- 1Pacific Northwest National Laboratory, Earth and Biological Sciences Directorate, Richland, WA, United States
- 2Department of Animal, Veterinary, and Food Sciences, University of Idaho, Moscow, ID, United States
- 3Margaret Ritchie School of Family and Consumer Sciences, University of Idaho, Moscow, ID, United States
There is growing interest in a functional understanding of milk-associated microbiota as there is ample evidence that host-associated microbial communities play an active role in host health and phenotype. Mastitis, characterized by painful inflammation of the mammary gland, is prevalent among lactating humans and agricultural animals and is associated with significant clinical and economic consequences. The etiology of mastitis is complex and polymicrobial and correlative studies have indicated alterations in milk microbial community composition. Recent evidence is beginning to suggest that a causal relationship may exist between the milk microbiota and host phenotype in mastitis. Multi-omic approaches can be leveraged to gain a mechanistic, molecular level understanding of how the milk microbiome might modulate host physiology, thereby informing strategies to prevent and ameliorate mastitis. In this paper, we review existing studies that have utilized omics approaches to investigate the role of the milk microbiome in mastitis. We also summarize the strengths and challenges associated with the different omics techniques including metagenomics, metatranscriptomics, metaproteomics, metabolomics and lipidomics and provide perspective on the integration of multiple omics technologies for a better functional understanding of the milk microbiome.
Introduction
The human body is host to trillions of bacterial cells that operate in connected and complex networks to potentially affect host health (Sharma and Gilbert, 2018). As the field of microbiome research advances through the refinement of analytical tools and methodologies, unexpected sites and secretions with diverse microbial communities have been identified including human milk. Except for specific cases of maternal systemic viral infection (e.g., HIV, CMV; Jones, 2001) or local inflammation (e.g., mastitis), milk was historically considered to be a sterile fluid unless contaminated via external sources (Fernandez et al., 2013). However, over the past two decades, numerous studies have confirmed the presence of bacteria in milk via culture-dependent (Martín et al., 2003; Perez et al., 2007; Delgado et al., 2009) and culture-independent methods (Collado et al., 2009; Hunt et al., 2011; Jiménez et al., 2015; Williams et al., 2017a,b; Moossavi et al., 2019b). This has propelled interest in this ecological niche for its potential to impact both maternal and offspring health (Fernandez et al., 2013; Oikonomou et al., 2020). Beyond that, alterations in the microbiota of milk produced by dairy animals are thought to influence milk production, processing, and spoilage, as well as overall consumer health - intriguing nutritionists, food scientists, and agriculturists alike (Quigley et al., 2013; Parente et al., 2020). The milk microbiome, however, is relatively understudied compared to other human microbiomes such as those of feces [as a proxy for the lower gastrointestinal (GI) tract (Shreiner et al., 2015)], skin (Grice and Segre, 2011), and vagina (France et al., 2022), which have been included in numerous investigations and large collaborative efforts like the Human Microbiome Project (Integrative, 2019). The growing understanding of the impact of host-associated microbial communities on host phenotype and health has increased interest in investigating the milk microbiome. This area of research represents an exciting frontier in maternal and infant health.
The microbial communities in milk are likely influenced by a complex interplay of exchanges among the mother, infant, and environment. Through metagenomics, metatranscriptomics, metaproteomics, and metabolomics studies, it is now possible to elucidate the microbes that constitute the microbial community, their functional potential, metabolic activity, and the products they participate in and produce (respectively) in close interaction with the host. Although the use of high-throughput analytical technologies to characterize the bacterial members of the milk microbiome has primarily focused on taxonomic profiling via 16S rRNA gene analysis and whole genome shotgun metagenomic sequencing (Zimmermann and Curtis, 2020). Functional profiling represents an intriguing future direction as demonstrated in more thoroughly studied microbial communities, such as those in the GI tract (Heintz-Buschart and Wilmes, 2018). Shifting the focus of milk microbiome investigations to gain functional understanding could lead to deeper insights into the causal relationships between composition of microbial communities and variation in maternal and infant health.
Omics technologies have been described as “high-throughput biochemical assays that measure comprehensively and simultaneously molecules of the same type from a biological sample” (Conesa and Beck, 2019). In this review, we aim to draw attention to the limited number of existing studies that have used omics approaches to investigate the milk microbiome, especially in the context of mastitis, which is inflammation of the mammary gland that can pose serious clinical and economic consequences for both dairy cattle and women. Clinically, mastitis is characterized by inflammation, often accompanied by breast pain and irritation, complicating the ability to breastfeed. Nearly 10 % of U.S. breastfeeding mothers experience clinical mastitis (Spencer, 2008), and it is of interest to uncover the nutritional and microbial impacts, as it is standard practice to encourage mothers to continue breastfeeding during a mastitis episode (Spencer, 2008). Sub-clinical mastitis in dairy cattle, is typically identified by high somatic cell count (SCC), a marker of mammary inflammation measurable in milk. As there may be no visible signs such as abnormal milk or soreness of the udder, sub-clinical mastitis can escape detection and result in decreased milk production, and poor animal health (Schukken et al., 2003). In humans, sodium/potassium the (Na+/K+) ratio in milk has been used as an indicator of sub-clinical mastitis (Willumsen et al., 2003; Pace et al., 2022). Clinical mastitis in dairy cattle is typically noted by observation of abnormalities in the milk or udder and a positive reaction in the California Mastitis Test. A variety of opportunistic pathogens that are members of the normal host microbiota (e.g., staphylococci and streptococci) have been associated with mastitis, which is characterized by a complex etiology that is likely polymicrobial and variable (Boix-Amorós et al., 2020). There is increasing interest in obtaining a mechanistic understanding of dysbiosis in the milk microbiome in the development and progression of mastitis (Hoque et al., 2020). A dysfunctional GI microbiota has been shown to lead to mastitis following fecal transplantations in animal models (Ma et al., 2018; Hoque et al., 2022), and probiotics that restore intestinal microbiota are effective in preventing and treating mastitis. These findings suggest that a holistic interrogation of host-associated microbial communities and their molecular crosstalk with the host is critical to uncover the microbial etiology of mastitis and develop effective prevention and treatment strategies. Here, we use the term “milk-omics” to describe the holistic and comprehensive characterization of both microbes and the complex composition (e.g., proteins, lipids, carbohydrates, metabolites, and myriad other substances) found in milk.
We review existing literature and discuss the strengths and challenges of each omics technique including metagenomics, metatranscriptomics, metaproteomics, metabolomics (which includes lipidomics), for understanding the role of the milk microbiome in mastitis (Figure 1). Additionally, we consider ways in which the field of milk microbiome research might benefit from multi-omics approaches that have been successfully used to gain systems-level understanding of other microbial communities.
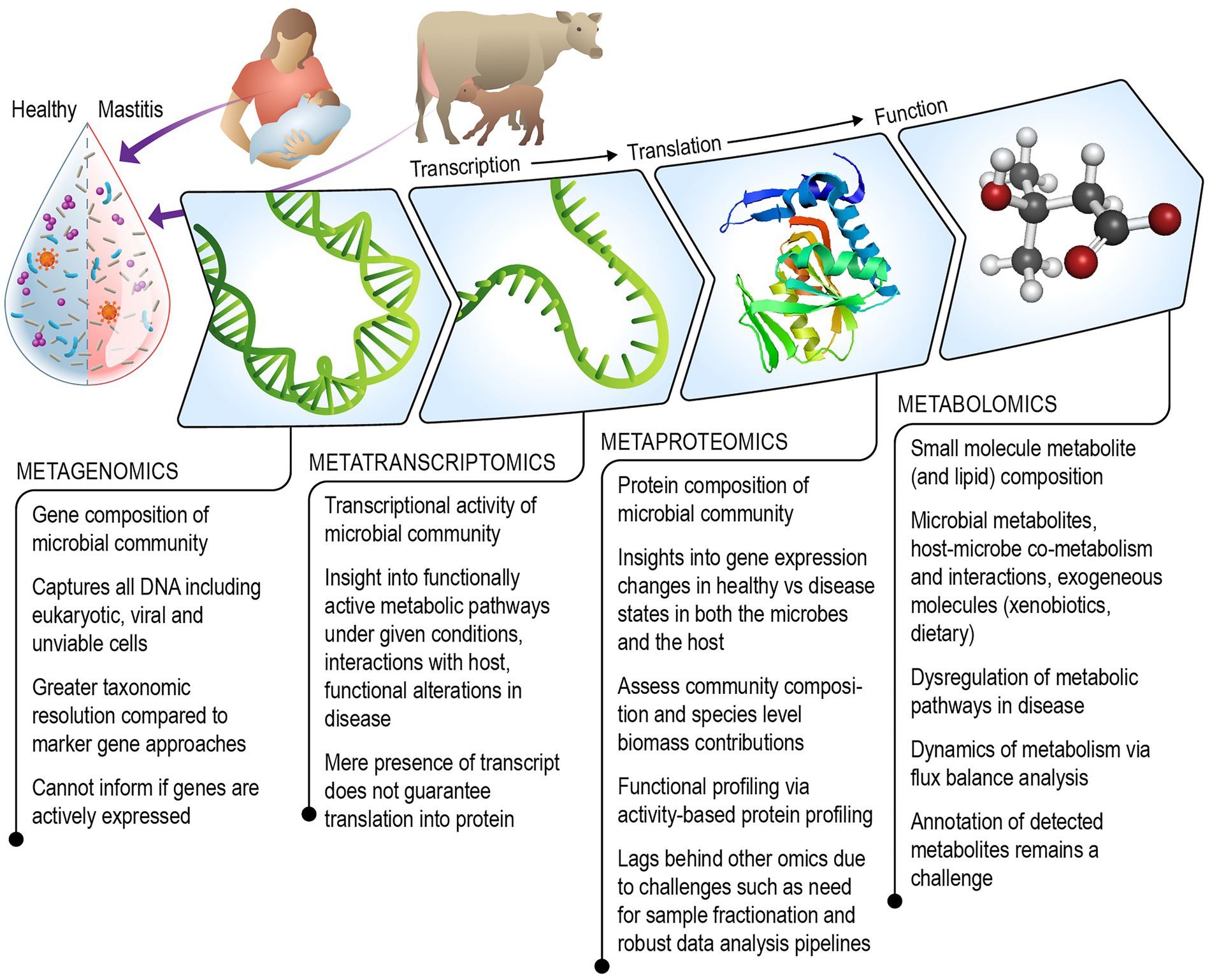
Figure 1. Schematic illustrating the combined multi-omics technologies that can be leveraged to gain a mechanistic understanding of the role of the milk microbiome in the onset and progression of mastitis in humans and agricultural animals.
Origin and importance of milk microbiota
The microbial communities of milk are hypothesized to arise through multiple routes, including exchange from infant to mother (Fernandez et al., 2013). It has been proposed that bacteria present in the infant’s oral cavity help to shape milk microbial communities via retrograde flow of bacteria from the infant’s mouth to the breast and mammary ducts. This hypothesis is supported, in part, by the finding that Streptococcus species are dominant in both the infant oral cavity and human milk (Cephas et al., 2011; Hunt et al., 2011; Fernandez et al., 2013). In addition, on a compositional basis, the complex milk microbiome is notably similar to that of the infant’s oral cavity. Of course, reverse causality might play a role here with milk’s microbiome colonizing the infant’s oral microbiome, and evidence exists to support this (Ruiz et al., 2019; Williams et al., 2019). Aside from retrograde flow of bacteria from the infant’s mouth to the mammary ducts, research also supports the concept that breast skin microbiota may contribute to the milk microbiome, in part because human milk and sebaceous breast skin share a notable number of phylotypes, including Staphylococcus species (Latuga et al., 2014). The intriguing presence of anaerobic bacteria (e.g., Bifidobacteria) in milk suggests that breast skin and the infant oral cavity may not be the only sources of milk microbiota and suggests the possibility that maternal GI microbiota may also be a source of commensal microbes eventually found in milk. This source of microbes is often referred to as being derived from the entero-mammary route of transfer (Gueimonde et al., 2007; Rodríguez, 2014; Addis et al., 2016). Evidence for the entero-mammary route has been shown in humans, mice, and ruminants (Perez et al., 2007; Treven et al., 2015; Young et al., 2015; de Andrés et al., 2017). Recent findings from animal models imply that a dysfunctional intestinal microbiota can cause mastitis, highlighting the importance of entero-mammary pathways and potential links between mammary and gut health (Ma et al., 2018; Hoque et al., 2022). The myriad ways in which the milk microbiome impacts maternal and infant health are in the early stages of being characterized and understood. Promoting GI health, immune system development, and neural maturation, milk microbiota are thought to aid in the early colonization of the infant GI tract (Jost et al., 2014; Latuga et al., 2014). Our ability to characterize microbial communities has vastly improved with advancements in high-throughput sequencing and omics-based functional profiling technologies (Di Bella et al., 2013). The unique contribution of each omics technique to understanding the milk microbiome, along with the challenges they present, are discussed next.
Sequencing-based omics approaches
Metagenomics is a powerful tool that has played a key role in the current understanding of the diversity of the human GI microbiome and microbial communities from other anatomical sites (Wang et al., 2015). In addition, metagenomics approaches have been used to successfully discover novel genes and microbial pathways and to identify functional dysbiosis (Hehemann et al., 2010; Qin et al., 2012; Vital et al., 2014), providing a useful template for its application in milk microbiome research. Metagenomes are also helpful for downstream metatranscriptomics and metaproteomics data analysis which depend on metagenomic reference databases. The most prevalent culture-independent approach for characterizing milk microbiota has been 16S rRNA gene amplicon sequencing (Hunt et al., 2011; Pannaraj et al., 2017; Lackey et al., 2019; Moossavi et al., 2019a,b). While this approach can provide information on taxonomic composition, metagenomics can go beyond this application and provide insight into both species and subspecies diversity and functional potential by examining the gene sequences that encode for proteins or functional RNAs (e.g., mRNAs and noncoding RNA). A handful of studies have utilized metagenomic sequencing to characterize the composition of the milk microbiome in bovine (Bhatt et al., 2012; Patel et al., 2017; Kusumawati et al., 2021) and human (Jiménez et al., 2015; Kordy et al., 2020; Seferovic et al., 2020; Olshan et al., 2021) milk but very few have interrogated the functional potential of the microbial communities. Metagenomic deep sequencing was used to compare the microbiomes of milk obtained from cows with clinical mastitis and healthy control cows where functional annotation of the metagenomic sequences was performed to identify differences in metabolic pathways (Hoque et al., 2019). Genes associated with bacterial colonization, proliferation, chemotaxis and invasion, oxidative stress, biofilm formation, and antimicrobial resistance, among others, were overrepresented in bacteria found in milk produced by cows with clinical mastitis (Hoque et al., 2019). Another study carried out metagenomic sequencing of milk produced by cows with subclinical mastitis and found genes associated with multidrug antibiotic resistance; however, no milk produced by healthy cows was included to assess impact of disease on functional gene presence (Bhatt et al., 2012). Milk is a challenging matrix for microbiome characterization using omics due to its relatively low microbial biomass and high ratio of host-to-microbial DNA, which complicates analyzes focusing on the microbiome (Seferovic et al., 2020; Yap et al., 2020; Moossavi et al., 2021). Metagenomics analysis requires a sufficient quantity of high-quality microbial DNA for high coverage (Wang et al., 2015). Contaminating DNA is also an issue for downstream analysis as it is inversely related to the microbial biomass of samples, underscoring the importance of including extraction and sequencing controls (e.g., extraction/PCR negative controls and mock community positive controls) when investigating milk microbial communities (Dahlberg et al., 2019; Moossavi et al., 2021; Pace et al., 2021). In-depth reviews of shotgun metagenomics that cover aspects such as best practices for study design, sample collection and analysis, library preparation, sequencing and metagenome assembly are available (Quince et al., 2017; Knight et al., 2018).
Metagenomic approaches describe the presence of genes and organisms but are unable to provide information on the transcriptional activity of individual microbes or the microbial community at large. Metatranscriptomics can be used to profile the genes expressed by the entire microbial community. Transcriptomic studies often target protein coding RNA [i.e., messenger RNA (mRNA)], but can also profile noncoding RNAs. Multiple studies have profiled the transcriptome of mammary epithelial cells and have been particularly interested in gene expression changes over the course of lactation stages (Bionaz et al., 2012; Lemay et al., 2013; Bhat et al., 2019; Ji et al., 2019; Lin et al., 2019; Martin Carli et al., 2020). Less is known about the milk metatranscriptome, as host RNA has primarily been mined in milk for information about host cell function rather than microbe function (Zorc et al., 2018). This may be due to several obstacles, such as the challenge of differentiating between host and microbial RNA given the low microbial biomass in milk. mRNA is highly unstable and the amount present in a sample can be obscured by the more abundant ribosomal RNA. Although mRNA enrichment is possible, it may cause potential biases (Li et al., 2019). Total RNA metatranscriptomics offers the possibility to gain taxonomic insights (Li et al., 2019; Xue et al., 2020) in addition to functional information. However, it should be noted that the mere presence of a transcript does not guarantee translation into protein and proteomic (or metaproteomic) analysis is required if an accurate characterization of protein expression is desired. Matched metagenomic data can be helpful for metatranscriptome analyzes and tools have been developed to facilitate metatranscriptome assembly (Ye and Tang, 2015). De novo assembly of high-quality reads into transcripts using bioinformatic tools designed for microbial communities is an alternative strategy (Leung et al., 2013; Celaj et al., 2014; Aguiar-Pulido et al., 2016; Shakya et al., 2019). Metatranscriptomics analyzes have been useful in the interrogation of other microbiomes, providing an idea of how this tool should be applied in milk research. Comprehensive reviews on the use of and best practices for metatranscriptomics are available (Bashiardes et al., 2016; Zhang et al., 2021). Integrated host and microbial transcriptome profiling of has been conducted to simultaneously characterize host and microbial functional responses and host–microbe interactions in pediatric asthma (Perez-Losada et al., 2015). This was done by separating transcriptomic (host) and metatranscriptomic (microbiota) sequences in silico and mapping to human or microbial databases. A similar approach could be used to uncover host–microbe interactions in the context of the milk microbiome in mastitis.
Mass spectrometry-based omics approaches
Much of the existing literature that aims to characterize the milk microbiome documents taxonomic population shifts under varying host or environmental conditions, but few studies have examined functional consequences of these shifts. Functional redundancy is known to exist in microbial communities across anatomical sites, and it is critical to go beyond taxonomic cataloging and examine the functional roles and phenotypes of microbial communities in milk and their phenotypic effects on the host (Moya and Ferrer, 2016). To better understand how the functional outputs of the milk microbiome impacts maternal and infant health, research must examine the biomolecules that have a closer relationship to phenotype. Mass spectrometry-based technologies, namely metaproteomics, metabolomics, and lipidomics, have enabled functional characterization of microbial communities in a variety of ecosystems.
Proteomics has been used as a tool to examine protein expression and proteolytic activity of microorganisms, providing insights on the function of fermenting microbiota in dairy products like cheese and yogurt (Gagnaire et al., 2004; Manso et al., 2005; Gagnaire et al., 2009). Several proteomic and peptidomic studies have been conducted to examine changes in milk peptides and proteins due to mastitis (Danielsen et al., 2010; Larsen et al., 2010; Chiaradia et al., 2013; Mansor et al., 2013; Zhang et al., 2015; Thomas et al., 2016). These proteomic studies have been useful for the identification of mastitis and general mastitis susceptibility biomarkers but are host focused unlike metaproteomic studies that assess functionality of the microbiome. One exception was Piras and colleagues who used metaproteomics analyzes of raw bovine milk to identify proteins related to antimicrobial resistance (Piras et al., 2020). Metaproteomics can bridge genetic information obtained from metagenomics and metatranscriptomics to the metabolic and phenotypic information contained in the metabolome (Van Den Bossche et al., 2021). Metaproteomic profiling has been used to understand the functional changes in the human gut microbiome during the pathogenesis of colorectal cancer which is known to be associated with taxonomic alterations in intestinal bacteria (Long et al., 2020). Recently, a free-flow isoelectric focusing (FFIEF) electrophoresis method was used to enrich low-abundance bacterial cells from human saliva where host cells are highly abundant (Jiang et al., 2022). This strategy reduced interference by host proteins and enabled deeper coverage of the oral metaproteome, indicating that it may be valuable in milk metaproteomic studies which face similar challenges of low microbial to host cell ratios and highly abundant host proteins such as casein.
Despite the widespread use of proteomics for single organisms, progress in metaproteomics has been slow. A few different factors have been barriers to the wider use of metaproteomics in studying microbial communities. The analysis of complex multispecies samples such as microbial communities often requires sample pre-fractionation to increase detection of peptides, and by association coverage of the metaproteome (Issa Isaac et al., 2019). This can result in significantly increasing overall sample numbers and therefore study costs. Data analysis is still a major challenge in metaproteomics. Peptide sequences, inferred from MS/MS fragmentation patterns, are computationally assigned to proteins. Mapping peptides to proteins and subsequently taxonomic association within a community containing numerous species that all have proteins with similar peptide sequences (i.e., protein sequence homology) does not discriminate the source of the protein (Kleiner et al., 2017). This is done using databases of predicted protein sequences whose quality depends on the availability and annotation of metagenomes (Hettich et al., 2013). While this is much easier for single organisms with sequenced genomes, microbial community data relies on the availability of sample specific metagenomes or high-quality public databases with comprehensive functional and taxonomic annotation. There is a growing emphasis on library-free methods of identifying and quantifying proteins in proteomics research through recent developments like Kaiko, a deep learning model that is trained to build protein databases directly from proteomics data without the aid of metagenomic sequencing (Lee et al., 2022). Library-free protein analysis could be a particularly advantageous method in milk research given the high degree of taxonomic variation in this biological niche and limited availability of existing protein reference databases. Further advancements in proteomics includes functional profiling of the proteome via activity-based protein profiling (ABPP). This method uses function-dependent covalent labeling with activity-based probes to pick out which proteins in a sample are active and has been successfully employed in fecal microbiome analysis (Whidbey et al., 2019; Couvillion et al., 2020). This technology could be useful for the analysis of the milk microbiome where low abundance, yet functionally significant microbial or host proteins are overshadowed by an intensely abundant casein and whey background (O’Donnell et al., 2004). Although some enrichment techniques have been previously developed, like 2D chromatography and fractionation, these methods can be costly and time intensive, and ABPP is a promising alternative (Couvillion et al., 2020). Salvato et al. have presented a detailed introduction of the use of metaproteomics to interrogate host-associated microbiomes (Salvato et al., 2021).
Interactions among the environment, host, and microbes are frequently relayed through small molecule metabolites, making metabolomics a key tool to elucidate host-microbial co-metabolism (Nicholson et al., 2005; D’Souza et al., 2018). Additionally, metabolomics can provide information on pathway regulation, signaling processes, and phenotype (Zhang et al., 2012). Metabolites can be introduced to milk through mammary epithelial cell secretions, somatic cell activity, serum, or microbial metabolism (Sundekilde et al., 2013b). The prevalence of certain metabolites in raw milk can influence storage stability, coagulation properties, heat stability, fermentation properties, and flavor (Cadwallader and Singh, 2009; Sundekilde et al., 2013a; Zhu et al., 2021b). Metabolomic approaches have been prevalent in studies that aim to understand the nutritional value of milk, comparing nutritional components between species, humans of varying geographical location, or milk substitutes such as infant formula (Qian et al., 2016; Scano et al., 2016; Gay et al., 2018; Perrone et al., 2019). Much less prevalent are investigations on the metabolome in mastitic milk. Xi et al. (2017) used untargeted liquid chromatography-quadrupole-time of flight mass spectrometry in the MSE mode to identify metabolites that were different in milk from cows that were healthy or had sub-clinical or clinical mastitis. Downregulation of carbohydrate, energy and lipid metabolism and upregulation of amino acid metabolism was observed in clinical mastitis. Untargeted nuclear magnetic resonance spectroscopy (1H-NMR) has also been used to characterize the milk metabolome in dairy cows with mastitis. Results suggest that mastitis was associated with alterations in the tricarboxylic acid cycle (TCA cycle) and phenylalanine, tyrosine and tryptophan biosynthesis (Zhu et al., 2021a). Some recent studies have looked at the milk metabolome and microbiome composition in the context of infant health and maternal diet. Shotgun metagenomics and metabolomics were used to identify significant differences in bacterial and viral species/strains and pathways in the breast milk of subjects with celiac disease on a gluten free diet (Olshan et al., 2021). In another study, metabolome and microbiome profiles of milk produced by women across several countries were compared using NMR and 16S rRNA gene sequencing to reveal specific metabolite profile associations with geographical locations (Gomez-Gallego et al., 2018). Correlations between milk metabolite prevalence and relative abundance bacteria were identified, suggesting potential functional relationships between the milk microbiome and metabolites (Gomez-Gallego et al., 2018).
Lipids are an important class of metabolites that could provide useful insights in mastitis research, given that many inflammatory responses are mediated by bioactive lipids (Dhankhar et al., 2016). Beyond resolving mastitis, milk lipids have been shown to have nutritional and immunomodulatory affects in the consumer, making milk microbiome lipidomics relevant to both dairy consumers and breastfeeding infants (Dhankhar et al., 2016; George et al., 2020, 2021; Hewelt-Belka et al., 2020). Despite how informative the milk lipidome can be, few studies have carried out comprehensive characterization of the milk lipidome in the context of mastitis. A recent study by Ceciliani and colleagues demonstrated significant changes in lipid classes such as triacylglycerols and sphingomyelins in milk from dairy cows with subclinical intramammary infection by non-aureus staphylococci (Ceciliani et al., 2021).
Given the challenges associated with small molecule annotation and identification (Chaleckis et al., 2019), user-friendly milk-specific metabolomic databases such as the Milk Composition Database (MCDB) are a valuable resource for researchers analyzing milk metabolomics data (Foroutan et al., 2019). The milk metabolome represents small molecule metabolites that might originate from the host, microbiome, their co-metabolism, and environmental exposures. This presents a unique analytical challenge of distinguishing between metabolites generated by host and microbial metabolism, which is further complicated by a high background of host-generated metabolites. Metabolic networks, which use databases that map metabolites back to enzyme of production, gene sequence, and then organism genomes, can help to untangle metabolite origin (Biggs et al., 2015; Kanehisa et al., 2017). However, these methods are generally only limited to genes and metabolites whose functions, identities, and relationships have already been extensively modeled. Annotation of metabolite origins via networks (AMON) is a recently developed analytical tool that uses genomic information to predict if a metabolite originated from a single organism (the host) or a group of organisms (bacteria), allowing for the analysis of complex untargeted metabolomics (Shaffer et al., 2019). These types of bioinformatic tools may be particularly useful when examining the complex milk metabolome. A recent review by Bauermeister et al. (2022) provides an informative overview on mass-spectrometry based metabolomics data and data analysis approaches.
Table 1 lists the references utilizing the omics techniques discussed above for studying the milk microbiome.
Integration of multi-omics data
In a multi-omics approach, a combination of the strategies mentioned in this review to characterize holistic “milk-omics” profiles can be integrated to investigate the complex dynamics of microbial communities and their impact on the host. By studying the interplay of genes, transcripts, proteins, and metabolites, researchers can obtain a more thorough systems-level understanding of microbial community functions, phenotypes, and their influence on host phenotype. Data generated by each omics approach can be informative about different aspects of the microbial community (Heintz-Buschart and Westerhuis, 2022) and integration of complementary omics data can provide a more holistic understanding (Nyholm et al., 2020). Although there is growing interest in integrating omics data, this is not a trivial task given that each ‘meta’-omics approach involves significant resources (financial and computational) and specialized expertise. Furthermore, studies using multiple platforms of analysis tend to generate disparate forms of high-dimensional data with varying amounts of missing values. Efficient data integration methods are necessary for meaningful interpretation of results, and ongoing development of analytical pipelines and software is making this endeavor more feasible (Noecker et al., 2016; Rohart et al., 2017; Jiang et al., 2019; Tarazona et al., 2020).
Multiple taxonomic and omics approaches have been used in tandem to assess previously mentioned factors of interest like lactation stage differences, dairy product quality, and bioactive molecules but have not focused on microbial functions and their impact on host phenotype (Lu et al., 2013, 2015; Morris et al., 2016; Afshari et al., 2021). Correlations between metagenomic compositional profiles and raw milk metabolites revealed potential associations between bacterial genera and metabolite markers of milk as related to feed practices (Bellassi et al., 2021). By focusing on the association of metabolite profiles and shifts in taxonomy representation due to host or environment perturbations rather than metabolic pathway analysis, these studies show correlation but do not confirm causation. A combination of untargeted metabolomics and 16S rRNA gene sequencing, enabled the analysis of correlative taxonomy and metabolite profile changes during sub-clinical Streptococcus agalactiae mastitis (GBS) (Tong et al., 2019). Specific bacteria were highly correlated with several metabolites, suggesting possible functional relationships and potential diagnostic biomarkers and pathway analysis suggested that GBS disrupts the TCA cycle in mammary gland cells (Tong et al., 2019).
Integrated multi-omics studies have been more common in the field of GI microbiome research. Longitudinal proteomics, metabolomics, and metagenomics data have been collected to examine microbiome composition and function (Gierse et al., 2020). Researchers found stability in the proteome and metabolome profiles of fecal samples despite fluctuations in microbe taxonomy, presenting an interesting story of functional redundancy that could not have been told through a single platform of analysis (Gierse et al., 2020). An integrated metagenomic, metatranscriptomic and metaproteomic approach was used to link microbial functional signatures to metabolic traits in distinct taxa in the fecal microbiome for type 1 diabetes mellitus (Heintz-Buschart et al., 2016). Similar longitudinal multi-omic approaches could prove insightful in milk microbiome research.
Conclusions and future directions
In this review we draw attention to a relative lack of research on relationships among the milk microbial community, its functional capacity, and ultimate impact on the proteome, lipidome and metabolome of milk – collectively referred to here as milk-omics. To more completely understand how the milk microbiome impacts maternal and infant health, research should incorporate multi-omics approaches that profile the analytes that have a closer relationship to phenotype, such as RNA, proteins, and metabolites. Although several omics studies have been conducted in milk, most have focused on molecules that are produced by the host. Despite the use of multi-omic approaches to study host-associated microbial communities in other anatomical sites, investigations of the milk microbiome have largely been limited to metagenomics and metabolomics. Uncovering the changes in microbial functional output could be a useful next step in understanding the development of mastitis. Future research must also encompass non-bacterial microbial community members such as fungi, viruses, archaea, and protists and their role in host health and disease. Mastitis that requires clinical intervention is generally treated with antibiotics, but recent studies conducted in other sites of dysbiosis, like the GI tract and vagina, suggest that it may be more beneficial to foster the colonization of health promoting bacteria as opposed to eliminating pathogenic bacteria through antibiotics (Spencer, 2008; Schmidt et al., 2020; Chen et al., 2021). Milk-omics research would also benefit from standardization of methods for recording metadata, sample collection/storage, analysis and data curation (Eloe-Fadrosh et al., 2021). This would increase the generalizability of findings across studies. Inter-individual variations in milk composition points to the need for factoring in metadata regarding maternal diet, genotype, environment, and birth mode to be able to make meaningful comparisons of findings between individuals, cohorts, or studies.
The field of milk microbiome research is an actively developing area of research, and the question of who is doing what in this ecological niche is still largely unexplored. As further investigations are conducted, we advocate for employing integrated multi-omics approaches that go beyond compositional profiling in order to gain a comprehensive mechanistic understanding for the impact of the milk microbiome on the lactating mother and the nursing infant or consumer of dairy products. There is an overarching need for standardization of methods, from sample and metadata collection to data integration and interpretation. The development and adoption of community-wide metadata standards and data generation pipelines, open-source bioinformatic tools and workflows and also making data FAIR (Wilkinson et al., 2016) will empower the milk microbiome research community and accelerate discovery and innovation.
Funding
This work was supported by the National Institutes of Health NICHD R01 HD092297, US Department of Agriculture, and the USDA National Institute of Food and Agriculture, Hatch project IDA01643.
Author contributions
SC and TM initiated and conceptualized the review topic. KM and SC worked on the initial draft. All authors contributed to the literature review, manuscript writing, and approved the submitted version.
Acknowledgments
The authors thank Pacific Northwest National Laboratory (PNNL) scientific illustrator, Nathan Johnson, for creating the figure.
Conflict of interest
The authors declare that the research was conducted in the absence of any commercial or financial relationships that could be construed as a potential conflict of interest.
Publisher’s note
All claims expressed in this article are solely those of the authors and do not necessarily represent those of their affiliated organizations, or those of the publisher, the editors and the reviewers. Any product that may be evaluated in this article, or claim that may be made by its manufacturer, is not guaranteed or endorsed by the publisher.
References
Addis, M. F., Tanca, A., Uzzau, S., Oikonomou, G., Bicalho, R. C., and Moroni, P. (2016). The bovine milk microbiota: insights and perspectives from -omics studies. Mol. BioSyst. 12, 2359–2372. doi: 10.1039/c6mb00217j
Afshari, R., Pillidge, C. J., Dias, D. A., Osborn, A. M., and Gill, H. (2021). Biomarkers associated with cheese quality uncovered by integrative multi-omic analysis. Food Control 123:107752. doi: 10.1016/j.foodcont.2020.107752
Aguiar-Pulido, V., Huang, W., Suarez-Ulloa, V., Cickovski, T., Mathee, K., and Narasimhan, G. (2016). Metagenomics, metatranscriptomics, and metabolomics approaches for microbiome analysis. Evol. Bioinformatics Online 12s1, EBO.S36436–EBO.S36416. doi: 10.4137/ebo.S36436
Bashiardes, S., Zilberman-Schapira, G., and Elinav, E. (2016). Use of metatranscriptomics in microbiome research. Bioinform. Biol. Insights 10, BBI.S34610–BBI.S34625. doi: 10.4137/BBI.S34610
Bauermeister, A., Mannochio-Russo, H., Costa-Lotufo, L. V., Jarmusch, A. K., and Dorrestein, P. C. (2022). Mass spectrometry-based metabolomics in microbiome investigations. Nat. Rev. Microbiol. 20, 143–160. doi: 10.1038/s41579-021-00621-9
Bellassi, P., Rocchetti, G., Nocetti, M., Lucini, L., Masoero, F., and Morelli, L. (2021). A combined metabolomic and metagenomic approach to discriminate raw milk for the production of hard cheese. Foods 10:109. doi: 10.3390/foods10010109
Bhat, S. A., Ahmad, S. M., Ibeagha-Awemu, E. M., Bhat, B. A., Dar, M. A., Mumtaz, P. T., et al. (2019). Comparative transcriptome analysis of mammary epithelial cells at different stages of lactation reveals wide differences in gene expression and pathways regulating milk synthesis between Jersey and Kashmiri cattle. PLoS One 14:e0211773. doi: 10.1371/journal.pone.0211773
Bhatt, V. D., Ahir, V. B., Koringa, P. G., Jakhesara, S. J., Rank, D. N., Nauriyal, D. S., et al. (2012). Milk microbiome signatures of subclinical mastitis-affected cattle analysed by shotgun sequencing. J. Appl. Microbiol. 112, 639–650. doi: 10.1111/j.1365-2672.2012.05244.x
Biggs, M. B., Medlock, G. L., Kolling, G. L., and Papin, J. A. (2015). Metabolic network modeling of microbial communities. Wiley Interdiscip. Rev. Syst. Biol. Med. 7, 317–334. doi: 10.1002/wsbm.1308
Bionaz, M., Periasamy, K., Rodriguez-Zas, S. L., Everts, R. E., Lewin, H. A., Hurley, W. L., et al. (2012). Old and new stories: revelations from functional analysis of the bovine mammary transcriptome during the lactation cycle. PLoS One 7:e33268. doi: 10.1371/journal.pone.0033268
Boix-Amorós, A., Hernández-Aguilar, M. T., Artacho, A., Collado, M. C., and Mira, A. (2020). Human milk microbiota in sub-acute lactational mastitis induces inflammation and undergoes changes in composition, diversity and load. Sci. Rep. 10:18521. doi: 10.1038/s41598-020-74719-0
Cadwallader, K., and Singh, T. K. (2009). Flavours and off-flavours in milk and dairy products. Adv. Dairy Chem. 3, 631–690. doi: 10.1007/978-0-387-84865-5_14
Ceciliani, F., Audano, M., Addis, M. F., Lecchi, C., Ghaffari, M. H., Albertini, M., et al. (2021). The untargeted lipidomic profile of quarter milk from dairy cows with subclinical intramammary infection by non-aureus staphylococci. J. Dairy Sci. 104, 10268–10281. doi: 10.3168/jds.2020-19975
Celaj, A., Markle, J., Danska, J., and Parkinson, J. (2014). Comparison of assembly algorithms for improving rate of metatranscriptomic functional annotation. Microbiome 2:39. doi: 10.1186/2049-2618-2-39
Cephas, K. D., Kim, J., Mathai, R. A., Barry, K. A., Dowd, S. E., Meline, B. S., et al. (2011). Comparative analysis of salivary bacterial microbiome diversity in edentulous infants and their mothers or primary care givers using pyrosequencing. PLoS One 6:e23503. doi: 10.1371/journal.pone.0023503
Chaleckis, R., Meister, I., Zhang, P., and Wheelock, C. E. (2019). Challenges, progress and promises of metabolite annotation for LC-MS-based metabolomics. Curr. Opin. Biotechnol. 55, 44–50. doi: 10.1016/j.copbio.2018.07.010
Chen, T., Xia, C., Hu, H., Wang, H., Tan, B., Tian, P., et al. (2021). Dysbiosis of the rat vagina is efficiently rescued by vaginal microbiota transplantation or probiotic combination. Int. J. Antimicrob. Agents 57:106277. doi: 10.1016/j.ijantimicag.2021.106277
Chiaradia, E., Valiani, A., Tartaglia, M., Scoppetta, F., Renzone, G., Arena, S., et al. (2013). Ovine subclinical mastitis: proteomic analysis of whey and milk fat globules unveils putative diagnostic biomarkers in milk. J. Proteome 83, 144–159. doi: 10.1016/j.jprot.2013.03.017
Collado, M. C., Delgado, S., Maldonado, A., and Rodríguez, J. M. (2009). Assessment of the bacterial diversity of breast milk of healthy women by quantitative real-time PCR. Lett. Appl. Microbiol. 48, 523–528. doi: 10.1111/j.1472-765X.2009.02567.x
Conesa, A., and Beck, S. (2019). Making multi-omics data accessible to researchers. Scientific Data 6:251. doi: 10.1038/s41597-019-0258-4
Couvillion, S. P., Agrawal, N., Colby, S. M., Brandvold, K. R., and Metz, T. O. (2020). Who is metabolizing what? Discovering novel biomolecules in the microbiome and the organisms who make them. Front. Cell. Infect. Microbiol. 10:388. doi: 10.3389/fcimb.2020.00388
Dahlberg, J., Sun, L., Persson Waller, K., Ostensson, K., McGuire, M., Agenas, S., et al. (2019). Microbiota data from low biomass milk samples is markedly affected by laboratory and reagent contamination. PLoS One 14:e0218257. doi: 10.1371/journal.pone.0218257
Danielsen, M., Codrea, M. C., Ingvartsen, K. L., Friggens, N. C., Bendixen, E., and Røntved, C. M. (2010). Quantitative milk proteomics – host responses to lipopolysaccharide-mediated inflammation of bovine mammary gland. Proteomics 10, 2240–2249. doi: 10.1002/pmic.200900771
de Andrés, J., Jiménez, E., Chico-Calero, I., Fresno, M., Fernández, L., and Rodríguez, J. M. (2017). Physiological translocation of lactic acid bacteria during pregnancy contributes to the composition of the Milk microbiota in mice. Nutrients 10:14. doi: 10.3390/nu10010014
Delgado, S., Arroyo, R., Jiménez, E., Marín, M. L., del Campo, R., Fernández, L., et al. (2009). Staphylococcus epidermidis strains isolated from breast milk of women suffering infectious mastitis: potential virulence traits and resistance to antibiotics. BMC Microbiol. 9:82. doi: 10.1186/1471-2180-9-82
Dhankhar, J., Sharma, R., and Indumathi, K. P. (2016). Bioactive lipids in milk. Int. Food Res. J. 23, 2326–2334.
Di Bella, J. M., Bao, Y., Gloor, G. B., Burton, J. P., and Reid, G. (2013). High throughput sequencing methods and analysis for microbiome research. J. Microbiol. Methods 95, 401–414. doi: 10.1016/j.mimet.2013.08.011
D’Souza, G., Shitut, S., Preussger, D., Yousif, G., Waschina, S., and Kost, C. (2018). Ecology and evolution of metabolic cross-feeding interactions in bacteria. Nat. Prod. Rep. 35, 455–488. doi: 10.1039/c8np00009c
Eloe-Fadrosh, E. A., Ahmed, F., Anubhav,, Babinski, M., Baumes, J., Borkum, M., et al. (2021). The National Microbiome Data Collaborative Data Portal: an integrated multi-omics microbiome data resource. Nucleic Acids Res. 50, D828–D836. doi: 10.1093/nar/gkab990
Fernandez, L., Langa, S., Martin, V., Maldonado, A., Jimenez, E., Martin, R., et al. (2013). The human milk microbiota: origin and potential roles in health and disease. Pharmacol. Res. 69, 1–10. doi: 10.1016/j.phrs.2012.09.001
Foroutan, A., Guo, A. C., Vazquez-Fresno, R., Lipfert, M., Zhang, L., Zheng, J., et al. (2019). Chemical composition of commercial Cow’s Milk. J. Agric. Food Chem. 67, 4897–4914. doi: 10.1021/acs.jafc.9b00204
France, M., Alizadeh, M., Brown, S., Ma, B., and Ravel, J. (2022). Towards a deeper understanding of the vaginal microbiota. Nat. Microbiol. 7, 367–378. doi: 10.1038/s41564-022-01083-2
Gagnaire, V., Jardin, J., Jan, G., and Lortal, S. (2009). Invited review: proteomics of milk and bacteria used in fermented dairy products: from qualitative to quantitative advances. J. Dairy Sci. 92, 811–825. doi: 10.3168/jds.2008-1476
Gagnaire, V., Piot, M., Camier, B., Vissers, J. P. C., Jan, G., and Leonil, J. (2004). Survey of bacterial proteins released in cheese: a proteomic approach. Int. J. Food Microbiol. 94, 185–201. doi: 10.1016/j.ijfoodmicro.2004.01.010
Gay, M. C. L., Koleva, P. T., Slupsky, C. M., Toit, E. D., Eggesbo, M., Johnson, C. C., et al. (2018). Worldwide variation in human Milk Metabolome: indicators of breast physiology and maternal lifestyle? Nutrients 10:1151. doi: 10.3390/nu10091151
George, A. D., Gay, M. C. L., Selvalatchmanan, J., Torta, F., Bendt, A. K., Wenk, M. R., et al. (2021). Healthy breastfeeding infants consume different quantities of Milk fat globule membrane lipids. Nutrients 13:2951. doi: 10.3390/nu13092951
George, A. D., Gay, M. C. L., Wlodek, M. E., Trengove, R. D., Murray, K., and Geddes, D. T. (2020). Untargeted lipidomics using liquid chromatography-ion mobility-mass spectrometry reveals novel triacylglycerides in human milk. Sci. Rep. 10:9255. doi: 10.1038/s41598-020-66235-y
Gierse, L. C., Meene, A., Schultz, D., Schwaiger, T., Karte, C., Schroder, C., et al. (2020). A multi-omics protocol for swine feces to elucidate longitudinal dynamics in microbiome structure and function. Microorganisms 8:1887. doi: 10.3390/microorganisms8121887
Gomez-Gallego, C., Morales, J. M., Monleon, D., du Toit, E., Kumar, H., Linderborg, K. M., et al. (2018). Human breast milk NMR metabolomic profile across specific geographical locations and its association with the milk microbiota. Nutrients 10:1355. doi: 10.3390/nu10101355
Grice, E. A., and Segre, J. A. (2011). The skin microbiome. Nat. Rev. Microbiol. 9, 244–253. doi: 10.1038/nrmicro2537
Gueimonde, M., Laitinen, K., Salminen, S., and Isolauri, E. (2007). Breast milk: a source of bifidobacteria for infant gut development and maturation? Neonatology 92, 64–66. doi: 10.1159/000100088
Hehemann, J. H., Correc, G., Barbeyron, T., Helbert, W., Czjzek, M., and Michel, G. (2010). Transfer of carbohydrate-active enzymes from marine bacteria to Japanese gut microbiota. Nature 464, 908–912. doi: 10.1038/nature08937
Heintz-Buschart, A., May, P., Laczny, C. C., Lebrun, L. A., Bellora, C., Krishna, A., et al. (2016). Integrated multi-omics of the human gut microbiome in a case study of familial type 1 diabetes. Nat. Microbiol. 2:16180. doi: 10.1038/nmicrobiol.2016.180
Heintz-Buschart, A., and Westerhuis, J. A. (2022). A beginner’s guide to integrating multi-omics data from microbial communities. Biochemist 44, 23–29. doi: 10.1042/bio_2022_100
Heintz-Buschart, A., and Wilmes, P. (2018). Human gut microbiome: function matters. Trends Microbiol. 26, 563–574. doi: 10.1016/j.tim.2017.11.002
Hettich, R. L., Pan, C., Chourey, K., and Giannone, R. J. (2013). Metaproteomics: harnessing the power of high performance mass spectrometry to identify the suite of proteins that control metabolic activities in microbial communities. Anal. Chem. 85, 4203–4214. doi: 10.1021/ac303053e
Hewelt-Belka, W., Garwolińska, D., Młynarczyk, M., and Kot-Wasik, A. (2020). Comparative lipidomic study of human milk from different lactation stages and milk formulas. Nutrients 12:2165. doi: 10.3390/nu12072165
Hoque, M. N., Istiaq, A., Clement, R. A., Sultana, M., Crandall, K. A., Siddiki, A. Z., et al. (2019). Metagenomic deep sequencing reveals association of microbiome signature with functional biases in bovine mastitis. Sci. Rep. 9:13536. doi: 10.1038/s41598-019-49468-4
Hoque, M. N., Istiaq, A., Rahman, M. S., Islam, M. R., Anwar, A., Siddiki, A. M. A. M. Z., et al. (2020). Microbiome dynamics and genomic determinants of bovine mastitis. Genomics 112, 5188–5203. doi: 10.1016/j.ygeno.2020.09.039
Hoque, M. N., Rahman, M. S., Islam, T., Sultana, M., Crandall, K. A., and Hossain, M. A. (2022). Induction of mastitis by cow-to-mouse fecal and milk microbiota transplantation causes microbiome dysbiosis and genomic functional perturbation in mice. Anim. Microbiome 4:43. doi: 10.1186/s42523-022-00193-w
Hunt, K. M., Foster, J. A., Forney, L. J., Schutte, U. M., Beck, D. L., Abdo, Z., et al. (2011). Characterization of the diversity and temporal stability of bacterial communities in human milk. PLoS One 6:e21313. doi: 10.1371/journal.pone.0021313
Integrative, H. M. P. R. N. C. (2019). The Integrative human microbiome project. Nature 569, 641–648. doi: 10.1038/s41586-019-1238-8
Issa Isaac, N., Philippe, D., Nicholas, A., Raoult, D., and Eric, C. (2019). Metaproteomics of the human gut microbiota: challenges and contributions to other OMICS. Clin. Mass Spectrom 14, 18–30. doi: 10.1016/j.clinms.2019.06.001
Ji, Z., Chao, T., Zhang, C., Liu, Z., Hou, L., Wang, J., et al. (2019). Transcriptome analysis of dairy goat mammary gland tissues from different lactation stages. DNA Cell Biol. 38, 129–143. doi: 10.1089/dna.2018.4349
Jiang, D., Armour, C. R., Hu, C., Mei, M., Tian, C., Sharpton, T. J., et al. (2019). Microbiome multi-omics network analysis: statistical considerations, limitations, and opportunities. Front. Genet. 10:995. doi: 10.3389/fgene.2019.00995
Jiang, X., Zhang, Y., Wang, H., Wang, Z., Hu, S., Cao, C., et al. (2022). In-depth metaproteomics analysis of oral microbiome for lung cancer. Research 2022:9781578. doi: 10.34133/2022/9781578
Jiménez, E., de Andrés, J., Manrique, M., Pareja-Tobes, P., Tobes, R., Martínez-Blanch, J. F., et al. (2015). Metagenomic analysis of Milk of healthy and mastitis-suffering women. J. Hum. Lact. 31, 406–415. doi: 10.1177/0890334415585078
Jones, C. (2001). Maternal transmission of infectious pathogens in breast milk. J. Paediatr. Child Health 37, 576–582. doi: 10.1046/j.1440-1754.2001.00743.x
Jost, T., Lacroix, C., Braegger, C. P., Rochat, F., and Chassard, C. (2014). Vertical mother-neonate transfer of maternal gut bacteria via breastfeeding. Environ. Microbiol. 16, 2891–2904. doi: 10.1111/1462-2920.12238
Kanehisa, M., Furumichi, M., Tanabe, M., Sato, Y., and Morishima, K. (2017). KEGG: new perspectives on genomes, pathways, diseases and drugs. Nucleic Acids Res. 45, D353–D361. doi: 10.1093/nar/gkw1092
Kleiner, M., Thorson, E., Sharp, C. E., Dong, X., Liu, D., Li, C., et al. (2017). Assessing species biomass contributions in microbial communities via metaproteomics. Nat. Commun. 8:1558. doi: 10.1038/s41467-017-01544-x
Knight, R., Vrbanac, A., Taylor, B. C., Aksenov, A., Callewaert, C., Debelius, J., et al. (2018). Best practices for analysing microbiomes. Nat. Rev. Microbiol. 16, 410–422. doi: 10.1038/s41579-018-0029-9
Kordy, K., Gaufin, T., Mwangi, M., Li, F., Cerini, C., Lee, D. J., et al. (2020). Contributions to human breast milk microbiome and enteromammary transfer of Bifidobacterium breve. PLoS One 15:e0219633. doi: 10.1371/journal.pone.0219633
Kusumawati, A., Mustopa, A. Z., Wibawan, I. W. T., Setiyono, A., and Sudarwanto, M. B. (2021). Metagenomic analysis of pathogen mastitis in cow’s milk from Cicurug, Sukabumi, West Java, Indonesia. IOP Conf. Ser. 762:012064. doi: 10.1088/1755-1315/762/1/012064
Lackey, K. A., Williams, J. E., Meehan, C. L., Zachek, J. A., Benda, E. D., Price, W. J., et al. (2019). What’s normal? Microbiomes in human milk and infant feces are related to each other but vary geographically: the INSPIRE study. Front. Nutr. 6:45. doi: 10.3389/fnut.2019.00045
Larsen, L. B., Hinz, K., Jørgensen, A. L. W., Møller, H. S., Wellnitz, O., Bruckmaier, R. M., et al. (2010). Proteomic and peptidomic study of proteolysis in quarter milk after infusion with lipoteichoic acid from Staphylococcus aureus. J. Dairy Sci. 93, 5613–5626. doi: 10.3168/jds.2010-3409
Latuga, M. S., Stuebe, A., and Seed, P. C. (2014). A review of the source and function of microbiota in breast milk. Semin. Reprod. Med. 32, 068–073. doi: 10.1055/s-0033-1361824
Lee, J. Y., Mitchell, H. D., Burnet, M. C., Wu, R., Jenson, S. C., Merkley, E. D., et al. (2022). Uncovering hidden members and functions of the soil microbiome using De novo Metaproteomics. J. Proteome Res. 21, 2023–2035. doi: 10.1021/acs.jproteome.2c00334
Lemay, D. G., Ballard, O. A., Hughes, M. A., Morrow, A. L., Horseman, N. D., and Nommsen-Rivers, L. A. (2013). RNA sequencing of the human Milk fat layer Transcriptome reveals distinct gene expression profiles at three stages of lactation. PLoS One 8:e67531. doi: 10.1371/journal.pone.0067531
Leung, H. C., Yiu, S.-M., Parkinson, J., and Chin, F. Y. (2013). IDBA-MT: de novo assembler for metatranscriptomic data generated from next-generation sequencing technology. J. Comput. Biol. 20, 540–550. doi: 10.1089/cmb.2013.0042
Li, F., Hitch, T. C. A., Chen, Y., Creevey, C. J., and Guan, L. L. (2019). Comparative metagenomic and metatranscriptomic analyses reveal the breed effect on the rumen microbiome and its associations with feed efficiency in beef cattle. Microbiome 7:6. doi: 10.1186/s40168-019-0618-5
Lin, Y., Lv, H., Jiang, M., Zhou, J., Song, S., and Hou, X. (2019). Functional analysis of the dairy cow mammary transcriptome between early lactation and mid-dry period. J. Dairy Res. 86, 63–67. doi: 10.1017/S0022029919000049
Long, S., Yang, Y., Shen, C., Wang, Y., Deng, A., Qin, Q., et al. (2020). Metaproteomics characterizes human gut microbiome function in colorectal cancer. NPJ Biofilms Microbiomes 6:14. doi: 10.1038/s41522-020-0123-4
Lu, J., Boeren, S., van Hooijdonk, T., Vervoort, J., and Hettinga, K. (2015). Effect of the DGAT1 K232A genotype of dairy cows on the milk metabolome and proteome. J. Dairy Sci. 98, 3460–3469. doi: 10.3168/jds.2014-8872
Lu, J., Fernandes, E. A., Cano, A. E. P., Vinitwatanakhun, J., Boeren, S., van Hooijdonk, T., et al. (2013). Changes in Milk proteome and metabolome associated with dry period length, energy balance, and lactation stage in postparturient dairy cows. J. Proteome Res. 12, 3288–3296. doi: 10.1021/pr4001306
Ma, C., Sun, Z., Zeng, B., Huang, S., Zhao, J., Zhang, Y., et al. (2018). Cow-to-mouse fecal transplantations suggest intestinal microbiome as one cause of mastitis. Microbiome 6:200. doi: 10.1186/s40168-018-0578-1
Manso, M. A., Leonil, J., Jan, G., and Gagnaire, V. (2005). Application of proteomics to the characterisation of milk and dairy products. Int. Dairy J. 15, 845–855. doi: 10.1016/j.idairyj.2004.07.021
Mansor, R., Mullen, W., Albalat, A., Zerefos, P., Mischak, H., Barrett, D. C., et al. (2013). A peptidomic approach to biomarker discovery for bovine mastitis. J. Proteome 85, 89–98. doi: 10.1016/j.jprot.2013.04.027
Martin Carli, J. F., Trahan, G. D., Jones, K. L., Hirsch, N., Rolloff, K. P., Dunn, E. Z., et al. (2020). Single cell RNA sequencing of human Milk-derived cells reveals sub-populations of mammary epithelial cells with molecular signatures of progenitor and mature states: a novel, non-invasive framework for investigating human lactation physiology. J. Mammary Gland Biol. Neoplasia 25, 367–387. doi: 10.1007/s10911-020-09466-z
Martín, R., Langa, S., Reviriego, C., Jimínez, E., Marín, M. L., Xaus, J., et al. (2003). Human milk is a source of lactic acid bacteria for the infant gut. J. Pediatr. 143, 754–758. doi: 10.1016/j.jpeds.2003.09.028
Moossavi, S., Atakora, F., Miliku, K., Sepehri, S., Robertson, B., Duan, Q. L., et al. (2019a). Integrated analysis of human Milk microbiota with oligosaccharides and fatty acids in the CHILD cohort. Front. Nutr. 6:58. doi: 10.3389/fnut.2019.00058
Moossavi, S., Fehr, K., Khafipour, E., and Azad, M. B. (2021). Repeatability and reproducibility assessment in a large-scale population-based microbiota study: case study on human milk microbiota. Microbiome 9:41. doi: 10.1186/s40168-020-00998-4
Moossavi, S., Sepehri, S., Robertson, B., Bode, L., Goruk, S., Field, C. J., et al. (2019b). Composition and variation of the human milk microbiota are influenced by maternal and early-life factors. Cell Host Microbe 25, 324–335.e4. doi: 10.1016/j.chom.2019.01.011
Morris, K. M., O’Meally, D., Zaw, T., Song, X. M., Gillett, A., Molloy, M. P., et al. (2016). Characterisation of the immune compounds in koala milk using a combined transcriptomic and proteomic approach. Sci. Rep. 6:5011. doi: 10.1038/srep35011
Moya, A., and Ferrer, M. (2016). Functional redundancy-induced stability of gut microbiota subjected to disturbance. Trends Microbiol. 24, 402–413. doi: 10.1016/j.tim.2016.02.002
Nicholson, J. K., Holmes, E., and Wilson, I. D. (2005). Gut microorganisms, mammalian metabolism and personalized health care. Nat. Rev. Microbiol. 3, 431–438. doi: 10.1038/nrmicro1152
Noecker, C., Eng, A., Srinivasan, S., Theriot, C. M., Young, V. B., Jansson, J. K., et al. (2016). Metabolic model-based integration of microbiome taxonomic and metabolomic profiles elucidates mechanistic links between ecological and metabolic variation. mSystems 1, e00013–e00015. doi: 10.1128/mSystems.00013-15
Nyholm, L., Koziol, A., Marcos, S., Botnen, A. B., Aizpurua, O., Gopalakrishnan, S., et al. (2020). Holo-Omics: integrated host-microbiota multi-omics for basic and applied biological research. iScience 23:101414. doi: 10.1016/j.isci.2020.101414
O’Donnell, R., Holland, J. W., Deeth, H. C., and Alewood, P. (2004). Milk proteomics. Int. Dairy J. 14, 1013–1023. doi: 10.1016/j.idairyj.2004.04.004
Oikonomou, G., Addis, M. F., Chassard, C., Nader-Macias, M. E. F., Grant, I., Delbès, C., et al. (2020). Milk microbiota: what are we exactly talking about? Front. Microbiol. 11:60. doi: 10.3389/fmicb.2020.00060
Olshan, K. L., Zomorrodi, A. R., Pujolassos, M., Troisi, J., Khan, N., Fanelli, B., et al. (2021). Microbiota and metabolomic patterns in the breast milk of subjects with celiac disease on a gluten-free diet. Nutrients 13:2243. doi: 10.3390/nu13072243
Pace, R. M., Pace, C. D. W., Fehrenkamp, B. D., Price, W. J., Lewis, M., Williams, J. E., et al. (2022). Sodium and potassium concentrations and somatic cell count of human milk produced in the first six weeks postpartum and their suitability as biomarkers of clinical and subclinical mastitis. Nutrients 14:4708. doi: 10.3390/nu14224708
Pace, R. M., Williams, J. E., Robertson, B., Lackey, K. A., Meehan, C. L., Price, W. J., et al. (2021). Variation in human milk composition is related to differences in milk and infant fecal microbial communities. Microorganisms 9:1153. doi: 10.3390/microorganisms9061153
Pannaraj, P. S., Li, F., Cerini, C., Bender, J. M., Yang, S., Rollie, A., et al. (2017). Association between breast milk bacterial communities and establishment and development of the infant gut microbiome. JAMA Pediatr. 171, 647–654. doi: 10.1001/jamapediatrics.2017.0378
Parente, E., Ricciardi, A., and Zotta, T. (2020). The microbiota of dairy milk: a review. Int. Dairy J. 107:104714. doi: 10.1016/j.idairyj.2020.104714
Patel, R. J., Pandit, R. J., Bhatt, V. D., Kunjadia, P. D., Nauriyal, D. S., Koringa, P. G., et al. (2017). Metagenomic approach to study the bacterial community in clinical and subclinical mastitis in buffalo. Meta Gene 12, 4–12. doi: 10.1016/j.mgene.2016.12.014
Perez, P. F., Dore, J., Leclerc, M., Levenez, F., Benyacoub, J., Serrant, P., et al. (2007). Bacterial imprinting of the neonatal immune system: lessons from maternal cells? Pediatrics 119, e724–e732. doi: 10.1542/peds.2006-1649
Perez-Losada, M., Castro-Nallar, E., Bendall, M. L., Freishtat, R. J., and Crandall, K. A. (2015). Dual transcriptomic profiling of host and microbiota during health and disease in pediatric asthma. PLoS One 10:e0131819. doi: 10.1371/journal.pone.0131819
Perrone, S., Longini, M., Zollino, I., Bazzini, F., Tassini, M., Vivi, A., et al. (2019). Breast milk: to each his own. From metabolomic study, evidence of personalized nutrition in preterm infants. Nutrition 62, 158–161. doi: 10.1016/j.nut.2018.12.015
Piras, C., Greco, V., Gugliandolo, E., Soggiu, A., Tilocca, B., Bonizzi, L., et al. (2020). Raw cow milk bacterial consortium as bioindicator of circulating anti-microbial resistance (AMR). Animals (Basel) 10:2378. doi: 10.3390/ani10122378
Qian, L., Zhao, A., Zhang, Y., Chen, T., Zeisel, S. H., Jia, W., et al. (2016). Metabolomic approaches to explore chemical diversity of human breast-milk, formula milk and bovine milk. Int. J. Mol. Sci. 17:128. doi: 10.3390/ijms17122128
Qin, J., Li, Y., Cai, Z., Li, S., Zhu, J., Zhang, F., et al. (2012). A metagenome-wide association study of gut microbiota in type 2 diabetes. Nature 490, 55–60. doi: 10.1038/nature11450
Quigley, L., O’Sullivan, O., Stanton, C., Beresford, T. P., Ross, R. P., Fitzgerald, G. F., et al. (2013). The complex microbiota of raw milk. FEMS Microbiol. Rev. 37, 664–698. doi: 10.1111/1574-6976.12030
Quince, C., Walker, A. W., Simpson, J. T., Loman, N. J., and Segata, N. (2017). Shotgun metagenomics, from sampling to analysis. Nat. Biotechnol. 35, 833–844. doi: 10.1038/nbt.3935
Rodríguez, J. M. (2014). The origin of human milk bacteria: is there a bacterial entero-mammary pathway during late pregnancy and lactation? Adv. Nutr. 5, 779–784. doi: 10.3945/an.114.007229
Rohart, F., Gautier, B., Singh, A., and Lê Cao, K. -A. (2017). mixOmics: An R package for ‘omics feature selection and multiple data integration. PLoS Comput. Biol. 13:e1005752. doi: 10.1371/journal.pcbi.1005752
Ruiz, L., Bacigalupe, R., García-Carral, C., Boix-Amoros, A., Argüello, H., Silva, C. B., et al. (2019). Microbiota of human precolostrum and its potential role as a source of bacteria to the infant mouth. Sci. Rep. 9:8435. doi: 10.1038/s41598-019-42514-1
Salvato, F., Hettich, R. L., and Kleiner, M. (2021).Five key aspects of metaproteomics as a tool to understand functional interactions in host-associated microbiomes. PLoS Pathog. 17:e1009245. doi: 10.1371/journal.ppat.1009245
Scano, P., Murgia, A., Demuru, M., Consonni, R., and Caboni, P. (2016). Metabolite profiles of formula milk compared to breast milk. Food Res. Int. 87, 76–82. doi: 10.1016/j.foodres.2016.06.024
Schmidt, E. K. A., Torres-Espin, A., Raposo, P. J. F., Madsen, K. L., Kigerl, K. A., Popovich, P. G., et al. (2020). Fecal transplant prevents gut dysbiosis and anxiety-like behaviour after spinal cord injury in rats. PLoS One 15:e0226128. doi: 10.1371/journal.pone.0226128
Schukken, Y. H., Wilson, D. J., Welcome, F., Garrison-Tikofsky, L., and Gonzalez, R. N. (2003). Monitoring udder health and milk quality using somatic cell counts. Vet. Res. 34, 579–596. doi: 10.1051/vetres:2003028
Seferovic, M. D., Mohammad, M., Pace, R. M., Engevik, M., Versalovic, J., Bode, L., et al. (2020). Maternal diet alters human milk oligosaccharide composition with implications for the milk metagenome. Sci. Rep. 10:22092. doi: 10.1038/s41598-020-79022-6
Shaffer, M., Thurimella, K., Quinn, K., Doenges, K., Zhang, X., Bokatzian, S., et al. (2019). AMON: annotation of metabolite origins via networks to integrate microbiome and metabolome data. BMC Bioinformatics 20:614. doi: 10.1186/s12859-019-3176-8
Shakya, M., Lo, C.-C., and Chain, P. S. G. (2019). Advances and challenges in metatranscriptomic analysis. Front. Genet. 10:904. doi: 10.3389/fgene.2019.00904
Sharma, A., and Gilbert, J. A. (2018). Microbial exposure and human health. Curr. Opin. Microbiol. 44, 79–87. doi: 10.1016/j.mib.2018.08.003
Shreiner, A. B., Kao, J. Y., and Young, V. B. (2015). The gut microbiome in health and in disease. Curr. Opin. Gastroenterol. 31, 69–75. doi: 10.1097/mog.0000000000000139
Spencer, J. P. (2008). Management of mastitis in breastfeeding women. Am. Fam. Physician 78, 727–731.
Sundekilde, U. K., Larsen, L. B., and Bertram, H. C. (2013a). NMR based milk metabolomics. Metabolites 3, 204–222. doi: 10.3390/metabo3020204
Sundekilde, U. K., Poulsen, N. A., Larsen, L. B., and Bertram, H. C. (2013b). Nuclear magnetic resonance metabonomics reveals strong association between milk metabolites and somatic cell count in bovine milk. J. Dairy Sci. 96, 290–299. doi: 10.3168/jds.2012-5819
Tarazona, S., Balzano-Nogueira, L., Gómez-Cabrero, D., Schmidt, A., Imhof, A., Hankemeier, T., et al. (2020). Harmonization of quality metrics and power calculation in multi-omic studies. Nat. Commun. 11:3092. doi: 10.1038/s41467-020-16937-8
Thomas, F. C., Mullen, W., Tassi, R., Ramírez-Torres, A., Mudaliar, M., McNeilly, T. N., et al. (2016). Mastitomics, the integrated omics of bovine milk in an experimental model of streptococcus uberis mastitis: 1. High abundance proteins, acute phase proteins and peptidomics. Mol. BioSyst. 12, 2735–2747. doi: 10.1039/C6MB00239K
Tong, J., Zhang, H., Zhang, Y., Xiong, B., and Jiang, L. (2019). Microbiome and Metabolome analyses of Milk from dairy cows with subclinical Streptococcus agalactiae mastitis-potential biomarkers. Front. Microbiol. 10:2547. doi: 10.3389/fmicb.2019.02547
Treven, P., Mrak, V., Bogovič Matijašić, B., Horvat, S., and Rogelj, I. (2015). Administration of probiotics lactobacillus rhamnosus GG and lactobacillus gasseri K7 during pregnancy and lactation changes mouse mesenteric lymph nodes and mammary gland microbiota. J. Dairy Sci. 98, 2114–2128. doi: 10.3168/jds.2014-8519
Van Den Bossche, T., Arntzen, M. Ø., Becher, D., Benndorf, D., Eijsink, V. G. H., Henry, C., et al. (2021). The Metaproteomics initiative: a coordinated approach for propelling the functional characterization of microbiomes. Microbiome 9:243. doi: 10.1186/s40168-021-01176-w
Vital, M., Howe, A. C., and Tiedje, J. M. (2014). Revealing the bacterial butyrate synthesis pathways by analyzing (meta)genomic data. MBio 5:e00889. doi: 10.1128/mBio.00889-14
Wang, W. L., Xu, S. Y., Ren, Z. G., Tao, L., Jiang, J. W., and Zheng, S. S. (2015). Application of metagenomics in the human gut microbiome. World J. Gastroenterol. 21, 803–814. doi: 10.3748/wjg.v21.i3.803
Whidbey, C., Sadler, N. C., Nair, R. N., Volk, R. F., DeLeon, A. J., Bramer, L. M., et al. (2019). A probe-enabled approach for the selective isolation and characterization of functionally active subpopulations in the gut microbiome. J. Am. Chem. Soc. 141, 42–47. doi: 10.1021/jacs.8b09668
Wilkinson, M. D., Dumontier, M., Aalbersberg, I. J., Appleton, G., Axton, M., Baak, A., et al. (2016). The FAIR guiding principles for scientific data management and stewardship. Scientific Data 3:160018. doi: 10.1038/sdata.2016.18
Williams, J. E., Carrothers, J. M., Lackey, K. A., Beatty, N. F., Brooker, S. L., Peterson, H. K., et al. (2019). Strong multivariate relations exist among Milk, Oral, and fecal microbiomes in mother-infant dyads during the first six months postpartum. J. Nutr. 149, 902–914. doi: 10.1093/jn/nxy299
Williams, J. E., Carrothers, J. M., Lackey, K. A., Beatty, N. F., York, M. A., Brooker, S. L., et al. (2017a). Human Milk microbial community structure is relatively stable and related to variations in macronutrient and micronutrient intakes in healthy lactating women. J. Nutr. 147, 1739–1748. doi: 10.3945/jn.117.248864
Williams, J. E., Price, W. J., Shafii, B., Yahvah, K. M., Bode, L., McGuire, M. A., et al. (2017b). Relationships among microbial communities, maternal cells, oligosaccharides, and macronutrients in human Milk. J. Hum. Lact. 33, 540–551. doi: 10.1177/0890334417709433
Willumsen, J. F., Filteau, S. M., Coutsoudis, A., Newell, M. L., Rollins, N. C., Coovadia, H. M., et al. (2003). Breastmilk RNA viral load in HIV-infected South African women: effects of subclinical mastitis and infant feeding. AIDS 17, 407–414. doi: 10.1097/00002030-200302140-00015
Xi, X., Kwok, L.-Y., Wang, Y., Ma, C., Mi, Z., and Zhang, H. (2017). Ultra-performance liquid chromatography-quadrupole-time of flight mass spectrometry MSE-based untargeted milk metabolomics in dairy cows with subclinical or clinical mastitis. J. Dairy Sci. 100, 4884–4896. doi: 10.3168/jds.2016-11939
Xue, Y., Lanzén, A., and Jonassen, I. (2020). Reconstructing ribosomal genes from large scale total RNA meta-transcriptomic data. Bioinformatics 36, 3365–3371. doi: 10.1093/bioinformatics/btaa177
Yap, M., Feehily, C., Walsh, C. J., Fenelon, M., Murphy, E. F., McAuliffe, F. M., et al. (2020). Evaluation of methods for the reduction of contaminating host reads when performing shotgun metagenomic sequencing of the milk microbiome. Sci. Rep. 10:21665. doi: 10.1038/s41598-020-78773-6
Ye, Y., and Tang, H. (2015). Utilizing de Bruijn graph of metagenome assembly for metatranscriptome analysis. Bioinformatics 32, 1001–1008. doi: 10.1093/bioinformatics/btv510
Young, W., Hine, B. C., Wallace, O. A., Callaghan, M., and Bibiloni, R. (2015). Transfer of intestinal bacterial components to mammary secretions in the cow. PeerJ 3:e888. doi: 10.7717/peerj.888
Zhang, L., Boeren, S., van Hooijdonk, A. C., Vervoort, J. M., and Hettinga, K. A. (2015). A proteomic perspective on the changes in milk proteins due to high somatic cell count. J. Dairy Sci. 98, 5339–5351. doi: 10.3168/jds.2014-9279
Zhang, A., Sun, H., Wang, P., Han, Y., and Wang, X. (2012). Modern analytical techniques in metabolomics analysis. Analyst 137, 293–300. doi: 10.1039/c1an15605e
Zhang, Y., Thompson, K. N., Branck, T., Yan, Y., Nguyen, L. H., Franzosa, E. A., et al. (2021). Metatranscriptomics for the human microbiome and microbial community functional profiling. Ann. Rev. Biomed. Data Sci. 4, 279–311. doi: 10.1146/annurev-biodatasci-031121-103035
Zhu, D., Kebede, B., McComb, K., Hayman, A., Chen, G., and Frew, R. (2021b). Milk biomarkers in relation to inherent and external factors based on metabolomics. Trends Food Sci. Technol. 109, 51–64. doi: 10.1016/j.tifs.2020.12.012
Zhu, C., Tang, K., Lu, X., Tang, J., and Laghi, L. (2021a). An untargeted metabolomics investigation of Milk from dairy cows with clinical mastitis by 1H-NMR. Foods 10:1707. doi: 10.3390/foods10081707
Zimmermann, P., and Curtis, N. (2020). Breast milk microbiota: a review of the factors that influence composition. J. Infect. 81, 17–47. doi: 10.1016/j.jinf.2020.01.023
Keywords: milk, multi-omics, mastitis, metagenomics, metatranscriptomics, metaproteomics, metabolomics, lipidomics
Citation: Couvillion SP, Mostoller KE, Williams JE, Pace RM, Stohel IL, Peterson HK, Nicora CD, Nakayasu ES, Webb-Robertson B-JM, McGuire MA, McGuire MK and Metz TO (2023) Interrogating the role of the milk microbiome in mastitis in the multi-omics era. Front. Microbiol. 14:1105675. doi: 10.3389/fmicb.2023.1105675
Edited by:
Yotsawat Pomyen, Chulabhorn Research Institute, ThailandReviewed by:
Alexandre Lamas, University of Santiago de Compostela, SpainRenée Maxine Petri, Agriculture and Agri-Food Canada (AAFC), Canada
Copyright © 2023 Couvillion, Mostoller, Williams, Pace, Stohel, Peterson, Nicora, Nakayasu, Webb-Robertson, McGuire, McGuire and Metz. This is an open-access article distributed under the terms of the Creative Commons Attribution License (CC BY). The use, distribution or reproduction in other forums is permitted, provided the original author(s) and the copyright owner(s) are credited and that the original publication in this journal is cited, in accordance with accepted academic practice. No use, distribution or reproduction is permitted which does not comply with these terms.
*Correspondence: Sneha P. Couvillion, ✉ sneha.couvillion@pnnl.gov; Thomas O. Metz, ✉ thomas.metz@pnnl.gov
†These authors have contributed equally to this work and share first authorship