and absence of BAC. See also Supplementary Figure S2.
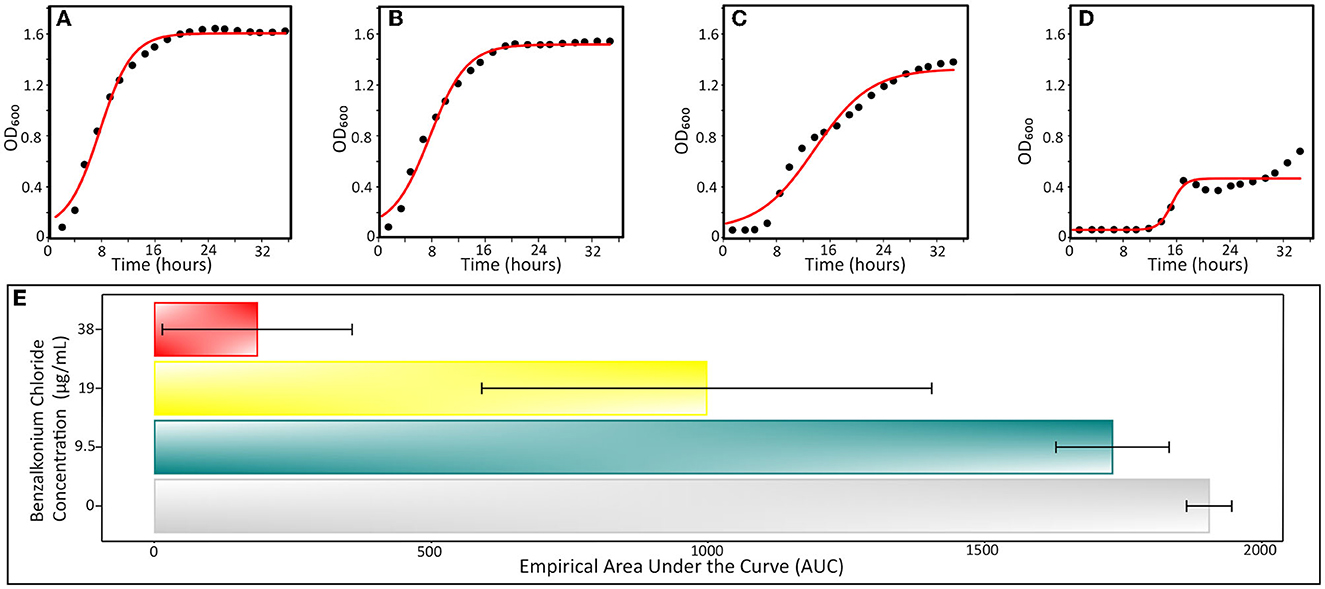
Figure 3. Typical growth curves of Aeromonas hydrophila INISA09 exposed to different BAC concentrations. (A) Non-treated control, (B) BAC 9.5 μg/mL, (C) BAC 19 μg/mL, (D) 38 μg/mL doses; and (E) empirical area under the curve, a global parameter that integrates other parameters such as growth rate, carrying capacity, death phase, and initial population size. The red line represents the logistic regression model obtained from the absorbance data. The empirical AUC was obtained from the experimental absorbance obtained curve from five independent repetitions according to the previously described procedure (Sprouffske and Wagner, 2016).
We also tested bacterial mobility using the swimming motility plate assay (Zorzano et al., 2005). A. hydrophila INISA09 showed a moderate swimming capacity (< 5 cm in diameter of swimming). A biofilm formation assay shows a substantial increase in biofilm mass in the first 48 h (Supplementary Figure S3). The statistical analysis showed differences in biofilm production in the three tested periods (p = 1.1 x 10−3). However, post-hoc analyses indicated that the differences occur among the first 48 h (p = 0.03), whereas the difference between 48 and 72 h is not significant (p = 0.16). This result indicates that INISA 09 biofilms mature within 48 h under experimental conditions.
To elucidate the mechanistic basis for the low susceptibility to BAC of the A. hydrophila INISA09, we performed a label-free quantification liquid chromatography–mass spectrometry proteomic analysis. To this end, we exposed the cells for 30 min to different concentrations of BAC and compared them to the non-treated control. The chosen BAC concentrations were 9.5, 19, and 38 μg/mL, corresponding to a quarter, half, and minimal inhibitory concentration; these experiments were carried out with a higher inoculum (~108 CFU/mL) to have more than enough cells for the protein extraction. We found 111, 379, and 233 proteins with a significant change in relative abundance when the bacteria were treated with ¼ MIC (9.5 μg/mL), ½ MIC (19 μg/mL), and the MIC (38 μg/mL), respectively (Figure 4). Volcano plots representing the differential expression of all quantified proteins are shown in Figure 5. Tables 3, 4 list 15 proteins with the largest fold change in each experiment. The whole dataset is shown in Supplementary Table S3.
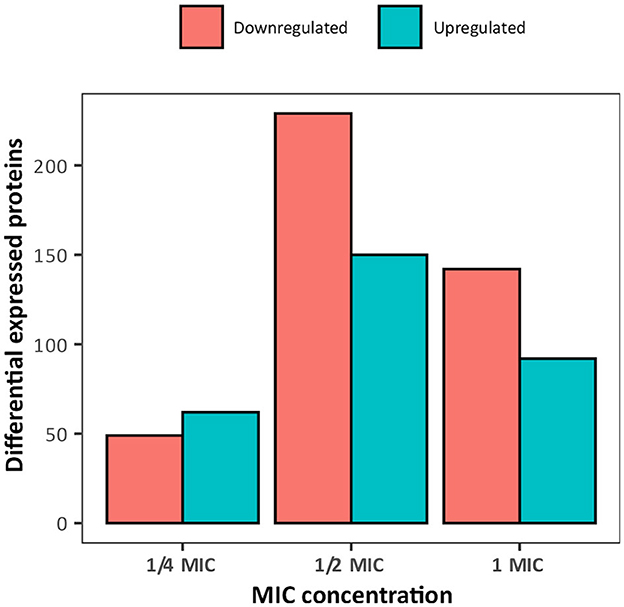
Figure 4. Overview of the number of differentially expressed proteins in Aeromonas hydrophila INSA09 after 30 min of exposure to BAC (¼ MIC: 9.5 μg/mL, ½ MIC: 19 μg/mL, and MIC: 38 μg/mL doses).
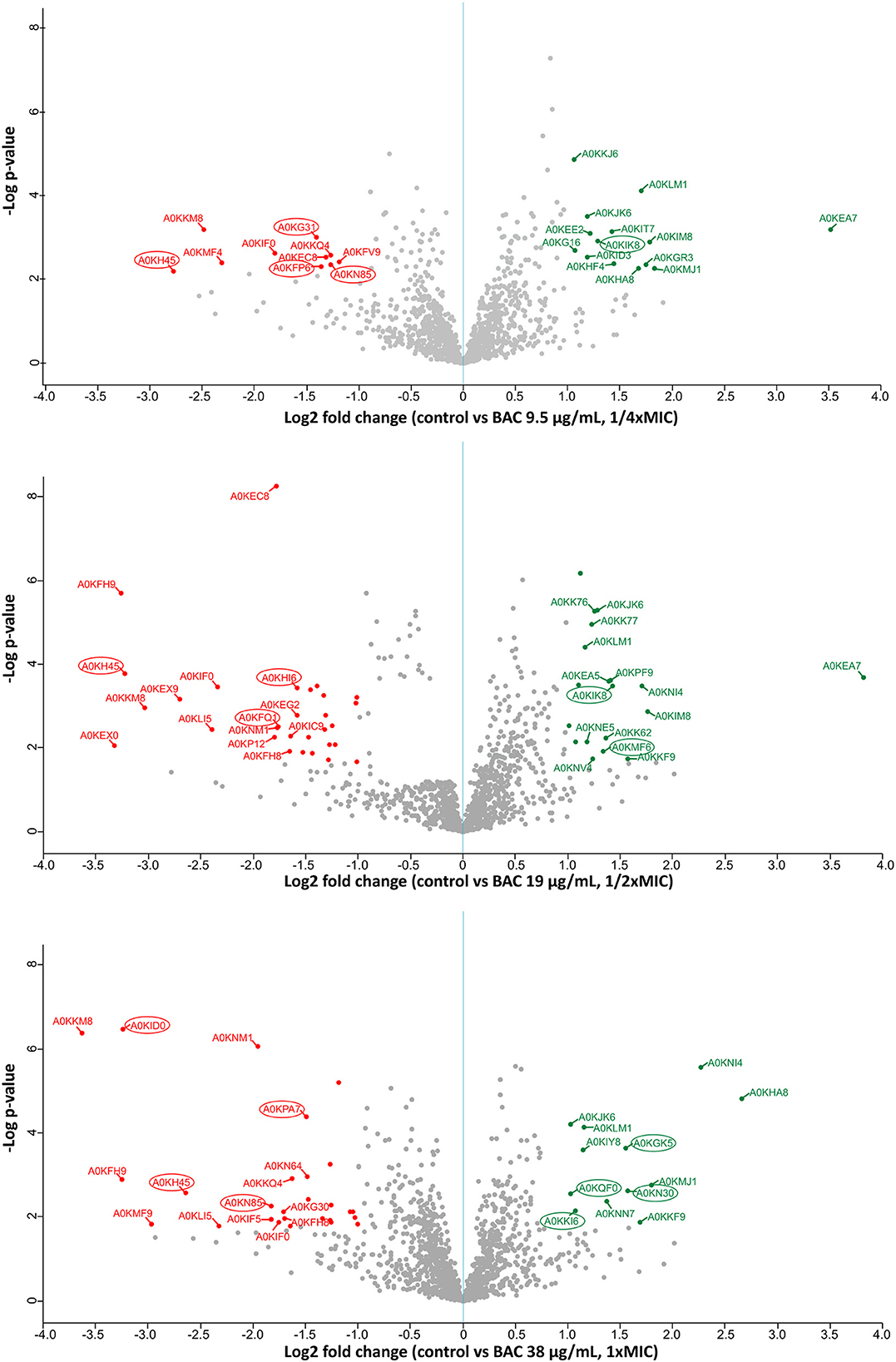
Figure 5. Label-free quantification LC-MS proteomics results. Volcano plot showing the log2 fold change of mean relative protein intensities of Aeromonas hydrophila determined by LC-MS and label-free quantification (BAC-treated vs. non-treated control). Cells have been treated with 9.5; 19; or 38 μg/mL BAC as indicated in the y-axis label. Proteins with significantly higher abundance in non-treated controls are colored in red (downregulated upon BAC treatment), while proteins with significantly higher abundance after BAC treatment are colored in green (upregulated). Proteins are defined as significantly regulated if they express a q-value < 0.05 (FDR adjusted p-value) and at least a 2-fold change in intensity. Proteins marked with a circle correspond to membrane-related proteins, according to the Uniprot database (Bateman et al., 2023).
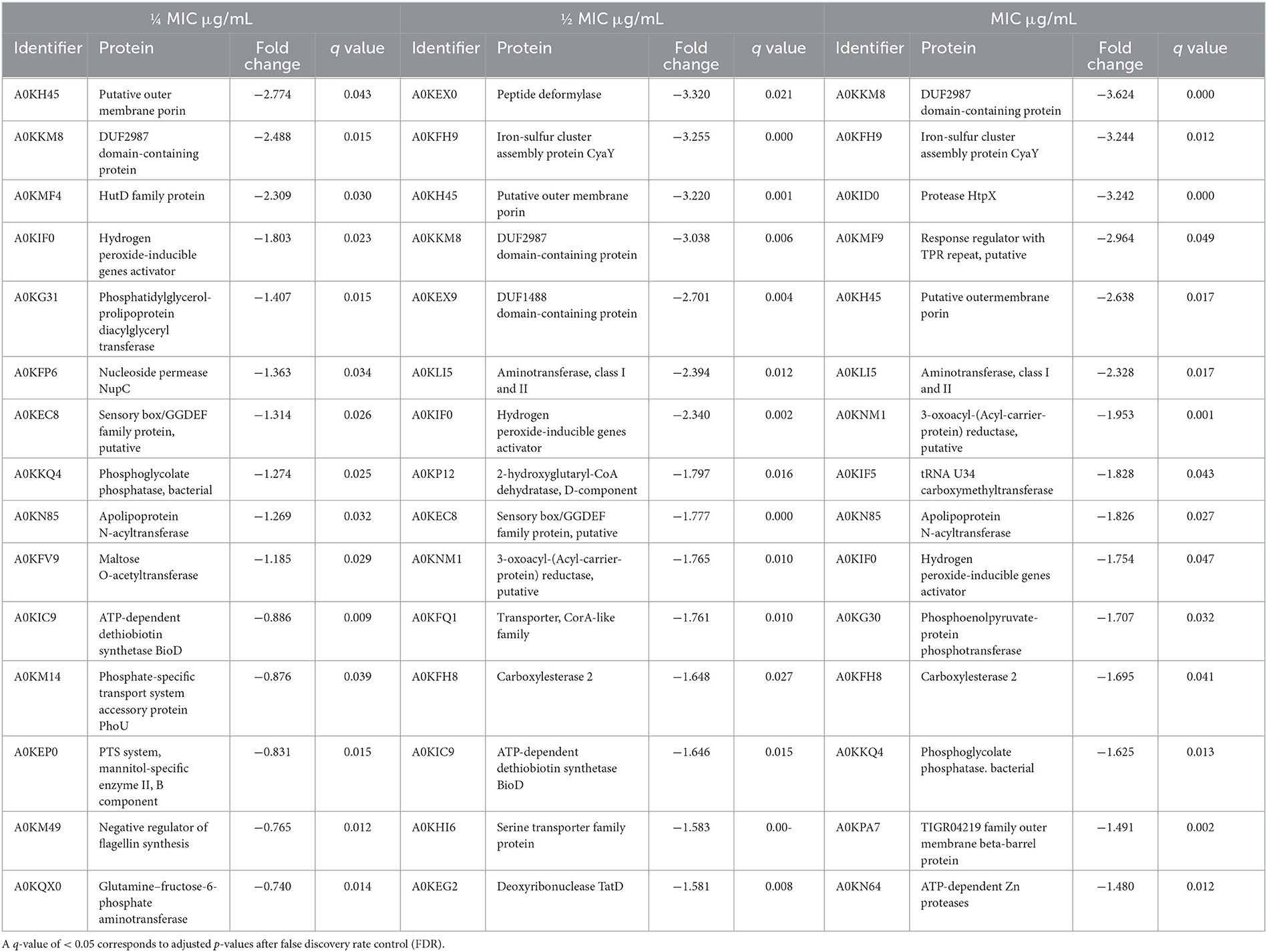
Table 3. Main downregulated proteins, measured by LC-MS using label-free quantification in Aeromonas hydrophila after exposure to three different BAC concentrations.
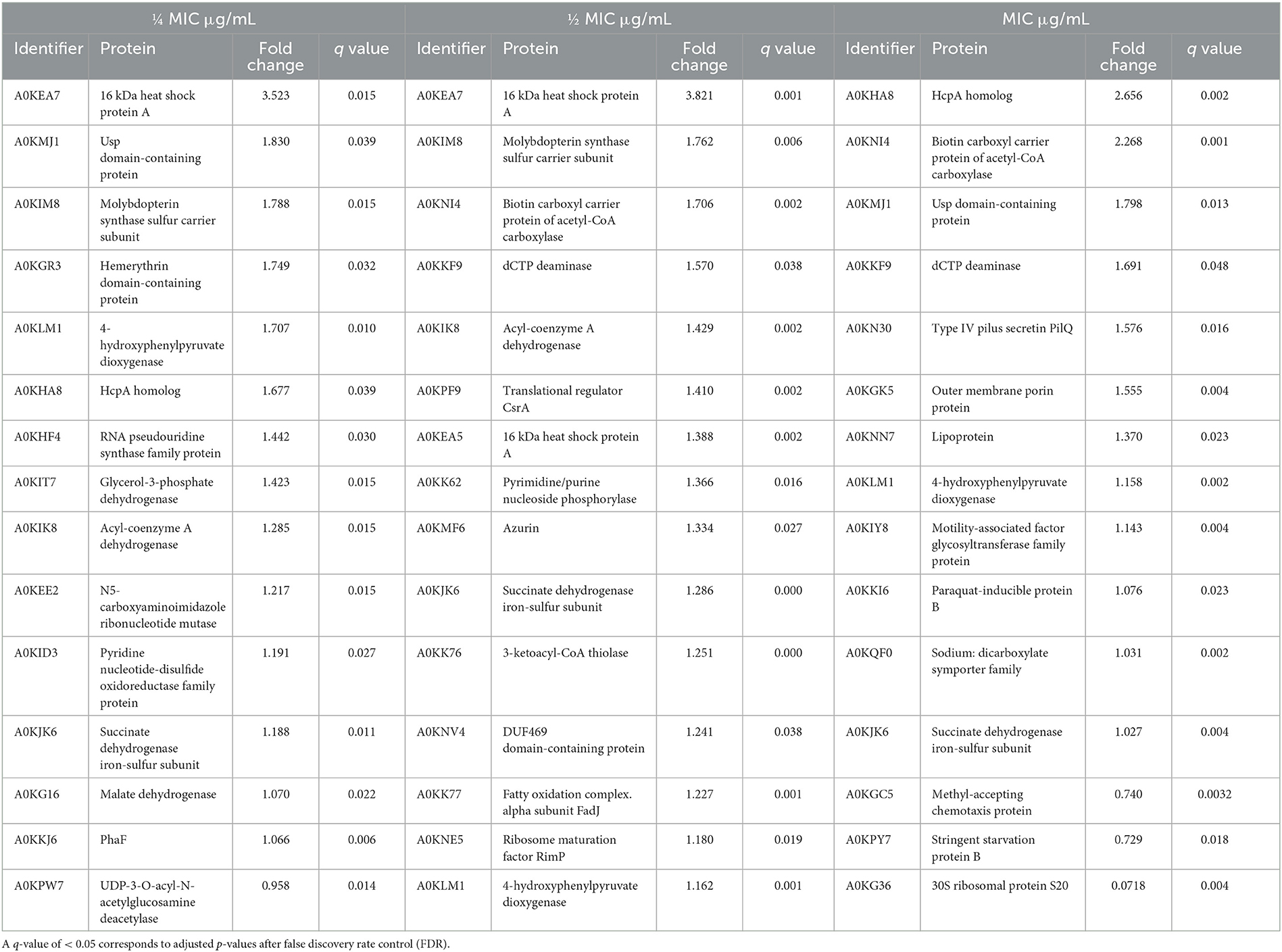
Table 4. Main upregulated proteins, measured by LC-MS using label-free quantification, in Aeromonas hydrophila after exposure to three different BAC concentrations.
In Figure 6, we show a Venn diagram illustrating the common core set of protein expressions and the dissimilarities among the three used BAC concentrations. A total of 21 downregulated proteins upon BAC treatment consistently coincide across all three conditions. These include five envelope-related proteins, including the membrane proteins A0KH45, A0KHA5, and A0KI39, the cell wall degradation protein A0KHL2, and a carbohydrate-associated transporter A0KEP0. Two of these proteins are related to different stress responses: A0KIF0 is an activator of hydrogen peroxide-inducible genes, and A0KM49 is a negative regulator of flagellin synthesis. Another 12 expressed genes are responsible for metabolic pathways, such as nucleic acid and fatty acid metabolism. One is a disulfide-reductase enzyme (A0KEH0), and the other is an undetermined function protein (DUF-domain protein) (A0KKM8). In addition, the upregulated proteins include six enzymes associated with metabolic processes (A0KN18, A0KLM1, A0KP19, A0KPW7, and A0KQG0), two membrane-related proteins (A0KHC8 and A0KKM0, both with oxidoreductase activity associated with electron transport), and three proteins with binding functions (A0KKE2 to ribosomes, and A0KIA0 and A0KJK6 to ion reductase enzymes). The last one, A0KM63, is a DUF-domain protein with an unknown function.
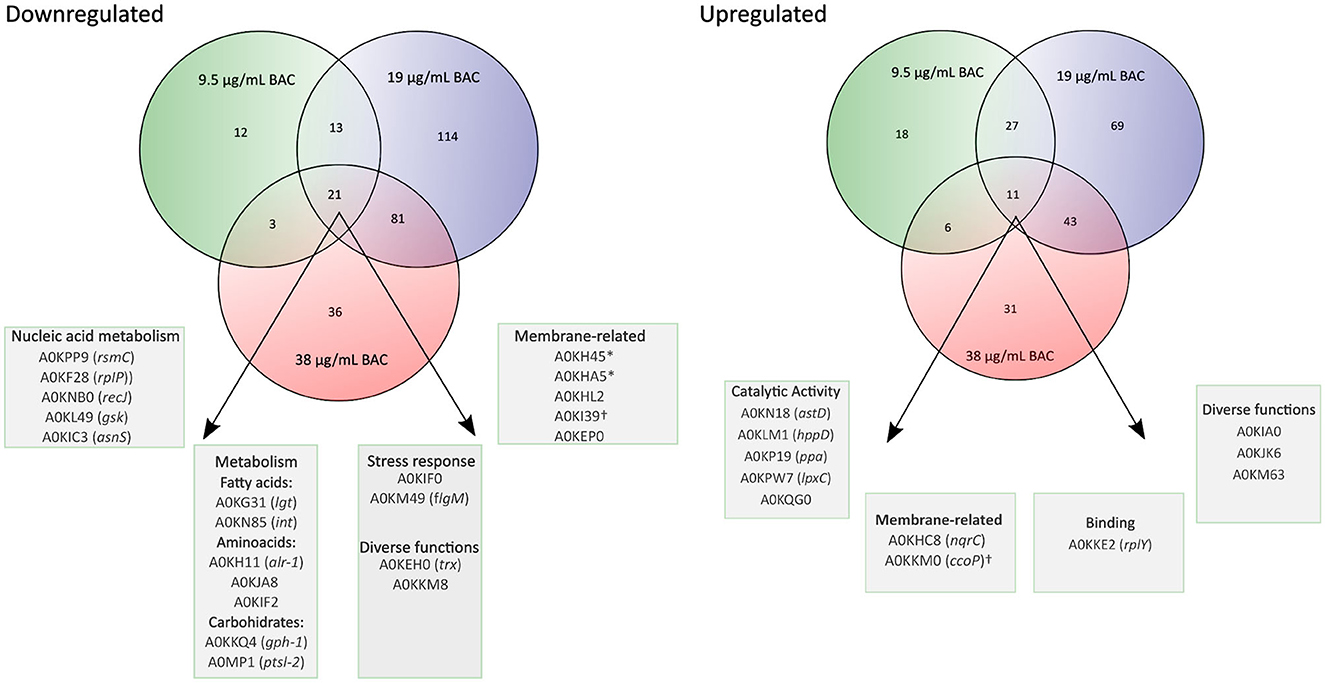
Figure 6. Venn diagrams showing the overlap of proteins that change significantly in their relative abundance (BAC-treated vs. non-treated control) at different BAC concentrations (¼ MIC −9.5 μg/mL-, ½ MIC−19 μg/mL-, and 1 MIC −38 μg/mL-). The porins are marked with an asterisk (*), and the cytochromes are marked with the symbol †.
The results of the quantitative proteome analysis indicate that the basal response of A. hydrophila INISA09 to BAC exposure includes membrane rearrangements and stabilization of the oxidative cell status. Although there are few examples of environmental isolates exposed to BAC for comparative analysis, these results are consistent with those previously reported in a transcriptomic study of Escherichia coli. In this study, exposure to BAC reduced the expression of outer membrane porins, decreased motility and chemotaxis, and increased the expression of respiration-associated genes (Forbes et al., 2019).
A closer examination of the proteins with the highest differential expression level (Tables 3, 4) reveals that three proteins are strongly downregulated across all three tested concentrations. The protein A0KH45 is described as a putative outer membrane porin and a member of the OprD family. This group of proteins is associated with the transport of essential amino acids, and mutations in its sequence can lead to carbapenem resistance (Bateman et al., 2023). A previous study reported that reduced levels of its expression in the outer membrane could allow carbapenem accumulation in outer membrane vesicles and reduce free carbapenems within the cell (Chevalier et al., 2017). Lowering the expression of porins, such as OmpC, OmpD, and OmpF, is one mechanism that decreases the susceptibility of Gram-negative bacteria to biocides (Ferenci and Phan, 2015). In this regard, the INISA09 strain presented mutations in ompD. It has been previously described that A. hydrophila downregulates porin expression under iron-restricted conditions (Wang et al., 2017). A. hydrophila may also use this mechanism to resist stress conditions, such as biocide exposure or starvation.
The protein A0KKM8 appears in the databases uncharacterized, containing a DUF2987 domain. According to the GenBank database, this domain is present in various Vibrio species, including V. parahaemolyticus, V. nigripulchritudo, V. natriegens, V. cholerae, V. cyclitrophicus, V. antiquaries, V. lentus, V. alginolyticus, and V. mimicus, as well as in other species such as Pseudoalteromonas rubia, Alteromonas macleodii, Shewanella baltica, and Alteromonas mediterranea. It is also present in several Aeromonas species, including A. caviae, A. dhakensis, A. jandei, A. media, A. salmonicida, A. veronii, A. sobria, and A. enteropelogenes. A protein–protein interaction analysis using the STRING database (Szklarczyk et al., 2019) reveals a co-occurrence with molecules such as BamC, a lipoprotein part of the Outer Membrane Protein Assembly Complex involved in the assembly and insertion of beta-barrel structures into the outer membrane, and some uncharacterized proteins. This protein has not been characterized in detail until now. However, it is likely that the membrane-related protein is common in aquatic bacteria and is necessary for responding to stress. Therefore, we recommend studying the role of the protein A0KKM8 in future research via functional genomics to improve our understanding of its function, especially in biocide survival conditions.
The A0KIF0 protein, also known as hydrogen peroxide-inducible gene activator, was downregulated in the presence of BAC. This protein has been described as part of the OxyR regulon since 1990, and its function is to defend the cell against oxidative damage caused by hydrogen peroxide (Storz et al., 1990). In A. hydrophila, the expression of OxyR can be modulated by the LahS transcriptional regulator. Under stress conditions, LahS diminishes OxyR expression and enhances biofilm formation (Dong et al., 2018). A similar regulation may occur in INISA09, which suggests that the stress response to BAC is not mediated by hydrogen peroxide-related genes.
Additionally, the protein A0KKM49 (FlgM) is also downregulated. This response under stress conditions has been observed previously in Salmonella spp. where it was shown that damages in the flagella and membrane integrity could repress nutrient acquisition and activate the cell envelope stress response due to environmental factors affecting the bacterial envelop (Spöring et al., 2018). A similar mechanism is likely activated in A. hydrophila under BAC exposure.
For moderate and high doses of BAC, downregulation of several membrane proteins was observed, including A0KFH9 (iron–sulfur cluster assembly protein CyaY), which is related to the electron transport chain, radical generation, and regulation of biosynthesis of sulfur derivatives (Iannuzzi et al., 2011) and transport proteins such as A0KFQ1 (a member of the CorA-like family of transporters with metal ion transporter activity), A0KID0 (with metalloendopeptidase activity and zinc ion binding), and A0KPA7 (an outer membrane protein). In addition, some proteins related to fatty acid syntheses, such as A0KN85, A0KFH8, and A0KNM1, were also downregulated, indicating bacterial membrane reorganization and an alternative response to oxidative stress as a consequence of BAC exposure.
In the INISA09 strain, several cytochrome-related and metal-binding proteins were upregulated during BAC treatment, including A0KIM8, A0KHC8, and A0KKM0. Cytochromes and respiratory chain proteins can stabilize the cell's redox status without metal loss through an independent pathway of OxyR regulation (Mishra and Imlay, 2012). These results suggest that in response to BAC, membrane proteins, such as those involved in oxidative stress response, could be upregulated in the strain INISA09.
Under BAC stress conditions, several proteins related to fatty acid metabolism and membrane proteins were upregulated in the INISA09 strain. A0KK76 (FadI) and A0KK77 (FadJ) are two proteins regulated by the operon CO03404902 (Okuda and Yoshizawa, 2011), and both are part of the fatty acid oxidation with acetyl-CoA. According to STRING (Szklarczyk et al., 2019), these proteins interact with protein NuoC (A0KJ65), a membrane protein associated with electron transport across the membrane to conserve the redox energy in a proton gradient. Another upregulated protein observed that interacts with NuoC is A0KJK6 (the iron–sulfur subunit of succinate dehydrogenase), which is also regulated by the operon CO006649937 that regulates the expression of the succinate dehydrogenase-related genes (dhC, AHA_1924, sdhA, and AHA_1926). The overexpressed protein A0KNI4 (AccB) is part of an operon composed of the genes aroQ, accB, and accC, which are part of the acetyl coenzyme A carboxylase complex and involved in the fatty acid synthesis pathways.
Two other upregulated proteins, namely NifJ-1 and NifJ-2, are oxidoreductases that transfer electrons from pyruvate to flavodoxin and have iron centers. They interact directly with FadI, FadJ, AccB, A0KJK6, AstD (A0KN18), Mdh (A0KG16), and A0KIA0, all of which are related to oxidoreductase activities and fatty acid metabolism. This pattern is remarkable because most of the proteins mentioned have metal centers and can participate in electron transfer processes as mechanisms to balance oxidative stress disequilibrium (Crack et al., 2012). In case of the INISA09 strain, the disturbance caused by BAC at the membrane level could be managed with a reorganization of the fatty acid composition since the primary redox reactions are related to fatty acid metabolism, using redox reactions to equilibrate the oxidative stress.
Finally, we found correspondences between the most critically differentially expressed proteins (Tables 3, 4) and the presence of missense SNPs (see Table 5) (stop codons, deletions, and insertions with frameshift changes were not found). The average number of missense SNPs is 3 per differentially expressed protein. However, it is remarkable that the downregulated proteins A0KH45 (porin), A0KHI6 (transporter), and A0KMF4 (HUT domain protein) present more than 10 missense SNPs. Regarding upregulated proteins, A0KKE3 (histidine kinase) and A0KNN7 (lipoprotein) present more than 15 missense mutations in their sequence. However, further research is required to determine the effect of these mutations on the global BAC response presented by INISA09 or other environmental bacteria.
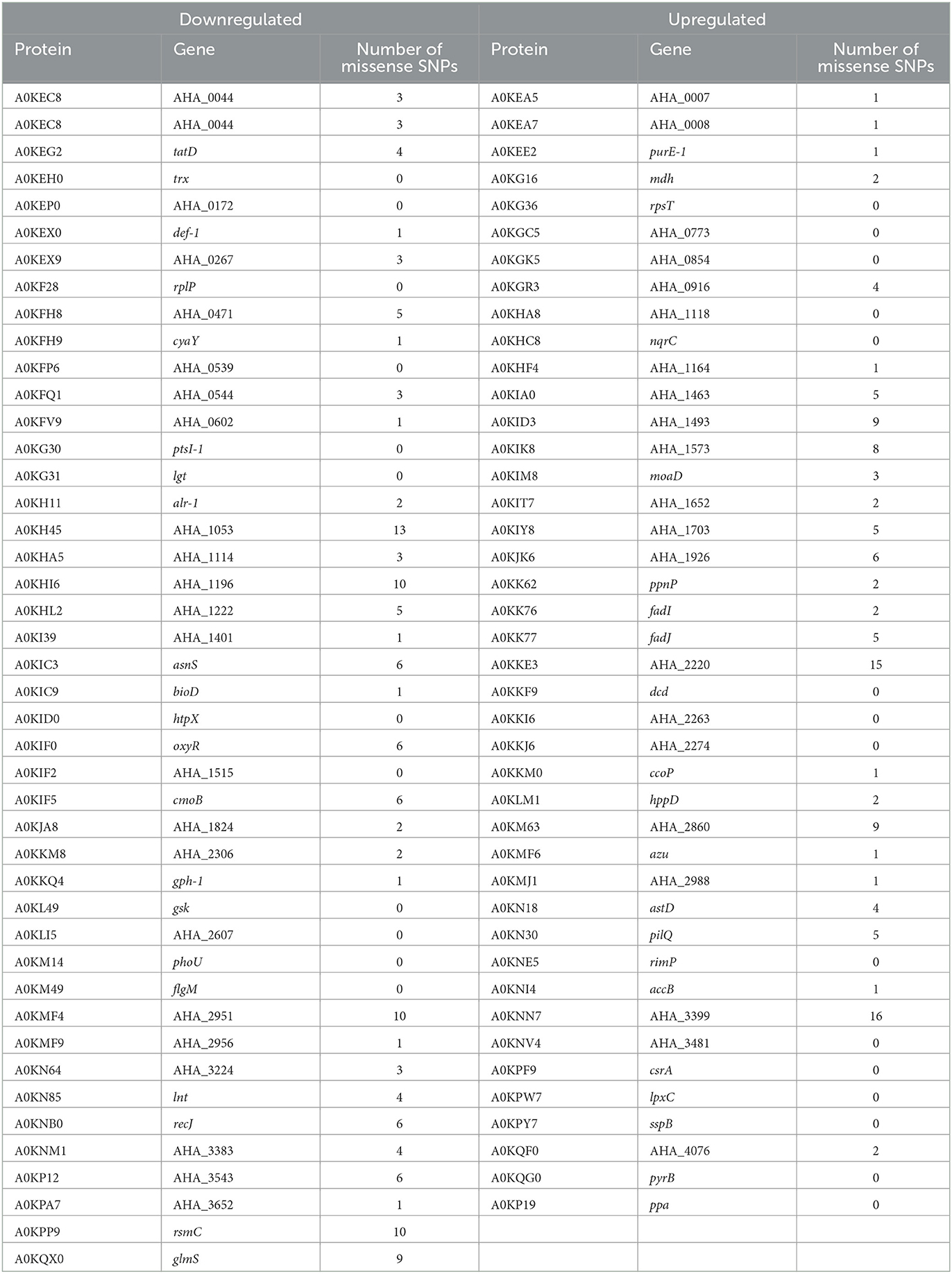
Table 5. Differentially expressed proteins and missense SNP present in Aeromonas hydrophila INISA09.
4. Conclusion
In summary, we have characterized the strain A. hydrophila INISA09 and its low susceptibility to BAC using various genomic, proteomic, and phenotypic techniques. This natural isolate from a Costa Rica domestic activated sludge underwent a profound genome rearrangement compared to other strains, probably fine-tuned by thousands of mutations for better survival in the heavily contaminated environment. The low susceptibility of A. hydrophila INISA09 to BAC can be related to changes in genes and proteins expression from the outer membrane, transmembrane transport, and fatty acid synthesis metabolic pathways (Figure 7). However, we recommend studying putative proteins such as A0KKM8, A0KH45, A0KGK5, A0KKI6, and A0KNV4 in future research using functional genomics strategies to identify or verify their function in biocide survival conditions. Finally, we provide information on mechanisms used by an environmental multidrug-resistant microorganism exposed to increasing amounts of substances such as disinfectants and other antimicrobials discharged into aquatic systems.
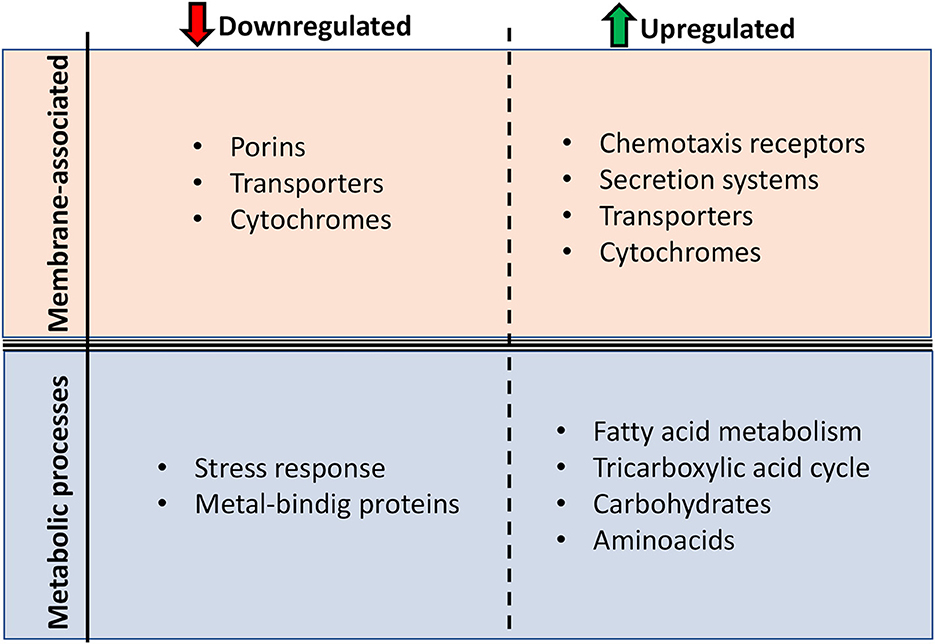
Figure 7. Main protein function changes presented by Aeromonas hydrophila INISA09 upon BAC exposure. The changes were divided into two levels: membrane composition and metabolic processes.
Data availability statement
The datasets presented in this study can be found in online repositories. The names of the repository/repositories and accession number(s) can be found below: https://www.ncbi.nlm.nih.gov/nuccore/NZ_JAPKVL000000000.1.
Author contributions
LC, FS, KR-J, and AR-R contributed to the conception and design of the study. LC organized the database. LC, EG-T, and BK performed the data analysis and wrote sections of the manuscript. BK performed the mass spectrometric measurements. LC, KR-J, and AR-R wrote the first draft of the manuscript. All authors contributed to the manuscript's revision, read, and approved the submitted version.
Funding
Deutscher Akademischer Austauschdienst (DAAD) Short-Term Grants, 2021 and the University o Costa Rica for the financial support of the LC internship in Germany. Vicerrectoría de Investigación of University of Costa Rica for the financial funds for the project B7-255. AR-R was supported by SFB973 from Deutsche Forschungsgemeinschaft (http://www.sfb973.de/), project C5 (https://www.sfb973.de/members_staff/c5/index.html).
Acknowledgments
We thank Jens Rolff from Freie Universität Berlin for his support. For mass spectrometry, we would like to acknowledge the assistance of the Core Facility BioSupraMol, supported by the Deutsche Forschungsgemeinschaft (DFG). Furthermore, we acknowledge support from the Open Access Publication Fund of Freie Universität Berlin.
Conflict of interest
The authors declare that the research was conducted in the absence of any commercial or financial relationships that could be construed as a potential conflict of interest.
Publisher's note
All claims expressed in this article are solely those of the authors and do not necessarily represent those of their affiliated organizations, or those of the publisher, the editors and the reviewers. Any product that may be evaluated in this article, or claim that may be made by its manufacturer, is not guaranteed or endorsed by the publisher.
Supplementary material
The Supplementary Material for this article can be found online at: https://www.frontiersin.org/articles/10.3389/fmicb.2023.1180128/full#supplementary-material
References
Abdelaziz, A., Sonbol, F., Elbanna, T., and El-Ekhnawy, E. (2019). Exposure to sublethal concentrations of benzalkonium chloride induces antimicrobial resistance and cellular changes in Klebsiellae pneumoniae clinical isolates. Microb. Drug Resist. 25, 631–638. doi: 10.1089/mdr.2018.0235
Alcock, B. P., Raphenya, A. R., Lau, T. T. Y., Tsang, K. K., Bouchard, M., Edalatmand, A., et al. (2019). CARD 2020: antibiotic resistome surveillance with the comprehensive antibiotic resistance database. Nucleic Acids Res. 3, 935. doi: 10.1093./nar/gkz935
Andrews, J. M. (2001). Determination of minimum inhibitory concentrations. J. Antimicrob. Chemother. 48, 5–16. doi: 10.1093/jac/48.suppl_1.5
Bankevich, A., Nurk, S., Antipov, D., Gurevich, A. A., Dvorkin, M., Kulikov, A. S., et al. (2012). SPAdes: a new genome assembly algorithm and its applications to single-cell sequencing. J. Comput. Biol. 19, 455–477. doi: 10.1089/cmb.2012.0021
Barber, O. W., and Hartmann, E. M. (2022). Benzalkonium chloride: a systematic review of its environmental entry through wastewater treatment, potential impact, and mitigation strategies. Crit. Rev. Environ. Sci. Technol. 52, 2691–2719. doi: 10.1080/10643389.2021.1889284
Bateman, A., Martin, M-. J., Orchard, S., Magrane, M., Ahmad, S., Alpi, E., et al. (2023). UniProt: the universal protein knowledgebase in 2023. Nucleic Acids Res. 51, D523–D531. doi: 10.1093/nar/gkac1052
Brown, J., Pirrung, M., and Mccue, L. A. (2017). FQC dashboard: integrates FastQC results into a web-based, interactive, and extensible FASTQ quality control tool. Bioinformatics 33, 3137–3139. doi: 10.1093/bioinformatics/btx373
Chacón, L., Arias-Andres, M., Mena, F., Rivera, L., Hernández, L., Achi, R., et al. (2021). Short-term exposure to benzalkonium chloride in bacteria from activated sludge alters the community diversity and the antibiotic resistance profile. J. Water Health 19, 895–906. doi: 10.2166/wh.2021.171
Chen, K. T., Liu, C. L., Huang, S. H., Shen, H-. T., Shieh, Y. K., Chiu, H. T., et al. (2018). CSAR: a contig scaffolding tool using algebraic rearrangements. Bioinformatics 34, 109–111. doi: 10.1093/bioinformatics/btx543
Chevalier, S., Bouffartigues, E., Bodilis, J., Maillot, O., Lesouhaitier, O., Feuilloley, M. G. J., et al. (2017). Structure, function and regulation of Pseudomonas aeruginosa porins. FEMS Microbiol. Rev. 41, 698–722. doi: 10.1093/femsre/fux020
CLSI (2018). CLSI supplement M 100, 28th. ed., ed. CLSI Wayne, PA: Clinical and Laboratory Standards Institute.
Conficoni, D., Losasso, C., Cortini, E., Cesare, D. i., Cibin, A., Giaccone, V., et al. (2016). Resistance to biocides in Listeria monocytogenes collected in meat-processing environments. Front. Microbiol. 7, 1–9. doi: 10.3389/fmicb.2016.01627
Couvin, D., Bernheim, A., Toffano-Nioche, C., Touchon, M., Michalik, J., Néron, B., et al. (2018). CRISPRCasFinder, an update of CRISRFinder, includes a portable version, enhanced performance and integrates search for Cas proteins. Nucleic Acids Res. 46, W246–W251. doi: 10.1093/nar/gky425
Crack, J. C., Green, J., Hutchings, M. I., Thomson, A. J., and Le Brun, N. E. (2012). Bacterial iron-sulfur regulatory proteins as biological sensor-switches. Antioxidants Redox Signal. 17, 1215–1231. doi: 10.1089/ars.2012.4511
Dong, Y., Wang, Y., Liu, J., Ma, S., Awan, F., Lu, C., et al. (2018). Discovery of lahS as a global regulator of environmental adaptation and virulence in Aeromonas hydrophila. Int. J. Mol. Sci. 19, 2709. doi: 10.3390/ijms19092709
Edirsana, M. R. C., Renata, A. C., Alberto, J. G. A., Ftima, C. T. C., Silvano, P. P., Oscarina, V. S., et al. (2014). Multiple antibiotic-resistance of Enterococcus isolated from coastal water near an outfall in Brazil. African J. Microbiol. Res. 8, 1825–1831. doi: 10.5897/ajmr2014.6672
El-Banna, T., Abd El-Aziz, A., Sonbol, F., and El-Ekhnawy, E. (2019). Adaptation of Pseudomonas aeruginosa clinical isolates to benzalkonium chloride retards its growth and enhances biofilm production. Mol. Biol. Rep. 46, 3437–3443. doi: 10.1007/s11033-019-04806-7
EUCAST (2022). EUCAST: Breaking point tables for interpretation of MICs and zone diameters. 1–110. Available online at: http://www.eucast.org
Feiner, R., Argov, T., Rabinovich, L., Sigal, N., Borovok, I., Herskovits, A. A., et al. (2015). A new perspective on lysogeny: prophages as active regulatory switches of bacteria. Nat. Rev. Microbiol. 13, 641–650. doi: 10.1038/nrmicro3527
Ferenci, T., and Phan, K. (2015). How porin heterogeneity and trade-offs affect the antibiotic susceptibility of gram-negative bacteria. Genes 6, 1113–1124. doi: 10.3390/genes6041113
Fernández-Bravo, A., and Figueras, M. J. (2020). An Update on the genus Aeromonas: taxonomy, epidemiology, and pathogenicity. Microorganisms 8, 129. doi: 10.3390/microorganisms8010129
Forbes, S., Morgan, N., Humphreys, G. J., Amézquita, A., Mistry, H., McBain, A. J., et al. (2019). Loss of function in Escherichia coli exposed to environmentally relevant concentrations of benzalkonium chloride. Appl. Environ. Microbiol. 85, 1–14. doi: 10.1128/AEM.02417-18
Gilbert, P., and Moore, L. E. (2005). Cationic antiseptics: diversity of action under a common epithet. J. Appl. Microbiol. 99, 703–715. doi: 10.1111/j.1365-200502664.x
Goñi-Urriza, M., Pineau, L., Capdepuy, M., Roques, C., Caumette, P., Quentin, C., et al. (2000). Antimicrobial resistance of mesophilic Aeromonas spp. isolated from two European rivers. J. Antimicrob. Chemother. 46, 297–301. doi: 10.1093/jac/46.2.297
Gu, Z., Eils, R., and Schlesner, M. (2016). Complex heatmaps reveal patterns and correlations in multidimensional genomic data. Bioinformatics 32, 2847–2849. doi: 10.1093/bioinformatics/btw313
Guo, W., Cui, S., Xu, X., and Wang, H. (2014). Resistant mechanism study of benzalkonium chloride selected Salmonella typhimurium mutants. Microb. Drug Resist. 20, 11–16. doi: 10.1089/mdr.2012.0225
Iannuzzi, C., Adinolfi, S., Howes, B. D., Garcia-Serres, R., Clémancey, M., Latour, J. M., et al. (2011). The role of cyay in iron sulfur cluster assembly on the E. coli iscu scaffold protein. PLoS One 6, 1–9. doi: 10.1371/journal.pone.0021992
Igbinosa, I. H., and Okoh, A. I. (2012). Antibiotic susceptibility profile of Aeromonas species isolated from wastewater treatment plant. Sci. World J. 2012, 1–6. doi: 10.1100/2012/764563
Jain, C., Rodriguez-, R. L. M , Phillippy, A. M., Konstantinidis, K. T., and Aluru, S. (2018). High throughput ANI analysis of 90K prokaryotic genomes reveals clear species boundaries. Nat. Commun. 9, 5114. doi: 10.1038/s41467-018-07641-9
Johnson, J. S., Spakowicz, D. J., Hong, B-. Y., Petersen, L. M., Demkowicz, P., Chen, L., et al. (2019). Evaluation of 16S rRNA gene sequencing for species and strain-level microbiome analysis. Nat. Commun. 10, 5029. doi: 10.1038/s41467-019-13036-1
Karkman, A., Do, T. T., Walsh, F., and Virta, M. P. J. (2018). Antibiotic-resistance genes in waste water. Trends Microbiol. 26, 220–228. doi: 10.1016/j.tim.09005
Knauf, G. A., Cunningham, A. L., Kazi, M. I., Riddington, I. M., Crofts, A. A., Cattoir, V., et al. (2018). Exploring the antimicrobial action of quaternary amines against Acinetobacter baumannii. MBio 9, 17. doi: 10.1128./mBio.02394-17
Langmead, B., and Salzberg, S. L. (2012). Fast gapped-read alignment with Bowtie 2. Nat. Methods 9, 357–359. doi: 10.1038/nmeth.1923
Larsson, D. G. J., and Flach, C. F. (2022). Antibiotic resistance in the environment. Nat. Rev. Microbiol. 20, 257–269. doi: 10.1038/s41579-021-00649-x
Lázár, V., Martins, A., Spohn, R., Daruka, L., Grézal, G., Fekete, G., et al. (2018). Antibiotic-resistant bacteria show widespread collateral sensitivity to antimicrobial peptides. Nat. Microbiol. 3, 718–731. doi: 10.1038/s41564-018-0164-0
Loughlin, M. F., Jones, M. V., and Lambert, P. A. (2002). Pseudomonas aeruginosa cells adapted to benzalkonium chloride show resistance to other membrane-active agents but not to clinically relevant antibiotics. J. Antimicrob. Chemother. 49, 631–639. doi: 10.1093/jac/49.4.631
Magiorakos, A-. P., Srinivasan, A., Carey, R. B., Carmeli, Y., Falagas, M. E., Giske, C. G., et al. (2012). Multidrug-resistant, extensively drug-resistant and pandrug-resistant bacteria: an international expert proposal for interim standard definitions for acquired resistance. Clin. Microbiol. Infect. 18, 268–281. doi: 10.1111/j.1469-0691.2011.03570.x
Mahzounieh, M., Khoshnood, S., Ebrahimi, A., Habibian, S., and Yaghoubian, M. (2014). Detection of antiseptic-resistance genes in Pseudomonas and Acinetobacter spp. isolated from burn patients. Jundishapur J. Nat. Pharm. Prod. 9, 1–4. doi: 10.17795/jjnpp-15402
Maiti, B., Shetty, M., Shekar, M., Karunasagar, I., and Karunasagar, I. (2012). (2012). Evaluation of two outer membrane proteins, Aha1 and OmpW of Aeromonas hydrophila as vaccine candidate for common carp. Vet. Immunol. Immunopathol. 149, 298–301. doi: 10.1016/j.vetimm.07013
Mishra, S., and Imlay, J. (2012). (2012). Why do bacteria use so many enzymes to scavenge hydrogen peroxide? Arch. Biochem. Biophys. 525, 145–160. doi: 10.1016/j.abb.04014
Mohapatra, S., Yutao, L., Goh, S. G., Ng, C., Luhua, Y., Tran, N. H., et al. (2023). Quaternary ammonium compounds of emerging concern: classification, occurrence, fate, toxicity and antimicrobial resistance. J. Hazard. Mater. 445, 130393. doi: 10.1016/j.jhazmat.2022.130393
Morrissey, I., Oggioni, M. R., Knight, D., Curiao, T., Coque, T., Kalkanci, A., et al. (2014). Evaluation of epidemiological cut-off values indicates that biocide resistant subpopulations are uncommon in natural isolates of clinically-relevant microorganisms. PLoS ONE 9, e86669. doi: 10.1371/journal.pone.0086669
Nadeem, A., and Wahl, L. M. (2017). Prophage as a genetic reservoir: Promoting diversity and driving innovation in the host community. Evolution (N. Y). 71, 2080–2089. doi: 10.1111/evo.13287
Nakano, Y., Yusaku, D., and Yamagishi, K. (2022). (2022). Phylogenetic trees of closely related bacterial species and subspecies based on frequencies of short nucleotide sequences. bioRxiv. 3, 1390. doi: 10.1101/0510.491390
Nordholt, N., Kanaris, O., Schmidt, S. B. I., and Schreiber, F. (2021). Persistence against benzalkonium chloride promotes rapid evolution of tolerance during periodic disinfection. Nat. Commun. 12, 8. doi: 10.1038./s41467-021-27019-8
Okuda, S., and Yoshizawa, A. C. (2011). ODB: a database for operon organizations, 2011 update. Nucleic Acids Res. 39, D552–D555. doi: 10.1093/nar/gkq1090
Oliveros, J. (2007–2015). Venny. An interactive tool for comparing lists with Venn's diagrams. Available online at: https://bioinfogp.cnb.csic.es/tools/venny/index20.2.html
Östman, M., Lindberg, R. H., Fick, J., Björn, E., and Tysklind, M. (2017). Screening of biocides, metals and antibiotics in Swedish sewage sludge and wastewater. Water Res. 115, 318–328. doi: 10.1016/j.watres.03011
O'Toole, G. A., and Kolter, R. (1998). Initiation of biofilm formation in Pseudomonas fluorescens WCS365 proceeds via multiple, convergent signalling pathways: a genetic analysis. Mol. Microbiol. 28, 449–461. doi: 10.1046/j.1365-199800797.x
Paradis, E., and Schliep, K. (2019). Ape 5.0: an environment for modern phylogenetics and evolutionary analyses in R. Bioinformatics 35, 526–528. doi: 10.1093/bioinformatics/bty633
Rappsilber, J., Mann, M., and Ishihama, Y. (2007). Protocol for micro-purification, enrichment, pre-fractionation and storage of peptides for proteomics using STAGETIps. Nat. Protoc. 2, 1896–906. doi: 10.1038/nprot.2007.261
Rodríguez-Rojas, A., Kim, J. J., Johnston, P. R., Makarova, O., Eravci, M., Weise, C., et al. (2020). Non-lethal exposure to H2O2 boosts bacterial survival and evolvability against oxidative stress. PLOS Genet. 16, e1008649. doi: 10.1371/journal.pgen.1008649
Rodríguez-Rojas, A., Rodríguez-Beltrán, J., Couce, A., and Blázquez, J. (2013). (2013). Antibiotics and antibiotic resistance: a bitter fight against evolution. Int. J. Med. Microbiol. 303, 293–297. doi: 10.1016/j.ijmm.02004
Rodríguez-Rojas, A., and Rolff, J. (2020). “Preparation of Insect Protein Samples for Label-Free Proteomic Quantification by LC-Mass Spectrometry,” in Immunity in Insects, eds. F. Sandrelli and G. Tettamanti (Springer Nature), 53–61. doi: 10.1007./978-1-0716-0259-1_3
Romanova, N. A., Wolffs, P. F. G. G., Brovko, L. Y., and Griffiths, M. W. (2006). Role of Efflux Pumps in Adaptation and Resistance of Listeria monocytogenes to Benzalkonium Chloride. Appl. Environ. Microbiol. 72, 3498–3503. doi: 10.1128/AEM.72.5.3498-3503.2006
Schliep, K., Potts, A. J., Morrison, D. A., and Grimm, G. W. (2017). Intertwining phylogenetic trees and networks. Methods Ecol. E8, 1212–1220. doi: 10.1111/2041-210X.12760
Schliep, K. P. (2011). phangorn: phylogenetic analysis in R. Bioinformatics 27, 592–593. doi: 10.1093/bioinformatics/btq706
Schmidt, S. B. I., Rodríguez-Rojas, A., Rolff, J., and Schreiber, F. (2022). Biocides used as material preservatives modify rates of de novo mutation and horizontal gene transfer in bacteria. J. Hazard. Mater. 437, 129280. doi: 10.1016/j.jhazmat.2022.129280
Schneider, V. A., Graves-Lindsay, T., Howe, K., Bouk, N., Chen, H-. C., Kitts, P. A., et al. (2017). Evaluation of GRCh38 and de novo haploid genome assemblies demonstrates the enduring quality of the reference assembly. Genome Res. 27, 849–864. doi: 10.1101/gr.213611.116
Seemann, T. (2014). Prokka: Rapid prokaryotic genome annotation. Bioinformatics 30, 2068–2069. doi: 10.1093/bioinformatics/btu153
Seemann, T. (2015). Snippy: fast bacterial variant calling from NGS reads. Available online at: https://github.com/tseemann/snippy
Seemann, T. (2020). Shovill. Available online at: https://github.com/tseemann/shovill
Seshadri, R., Joseph, S. W., Chopra, A. K., Sha, J., Shaw, J., Graf, J., et al. (2006). Genome sequence of Aeromonas hydrophila ATCC 7966 T : jack of all trades. J. Bacteriol. 188, 8272–8282. doi: 10.1128/JB.00621-06
Shen, W., Le, S., Li, Y., and Hu, F. (2016). SeqKit: a cross-platform and ultrafast toolkit for FASTA/Q file manipulation. PLoS ONE 11, 1–10. doi: 10.1371/journal.pone.0163962
Spöring, I., Felgner, S., Preuße, M., Eckweiler, D., Rohde, M., Häussler, S., et al. (2018). Regulation of flagellum biosynthesis in response to cell envelope stress in Salmonella enterica serovar Typhimurium. MBio 9, 1–17. doi: 10.1128/mBio.00736-17
Sprouffske, K., and Wagner, A. (2016). Growthcurver: an R package for obtaining interpretable metrics from microbial growth curves. BMC Bioinformatics 17, 172. doi: 10.1186/s12859-016-1016-7
Storz, G., Tartaglia, L. A., and Ames, B. N. (1990). The OxyR regulon. Antonie Van Leeuwenhoek 58, 157–161. doi: 10.1007/BF00548927
Stothard, P., and Wishart, D. S. (2005). Circular genome visualization and exploration using CGView. Bioinformatics 21, 537–539. doi: 10.1093/bioinformatics/bti054
Stratev, D., and Vashin, I. (2014). Aeromonas hydrophila sensitivity to disinfectants. J. Fish. 8, 324–330. doi: 10.3153/jfscom.201439
Swain, P. S., Stevenson, K., Leary, A., Montano-Gutierrez, L. F., Clark, I. B. N., Vogel, J., et al. (2016). Inferring time derivatives including cell growth rates using Gaussian processes. Nat. Commun. 7, 1–8. doi: 10.1038/ncomms13766
Szklarczyk, D., Gable, A. L., Lyon, D., Junge, A., Wyder, S., Huerta-Cepas, J., et al. (2019). STRING v11: protein–protein association networks with increased coverage, supporting functional discovery in genome-wide experimental datasets. Nucleic Acids Res. 47, D607–D613. doi: 10.1093/nar/gky1131
Tezel, U., and Pavlostathis, S. G. (2015). (2015). Quaternary ammonium disinfectants: microbial adaptation, degradation and ecology. Curr. Opin. Biotechnol. 33, 296–304. doi: 10.1016/j.copbio.03,18
Tikhonova, E. B., and Zgurskaya, H. I. (2004). AcrA, AcrB, and TolC of Escherichia coli form a stable intermembrane multidrug efflux complex. J. Biol. Chem. 279, 32116–32124. doi: 10.1074/jbc.M402230200
Tyanova, S., Temu, T., and Cox, J. (2016a). The MaxQuant computational platform for mass spectrometry-based shotgun proteomics. Nat. Protoc. 11, 2301–2319. doi: 10.1038/nprot.2016.136
Tyanova, S., Temu, T., Sinitcyn, P., Carlson, A., Hein, M. Y., Geiger, T., et al. (2016b). The Perseus computational platform for comprehensive analysis of (prote)omics data. Nat. Methods 13, 731–40. doi: 10.1038/nmeth3901
U.S. Environmental Protection Agency (2018). EPA Unable to Assess the Impact of Hundreds of Unregulated Pollutants in Land-Applied Biosolids on Human Health and the Environment (19P0002).
Wang, J., Qin, T., Chen, K., Pan, L., Xie, J., Xi, B., et al. (2022). Antimicrobial and Antivirulence Activities of Carvacrol against Pathogenic Aeromonas hydrophila. Microorganisms 10, 2170. doi: 10.3390/microorganisms10112170
Wang, Y., Chen, H., Guo, Z., Sun, L., Fu, Y., Li, T., et al. (2017). Quantitative proteomic analysis of iron-regulated outer membrane proteins in Aeromonas hydrophila as potential vaccine candidates. Fish Shellfish Immunol. 68, 1–9. doi: 10.1016/j.fsi.07002
Worthing, K. A., Marcus, A., Abraham, S., Trott, D. J., and Norris, J. M. (2018). (2018). Qac genes and biocide tolerance in clinical veterinary methicillin-resistant and methicillin-susceptible Staphylococcus aureus and Staphylococcus pseudintermedius. Vet. Microbiol. 216, 153–158. doi: 10.1016/j.vetmic.02004
Yu, T., Jiang, X., Zhang, Y., Ji, S., Gao, W., Shi, L., et al. (2018). Effect of benzalkonium chloride adaptation on sensitivity to antimicrobial agents and tolerance to environmental stresses in Listeria monocytogenes. Front. Microbiol. 9, 2906. doi: 10.3389./fmicb.2018.02906
Zhang, C., Tezel, U., Li, K., Liu, D., Ren, R., Du, J., et al. (2011). Evaluation and modeling of benzalkonium chloride inhibition and biodegradation in activated sludge. Water Res. 45, 1238–1246. doi: 10.1016/j.watres.09037
Keywords: benzalkonium chloride, wastewater, biocide, quaternary ammonium disinfectants, low-susceptibility, primary response
Citation: Chacón L, Kuropka B, González-Tortuero E, Schreiber F, Rojas-Jiménez K and Rodríguez-Rojas A (2023) Mechanisms of low susceptibility to the disinfectant benzalkonium chloride in a multidrug-resistant environmental isolate of Aeromonas hydrophila. Front. Microbiol. 14:1180128. doi: 10.3389/fmicb.2023.1180128
Received: 05 March 2023; Accepted: 04 May 2023;
Published: 02 June 2023.
Edited by:
Maoda Pang, Jiangsu Academy of Agricultural Sciences (JAAS), ChinaReviewed by:
Jose Ramos-Vivas, Universidad Europea del Atlántico, SpainJhih-Hang Jiang, Monash University, Australia
Copyright © 2023 Chacón, Kuropka, González-Tortuero, Schreiber, Rojas-Jiménez and Rodríguez-Rojas. This is an open-access article distributed under the terms of the Creative Commons Attribution License (CC BY). The use, distribution or reproduction in other forums is permitted, provided the original author(s) and the copyright owner(s) are credited and that the original publication in this journal is cited, in accordance with accepted academic practice. No use, distribution or reproduction is permitted which does not comply with these terms.
*Correspondence: Keilor Rojas-Jiménez, a2VpbG9yLnJvamFzQHVjci5hYy5jcg==; Alexandro Rodríguez-Rojas, YS5yb2phc0BmdS1iZXJsaW4uZGU=; QWxleGFuZHJvLlJvamFzQHZldG1lZHVuaS5hYy5hdA==