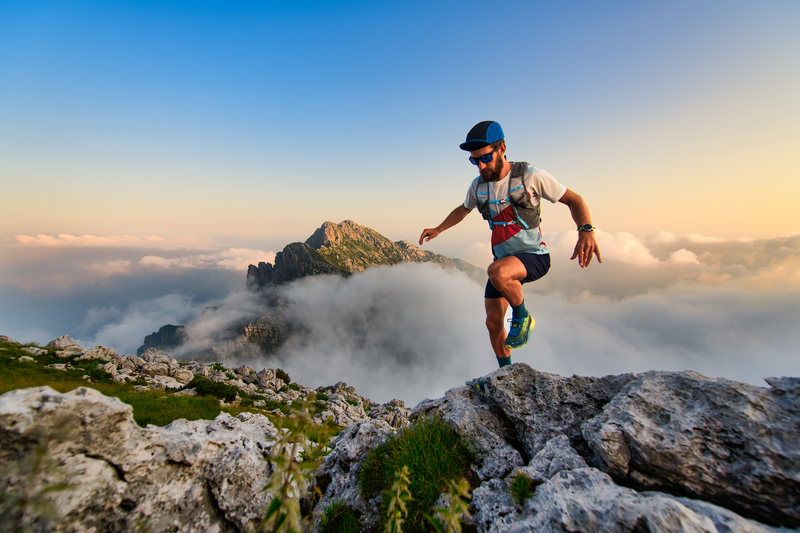
94% of researchers rate our articles as excellent or good
Learn more about the work of our research integrity team to safeguard the quality of each article we publish.
Find out more
ORIGINAL RESEARCH article
Front. Microbiol. , 09 June 2023
Sec. Microbe and Virus Interactions with Plants
Volume 14 - 2023 | https://doi.org/10.3389/fmicb.2023.1127606
Introduction: Viruses generally cause disease, but some viruses may be beneficial as resident regulators of their hosts or host microbiomes. Plant-associated viruses can help plants survive by increasing stress tolerance or regulating endophytic communities. The goal of this study was to characterize endophytic virus communities in banana and plantain (Musa spp.) genotypes, including cultivated and wild species, to assess virome repertoires and detect novel viruses.
Methods: DNA viral communities were characterized by shotgun sequencing of an enriched endosphere extract from leaves and roots or corm of 7 distinct Musa genotypes (M. balbisiana, Thai Black, M. textilis, M. sikkimensis, Dwarf Cavendish, Williams Hybrid, and FHIA-25 Hybrid).
Results: Results showed abundant virus-like contigs up to 108,191 bp long with higher relative abundance in leaves than roots. Analyses predicted 733 phage species in 51 families, with little overlap in phage communities among plants. Phage diversity was higher in roots and in diploid wild hosts. Ackermanniviridae and Rhizobium phage were generally the most abundant taxa. A Rhizobium RR1-like phage related to a phage of an endophytic tumor-causing rhizobium was found, bearing a holin gene and a partial Shiga-like toxin gene, raising interest in its potential to regulate endophytic Rhizobiaceae. Klebsiella phages were of interest for possible protection against Fusarium wilt, and other phages were predicted with potential to regulate Erwinia, Pectobacterium, and Ralstonia-associated diseases. Although abundant phage-containing contigs were functionally annotated, revealing 1,038 predicted viral protein domains, gene repertoires showed high divergence from database sequences, suggesting novel phages in these banana cultivars. Plant DNA viruses included 56 species of Badnavirus and 26 additional non-Musa plant viruses with distributions that suggested a mixture of resident and transient plant DNA viruses in these samples.
Discussion: Together, the disparate viral communities in these plants from a shared environment suggest hosts drive the composition of these virus communities. This study forms a first step in understanding the endophytic virome in this globally important food crop, which is currently threatened by fungal, bacterial, and viral diseases.
Pathogenic plant viruses have received much attention; however, some viruses can play important beneficial roles. For example, various DNA and RNA viruses appear to help plants survive restrictive environmental conditions and increase biotic and abiotic stress tolerance (Schoelz and Stewart, 2018; Seo and Kweon, 2019; Pratama et al., 2020; Siddique, 2020). Moreover, interactions between viruses, microbes, and hosts can create complex dynamics (Knief et al., 2012; Van Belleghem et al., 2018; Rodriguez et al., 2019) in which viruses may serve as resident regulators impacting hosts or their endophytic populations to alter net secondary metabolite biosynthesis (Roossinck et al., 2015; Seo and Kweon, 2019). Using whole-genome shotgun genomics (WGS) can reveal hidden viral diversity within plants (Forero-Junco et al., 2021). However, most endophyte WGS studies focus on bacterial or fungal communities, with limited analysis of DNA viruses (Regalado et al., 2020; Akinola et al., 2021; Soto-Giron et al., 2021; Fadiji et al., 2021a,b).
Among plant virome communities, bacteriophage (hereafter phage) communities may be the least studied but most impactful on host biology (Dion et al., 2020). Phages may impact hosts by controlling bacterial diseases or modifying beneficial bacterial communities (Buttimer et al., 2017; Koskella and Taylor, 2018; Morella et al., 2018; Koskella, 2019). Using phages as a therapy against pathogenic bacteria has been widely explored (Tian et al., 2015; Addy et al., 2016; Murthi et al., 2021), with some studies indicating plant bacterial diseases may be efficiently controlled by phages (Koskella and Taylor, 2018). For example, onion leaf blight caused by Xanthomonas axonopodis pv. allii showed a 50% reduction in disease severity when phages were applied weekly or biweekly (Lang et al., 2007). Peach bacterial spot caused by X. campestris pv. pruni was controlled by spraying trees with phages (Saccardi et al., 1993). Impacts of phages on beneficial bacteria in animal guts (Federici et al., 2021) and in soils (Kuzyakov and Mason-Jones, 2018; Blazanin and Turner, 2021) are well-established, but few studies have examined phage effects within plant microbiomes, although at least one study has shown that phages can alter phyllosphere diversity (Morella et al., 2018).
Plant dsDNA viruses, many of which alternate between circulating infectious virions and integrated endogenous virus elements (EVEs), are also inadequately studied (Gayral et al., 2008; Flynn and Moreau, 2019; Tripathi et al., 2019). Some EVEs can be activated and lead to systemic infection of the host when the plants are stressed (Muller et al., 2021), while others are ‘molecular fossils’ in domesticated plants (Jones et al., 1999). Latent plant viruses can benefit host plants (Pagán et al., 2012; Tripathi et al., 2019) by contributing to plant virus resistance through induction of transcriptional or post-transcriptional gene silencing of homologous sequences (Harper et al., 2002). The genomes of bitter orange (Poncirus trifoliata), potato (Solanum tuberosum), rice (Oryza sativa), tomato (Lycopersicon sp.), petunia (Petunia sp.), tobacco (Nicotiana sp.), and banana (Musa spp.) have been shown to harbor such integrates. Banana streak Obino l’Ewai virus has severely hindered international banana (Musa spp.) breeding programs, as new hybrids become infected through the integrated viruses from the wild M. balbisiana (BB genotype; Geering et al., 2005). Insertion of a badnavirus promoter next to an endogenous plant gene may change its transcription levels and alter the tissue specificity of expression, hindering banana breeding programs (Matzke et al., 2004).
Banana and plantain (Musa spp.) are a model group for which natural endophytic viromes have not been well-characterized. Banana is an important food staple and commercial product in over 130 countries (FAO, 2016). While common pathogenic viruses, such as banana streak virus (BSV), banana bunchy top virus (BBTV), and banana bract mosaic virus (BBrMV) have been studied extensively (Dale, 1987; Bateson and Dale, 1995; Lockhart, 1995), natural viral community variance is not well understood. Although plant tissue and cultivar shape microbiomes (Hirsch and Mauchline, 2012), the host impact on plant viromes is less clear (Koskella and Taylor, 2018; Harrison and Griffin, 2020). Furthermore, domestication appears to deplete bacterial and fungal microbiomes (Xue et al., 2015; Köberl et al., 2017), but its impacts on banana viromes are unknown.
The current study examined DNA viruses co-collected with enriched microbiomes from Musa spp. grown in sympatry, to assess the impacts of hosts on viral communities. Comparing viromes from endophytic tissues of both roots and leaves of wild Musa diploid genotypes (Musa balbisiana, Musa textilis, and Musa sikkimensis) and domesticated triploid genotypes (Dwarf Cavendish, Williams Hybrid, and FHIA-25 Hybrid), we identified putative phages and endogenous viral elements, illuminating distinct community profiles and predicted numerous potentially novel DNA viruses.
To compare endophytic virus-like sequences to estimate viral communities from different banana (Musa spp.) genotypes, seven cultivars and species of banana were collected from a farm in Homestead, Florida (Table 1). While some plants at the farm appeared to have low-level symptoms suggesting the presence of Black sigatoka (Mycosphaerella fijiensis) disease, the farm used standard intermittent control measures to limit this and other pathogens. Collected plants used in this study appeared to be symptom-free before, during, and after collection. To assess the differences in viruses present in above-ground and below-ground tissues, two samples were taken from each plant: one from the leaves and another from the root or corm of the same plant. Plant samples were first washed in tap water for 10 min to remove any loose material. Then tissues were surface-sterilized (Refaei et al., 2011) by immersing them for 1 min in 70% ethanol, then for 3 min in 2.5% sodium hypochlorite solution, followed by 1 min in 70% ethanol and finally rinsed three times with sterile distilled water.
Table 1. Banana (Musa spp.) sampled in this study, showing species or cultivar name, genotype, tissues sampled, and domestication status.
To reduce sequence yield of non-target plant genomes while obtaining microbial endophytes and potentially their associated viruses, we used a culture-free filtration and density gradient method previously used for soybean (Ikeda et al., 2009). One of the goals here was to examine for the first time whether this method could recover plant and endophyte DNA viromes while recovering cellular endophytic microbiota. Briefly, the method used 100 g of each plant sample, which was homogenized in a sterilized blender in 400 mL of ice-cold “BCE” buffer (50 mM Tris–HCl pH 7.5, 1% Triton X-100, 2 mM 2-mercaptoethanol). To preserve cell and DNA integrity, all steps were performed at 4°C. Resulting homogenate was filtered through a UV-sterilized Miracloth (EMD Millipore) rayon-polyester mesh, then centrifuged for 5 min at 500xg. Flow-through was transferred to sterile tubes and centrifuged for 20 min at 5,000× g. Resulting supernatant was discarded and pellets were resuspended in 50 mL of BCE. This slurry was then filtered through a UV-sterilized Kimwipe (Kimberly-Clark) and the filtrate was centrifuged for 10 min at 5,000× g. Resulting supernatants were discarded and pellets were resuspended in 35 mL of BCE. This Kimwipe filtration was repeated. Final pellets were resuspended in 6 mL of 50 mM Tris–HCl (pH 7.5). Each 1 mL aliquot was pipetted over 4 mL of Nycodenz® (3.2 g of Nycodenz® + 4 mL of 50 mM Tris–HCl, pH 7.5), then gradients were centrifuged for 40 min at 5,000× g to collect a layer of microbial cells at the interface. While this method may result in pelleting of free viral particles; our goal was to see whether virome members such as phages associated with bacteria or viruses associated with plants/fungi may be retained in this microbiome layer.
DNA was isolated from the enriched microbial layers using the DNeasy Blood and Tissue Kit from QIAGEN (Valencia, CA) following the manufacturer’s directions. DNA quantity and quality were assessed on the Nanodrop spectrophotometer. Shotgun metagenomic libraries were prepared from ~0.5 to 1 μg of DNA with the QIAseq FX 96 DNA Library Kit (Valencia, CA). Libraries were checked for quality on the TapeStation 2200 (Agilent) then normalized and pooled for sequencing. Sequencing was performed at the Center for Biotechnology and Genomics (CGB) at Texas Tech University and Genewiz, Inc. (NJ) with Illumina paired-end reads of 105 and 150 bp, respectively.
Paired-end read overlaps were merged using PEAR v0.9.1 (Zhang et al., 2014), then Trimmomatic v0.38 (Bolger et al., 2014) was used to trim and filter reads prior to assembly with metaSPAdes in the SPAdes software v3.13.0 (Bankevich et al., 2012; Nurk et al., 2017), with read-error correction and using kmers 21, 33, 45, 59, 73, and 99. Assembly statistics were calculated using QUAST v5.0. (Gurevich et al., 2013). Initial classification of taxa was performed by using DIAMOND v2.0.9 (Buchfink et al., 2014) blastx on assembled contigs against the NCBI nr database (accessed December 2020) with options sensitive, minimum e-value 1e-05, block size 16 and single chunk used for the seed index. Reads were mapped to the taxon-annotated contigs using BWA-MEM to assess relative abundances and normalized for relative average genome sizes among groups (e.g., banana haploid genome 523 Mbp, fungi 40 Mbp, bacteria/archaea 4 Mbp, viruses 60 kbp). All contigs were then searched for virus-like regions using a more sensitive custom two-part blastn approach, as follows. First, to generate a custom viral nucleotide blast database consisting of 3,250,606 viral sequences, all viral sequences on NCBI were downloaded (db date 02 January 2020) to generate a custom viral nucleotide blast database consisting of 3,250,606 viral sequences. Then, assembled contigs were analyzed against this custom virus database with blastn in BLAST+ v2.10.0 (Camacho et al., 2009) with the following parameters: e-value cutoff 10, maximum target sequences 500, keeping all blastn hits for the next step. Fasta sequences for all initial blastn hits were trimmed to only the hit regions using samtools faidx (Li et al., 2009), and resulting virus-like regions were subjected to a second blast to the full NCBI nucleotide database (nt) with e-value cutoff 0.01 and maximum target sequences 20, saving only contigs with top bitscore matching virus, phage, or prophage/provirus in the taxonomic field or elsewhere in the text of the blast hit.
To more sensitively and rigorously search for viruses, we employed recently validated virus identification pipelines (Gregory et al., 2019; Pratama et al., 2021), using assembled contigs as input and performing an initial virus scan using VirSorter2 v.2.2.1 (Roux et al., 2015; Guo et al., 2021), then assessing quality and completeness of the VirSorter2 outputs using CheckV (Nayfach et al., 2021), trimming any host DNA before repeating VirSorter2 with modified parameters. For the initial VirSorter2, we used a loose cutoff of 0.5 for maximal sensitivity with minimal length 500 bp, and limited the search to dsDNA and ssDNA phage. CheckV analysis of VirSorter2 outputs used minimal score cutoff of 0.5. Resulting viral contigs were run in VirSorter2 with a minimum score of 0.9 to detect more confident contigs, using parameters --seqname-suffix-off and --viral-gene-enrich-off. Next, potential duplications in the form of populations of similar viral fragments were removed using the deduplication program dRep (Olm et al., 2017) with parameters -con 10 and -sa 0.95. Dereplicated contigs were then taxonomically classified by two methods shown recently to give slightly different results (Lee et al., 2022): vConTACT2 (Bin Jang et al., 2019) which uses a distance-based hierarchical clustering; and Genome Detective Virus Tool (GDV) v.2.52 (Vilsker et al., 2019). GDV virus annotations were classified to higher taxonomic levels by referencing NCBI taxonomy databases (“fullnamelineage.dmp” downloaded April 2023).
The relative abundance of each predicted virus in each sample was estimated based on kmer coverage of each contig matched to a virus in blast or with vConTACT2 or Genome Detective Virus Tool (Supplementary Table 1), modified by the following equation: C = (CK R)/(R − K + 1), where C is absolute coverage, CK is kmer coverage estimated by metaSPAdes, K is the length of kmers used in metaSPAdes, and R is read length. Before analysis of diversity, alpha rarefaction curves were generated from total reads not mapped to Musa spp. (i.e., from reads derived from the microbiome fraction). For samples with sufficient data based on asymptote in rarefaction curves, data were then normalized to adjust for differences in sequencing effort among samples as follows: (a) for phages, this normalization was performed by scaling each sample’s coverage per contig to the highest sample read count from reads that were not mapped to Musa spp., under the premise that phage abundance should be linked to microbiome abundance rather than plant DNA (see right column in Supplementary Table 2), or (b) for endogenous viruses, this normalization was scaled to the coverage to the highest sample read count from reads that mapped to Musa spp. (see second to right column in Supplementary Table 2), under the premise that endogenous virus abundance should be linked to host plant DNA levels. Relative abundances were plotted as heatmaps. Viral community overlap among samples was depicted with the proportional Venn drawing tool nVenn (Pérez-Silva et al., 2018). Alpha diversity analysis was performed in R to assess Shannon diversity with the Hutcheson t-test using “ecolTest” v. 0.0.1 (Hutcheson, 1970; Zar, 2010).
Basic initial gene annotation of virus-like contigs identified either by the two-part blast beginning with the virus database or by the DIAMOND blast described above were annotating in Prokka v.1.14.6 (Seemann, 2014) using the parameter—kingdom Viruses. Prokka uses Prodigal for ab initio gene prediction, HMMER3 for protein family profiles, and BLAST+ for comparative annotation. A more refined virus-specific gene annotation was performed on the output viral contigs from the VirSorter2-dRep-GDV pipeline. This functional annotation used the DRAM-v software (Shaffer et al., 2020) using the parameter -min_contig_size 1,000.
A small subset of longer contigs were examined for orthologs and gene synteny as follows. Annotated viral gene orthologs were determined using Roary v3.13.0 (Page et al., 2015) on gff outputs from Prokka, with parameters -e for codon-aware alignment in PRANK (Löytynoja, 2014) and -i 50 to detect distant orthologs. Annotated contigs were then imported into Geneious Prime v2020.0.4 (Biomatters, Ltd), and subjected to between-sample assembly using the Geneious de novo assembler with default parameters. Resulting contigs were analyzed using the online platform PHASTER (Zhou et al., 2011; Arndt et al., 2016). Output phage/prophage were re-analyzed with DIAMOND blastx to predict taxonomy and function of phage-like regions. For the two longest genes in these phages, phylogenetic analysis was performed. First, blastn to the nt database at NCBI was used to find and download homologous genes. These homologs were then aligned in Geneious with CLUSTAL Omega, and phylogenetic analysis was performed using maximum likelihood (ML) phylogeny reconstruction was performed in RAxML v4.0 (Stamatakis, 2014) using the GTR Gamma nucleotide model, with rate heterogeneity alpha estimated, and with rapid bootstrapping and search for the best-scoring ML tree (−f a -x 1) assessing bootstrap support from 1,000 replicates. Annotated phage or prophage regions were then compared for synteny and similarity to the most similar full-length sequences available in GenBank using Easyfig v2.2.2 (Sullivan et al., 2011).
After overlap merging, filtering, and trimming, the total enriched microbiome layers yielded a total of ~576 million high-quality reads from 14 samples (Supplementary Table 2), with a range between 13 and 84 million reads per sample. Metagenomic assemblies from these reads produced a total of ~5 million contigs, with between ~4,000 and ~300,000 contigs per sample with assembly N50s of 670 to 9,897 per sample (Supplementary Table 2). Rarefaction curves indicated sufficient sampling of microbiomes for each genotype, except for leaves of Williams Hybrid, which appeared undersampled and did not have detectable viruses.
Initial taxonomic classification of the enriched microbiome layers, using DIAMOND blastx and controlling for average genome sizes showed these microbiomes had very few plant or fungal genomes (0.11 and 0.28%, respectively) compared to bacterial and viral genomes (92.4% and 7.2%, respectively) on average. There were consistently higher relative levels of predicted virus matches in leaves than in roots (Figure 1) and plant genotypes differed slightly in these proportions. Notably, 20.5% of all reads before normalizing for genome sizes could not be assigned to any taxonomic group, even at the kingdom level. It is unknown if these reads contain additional undescribed viruses.
Figure 1. Relative proportion of reads from extracted Musa microbiome layers that mapped to plant, fungi, bacteria and archaea, and viruses, controlling for differences in average genome sizes and showing reads mapped to contigs that could not be classified to any taxon. Average genome sizes used: banana haploid genome 523 Mbp, fungi 40 Mbp, bacteria/archaea 4 Mbp, viruses 60 kbp. Samples: BBC and BBL = Musa balbisiana Thai Black corm and leaf, DCR and DCL = Dwarf Cavendish root, and leaf, FHC and FHL = FHIA-25 corm and leaf, MBR and MBL = Musa balbisiana root and leaf, MSR and MSL = Musa sikkimensis root and leaf, MTC and MTL = Musa textilis corm and leaf, WHC and WHL = Williams Hybrid corm and leaf.
The results of the two-step blastn of assembled contigs to viral databases showed 864 contigs with highest blast hits to viruses, comprising ~1.13 Mbp of assembled sequence length with maximum contig length 53,615 bp (Table 2). Among these, 156 contigs matched phage and 708 contigs matched plant DNA viruses of the family Caulimoviridae, which likely comprise both endogenous (i.e., integrated) sequences such as endogenous pararetroviruses, and episomal (i.e., nonintegrated) viruses. In total, read counts across all samples (non-normalized across samples) at the order level mostly matched Ortervirales (95.16% of reads) which are a broad group containing almost all dsDNA viruses that replicate through an RNA intermediate, followed by Caudovirales (4.8% of reads) which are tailed bacteriophages, and Tubulavirales (0.02% of reads).
Table 2. Musa samples and their endophytic virus-like sequences matches, showing numbers of contigs resulting from using two different virus detection pipelines (i.e., a blastn-based pipeline and more sensitive pipeline using the software VirSorter2 with vConTACT2 and GDV).
By comparison, the more sensitive viral detection pipeline using VirSorter2, vConTACT2, and GDV viral prediction pipelines yielded significantly more candidate viruses (Table 2 and compare Supplementary Tables 1–3), with ~23.870 Mbp of contigs containing predicted viruses, comprising 733 predicted phages. vConTACT2 clustering was able to taxonomically assign 22.51% of the contigs, whereas GDV was able to taxonomically assign 94.27% of viral contigs. Compared to the blastn pipeline, this more sensitive pipeline resulted in identifying more long contigs, with an average of maximum contig lengths across samples of 51,312 bp (maximum 108,191 bp for sample BBC) with an average of the median contig lengths of 6,713 bp (maximum 16,828 for sample FHC). The sample WHC had notably shorter contigs than others (maximum 4,552 bp, median 807 bp).
After normalizing for relative numbers of reads, phage abundances at the family level varied among sampled genotypes and tissues (Figure 2). Predicted phages from the GDV pipeline were classified to 51 families, with the most common classification, at ~30% abundance, being “unclassified Caudoviricetes.” The next most abundant group was family Ackermannviridae (~27% abundance), followed by Peduoviridae (15.7%), and Autographiviridae, Perisivirus, Naomviridae, Stephanstirmvirinae, and Casjensviridae (at 6%, 4.2%, 3.9%, 2%, and 1.7% abundances, respectively). Among Musa genotypes and tissues, the pattern of phage abundance at the family level was variable (Figure 2), with Williams Hybrid roots (WHC) having a larger proportion of unassigned viruses than the others, FHIA-25 Hybrid leaves (FHL) having a larger portion of Kalamavirales, M. balbisiana Thai Black roots (BBC) having a larger portion of Aguilavirus, and MBR, MSR, and MTL having larger proportions of Casjensviridae.
Figure 2. Relative abundance of putative phages detected from microbiomes of Musa genotypes and tissues, classified to the family level using GDV Tool, calculated after normalizing read coverage among samples. For ease of visualization, arrows are used to point to the most abundant groups, including unclassified Caudoviricetes, and the five most abundant virus families across samples. Samples on the x-axis: BBC and BBL = Musa balbisiana Thai Black corm and leaf, DCR and DCL = Dwarf Cavendish root, and leaf, FHC and FHL = FHIA-25 corm and leaf, MBR and MBL = Musa balbisiana root and leaf, MSR and MSL = Musa sikkimensis root and leaf, MTC and MTL = Musa textilis corm and leaf, WHC and WHL = Williams Hybrid corm and leaf.
At the genus-level, predicted phage presence/absence and abundances from the GDV pipeline differed among samples, with most phage genera being unique to each sample. Overall, the most abundant phage genera were Rhizobium phage, Vhulanivirus, Salmonella phage, Brucella phage, Noahvirus, Rhodovulum phage, Eganvirus, Peduovirus, and Nanhaivirus. No genera were universally shared among all samples, but those most commonly found across samples included Salmonella phage, Rhizobium phage, Pseudomonas phage, Ochrobactrum phage, and Rhodovulum phage. In roots, the most abundant phages were Salmonella phage, Rhizobium phage, Peduovirus, Quadragintavirus, Yersinia phage, Brunovirus, Eganvirus, and Klebsiella phage. In leaves, the most abundant phages were Rhizobium phage, Vhulanivirus, Brucella phage, and Noahvirus. Overall phage taxa identification and abundances were similar for the blastn and GDV approaches except that the blastn method failed to detect about one- to two-thirds of the phages detected with GDV (Table 2; Supplementary Table 1).
Phage genera abundances plotted as a clustered heatmap scaled across rows (Figure 3) showed sets of 6 to 20 phages (numbered boxes 1 to 16 in Figure 3) which clustered together with high abundance (columns of dark boxes in heatmap). These sets of phages at higher abundance showed limited overlap across genotypes and tissues, i.e., most samples had unique patterns in highly abundant phages.
Figure 3. Relative abundance of putative phages detected from microbiomes of Musa samples classified to the genus level using GDV Tool, calculated after normalizing read coverage among samples. Heatmap has been scaled across rows and clustered by similar rows to reveal similarities in abundance patterns among sets of phage and host plant. Heatmap color: light to dark represents lowest to highest abundance. Boxes enclose 16 predominant abundance clusters. Samples on the x-axis: BBC and BBL = Musa balbisiana Thai Black corm and leaf, DCR and DCL = Dwarf Cavendish root, and leaf, FHC and FHL = FHIA-25 corm and leaf, MBR and MBL = Musa balbisiana root and leaf, MSR and MSL = Musa sikkimensis root and leaf, MTC and MTL = Musa textilis corm and leaf.
Comparing predicted phages at the lowest taxonomic level (species or isolate) across Musa genotypes, showed that most predicted phages were unique to each plant genotype with only a few occurring in more than one plant host (Figure 4). The specific predicted phages that were universal to all sampled Musa genotypes were Rhodovulum phage RS1, Stenotrophomonas phage S1, Ochrobactrum phage POA1180, Brunovirus SEN34, Salmonella phage 118,970 sal3, and unassigned phages, whereas predicted phages that were nearly universal among Musa genotypes included Rhizobium phage RR1-B, Rhizobium phage RR1-A, Rhizobium phage 16–3, Pectobacterium phage ZF40, Felsduovirus RE2010, Aguilavirus mEp043, Vhulanivirus Shpa, Nanhaivirus D5C, Eganvirus PsP3, and Yersinia phage P37. There were 36 phages that were uniquely shared among sets of diploid genotypes (BB, MB, MT, MS), but we did not detect any uniquely shared phages among triploids (FH, DC; Supplementary Table 1).
Figure 4. Putative phage community overlap at the lowest taxonomic-levels (species or isolates) within the endosphere microbiomes of 6 Musa genotypes, combining leaf and root tissues for each genotype, shown as a proportional Venn diagram, with each Musa genotype depicted with a separate color. Numbers in circles indicate numbers of shared phages between Musa genotypes or phages unique to a genotype, while numbers in parentheses within circles indicate numbers of shared or unshared orthologous genes among phages in each circle.
Diversity analysis showed that diploid Musa plants (genotypes BB and other diploids) had higher phage alpha diversity compared to triploid (domesticated) Musa plants (genotypes AAA and AAB): Shannon diversity (p < 0.01, Hutcheson t-test) ranging from 4.9 (diploid plants) to 4.5 (triploid plants). Furthermore, statistical analyses showed that root tissues had significantly higher alpha diversity than leaf tissues: Shannon diversity (p < 0.01, Hutcheson t-test) ranging from 6.5 (root samples) to 4.4 (leaf samples).
Using the less-sensitive blastn pipeline and annotating genes with prokka, among contigs with strong blast similarity to viruses, many (52.7%) contained no predicted genes, either because candidate open reading frames did not pass similarity filters of the prokka software or because contigs were too short (e.g., 63.8% of contigs were <1,000 bp). Nevertheless, prokka annotation resulted in 887 predicted genes among these contigs. Of these, 733 (82.6%) were annotated as “hypothetical protein.” Phage gene orthologs, analyzed in Roary, showed a similar pattern, with most orthologous gene clusters being unique to individual Musa genotypes. Using the contigs identified with the VirSorter2-vConTACT2-GDV pipeline, far more contigs could be annotated with virus genes or virus-like protein coding motifs (pfam) using the DRAMv software (Supplementary Table 4), resulting in 1,038 unique pfam domains across samples with ~400 to 600 unique viral pfams in most samples. There were up to 1,901 contigs per sample with predicted viral pfam domains. The most abundant of these genes or pfam domains, normalizing among samples and accounting for read coverage were: phage portal protein, Integrase core domain, Phage integrase family, Baseplate J-like protein, ABC transporter, Helix-turn-helix domain, Phage capsid family, Phage virion morphogenesis family, Transposase, and Caudovirus prohead serine protease. Comparing relative abundances of phage pfam domains across samples (Figure 5) showed no particular pattern across Musa genotypes or tissue types; however, assemblages of pfams did show some clustering within samples (e.g., MBR, MBL, MTC, and FHC had numerous abundant phage tail proteins in common, compared with other samples).
Figure 5. Relative abundances of predicted phage protein domains (pfams) for the 150 most abundant pfams detected from Musa samples microbiomes using the DRAMv software. Heatmap color: light to dark represents lowest to highest abundance. Samples on the x-axis: BBC and BBL = Musa balbisiana Thai Black corm and leaf, DCR and DCL = Dwarf Cavendish root, and leaf, FHC and FHL = FHIA-25 corm and leaf, MBR and MBL = Musa balbisiana root and leaf, MSR and MSL = Musa sikkimensis root and leaf, MTC and MTL = Musa textilis corm and leaf.
To assess predicted phage synteny for several of the most common and well-characterized phages, 8 predicted phage or prophage regions detected by both the GDV pipeline and PHASTER were analyzed compared to references in a gene-by-gene manner and with phylogenetics. These predicted phages ranged from 12.1 to 49.3 kb, with 16 to 56 predicted genes, with two of these phage regions classified as “intact,” 4 as “incomplete,” and 2 as “questionable” in PHASTER. Comparative synteny analysis with closest reference sequences from GenBank is shown in Figure 6 and phylogenetic analysis of the two longest predicted genes from each of these 8 phages is shown in Supplementary Figures 1–15.
Figure 6. Annotated PHASTER-predicted phages or prophages from Musa microbiomes compared to similar full-length reference sequences from GenBank, showing synteny and tblastx similarity (in grayscale bands) between predicted proteins of known (blue arrow) or unknown (yellow arrow) function, showing proteins that were analyzed phylogenetically (purple arrow) in Supplementary Figures 1–15. (A) Predicted phages 56 and 68 aligned to Rhizobium phage RR1-B. (B) Predicted phages 114 and phage 88 aligned to Agrobacterium phage Atu ph08. (C) Predicted phage 113 aligned to Klebsiella phage KMI6. (D) Predicted phage 116 aligned to Shinella sp. phage HZN7. (E) Predicted “FHC phage” aligned to Pseudomonas monteillii plasmid pSTW0522-72-1.
Intact predicted “Phage 56” from samples BBC and MTC, and incomplete “Phage 68” from samples FHL and FHC were most similar to Rhizobium phage RR1-B (NCBI accession JF74315.1; Figure 6A), with moderately high synteny conservation, and higher blastx identity to one another than to the reference sequence. Notable genes present in these Musa microbiome phages that were not present in the reference phage (Supplementary Table 5) include genes encoding an ISL3 family transposase, a partial Shiga-like toxin 2 subunit A (63 amino acids long, with 66% identity to prophage Shiga-like toxin sequences integrated into Agrobacterium species), and a holin. Phylogenetic analysis of the DNA methyltransferase/methylase N-4/N-6 gene showed these predicted phages to be most closely related to Rhizobium skierniewicense and Agrobacterium sp. AGB01 in a well-supported clade with other Agrobacterium/Rhizobium spp. Supplementary Figure 1, which was nested between clades comprised Agrobacterium vitis and Agrobacterium leguminosarum. Phylogenetic analysis of the “Phage 68” C-5 cytosine specific DNA methylase gene showed a similar pattern to that of the DNA methytransferase gene described above, whereas the “Phage 56” DNA primase gene clustered with Rhizobium straminoryzae phage/plasmid sequences and other Rhizobium spp. (Supplementary Figures 2, 3).
Incomplete predicted “Phage 114” from sample BBC and “Phage 88” from samples BBC and MBR were most similar to the flanking ends of Agrobacterium phage Atu ph08 (NCBI accession MF403009.1), with relatively high synteny (Figure 6B). Notable genes present in these phages that were absent in the reference phage (Supplementary Table 5) include a DNA adenine methyltransferase gene, a CzcR-like response regulator gene, and an endolysin gene. Phylogenetic analysis showed a ‘Phage 88’ gene annotated as encoding an integrase arm-type DNA binding domain protein clustered closely with an undescribed Proteobacteria bacterium within a clade of Agrobacterium tumefaciens (=A. radiobacter) complex while its gene for a UvrB/UvrC motif containing protein clustered similarly (Supplementary Figures 4, 5). Similarly, genes from “Phage 114” annotated as hypothetical proteins clustered with A. tumefaciens and other Agrobacterium, including Agrobacterium salinitolerans (Supplementary Figures 8, 9).
A very long phage-like region denoted as “questionable,” denoted here as “Phage 113” from sample MTC, had high gene conservation and synteny conservation to Klebsiella phage KMI6 (NCBI accession MN101220.1; Figure 6C). There were few genes that differed substantially between this phage and the reference, except annotated gene 12 in “Phage 113” (Supplementary Table 5) encoding a putative tail fiber protein which appeared to be absent in the reference. Phylogenetic analysis of two putative internal core protein genes indicated clustering with a clade containing a variety of Klebsiella phages, Escherichia phages, and undescribed Caudovirales sp. with high bootstrap support but large sequence divergence (i.e., long branch lengths; Supplementary Figures 6, 7).
Incomplete “Phage 116,” from sample MTC, was most similar to a prophage of Shinella sp. HZN7 (NCBI accession CP015736.1), but with a middle segment of uniquely missing and different genes and some regions with highly diverged (low blastx identity) genes (Figure 6D). Genes present in this phage that were absent in the reference (Supplementary Table 5) included a conserved transposable phage protein gene, a host-nuclease inhibitor protein “gam” gene, and a putative DNA ends-protecting protein. Phylogenetic analysis of a gene for a peptidoglycan-binding domain protein and a gene for a transposase C-terminal domain-containing protein showed this phage to be closest to Shinella sp. phage HZN7, with very weak bootstrap support for various sister clades (Supplementary Figures 10, 11).
Intact “Phage FHC” from sample FHC, which assembled as one complete contig, was most similar to Pseudomonas monteillii plasmid pSTW0522-72-1 (NCBI accession AP022474.1) with very high gene content similarity (Figure 6E). Phylogenetic analysis of the predicted phage tail tape measure protein gene and a hypothetical protein gene showed a strongly supported cluster with Pseudomonas sp. WS 5027, within clades containing P. monteillii, Pseudomonas aeruginosa, and Pseudomonas alloputida (Supplementary Figures 14, 15).
Finally, predicted “Phage DCR,” which was assembled as one large contig and was denoted as “questionable,” had no significantly similar full-length reference match, and so could not be analyzed with Easyfig. Nevertheless, this predicted phage had 17 of 19 predicted genes matching phage-like proteins. Phylogenetic analyses of genes for a phage tail tape measure protein and a head maturation protease showed strong bootstrap support for clustering with A. tumefaciens (=A. radiobacter) and Rhizobium tropici, and Rhizobium metallidurans and Rhizobium pusense, respectively. However, the branch lengths were quite long, suggesting significant sequence divergence in both cases (Supplementary Figures 12, 13).
The contigs matching endogenous viruses included both short and long contigs, many of which had high blastn similarity to references in NCBI databases (Supplementary Table 6). For example, the maximum length of a contig that matched endogenous viruses was 37,479 bp, whereas 41 of these endogenous virus-like contigs were >3,000 bp, 41.6% of contigs were >900 bp, and 90% of contigs were >300 bp. Blast hit regions were relatively long, with 81.9% of hits being >300 bp and 112 hits being >900 bp. Nucleotide identities for hit regions were variable, with 55.5% of hit regions being >95% identity and 34% of hits being >98% identity. Among matches to reference endogenous viruses annotated as “complete,” 39% of the matching contigs showed 100% coverage (i.e., indicating the contig included the complete endogenous virus genome; see Supplementary Table 6, which includes sequences) and inspection of predicted ORFs in Geneious showed full, intact predicted genes. The sum of read coverage of predicted endogenous viruses (Table 2) was quite high (40,481 X) despite low levels of banana genomic DNA in these enriched microbiome layers (Figure 1). Endogenous virus-like reads, calculated across all banana genotypes (Figure 7; Supplementary Table 7), were mostly from the order Ortervirales, with most matches to family Caulimoviridae, in the genus Badnavirus. Among Badnavirus matches, 56 species were identified. In total, 42.13% of reads matched the broad group of badnaviruses denoted endogenous banana streak viruses (eBSVs). Seventeen distinct species of eBSVs were identified from across sampled Musa genotypes. The most abundant Badnavirus species were Banana streak virus (BSV; 60.97% of eBSVs reads) and Banana streak MY virus (BSMYV; 22.42% of eBSVs reads). Other eBSVs were detected at less than 5% read coverage. Additionally, 14 species matched other Musa-group endogenous badnaviruses (Figure 6; Supplementary Table 7).
Figure 7. Abundance of putative endogenous viruses in 12 Musa samples from leaf and corm tissues, based on blastn hits to assembly contigs shown as a heatmap of coverage, shown in color legend, after normalization to control for differences in reads across samples.
Other non-Musa plant viruses detected include 26 additional Badnavirus species, including several that were present in multiple samples, such as Grapevine Roditis leaf discoloration-associated virus, Taro bacilliform CH virus, Piper yellow mottle virus, Sugarcane bacilliform virus, and Sugarcane bacilliform Guadeloupe A virus.
Analyses of predicted endogenous plant virus diversity among samples showed that only Banana streak virus was universal to all root and leaf samples for all Musa genotypes. Overall, 71.68% of endogenous plant virus reads were from leaf samples, from which 17 species were unique to leaf tissues, whereas 9 species were unique to root samples. Comparing Musa genotypes, 17 endogenous viruses were unique to Thai Black roots (BBC), while 3 viruses were unique to M. balbisiana roots (MBR). Among BB genotypes (MBR and BBC) only 3 viruses where shared. Also, 14 viruses were unique to M. textilis roots (MTC), 3 viruses were unique to Musa sikkimensis root (MSR), 6 viruses were unique to Dwarf Cavendish roots (DCR; AAA genotype), and 4 viruses unique to FHIA-25 Hybrid roots (FHC; AAB genotype; Figure 7; Supplementary Table 7).
Several candidate viruses were exclusively detected from diploid (wild) genotypes (BB/MB, M. textilis, and M. sikkimensis; Figure 7): Banana streak Brazilian B virus, Banana streak CA virus, Banana streak UA virus, Banana streak UI virus, and Banana streak UL virus. Some viruses were exclusively detected from diploids and FHIA-25 (AAB genotype) samples: Banana streak GF virus, Banana streak IM virus, Banana streak MY virus, Banana streak OL virus, Banana streak virus acuminata Yunnan, and Banana streak VN virus. Other viruses were found exclusively in Dwarf Cavendish (AAA genotype): Banana streak Uganda B virus, Banana streak Uganda E virus, Banana streak Uganda G virus, Banana streak Uganda H virus, and Banana streak Uganda virus C.
Diversity analyses of candidate endogenous viruses showed that diploid (wild) plants had higher alpha diversity compared to triploid domesticated (AAA and AAB) plants: Shannon diversity (p < 0.01, Hutcheson t-test) ranged from 3.32 for triploid plants to 4.02 for diploid plants. Furthermore, statistical analyses showed that leaf tissues had higher alpha diversity than root tissues. Shannon diversity (p < 0.01, Hutcheson t-test) ranged from 3.9 (leaf samples) to 3.4 (root samples). The same pattern and similar statistically significant differences were found after removing Banana streak virus which occurs in every plant and tissue and is therefore more likely incorporated into the banana genome.
We compared endophytic virus-like sequences from sympatric banana plants including wild diploid and domesticated triploid varieties as a critical first step toward assessing how these viromes may impact microbiomes. Results revealed numerous novels or highly divergent predicted phages and dsDNA viruses or endogenous viral elements (EVEs) with generally non-overlapping community structures despite the shared environments of these plants, suggesting hosts drive the composition of these DNA virus communities.
Comparing phage communities between banana genotypes demonstrated clear differences at the phage family-, genus-, and species/isolate-levels, suggesting an influence of plant host history (wild diploid vs. domesticated triploid), as expected given previous studies of domesticated vs. wild relative microbiomes (Liu et al., 2017; Mertens et al., 2021; Nakkeeran et al., 2021). The lack of overall lower-level taxonomic overlap among Musa plants grown together in the small-scale farm suggests that these endophytic phages either do not easily transfer among plants or that plant hosts control or limit their communities. Furthermore, data showed a pattern of phage communities unique to each plant (shown in Figure 3) suggesting possible “phage consortia” may be able to infect or persist within these endospheres. If these distinct endosphere phageomes persist in healthy plants over the long term, presumably actively infecting endophytic bacteria. Despite our efforts to functionally annotate these phage communities and the great diversity of predicted pfam domains, the resulting lists of pfams provided limited insight: most pfams represent standard viral components. However, and the clustering of several samples (M. balbisiana, M. textilis, and FHIA-25 Hybrid) in their abundance profiles for many phage tail components raises interest in the possible activity of these phage. Further work will be essential to explore these possibilities as part of a broader investigation of the impact of endophytic phages on the plant microbiome’s contribution to disease resistance.
Plant tissue and genotype influenced the composition of predicted phage communities, as expected based on studies of bacterial endophyte communities (Lundberg et al., 2012; Liu et al., 2017; Maheshwari and Annapurna, 2017; Fitzpatrick et al., 2018; Afzal et al., 2019; Mertens et al., 2021; Nakkeeran et al., 2021; Wippel et al., 2021). For example, even though there were higher relative levels of viruses in leaves than roots, these virus blast hits were predominantly endogenous plant viruses, with few phage or prophage hits. This result is consistent with roots being primary sites of bacterial colonization, with leaves hosting a subset of root-colonizing microbiota (Fitzpatrick et al., 2018; Wippel et al., 2021). However, several predicted phage phages appeared to be unique to leaf tissues, for example, Burkholderia phage DC1. Its host, members of the Burkholderia cepacia complex, are important intracellular endophytes that are often integral to plants, conferring key protective benefits (Pan et al., 1997; Mendes et al., 2007; Mercado-Blanco and Lugtenberg, 2014).
In the endophytic microbiome of Musa spp., we found predicted phage or prophage sequences that were diverged from those of reference phage, suggesting that these phages may be unique to bacterial endophytes or pathogens of banana. Among the abundant and widely shared phages identified in these Musa samples, several resembled Rhizobium phage RR1-A and RR1-B, which are temperate phages first characterized from deep subseafloor sediments (Engelhardt et al., 2013), but also found associated with the rhizosphere of plants, such as Agave americana (Ruíz-Valdiviezo et al., 2017). Phylogenetic comparisons suggested that this phage may be related to phage infecting R. skierniewicense, an endophytic tumor-causing rhizobium (Pulawska et al., 2012). We found two diverged variants of this phage, one in diploids M. balbisiana and M. textilis (denoted “Phage 56”) and the other in the triploid FHIA-25 Hybrid (denoted “Phage 68”), with higher sequence similarity between these contigs than to the reference, suggesting possible Musa-specific variants and possible phylodiversification of this phage or its bacterial host. Detection of such RR1 “predatory” lysogenic phages (Engelhardt et al., 2013) in Musa spp. combined with our detection of an additional a holin gene and a partial Shiga-like toxin gene in these phages raises interest in their capacity to regulate Rhizobiaceae in the endophytic microbiome.
Another widely observed group of predicted phage in these Musa samples matched Klebsiella phage, targeting Klebsiella bacteria which are a focus for their plant growth promoting properties. Although initial blast similarity suggested the numerous Klebsiella phages in these Musa microbiomes were most similar to prophage in clinically important Klebsiella pneumoniae (Bleriot et al., 2020), the sequences were quite diverged from known clinical and agricultural strains in GenBank, likely reflecting distinct phage variants. Diverse Klebsiella strains including strains of K. pneumoniae are nitrogen-fixing diazotrophs which colonize plants well, especially monocots (Dong et al., 2003). Many strains promote plant growth and some have been shown to be protective against diseases such as Fusarium wilt in banana (Nakkeeran et al., 2021). Together, these findings raise interest in the novel Klebsiella phage variants found in this study. As with the RR1-like sequences, the divergence among Klebsiella phage-like sequences among our sampled Musa plants suggest Musa-specific variants and possible phylodiversification in these hosts.
A predicted phage found only in the M. textilis microbiome distantly matched a phage from the nicotine-degrading alphaproteobacterial strain Shinella sp. HZN7 (Ma et al., 2014). Shinella species have been found as endophytes in several studies and are remarkable for their diverse biosynthetic properties supported by large genomes (~7.35 Mbp), including electroproducing endophytes (Qiu et al., 2016; Ling et al., 2022). The additional transposable phage protein gene detected here may suggest this is a transposable phage with the ability to rearrange host genomes (Toussaint and Rice, 2017), while the other extra genes (a host-nuclease inhibitor protein ‘gam’ gene and a DNA ends protecting protein) which protect the phage against host RecBCD-driven degradation suggest this could be a formidable regulator of its host endophytes.
Pseudomonas spp. are common in endophytic microbiomes, conferring a wide range of plant-beneficial traits (Nakkeeran et al., 2021), hence, our discovery of an intact phage-like region matching a plasmid from Pseudomonas monteillii (an environmental and human pathogenic species) or Pseudomonas sp. WS 5027 (a psychrophilic species initially found in milk; Maier et al., 2020) was of interest. Curiously, this predicted phage/plasmid was only found in the FHIA-25 Hybrid banana, suggesting specificity of this phage’s host. We also detected at lower coverage, a Pseudomonas fluorescens phage KNP-like sequence. This sequence matched a cluster of phages of interest for their potentially important role in regulating plant-specific P. fluorescens (Nowicki et al., 2017).
The discovery of two fragments matching phage Atu_ph08 from the plant pathogen Agrobacterium tumefaciens (=A. radiobacter) in Thai Black M. balbisiana is of interest due to recent evidence suggesting that these novel T7-like phages may be lysogenic and could regulate pathogenic Agrobacterium (Attai and Brown, 2019). However, our seqeunces from banana lacked the large helicase gene found in the Atu_ph08 phage: it is unclear whether this indicates assembly artifacts due to repeat elements or diversity among metagenomic variants, or whether these prophage regions have lost the helicase and are degrading. Another candidate A. radiobacter phage, “Phage DCR” from Dwarf Cavendish, was too diverged from reference sequences in GenBank to comparatively analyze gene synteny, and may represent another novel host-specific virus or prophage.
We also found predicted phages with potential to regulate Erwinia-, Pectobacterium-, and Ralstonia-associated diseases which have been reported as the most devastating bacterial diseases of banana worldwide, after Xanthomonas wilt (Blomme et al., 2017). We found a match to Erwinia phage EtG, which clusters with Enterobacteriales phages of wide host ranges (Thompson et al., 2019), and a match to the Pectobacterium phage ZF40, hosts of which cause Enterobacteraceae derived soft rot diseases (Czajkowski, 2015; Comeau et al., 2017). We also found low levels of Ralstonia phage in M. sikkimensis and Thai Black roots, which is important given previous studies that showed phages isolated from banana soils may control R. solanacearum, causing devastating banana Moko disease (Ramírez et al., 2020) and similarly, phages appear effective in controlling Ralstonia syzygii sub sp. celebesensis (Rsc), causing banana blood disease (Murthi et al., 2021).
In particular, our results showing a large proportion of the reads from sequencing the bacterial microbiome layer were unclassified even at kingdom level supports the hypothesis that there may be many undescribed DNA viruses in Musa tissues. Consistent with this result, the most abundant phages were “uncharacterized Caudoviricetes” and a majority of blast-detected phage-like regions could not be annotated to known genes, although GDV performed somewhat better at function prediction than the simple prokka/DIAMOND approaches. Furthermore, we suspect that our Nycodenz enrichment protocol, which was developed for isolating bacteria may not be optimal for phage particles, some of which we speculate may be lost in the pellet, further reducing the measured abundance and diversity of phage in our study. Together, these arguments all point to hidden undiscovered phage diversity in the Musa endosphere. This is on the one hand surprising, given the challenges of phage transmission one might imagine within the confines of plant tissues. Although metagenomic virus detection workflows are improving (Bin Jang et al., 2019; Roux et al., 2019; Vilsker et al., 2019; Pratama et al., 2021; Turner et al., 2021), MAG approaches are still less often used for detecting phage communities compared to bacterial communities, and sequence databases for phage genes still lag behind that of bacterial genes (Dion et al., 2020). Although we found the VirSorter2-vConTACT2-GDV pipeline to be several times more sensitive at detective viruses than our initial blastn-based pipeline, we note that vConTACT2 was somewhat poorer at taxonomic classification than GDV, as has been reported by others (Zhu et al., 2022). This performance difference is likely due to an abundance of short contigs in our samples.
Although detection of dsDNA plant viruses was not the primary goal of this study, given the methods used to enrich microbial cells, we found a surprising proportion of reads (e.g., at a total of >40,000 × coverage) and assembled contigs (e.g., 708 distinct taxa) matched plant DNA viruses, including both predicted intact plant viruses and fragmented or degrading viral fragments. This result was more surprising, given the tiny relative number of copies of Musa genomic DNA in our data. Unlike the diversity of phage, which was higher in root tissues, plant viruses were richer in leaves, and unlike the largely non-overlapping phage communities among genotypes, abundant plant viruses tended to be shared across all tissues and Musa genotypes. This is likely because these DNA plant viruses, such as the banana streak viruses, largely occur as endogenous, replicating forms universally integrated into Musa genomes (Geering et al., 2005; Gayral et al., 2008). The high coverage and abundance of these in our data, despite limited host DNA levels, is consistent with their high copy number in the host DNA. In addition, we found differences among genotypes: diploids had more diverse DNA plant virus communities than triploids, with many unshared viruses, including numerous non-streak and non-Musa viruses, suggesting a mixture of resident and transient DNA viruses in these samples. These findings are important because cultivars of commercial banana (triploid M. acuminata) are susceptible to infection by a broad range of badnaviruses (Harper et al., 2002; Geering et al., 2005), impacting breeding programs as viral DNA integrated into the nuclear genome of M. balbisiana (BB genotype) spreads (Geering et al., 2005) leading to systemic infection when the plants are stressed (Muller et al., 2021). Insertion of a badnavirus promoter next to an endogenous plant gene may change transcription levels and alter tissue specificity of expression and give rise to infection (Matzke et al., 2004). Yet, most EVEs are thought to be “viral molecular fossils” in wild plant (Jones et al., 1999), with some latent plant viruses potentially providing a benefit to wild host plants (Pagán et al., 2012; Tripathi et al., 2019). Given our findings of differences in EVEs and other relatively abundant non-streak disease-causing dsDNA viruses in these Musa plants (e.g., Grapevine Roditis leaf discoloration-associated virus, Fig badnavirus 1, Taro bacilliform CH virus, Yacon necrotic mottle virus, and Dioscorea bacilliform RT virus), we propose future focused work to improve enrichment protocols, including target capture, to economically assay the diversity of these plant DNA viruses.
In conclusion, our results contribute to the small but growing collection of studies that characterize DNA virus communities within plants, a first step toward examining how endophytic viruses may regulate plant microbiomes. While we found several predicted viruses with similarity to known pathogens, most predicted virus-like sequences did not closely match pathogens. Furthermore, the sampled plants appeared healthy, raising questions about the potential for some of these viruses to be neutral or perhaps beneficial under some conditions. This study successfully characterized putative endophytic viromes from sympatric banana plants, showing differences among host tissues and genotypes with largely non-overlapping viromes, suggesting plant hosts drive or limit viral community structure. Future studies with long-read approaches should better-uncover longer regions to help distinguish active virions from integrated prophages or endogenous plant dsDNA viruses. Importantly, our data suggest that a Nycodenz-based microbiome enrichment method should make such long-read sequencing approaches more cost-effective for in planta virus community analysis.
The names of the repository/repositories and accession number(s) can be found in NCBI BioProject PRJNA837781, BioSamples SAMN28230877 to SAMN28230890.
AB led the sample collection and sequencing. SA and RL developed and ran bioinformatic analyses pipelines. SA and AB drafted the manuscript. All authors contributed to the article and approved the submitted version.
This research was funded by graduate funding support to SA through the Texas Tech Association of Biologists, Tech ASM, the TTU Graduate Student Research Award, Biology Graduate Summer Research Award, and the Helen DeVitt Jones Graduate Fellowship.
We thank Tammy Laberge for assistance with sample collection and undergraduate researchers Colton Eaton, Kimberly Morales, and others in the Brown Lab for assisting with microbiome isolation and enrichment, Simrandeep Singh and Sydney Pittignano for assistance with code, and the Center for Biotechnology and Genomics at Texas Tech University for use of the TapeStation. We also thank reviewers for invaluable suggestions for improving our analyses.
The authors declare that the research was conducted in the absence of any commercial or financial relationships that could be construed as a potential conflict of interest.
All claims expressed in this article are solely those of the authors and do not necessarily represent those of their affiliated organizations, or those of the publisher, the editors and the reviewers. Any product that may be evaluated in this article, or claim that may be made by its manufacturer, is not guaranteed or endorsed by the publisher.
The Supplementary material for this article can be found online at: https://www.frontiersin.org/articles/10.3389/fmicb.2023.1127606/full#supplementary-material
SUPPLEMENTARY TABLE S1 | List of detected putative phage and endogenous species from each sample, non-normalized and normalized read counts.
SUPPLEMENTARY TABLE S4 | Predicted genes and protein motifs (pfams) using the DRAM-v software.
SUPPLEMENTARY TABLE S6 | List of putative endogenous plant viruses detected from Musa spp. with taxonomic data and associated NCBI metadata.
Addy, H. S., Azizi, N. F., and Mihardjo, P. A. (2016). Detection of bacterial wilt pathogen and isolation of its bacteriophage from banana in Lumajang area, Indonesia. Int. J. Agron. 2016, 1–7. doi: 10.1155/2016/5164846
Afzal, I., Shinwari, Z. K., Sikandar, S., and Shahzad, S. (2019). Plant beneficial endophytic bacteria: mechanisms, diversity, host range and genetic determinants. Microbiol. Res. 221, 36–49. doi: 10.1016/j.micres.2019.02.001
Akinola, S. A., Ayangbenro, A. S., and Babalola, O. O. (2021). The immense functional attributes of maize rhizosphere microbiome: a shotgun sequencing approach. Agriculture 11, 1–14. doi: 10.3390/agriculture11020118
Arndt, D., Grant, J. R., Marcu, A., Sajed, T., Pon, A., Liang, Y., et al. (2016). PHASTER: a better, faster version of the PHAST phage search tool. Nucleic Acids Res. 44, W16–W21. doi: 10.1093/nar/gkw387
Attai, H., and Brown, P. J. B. (2019). Isolation and characterization T4-and T7-like phages that infect the bacterial plant pathogen agrobacterium tumefaciens. Viruses 11, 1–14. doi: 10.3390/v11060528
Bankevich, A., Nurk, S., Antipov, D., Gurevich, A. A., Dvorkin, M., Kulikov, A. S., et al. (2012). SPAdes: a new genome assembly algorithm and its applications to single-cell sequencing. J. Comput. Biol. 19, 455–477. doi: 10.1089/cmb.2012.0021
Bateson, M. F., and Dale, J. L. (1995). Banana bract mosaic virus: characterisation using potyvirus specific degenerate PCR primers. Arch. Virol. 140, 515–527. doi: 10.1007/BF01718428
Bin Jang, H., Bolduc, B., Zablocki, O., Kuhn, J. H., Roux, S., Adriaenssens, E. M., et al. (2019). Taxonomic assignment of uncultivated prokaryotic virus genomes is enabled by gene-sharing networks. Nat. Biotechnol. 37, 632–639. doi: 10.1038/s41587-019-0100-8
Blazanin, M., and Turner, P. E. (2021). Community context matters for bacteria-phage ecology and evolution. ISME J. 15, 3119–3128. doi: 10.1038/s41396-021-01012-x
Bleriot, I., Trastoy, R., Blasco, L., Fernández-Cuenca, F., Ambroa, A., Fernández-García, L., et al. (2020). Genomic analysis of 40 prophages located in the genomes of 16 carbapenemase-producing clinical strains of Klebsiella pneumoniae. Microb. Genomics 6, 1–18. doi: 10.1099/mgen.0.000369
Blomme, G., Dita, M., Jacobsen, K. S., Vicente, L. P., Molina, A., Ocimati, W., et al. (2017). Bacterial diseases of bananas and enset: current state of knowledge and integrated approaches toward sustainable management. Front. Plant Sci. 8, 1–25. doi: 10.3389/fpls.2017.01290
Bolger, A. M., Lohse, M., and Usadel, B. (2014). Trimmomatic: a flexible trimmer for Illumina sequence data. Bioinformatics 30, 2114–2120. doi: 10.1093/bioinformatics/btu170
Buchfink, B., Xie, C., and Huson, D. H. (2014). Fast and sensitive protein alignment using DIAMOND. Nat. Methods 12, 59–60. doi: 10.1038/nmeth.3176
Buttimer, C., McAuliffe, O., Ross, R. P., Hill, C., O’Mahony, J., and Coffey, A. (2017). Bacteriophages and bacterial plant diseases. Front. Microbiol. 8, 1–15. doi: 10.3389/fmicb.2017.00034
Camacho, C., Coulouris, G., Avagyan, V., Ma, N., Papadopoulos, J., Bealer, K., et al. (2009). BLAST+: architecture and applications. BMC Bioinformatics 10, 1–9. doi: 10.1186/1471-2105-10-421
Comeau, A. M., Douglas, G. M., and Langille, M. G. I. (2017). Microbiome helper: a custom and streamlined workflow for Microbiome Research. mSystems 2, e00127–e00116. doi: 10.1128/mSystems.00127-16
Czajkowski, R. (2015). Bacteriophages of soft rot Enterobacteriaceae—a minireview. FEMS Microbiol. Lett. 363, 1–6. doi: 10.1093/femsle/fnv230
Dale, J. L. (1987). Banana bunchy top: an economically important tropical plant virus disease. Adv. Virus Res. 33, 301–325. doi: 10.1016/S0065-3527(08)60321-8
Dion, M. B., Oechslin, F., and Moineau, S. (2020). Phage diversity, genomics and phylogeny. Nat. Rev. Microbiol. 18, 125–138. doi: 10.1038/s41579-019-0311-5
Dong, Y., Iniguez, A. L., and Triplett, E. W. (2003). Quantitative assessments of the host range and strain specificity of endophytic colonization by Klebsiella pneumoniae 342. Plant and Soil 257, 49–59. doi: 10.1023/A:1026242814060
Engelhardt, T., Sahlberg, M., Cypionka, H., and Engelen, B. (2013). Biogeography of Rhizobium radiobacter and distribution of associated temperate phages in deep subseafloor sediments. ISME J. 7, 199–209. doi: 10.1038/ismej.2012.92
Fadiji, A. E., Ayangbenro, A. S., and Babalola, O. O. (2021a). Shotgun metagenomics reveals the functional diversity of root-associated endophytic microbiomes in maize plant. Curr. Plant Biol. 25:100195. doi: 10.1016/j.cpb.2021.100195
Fadiji, A. E., Ayangbenro, A. S., and Babalola, O. O. (2021b). Unveiling the putative functional genes present in root-associated endophytic microbiome from maize plant using the shotgun approach. J. Appl. Genet. 62, 339–351. doi: 10.1007/s13353-021-00611-w
FAO (2016). All about bananas: Things you should know about the tropical fruit. Rome Food Agric. Organ. United Nations, FAO.
Federici, S., Nobs, S. P., and Elinav, E. (2021). Phages and their potential to modulate the microbiome and immunity. Cell. Mol. Immunol. 18, 889–904. doi: 10.1038/s41423-020-00532-4
Fitzpatrick, C. R., Copeland, J., Wang, P. W., Guttman, D. S., Kotanen, P. M., and Johnson, M. T. J. (2018). Assembly and ecological function of the root microbiome across angiosperm plant species. Proc. Natl. Acad. Sci. U. S. A. 115, E1157–E1165. doi: 10.1073/pnas.1717617115
Flynn, P. J., and Moreau, C. S. (2019). Assessing the diversity of endogenous viruses throughout ant genomes. Front. Microbiol. 10:e01139. doi: 10.3389/fmicb.2019.01139
Forero-Junco, L. M., Alanin, K. W. S., Djurhuus, A. M., Kot, W., Gobbi, A., and Hansen, L. H. (2021). Viruses roam the wheat phyllosphere bioRxiv [Preprint], 1–11.
Gayral, P., Noa-Carrazana, J.-C., Lescot, M., Lheureux, F., Lockhart, B. E. L., Matsumoto, T., et al. (2008). A single Banana streak virus integration event in the Banana genome as the origin of infectious endogenous Pararetrovirus. J. Virol. 82, 6697–6710. doi: 10.1128/jvi.00212-08
Geering, A. D. W., Olszewski, N. E., Harper, G., Lockhart, B. E. L., Hull, R., and Thomas, J. E. (2005). Banana contains a diverse array of endogenous badnaviruses. J. Gen. Virol. 86, 511–520. doi: 10.1099/vir.0.80261-0
Gregory, A. C., Zayed, A. A., Conceição-Neto, N., Temperton, B., Bolduc, B., Alberti, A., et al. (2019). Marine DNA viral macro- and micro-diversity from pole to pole. Cells 177, 1109–1123.e14. doi: 10.1016/j.cell.2019.03.040
Guo, J., Bolduc, B., Zayed, A. A., Varsani, A., Dominguez-huerta, G., Delmont, T. O., et al. (2021). VirSorter2: a multi-classifier, expert-guided approach to detect diverse DNA and RNA viruses. Microbiome 9, 1–13. doi: 10.1186/s40168-020-00990-y
Gurevich, A., Saveliev, V., Vyahhi, N., and Tesler, G. (2013). QUAST: quality assessment tool for genome assemblies. Bioinformatics 29, 1072–1075. doi: 10.1093/bioinformatics/btt086
Harper, G., Hull, R., Lockhart, B., and Olszewski, N. (2002). Viral sequences integrated into plant genomes. Annu. Rev. Phytopathol. 40, 119–136. doi: 10.1146/annurev.phyto.40.120301.105642
Harrison, J. G., and Griffin, E. A. (2020). The diversity and distribution of endophytes across biomes, plant phylogeny and host tissues: how far have we come and where do we go from here? Environ. Microbiol. 22, 2107–2123. doi: 10.1111/1462-2920.14968
Hirsch, P. R., and Mauchline, T. H. (2012). Who’s who in the plant root microbiome? Nat. Biotechnol. 30, 961–962. doi: 10.1038/nbt.2387
Hutcheson, K. (1970). A test for comparing diversities based on the Shannon formula. J. Theor. Biol. 29, 151–154. doi: 10.1016/0022-5193(70)90124-4
Ikeda, S., Kaneko, T., Okubo, T., Rallos, L. E. E., Eda, S., Mitsui, H., et al. (2009). Development of a bacterial cell enrichment method and its application to the community analysis in soybean stems. Microb. Ecol. 58, 703–714. doi: 10.1007/s00248-009-9566-0
Jones, L., Hamilton, A. J., Voinnet, O., Thomas, C. L., Maule, A. J., and Baulcombe, D. C. (1999). RNA-DNA interactions and DNA methylation in post-transcriptional gene silencing. Plant Cell 11, 2291–2301. doi: 10.1105/tpc.11.12.2291
Knief, C., Delmotte, N., Chaffron, S., Stark, M., Innerebner, G., Wassmann, R., et al. (2012). Metaproteogenomic analysis of microbial communities in the phyllosphere and rhizosphere of rice. ISME J. 6, 1378–1390. doi: 10.1038/ismej.2011.192
Köberl, M., Dita, M., Martinuz, A., Staver, C., and Berg, G. (2017). Members of Gammaproteobacteria as indicator species of healthy banana plants on fusarium wilt-infested fields in Central America. Sci. Rep. 7, 1–9. doi: 10.1038/srep45318
Koskella, B. (2019). New approaches to characterizing bacteria–phage interactions in microbial communities and microbiomes. Environ. Microbiol. Rep. 11, 15–16. doi: 10.1111/1758-2229.12706
Koskella, B., and Taylor, T. B. (2018). Multifaceted impacts of bacteriophages in the plant microbiome. Annu. Rev. Phytopathol. 56, 361–380. doi: 10.1146/annurev-phyto-080417-045858
Kuzyakov, Y., and Mason-Jones, K. (2018). Viruses in soil: Nano-scale undead drivers of microbial life, biogeochemical turnover and ecosystem functions. Soil Biol. Biochem. 127, 305–317. doi: 10.1016/j.soilbio.2018.09.032
Lang, J. M., Gent, D. H., and Schwartz, H. F. (2007). Management of Xanthomonas leaf blight of onion with bacteriophages and a plant activator. Plant Dis. 91, 871–878. doi: 10.1094/PDIS-91-7-0871
Lee, C. Z., Zoqratt, M. Z. H. M., Phipps, M. E., Barr, J. J., Lal, S. K., Ayub, Q., et al. (2022). The gut virome in two indigenous populations from Malaysia. Sci. Rep. 12, 1824–1810. doi: 10.1038/s41598-022-05656-3
Li, H., Handsaker, B., Wysoker, A., Fennell, T., Ruan, J., Homer, N., et al. (2009). The sequence alignment/map format and SAMtools. Bioinformatics 25, 2078–2079. doi: 10.1093/bioinformatics/btp352
Ling, L., Luo, H., Li, Z., Yang, C., Pang, M., Tu, Y., et al. (2022). Isolation, identification and characteristic analysis of plant endophyte Electrogenic Bacteria Shinella zoogloeoides SHE10. Curr. Microbiol. 79:268. doi: 10.1007/s00284-022-02964-9
Liu, H., Carvalhais, L. C., Crawford, M., Singh, E., Dennis, P. G., Pieterse, C. M. J., et al. (2017). Inner plant values: diversity, colonization and benefits from endophytic bacteria. Front. Microbiol. 8, 1–17. doi: 10.3389/fmicb.2017.02552
Lockhart, B. E. (1995). Banana streak Badnavirus infection in Musa: epidemiology, diagnosis and control. Taipei, Taiwan: ASPAC Food Fertilizer Technology Center. 143, 1–11.
Löytynoja, A. (2014). Phylogeny-aware alignment with PRANK. Methods Mol. Biol. 1079, 155–170. doi: 10.1007/978-1-62703-646-7_10
Lundberg, D. S., Lebeis, S. L., Paredes, S. H., Yourstone, S., Gehring, J., Malfatti, S., et al. (2012). Defining the core Arabidopsis thaliana root microbiome. Nature 488, 86–90. doi: 10.1038/nature11237
Ma, Y., Wei, Y., Qiu, J., Wen, R., Hong, J., and Liu, W. (2014). Isolation, transposon mutagenesis, and characterization of the novel nicotine-degrading strain Shinella sp. HZN7. Appl. Microbiol. Biotechnol. 98, 2625–2636. doi: 10.1007/s00253-013-5207-0
Maheshwari, D. K., and Annapurna, K. (Eds.). (2017). Endophytes: Crop productivity and protection. Vol. 2. Springer.
Maier, C., Huptas, C., von Neubeck, M., Scherer, S., Wenning, M., and Lücking, G. (2020). Genetic organization of the aprX-lipA2 operon affects the proteolytic potential of Pseudomonas species in Milk. Front. Microbiol. 11, 1–13. doi: 10.3389/fmicb.2020.01190
Matzke, M., Gregor, W., Mette, M. F., Aufsatz, W., Kanno, T., Jakowitsch, J., et al. (2004). Endogenous pararetroviruses of allotetraploid Nicotiana tabacum and its diploid progenitors, N. sylvestris and N. tomentosiformis. Biol. J. Linn. Soc. 82, 627–638. doi: 10.1111/j.1095-8312.2004.00347.x
Mendes, R., Pizzirani-Kleiner, A. A., Araujo, W. L., and Raaijmakers, J. M. (2007). Diversity of cultivated endophytic bacteria from sugarcane: genetic and biochemical characterization of Burkholderia cepacia complex isolates. Appl. Environ. Microbiol. 73, 7259–7267. doi: 10.1128/AEM.01222-07
Mercado-Blanco, J., and Lugtenberg, B. J. J. (2014). Biotechnological applications of bacterial endophytes. Curr. Biotechnol. 3, 60–75. doi: 10.2174/22115501113026660038
Mertens, A., Bawin, Y., Abeele, S., Kallow, S., Vu, D. T., Le, L. T., et al. (2021). Genetic diversity and structure of Musa balbisiana populations in Vietnam and its implications for the conservation of banana crop wild relatives. PLoS One 16, e0253255–e0253222. doi: 10.1371/journal.pone.0253255
Morella, N. M., Gomez, A. L., Wang, G., Leung, M. S., and Koskella, B. (2018). The impact of bacteriophages on phyllosphere bacterial abundance and composition. Mol. Ecol. 27, 2025–2038. doi: 10.1111/mec.14542
Muller, E., Ullah, I., Dunwell, J. M., Daymond, A. J., Richardson, M., Allainguillaume, J., et al. (2021). Identification and distribution of novel badnaviral sequences integrated in the genome of cacao (Theobroma cacao). Sci. Rep. 11, 8270–8213. doi: 10.1038/s41598-021-87690-1
Murthi, R. S., Safni, I., and Lisnawita, A. (2021). Isolation and selection specific bacteriophage from banana in north Sumatera to biologically control Ralstonia syzygii sub sp. celebesensis in vitro. IOP Conf. Ser. Earth Environ. Sci. 782:042018. doi: 10.1088/1755-1315/782/4/042018
Nakkeeran, S., Rajamanickam, S., Saravanan, R., Vanthana, M., and Soorianathasundaram, K. (2021). Bacterial endophytome-mediated resistance in banana for the management of fusarium wilt. 3 Biotech 11, 267–213. doi: 10.1007/s13205-021-02833-5
Nayfach, S., Camargo, A. P., Schulz, F., Eloe-Fadrosh, E., Roux, S., and Kyrpides, N. C. (2021). CheckV assesses the quality and completeness of metagenome-assembled viral genomes. Nat. Biotechnol. 39, 578–585. doi: 10.1038/s41587-020-00774-7
Nowicki, G., Walkowiak-Nowicka, K., Zemleduch-Barylska, A., Mleczko, A., Frąckowiak, P., Nowaczyk, N., et al. (2017). Complete genome sequences of two novel autographiviruses infecting a bacterium from the Pseudomonas fluorescens group. Arch. Virol. 162, 2907–2911. doi: 10.1007/s00705-017-3419-9
Nurk, S., Meleshko, D., Korobeynikov, A., and Pevzner, P. A. (2017). MetaSPAdes: a new versatile metagenomic assembler. Genome Res. 27, 824–834. doi: 10.1101/gr.213959.116
Olm, M. R., Brown, C. T., Brooks, B., and Banfield, J. F. (2017). DRep: a tool for fast and accurate genomic comparisons that enables improved genome recovery from metagenomes through de-replication. ISME J. 11, 2864–2868. doi: 10.1038/ismej.2017.126
Pagán, I., González-Jara, P., Moreno-Letelier, A., Rodelo-Urrego, M., Fraile, A., Piñero, D., et al. (2012). Effect of biodiversity changes in disease risk: exploring disease emergence in a plant-virus system. PLoS Pathog. 8:e1002796. doi: 10.1371/journal.ppat.1002796
Page, A. J., Cummins, C. A., Hunt, M., Wong, V. K., Reuter, S., Holden, M. T. G., et al. (2015). Roary: rapid large-scale prokaryote pan genome analysis. Bioinformatics 31, 3691–3693. doi: 10.1093/bioinformatics/btv421
Pan, M. J., Rademan, S., Kunert, K., and Hastings, J. W. (1997). Ultrastructural studies on the colonization of banana tissue and fusarium oxysporum f. sp. cubense race 4 by the endophytic bacterium Burkholderia cepacia. J. Phytopathol. 145, 479–486. doi: 10.1111/j.1439-0434.1997.tb00354.x
Pérez-Silva, J. G., Araujo-Voces, M., and Quesada, V. (2018). nVenn: Generalized, quasi-proportional Venn and Euler diagrams. Bioinformatics 34, 2322–2324. doi: 10.1093/bioinformatics/bty109
Pratama, A. A., Bolduc, B., Zayed, A. A., Zhong, Z. P., Guo, J., Vik, D. R., et al. (2021). Expanding standards in viromics: in silico evaluation of dsDNA viral genome identification, classification, and auxiliary metabolic gene curation. PeerJ 9, e11447–e11430. doi: 10.7717/peerj.11447
Pratama, A. A., Terpstra, J., de Oliveria, A. L. M., and Salles, J. F. (2020). The role of rhizosphere bacteriophages in plant health. Trends Microbiol. 28, 709–718. doi: 10.1016/j.tim.2020.04.005
Pulawska, J., Willems, A., and Sobiczewski, P. (2012). Rhizobium skierniewicense sp. nov., isolated from tumours on chrysanthemum and cherry plum. Int. J. Syst. Evol. Microbiol. 62, 895–899. doi: 10.1099/ijs.0.032532-0
Qiu, J., Yang, Y., Zhang, J., Wang, H., Ma, Y., He, J., et al. (2016). The complete genome sequence of the nicotine-degrading bacterium Shinella sp. HZN7. Front. Microbiol. 7, 2–5. doi: 10.3389/fmicb.2016.01348
Ramírez, M., Neuman, B. W., and Ramírez, C. A. (2020). Bacteriophages as promising agents for the biological control of Moko disease (Ralstonia solanacearum) of banana. Biol. Control 149:104238. doi: 10.1016/j.biocontrol.2020.104238
Refaei, J., Jones, E., Sakayaroj, J., and Santhanam, J. (2011). Endophytic fungi from Rafflesia cantleyi: species diversity and antimicrobial activity. Mycosphere 2, 429–447.
Regalado, J., Lundberg, D. S., Deusch, O., Kersten, S., Karasov, T., Poersch, K., et al. (2020). Combining whole-genome shotgun sequencing and rRNA gene amplicon analyses to improve detection of microbe–microbe interaction networks in plant leaves. ISME J. 14, 2116–2130. doi: 10.1038/s41396-020-0665-8
Rodriguez, P. A., Rothballer, M., Chowdhury, S. P., Nussbaumer, T., Gutjahr, C., and Falter-Braun, P. (2019). Systems biology of plant-microbiome interactions. Mol. Plant 12, 804–821. doi: 10.1016/j.molp.2019.05.006
Roossinck, M. J., Martin, D. P., and Roumagnac, P. (2015). Plant virus metagenomics: advances in virus discovery. Phytopathology 105, 716–727. doi: 10.1094/PHYTO-12-14-0356-RVW
Roux, S., Adriaenssens, E. M., Dutilh, B. E., Koonin, E. V., Kropinski, A. M., Krupovic, M., et al. (2019). Minimum information about an uncultivated virus genome (MIUVIG). Nat. Biotechnol. 37, 29–37. doi: 10.1038/nbt.4306
Roux, S., Enault, F., Hurwitz, B. L., and Sullivan, M. B. (2015). VirSorter: mining viral signal from microbial genomic data. PeerJ 3, e985–e920. doi: 10.7717/peerj.985
Ruíz-Valdiviezo, V. M., Rogel-Hernandez, M. A., Guerrero, G., Rincón-Molina, C. I., García-Perez, L. G., Gutiérrez-Miceli, F. A., et al. (2017). Complete genome sequence of a novel nonnodulating Rhizobium species isolated from Agave americana L. rhizosphere. Genome Announc. 5:e01280–17. doi: 10.1128/genomeA.01280-17
Saccardi, A., Gambin, E., Zaccardelli, M., Barone, G., and Mazzucchi, U. (1993). Xanthomonas campestris pv. Pruni control trials with phage treatments on peaches in the orchard. Phytopathol. Mediterr. 32, 206–210.
Schoelz, J. E., and Stewart, L. R. (2018). The role of viruses in the phytobiome. Annu. Rev. Virol. 5, 93–111. doi: 10.1146/annurev-virology-092917-043421
Seemann, T. (2014). Prokka: rapid prokaryotic genome annotation. Bioinformatics 30, 2068–2069. doi: 10.1093/bioinformatics/btu153
Seo, S. U., and Kweon, M. N. (2019). Virome–host interactions in intestinal health and disease. Curr. Opin. Virol. 37, 63–71. doi: 10.1016/j.coviro.2019.06.003
Shaffer, M., Borton, M. A., McGivern, B. B., Zayed, A. A., La Rosa, S. L., Solden, L. M., et al. (2020). DRAM for distilling microbial metabolism to automate the curation of microbiome function. Nucleic Acids Res. 48, 8883–8900. doi: 10.1093/nar/gkaa621
Siddique, A. B. (2020). Viruses of endophytic and pathogenic forest fungi. Virus Genes 56, 407–416. doi: 10.1007/s11262-020-01763-3
Soto-Giron, M. J., Kim, J. N., Schott, E., Tahmin, C., Ishoey, T., Mincer, T. J., et al. (2021). The edible plant microbiome represents a diverse genetic reservoir with functional potential in the human host. Sci. Rep. 11, 24017–24014. doi: 10.1038/s41598-021-03334-4
Stamatakis, A. (2014). RAxML version 8: a tool for phylogenetic analysis and post-analysis of large phylogenies. Bioinformatics 30, 1312–1313.
Sullivan, M. J., Petty, N. K., and Beatson, S. A. (2011). Easyfig: A genome comparison visualizer. Bioinformatics 27, 1009–1010. doi: 10.1093/bioinformatics/btr039
Thompson, D. W., Casjens, S. R., Sharma, R., and Grose, J. H. (2019). Genomic comparison of 60 completely sequenced bacteriophages that infect Erwinia and/or Pantoea bacteria. Virology 535, 59–73. doi: 10.1016/j.virol.2019.06.005
Tian, B. Y., Cao, Y., and Zhang, K. Q. (2015). Metagenomic insights into communities, functions of endophytes, and their associates with infection by root-knot nematode, Meloidogyne incognita, in tomato roots. Sci. Rep. 5, 1–15. doi: 10.1038/srep17087
Toussaint, A., and Rice, P. A. (2017). Transposable phages, DNA reorganization and transfer. Curr. Opin. Microbiol. 38, 88–94. doi: 10.1016/j.mib.2017.04.009
Tripathi, J. N., Ntui, V. O., Ron, M., Muiruri, S. K., Britt, A., and Tripathi, L. (2019). CRISPR/Cas9 editing of endogenous banana streak virus in the B genome of Musa spp. overcomes a major challenge in banana breeding. Commun. Biol. 2, 46–11. doi: 10.1038/s42003-019-0288-7
Turner, D., Kropinski, A. M., and Adriaenssens, E. M. (2021). A roadmap for genome-based phage taxonomy. Viruses 13, 1–10. doi: 10.3390/v13030506
Van Belleghem, J. D., Dąbrowska, K., Vaneechoutte, M., Barr, J. J., and Bollyky, P. L. (2018). Interactions between bacteriophage, bacteria, and the mammalian immune system. Viruses 11:10. doi: 10.3390/v11010010
Vilsker, M., Moosa, Y., Nooij, S., Fonseca, V., Ghysens, Y., Dumon, K., et al. (2019). Genome detective: an automated system for virus identification from high-throughput sequencing data. Bioinformatics 35, 871–873. doi: 10.1093/bioinformatics/bty695
Wippel, K., Tao, K., Niu, Y., Zgadzaj, R., Kiel, N., Guan, R., et al. (2021). Host preference and invasiveness of commensal bacteria in the Lotus and Arabidopsis root microbiota. Nat. Microbiol. 6, 1150–1162. doi: 10.1038/s41564-021-00941-9
Xue, C., Penton, C. R., Shen, Z., Zhang, R., Huang, Q., Li, R., et al. (2015). Manipulating the banana rhizosphere microbiome for biological control of Panama disease. Sci. Rep. 5, 1–11. doi: 10.1038/srep11124
Zhang, J., Kobert, K., Flouri, T., and Stamatakis, A. (2014). PEAR: a fast and accurate Illumina paired-end reAd mergeR. Bioinformatics 30, 614–620. doi: 10.1093/bioinformatics/btt593
Zhou, Y., Liang, Y., Lynch, K. H., Dennis, J. J., and Wishart, D. S. (2011). PHAST: a fast phage search tool. Nucleic Acids Res. 39, W347–W352. doi: 10.1093/nar/gkr485
Keywords: viral community, bacteriophage, endogenous virus, Musa, diversity, microbiome
Citation: Aghdam SA, Lahowetz RM and Brown AMV (2023) Divergent endophytic viromes and phage genome repertoires among banana (Musa) species. Front. Microbiol. 14:1127606. doi: 10.3389/fmicb.2023.1127606
Received: 19 December 2022; Accepted: 02 May 2023;
Published: 09 June 2023.
Edited by:
Ashish Srivastava, Amity University, IndiaReviewed by:
Aditya Kulshreshtha, Texas A&M AgriLife Research and Extension Center at Weslaco, United StatesCopyright © 2023 Aghdam, Lahowetz and Brown. This is an open-access article distributed under the terms of the Creative Commons Attribution License (CC BY). The use, distribution or reproduction in other forums is permitted, provided the original author(s) and the copyright owner(s) are credited and that the original publication in this journal is cited, in accordance with accepted academic practice. No use, distribution or reproduction is permitted which does not comply with these terms.
*Correspondence: Amanda M. V. Brown, YW1hbmRhLm12LmJyb3duQHR0dS5lZHU=
Disclaimer: All claims expressed in this article are solely those of the authors and do not necessarily represent those of their affiliated organizations, or those of the publisher, the editors and the reviewers. Any product that may be evaluated in this article or claim that may be made by its manufacturer is not guaranteed or endorsed by the publisher.
Research integrity at Frontiers
Learn more about the work of our research integrity team to safeguard the quality of each article we publish.