- 1National Institute for Communicable Disease Control and Prevention, Chinese Center for Disease Control and Prevention, Beijing, China
- 2Hunan Provincial Chest Hospital, Tuberculosis Control Institution of Hunan Province, Changsha, Hunan, China
- 3National Tuberculosis Reference Laboratory, Chinese Center for Disease Control and Prevention, Beijing, China
- 4Laboratory of Respiratory Diseases, Beijing Key Laboratory of Pediatric Respiratory Infection Diseases, Beijing Pediatric Research Institute, Beijing Children’s Hospital, Capital Medical University, Beijing, China
- 5School of Public Health, Peking University, Beijing, China
- 6Shenzhen Third People’s Hospital, Shenzhen, China
Background: Tuberculosis may reoccur due to reinfection or relapse after initially successful treatment. Distinguishing the cause of TB recurrence is crucial to guide TB control and treatment. This study aimed to investigate the source of TB recurrence and risk factors related to relapse in Hunan province, a high TB burden region in southern China.
Methods: A population-based retrospective study was conducted on all culture-positive TB cases in Hunan province, China from 2013 to 2020. Phenotypic drug susceptibility testing and whole-genome sequencing were used to detect drug resistance and distinguish between relapse and reinfection. Pearson chi-square test and Fisher exact test were applied to compare differences in categorical variables between relapse and reinfection. The Kaplan–Meier curve was generated in R studio (4.0.4) to describe and compare the time to recurrence between different groups. p < 0.05 was considered statistically significant.
Results: Of 36 recurrent events, 27 (75.0%, 27/36) paired isolates were caused by relapse, and reinfection accounted for 25.0% (9/36) of recurrent cases. No significant difference in characteristics was observed between relapse and reinfection (all p > 0.05). In addition, TB relapse occurs earlier in patients of Tu ethnicity compared to patients of Han ethnicity (p < 0.0001), whereas no significant differences in the time interval to relapse were noted in other groups. Moreover, 83.3% (30/36) of TB recurrence occurred within 3 years. Overall, these recurrent TB isolates were predominantly pan-susceptible strains (71.0%, 49/69), followed by DR-TB (17.4%, 12/69) and MDR-TB (11.6%, 8/69), with mutations mainly in codon 450 of the rpoB gene and codon 315 of the katG gene. 11.1% (3/27) of relapse cases had acquired new resistance during treatment, with fluoroquinolone resistance occurring most frequently (7.4%, 2/27), both with mutations in codon 94 of gyrA.
Conclusion: Endogenous relapse is the main mechanism leading to TB recurrences in Hunan province. Given that TB recurrences can occur more than 4 years after treatment completion, it is necessary to extend the post-treatment follow-up period to achieve better management of TB patients. Moreover, the relatively high frequency of fluoroquinolone resistance in the second episode of relapse suggests that fluoroquinolones should be used with caution when treating TB cases with relapse, preferably guided by DST results.
1. Introduction
Tuberculosis (TB) remains a major global public health issue, with an estimated 10.0 million new cases and more than 1.2 million deaths from TB worldwide in 2019 [World Health Organization (WHO), 2020]. Although most TB patients can be cured after the introduction of a standard combination of chemotherapy, some patients who complete an appropriate course of treatment still experience a subsequent episode, or TB recurrence (Zong et al., 2018). Patients with recurrent TB often require longer rounds of treatment with more toxic drugs, which reduces the success of treatment, leads to further transmission of Mycobacterium tuberculosis (MTB), and increases the burden of TB (Liu et al., 2020).
Recurrence of TB can be caused by relapse, also known as endogenous reactivation of the initial infection, or by exogenous reinfection with new MTB strains (Ruan et al., 2022). The proper discrimination between relapse and reinfection is essential for adjusting TB control measures. High relapse rates indicate inadequate TB treatment, whereas high rates of reinfection reveal poor TB cases management with many missed TB cases circulating in the community (Folkvardsen et al., 2020; Du et al., 2021).
The advent of molecular genotyping techniques for MTB has made it possible to assess the magnitude of endogenous relapse versus exogenous reinfection (Bandera et al., 2001; Lambert et al., 2003). These genomic-based typing methods include IS6110 fingerprinting, mycobacterial interspersed repetitive unit-variable number of tandem repeat (MIRU-VNTR), spoligotyping, and whole-genome-sequencing (WGS) (Barbier and Wirth, 2016). However, different genotyping methods often affect the reinfection rate due to different resolutions (Jagielski et al., 2016). Compared to traditional genotyping methods, WGS based on the full-genome of MTB strains has the distinct advantage by allowing the discrimination of MTBC strains at the highest resolution and simultaneously enabling detailed resistance predictions for almost all drugs (Roetzer et al., 2013; Walker et al., 2015).
Despite tremendous progress in TB control, China still has the second-highest TB burden worldwide [World Health Organization (WHO), 2020]. In addition, the presence of TB recurrence can further increase the burden of TB. A better understanding of the sources of recurrent TB and its related risk factors is essential for targeted interventions and for reducing the frequency of TB (Shen et al., 2017). However, limited efforts have been made to identify the major cause of TB recurrence in China, particularly in Hunan province, which has one of the highest TB burdens in China, with an estimated annual TB incidence of 94 cases per 100,000 population (He et al., 2022). To address this concern, we conducted a retrospective study among recurrent TB cases from five counties in Hunan province. We used WGS to determine whether TB recurrence was mainly caused by reinfection or relapse. We performed phenotypic drug susceptibility testing (DST) to compare in vitro DST results between the first and second TB episodes. We also collected demographic information and clinical characteristics of recurrent TB cases to analyze risk factors associated with reinfection and relapse.
2. Materials and methods
2.1. Study population
This retrospective study was conducted based on five DRS (drug resistance surveillance) sites (5 counties: Hecheng, Yongshun, Qidong, Taojiang, and Leiyang) in Hunan province, which were established according to the first national survey of drug resistance in China (Zhao et al., 2012). In these five counties, all suspected pulmonary TB cases from general hospitals or health centers are referred to local designated TB hospitals for confirmed diagnosis and treatment. All TB cases aged 15 years or older with bacteriologically confirmed (sputum-smear positive or culture positive) by local designated TB hospitals or clinics between January 1, 2013 to December 31, 2020 were included in this study. Positive sputum samples were cultured and isolated on Lowenstein-Jensen medium at the county-level and then sent to National Tuberculosis Reference Laboratory (NTRL). Information on these TB cases, including demographic characteristics and medical records, is collected at the time of patients’ visits and stored electronically in the National Tuberculosis Information Management System (TBIMS). To identify recurrent TB cases, the medical records of TB patients diagnosed between 2013 and 2020 were extracted from TBIMS on June 30, 2022 and collated using the method described previously (Shen et al., 2017). TB cases with any of the followings were excluded from the further study: (1) unsuccessful treatment outcomes of their initial TB episode (e.g., lose to follow-up, death, treatment failure, etc.); (2) less than 6 months of the recurrent interval (the time interval between the recorded end date of the treatment and the date of the re-diagnosis of active TB); (3) strains with subculture failure or contamination; (4) failed extraction of DNA or WGS errors.
2.2. Drug susceptibility testing
All MTB strains isolated from recurrent TB cases were previously stored in 7H9 medium containing 25% glycerin at–80°C refrigerator, and then were thawed and re-cultured on L-J medium for further study. MTB isolates in the logarithmic phase were subjected to drug susceptibility testing against rifampicin, isoniazid, ethambutol, streptomycin, ofloxacin, moxifloxacin, kanamycin, and amikacin using MYCOTB plate (Thermo Fisher Scientific, United States). Previous studies have demonstrated the good accuracy and reproducibility of the MYCOTB plate, which can be used as an alternative method for DST (Xia et al., 2017; Wu et al., 2019). All procedures were performed by trained staff at the national TB reference laboratory of China, as described elsewhere (He et al., 2022). H37Rv (ATCC 27294) was used as pan-susceptible control in each batch of DST. The concentration ranges and cut-off values for determining resistance or sensitivity for each drug used in this study were depicted previously (He et al., 2022). All DSTs were conducted twice to ensure the accuracy of DST results.
2.3. DNA extraction and sequencing
MTB strains were scraped from L-J solid slants, and genomic DNA was obtained from isolates with the cetyltrimethylammonium bromide (CTAB) method as described previously (Shao et al., 2021). The quality and concentration of genomic DNA were assessed by NanoDrop 2000c spectrophotometer (Thermo Fisher Scientific, USA) and Qubit 2.0 fluorometer (Invitrogen, Thermo Fisher Scientific, USA), respectively. Whole genome sequencing was performed by Annoroad Gene Technology company (Beijing, China) using Illumina Hiseq X10 (Illumina, Inc.) with 2 × 150 paired-end (PE) strategies.
2.4. Phylogenetic analysis
In brief, the quality control of raw reads was examined by FastQC (v0.11.9),1 and reads were filtered with Trimmomatic (v 0.38) using default values and minimum Phred Quality score of 20 (Bolger et al., 2014). Retained paired-end reads were mapped to the reference genome H37Rv (GenBank accession NC_000962.3) using BWA-MEM software (v. 0.7.17) (Freschi et al., 2021). Variants information including single nucleotide polymorphisms (SNPs) and small insertion/deletions (Indel) were detected using SAMtools (v1.3.1) and GATK (v.3.8.0) (He et al., 2022). The variants that met the following criteria were kept for further analysis: minimum coverage depth of 10X, Q20 minimum quality score for each variant, and more than 75% allele frequency (He et al., 2022).
SNPs located in repeating regions of the genome such as PE/PPE/PGRs genes, phage sequence, insertions, and mobile elements were excluded. The remaining SNPs in each isolate were pooled into a sequence based on the position, and SNP positions present in at least 95% of isolates were integrated into a sequence alignment (Liu et al., 2022). The maximum likelihood trees were constructed using a general time reversible model in MEGA-X (v.10.1.8) with bootstraps of 1,000 replicates (He et al., 2022). The phylogenetic tree was visualized and annotated using iTOL.2 Snp-dists (v.0.8.2) was used to calculate the SNP distance between pairs of isolates. QuantTB (v. 1.01)3 was used to identify mixed infection of MTB (Anyansi et al., 2020).
2.5. Lineage and genotypic drug resistance prediction
Fast-lineage-caller package (v.3.2)4 was used to call lineage and sub-lineage information of M. tuberculosis. TB Profiler (v.3.0.8)5 was used to predict genotypic drug susceptibility.
2.6. Statistical analysis
Pearson chi-square test and Fisher exact test were used to compare differences in categorical variables between relapse and reinfection. The Kaplan–Meier curve was generated in R studio (4.0.4) to describe and compare the time to recurrence between different groups. All statistical analysis was performed in the SPSS version 18.0 software (SPSS Inc., Chicago, Illinois). p < 0.05 was considered statistically significant.
2.7. Definition
TB recurrence was defined as a patient who was cured or completed treatment during the most recent course of treatment and then was re-diagnosed with a new TB episode [World Health Organization (WHO), 2013]. Reinfection was defined as a recurrent disease episode caused by a new TB strain with a genetic distance of more than 12 SNPs compared with the strain that caused the original episode. Relapse was defined as a genetic distance of 12 or fewer SNPs between paired strains isolated from two episodes in TB recurrence (Li et al., 2022). The recurrent interval was defined as the time interval between the recorded end date of the initial TB treatment and the date of the re-diagnosis of active TB (Ruan et al., 2022). Based on the phenotypic drug susceptibility testing, Pan-Susceptible was defined as MTB strains that were susceptible to all anti-TB drugs tested in this study (including rifampicin, isoniazid, ethambutol, streptomycin, moxifloxacin, ofloxacin, kanamycin and amikacin), whereas Drug-resistant was defined as MTB strains that were resistant to at least one of these anti-TB drugs but not include the concurrent resistance to rifampicin and isoniazid. MDR-TB was defined as MTB resistance to at least isoniazid and rifampicin. Pre-XDR-TB was defined as MDR-TB with additional resistance to any fluoroquinolones (moxifloxacin or ofloxacin) or any second-line injectable drugs (amikacin or kanamycin), but not both. XDR-TB was defined as MDR-TB with additional resistance to any fluoroquinolones and any second-line injectable drugs.
3. Results
3.1. Description of the study population
A total of 2,416 bacteriologically confirmed TB cases aged 15 years or older were collected between Jan. 2013 and Dec. 2020. Of which, 88.6% (2141/2416) cases were successfully treated, while 275 (11.4%) patients experienced treatment failure, loss to follow-up, treatment interruption, adverse reactions, or death. Overall, 117 (5.5%, 117/2141) successfully treated cases that experienced TB recurrences, 25 recurrent TB cases were excluded due to their recurrent interval being less than 6 months, and finally, 92 recurrent TB cases were included in further analysis. Among them, 56.5% (52/92) had recurrent strains with both episodes. After excluding subculture failure or contamination of any paired isolates (n = 7) and failure to extract DNA or WGS (n = 6). Finally, 39 recurrent TB patients with paired strains were enrolled in the final analysis. Of these, one patient had a third episode during the study period, for a total of 79 MTB isolates and 41 recurrent events (Figure 1).
3.2. Patient characteristics
The characteristics of recurrent TB cases at their primary episode were described below. Among the 39 recurrent TB cases included in this study, the median and mean age of patients was 54.0 [interquartile range (IQR), 45.0–65.0] and 54.4 ± 12.6 years old. The majority of patients were male (84.6%, 33/39) while 15.4% (6/39) were female. Almost 90% (89.7%, 35/39) of patients were farmers. In terms of treatment history, new cases accounted for 94.9% (37/39) of the total. 7.7% (3/39) and 12.8% (5/39) of recurrent TB cases had complications of hepatitis B and diabetes, respectively. The chest X-ray showed that 30.8% (12/39) of patients had cavitation in the first episode of TB. As for HIV status, 12 (30.8%, 12/39) patients were HIV-negative, while 27 (69.2%, 27/39) patients had unknown HIV infection status.
3.3. TB relapse and reinfection identified by SNP distance
The whole genome sequencing data of 79 MTB strains collected from 39 recurrent TB cases were first analyzed to determine the presence of mixed infections. Five recurrent TB cases (7 strains of mixed infections in total) were excluded from further analysis because any of their paired strains were identified as having at least two different strains. The paired SNP distances were calculated on the remaining 34 recurrent TB cases, including one patient (patient 15) with three TB episodes (patient 15–1, 15–2, 15–3), thus involving a total of 69 MTB strains and 36 recurrent events (Supplementary Figure S1). Identical genotypes were defined as strains that differed by no more than 12 SNPs (Li et al., 2022). Of 36 recurrent events, 27 (75.0%, 27/36) paired isolates (patient 1, 2, 3, 4, 5, 7, 8, 9, 11, 12, 13, 14, 15[1–3], 16, 20, 21, 22, 23, 24, 25, 26, 27, 28, 30, 31, 32 and 33) had 5 or fewer SNP differences (Supplementary Figure S1), indicating relapse. Nine (25.0%, 9/36) paired isolates (patient 6, 10, 15[1–2], 15[2–3], 17, 18, 19, 29, and 34) differed by >12 SNPs, suggesting exogenous reinfection with a new strain of MTB (Supplementary Figure S1). Comparison with SNP differences was also shown in Figure 2, identifying two major groups: paired isolates from relapsed cases had five or fewer SNP differences, whereas paired strains from reinfected cases had a dramatically higher number of SNP differences (range 185–1,074) except for 2 paired isolates (patient 17 and 18) with SNP differences of 14 (Supplementary Figure S1; Figure 2).
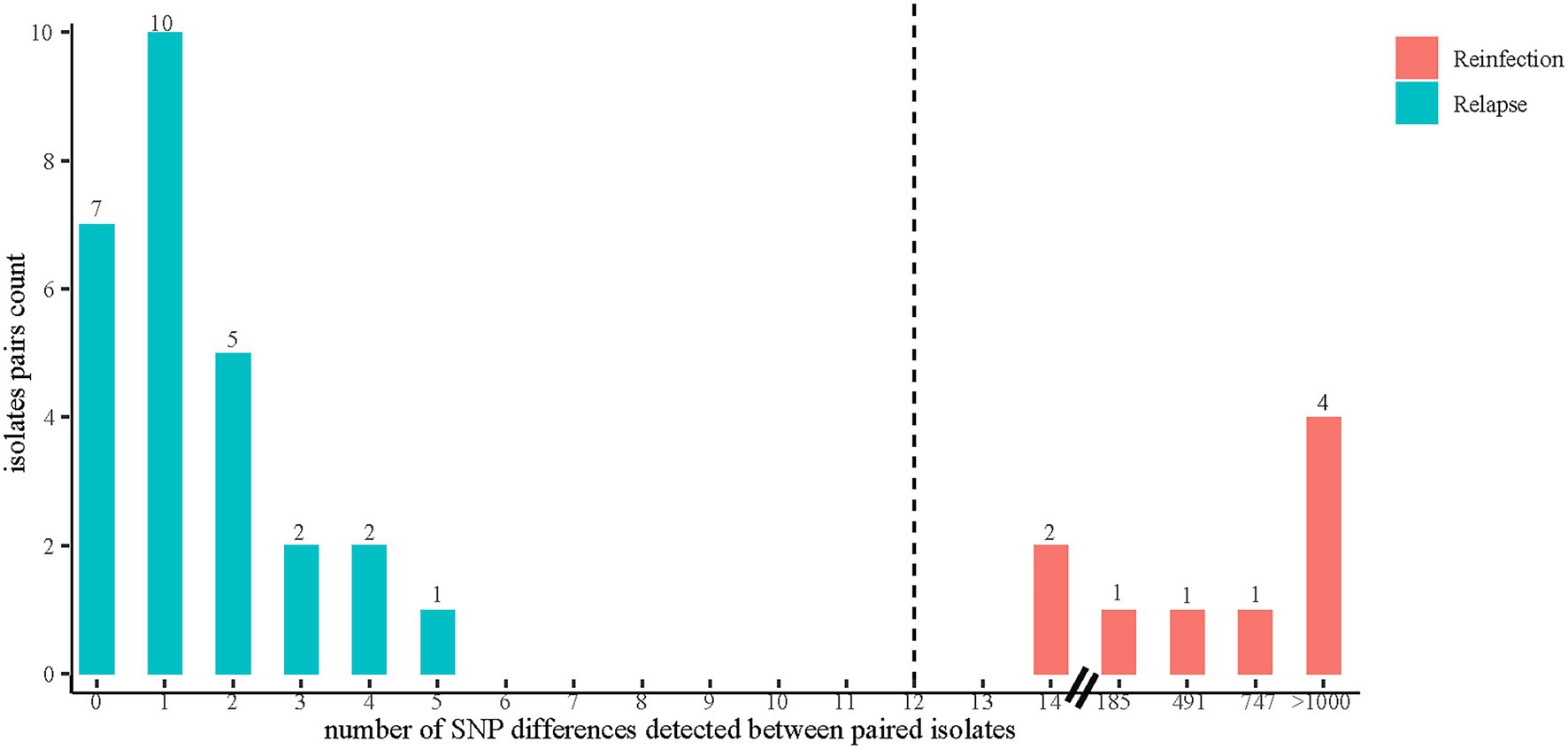
Figure 2. The distribution of SNP differences between paired isolates. Reinfection was defined as a recurrent disease episode caused by a new TB strain with a genetic distance of more than 12 SNPs compared with the strain that caused the original episode. Relapse was defined as a genetic distance of 12 or fewer SNPs between paired strains isolated from two episodes in TB recurrence. The SNP differences between paired isolates were calculated by using Snp-dists (v.0.8.2).
3.4. Phylogenetic reconstructions and drug-resistant profile
The phylogenetic tree was constructed based on 6,847 high-quality SNPs (Figure 3). Fast-lineage-caller analysis showed that the majority (59.4%, 41/69) of recurrent TB isolates were lineage 2, and 40.6% (28/69) were lineage 4. All the isolate pairs from relapse cases were close together on the tree, whereas almost the reinfected isolate pairs appeared quite divergent on the tree (marked in different colors) (Figure 3). We also analyzed the community transmission of these recurrent TB cases, as demonstrated in Figure 3, TB strains collected from different individuals did not show high sequence similarity. Among these recurrent TB isolates, pan-susceptible predominated, accounting for 71.0% (49/69), with only 8 (11.6%, 8/69) and 12 (17.4%, 12/69) were identified as MDR-TB and DR-TB, respectively. To rationalize these phenotypic drug-resistance, genetic mutations were predicted based on WGS data. A total of 9 recurrent TB strains were identified as genomic MDR-TB, mainly with mutations in codon 450 of the rpoB gene and codon 315 of the katG gene (Table 1). Moreover, 2 strains had detectable drug-resistant mutations to four first-line anti-TB drugs (rifampicin, isoniazid, pyrazinamide, and ethambutol) simultaneously. We further compared the drug-resistant profiles between paired isolates to clarify the development of acquired resistance during treatment. As shown in Figure 3, three relapse cases had acquired new resistance during treatment: two (patient 21 and 26) to fluoroquinolones were both due to mutations in codon 94 of gyrA and one (patient 27) to ethambutol due to a mutation in codon 406 of embB, resulting in amino acid substitution from Gly to Asp (Table 1). Of note, the strain from patient 21, which was MDR with additional resistance to ethambutol and pyrazinamide in the first isolate, had progressed to pre-XDR in their second relapsed isolate. Interestingly, one patient (patient 15) had three TB episodes between 2015 and 2019, of which the first and third episodes isolated the identical TB strain, both MDR-TB, while the new strain isolated from the second was pan-susceptible (Figure 3, Table 1). In addition, one patient (patient 10) was initially infected with a pan-susceptible strain and subsequently reinfected with a new strain that harbored gene mutations related to rifampin and isoniazid resistance (Table 1).
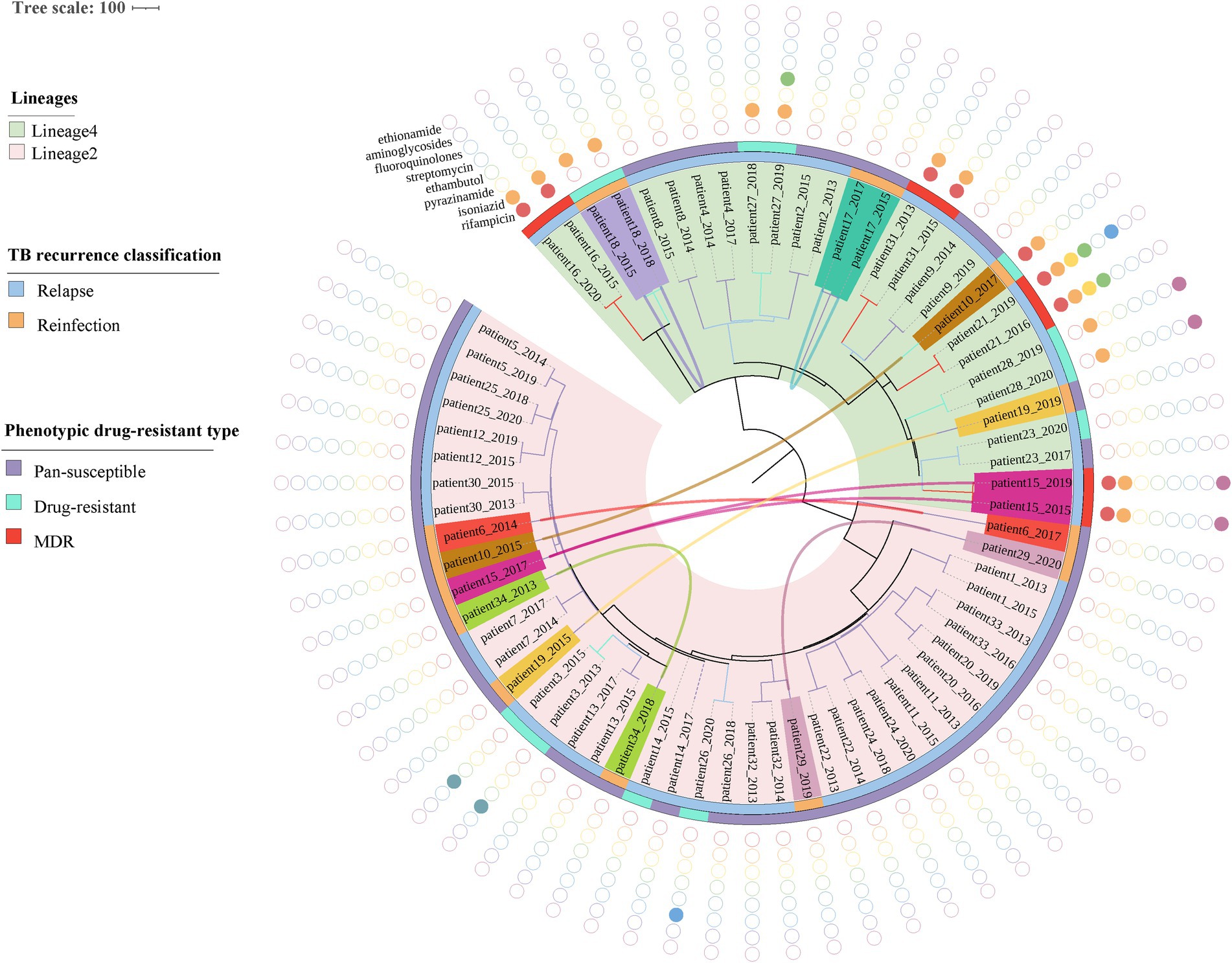
Figure 3. Phylogenetic tree and drug-resistant profile of 69 MTB strains from 34 recurrent patients. Inner band indicates TB recurrence classification (reinfection represents strain pairs differences >12 SNPs, whereas relapse represents strain pairs differences ≤12 SNPs) and the outer band suggests phenotypic drug-resistant type (see legend). Solid circles indicate genetic drug resistance detected by TB-profiler. Reinfected patients are highlighted with different colors and curves connecting patients’ samples in the phylogeny indicating paired strains isolated from the same patient.
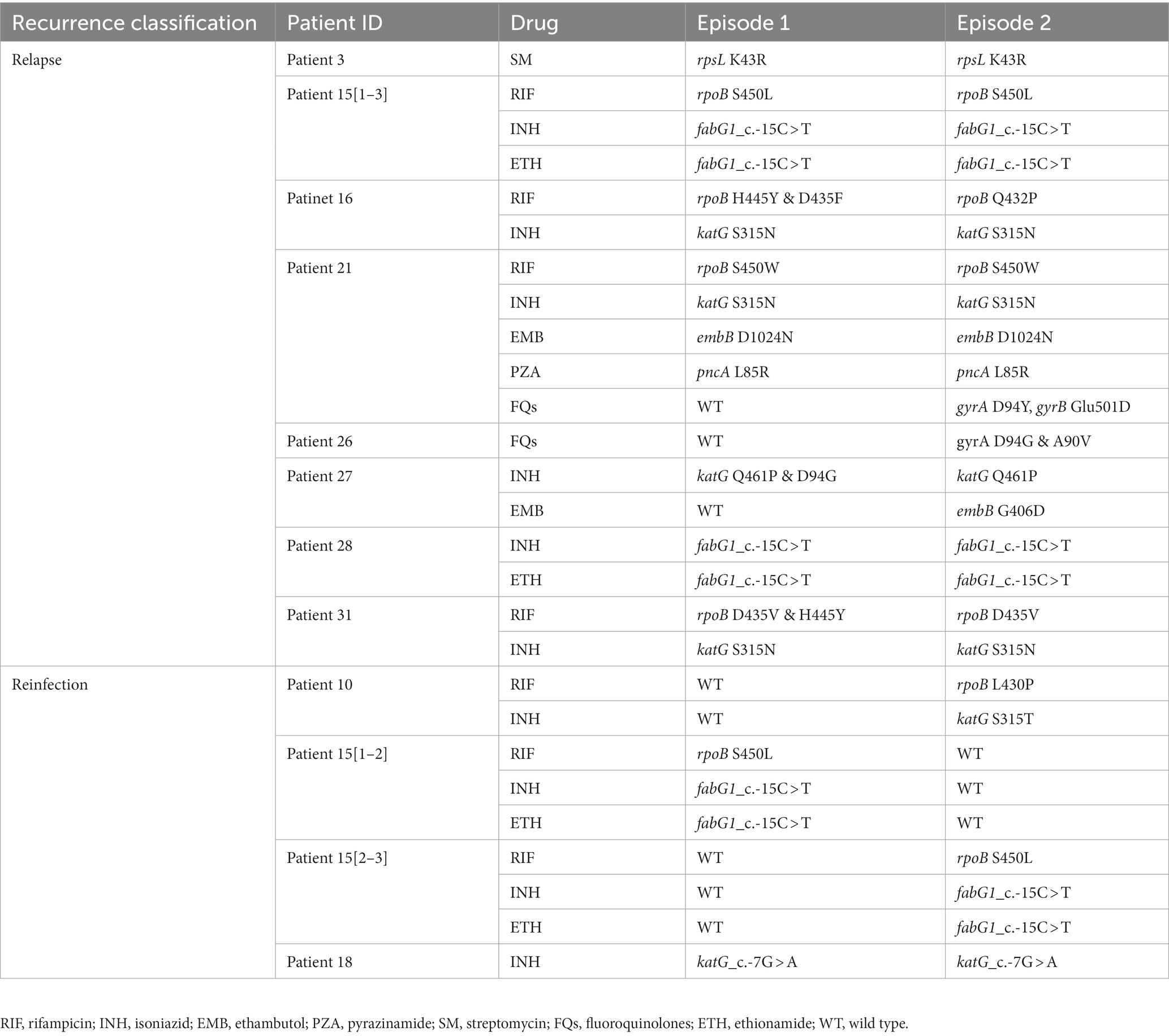
Table 1. Genetic mutations related to drug-resistance identified in the first and second episodes of recurrent TB isolates.
3.5. Comparison of the characteristics between relapse and reinfection
We analyzed the differences in the characteristics between TB relapse and reinfection. As summarized in Table 2, all these demographic factors and clinical characteristics of patients, such as gender, age, occupation, and comorbidities et al., as well as genetic background and drug-resistant type of strains, had no significant effect on the proportion of TB relapse (all p > 0.05). In addition, more than 80% of TB recurrence occurred within three years after completion of treatment for the index episode (Table 2). The median of the recurrent time interval to relapse was 17.6 months (IQR, 12.9–28.3 months) compared with 24.3 months (IQR, 12.9–31.5 months) for reinfection cases, and there was no significant association between relapse and earlier recurrence (p = 0.51) (Figure 4A). We further assessed the time interval to relapse stratified by gender, nationality, pulmonary cavity, strain drug-resistant type, and genetic background. As shown in Figure 4, TB relapse occurs earlier in patients of Tu ethnicity compared to patients of Han ethnicity (p < 0.0001), whereas no significant differences in the time interval to relapse were noted in other groups.
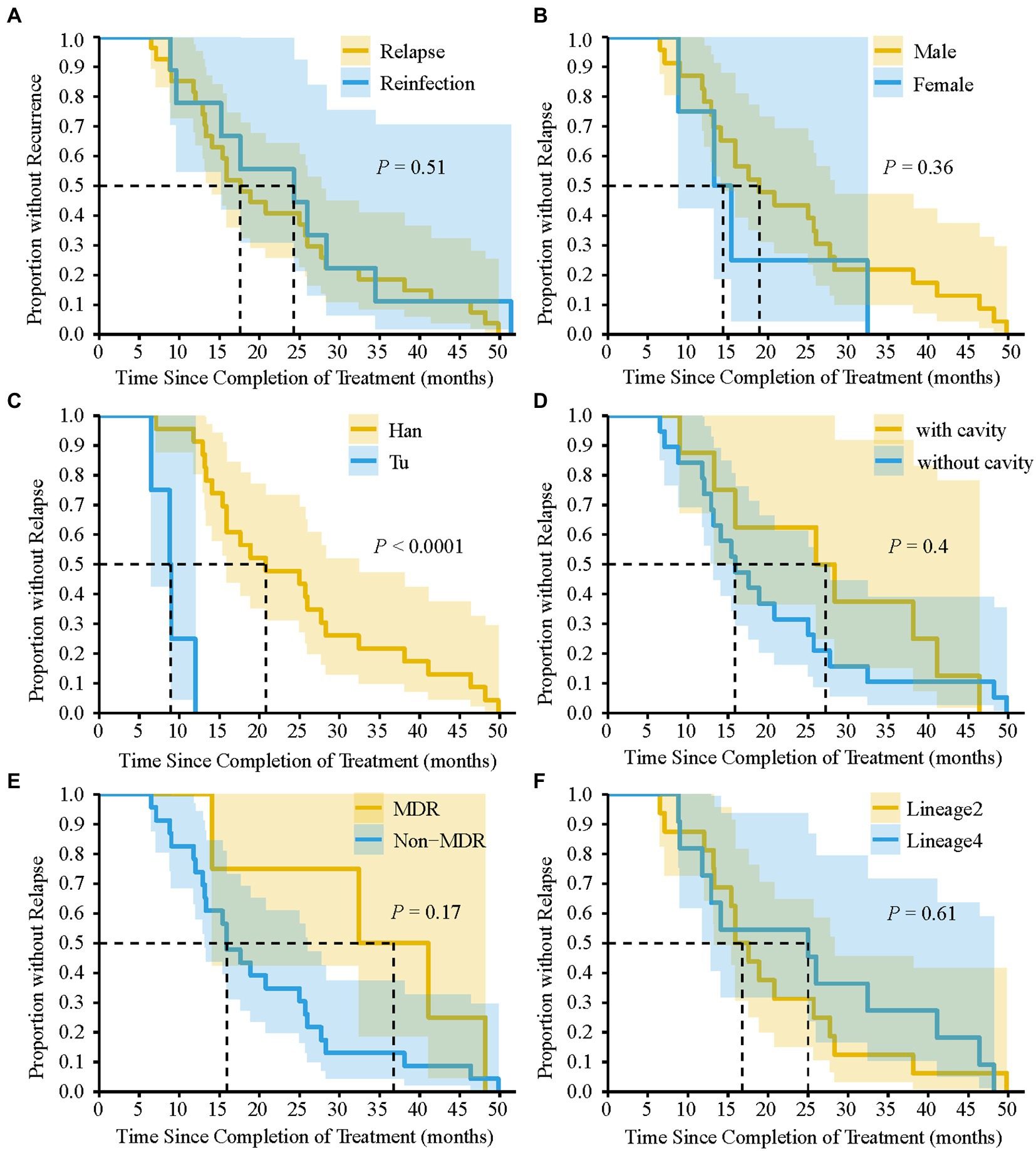
Figure 4. Kaplan–Meier survival estimates for TB recurrence. Comparison of the time interval required for relapse and reinfection (A). The time interval required for relapse to occur by gender (B), nationality (C), pulmonary cavity (D), MDR-TB (E), and Lineage of TB strains (F). dashed line indicate that the time interval required for 50% of TB recurrence or relapse to occur. p-value was calculated by Log-rank test.
3.6. SNPs in relapse isolate
Of 39 SNPs and small indels (insertion–deletion) identified between the relapse pairs, 23 were non-synonymous polymorphisms. These mutations are located in genes encoding proteins with various functions, such as cell wall and cellular process, lipid metabolism, and information pathways (Supplementary Table S1). In three and four cases, these mutations were involved in drug-resistant related genes and growth advantage regions, respectively. We also identified 6 indels differences that result in frame-shifts within protein-coding regions, but all of these indels were located in non-essential regions (Supplementary Table S1).
4. Discussion
To our knowledge, this is the first longitudinal population-based study of sufficient duration to investigate TB recurrence using WGS in Hunan, China. The current study found a relatively high frequency of mixed infections among recurrent TB cases. After excluding patients with mixed infections, our study demonstrated that TB recurrence in Hunan province is mainly caused by endogenous reactivation of the initial infection (relapse), and reinfection accounted for a quarter of recurrent cases. In addition, our study found TB recurrence can occur even more than 4 years after treatment completion of the most recent episode, mainly within 3 years. Evidence of acquired resistance during treatment was also observed in this study, with fluoroquinolone resistance occurring most frequently.
Understanding the proportion of reinfection and relapse will help to implement better post-treatment follow-up and reduce TB burden. Unexpectedly high rates of reinfection suggest that reducing the risk of TB transmission is fundamental, while higher rates of relapse suggest that TB control should focus on improving the efficacy of the first-episode treatment regimen (Folkvardsen et al., 2020; Du et al., 2021). Numerous studies have shown that the proportion of TB recurrence due to exogenous reinfection varies by regions (Bandera et al., 2001; Verver et al., 2005; Zong et al., 2018; Liu et al., 2022). It is generally accepted that the proportion of reinfection in TB recurrence is higher in settings with a high prevalence of TB (Vega et al., 2021; Li et al., 2022), but there are exceptions (Shamputa et al., 2007). Studies of countries with low to moderate TB incidence found that the percentage of reinfection ranging from 10% in Switzerland to 33% in Spain (Schiroli et al., 2015), while reinfection was common in studies of high-burden countries, ranging from 23% in India to 68–77% in South Africa (Sahadevan et al., 1995; van Rie et al., 1999; Charalambous et al., 2008). Our study found that 25% of TB recurrence were attributed to reinfection, which was comparable to the proportion reported in Jiangsu (28.9%) (Liu et al., 2022), but much higher than that reported in Beijing (8.8%) (Du et al., 2021). Several reasons could be responsible for such variation of the percentage of reinfection. Firstly, the varied duration of follow-up would potentially affect the proportion of recurrence due to reactivation and reinfection (Liu et al., 2022). In general, relapse occurs earlier than reinfection, and if cases were followed up for an insufficient period, reinfections would not be captured (Vega et al., 2021), leading to a relatively lower proportion of reinfection. Secondly, different genomic-based typing methods, such as MIRU-VNTR, IS6110 fingerprinting, and whole genome sequencing, have different discriminatory power that can make a difference in the classification of TB recurrence (Shao et al., 2021). In addition, some of the patients’ complications could increase the risk from infection to disease, resulting in more reinfection cases (Lieberman et al., 2016). Moreover, transmission dynamics were also analyzed in our study and community transmission was not observed among these recurrent TB cases, which might due to transmission occurring in a broader population that was not included in our study population.
Mixed infections can complicate TB diagnosis and treatment, and it is also one of the potential confounders in distinguishing relapse from reinfection (Witney et al., 2017). To reduce the misclassification of recurrent TB cases, detection of mixed infection based on whole genome sequencing before determining the main source of TB recurrence is very essential. By using QuantTB, a method for identifying and quantifying individual MTB strains at high resolution (Anyansi et al., 2020), 7 of 79 (8.9%) isolates in this study were identified as mixed infections. Although the sampling and culture methods used in this study may lower the diversity of strains (Liu et al., 2020), a relatively high proportion of mixed infections were still detected, which warned of the urgent need for further studies to determine the prevalence of mixed infections in different settings and its impact on heterogeneous drug-resistance.
Of note, two patients’ pair (patient 17 and patient 18) of isolates in this study displayed 14 SNPs (SNP > 12) between two episodes and were therefore initially classified as reinfection. However, further analysis showed that the strain pairs were located next to each other on the phylogenetic tree and shared the same drug-resistant profile (Figure 2), suggesting that these two recurrent cases were likely caused by relapse. This would leave 7 recurrent cases with paired isolates differing by more than 180 SNPs that were clearly identified as the result of reinfection, indicating that 80.6% of TB recurrences were caused by relapse. The data here was supported by the findings of Walker and colleagues that the diversity between the initial and later isolates from relapsed patients does not generally exceed 14 SNPs, with most cases differing by less than five (Walker et al., 2013). Based on these results, it is reasonable to assume that strains with SNP differences slightly exceeding the thresholds (commonly 6 or 12 SNPs) used to define a cluster may occasionally belong to the same transmission chain and should be taken into account during the epidemiological investigation (Liu et al., 2020). More importantly, similar to previous studies, our study only found reinfections with large phylogenetic distances (range 185–1,074), but nothing at an intermediary level (Witney et al., 2017). This suggests that primary infection does not provide sufficient immune protection against genetically distant strains, which has important implications for future vaccine design (Bryant et al., 2013).
The emergence of drug resistance in relapsed TB weakens the effectiveness of subsequent treatment. In the present study, we found that the acquisition of resistance to fluoroquinolones was the most common during treatment, and this observation was further rationalized by genotypic resistance prediction based on whole-genome sequencing. Similar results have been reported elsewhere (Zong et al., 2018; Du et al., 2021). Although the exact cause of this phenomenon remains unclear, it can be partially explained by the abuse and misuse of fluoroquinolones. In China, because of their broad-spectrum antimicrobial activity, fluoroquinolones are always used as empirical treatment for suspected TB patients and various other types of infections (Du et al., 2021). Consequently, the selection pressure on MTB generated by residual drugs in the host allows the survival and accumulation of drug-resistant strains, resulting in strains with drug-resistance becoming the dominant population. Consistent with our findings, numerous previous studies have confirmed significantly increased prevalence of fluoroquinolones resistance in recent years in China (Xia et al., 2021; Mave et al., 2022). In addition, experimental data showed that fluoroquinolones activate the SOS response, which is likely to be associated with an elevated mutation rate. This may be another important factor contributing to the high frequency of fluoroquinolones resistance (Iacobino et al., 2021).
TB relapse was determined by a wide range of factors, such as socio-demographic and clinical features of TB cases, drug resistance and genetic background of the bacteria, and the disease burden of the study settings (Romanowski et al., 2019). Previous studies have shown that patients infected with Beijing genotype or isoniazid resistant strains were more susceptible to relapse (Hang et al., 2015; Thai et al., 2018). Besides, Romanowski et al. already found that despite poor predictive ability, cavitary disease and 2-month smear positivity could be used as markers for higher risk of relapse (Romanowski et al., 2019). However, in our current study, the relatively small sample size of recurrent TB cases limits our ability to detect significant difference between relapse and reinfection. To make follow-up for TB relapse more practical, future studies could identify socio-environmental and bio-medical factors associated with relapse by using modeling studies or genome-wide association analysis (GWAS), so these can be addressed or guide care after cure. Understanding the time interval distribution of recurrence is important for developing post-treatment control strategies and designing clinical trial studies (Marx et al., 2014). A meta-analysis reported that relapse occurred mainly in the first year after the end of treatment, while late recurrences tended to be reinfections (Romanowski et al., 2019). However, in our study, there was no significant difference in the time interval between relapse and reinfection. TB recurrences, whether caused by relapse or reinfection, occur predominantly within 3 years after completion of therapy. Therefore, for better management of TB patients in this region, we recommend that patients should be followed-up for at least 3 years after completion of therapy. Moreover, we further assessed the time interval to relapse stratified by gender, nationality, pulmonary cavity, et al. Despite the small sample size, a correlation was observed in the present study between Tu nationality and earlier relapse. Further study with an expanded sample size is needed to explore whether there is a genuine correlation between nationality and time interval to relapse.
A major strength of this study is that we conducted a retrospective study of sufficient duration by using whole-genome sequencing data of serial strains from recurrent TB patients, which allowed us to get a more accurate picture of the proportion of recurrence caused by reinfection after excluding mixed infections, as well as to understand the drug resistance acquired during treatment. We must acknowledge several limitations of this study. First, this study was based on routinely collected information and specimens. Some TB recurrent cases might be lost due to death or moving out of the region, which would reduce the accuracy of our results. Second, recurrent TB cases who were excluded from the final analysis due to subculture failure and contamination of any paired isolates may introduce selection bias into this study. Third, the relatively small sample size of drug-resistant TB strains restricted us from exploring the underlying mechanism of acquired drug resistance during treatment. Lastly, the HIV status of most recurrent TB cases in this study is unknown, but given the low prevalence of HIV in this area, we believe this is unlikely to introduce bias to the results of our study.
In conclusion, our data demonstrate that endogenous relapse is the main mechanism leading to TB recurrences in Hunan province. Additionally, our study found TB recurrence can occur even more than 4 years after treatment completion of the most recent episode, mainly within 3 years. Therefore, it is necessary to extend the post-treatment follow-up period to achieve better management of TB patients. Moreover, the relatively high frequency of fluoroquinolone-resistance in the second episode of relapse suggests that fluoroquinolones should be used with caution when treating TB cases with relapse, preferably guided by DST results.
Data availability statement
The datasets presented in this study can be found in online repositories. The names of the repository/repositories and accession number(s) can be found in the article/Supplementary material.
Ethics statement
Ethical approval was not provided for this study on human participants because National TB drug-resistant surveillance (DRS) was ethically approved by the Ethics Committee of Chinese Center for Disease Control and Prevention since the first national survey in 2007 (Zhao et al., 2012). Ethics approval of the present study was waived because all TB isolates used in this study were obtained from previous DRS routine work, and patient information was extracted from the previous database, no additional data and specimens were collected. Patients/participants provided their written informed consent at the time of their first visit to the designated TB clinics or centers.
Author contributions
WH and YZ contributed to study design, data analysis, and manuscript writing. YT, ZS, BL, CL, HZ, DL, SP, and FH participated in study design, data collection, and analysis. YW, PH, AM, XC, and BZ conducted laboratory testing. HX, SW, and XO revised and polished the manuscript. All the authors have read the final version of the manuscript and have approved it.
Funding
This work was supported by the National Key R&D Program (No. 2022YFC2305200) and Natural Science Foundation of Xinjiang Uygur Autonomous Region (No. 2022D01A115).
Acknowledgments
We would like to show our great gratitude to the local medical staff for their hard work in collecting clinical data and tuberculosis isolates.
Conflict of interest
The authors declare that the research was conducted in the absence of any commercial or financial relationships that could be construed as a potential conflict of interest.
Publisher’s note
All claims expressed in this article are solely those of the authors and do not necessarily represent those of their affiliated organizations, or those of the publisher, the editors and the reviewers. Any product that may be evaluated in this article, or claim that may be made by its manufacturer, is not guaranteed or endorsed by the publisher.
Supplementary material
The Supplementary material for this article can be found online at: https://www.frontiersin.org/articles/10.3389/fmicb.2023.1115295/full#supplementary-material
Footnotes
1. ^https://www.bioinformatics.babraham.ac.uk/projects/fastqc/
3. ^https://github.com/AbeelLab/quanttb
References
Anyansi, C., Keo, A., Walker, B. J., Straub, T. J., Manson, A. L., Earl, A. M., et al. (2020). QuantTB – a method to classify mixed Mycobacterium tuberculosis infections within whole genome sequencing data. BMC Genomics 21:80. doi: 10.1186/s12864-020-6486-3
Bandera, A., Gori, A., Catozzi, L., Degli Esposti, A., Marchetti, G., Molteni, C., et al. (2001). Molecular epidemiology study of exogenous reinfection in an area with a low incidence of tuberculosis. J. Clin. Microbiol. 39, 2213–2218. doi: 10.1128/jcm.39.6.2213-2218.2001
Barbier, M., and Wirth, T. (2016). The evolutionary history, demography, and spread of the Mycobacterium tuberculosis complex. Microbiol. Spectr. 4:4. doi: 10.1128/microbiolspec.TBTB2-0008-2016
Bolger, A. M., Lohse, M., and Usadel, B. (2014). Trimmomatic: a flexible trimmer for Illumina sequence data. Bioinformatics 30, 2114–2120. doi: 10.1093/bioinformatics/btu170
Bryant, J. M., Harris, S. R., Parkhill, J., Dawson, R., Diacon, A. H., van Helden, P., et al. (2013). Whole-genome sequencing to establish relapse or re-infection with mycobacterium tuberculosis: a retrospective observational study. Lancet Respir. Med. 1, 786–792. doi: 10.1016/s2213-2600(13)70231-5
Charalambous, S., Grant, A. D., Moloi, V., Warren, R., Day, J. H., van Helden, P., et al. (2008). Contribution of reinfection to recurrent tuberculosis in south African gold miners. Int. J. Tuberc. Lung Dis. 12, 942–948.
Du, J., Li, Q., Liu, M., Wang, Y., Xue, Z., Huo, F., et al. (2021). Distinguishing relapse from reinfection with whole-genome sequencing in recurrent pulmonary tuberculosis: a retrospective cohort study in Beijing, China. Front. Virol. 12:754352. doi: 10.3389/fmicb.2021.754352
Folkvardsen, D. B., Norman, A., Rasmussen, E. M., Lillebaek, T., Jelsbak, L., Andersen, Å., et al. (2020). Recurrent tuberculosis in patients infected with the predominant mycobacterium tuberculosis outbreak strain in Denmark. New insights gained through whole genome sequencing. Infect. Genet. Evol. 80:104169. doi: 10.1016/j.meegid.2020.104169
Freschi, L., Vargas, R. Jr., Husain, A., Kamal, S. M. M., Skrahina, A., Tahseen, S., et al. (2021). Population structure, biogeography and transmissibility of Mycobacterium tuberculosis. Nat. Commun. 12:6099. doi: 10.1038/s41467-021-26248-1
Hang, N. T., Maeda, S., Keicho, N., Thuong, P. H., and Endo, H. (2015). Sublineages of Mycobacterium tuberculosis Beijing genotype strains and unfavorable outcomes of anti-tuberculosis treatment. Tuberculosis 95, 336–342. doi: 10.1016/j.tube.2015.02.040
He, W., Tan, Y., Liu, C., Wang, Y., He, P., Song, Z., et al. (2022). Drug-resistant characteristics, genetic diversity, and transmission dynamics of rifampicin-resistant mycobacterium tuberculosis in Hunan, China, revealed by whole-genome sequencing. Microbiol. Spectr. 10:e0154321. doi: 10.1128/spectrum.01543-21
Iacobino, A., Piccaro, G., Pardini, M., Fattorini, L., and Giannoni, F. (2021). Moxifloxacin activates the SOS response in Mycobacterium tuberculosis in a dose-and time-dependent manner. Microorganisms 9:255. doi: 10.3390/microorganisms9020255
Jagielski, T., Minias, A., van Ingen, J., Rastogi, N., Brzostek, A., Żaczek, A., et al. (2016). Methodological and clinical aspects of the molecular epidemiology of Mycobacterium tuberculosis and other mycobacteria. Clin. Microbiol. Rev. 29, 239–290. doi: 10.1128/cmr.00055-15
Lambert, M. L., Hasker, E., Van Deun, A., Roberfroid, D., Boelaert, M., and Van der Stuyft, P. (2003). Recurrence in tuberculosis: relapse or reinfection? Lancet Infect. Dis. 3, 282–287. doi: 10.1016/s1473-3099(03)00607-8
Li, M., Qiu, Y., Guo, M., Zhang, S., Wang, G., Wang, Y., et al. (2022). Investigation on the cause of recurrent tuberculosis in a rural area in China using whole-genome sequencing: a retrospective cohort study. Tuberculosis 133:102174. doi: 10.1016/j.tube.2022.102174
Lieberman, T. D., Wilson, D., Misra, R., Xiong, L. L., Moodley, P., Cohen, T., et al. (2016). Genomic diversity in autopsy samples reveals within-host dissemination of HIV-associated Mycobacterium tuberculosis. Nat. Med. 22, 1470–1474. doi: 10.1038/nm.4205
Liu, Q., Qiu, B., Li, G., Yang, T., Tao, B., Martinez, L., et al. (2022). Tuberculosis reinfection and relapse in eastern China: a prospective study using whole-genome sequencing. Clin. Microbiol. Infect. 28, 1458–1464. doi: 10.1016/j.cmi.2022.05.019
Liu, Q., Wei, J., Li, Y., Wang, M., Su, J., Lu, Y., et al. (2020). Mycobacterium tuberculosis clinical isolates carry mutational signatures of host immune environments. Sci. Adv. 6:eaba4901. doi: 10.1126/sciadv.aba4901
Marx, F. M., Dunbar, R., Enarson, D. A., Williams, B. G., Warren, R. M., van der Spuy, G. D., et al. (2014). The temporal dynamics of relapse and reinfection tuberculosis after successful treatment: a retrospective cohort study. Clin. Infect. Dis. 58, 1676–1683. doi: 10.1093/cid/ciu186
Mave, V., Chen, L., Ranganathan, U. D., Kadam, D., Vishwanathan, V., Lokhande, R., et al. (2022). Whole genome sequencing assessing impact of diabetes mellitus on tuberculosis mutations and type of recurrence in India. Clin. Infect. Dis. 75, 768–776. doi: 10.1093/cid/ciab1067
Roetzer, A., Diel, R., Kohl, T. A., Rückert, C., Nübel, U., Blom, J., et al. (2013). Whole genome sequencing versus traditional genotyping for investigation of a Mycobacterium tuberculosis outbreak: a longitudinal molecular epidemiological study. PLoS Med. 10:e1001387. doi: 10.1371/journal.pmed.1001387
Romanowski, K., Balshaw, R. F., Benedetti, A., Campbell, J. R., Menzies, D., Ahmad Khan, F., et al. (2019). Predicting tuberculosis relapse in patients treated with the standard 6-month regimen: an individual patient data meta-analysis. Thorax 74, 291–297. doi: 10.1136/thoraxjnl-2017-211120
Ruan, Q. L., Yang, Q. L., Sun, F., Liu, W., Shen, Y. J., Wu, J., et al. (2022). Recurrent pulmonary tuberculosis after treatment success: a population-based retrospective study in China. Clin. Microbiol. Infect. 28, 684–689. doi: 10.1016/j.cmi.2021.09.022
Sahadevan, R., Narayanan, S., Paramasivan, C. N., Prabhakar, R., and Narayanan, P. R. (1995). Restriction fragment length polymorphism typing of clinical isolates of Mycobacterium tuberculosis from patients with pulmonary tuberculosis in Madras, India, by use of direct-repeat probe. J. Clin. Microbiol. 33, 3037–3039. doi: 10.1128/jcm.33.11.3037-3039.1995
Schiroli, C., Carugati, M., Zanini, F., Bandera, A., Di Nardo Stuppino, S., Monge, E., et al. (2015). Exogenous reinfection of tuberculosis in a low-burden area. Infection 43, 647–653. doi: 10.1007/s15010-015-0759-9
Shamputa, I. C., Van Deun, A., Salim, M. A., Hossain, M. A., Fissette, K., de Rijk, P., et al. (2007). Endogenous reactivation and true treatment failure as causes of recurrent tuberculosis in a high incidence setting with a low HIV infection. Tropical Med. Int. Health 12, 700–708. doi: 10.1111/j.1365-3156.2007.01840.x
Shao, Y., Song, H., Li, G., Li, Y., Li, Y., Zhu, L., et al. (2021). Relapse or re-infection, the situation of recurrent tuberculosis in eastern China. Front. Cell. Infect. Microbiol. 11:638990. doi: 10.3389/fcimb.2021.638990
Shen, X., Yang, C., Wu, J., Lin, S., Gao, X., Wu, Z., et al. (2017). Recurrent tuberculosis in an urban area in China: relapse or exogenous reinfection? Tuberculosis 103, 97–104. doi: 10.1016/j.tube.2017.01.007
Thai, P. V. K., Ha, D. T. M., Hanh, N. T., Day, J., Dunstan, S., Nhu, N. T. Q., et al. (2018). Bacterial risk factors for treatment failure and relapse among patients with isoniazid resistant tuberculosis. BMC Infect. Dis. 18:112. doi: 10.1186/s12879-018-3033-9
van Rie, A., Warren, R., Richardson, M., Victor, T. C., Gie, R. P., Enarson, D. A., et al. (1999). Exogenous reinfection as a cause of recurrent tuberculosis after curative treatment. N. Engl. J. Med. 341, 1174–1179. doi: 10.1056/nejm199910143411602
Vega, V., Rodríguez, S., Van der Stuyft, P., Seas, C., and Otero, L. (2021). Recurrent TB: a systematic review and meta-analysis of the incidence rates and the proportions of relapses and reinfections. Thorax 76, 494–502. doi: 10.1136/thoraxjnl-2020-215449
Verver, S., Warren, R. M., Beyers, N., Richardson, M., van der Spuy, G. D., Borgdorff, M. W., et al. (2005). Rate of reinfection tuberculosis after successful treatment is higher than rate of new tuberculosis. Am. J. Respir. Crit. Care Med. 171, 1430–1435. doi: 10.1164/rccm.200409-1200OC
Walker, T. M., Ip, C. L., Harrell, R. H., Evans, J. T., Kapatai, G., Dedicoat, M. J., et al. (2013). Whole-genome sequencing to delineate Mycobacterium tuberculosis outbreaks: a retrospective observational study. Lancet Infect. Dis. 13, 137–146. doi: 10.1016/s1473-3099(12)70277-3
Walker, T. M., Kohl, T. A., Omar, S. V., Hedge, J., Del Ojo Elias, C., Bradley, P., et al. (2015). Whole-genome sequencing for prediction of Mycobacterium tuberculosis drug susceptibility and resistance: a retrospective cohort study. Lancet Infect. Dis. 15, 1193–1202. doi: 10.1016/s1473-3099(15)00062-6
Witney, A. A., Bateson, A. L., Jindani, A., Phillips, P. P., Coleman, D., Stoker, N. G., et al. (2017). Use of whole-genome sequencing to distinguish relapse from reinfection in a completed tuberculosis clinical trial. BMC Med. 15:71. doi: 10.1186/s12916-017-0834-4
World Health Organization (WHO) (2020). Global Tuberculosis Report 2020. Geneva: World Health Organization.
World Health Organization (WHO) (2013). Definitions and reporting framework for tuberculosis–2013 revision: Updated December 2014 and January 2020. Geneva: World Health Organization.
Wu, X., Yang, J., Tan, G., Liu, H., Liu, Y., Guo, Y., et al. (2019). Drug resistance characteristics of Mycobacterium tuberculosis isolates from patients with tuberculosis to 12 antituberculous drugs in China. Front. Cell. Infect. Microbiol. 9:345. doi: 10.3389/fcimb.2019.00345
Xia, H., Zheng, Y., Liu, D., Wang, S., He, W., Zhao, B., et al. (2021). Strong increase in moxifloxacin resistance rate among multidrug-resistant mycobacterium tuberculosis isolates in China, 2007 to 2013. Microbiol. Spectr. 9:e0040921. doi: 10.1128/Spectrum.00409-21
Xia, H., Zheng, Y., Zhao, B., van den Hof, S., Cobelens, F., and Zhao, Y. (2017). Assessment of a 96-well plate assay of quantitative drug susceptibility testing for Mycobacterium tuberculosis complex in China. PLoS One 12:e0169413. doi: 10.1371/journal.pone.0169413
Zhao, Y., Xu, S., Wang, L., Chin, D. P., Wang, S., Jiang, G., et al. (2012). National survey of drug-resistant tuberculosis in China. N. Engl. J. Med. 366, 2161–2170. doi: 10.1056/NEJMoa1108789
Keywords: tuberculosis, recurrence, relapse, reinfection, whole genome sequencing
Citation: He W, Tan Y, Song Z, Liu B, Wang Y, He P, Xia H, Huang F, Liu C, Zheng H, Pei S, Liu D, Ma A, Cao X, Zhao B, Ou X, Wang S and Zhao Y (2023) Endogenous relapse and exogenous reinfection in recurrent pulmonary tuberculosis: A retrospective study revealed by whole genome sequencing. Front. Microbiol. 14:1115295. doi: 10.3389/fmicb.2023.1115295
Edited by:
Sivakumar Shanmugam, National Institute of Research in Tuberculosis (ICMR), IndiaReviewed by:
Balaji Pathakumari, Mayo Clinic, United StatesArunkumar Venkatesan, Upstate Medical University, United States
Copyright © 2023 He, Tan, Song, Liu, Wang, He, Xia, Huang, Liu, Zheng, Pei, Liu, Ma, Cao, Zhao, Ou, Wang and Zhao. This is an open-access article distributed under the terms of the Creative Commons Attribution License (CC BY). The use, distribution or reproduction in other forums is permitted, provided the original author(s) and the copyright owner(s) are credited and that the original publication in this journal is cited, in accordance with accepted academic practice. No use, distribution or reproduction is permitted which does not comply with these terms.
*Correspondence: Yanlin Zhao, ✉ emhhb3lsQGNoaW5hY2RjLmNu
†These authors have contributed equally to this work and share first authorship