- 1Key Laboratory of Resource Biology and Biotechnology in Western China, Ministry of Education, Northwest University, Xi’an, China
- 2College of Life Science, Northwest University, Xi’an, China
- 3State Key Laboratory of Water Resources and Hydropower Engineering Sciences, Institute for Water-Carbon Cycles and Carbon Neutrality, Wuhan University, Wuhan, China
- 4Department of Biology Sciences, Institute of Environment Sciences, University of Quebec at Montreal, Montreal, QC, Canada
- 5Shuanglong State-Owned Ecological Experimental Forest Farm of Qiaoshan State-Owned Forestry Administration of Yan'an City, Yan'an, Shaanxi, China
Soil microorganisms are critical biological indicators for evaluating soil health and play a vital role in carbon (C)-climate feedback. In recent years, the accuracy of models in terms of predicting soil C pools has been improved by considering the involvement of microbes in the decomposition process in ecosystem models, but the parameter values of these models have been assumed by researchers without combining observed data with the models and without calibrating the microbial decomposition models. Here, we conducted an observational experiment from April 2021 to July 2022 in the Ziwuling Mountains, Loess Plateau, China, to explore the main influencing factors of soil respiration (RS) and determine which parameters can be incorporated into microbial decomposition models. The results showed that the RS rate is significantly correlated with soil temperature (TS) and moisture (MS), indicating that TS increases soil C loss. We attributed the non-significant correlation between RS and soil microbial biomass carbon (MBC) to variations in microbial use efficiency, which mitigated ecosystem C loss by reducing the ability of microorganisms to decompose organic resources at high temperatures. The structural equation modeling (SEM) results demonstrated that TS, microbial biomass, and enzyme activity are crucial factors affecting soil microbial activity. Our study revealed the relations between TS, microbial biomass, enzyme activity, and RS, which had important scientific implications for constructing microbial decomposition models that predict soil microbial activity under climate change in the future. To better understand the relationship between soil dynamics and C emissions, it will be necessary to incorporate climate data as well as RS and microbial parameters into microbial decomposition models, which will be important for soil conservation and reducing soil C loss in the Loess Plateau.
1. Introduction
The Intergovernmental Panel on Climate Change (IPCC) assessment reports that global average temperatures will rise by 2.1–3.5°C, and the frequency and intensity of extreme heatwaves and precipitation events are also likely to increase (Tollefson, 2021). This climate change is expected to put general stress on ecosystems. The soil ecosystem is an important part of the terrestrial ecosystem and the hub of material and energy flow in the biosphere (Piao et al., 2010b). Carbon (C) is the basic element of life forms, without which life cannot exist, so the C cycle is one of the most important biogeochemical cycles (Bot and Bernites, 2005), and terrestrial soil C cycle research is an important component of global change research. Soil microbes are largely involved in the soil C cycle and play a crucial role in climate feedback (Jansson and Hofmockel, 2020), including CO2, N2O, and other greenhouse gas emissions. As the most active component of soil, microorganisms are significant biological indicators for evaluating soil health (Fierer et al., 2021). In recent years, it has been proposed that soil microbial characteristics can be used as biological indicators of soil health to guide soil ecosystem management (Schloter et al., 2003; Cardoso et al., 2013). Sicardi et al. (2004) believe that soil microbial characteristics, such as soil respiration (RS), microbial biomass, and enzyme activity, vary significantly from season to season, suggesting that they are sensitive and reliable indicators of changes in soil physicochemical properties.
RS refers to the process by which soil releases CO2 into the atmosphere and the most important component of RS is the heterotrophic respiration of soil microorganisms (Wang et al., 2019). C is stored in the soil as organic matter, its storage is approximately twice that of the atmospheric C pool and it plays a significant role in the C cycle of the terrestrial ecosystem (Mahajan et al., 2021). Therefore, RS can significantly affect the global C cycle in terrestrial ecosystems (Zhou et al., 2009). The world is now experiencing a period of rapid warming due to the effects of human activities and CO2 emissions, and RS, which releases more than 10 times more CO2 into the atmosphere than the combustion of fossil fuel (Marland, 1983), is the second-largest source of continental C fluxes (Hu et al., 2019). Due to the enormous storage capacity of soil organic carbon (SOC), even a small change in soil C storage and RS will significantly affect the CO2 concentration in the atmosphere, thereby affecting the feedback effect of terrestrial ecosystems on climate change (Davidson et al., 2006).
In ecosystems, microorganisms play a crucial role in soil metabolism as decomposers that drive nutrient turnover in soil ecosystems by mineralizing organic matter (Wieder et al., 2015). Soil microbial biomass is the active component of soil organic matter (SOM) and the most active soil factor (Jenkinson and Ladd, 1981). Since soil microbial biomass is very sensitive to environmental factors, slight changes in soil can change it (Chander et al., 1998), so various environmental disturbances can be predicted earlier.
All soil biochemical processes proceed because soil enzymes act as the driving force. An essential soil microbial function is to decompose key nutrients in litter and accumulate organic matter through soil enzymes (Caldwell, 2005). For example, cellobiohydrolase (CBH) and β-1,4-glucosidase (βG) are required to decompose cellulose in a litter (Sinsabaugh et al., 1992), and peroxidase (PER) and polyphenol oxidase (PPO) also play important roles in lignin decomposition (Lucas et al., 2007). Green et al. demonstrated that oxidase is an important factor affecting soil microbial respiration (Green and Oleksyszyn, 2002). In addition, Sinsabaugh et al. (2008) also demonstrated that soil microbial biomass determines the organic matter decomposition process of soil enzymes. Therefore, soil enzyme activity and other soil microbial indicators can be used to identify early warnings of soil ecosystems under stress conditions and anthropogenic disturbances (Boerner R. et al., 2005).
The results of most ecosystem models show that climate change will stimulate the microbial decomposition of SOM and generate feedback on global climate change (Friedlingstein et al., 2006). The positive feedback system model for climate change over time has a poor effect in simulating the global SOC pool and has great uncertainty (Voigt et al., 2016). Therefore, the global ecosystem model needs to consider microbial effects to accurately predict the feedback relationship between climate warming and SOM decomposition (Ji et al., 2018). In recent researches, the accuracy of the models in predicting soil C pools has been improved by considering microbial involvement in the decomposition process in ecosystem models (Abs et al., 2020; Guo et al., 2020), but the parameter values of these models are assumed by researchers without integrating the observed data with the models and calibrating the microbial decomposition models. Therefore, to improve the accuracy of microbial ecosystem models, it is also necessary to calibrate microbial parameters, and RS, microbial biomass, and enzyme activity are the most reliable observations for model calibration and validation (Hanson et al., 2000; Wang et al., 2015). In addition, dynamic data (e.g., soil temperature and moisture) can represent real-world climatic and environmental conditions, which can be beneficial for the model and understanding soil C cycling more realistically (Wang et al., 2020).
Forest soil microorganisms, which are vital part of forest ecosystems, play an important role in the decomposition of litter and soil nutrient cycling (Barberan et al., 2015). Forest RS occupies an important proportion of terrestrial ecosystems, and its dynamic changes will have an important impact on the global C balance (Laganière et al., 2012). Forest RS is also one of the important research objects of the long-term monitoring CO2 flux network currently being established, which is of great significance to scientific ecology and earth system research (Schlesinger and Andrews, 2000).
The Loess Plateau is a mixture of arid, semiarid and semihumid areas but is generally considered a semiarid area (Yu et al., 2020) and has always been known for severe land degradation, low land productivity, and soil erosion (Fu et al., 2016). The Ziwuling Mountains are located in the hinterland of the Loess Plateau, which is a well-preserved natural secondary forest area that plays a critical role in improving the surrounding ecological environment and climate regulation (Kang et al., 2014). From April 2021 to July 2022, we carried out an observational experiment in the Ziwuling Mountains, Loess Plateau, China, to record the monthly diurnal changes in RS and the monthly dynamic changes in soil microbial biomass and enzyme activity. Since soil physicochemical properties can vary significantly at different soil depths (Rahman et al., 2022), we collected topsoil (0–30 cm) and subsoil (30–100 cm) respectively in the process of collecting soil samples. We hypothesized that the topsoil and subsoil physicochemical and microbial properties would be significantly different, and soil microbial properties would also change significantly in different months or seasons. The main goals of this study were to I) explore the main influencing factors of RS and II) determine which parameters can be incorporated into a microbial decomposition model.
2. Materials and methods
2.1. Study site
Field sampling and observation experiments were conducted from April 2021 to July 2022. The study site (Figure 1) was located in the Shuanglong Forest Farm (35°39′ ~ 35°43’N, 108°56′ ~ 108°58′E), a natural secondary forest in the Ziwuling Mountains of North China (Zhang et al., 2022). Our study site was 100 × 100 m. The climate of this site was a warm temperate semihumid climate, with a mean annual temperature of approximately 7.4°C and a mean annual precipitation of 587.6 mm (Chai et al., 2016). The main soil type was loessial soil, which was turbid brown or orange. The soil texture was loose and soft with few roots and pores, which indicated silt loam. The typical arbor species include Betula platyphylla, Swida macrophylla, Carpinus turczaninowii Hance, Quercus aliena Bl, Quercus liaotungensis, Rhus potaninii Maxim, and cer davidii Franch. The typical shrub species include Acer tataricum subsp.ginnala, Viburnum dilatatum Thunb, Cotoneaster multiflorus Bge, Rhamnus leptophylla Schneid, Lonicera hispida Pall. ex Roem. et Schult.
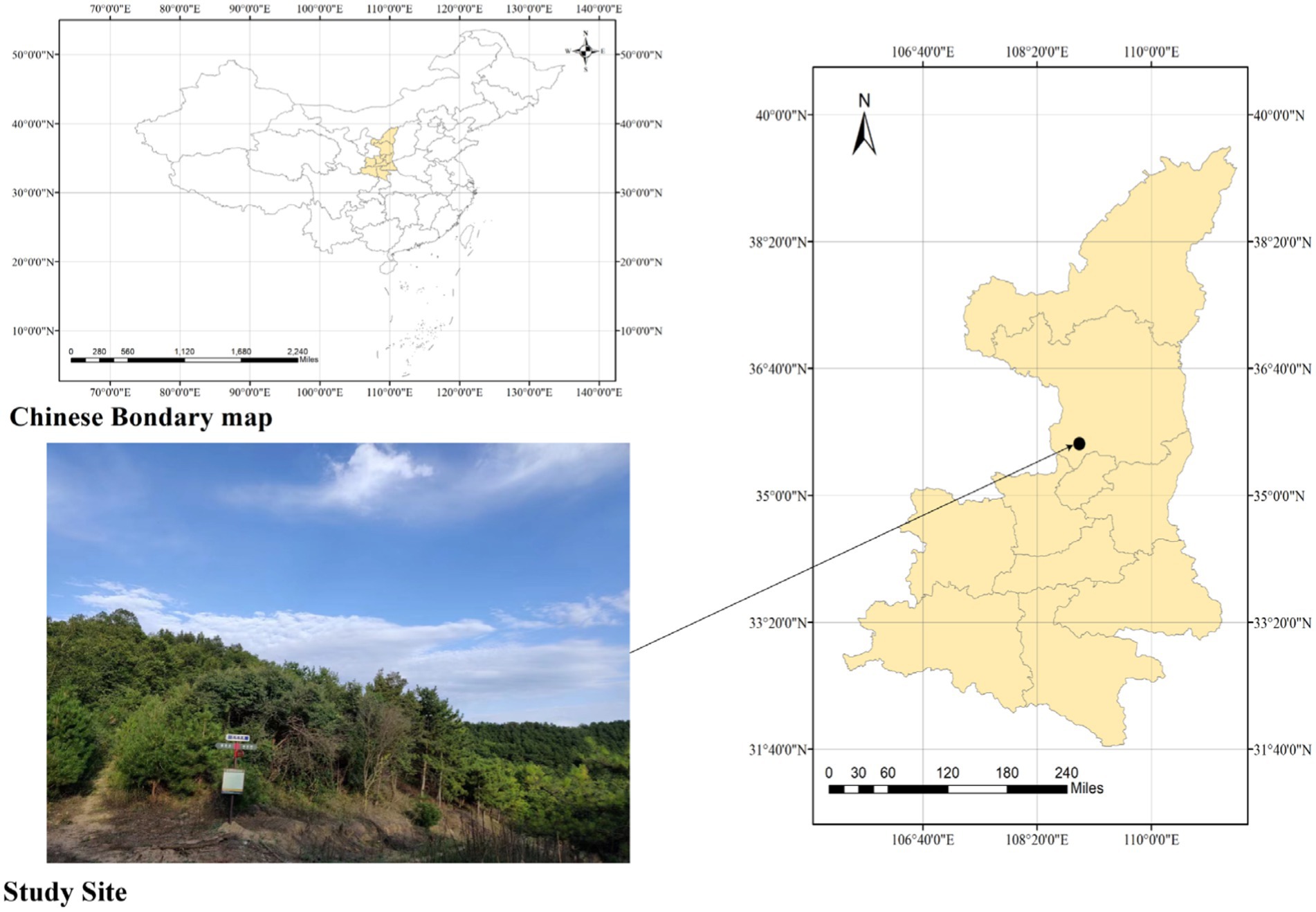
Figure 1. Map of study site. The Shuanglong Forest Farm is located in Shuanglong Town, Huangling County, Yan’an City, Shaanxi Province, China. It is located in the Ziwuling Mountains and is a natural secondary forest.
2.2. RS observation experiment
Three sites with flat terrain were selected as sampling points for the measurement of the RS rate (μ mol m−2 s−1). We installed ACE automatic RS monitoring systems on iron rings with the inner diameter of 20 cm and the height of 10 cm (ACE-200, Ecotech Ecological Technology Ltd) and inserted 4–5 cm into the soil at each sampling point. RS measurement sites were chosen to be more than 50 cm away from the surrounding vegetation, with each site being more than three meters away. To reduce soil disturbance, we inserted the iron rings at least 24 h before the measurement, and the broken roots and litter on the soil surface were removed. From April 2021 to July 2022, we used automatic RS monitoring systems to monitor the RS rate every 30 min for all sample points every month for 24 h. The RS monitoring systems could simultaneously measure and record the soil temperature (TS, °C) and soil moisture (MS, %vol) within 0–10 cm below the surface soil of the sampling site. A meteorological monitoring station (CR200Series) was established at the research site to collect air temperature and moisture data from April 2021 to July 2022.
2.3. Soil sample collection
During the study period, from April 2021 to July 2022, soil sampling was performed every 2 months. Five sampling points were set at the research site using the five-point sampling method. To avoid edge effects, the sampling points were neither close to the edge of the plot nor far from the edge of the sample plot. Each sampling site was 5 × 0.5 m plot. The sampling sites were surrounded by abundant vegetation and the soil surface had obvious humus layers. We divided each sampling point into 12 areas, assigned a random block to all the sampling points, and conducted sampling according to the random block order (Supplementary Figure S1). The sampling depth was divided into two types. The soil at a depth of 0–30 cm below the surface soil was used as topsoil, and the soil at a depth of 30–100 cm was used as subsoil. A total of five replicates were collected separately for topsoil and subsoil. The soil from each depth at each sampling point was mixed evenly after collection, and the broken roots and litter in the soil were removed to reduce errors in the analysis process. Sterile gloves were worn during soil collection to prevent soil contamination. The soil samples were transported in sterile sampling bags, stored in a freezer, and taken to a laboratory by car for further analysis.
2.4. Soil sample analysis
We used the Kjeldahl method (Bradstreet, 1965) to determine the total soil nitrogen (TN), and the soil was hydrolyzed under alkaline conditions in a diffusion dish (Wang, 2010) to calculate the content of alkaline hydrolyzed nitrogen (HN). We used the alkali fusion-Mo-Sb antispectrophotometric method (Chen et al., 2018) to determine the total phosphorus (TP) and sodium bicarbonate solution (Cade-Menun and Lavkulich, 1997) to determine the available phosphorus (AP). The soil-available potassium (AK) was determined by ammonium acetate flame photometry (Zanati et al., 1973).The potassium dichromate oxidation-external heating method was used to determine soil organic matter (SOM), and then the SOM was determined by titration with a standard ferrous iron solution (Zhu et al., 2020). The soil pH was measured by using a pH meter. Microbial biomass carbon (MBC), microbial biomass nitrogen (MBN), and microbial biomass phosphorus (MBP) were determined by using the chloroform fumigation extraction method (Vance and Brookes, 1987). The soil samples were leached with KCL solution and then analyzed using a continuous flow analyzer to determine NH4+--N and NO3−--N (Liu et al., 2014).
We used a fluorometric method (Eivazi and Tabatabai, 1988) to measure the β-1,4-glucosidase (βG) activity in the soil and a nitrophenol colorimetric method (Wood and Bhat, 1988) to measure the cellobiohydrolase (CBH) activity. Polyphenol oxidase (PPO) was determined spectrophotometrically by using pyrogallol (1,2,3-trihydroxy benzene) as a substrate (Bach et al., 2013). Peroxidase (PER) was measured by calculating the rate of substrate oxidation after the addition of H2O2 (Burns et al., 2013).
2.5. Statistical analysis
The RS mean value and error were calculated from three replicate measurements. The mean values and errors of soil physicochemical and microbial properties were calculated from five replicate measurements. Pearson correlation analysis was used to examine the correlation of RS with TS and MS. Origin 2017 software was used to obtain the regression equations between RS rate, TS, and soil MS, and then these regression relationships were plotted. Monthly and seasonal differences in RS and soil physicochemical and microbial properties were tested by ANOVA. The datasets were checked for normality and homogeneity assumptions before performing ANOVA. The magnitude of this feedback largely depends on the temperature sensitivity of SOM decomposition (Q10).
Q10 was measured by the exponential relationship between RS and TS and was calculated as follows:
where TS is the soil temperature, a is the RS rate when the soil temperature is 0°C, and b is the temperature coefficient reflecting the temperature sensitivity of RS.
To examine how soil microbial characteristics influenced RS, structural equation modeling (SEM) was performed with Amos software (IBM SPSS Amos 26.0.0) for different soil layers. In stepwise multiple regression (Supplementary Tables S1, S2), in order to optimize the model, we removed the non-significant variables and paths. We evaluated the goodness of fit of the model according to the low chi-square (χ2; the model is a great fit when 0 ≤ χ2/df ≤ 2) (Tabachnick and Fidell, 2007), the high whole-model p value (if p > 0.05, there is no path loss and the model was a great fit), the comparative fit index (CFI; the model is a great fit when 0.97 ≤ CFI ≤ 1) (Hu and Bentler, 1999), and a root mean square error of approximation (RMSEA; the model is a great fit when 0 ≤ RMSEA ≤0.05) (Vile et al., 2006).
3. Results
3.1. Atmospheric temperature and humidity observation values and soil physicochemical and microbial properties
The monthly variations in air temperature and air moisture are shown in Figure 2. During the observation period from April 2021 to July 2022, the average air temperature was 16.1°C, the highest temperature was 22.2°C, and the lowest temperature was 4.9°C. The average air moisture was 61.7%, the maximum moisture was 75.68%, and the minimum moisture was 46.70% (Figure 2A).
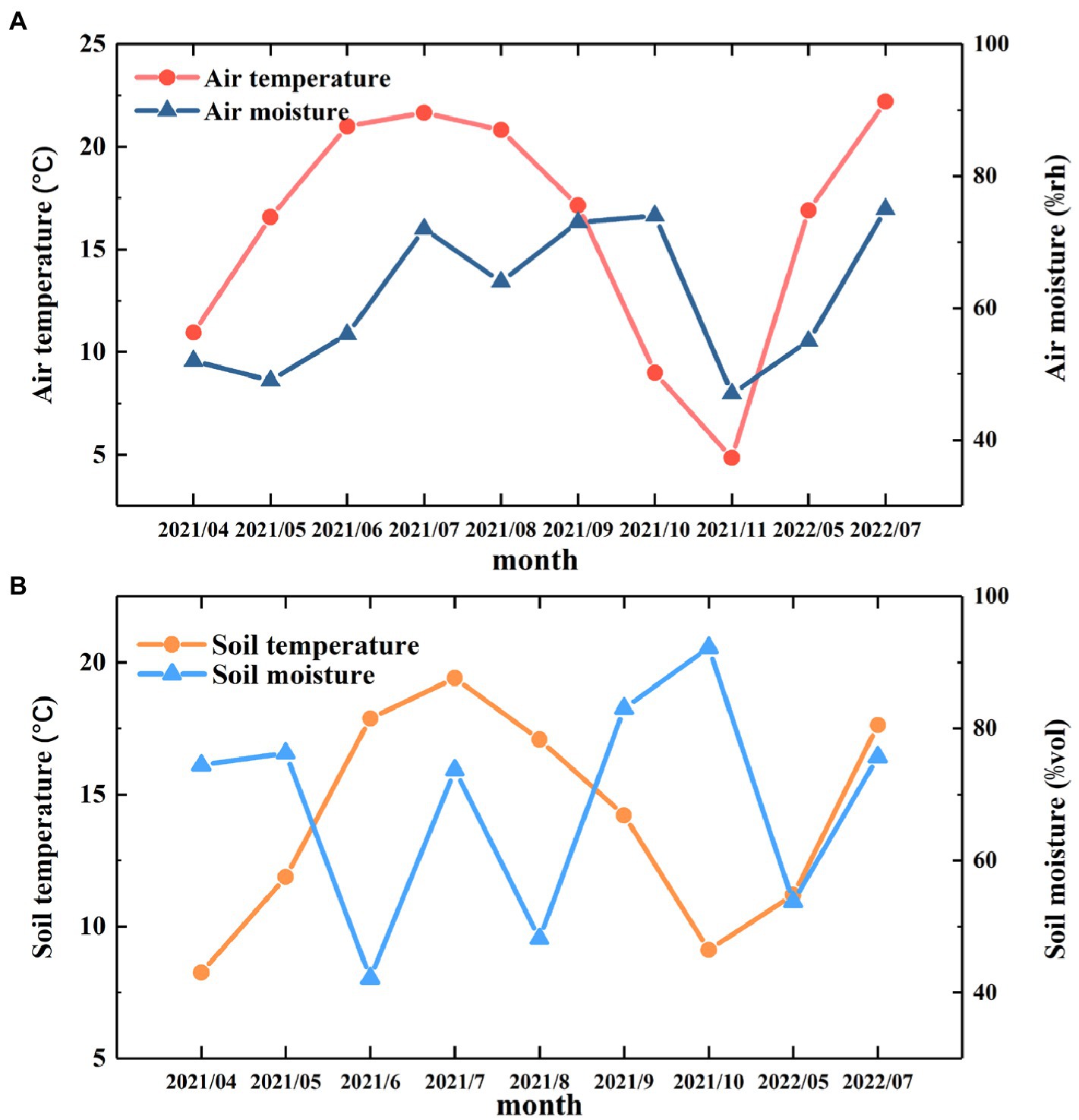
Figure 2. Monthly variations in temperature and moisture. (A) The monthly changes in air temperature and moisture from April 2021 to July 2022. (B) The monthly variations in soil temperature and moisture from April 2021 to July 2022.
During the observation period, the TS showed a pattern consistent with the seasonal variations in air temperature and air moisture. That is, the TS gradually increased from April to July 2021, reaching a maximum value of 19.4°C in July 2021, and then the TS decreased for the rest of the year. The MS had obvious monthly variations during the observation period, reaching a maximum value in October 2021 and minimum value in June 2021 (Figure 2B). The maximum and minimum values were 92.44 and 42.20%, respectively.
The soil at the study site was alkaline, and there was no significant difference in pH between the topsoil (0–30 cm) and the subsoil (30–100 cm) (Table 1). The TP content in the subsoil was significantly higher than that in the topsoil, while the other soil physicochemical properties in the subsoil were lower than those in the topsoil, and AK and SOM were significantly reduced in the subsoil (p < 0.01).
3.2. Diurnal, monthly and seasonal variations in RS
The diurnal variation in the RS rate in different months is shown in Supplementary Figure S2. The RS rate showed a multi-peak distribution trend, and the RS rate reached its peak at noon every day except in April and May within the 24 h observation period of each month. Except in June and August 2021, the RS rate showed a minimum value in the morning (approximately 5:00 to 8:00 AM), and then the RS rate gradually increased. The monthly variation in the RS rate showed a trend of increasing and then decreasing. The RS rate gradually increased after April, reached a maximum in July 2021, and then gradually decreased (Supplementary Figure S3).
The seasonal changes in the RS rate are shown in Figure 3. On the seasonal scale, there were significant differences between the RS rates in different seasons. In 2021, the average RS rate in summer was 0.75 μmol m−2 s−1, which was significantly higher than that in spring (0.52 μmol m−2 s−1) and autumn (0.37 μmol m−2 s−1) (p < 0.05). In 2022, the average RS rate in summer was 0.97 μmol m−2 s−1 (Figure 3).
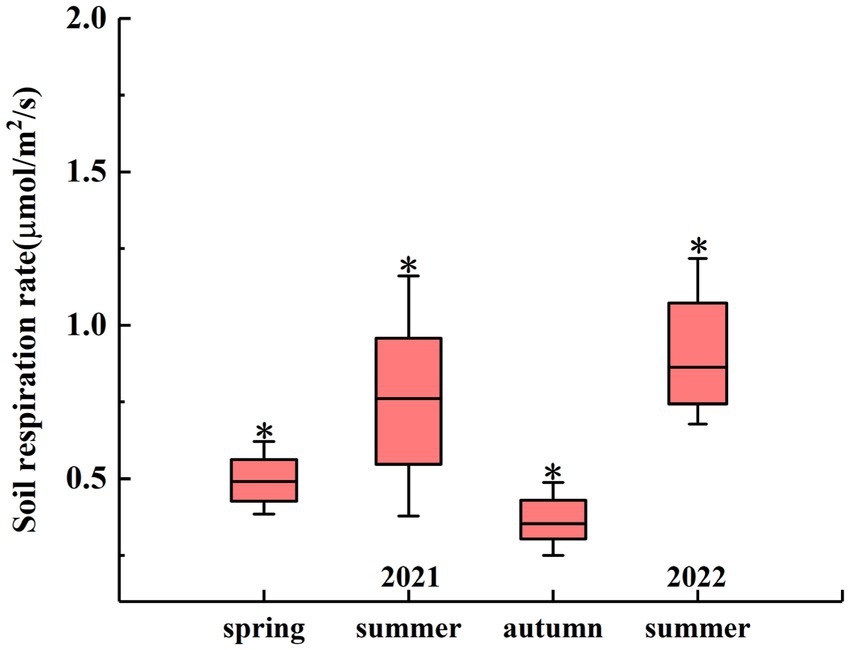
Figure 3. Seasonal RS rates from April 2021 to July 2022. The asterisk (*) indicates significant differences in RS rates between different seasons at a significance level of 0.05.
3.3. Relationship between TS, MS, and RS
The correlation analysis results showed that there were significant correlations between TS, MS, and RS (p < 0.01). The relationship between TS and the linear equation fitting the diurnal-scale variation in RS is shown in Figure 4, and the relationship between MS and the linear equation fitting the diurnal-scale variation in RS is shown in Supplementary Figure S4. The RS rate increased with TS and decreased with MS. According to Eq. (1), Q10 is 2.61, which is within the normal range (Zhou et al., 2009).
3.4. Soil inorganic nitrogen, microbial biomass, and enzyme activity
In the topsoil, the NO3−--N from August 2021 to July 2022 was significantly higher than that from April to June 2021, and the MBC from October 2021 to July 2022 was significantly higher than that from April to August 2021. NH4+--N reached a maximum (12.59 mg/kg) in August 2021, and there were no significant differences in NH4+--N between other months except in August 2021. The MBP in the topsoil in August 2021 was significantly higher than that in the other months. The MBN reached the maximum (34.95 mg/kg) in May 2022 (Figure 5A). In the subsoil, the NO3−--N from April 2021 to June 2021 was significantly higher than that in other months, and there was no significant difference in NH4+--N among the 6 months. The MBC reached a maximum (113.88 mg/kg) in October 2021. The MBN from May to July 2022 was significantly higher than that from April 2021 to August 2021. There were no significant changes in MBP from April 2021 to July 2022 (Figure 5B).
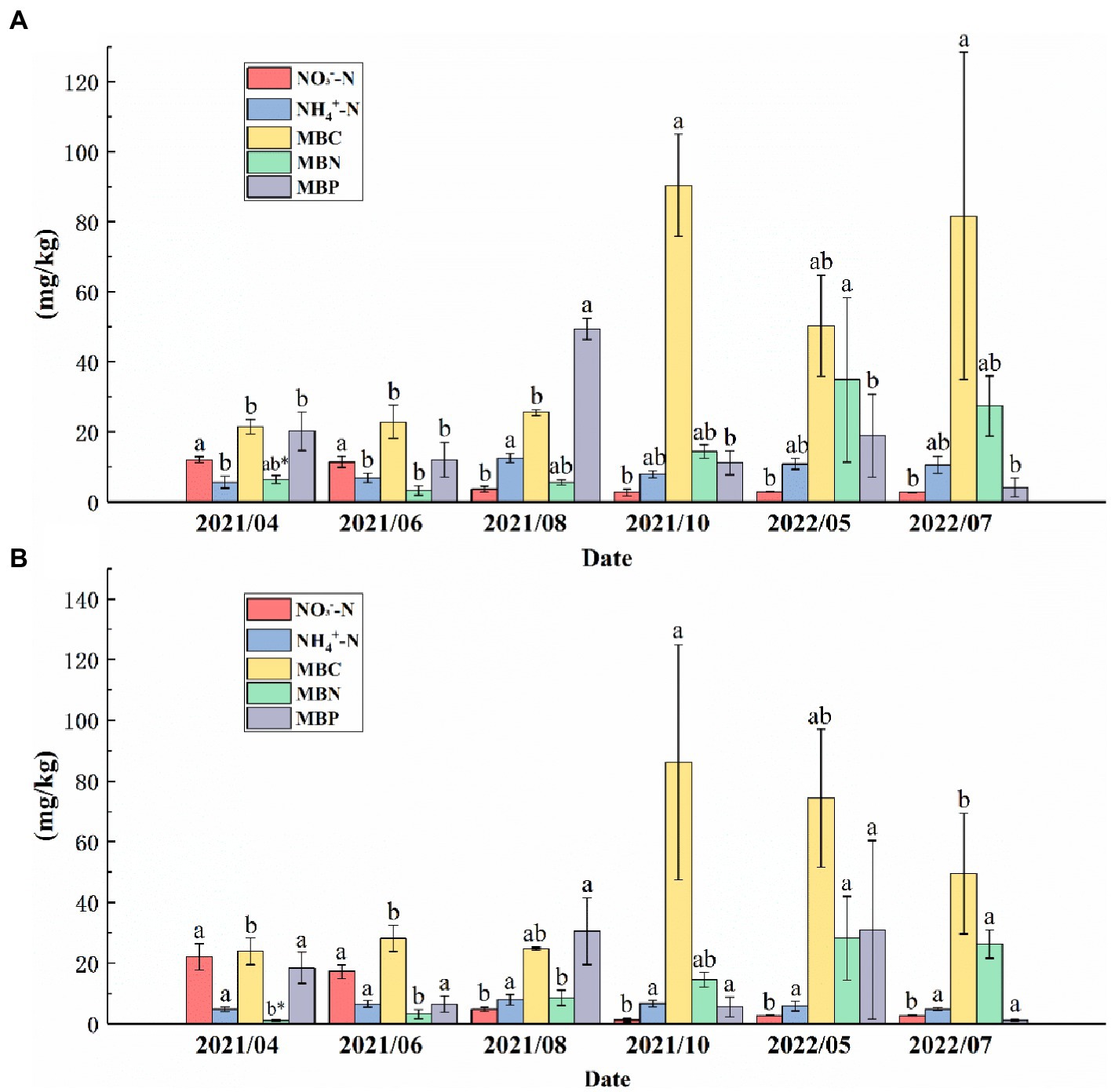
Figure 5. NH4+--N, NO3−--N, microbial biomass carbon (MBC), microbial biomass nitrogen (MBN), and microbial biomass phosphorus (MBP) contents in the (A) topsoil and (B) subsoil from April 2021 to July 2022. Different letters indicate significant differences between months for the same variable (p < 0.05).* indicates that the same variable in the same month is significantly different in different soil layers (p < 0.01).
The soil enzyme activity in the topsoil and subsoil varied significantly among different months (p < 0.05). The PER activity in the topsoil in May 2022 and July 2022 was significantly lower than that in the other months, and in the subsoil there were significant differences in the PER activity between April 2021 and July 2022. The PPO activity in both the topsoil and subsoil varied significantly among different months. Similar to that in the topsoil, in the subsoil, the βG activity in May 2022 was significantly higher than that in other months. The βG activity in the topsoil in April, June, and August 2021 was significantly different from the βG activity in the subsoil. The CBH activity in the topsoil in August 2021 was significantly lower than that in other months, and in the subsoil, the CBH activity in May 2022 was significantly higher than that from April to August 2021 but not significantly different from that in other months. In addition, from April 2021 to August 2021, the CBH and βG activities were significantly different in the topsoil and subsoil, so we believe that the CBH and βG activities in the topsoil were generally greater than those in the subsoil (Table 2).
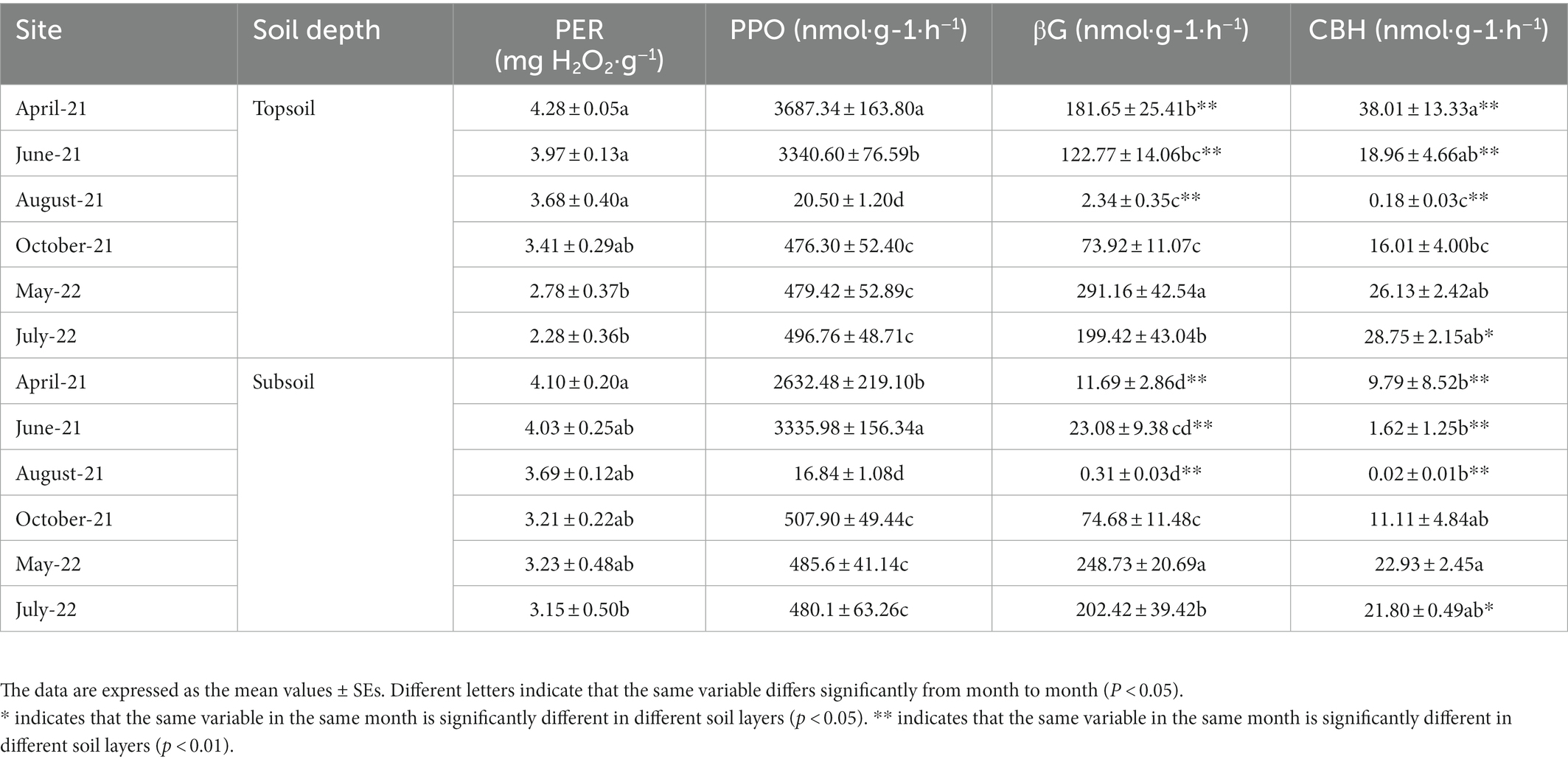
Table 2. Peroxidase (PER), polyphenol oxidase (PPO), β-1,4-glucosidase (βG), and cellobiohydrolase (CBH) activities in the topsoil (0–30 cm) and subsoil (30–100 cm) from April 2021 to July 2022.
3.5. Relationship between soil microbial biomass, enzyme activity and RS
Based on the stepwise multiple regression results (Supplementary Tables S1, S2), we determined the variables that mostly explained the variation in RS. Model optimization was performed continually until the model fits well. SEM demonstrated the influence of RS in different soil layers (Figure 6). The model for topsoil showed values of χ2 = 2.809, p = 0.422, df = 3, RMSEA = 0, and CFI = 1 (Figure 6A); the model for whole soil showed values of χ2 = 4.339, p = 0.362, df = 4, RMSEA = 0, and CFI = 1 (Figure 6B).
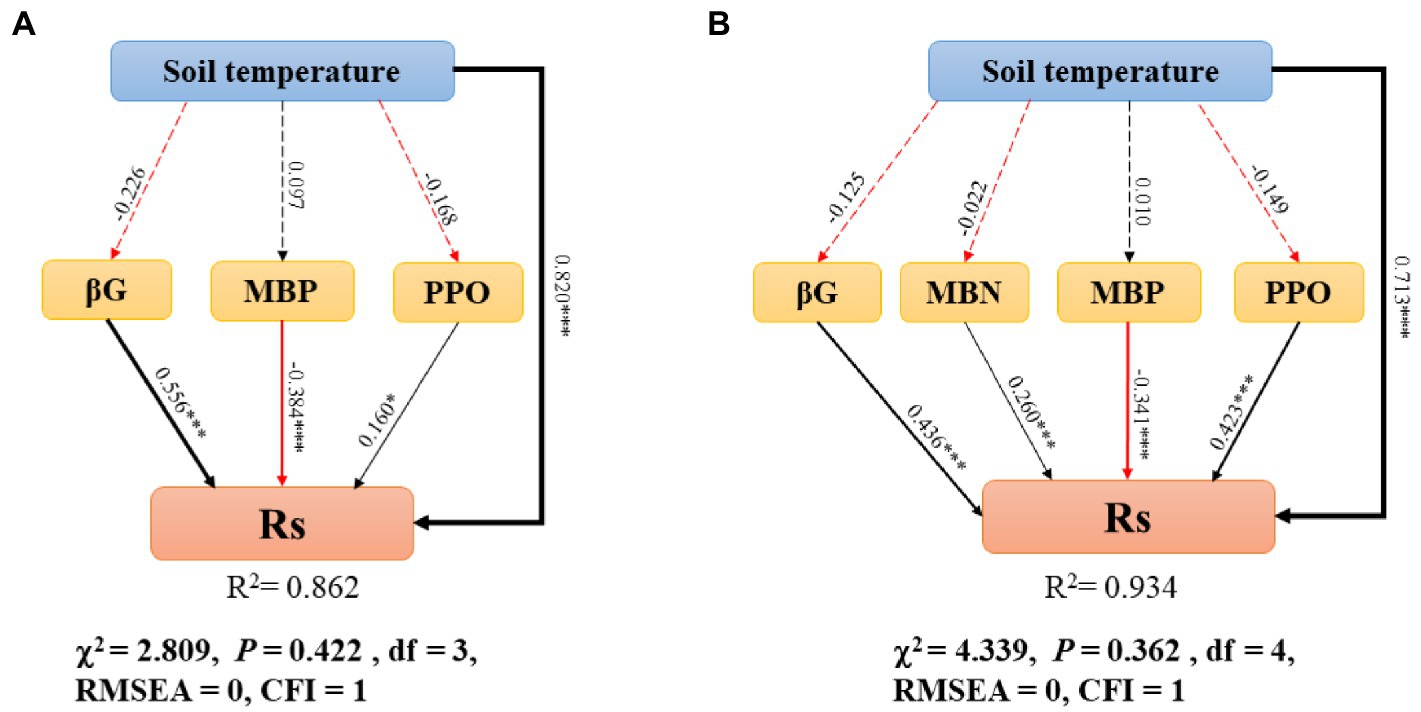
Figure 6. Structural equation model describes the relationship between variables and RS in the topsoil (A) and whole soil (B). RS, soil respiration; MBN, microbial biomass nitrogen; MBP, microbial biomass phosphorus; PPO, polyphenol oxidase; βG, β-1,4-glucosidase; soil temperature (TS). Arrows represent the assumed direction of causation. The width of arrow is proportional to the path coefficient. The red and black solid lines represent negative and positive pathways, respectively. Insignificant pathways are indicated by grey dashed lines. The importance of the variables is reflected by standardized path coefficients. R2 reflects the proportion of variance explained for all variables in the model. The significance levels are as follows: *p < 0.05, **p < 0.01, and ***p < 0.001.
In the topsoil, TS, βG, MBP, and PPO all directly affected RS, except for MBP, TS, βG and PPO, which were significantly and positively correlated with RS, and TS, βG were the variables that had the strongest effects on RS. In the whole soil, TS, βG, MBP, MBN, and PPO all directly affected RS, except for MBP, TS, βG, PPO, MBN, which were significantly and positively correlated with RS, and TS, βG, PPO were the variables that had the strongest effects on RS.
4. Discussion
4.1. Effects of TS and MS on RS
Global warming not only increases the temperature of the atmosphere but also leads to changes in precipitation, which in turn causes greater variation in TS and MS (Zhang et al., 2016). In this study, the air temperature reached its highest value in summer and then gradually decreased, and the atmospheric moisture showed a multipeak trend, which experienced a decrease followed by an increase in the summer (Figure 1A). This may be related to the specific climate of the Loess Plateau. Since the Loess Plateau is an area sensitive to climate change, changes in atmospheric temperature and precipitation caused by global warming often lead to frequent droughts in many areas (Piao et al., 2010a). The climate of the Loess Plateau showed a trend of aridity in spring and summer (Hou et al., 2021) and then experienced violent precipitation in autumn, causing the air temperature to decrease after August, while air moisture began to increase significantly after August, reaching a maximum in October, and then gradually decreased again. TS and MS also show similar trends to air temperature and moisture (Figure 1B).
It has been demonstrated in previous studies that RS is closely related to TS (Wang et al., 2006). During the observation period, the monthly diurnal variation in RS showed a multi-peak trend, and the RS rate reached its peak value at noon and decreased to a minimum value in the morning (Supplementary Figure S2). This is consistent with the results of Wang et al. (2018). A possible explanation for this phenomenon is that the reaction process of RS is mainly catalyzed by soil enzymes, and temperature is the main limiting factor affecting soil enzyme activity (Melillo et al., 2018). When the TS is low, the activities of some critical enzymes that control RS decrease, resulting in a lower RS rate. On the monthly scale, with increasing TS, the RS rate starts to increase in April, reaches a maximum value in July, and then gradually decreases to a lower value in October, which is consistent with the results of Tong et al. (2021). Our results differ from the results of Wen et al. (2018). A possible explanation for this phenomenon is the sensitivity of the research site to climate warming, resulting in a significantly higher rate of temperature change than in the other areas (Cao et al., 2016), thereby increasing the TS to a maximum at an earlier time and leading to an increase in the RS rate. The temperature sensitivity (Q10) of RS is often used as an important parameter to measure the feedback of RS to global warming (Hu et al., 2013). During the whole study period, there was a significant statistical relationship between the RS rate and TS (p < 0.01; Figure 4), and the fitting effect between the RS rate and TS also had some explanatory significance (R2 = 0.2654). Increased temperature stimulates RS because climate warming may enhance the activity of soil microorganisms and promote soil organic C and litter decomposition, which partly explains why the RS rate in summer is significantly higher than that in spring and autumn (Figure 3). Our findings suggest that a sustained increase in temperature may lead to greater soil C loss; that is, climate warming reduces soil C sinks.
MS has been identified in previous studies as a major factor affecting RS, especially after drought rewetting events stimulate RS (Hu et al., 2019). Our results show that MS is significantly negatively correlated with RS (Supplementary Figure S4), proving that MS limits CO2 emissions from soil in a shorter period, which is different from the results of Yu et al. (2021). There may be several reasons for this: first, higher MS inhibits the CO2 transportation process from the atmosphere to the soil (Yan et al., 2018). During the study period, the MS variation range was 92.44% ~42.20%, especially in summer and autumn, and the MS remained at a high level (Figure 2B). It has been suggested in previous studies that under anoxic conditions, soil organic carbon (SOC) may be more persistent (Li et al., 2021), resulting in a decrease in RS rate. Second, differences in SOC and microbial communities that decompose specific soil SOC lead to different relationships between MS and RS (Yan et al., 2018). Third, in this study, MS may not be the main factor affecting the RS rate because RS is often regulated by multiple factors (Duan et al., 2021). For example, soil salinity and RS show a negative correlation. When in a salt-stress environment, the activities of plant roots and soil microorganisms are severely affected (Song et al., 2021).
The correlations between TS, MS, and RS demonstrate that TS and MS data are useful for optimizing microbial decomposition models, which facilitates soil microbial activity prediction much better in the context of future climate change.
4.2. Physical and chemical properties in different soil layers
Most previous studies have focused on the topsoil physicochemical properties (Liu et al., 2021). In this study, there were significant differences in the TP, AK, and SOM contents of different soil layers; TP was higher in the subsoil, and AK and SOM were higher in the topsoil (Table 1). The soil physicochemical properties changed with increasing soil depth, and our results are consistent with the results of Rahman et al. (2022). Microbial secretions can significantly affect soil potassium availability (Zorb et al., 2014), and factors such as microbial abundance and activity determine the pathway of soil litter conversion into SOM (Witzgall et al., 2021). In addition, plant roots and soil microbial communities can also dissolve P in the soil (Yang et al., 2021). Litter and most of the plant roots in the study site are concentrated in the topsoil. As shown in Figure 7, the microbial enzyme activity in the topsoil is higher than that in the subsoil, which proves that the microorganisms in the surface soil may be more active. Therefore, the AK and SOM contents were significantly higher than those in the subsoil, and the TP content was significantly lower than that in the subsoil. C and N cycle and nutrient turnover in soil are carried out by microorganisms through substrate (organic matter and litter) decomposition. In recent years, microbial decomposition models have been commonly used to explore the role of soil microorganisms in the coupled C and N cycle (Wang et al., 2014; Buchkowski et al., 2015). Our results can provide a reference for describing C, N, and P stocks and stoichiometry as well as soil nutrient distribution patterns in Loess Plateau soils and provide initial response data for soil microbial decomposition models.
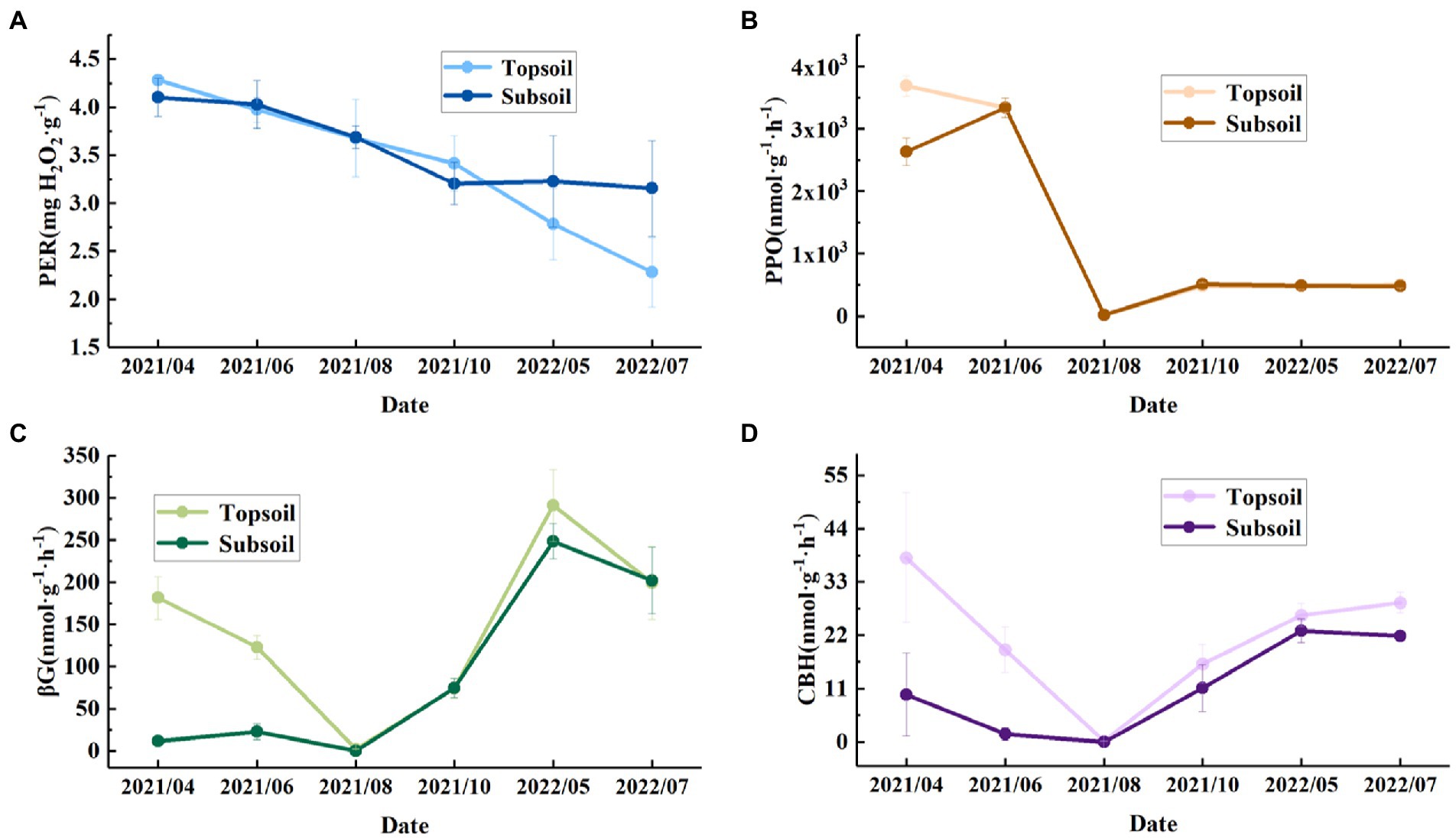
Figure 7. Monthly variations in enzyme activities in the topsoil (0–30 cm) and subsoil (30–100 cm) from April 2021 to July 2022. (A) PER, peroxidase. (B) PPO, polyphenol oxidase. (C) βG, β-1,4-glucosidase. (D) CBH, cellobiohydrolase.
4.3. Effects of soil microbial biomass and enzyme activity on RS
The soil microbial biomass can regulate microbial biochemical processes and nutrient cycling and affect soil physical and chemical properties, which in turn affects soil quality (He et al., 2003). MBC is often considered to be a crucial factor affecting RS, reflecting the ability of microorganisms to utilize SOC (Zeng et al., 2018). We found that, whether in the subsoil or topsoil, the MBC content showed a gradually increasing trend, and the MBC in May 2022 was significantly higher than in the other 3 months (Figure 5). We found that the trend of RS was out of sync with that of the MBC. RS decreased to a minimum value in autumn 2021, but the MBC reached a peak value in 2021. Our multiple stepwise regression results demonstrate that MBC is not a crucial factor affecting RS (Supplementary Tables S1, S2). The reason for this phenomenon may be due to the decrease in the use efficiency of microorganisms. Microbial carbon use efficiency (CUE) refers to the distribution ratio between the MBC produced by organic matter catabolism and the C allocated by microorganisms for aerobic respiration (Schimel et al., 2022). The results of Manzoni et al. demonstrated that high temperature reduces the CUE of microorganisms (Manzoni et al., 2012) because the increase in TS results in less C being allocated for microbial growth, which in turn reduces the ability of microbes to decompose organic resources to mitigate ecosystem C loss (Allison et al., 2010). During the growing season, TS gradually increased, and microbes probably allocated more C for RS than for MBC.
According to the results of the SEM, in addition to TS, βG and PPO also significantly influenced RS. Soil enzymes and microorganisms are involved in regulating the transformation of various organic matter and material circulation processes. Enzyme activity can be used as an indicator of microbial activity and plays a crucial role in decomposing organic compounds (Boerner R. E. J. et al., 2005). Previous studies have demonstrated that βG could participate in the decomposition of cellulose in litter (Caldwell, 2005). Litter is the most important source of organic matter input to the soil, and it can influence RS by affecting the amount of labile C in the soil (Zhang et al., 2020). Therefore, βG has a significant positive effect on RS. In addition, PPO can promote the accumulation of SOC by depolymerizing or aggregating lignin molecules and phenolic compounds in the soil (KIRK et al., 1987), thus positively influencing RS.
Our results suggest that TS, microbial biomass, and enzyme activity may be the main factors influencing soil microbial activity, which has important scientific implications for constructing microbial decomposition models that predict soil microbial activity under climate change in the future. To better understand the relationship between soil dynamics and C emissions, it will be necessary to incorporate climate data as well as RS and microbial parameters into microbial decomposition models, which will be important for soil conservation and reducing soil C loss in the Loess Plateau.
5. Conclusion
We examined the soil physical and chemical properties at different depths in the Ziwuling Mountains, Loess Plateau, China, and conducted a soil observation experiment to record the temporal and spatial dynamic changes in the soil microbial characteristics in this area. Our results prove that RS in the Ziwuling area has obvious diurnal and seasonal variations and that the RS rate is significantly correlated with TS and MS. The strong effect of temperature on RS leads to increased CO2 emissions from the soil to the atmosphere, which in turn leads to greater forest soil C loss. Our study reveals the main factors affecting RS, in order to better understand and predict changes in soil carbon dynamics in the future, incorporating TS, MBN, MBP, βG, and PPO data into microbial decomposition models is necessary.
Data availability statement
The original contributions presented in the study are included in the article/Supplementary material, further inquiries can be directed to the corresponding author.
Author contributions
KW conceived the idea and plotted the figures. RQ and GL conducted field sampling and sample determination. MY, GW, and CP conducted the statistical analysis. RQ and KW wrote the first draft of the manuscript. All authors contributed substantially to revisions, intellectual input and assistance to this study, and manuscript preparation.
Funding
This research was supported in part by the National Natural Science Foundation of China (NSFC) (grant no. 41901059), Natural Science Foundation of Shaanxi Provincial Department (grant no. 2020JQ-593), and China Scholarship Council (CSC) (grant no: 202006970003).
Acknowledgments
We are grateful to Dong Hu, JiaYi Zhong, and JinHan Li for their help in soil sampling and data analysis.
Conflict of interest
The authors declare that the research was conducted in the absence of any commercial or financial relationships that could be construed as a potential conflict of interest.
Publisher’s note
All claims expressed in this article are solely those of the authors and do not necessarily represent those of their affiliated organizations, or those of the publisher, the editors and the reviewers. Any product that may be evaluated in this article, or claim that may be made by its manufacturer, is not guaranteed or endorsed by the publisher.
Supplementary material
The Supplementary Material for this article can be found online at: https://www.frontiersin.org/articles/10.3389/fmicb.2023.1105723/full#supplementary-material
References
Abs, E., Leman, H., and Ferriere, R. (2020). A multi-scale eco-evolutionary model of cooperation reveals how microbial adaptation influences soil decomposition. Commun. Biol. 3:520. doi: 10.1038/s42003-020-01198-4
Allison, S. D., Wallenstein, M., and Bradford, M. A. J. N. G. (2010). Soil-carbon response to warming dependent on microbial physiology Nature Geoscience 3, 336–340. doi: 10.1038/ngeo846,
Bach, C. E., Warnock, D. D., Van Horn, D. J., Weintraub, M. N., Sinsabaugh, R. L., Allison, S. D., et al. (2013). Measuring phenol oxidase and peroxidase activities with pyrogallol, l-DOPA, and ABTS: effect of assay conditions and soil type. Soil Biol. Biochem. 67, 183–191. doi: 10.1016/j.soilbio.2013.08.022
Barberan, A., McGuire, K. L., Wolf, J. A., Jones, F. A., Wright, S. J., Turner, B. L., et al. (2015). Relating belowground microbial composition to the taxonomic, phylogenetic, and functional trait distributions of trees in a tropical forest. Ecol. Lett. 18, 1397–1405. doi: 10.1111/ele.12536
Boerner, R., Brinkman, J., and Smith, A. (2005). Seasonal variations in enzyme activity and organic carbon in soil of a burned and unburned hardwood forest. Soil Biol. Biochem. 37, 1419–1426. doi: 10.1016/j.soilbio.2004.12.012
Boerner, R. E. J., Brinkman, J. A., and Smith, A. (2005). Seasonal variations in enzyme activity and organic carbon in soil of a burned and unburned hardwood forest. Soil Biol. Biochem. 37, 1419–1426. doi: 10.1016/j.soilbio.2004.12.012
Bot, A., and Bernites, J. J. J. O. G. (2005). The importance of soil organic matter. Journal of Geology. 117, 241–262. doi: 10.1086/597364
Bradstreet, R. B. (1965). Distillation and determination of nitrogen - ScienceDirect Kjeldahl method for organic nitrogen. Science Direct. 147–168. Available at https://www.elsevier.com/books/the-kjeldahl-method-for-organic-nitrogen/bradstreet/978-1-4832-3298-0
Buchkowski, R. W., Schmitz, O. J. B., and Ecology, M. J. (2015). Microbial stoichiometry overrides biomass as a regulator of soil carbon and nitrogen cycling. Ecology. 96, 1139–1149. doi: 10.1890/14-1327.1,
Burns, R. G., DeForest, J. L., Marxsen, J., Sinsabaugh, R. L., Stromberger, M. E., Wallenstein, M. D., et al. (2013). Soil enzymes in a changing environment: current knowledge and future directions. Soil Biol. Biochem. 58, 216–234. doi: 10.1016/j.soilbio.2012.11.009
Cade-Menun, B. J., and Lavkulich, L. M. (1997). A comparison of methods to determine total, organic, and available phosphorus in forest soils. Commun. Soil Sci. Plant Anal. Taylor & Francis Inc. 28, 651–663. doi: 10.1080/00103629709369818
Caldwell, B. A. (2005). Enzyme activities as a component of soil biodiversity: a review. Pedobiologia 49, 637–644. doi: 10.1016/j.pedobi.2005.06.003
Cao, L., Zhu, Y., Tang, G., Yuan, F., and Yan, Z. J. I. J. O. C. (2016). Climatic warming in China according to a homogenized data set from 2419 stations, Wiley. 4384–4392.
Cardoso, E.J.B.N., Bini, D., Miyauchi, M.Y.H., Santos, C.A.D., Alves, P.R.L., Paula, A.M.D., et al. (2013). Soil health: Looking for suitable indicators: What should be considered to assess the effects of use and management on soil health? Univ Sao Paulo, Esalq.
Chai, Y., Yue, M., Liu, X., Guo, Y., Wang, M., Xu, J., et al. (2016). Patterns of taxonomic, phylogenetic diversity during a long-term succession of forest on the loess plateau, China: insights into assembly process. Sci. Rep. 6:27087. doi: 10.1038/srep27087
Chander, K., Goyal, S., Nandal, D. P., Kapoor, K. K. J. B., and Soils, F.o., (1998). Soil organic matter, microbial biomass and enzyme activities in a tropical agroforestry system. Biology and Fertility of Soils. 27, 168–172. doi: 10.1007/s003740050416,
Chen, Y. S., Hou, M. T., Dan, M. A., Han, X. H., Zhang, R. Y., and Zhang, X. Z. (2018). Determination of Total phosphorus in soil by alkali fusion-Mo-Sb anti spectrophotometric method. China Stand. 4,193–196. Avilable at: http://en.cnki.com.cn/Article_en/CJFDTotal-ZGBZ2018S1041.htm
Davidson, E. A., Janssens, I. A., and Luo, Y. (2006). On the variability of respiration in terrestrial ecosystems: moving beyond Q 10. Glob. Chang. Biol. 12, 154–164. doi: 10.1111/j.1365-2486.2005.01065.x
Duan, L., Liu, T., Ma, L., Lei, H., and Singh, V. P. (2021). Analysis of soil respiration and influencing factors in a semiarid dune-meadow cascade ecosystem. Sci. Total Environ. 796:148993. doi: 10.1016/j.scitotenv.2021.148993
Eivazi, F., and Tabatabai, M. (1988). Glucosidases and galactosidases in soils. Soil biol. Biochem. 20, 601-606. Soil Biol. Biochem. 20, 601–606. doi: 10.1016/0038-0717(88)90141-1
Friedlingstein, P., Cox, P., Betts, R., Bopp, L., Bloh, W. V., Brovkin, V., et al. (2006). Climate–Carbon cycle feedback analysis: Results from the C4MIP model intercomparison. Journal of Climate 19, 3337.
Fierer, N., Wood, S. A., and Bueno de Mesquita, C. P. (2021). How microbes can, and cannot, be used to assess soil health. Soil Biol. Biochem. 153:8111. doi: 10.1016/j.soilbio.2020.108111
Fu, B., Wang, S., Liu, Y., Liu, J., Liang, W., Miao, C. J. A. R. O. E., et al. (2016). Hydrogeomorphic ecosystem responses to natural and anthropogenic changes in the Loess Plateau of China. Annual Review of Earth & Planetary Sciences. 45, 223–243. doi: 10.1146/annurev-earth-063016-020552
Green, D. M., and Oleksyszyn, M. J. S. S. S. O. A. J. (2002). Enzyme activities and carbon dioxide flux in a Sonoran. Des. Urban Ecosyst. 66, 2002–2008. doi: 10.2136/sssaj2002.2002
Guo, X., Gao, Q., Yuan, M., Wang, G., Zhou, X., Feng, J., et al. (2020). Gene-informed decomposition model predicts lower soil carbon loss due to persistent microbial adaptation to warming. Nat. Commun. 11:4897. doi: 10.1038/s41467-020-18706-z
Hanson, P. J., Edwards, N. T., Garten, C. T., and Andrews, J. A. (2000). Separating root and soil microbial contributions to soil respiration: a review of methods and observations. Biogeochemistry 48: 115-146. Biogeochemistry 48, 115–146. doi: 10.1023/A:1006244819642
He, Z. L., Yang, X. E., Baligar, V. C., and Calvert, D. V. (2003). Microbiological and biochemical indexing systems for assessing quality of acid soils, Academic Press Inc. 89–138.
Hou, Q. Q., Pei, T. T., Chen, Y., Ji, Z. X., and Xie, B. P. J. Y. Y. S. T. X. B. T. J. O. A. E. Z. S. T. X. X. H., Zhongguo ke xue yuan Shenyang ying yong sheng tai yan jiu suo zhu ban (2021). Variations of drought and its trend in the Loess Plateau from 1986 to 2019. Chinese Journal of Applied Ecology. 32, 649–660. doi: 10.13287/j.1001-9332.202102.012
Hu, L. T., and Bentler, P. M. (1999). Cutoff criteria for fit indexes in covariance structure analysis: conventional criteria versus new alternatives. Struct. Equ. Model. 6, 1–55. doi: 10.1080/10705519909540118
Hu, Z., Cui, H., Chen, S., Shen, S., Li, H., Yang, Y., et al. (2013). Soil respiration and N2O flux response to UV-B radiation and straw incorporation in a soybean–winter wheat rotation. System 224:1394. doi: 10.1007/s11270-012-1394-z
Hu, Z., Towfiqul Islam, A. R. M., Chen, S., Hu, B., Shen, S., Wu, Y., et al. (2019). Effects of warming and reduced precipitation on soil respiration and N2O fluxes from winter wheat-soybean cropping systems. Geoderma 337, 956–964. doi: 10.1016/j.geoderma.2018.10.047
Jansson, J. K., and Hofmockel, K. S. (2020). Soil microbiomes and climate change. Nat. Rev. Microbiol. 18, 35–46. doi: 10.1038/s41579-019-0265-7
Ji, C., Luo, Y., Jan, V., Hungate, B. A., Cao, J., Zhou, X., et al. (2018). A keystone microbial enzyme for nitrogen control of soil carbon storage. Science Advances 4, eaaq1689.
Kang, D., Guo, Y., Ren, C., Zhao, F., Feng, Y., Han, X., et al. (2014). Population structure and spatial pattern of main tree species in secondary Betula platyphylla forest in Ziwuling Mountains China. Sci. Rep. 4:6873. doi: 10.1038/srep06873
Kirk, T. K., Farrell,, and Microbiol, R. J. A. R. (1987). Enzymatic combustion - the microbial-degradation of lignin, Annual Reviews: Copernicus Gesellschaft Mbh vol. 41, 465–505.
Laganière, J., Paré, D., Bergeron, Y., and Chen, H. Y. H. (2012). The effect of boreal forest composition on soil respiration is mediated through variations in soil temperature and C quality. Soil Biol. Biochem. 53, 18–27. doi: 10.1016/j.soilbio.2012.04.024
Li, Y., Shahbaz, M., Zhu, Z., Deng, Y., Tong, Y., Chen, L., et al. (2021). Oxygen availability determines key regulators in soil organic carbon mineralization in paddy soils. Soil Biol. Biochem. 153:8106. doi: 10.1016/j.soilbio.2020.108106
Liu, D., Fang, Y., Tu, Y., and Pan, Y. (2014). Chemical method for nitrogen isotopic analysis of ammonium at natural abundance. Anal. Chem. 86, 3787–3792. doi: 10.1021/ac403756u
Liu, M., Li, X., Zhu, R., Chen, N., Ding, L., and Chen, C. (2021). Vegetation richness, species identity and soil nutrients drive the shifts in soil bacterial communities during restoration process. Environ. Microbiol. Rep. 13, 411–424. doi: 10.1111/1758-2229.12913
Lucas, R. W., Casper, B. B., Jackson, J. K., and Balser, T. C. (2007). Soil microbial communities and extracellular enzyme activity in the New Jersey pinelands. Soil Biol. Biochem. 39, 2508–2519. doi: 10.1016/j.soilbio.2007.05.008
Mahajan, G. R., Das, B., Manivannan, S., Manjunath, B. L., Verma, R. R., Desai, S., et al. (2021). Soil and water conservation measures improve soil carbon sequestration and soil quality under cashews, Irtces. 36, 190–206 doi: 10.1016/j.ijsrc.2020.07.009.
Manzoni, S., Taylor, P., Richter, A., Porporato, A., and Agren, G. I. (2012). Environmental and stoichiometric controls on microbial carbon-use efficiency in soils. New Phytol. 196, 79–91. doi: 10.1111/j.1469-8137.2012.04225.x
Marland, G. (1983). Relationship between global fossil-fuel consumption and the concentration of carbon dioxide in the atmosphere. Part. 1, 1–133.
Melillo, J. M., Frey, S. D., Deangelis, K. M., Werner, W. J., Bernard, M. J., Bowles,, et al. (2018). Long-term pattern and magnitude of soil carbon feedback to the climate system in a warming world Science. 2, 101–105. doi: 10.1126/science.aan2874
Piao, S., Ciais, P., Huang, Y., Shen, Z., Peng, S., Li, J., et al. (2010a). The impacts of climate change on water resources and agriculture in China. Nature. 467, 43–51. doi: 10.1038/nature07944
Piao, S., Fang, J., Ciais, P., Peylin, P., Huang, Y., Sitch, S., et al. (2010b). The carbon balance of terrestrial ecosystems in China. Nature. 458, 1009–1013. doi: 10.1038/nature07944
Rahman, M., Zhang, K., Wang, Y., Ahmad, B., Ahmad, A., Zhang, Z., et al. (2022). Variations in soil physico-chemical properties, soil stocks, and soil stoichiometry under different soil layers, the major forest region Liupan Mountains of Northwest China. Braz. J. Biol. 84:e256565. doi: 10.1590/1519-6984.256565
Schimel, J., Weintraub, M. N., and Moorhead, D. (2022). Estimating microbial carbon use efficiency in soil: isotope-based and enzyme-based methods measure fundamentally different aspects of microbial resource use. Soil Biol. Biochem. 169:108677. doi: 10.1016/j.soilbio.2022.108677
Schlesinger, W. H., and Andrews, J. A. J. B. (2000). Soil respiration and the global carbon cycle. Biogeochemistry. 48, 7–20.
Schloter, M., Dilly, O., and Munch, J. C. J. A. E. (2003). Environment indicators for evaluating soil quality. Agriculture Ecosystems & Environment. 98, 255–262. doi: 10.1016/S0167-8809(03)00085-9,
Sicardi, M., Garcia-Prechac, F., and Frioni, L. (2004). Soil microbial indicators sensitive to land use conversion from pastures to commercial Eucalyptus grandis (hill ex maiden) plantations in Uruguay. Appl. Soil Ecol. 27, 125–133. doi: 10.1016/j.apsoil.2004.05.004
Sinsabaugh, R. L., Antibus, R. K., Linkins, A. E., Mcclaugherty, C. A., Rayburn, L., Repert, D., et al. (1992). Wood decomposition over a first-order watershed: Mass loss as a function of lignocellulase activity. Soil Biology & Biochemistry. 24, 743–749. doi: 10.1016/0038-0717(92)90248-V,
Sinsabaugh, R. L., Lauber, C. L., Weintraub, M. N., Ahmed, B., Allison, S. D., Crenshaw, C., et al. (2008). Stoichiometry of soil enzyme activity at global scale. Ecol. Lett. 11, 1252–1264. doi: 10.1111/j.1461-0248.2008.01245.x
Song, X., Zhu, Y., and Chen, W. (2021). Dynamics of the soil respiration response to soil reclamation in a coastal wetland. Sci. Rep. 11:2911. doi: 10.1038/s41598-021-82376-0
Tabachnick, B. G., and Fidell, L. S. (2007). Using multivariate statistics. 5th Edn. Lippincott Williams & Wilkins
Tollefson, J. J. N. (2021). IPCC climate report: Earth is warmer than it's been in 125,000 years. Nature Portfolio. 596.
Tong, D., Li, Z., Xiao, H., Nie, X., Liu, C., and Zhou, M. (2021). How do soil microbes exert impact on soil respiration and its temperature sensitivity? Environ. Microbiol. 23, 3048–3058. doi: 10.1111/1462-2920.15520
Vance, E. D., and Brookes, P. C. (1987). “Measurement of microbial biomass in soil” in Chemical analysis in environmental research. (Rowland: Abbots Ripton, Institute of Terrestrial Ecology).
Vile, D., Shipley, B., and Garnier, E. (2006). A structural equation model to integrate changes in functional strategies during old-field succession. Ecology 87, 504–517. doi: 10.1890/05-0822
Voigt, C., Lamprecht, R. E., Marushchak, M. E., Lind, S. E., Novakovskiy, A., Aurela, M., et al. (2016). Warming of subarctic tundra increases emissions of all three important greenhouse gases – carbon dioxide, methane, and nitrous oxide. Global Change Biology.
Wang, X. (2010). Comparison of methods for determining alkali-hydrolyzed nitrogen in soil. J. Beijing Norm. Univ. Nat. Sci. 46, 76–78. http://en.cnki.com.cn/article_en/cjfdtotal-bsdz201001021.htm
Wang, Y. P., Chen, B. C., Wieder, W.R., Leite, M., and Luo, Y. Q. J. B. (2014). Oscillatory behavior of two non-linear microbial models of soil carbon decomposition. 11. Copernicus Gesellschaft Mbh.
Wang, G., Huang, W., Zhou, G., Mayes, M. A., and Zhou, J. (2020). Modeling the processes of soil moisture in regulating microbial and carbon-nitrogen cycling. J. Hydrol. 585:124777. doi: 10.1016/j.jhydrol.2020.124777
Wang, G., Jagadamma, S., Mayes, M. A., Schadt, C. W., Steinweg, J. M., Gu, L., et al. (2015). Microbial dormancy improves development and experimental validation of ecosystem model. ISME J. 9, 226–237. doi: 10.1038/ismej.2014.120
Wang, X., Luo, X., Jia, H., and Zheng, H. (2018). Dynamic characteristics of soil respiration in Yellow River Delta wetlands China. Physics and chemistry of the earth. 103, 11–18. doi: 10.1016/j.pce.2017.06.008,
Wang, J., Song, B., Ma, F., Tian, D., Li, Y., Yan, T., et al. (2019). Nitrogen addition reduces soil respiration but increases the relative contribution of heterotrophic component in an alpine meadow running head: A rising r h /r s ratio along with n addition gradient.
Wang, C., Yang, J., and Zhang, Q. (2006). Soil respiration in six temperate forests in China. Glob. Chang. Biol. 12, 2103–2114. doi: 10.1111/j.1365-2486.2006.01234.x
Wen, L., Jinlan, W., Xiaojiao, Z., Shangli, S., and Wenxia, C. (2018). Effect of degradation and rebuilding of artificial grasslands on soil respiration and carbon and nitrogen pools on an alpine meadow of the Qinghai-Tibetan plateau. Ecol. Eng. 111, 134–142. doi: 10.1016/j.ecoleng.2017.10.013
Wieder, W. R., Cleveland, C. C., Smith, W. K., and Todd-Brown, K. J. N. G. (2015). Future productivity and carbon storage limited by terrestrial nutrient availability. Nature Geoscience. 8, 441–444. doi: 10.1038/ngeo2413,
Witzgall, K., Vidal, A., Schubert, D. I., Hoschen, C., Schweizer, S. A., Buegger, F., et al. (2021). Particulate organic matter as a functional soil component for persistent soil organic carbon. Nat. Commun. 12:4115. doi: 10.1038/s41467-021-24192-8
Wood, T. M., and Bhat, K. M. (1988). “Methods for measuring cellulase activities” in Methods in enzymology (United States: Academic Press), 87–112.
Yan, Z., Bond-Lamberty, B., Todd-Brown, K. E., Bailey, V. L., Li, S., Liu, C., et al. (2018). A moisture function of soil heterotrophic respiration that incorporates microscale processes. Nature. Communications 9:4971. doi: 10.1038/s41467-018-04971-6
Yang, F., Sui, L., Tang, C., Li, J., Cheng, K., and Xue, Q. (2021). Sustainable advances on phosphorus utilization in soil via addition of biochar and humic substances. Sci. Total Environ. 768:145106. doi: 10.1016/j.scitotenv.2021.145106
Yu, H., Liu, X., Ma, Q., Yin, Z., Wang, Y., Xu, Z., et al. (2021). Climatic warming enhances soil respiration resilience in an arid ecosystem. Sci. Total Environ. 756:144005. doi: 10.1016/j.scitotenv.2020.144005
Yu, Y., Zhao, W., Martinez-Murillo, J. F., and Pereira, P. (2020). Loess plateau: from degradation to restoration. Sci. Total Environ. 738:140206. doi: 10.1016/j.scitotenv.2020.140206
Zanati, M. R., Guirguis, M. A., and Saber, M. S. M. (1973). Biological and chemical determination of available potassium in soil. Zentralblatt für Bakteriologie, Parasitenkunde, Infektionskrankheiten und hygiene. Zweite Naturwissenschaftliche Abteilung: Allgemeine, Landwirtschaftliche und Technische Mikrobiologie 128, 572–577. doi: 10.1016/s0044-4057(73)80079-6
Zeng, W., Chen, J., Liu, H., and Wang, W. (2018). Soil respiration and its autotrophic and heterotrophic components in response to nitrogen addition among different degraded temperate grasslands. Soil Biol. Biochem. 124, 255–265. doi: 10.1016/j.soilbio.2018.06.019
Zhang, H., Wang, E., Zhou, D., Luo, Z., and Zhang, Z. (2016). Rising soil temperature in China and its potential ecological impact. Sci. Rep. 6:35530. doi: 10.1038/srep35530
Zhang, Y., Zhao, J., Xu, J., Chai, Y., Liu, P., Quan, J., et al. (2022). Effects of water availability on the relationships between hydraulic and economic traits in the Quercus wutaishanica forests. Front. Plant Sci. 13:902509. doi: 10.3389/fpls.2022.902509
Zhang, Y., Zou, J., Meng, D., Dang, S., Zhou, J., Osborne, B., et al. (2020). Effect of soil microorganisms and labile C availability on soil respiration in response to litter inputs in forest ecosystems: a meta-analysis. Ecol. Evol. 10, 13602–13612. doi: 10.1002/ece3.6965
Zhou, T., Shi, P., Hui, D., and Luo, Y. (2009). Global pattern of temperature sensitivity of soil heterotrophic respiration (Q10) and its implications for carbon-climate feedback. Journal of geophysical research. Biogeosciences 114:850. doi: 10.1029/2008jg000850
Zhu, C., Zhang, Z., Wang, H., Wang, J., and Yang, S. (2020). Assessing soil organic matter content in a coal mining area through spectral variables of different numbers of dimensions. Sensors 20:1795. doi: 10.3390/s20061795
Keywords: climate change, carbon cycle, soil microbial activity, microbial decomposition model, soil respiration (CO2)
Citation: Qu R, Liu G, Yue M, Wang G, Peng C, Wang K and Gao X (2023) Soil temperature, microbial biomass and enzyme activity are the critical factors affecting soil respiration in different soil layers in Ziwuling Mountains, China. Front. Microbiol. 14:1105723. doi: 10.3389/fmicb.2023.1105723
Edited by:
Zengming Chen, Institute of Soil Science (CAS), ChinaReviewed by:
Yangquanwei Zhong, Northwestern Polytechnical University, ChinaKerou Zhang, Chinese Academy of Forestry, China
Zihan Jiang, Chinese Academy of Sciences, China
Copyright © 2023 Qu, Liu, Yue, Wang, Peng, Wang and Gao. This is an open-access article distributed under the terms of the Creative Commons Attribution License (CC BY). The use, distribution or reproduction in other forums is permitted, provided the original author(s) and the copyright owner(s) are credited and that the original publication in this journal is cited, in accordance with accepted academic practice. No use, distribution or reproduction is permitted which does not comply with these terms.
*Correspondence: Kefeng Wang, ✉ d2FuZ2tmQG53dS5lZHUuY24=