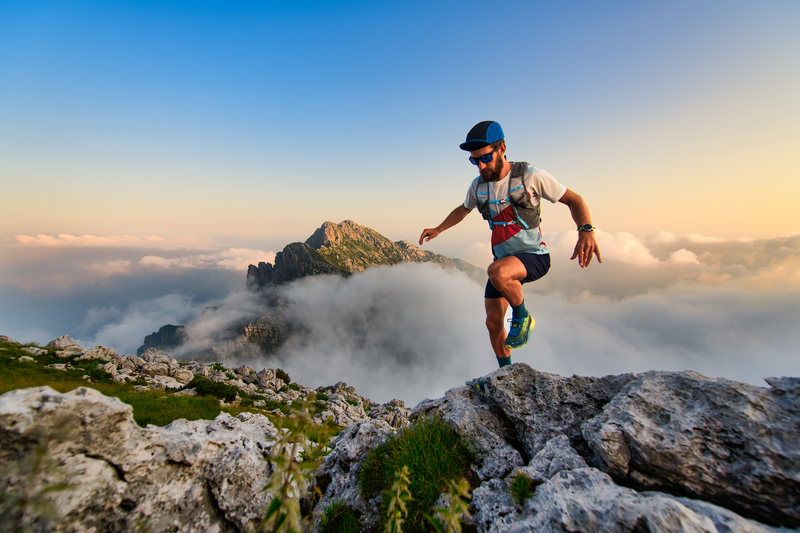
94% of researchers rate our articles as excellent or good
Learn more about the work of our research integrity team to safeguard the quality of each article we publish.
Find out more
ORIGINAL RESEARCH article
Front. Microbiol. , 12 May 2023
Sec. Terrestrial Microbiology
Volume 14 - 2023 | https://doi.org/10.3389/fmicb.2023.1167353
This article is part of the Research Topic Microbial Regulation of Soil Carbon Cycling in Terrestrial Ecosystems View all 12 articles
Changes in the water regime are among the crucial factors controlling soil carbon dynamics. However, at the aggregate scale, the microbial mechanisms that regulate soil respiration under flooding and drying conditions are obscure. In this research, we investigated how the shift from flooding to drying changes the microbial respiration of soil aggregates by affecting microbial community composition and their co-occurrence patterns. Soils collected from a riparian zone of the Three Gorges Reservoir, China, were subjected to a wet-and-dry incubation experiment. Our data illustrated that the shift from flooding to drying substantially enhanced soil respiration for all sizes of aggregate fractions. Moreover, soil respiration declined with aggregate size in both flooding and drying treatments. The keystone taxa in bacterial networks were found to be Acidobacteriales, Gemmatimonadales, Anaerolineales, and Cytophagales during the flooding treatment, and Rhizobiales, Gemmatimonadales, Sphingomonadales, and Solirubrobacterales during the drying treatment. For fungal networks, Hypocreales and Agaricalesin were the keystone taxa in the flooding and drying treatments, respectively. Furthermore, the shift from flooding to drying enhanced the microbial respiration of soil aggregates by changing keystone taxa. Notably, fungal community composition and network properties dominated the changes in the microbial respiration of soil aggregates during the shift from flooding to drying. Thus, our study highlighted that the shift from flooding to drying changes keystone taxa, hence increasing aggregate-scale soil respiration.
Soil aggregates, the fundamental building blocks of soil structure, perform a vital function in SOC turnover and nutrient cycling by providing different habitats for microbial activity (Six et al., 2004; Wang et al., 2021). According to the hierarchical model, aggregates are classified as macroaggregates (> 0.25 mm) and microaggregates (< 0.25 mm; Tisdall and Oades, 1982). Different aggregate size classes have distinct roles in soil nutrient supply and retention by influencing soil biological processes and pore characteristics (Mangalassery et al., 2013; Chen et al., 2023), thereby leading to small-scale heterogeneity in SOC mineralization (i.e., soil respiration) (Wang et al., 2019). Macroaggregates generally comprise labile young SOC, predominantly originating from fresh SOC inputs, fungal hyphae, and plant residues (Six et al., 2004). However, the most recalcitrant SOC formed by microbial-induced bonding of clay particles and organometallic complexes is stored in microaggregates (Zhang et al., 2018). Although macroaggregates are considered to have higher soil respiration than microaggregates (Noellemeyer et al., 2008; Fernández et al., 2010; Bandyopadhyay and Lal, 2014), macroaggregates have been shown to reduce soil respiration in contrast to microaggregates in various studies (Drury et al., 2004; Sey et al., 2008) or the same among aggregate size fractions (Razafimbelo et al., 2008; Rabbi et al., 2014). These conflicting results suggest that further research is required on the regulatory mechanisms of aggregate-scale soil respiration.
Soil respiration is profoundly affected by soil water regimes (Luo and Zhou, 2006; Zhu et al., 2020, 2022a). The changes in water regimes, such as intensive rain after a long drought or drying after intensive flooding, are dominant factor regulating biogeochemical processes in soils (Bodner et al., 2013; Evans and Wallenstein, 2014). These changes have a vital impact on soil aggregate stability, nutrient cycling, and microbial community composition and activity (Denef et al., 2001; Jansson and Hofmockel, 2020). Various microbial communities may colonize a given environment because varied sizes of aggregates provide unique niches for them to thrive in (including aerobic and anaerobic environments, for example) (Trivedi et al., 2017). Microaggregates have a high fungal abundance and a low bacterial abundance (Gupta and Germida, 1988; Jiang et al., 2018; Wang et al., 2021). Microaggregates amass more recalcitrant carbon, which is more favorable to oligotrophs, while macroaggregates contain comparatively much labile carbon, which favors copiotrophs (Trivedi et al., 2017). Increased soil water content generally promotes bacterial abundance, whereas drought decreases bacterial activity (Johan et al., 1986; Meisner et al., 2013). Owing to their thick cell walls, fungi are insufficiently sensitive to changes in soil moisture (Holland and Coleman, 1987; Umair et al., 2020; Dacal et al., 2022). Therefore, soil bacteria and fungi may respond differently to water regime changes at the aggregate scale (Navas et al., 2021), thereby affecting soil respiration. However, at the aggregate scale, our knowledge of how soil microbe communities respond to changes in water availability is still rather restricted.
Riparian zones, or the transition zones between aquatic and terrestrial ecosystems, facilitate critical ecological functions, such as supplying corridors for species migration and improving biodiversity (Jones et al., 2010; de Sosa et al., 2018). Water-level fluctuations have a significant impact on riparian ecosystems’ functions (Leira and Cantonati, 2008). These fluctuations are the major drivers of water regime changes (the shift from aerobic to anaerobic environments), which substantially affects soil respiration, soil aggregates and soil microbial community structure (Fierer et al., 2003; Zhu et al., 2022a). Nevertheless, it is not clear how microbial community changes at the aggregate scale due to water regime changes and how these changes affect respiration.
To date, research on the impacts of water regime changes on soil respiration and its mechanisms have primarily focused on wet–dry cycles (Jarvis et al., 2007), rewetting (i.e., rainfall) after long-term drought (De Nijs et al., 2019), peatland drainage, and water level decline (Silvola et al., 1996; Danevčič et al., 2010). Among these studies, the focus has been on bulk soil. However, little is known about the impact of soil microbes on aggregate-scale soil respiration under water regime changes in the riparian zone. Filling this knowledge gap will improve our understanding of the SOC dynamics in water regime changes, which thus contributing to the realization of riparian ecosystem carbon sequestration and emission reduction targets.
The riparian zones of the Three Gorges Reservoir (TGR) are an excellent location for investigating the influence of soil microbes in soil respiration in the face of extreme shifts in the water regime. The water level of the TGR in China varies from 145 m to 175 m during summer and winter, respectively, due to dam activities, which results in the formation of a new riparian zone that is 349 km2 (Zhu et al., 2020). In the TGR riparian zone, yearly disruption from fluctuating water levels, soil erosion and deposition caused by periodical draining and flooding, and the presence of microorganisms all have the potential to significantly alter soil aggregation (Xiang et al., 2018; Zhu et al., 2022a). She et al. (2022) recently reported that water regime changes result in distinct microbial community compositions and functions between the drainage and flooding periods, thereby controlling CH4 and CO2 emissions in the TGR. Zhu et al. (2022a) reported that in the riparian zone of the TGR, intense wet–dry oscillations reduce the soil’s aggregate stability while simultaneously increasing soil respiration. However, in this zone, the microbial mechanisms of aggregate-scale soil respiration under water regime changes remain unclear.
This research sought to examine how aggregate-scale soil respiration is regulated by microbial community structure in response to changes in the water regime (i.e., from flooding to drying), with a specific focus on microbiota population, microbial co-occurrence tendencies, and their keystone taxa in networks. Specifically, this study investigates (1) how varying aggregate sizes influence soil respiration, bacterial and fungal community composition, and co-occurrence networks during flooding and drying; (2) how the microbial keystone taxa change during the flooding and drying periods and whether soil respiration is regulated by keystone taxa at the aggregate scale; and (3) how to reveal the regulatory mechanisms of aggregate-scale soil respiration for different flooding and drying treatments. Considering that soil respiration and microbial communities are susceptible to disturbance due to water regime changes, we hypothesized that (1) soil respiration rate and microbial community richness would decline with the decrease in soil aggregate size, and their respiration would increase with the shift from flooding to drying, and (2) the microbial keystone taxa would predominantly regulate soil respiration in both flooding and drying treatments.
In June 2018, soil samples for this research were taken from the Wuyangwan riparian zone (31°11′20″N, 108°27′40″E), which is representative of the riparian zones along the Pengxi River. As a result of the activities of the Three Gorges Dam, the water level of the Pengxi River, which is a secondary branch in the TGR of the Yangtze River, varies between 145 and 175 meters above sea level (m.a.s.l) in the summer and winter, respectively (Figure 1) since the TGR was fully impounded in 2010 (Zhu et al., 2020). With average annual temperatures of 18.2 ° C and average annual precipitation of 1,200 mm, the climate of this region may be described as a humid and mid-subtropical monsoon. The period from April through September, which is considered to be the plant growth season, receives over 60% of the total yearly precipitation (Zhu et al., 2020). Purple soil, formed from purple sandstone, is the dominant zonal soil type (Entisols in the World Reference Base) (Chen et al., 2014) with a texture dominated by silt loam (2.50% clay, 65.41% silt, and 30.09% sand) (Zhu et al., 2022b). Most native trees have died because of periodic flooding. Moreover, tillage is not allowed in the TGR riparian zone owing to environmental concerns. Thus, grasslands are the dominant land-use type, comprising flood-tolerant grasses such as Xanthium sibiricum, Paspalum thunbergii, and Cynodon dactylon (Ye et al., 2019). Since 2010, corn fields have been converted to selected grasslands, which were used as the study sites.
Figure 1. Water level changes in the Three Gorges Reservoir and sampling frequency in the riparian zone (A); Schematic design and flow chart of the lab incubation experiment (B).
Based on an S-shaped sampling strategy and using a soil corer (5.7 cm diameter), nine soil samples, weighing approximately 1.5 kg were obtained at random from the grasslands from 0 to 10 cm depths. To avoid any impacts that might break the macroaggregates, we used a wooden mallet to carefully drive the corer into the soil.
The soil that had been dried in an oven was used in the calculation of the soil bulk density (SBD). After mixing the sample with distilled water at a ratio of 1:2.5 soil to water, the pH of the soil was determined. SOC and TN were determined by the dry combustion method with a CN elemental analyzer (Vario Max CN Macro Elemental Analyzer, Elementar Analysensysteme GmbH, Hanau, Germany) (Nelson and Sommers, 1996).
Bulk soil (BS) samples were broken by hand along the fractures of the peds (> 8 mm). Plant residues, stones, visible fauna, and roots were excluded from the samples. Aggregate fractions were measured using wet sieving as described by Márquez et al. (2004). Briefly, a stack of sieves (2-, 0.25-and 0.053-mm) was used to manually fractionate air-dried samples of soil into four size classes in distilled water for 2 min at a rate of 30 times/min. The bulk soil was broken down into 4 aggregate fractions: > 2 mm (large macroaggregates, LM), 0.25–2 mm (small macroaggregates, SM), 0.053–0.25 mm (microaggregates, MI), and < 0.053 mm (silt and clay, SC). As soon as wet sieving was complete, the aggregates were given a gentle rinse in sterile water.
The prepared soils were introduced into 1,000 ml incubation jars (equivalent to 200 g of oven-dried soil in each), with three replicate jars of each treatment. In total, 15 glass jars were used for the incubation experiments, including three jars each for BS, LM, SM, MI, and SC. To accomplish soil microbial stability, we pre-incubated all soil samples at 15°C for 7 days with 50% water-filled pore space (Butterly et al., 2010; Jiang et al., 2021).
To simulate the soil moisture changes in the TGR riparian zone, the experiment was conducted at 15°C with two treatments: (a) flooding at a ratio of 1:2.5 soil to water and (b) drying with a moisture content of 50% field capacity. All incubated samples were placed in a constant-temperature incubator at 15°C, and in case it was deemed essential, deionized water was introduced into the mixture. Following the pre-incubation period, gas samples were withdrawn from the flooding incubation using a syringe on days 7, 14, 21, 28, 35, and 42. Subsequently, the flooding incubation experiment was terminated, and the soil samples were naturally dried to a moisture content of 50% field capacity on the 55th day. Gas samples were extracted on days 56, 63, 70, 77, and 84 of the drying incubation. Twice weekly, the jars were weighed, and water was replenished to prevent excessive evaporation and keep the humidity level consistent. Following the removal of the polyethylene film from the jars, their headspaces were purged with fresh air for approximately 15 min. Gas was collected in the jars after they were hermetically sealed with rubber septum covers. At 0 and 1 h after the jar had been sealed, a gas-tight syringe was used to extract about 30 ml of gas from the headspace. The levels of carbon dioxide in the samples were determined by the use of Gas chromatography (GC-2014, Shimadzu, Japan).
Following the guidelines provided by the manufacturer of the MoBio PowerSoil DNA extraction kit, total DNA was extracted from 0.25 g of soil. Following amplification with fungal primers, ITS1F/ITS2R (Adams et al., 2013) and bacterial primers, 338\u00B0F/806R (Lee et al., 2012), samples were sent to the Novogene Biotechnology Co., Ltd. (Beijing, China) for sequencing via Illumina® MiSeq. To extract the valid data (clean data) after sequencing, the raw data were first demultiplexed and then subjected to quality filtering using the Trimmomatic program (Magoč and Salzberg, 2011). A table of operational taxonomic units (OTUs) was created by clustering the sequences. Using the UPARSE program (UPARSE v7.0.10011; Edgar, 2013) Sequences from bacteria and fungi with a similarity of ≥97% were placed in the same OTU. BLAST was employed to search the UNITE (fungi) and RDP (bacteria) databases for matching sequences, and then representatives of each OTU were chosen for taxonomic annotation (Wang et al., 2007; Kõljalg et al., 2013). There were a total of 17,115 16S OTUs and 5,033 ITS OTUs discovered across all samples. Bacterial and fungal raw sequencing data were jointly submitted to the National Center for Biotechnology Information (NCBI) Sequence Read Archive (SRA) database under BioProject accession number PRJNA844584.
The soil microbial respiration was calculated according to the following formula (Wang et al., 2014):
Whereby, F denotes the CO2 flux (mg m−2 h−1); ρ (kg m−3) represents the CO2 density under normal circumstances; the effective volume is denoted by v (m3) while the bottom of the incubation jar is denoted by (m2); t0 represents the absolute temperature when circumstances are normalized; t signifies the absolute temperature within the jar; and is the shift in the level of CO2 (m3 m−3) that occurred within the jar throughout the sampling duration (h).
R software (version 4.1.0) was used for every computation along with the data analyzes. The Levene’s and Kolmogorov–Smirnov tests were completed to correspondingly ensure the homogeneity of variances and the normality of the data before proceeding with the analysis. If the conditions were not met, a log or square-root transformation was applied. Soil aggregate fractions were separated into four groups, and their individual attributes were compared via analysis of variance (ANOVA). Soil respiration variations between aggregate fractions were analyzed through repeated-measure ANOVA. Also, an independent sample t-test was conducted to contrast the impacts of flooding and drying treatments on the aforementioned characteristics at the same aggregate scale. Two-way ANOVA was executed to determine the different moisture treatments, soil aggregate sizes, and their interactions with soil respiration.
Using the cmdscale function in the vegan package, principal coordinate analysis (PCoA) was utilized to investigate the differences in bacterial and fungal community architectures across the various treatments and aggregate fractions. To show the connections between microbial populations and to compute their topological features, we adopted the co-occurrence network inference (CoNet) in Gephi 0.9.2. Keystone species were identified as OTUs exhibiting a high degree, high eigenvector centrality, and high closeness/betweenness centrality (Wang et al., 2021). The highest average degree was considered a complex microbial network (Wagg et al., 2019). Soil respiration and keystone species abundance were also subjected to regression analyzes (Banerjee et al., 2016).
To determine how various predictor factors may be influencing soil respiration, partial least squares path modeling (PLS-PM) was carried out using the plspm program (Sanchez, 2013). Fourteen manifest variables [SOC, C/N ratio, pH, soil respiration, positive to negative edges (P/N) of the overall network (BPN), bacterial P/N related to keystone taxa (BKPN), bacterial average clustering coefficients (BACC), bacterial richness (BR), bacterial first dominant eigengenes BFDE, fungi richness (FR), fungal first dominant eigengenes (FFDE), fungal P/N of the overall network (FPN), fungal P/N related to keystone taxa (FKPN), and fungal average clustering coefficients (FACC)] and four latent variables (bacterial network, bacterial community composition, fungal network, and fungal community composition) were condensed for use in the PLS-PM. There were two or three manifest variables associated with each latent variable. The bacterial networks encompassed BPN, BKPN, and BACC. The bacterial community composition comprised BR and BFDE. Fungal networks included FR, FFDE, and FPN. The fungal community composition included FR and FFDE. We then computed the models’ path coefficients, which describe the direction and intensity of the linear correlations between the variables, as well as the explained variability (R2). Based on this information, we determined the overall influence (both direct and indirect) that each variable had on soil respiration. The path coefficients represent the direct impacts, while the indirect effects may be calculated by multiplying the direct effects by the indirect path’s path coefficients. The model’s overall prediction accuracy was measured by calculating its goodness of fit. Before running PLS-PM, we used a car package to ensure there wasn’t any multicollinearity between our chosen independent variables. In this investigation, we conducted regression random forest analysis with the rfPermute program to determine the most important soil variables for soil respiration. Variables with low levels of intercorrelation, a variance inflation factor of <10, significance levels of <0.05, and greater levels of mean squared error (MSE percent) were retained (Liaw and Wiener, 2002). Consequently, the proportion of soil aggregates, TN, and SBD was excluded (Supplementary Figure S1).
Flooding and drying influenced soil properties (Table 1). Flooding to drying generally decreased SOC and TN contents but slightly increased soil pH in all aggregate fractions. The SOC and TN contents of >2 mm aggregate fractions were substantially elevated relative to those of the other aggregate fractions in both the flooding and drying treatments. The SBD decreased as the aggregate size increased in both the flooding and drying treatments (i.e., LM < SM < MI < SC).
The soil CO2 flux (i.e., soil respiration) slightly increased with incubation time during the flooding phase (Figure 2). In contrast, soil respiration slightly decreased with increasing incubation time during the drying phase (Figure 2). Mean soil respiration dropped as the aggregate size became smaller in both the flooding and drying treatments (i.e., LM > SM > MI > SC). Notably, flooding to drying substantially enhanced the mean soil respiration (p < 0.05; Figure 3).
Figure 2. Flux of CO2 among soil aggregates in flooding and drying status. BS, bulk soil; LM, large macroaggregate, > 2 mm; SM, small macroaggregate, 0.25–2 mm; MI, microaggregate, 0.053–0.25 mm; SC, silt and clay, < 0.053 mm.
Figure 3. Mean soil respiration of different aggregate sizes in flooding and drying treatments during the incubation period. BS, bulk soil; LM, large macroaggregate, > 2 mm; SM, small macroaggregate, 0.25–2 mm; MI, microaggregate, 0.053–0.25 mm; SC, silt and clay, < 0.053 mm. A significant difference in soil respiration across aggregate sizes (p 0.05) in both the flooded and dried conditions is shown by the bars designated with capital letters and lowercase letters, correspondingly.
The soil microbial community richness is presented in Supplementary Table S1. The bacterial richness considerably decreased in response to the shift from flooding to drying. In contrast, the fungal richness substantially increased in response to the shift from flooding to drying. The maximum bacterial richness among the aggregate fractions in the flooding and drying conditions were MI (i.e., 5139.00 ± 38.73) and SC (i.e., 4290.67 ± 152.78), respectively. The minimum bacterial richness among the aggregate fractions in the flooding and drying conditions were SC (i.e., 4617.00 ± 107.41) and MI (i.e., 3671.33 ± 93.38), respectively. The maximum fungal richness among the aggregate fractions in the flooding and drying conditions were SM (i.e., 611.00 ± 22.15) and SC (i.e., 723.33 ± 86.33), respectively. The minimum fungal richness among the aggregate fractions in the flooding and drying conditions were MI (i.e., 512.67 ± 61.06) and SM (i.e., 663.67 ± 27.28), respectively. Nevertheless, no significant variance in fungal richness was discovered among the aggregate fractions under the drying treatment. Moreover, the shift from flooding to drying reduced the bacteria-to-fungi ratio in all the aggregate fractions.
The relative abundance of the dominant bacterial and fungal phyla in soil aggregates under flooding and drying treatments are shown in Figure 4. The bacterial communities in soil aggregates predominately comprised Proteobacteria (34.6–43.3%), Acidobacteria (11.4–16.3%), Actinobacteria (6.8–12.1%), Firmicutes (3.6–7.7%), and Planctomycetes (1.9–2.2%). Compared with the flooding, the drying treatment resulted in an increase of 38.4–57.5% in the relative abundance of actinobacteria. The bacterial communities primarily included Ascomycota (9.3–36.5%), Basidiomycota (1.9–26.5%), Rozellomycota (0.1–5.2%), and Chytridiomycota (0.1–4.1%). Compared with the flooding treatment, the drying treatment generally enhanced the relative abundance of Basidiomycota and Ascomycota in soil aggregates.
Figure 4. Aggregate size fractions during flooding and drying conditions and the relative abundance of key phyla within fungi and bacteria communities.
The PCoA revealed that both bacterial and fungal community compositions were distinct among the aggregate fractions and treatments (Figure 5). Three samples from the same moisture conditions were grouped, regardless of the aggregate size. Moreover, the samples from different moisture conditions were completely separated from each other. The first two principal coordinates together explained 34.29 and 29.24% of the differences in soil bacteria and fungi community compositions, correspondingly.
Figure 5. The impacts of moisture status and aggregate fractions on bacterial (A) and fungal (B) populations are shown using principal coordinate analysis predicated on the Bray-Curtis distances. Symbols of varying hues depict various aggregate fractions, each corresponding to a certain treatment of soil moisture. FBS, bulk soil in the flooding treatment; FLM, large macroaggregates in the flooding treatment; FSM, small macroaggregates in the flooding treatment; FMI, microaggregates in the flooding treatment; FSC, silt and clay in the flooding treatment; DBS, bulk soil in the drying treatment; DLM, large macroaggregates in the drying treatment; DSM, small macroaggregates in the drying treatment; DMI, microaggregates in the drying treatment; DSC, silt, and clay in the drying treatment.
To evaluate differences in network features between individual treatments and for every aggregate fraction, we generated 20 subnetworks from the bacterial and fungal populations. Supplementary Table S3 displays the results of the ANOVA. Both bacteria and fungi community compositions exhibited modified co-occurrence patterns in response to the varying soil moisture treatments (Figure 6). The changes in the soil moisture conditions substantially affected the dynamics of the microbial and fungal populations’ interplay. The shift from flooding to drying increased the ratio of positive to negative edges (P/N), average degree, and average clustering coefficient in all bacteria and fungi networks and most subnetworks (Table 2; Supplementary Tables S2, S3).
Figure 6. Networks showing the co-occurrence of bacterial (A,B) and fungal (C,D) populations under flooding and drying, respectively. A connection represents a statistically significant (p < 0.005) relationship between two OTUs. In a network, a node’s size is equivalent to the number of its connections (i.e., degree), and the Pearson correlation coefficients are used to determine the thickness of the edges connecting the nodes. Positive and negative interactions are denoted by the red and green edges, correspondingly.
The changes in soil moisture conditions changed the keystone taxa (Table 3). For bacterial networks, Acidobacteria (unclassified Acidobacteria at the order level), Acidobacteria (Acidobacteriales), Gemmatimonadetes (Gemmatimonadales), Chloroflexi (Anaerolineales), and Bacteroidetes (Cytophagales) were the keystone taxa in the flooding treatment, whereas, in the drying treatment, the keystone taxa were Proteobacteria (unclassified), Proteobacteria (Rhizobiales), Gemmatimonadetes (Gemmatimonadales), Proteobacteria (Sphingomonadales), and Actinobacteria (Solirubrobacterales). For fungal networks, the keystone taxa in the flooding and drying treatments were Ascomycota (Hypocreales) and Basidiomycota (Agaricales), respectively.
Variation in the keystone taxa was observed across soil moisture manipulations (Supplementary Table S3). Bacterial subnetworks showed changes in their keystone taxa in response to manipulations of soil moisture and particle size distributions. P/N was solely influenced by soil moisture treatments for fungal subnetworks, whereas the average clustering coefficient was influenced by the interplay of aggregate sizes and soil moisture treatments.
The keystone taxa abundance was shown to have a strong correlation with soil respiration in regression analyzes (Figure 7). For bacteria, soil respiration was positively influenced by OTU88 (Proteobacteria; R2 = 0.75, p < 0.01) under flooding treatment. Under drying treatment, soil respiration was positively influenced by OTU88 (i.e., Proteobacteria; R2 = 0.32, p < 0.01), OTU89 (Gemmatimonadetes; R2 = 0.88, p < 0.01), OUT144 (Actinobacteria; R2 = 0.95, p < 0.01), OTU162 (Actinobacteria; R2 = 0.60, p < 0.01), and OTU13562 (Actinobacteria; R2 = 0.21, p < 0.05). For fungi, soil respiration was positively influenced by OTU2732 (Ascomycota; R2 = 0.29, p < 0.05) under flooding treatment. Under flooding treatment, soil respiration was negatively influenced by OTU13 (unclassified fungus; R2 = 0.68, p < 0.01). Moreover, under drying treatment soil respiration was positively influenced by OTU11 (Basidiomycota; R2 = 0.32, p < 0.01), OTU12 (Ascomycota; R2 = 0.88, p < 0.01), OTU3 (Basidiomycota; R2 = 0.95, p < 0.01), OTU222 (Basidiomycota; R2 = 0.60, p < 0.01), and OTU477 (unclassified fungus; R2 = 0.21, p < 0.05).
Figure 7. The relationships of soil respiration with the richness of key bacterial (A,C) and fungal members (B,D).
A regression random forest analysis was showed that the key soil parameters that were linked to soil respiration were SOC, C/N ratio, and pH (Supplementary Figure S1). Soil respiration was also significantly influenced by the make-up of the microbial population (as measured by the first dominant eigengenes, FDE) and the features of the microbial network (as measured by the P/N ratio related to keystone taxa and the mean cluster coefficients).
In both the flooded and dried soils, PLS-PM analysis showed causative links between soil respiration, soil microbial network, soil microbial community composition, and environmental factors (Figure 8). In the flooding treatment, SOC, bacterial network, and fungal community composition exhibited a remarkable direct effect on soil respiration, with path coefficients of 0.71, 0.21, and 0.26, correspondingly (p < 0.05). Additionally, the SOC and C/N ratios both exhibited substantial indirect effects on soil respiration through fungal community composition, with path coefficients of 0.15 and-0.20, correspondingly (p < 0.05). Consequently, SOC had a strong beneficial effect on soil respiration, as measured by a path coefficient of 0.86. Bacterial networks predominantly and significantly relied on BPN and BACC with loading coefficients of 0.40 and 0.56, correspondingly (p < 0.05). Fungal community composition dominantly relied on their FFDE with a loading coefficient of 0.85 (p < 0.05).
Figure 8. Soil respiration derived from different sizes of aggregates after exposure to flooding (A) and drying (B) treatments were modeled using a partial least squares path model of the impacts of soil characteristics, microbial population structure, and microbial network topological parameters (B). Positive paths (p < 0.05) are denoted by solid arrows, while insignificant paths (p > 0.05) are illustrated by the dotted arrows. The goodness-of-fit (GoF) statistic was employed to evaluate the model. SOC, soil organic carbon; C:N, the ratios of total carbon and nitrogen; BPN, P/N of the entire network; BKPN, P/N of the bacterial network related to keystone taxa; BACC, average clustering coefficients of the whole network; BR, the relative abundance of bacteria; BFDE, first dominant eigengenes of the bacterial community composition; FPN, P/N of the entire network; FKPN, P/N of the bacterial network related to keystone taxa; FACC, average clustering coefficients of the fungal network; FR, the relative abundance of fungi; FFDE, first dominant eigengenes of fungal community composition.
In the drying treatment, SOC, bacterial network, fungal community composition, and the fungal network exhibited substantial direct effects on soil respiration, as indicated by path coefficients of 0.57, 0.32, 0.25, and 0.56, correspondingly (p < 0.05). Moreover, the SOC and pH exhibited substantial indirect effects on soil respiration through fungal community composition, as illustrated by coefficients of 0.14 and 0.11, correspondingly (p < 0.05). By way of the microbial community, the C/N ratio strongly influenced soil respiration (path coefficient = −0.19) (p < 0.05). Furthermore, SOC had a path coefficient of 0.71, indicating that it considerably and positively influenced soil respiration. The bacterial network dominantly and significantly relied on BKPN with a loading coefficient of 0.61 (p < 0.05). Moreover, fungal community composition predominantly relied on FR with a loading coefficient of 0.94 (p < 0.05). The fungal network predominantly relied on FACC with a loading coefficient of 0.79 (p < 0.05).
Soil respiration reflects short-term dynamics of SOC, which are primarily affected by several biotic and abiotic factors, like microorganisms, substrate availability, and water content (Luo and Zhou, 2006; Wang et al., 2020). In this study, the respiration of different-sized soil aggregates slightly increased with the incubation time during the flooding phase, whereas it slightly decreased with incubation time during the drying phase (Figure 2). These differences may be caused by the discrepancy between the labile and recalcitrant carbon fractions used by microbes (Rusalimova and Barsukov, 2006). Generally, labile organic carbon is preferentially decomposed by soil microorganisms during the early stages of incubation. However, microorganisms began to use the recalcitrant organic carbon fraction which is more difficult to decompose in the late stage of incubation (Hao et al., 2008; Rabbi et al., 2014; Bimüller et al., 2016). In addition, the soil respiration rate fluctuated during the incubation process, which was likely because of soil disturbance caused by the pretreatment process, such as the collection of soil samples (Hao et al., 2008).
The variations in soil respiration at different aggregate sizes reflect the dominant roles of carbon and nitrogen contents and microbial activity within the aggregates under relatively uniform incubation conditions (Gupta and Germida, 1988; Kan et al., 2020). Our results showed that significant differences in the respiration of different size fractions of soil aggregates were observed under the same incubation conditions, and the mean soil respiration rate generally increased with the soil aggregate size-classes. These results support our first hypothesis, conforming to the conclusions drawn by Noellemeyer et al. (2008). The reasons for this are as follows. First, in the absence of plant roots, soil respiration is primarily derived from the decomposition of organic carbon by soil microorganisms (Ontl and Schulte, 2012). Further, the activities of microorganisms responded to the discrepancy in the amount and stability of organic carbon in different soil aggregate sizes, thereby leading to variations in soil respiration among different aggregate size-classes (Trivedi et al., 2017). Second, macroaggregate-associated carbon primarily derives from labile organic carbon (e.g., fresh plant residues) that easily decomposes, whereas microaggregate-associated carbon primarily comprises humus that is difficult to decompose and use (Six et al., 1999). Moreover, because the pore necks in microaggregates are <0.2 μm in width, the pores in these structures are impenetrable to bacteria, thereby inhibiting biological activity (Erktan et al., 2020; Zhu et al., 2022b).
The mean soil respiration of all aggregate size fractions in the drying treatment was substantially elevated in contrast to the flooding treatment under a constant temperature (Figure 3). The results primarily showed the inhibition of organic matter decomposition, as soil pore spaces are occupied by water in the flooding treatment (Gorham, 1995); Additionally, during the drying treatment, 50% field water retention capacity was maintained in the soil samples since it is suitable for microbial activity, particularly in the early stage of measurement (Manzoni et al., 2012).
Soil aggregates act as heterogeneous microhabitats for highly spatially organized microorganism communities (Upton et al., 2019). In this study, the maximum bacterial abundances among the aggregate fractions under flooding and drying conditions were MI and SC, respectively. SM had the maximum fungal abundance under flooded conditions. These findings were comparable to those obtained in earlier investigations which revealed that the bacterial and fungal abundances are maximum in <0.25 mm and > 0.25 mm aggregates, correspondingly (Zhang et al., 2014; Bach et al., 2018; Wilpiszeski et al., 2019). The presence of protected habitats in microaggregates may facilitate niche creation for bacteria by excluding their predators (protozoa) and competing with fungi, since predation serves as a crucial structuring force for bacterial communities (Zhang et al., 2014). Additionally, due to the narrow gaps that exist between microaggregates, silt, and clay fractions, it is physically impossible for fungi to penetrate the interior of these habitats (Zhang et al., 2014; Erktan et al., 2020).
Soil water regimes, which are associated with most soil properties and processes, have a profound influence on the structure and function of the soil microbial population in the soil (Brockett et al., 2012; Schimel, 2018). In this study, the shift from flooding to drying decreased the bacteria–fungi ratio in all aggregate size fractions. Notably, fungi are heterotrophic and essentially aerobic with limited anaerobic capabilities (McGinnis and Tyring, 1996). The shift from flooding to drying changed the soil microenvironment and improved the exchange of air and heat, which was conducive to soil fungal aeration. Fungal communities are generally more resistant to environmental alterations than bacterial communities (Lin et al., 2019). Consequently, fungi rapidly reproduce, thereby improving their diversity (Zhang, 2010). In addition, soil bacteria might adjust their expression of certain metabolic processes (e.g., resulting in the synthesis of compounds with osmoprotective properties) in response to the stress environment and maintain their growth during flooding (Chowdhury et al., 2019). For bacteria, Proteobacteria were the dominant taxa in both flooding and drying treatments. Acidobacteria and Bacteroidetes were the subdominant groups in the flooding and drying treatments, respectively. Among fungi, the dominant taxa were Ascomycetes and Basidiomycetes. However, the shift from flooding to drying considerably increased the relative abundance of Basidiomycetes. These results were in line with those found in earlier investigations, which revealed that the microbial communities Proteobacteria, Acidobacteria, Bacteroidetes, Ascomycetes, and Basidiomycetes are abundant in wetland soils which are frequently anaerobic (Tait et al., 2007; Peralta et al., 2013). Moreover, the relative abundance of dominant microbial taxa generally decreased with an increase in aggregate size, which might be related to the nutrient strategy of the microbial community (Fierer et al., 2007; Zhang et al., 2016) where macroaggregates tend to be nutrient-rich, whereas microaggregates are relatively barren (Fonte et al., 2014).
The co-occurrence patterns of soil microbes could be revealed by using network analysis, which is conducive to acquiring a more comprehensive knowledge of the structure of microbial communities and the biological principles that guide community formation (Barberán et al., 2012). Herein, in both the flooding and drying conditions, Proteobacteria and Actinobacteria predominated in the bacterial network, while Ascomycota predominated in the fungus network, indicating that these phyla could be the most influential in determining the overall architecture of microbiomes. This is likely because Proteobacteria, Actinobacteria, and Ascomycota can resist external pressure and disturbances (Zhou et al., 2020; Faitová, 2021). This finding aligns with the conclusions drawn by Ye et al. (2021) in their study of co-occurrence patterns in the soil microbial network within the riparian zone of the TGR. In addition, the fungal network exhibited higher average degrees, and more nodes and edges in the drying treatment than in the flooding treatment, suggesting that the shift from flooding to drying enhanced the connectivity and complexity of the fungal network (Wagg et al., 2019; Ye et al., 2021). These results are in agreement with those of earlier research showing that flooding drastically decreases the complexity of co-occurrence networks (Zhang et al., 2019; Gao et al., 2021) owing to resource limitations and environmental stresses, such as severe flooding stress, reduced water, and availability of nutrients (Malik et al., 2020; Qiu et al., 2021). In addition, positive linkages in the bacterial network, especially those linked to keystone taxa, rose as flooding duration reduced, whereas negative ones dropped as a result of the shift from flooding to drying (i.e., the P/N of the overall network and that related to keystone taxa) (Table 2). This indicates that the shift from flooding to drying increased niche breadths by eliminating flooding stress and reactivating aerobic microbes to enhance the availability of organic materials (Malik et al., 2020), thereby mitigating competition (Banerjee et al., 2016) and exhibiting favorable co-occurrence patterns with selected copiotrophic keystone taxa (Wang et al., 2021).
Soil respiration is determined by the richness of certain taxa (Banerjee et al., 2016). Confirming our second hypothesis, both bacterial and fungal keystone taxa were found using the network analysis, and they were shown to have substantial ties to soil respiration (Figure 7). The abundance of Proteobacteria in bacteria and Ascomycota (Hypocreales) in fungi exhibited remarkably favorable impact on soil respiration during flooding treatment. Proteobacteria, Proteobacteria (Rhizobiales), Gemmatimonadetes (Gemmatimonadales), Proteobacteria (Sphingomonadales), and Actinobacteria (Solirubrobacterales) in bacteria and Ascomycota (Hypocreales), Basidiomycota (Agaricales), an unclassified taxon, in fungi, exhibited strong positive effects on soil respiration in the drying treatment. Proteobacteria, Firmicutes, and Actinobacteria have been found to decompose plant polymers by releasing soil enzymes like xylanases and β-glucosidase (Wang et al., 2010; Tiwari et al., 2016; Zheng et al., 2018). Earlier literature has shown that the families of α-Proteobacteria are decomposers of both fresh and soil organic matter (Bernard et al., 2012; Liu et al., 2021). Herein, both Rhizobiales and Sphingomonadales belong to α-Proteobacteria, which positively affected soil respiration. Actinobacteria are an essential bacterium class that participates in many activities throughout ecosystems, including the breakdown of organic molecules, which can mineralize fused aromatic C-ring structures (Faitová, 2021). Gemmatimonadetes are typically abundant and active in low-moisture soils, playing a vital role in driving soil carbon cycling processes (He et al., 2020; Fan et al., 2021). Thus, our findings illustrated that Gemmatimonadetes positively affected soil respiration during the drying treatment rather than during the flooding treatment. In addition, an unclassified keystone fungus exhibited a considerable negative impact on soil respiration in the flooding treatment, which could explain why negative rates of soil respiration were detected during the drying treatment. Agaricales, an order of Basidiomycota, is an extremely common soft-rot fungus that aids in decomposing dead wood and litter and may generate a spectrum of hydrolytic enzymes capable of breaking down humic and lignin acids (Voříšková and Baldrian, 2013). During decomposition, the prevalent and persistent ascomycetous fungi (Hypocreales) are the endophytes of a wide variety of plants and also include extracellular fungi that produce enzymes (Voříšková and Baldrian, 2013; Herzog et al., 2019). However, the keystone taxa Rhizobiales, Gemmatimonadales, Sphingomonadales, and Solirubrobacterales, Hypocreales, Agaricales are uncultured bacterial or fungal orders. Therefore, further studies are required to determine how they affect the composition and function of soil microbes (Wang et al., 2021). Additionally, keystone taxa should be selectively excluded in future studies to verify the changes in species interactions affecting soil respiration (Zheng et al., 2021).
Microbial community structure and network properties assume critical roles in the dynamics of SOC (Banerjee et al., 2016; Zheng et al., 2021). In both flooding and drying treatments, aggregate fractions remarkably modified the composition of microbial communities and microbial networks, primarily by altering their associated SOC levels (Cookson et al., 2005). In addition, SOC was the dominant regulator of soil respiration, with a path coefficient of 0.86 in the flooding treatment and 0.71 in the drying treatment (Figure 8), suggesting that substrate supply was the major factor affecting CO2 release in this study (Luo and Zhou, 2006). Furthermore, the mechanisms regulating soil respiration were distinct between the flooding and drying treatments (Figure 8).
In the flooding treatment, except for SOC, soil respiration was principally modulated by the ACC and P/N of the entire network and FFDE. However, in the drying treatment, soil respiration was predominantly modulated by FR, FACC, and P/N associated with the bacterial keystone taxa. These results show that the shift from flooding to drying changed the regulatory mechanisms of soil respiration, particularly the fungal network. Archived literatures illustrates that decreasing competitive interactions with keystone taxa enhances soil respiration (Wang et al., 2021), whereas soil respiration diminishes when competitive interactions increase among keystone taxa (Chen et al., 2019). The shift from flooding to drying relieved water stress, thereby alleviating interactions that are competitive with the co-occurrence networks’ keystone taxa. Moreover, C mineralization is less energy-efficient during anaerobic degradation (Wang et al., 2021). Therefore, the shift from flooding to drying increased the average soil respiration rate (Figure 3). Notably, the relieved water stress increases oxygen availability, which creates copiotrophic environments for fungi that prefer aerobic conditions (McGinnis and Tyring, 1996) and interacts with other species by using both labile and recalcitrant carbon fractions (Bian et al., 2022). Also, the effect of the bacterial network on soil respiration increased from a path coefficient of 0.21 to 0.32 (Figure 8), which revealed that the dominant bacterial network with positive interaction in aerobic conditions facilitated the utilization of carbon sources by microorganisms, thereby stimulating soil respiration (Wang et al., 2021).
Our study reveals that the shift from flooding to drying changes the microbial community composition and keystone taxa, thereby enhancing the microbial respiration of soil aggregates. Specifically, soil respiration decreases with a decrease in aggregate size in both flooding and drying treatments. Additionally, the microbial respiration of soil aggregates is substantially higher in the drying treatments than in the flooding treatment as a result of the changes in keystone taxa. Notably, the fungal community composition and network properties dominate the changes in microbial respiration of soil aggregates during the flooding to the drying process. This study reveals the crucial roles of fungal community composition and co-occurrence network properties in regulating soil respiration during the shift from flooding to drying conditions. Moreover, this analysis offers valuable knowledge of the mechanisms of soil respiration changes at the aggregate scale under different water regimes.
The original contributions presented in the study are included in the article/Supplementary material, further inquiries can be directed to the corresponding authors.
KZ: conceptualization, methodology, validation, formal analysis, investigation, writing–original draft, visualization, and funding acquisition. WJ: writing–review and editing. YM: investigation and data curation. SW: methodology and funding acquisition. PH: conceptualization, resources, writing–review and editing, supervision, and project administration. All authors contributed to the article and approved the submitted version.
This study was sponsored by Natural Science Foundation of Chongqing, China (2022NSCQ-MSX1111), “Through Train” for Doctors in Chongqing (sl202100000124), the National Natural Science Foundation of China (41771266), the Three Gorges’ follow-up scientific research project from Chongqing Municipal Bureau of Water Resources (No. 5000002021BF40001). PH is also supported by the “Light of West China” Program funded by the Chinese Academy of Sciences. China Postdoctoral Science Foundation (2021M703137), Chongqing Postdoctoral Science Foundation (cstc2021jcyj-bsh0080).
The authors declare that the research was conducted in the absence of any commercial or financial relationships that could be construed as a potential conflict of interest.
All claims expressed in this article are solely those of the authors and do not necessarily represent those of their affiliated organizations, or those of the publisher, the editors and the reviewers. Any product that may be evaluated in this article, or claim that may be made by its manufacturer, is not guaranteed or endorsed by the publisher.
The Supplementary material for this article can be found online at: https://www.frontiersin.org/articles/10.3389/fmicb.2023.1167353/full#supplementary-material
Adams, R. I., Miletto, M., Taylor, J. W., and Bruns, T. D. (2013). Dispersal in microbes: fungi in indoor air are dominated by outdoor air and show dispersal limitation at short distances. ISME J. 7, 1262–1273. doi: 10.1038/ismej.2013.28
Bach, E. M., Williams, R. J., Hargreaves, S. K., Yang, F., and Hofmockel, K. S. (2018). Greatest soil microbial diversity found in micro-habitats. Soil Biol. Biochem. 118, 217–226. doi: 10.1016/j.soilbio.2017.12.018
Bandyopadhyay, K. K., and Lal, R. (2014). Effect of land use management on greenhouse gas emissions from water stable aggregates. Geoderma 232-234, 363–372. doi: 10.1016/j.geoderma.2014.05.025
Banerjee, S., Kirkby, C. A., Schmutter, D., Bissett, A., Kirkegaard, J. A., and Richardson, A. E. (2016). Network analysis reveals functional redundancy and keystone taxa amongst bacterial and fungal communities during organic matter decomposition in an arable soil. Soil Biol. Biochem. 97, 188–198. doi: 10.1016/j.soilbio.2016.03.017
Barberán, A., Bates, S. T., Casamayor, E. O., and Fierer, N. (2012). Using network analysis to explore co-occurrence patterns in soil microbial communities. ISME J. 6, 343–351. doi: 10.1038/ismej.2011.119
Bernard, L., Chapuis-Lardy, L., Razafimbelo, T., Razafindrakoto, M., Pablo, A.-L., Legname, E., et al. (2012). Endogeic earthworms shape bacterial functional communities and affect organic matter mineralization in a tropical soil. ISME J. 6, 213–222. doi: 10.1038/ismej.2011.87
Bian, Q., Wang, X., Bao, X., Zhu, L., Xie, Z., Che, Z., et al. (2022). Exogenous substrate quality determines the dominant keystone taxa linked to carbon mineralization: evidence from a 30-year experiment. Soil Biol. Biochem. 169:108683. doi: 10.1016/j.soilbio.2022.108683
Bimüller, C., Kreyling, O., Kölbl, A., von Lützow, M., and Kögel-Knabner, I. (2016). Carbon and nitrogen mineralization in hierarchically structured aggregates of different size. Soil Tillage Res. 160, 23–33. doi: 10.1016/j.still.2015.12.011
Bodner, G., Scholl, P., and Kaul, H. P. (2013). Field quantification of wetting-drying cycles to predict temporal changes of soil pore size distribution. Soil Tillage Res. 133, 1–9. doi: 10.1016/j.still.2013.05.006
Brockett, B. F. T., Prescott, C. E., and Grayston, S. J. (2012). Soil moisture is the major factor influencing microbial community structure and enzyme activities across seven biogeoclimatic zones in western Canada. Soil Biol. Biochem. 44, 9–20. doi: 10.1016/j.soilbio.2011.09.003
Butterly, C. R., Marschner, P., McNeill, A. M., and Baldock, J. A. (2010). Rewetting CO2 pulses in Australian agricultural soils and the influence of soil properties. Biol. Fertil. Soils 46, 739–753. doi: 10.1007/s00374-010-0481-9
Chen, L., Jiang, Y., Liang, C., Luo, Y., Xu, Q., Han, C., et al. (2019). Competitive interaction with keystone taxa induced negative priming under biochar amendments. Microbiome 7:77. doi: 10.1186/s40168-019-0693-7
Chen, C., Meurk, C., Chen, J., Lv, M., Wen, Z., Jiang, Y., et al. (2014). Restoration design for three gorges reservoir shorelands, combining Chinese traditional agro-ecological knowledge with landscape ecological analysis. Ecol. Eng. 71, 584–597. doi: 10.1016/j.ecoleng.2014.07.008
Chen, J., Zhang, Y., Kuzyakov, Y., Wang, D., and Olesen, J. E. (2023). Challenges in upscaling laboratory studies to ecosystems in soil microbiology research. Glob. Chang. Biol. 29, 569–574. doi: 10.1111/gcb.16537
Chowdhury, T. R., Lee, J.-Y., Bottos, E. M., Brislawn, C. J., White, R. A., Bramer, L. M., et al. (2019). Metaphenomic responses of a native prairie soil microbiome to moisture perturbations. mSystems 4, e00061–e00019. doi: 10.1128/mSystems.00061-19
Cookson, W. R., Abaye, D. A., Marschner, P., Murphy, D. V., Stockdale, E. A., and Goulding, K. W. T. (2005). The contribution of soil organic matter fractions to carbon and nitrogen mineralization and microbial community size and structure. Soil Biol. Biochem. 37, 1726–1737. doi: 10.1016/j.soilbio.2005.02.007
Dacal, M., García-Palacios, P., Asensio, S., Wang, J., Singh, B. K., and Maestre, F. T. (2022). Climate change legacies contrastingly affect the resistance and resilience of soil microbial communities and multifunctionality to extreme drought. Funct. Ecol. 36, 908–920. doi: 10.1111/1365-2435.14000
Danevčič, T., Mandic-Mulec, I., Stres, B., Stopar, D., and Hacin, J. (2010). Emissions of CO2, CH4 and N2O from southern European peatlands. Soil Biol. Biochem. 42, 1437–1446. doi: 10.1016/j.soilbio.2010.05.004
De Nijs, E. A., Hicks, L. C., Leizeaga, A., Tietema, A., and Rousk, J. (2019). Soil microbial moisture dependences and responses to drying–rewetting: the legacy of 18 years drought. Glob. Chang. Biol. 25, 1005–1015. doi: 10.1111/gcb.14508
de Sosa, L. L., Glanville, H. C., Marshall, M. R., Prysor Williams, A., and Jones, D. L. (2018). Quantifying the contribution of riparian soils to the provision of ecosystem services. Sci. Total Environ. 624, 807–819. doi: 10.1016/j.scitotenv.2017.12.179
Denef, K., Six, J., Bossuyt, H., Frey, S. D., Elliott, E. T., Merckx, R., et al. (2001). Influence of dry–wet cycles on the interrelationship between aggregate, particulate organic matter, and microbial community dynamics. Soil Biol. Biochem. 33, 1599–1611. doi: 10.1016/S0038-0717(01)00076-1
Drury, C. F., Yang, X. M., Reynolds, W. D., and Tan, C. S. (2004). Influence of crop rotation and aggregate size on carbon dioxide production and denitrification. Soil Tillage Res. 79, 87–100. doi: 10.1016/j.still.2004.03.020
Edgar, R. C. (2013). UPARSE: highly accurate OTU sequences from microbial amplicon reads. Nat. Methods 10, 996–998. doi: 10.1038/nmeth.2604
Erktan, A., Or, D., and Scheu, S. (2020). The physical structure of soil: determinant and consequence of trophic interactions. Soil Biol. Biochem. 148:107876. doi: 10.1016/j.soilbio.2020.107876
Evans, S. E., and Wallenstein, M. D. (2014). Climate change alters ecological strategies of soil bacteria. Ecol. Lett. 17, 155–164. doi: 10.1111/ele.12206
Faitová, A.. (2021). Actinobacteria communities in natural and anthropogenic environments. Univerzita Karlova, Prague.
Fan, J., Jin, H., Zhang, C., Zheng, J., Zhang, J., and Han, G. (2021). Grazing intensity induced alternations of soil microbial community composition in aggregates drive soil organic carbon turnover in a desert steppe. Agric. Ecosyst. Environ. 313:107387. doi: 10.1016/j.agee.2021.107387
Fernández, R., Quiroga, A., Zorati, C., and Noellemeyer, E. (2010). Carbon contents and respiration rates of aggregate size fractions under no-till and conventional tillage. Soil Tillage Res. 109, 103–109. doi: 10.1016/j.still.2010.05.002
Fierer, N., Bradford, M. A., and Jackson, R. B. (2007). Toward an ecological classification of soil bacteria. Ecology 88, 1354–1364. doi: 10.1890/05-1839
Fierer, N., Schimel, J. P., and Holden, P. A. (2003). Influence of drying–rewetting frequency on soil bacterial community structure. Microb. Ecol. 45, 63–71. doi: 10.1007/s00248-002-1007-2
Fonte, S. J., Nesper, M., Hegglin, D., Velásquez, J. E., Ramirez, B., Rao, I. M., et al. (2014). Pasture degradation impacts soil phosphorus storage via changes to aggregate-associated soil organic matter in highly weathered tropical soils. Soil Biol. Biochem. 68, 150–157. doi: 10.1016/j.soilbio.2013.09.025
Gao, G.-F., Peng, D., Zhang, Y., Li, Y., Fan, K., Tripathi, B. M., et al. (2021). Dramatic change of bacterial assembly process and co-occurrence pattern in Spartina alterniflora salt marsh along an inundation frequency gradient. Sci. Total Environ. 755:142546. doi: 10.1016/j.scitotenv.2020.142546
Gorham, E. (1995). “The biogeochemistry of northern peatlands and its possible responses to global warming” in Biotic feedbacks in the global climate system: will the warming feed the warming. eds. G. M. Woodwell and F. T. MacKenzie (New York: Oxford University), 169–187.
Gupta, V. V. S. R., and Germida, J. J. (1988). Distribution of microbial biomass and its activity in different soil aggregate size classes as affected by cultivation. Soil Biol. Biochem. 20, 777–786. doi: 10.1016/0038-0717(88)90082-X
Hao, R. J., Li, Z. P., Che, Y. P., and Fang, H. L. (2008). Organic carbon mineralization in various size aggregates of paddy soil under aerobic and submerged conditions. Ying. Yong. Sheng. Xue Bao 19, 1944–1950.
He, H., Miao, Y., Gan, Y., Wei, S., Tan, S., Rask, K. A., et al. (2020). Soil bacterial community response to long-term land use conversion in Yellow River Delta. Appl. Soil Ecol. 156:103709. doi: 10.1016/j.apsoil.2020.103709
Herzog, C., Hartmann, M., Frey, B., Stierli, B., Rumpel, C., Buchmann, N., et al. (2019). Microbial succession on decomposing root litter in a drought-prone scots pine forest. ISME J. 13, 2346–2362. doi: 10.1038/s41396-019-0436-6
Holland, E. A., and Coleman, D. C. (1987). Litter placement effects on microbial and organic matter dynamics in an agroecosystem. Ecology 68, 425–433. doi: 10.2307/1939274
Jansson, J. K., and Hofmockel, K. S. (2020). Soil microbiomes and climate change. Nat. Rev. Microbiol. 18, 35–46. doi: 10.1038/s41579-019-0265-7
Jarvis, P., Rey, A., Petsikos, C., Wingate, L., Rayment, M., Pereira, J., et al. (2007). Drying and wetting of Mediterranean soils stimulates decomposition and carbon dioxide emission: the birch effect†. Tree Physiol. 27, 929–940. doi: 10.1093/treephys/27.7.929
Jiang, Y., Qian, H., Wang, X., Chen, L., Liu, M., Li, H., et al. (2018). Nematodes and microbial community affect the sizes and turnover rates of organic carbon pools in soil aggregates. Soil Biol. Biochem. 119, 22–31. doi: 10.1016/j.soilbio.2018.01.001
Jiang, M., Yang, N., Zhao, J., Shaaban, M., and Hu, R. (2021). Crop straw incorporation mediates the impacts of soil aggregate size on greenhouse gas emissions. Geoderma 401:115342. doi: 10.1016/j.geoderma.2021.115342
Johan, S., Clarholm, M., Sven, B., and Rosswall, T. (1986). Effects of moisture on soil microorganisms and nematodes: a field experiment. Microb. Ecol. 12, 217–230. doi: 10.1007/BF02011206
Jones, K. B., Slonecker, E. T., Nash, M. S., Neale, A. C., Wade, T. G., and Hamann, S. (2010). Riparian habitat changes across the continental United States (1972–2003) and potential implications for sustaining ecosystem services. Landsc. Ecol. 25, 1261–1275. doi: 10.1007/s10980-010-9510-1
Kan, Z. R., Ma, S. T., Liu, Q. Y., Liu, B. Y., Virk, A. L., Qi, J. Y., et al. (2020). Carbon sequestration and mineralization in soil aggregates under long-term conservation tillage in the North China plain. Catena 188:104428. doi: 10.1016/j.catena.2019.104428
Kõljalg, U., Nilsson, R. H., Abarenkov, K., Tedersoo, L., Taylor, A. F. S., Bahram, M., et al. (2013). Towards a unified paradigm for sequence-based identification of fungi. Mol. Ecol. 22, 5271–5277. doi: 10.1111/mec.12481
Lee, C. K., Barbier, B. A., Bottos, E. M., McDonald, I. R., and Cary, S. C. (2012). The inter-valley soil comparative survey: the ecology of dry valley edaphic microbial communities. ISME J. 6, 1046–1057. doi: 10.1038/ismej.2011.170
Leira, M., and Cantonati, M. (2008). Effects of water-level fluctuations on lakes: an annotated bibliography. Hydrobiologia 613, 171–184. doi: 10.1007/s10750-008-9465-2
Lin, Y., Ye, G., Kuzyakov, Y., Liu, D., Fan, J., and Ding, W. (2019). Long-term manure application increases soil organic matter and aggregation, and alters microbial community structure and keystone taxa. Soil Biol. Biochem. 134, 187–196. doi: 10.1016/j.soilbio.2019.03.030
Liu, X. J. A., Hayer, M., Mau, R. L., Schwartz, E., Dijkstra, P., and Hungate, B. A. (2021). Substrate stoichiometric regulation of microbial respiration and community dynamics across four different ecosystems. Soil Biol. Biochem. 163:108458. doi: 10.1016/j.soilbio.2021.108458
Magoč, T., and Salzberg, S. L. (2011). FLASH: fast length adjustment of short reads to improve genome assemblies. Bioinformatics 27, 2957–2963. doi: 10.1093/bioinformatics/btr507
Malik, A. A., Martiny, J. B. H., Brodie, E. L., Martiny, A. C., Treseder, K. K., and Allison, S. D. (2020). Defining trait-based microbial strategies with consequences for soil carbon cycling under climate change. ISME J. 14, 1–9. doi: 10.1038/s41396-019-0510-0
Mangalassery, S., Sjögersten, S., Sparkes, D. L., Sturrock, C. J., and Mooney, S. J. (2013). The effect of soil aggregate size on pore structure and its consequence on emission of greenhouse gases. Soil Tillage Res. 132, 39–46. doi: 10.1016/j.still.2013.05.003
Manzoni, S., Schimel, J. P., and Porporato, A. (2012). Responses of soil microbial communities to water stress: results from a meta-analysis. Ecology 93, 930–938. doi: 10.1890/11-0026.1
Márquez, C. O., Garcia, V. J., Cambardella, C. A., Schultz, R. C., and Isenhart, T. M. (2004). Aggregate-size stability distribution and soil stability. Soil Sci. Soc. Am. J. 68, 725–735. doi: 10.2136/sssaj2004.7250
McGinnis, M. R., and Tyring, S. K.. (1996). Introduction to mycology. Medical microbiology. 4th Edn. Galveston, TX: University of Texas Medical Branch at Galveston.
Meisner, A., Bååth, E., and Rousk, J. (2013). Microbial growth responses upon rewetting soil dried for four days or one year. Soil Biol. Biochem. 66, 188–192. doi: 10.1016/j.soilbio.2013.07.014
Navas, M., Martín-Lammerding, D., Hontoria, C., Ulcuango, K., and Mariscal-Sancho, I. (2021). The distinct responses of bacteria and fungi in different-sized soil aggregates under different management practices. Eur. J. Soil Sci. 72, 1177–1189. doi: 10.1111/ejss.12997
Nelson, D. W., and Sommers, L. E. (1996). “Total carbon, organic carbon, and organic matter” in Methods of soil analysis. eds. D. L. Sparks, A. L. Page, P. A. Helmke, R. H. Loeppert, P. N. Soltanpour, and M. A. Tabatabai (Madison: SSSA Book Series), 961–1010.
Noellemeyer, E., Frank, F., Alvarez, C., Morazzo, G., and Quiroga, A. (2008). Carbon contents and aggregation related to soil physical and biological properties under a land-use sequence in the semiarid region of Central Argentina. Soil Tillage Res. 99, 179–190. doi: 10.1016/j.still.2008.02.003
Peralta, R. M., Ahn, C., and Gillevet, P. M. (2013). Characterization of soil bacterial community structure and physicochemical properties in created and natural wetlands. Sci. Total Environ. 443, 725–732. doi: 10.1016/j.scitotenv.2012.11.052
Qiu, L., Zhang, Q., Zhu, H., Reich, P. B., Banerjee, S., van der Heijden, M. G. A., et al. (2021). Erosion reduces soil microbial diversity, network complexity and multifunctionality. ISME J. 15, 2474–2489. doi: 10.1038/s41396-021-00913-1
Rabbi, S. M. F., Wilson, B. R., Lockwood, P. V., Daniel, H., and Young, I. M. (2014). Soil organic carbon mineralization rates in aggregates under contrasting land uses. Geoderma 216, 10–18. doi: 10.1016/j.geoderma.2013.10.023
Razafimbelo, T. M., Albrecht, A., Oliver, R., Chevallier, T., Chapuis-Lardy, L., and Feller, C. (2008). Aggregate associated-C and physical protection in a tropical clayey soil under Malagasy conventional and no-tillage systems. Soil Tillage Res. 98, 140–149. doi: 10.1016/j.still.2007.10.012
Rusalimova, O., and Barsukov, P. (2006). “Decomposition of labile and recalcitrant soil organic matter of Gleyic Cryosols in permafrost region of Siberia” in Symptom of environmental change in Siberian permafrost region. eds. R. Hatano and G. Guggenberger (Sapporo: Hokkaido University Press), 93–102.
Schimel, J. P. (2018). Life in dry soils: effects of drought on soil microbial communities and processes. Annu. Rev. Ecol. Evol. Syst. 49, 409–432. doi: 10.1146/annurev-ecolsys-110617-062614
Sey, B. K., Manceur, A. M., Whalen, J. K., Gregorich, E. G., and Rochette, P. (2008). Small-scale heterogeneity in carbon dioxide, nitrous oxide and methane production from aggregates of a cultivated sandy-loam soil. Soil Biol. Biochem. 40, 2468–2473. doi: 10.1016/j.soilbio.2008.05.012
She, W., Yang, J., Wu, G., and Jiang, H. (2022). The synergy of environmental and microbial variations caused by hydrologic management affects the carbon emission in the three gorges reservoir. Sci. Total Environ. 821:153446. doi: 10.1016/j.scitotenv.2022.153446
Silvola, J., Alm, J., Ahlholm, U., Nykanen, H., and Martikainen, P. J. (1996). CO2 fluxes from peat in boreal mires under varying temperature and moisture conditions. J. Ecol. 84, 219–228. doi: 10.2307/2261357
Six, J., Bossuyt, H., Degryze, S., and Denef, K. (2004). A history of research on the link between (micro)aggregates, soil biota, and soil organic matter dynamics. Soil Tillage Res. 79, 7–31. doi: 10.1016/j.still.2004.03.008
Six, J., Elliott, E. T., and Paustian, K. (1999). Aggregate and soil organic matter dynamics under conventional and no-tillage systems. Soil Sci. Soc. Am. J. 63, 1350–1358. doi: 10.2136/sssaj1999.6351350x
Tait, E., Carman, M., and Sievert, S. M. (2007). Phylogenetic diversity of bacteria associated with ascidians in eel pond (woods hole, Massachusetts, USA). J. Exp. Mar. Biol. Ecol. 342, 138–146. doi: 10.1016/j.jembe.2006.10.024
Tisdall, J. M., and Oades, J. M. (1982). Organic matter and water-stable aggregates in soils. J. Soil Sci. 33, 141–163. doi: 10.1111/j.1365-2389.1982.tb01755.x
Tiwari, R., Kumar, K., Singh, S., Nain, L., and Shukla, P. (2016). Molecular detection and environment-specific diversity of Glycosyl hydrolase family 1 β-Glucosidase in different habitats. Front. Microbiol. 7:1597. doi: 10.3389/fmicb.2016.01597
Trivedi, P., Delgado-Baquerizo, M., Jeffries, T. C., Trivedi, C., Anderson, I. C., Lai, K., et al. (2017). Soil aggregation and associated microbial communities modify the impact of agricultural management on carbon content. Environ. Microbiol. 19, 3070–3086. doi: 10.1111/1462-2920.13779
Umair, M., Sun, N., Du, H., Hui, N., Altaf, M., Du, B., et al. (2020). Bacterial communities are more sensitive to water addition than fungal communities due to higher soil K and Na in a degraded karst ecosystem of southwestern China. Front. Microbiol. 11:562546. doi: 10.3389/fmicb.2020.562546
Upton, R. N., Bach, E. M., and Hofmockel, K. S. (2019). Spatio-temporal microbial community dynamics within soil aggregates. Soil Biol. Biochem. 132, 58–68. doi: 10.1016/j.soilbio.2019.01.016
Voříšková, J., and Baldrian, P. (2013). Fungal community on decomposing leaf litter undergoes rapid successional changes. ISME J. 7, 477–486. doi: 10.1038/ismej.2012.116
Wagg, C., Schlaeppi, K., Banerjee, S., Kuramae, E. E., and van der Heijden, M. G. A. (2019). Fungal-bacterial diversity and microbiome complexity predict ecosystem functioning. Nat. Commun. 10:4841. doi: 10.1038/s41467-019-12798-y
Wang, X., Bian, Q., Jiang, Y., Zhu, L., Chen, Y., Liang, Y., et al. (2021). Organic amendments drive shifts in microbial community structure and keystone taxa which increase C mineralization across aggregate size classes. Soil Biol. Biochem. 153:108062. doi: 10.1016/j.soilbio.2020.108062
Wang, B., Brewer, P. E., Shugart, H. H., Lerdau, M. T., and Allison, S. D. (2019). Soil aggregates as biogeochemical reactors and implications for soil–atmosphere exchange of greenhouse gases–a concept. Glob. Chang. Biol. 25, 373–385. doi: 10.1111/gcb.14515
Wang, D., Chi, Z., Yue, B., Huang, X., Zhao, J., Song, H., et al. (2020). Effects of mowing and nitrogen addition on the ecosystem C and N pools in a temperate steppe: a case study from northern China. Catena 185:104332. doi: 10.1016/j.catena.2019.104332
Wang, Q., Garrity, G. M., Tiedje, J. M., and Cole, J. R. (2007). Naïve Bayesian classifier for rapid assignment of rRNA sequences into the new bacterial taxonomy. Appl. Environ. Microbiol. 73, 5261–5267. doi: 10.1128/AEM.00062-07
Wang, Z., Li, Y., Chang, S. X., Zhang, J., Jiang, P., Zhou, G., et al. (2014). Contrasting effects of bamboo leaf and its biochar on soil CO2 efflux and labile organic carbon in an intensively managed Chinese chestnut plantation. Biol. Fertil. Soils 50, 1109–1119. doi: 10.1007/s00374-014-0933-8
Wang, G., Wang, Y., Yang, P., Luo, H., Huang, H., Shi, P., et al. (2010). Molecular detection and diversity of xylanase genes in alpine tundra soil. Appl. Microbiol. Biotechnol. 87, 1383–1393. doi: 10.1007/s00253-010-2564-9
Wilpiszeski, R. L., Aufrecht, J. A., Retterer, S. T., Sullivan, M. B., Graham, D. E., Pierce, E. M., et al. (2019). Soil aggregate microbial communities: towards understanding microbiome interactions at biologically relevant scales. Appl. Environ. Microbiol. 85, e00324–e00319. doi: 10.1128/AEM.00324-19
Xiang, Y., Wang, Y., Zhang, C., Shen, H., and Wang, D. (2018). Water level fluctuations influence microbial communities and mercury methylation in soils in the three gorges reservoir, China. J. Environ. Sci. 68, 206–217. doi: 10.1016/j.jes.2018.03.009
Ye, F., Ma, M. H., Wu, S. J., Jiang, Y., Zhu, G. B., Zhang, H., et al. (2019). Soil properties and distribution in the riparian zone: the effects of fluctuations in water and anthropogenic disturbances. Eur. J. Soil Sci. 70, 664–673. doi: 10.1111/ejss.12756
Ye, F., Wang, X., Wang, Y., Wu, S., Wu, J., and Hong, Y. (2021). Different pioneer plant species have similar rhizosphere microbial communities. Plant Soil 464, 165–181. doi: 10.1007/s11104-021-04952-7
Zhang, G. (2010). Changes of soil labile organic carbon in different land uses in Sanjiang plain, Heilongjiang Province. Chin. Geogr. Sci. 20, 139–143. doi: 10.1007/s11769-010-0139-4
Zhang, L., Adams, J. M., Dumont, M. G., Li, Y., Shi, Y., He, D., et al. (2019). Distinct methanotrophic communities exist in habitats with different soil water contents. Soil Biol. Biochem. 132, 143–152. doi: 10.1016/j.soilbio.2019.02.007
Zhang, H., Ding, W., He, X., Yu, H., Fan, J., and Liu, D. (2014). Influence of 20–year organic and inorganic fertilization on organic carbon accumulation and microbial community structure of aggregates in an intensively cultivated sandy loam soil. PLoS One 9:e92733. doi: 10.1371/journal.pone.0092733
Zhang, C., Liu, G., Xue, S., and Wang, G. (2016). Soil bacterial community dynamics reflect changes in plant community and soil properties during the secondary succession of abandoned farmland in the loess plateau. Soil Biol. Biochem. 97, 40–49. doi: 10.1016/j.soilbio.2016.02.013
Zhang, Q., Shao, M. A., Jia, X., and Zhang, C. (2018). Understory vegetation and drought effects on soil aggregate stability and aggregate-associated carbon on the loess plateau in China. Soil Sci. Soc. Am. J. 82, 106–114. doi: 10.2136/sssaj2017.05.0145
Zheng, H., Yang, T., Bao, Y., He, P., Yang, K., Mei, X., et al. (2021). Network analysis and subsequent culturing reveal keystone taxa involved in microbial litter decomposition dynamics. Soil Biol. Biochem. 157:108230. doi: 10.1016/j.soilbio.2021.108230
Zheng, W., Zhao, Z., Gong, Q., Zhai, B., and Li, Z. (2018). Effects of cover crop in an apple orchard on microbial community composition, networks, and potential genes involved with degradation of crop residues in soil. Biol. Fertil. Soils 54, 743–759. doi: 10.1007/s00374-018-1298-1
Zhou, H., Gao, Y., Jia, X., Wang, M., Ding, J., Cheng, L., et al. (2020). Network analysis reveals the strengthening of microbial interaction in biological soil crust development in the mu us Sandy land, northwestern China. Soil Biol. Biochem. 144:107782. doi: 10.1016/j.soilbio.2020.107782
Zhu, K., Li, W., Yang, S., Ran, Y., Lei, X., Ma, M., et al. (2022a). Intense wet-dry cycles weakened the carbon sequestration of soil aggregates in the riparian zone. Catena 212:106117. doi: 10.1016/j.catena.2022.106117
Zhu, K., Ma, M., Ran, Y., Liu, Z., Wu, S., and Huang, P. (2020). In mitigating CO2 emission in the reservoir riparian: the influences of land use and the dam-triggered flooding on soil respiration. Soil Tillage Res. 197:104522. doi: 10.1016/j.still.2019.104522
Keywords: soil respiration, soil aggregates, water regime changes, microbial community, co-occurrence network, keystone taxa
Citation: Zhu K, Jia W, Mei Y, Wu S and Huang P (2023) Shift from flooding to drying enhances the respiration of soil aggregates by changing microbial community composition and keystone taxa. Front. Microbiol. 14:1167353. doi: 10.3389/fmicb.2023.1167353
Received: 16 February 2023; Accepted: 19 April 2023;
Published: 12 May 2023.
Edited by:
Yu Luo, Zhejiang University, ChinaReviewed by:
Dong Wang, Henan University, ChinaCopyright © 2023 Zhu, Jia, Mei, Wu and Huang. This is an open-access article distributed under the terms of the Creative Commons Attribution License (CC BY). The use, distribution or reproduction in other forums is permitted, provided the original author(s) and the copyright owner(s) are credited and that the original publication in this journal is cited, in accordance with accepted academic practice. No use, distribution or reproduction is permitted which does not comply with these terms.
*Correspondence: Ping Huang, aHVhbmdwaW5nQGNpZ2l0LmFjLmNu
Disclaimer: All claims expressed in this article are solely those of the authors and do not necessarily represent those of their affiliated organizations, or those of the publisher, the editors and the reviewers. Any product that may be evaluated in this article or claim that may be made by its manufacturer is not guaranteed or endorsed by the publisher.
Research integrity at Frontiers
Learn more about the work of our research integrity team to safeguard the quality of each article we publish.