- 1Department of Medical Research, PLA Strategic Support Force Medical Center, Beijing, China
- 2Center for Applied Molecular Biology, University of the Punjab, Lahore, Pakistan
- 3Department of Traditional Chinese Medicine, PLA Strategic Support Force Medical Center, Beijing, China
Spaceflight and microgravity has a significant impact on the immune, central nervous, bone, and muscle support and cardiovascular systems. However, limited studies are available on the adverse effects of long-term microgravity on the intestinal microbiota, metabolism, and its relationships. In this study, a ground-based simulated microgravity (SMG) mouse model was established to evaluate the impact of long-term microgravity on gut microbiota and metabolome. After 8 weeks of SMG, alterations of the intestinal microbiota and metabolites were detected using 16S rRNA sequencing and untargeted metabolomics. Compared to the control, no significant differences in α-diversity were observed at weeks 2, 4 and 8. Nevertheless, there were clear differences in community structures at different time points. The phylum Verrucomicrobia significantly declined from 2 to 8 weeks of SMG, yet the relative abundance of Actinobacteria and Deferribacteres expanded remarkably at weeks 8. SMG decreased the genus of Allobaculum and increased Bacteroides significantly throughout the period of 8 weeks. Besides, Genus Akkermansia, Gracilibacter, Prevotella, Odoribacter, Rothia, Sporosarcina, Gracilibacter, Clostridium, and Mucispirillum were identified as biomarkers for SMG group. Desulfovibrio_c21_c20, Akkermansia_muciniphila, and Ruminococcus_gnavus dropped at week 2, which tend to recover at week 4, except for Akkermansia_muciniphila. Bacteroides_uniformis and Faecalibacterium_prausnitzii declined significantly, while Ruminococcus_flavefaciens and Mucispirillum_schaedleri elevated at week 8. Furthermore, intestinal metabolome analysis showed that 129 were upregulated and 146 metabolites were downregulated in SMG. Long-term SMG most affected steroid hormone biosynthesis, tryptophan, cysteine, methionine, arginine, proline metabolism, and histidine metabolism. Correlated analysis suggested that the potential beneficial taxa Allobaculum, Akkermansia, and Faecalibacterium were negatively associated with tryptophan, histidine, arginine, and proline metabolism, but positively with steroid hormone biosynthesis. Yet Bacteroides, Lachnospiraceae_Clostridium, Rothia, Bilophila, and Coprococcus were positively correlated with arginine, proline, tryptophan, and histidine metabolism, while negatively associated with steroid hormone biosynthesis. These results suggest that Long-term SMG altered the community of intestinal microbiota, and then further disturbed intestinal metabolites and metabolic pathways, which have great potential to help understand and provide clues for revealing the mechanisms of long-term SMG involved diseases.
Introduction
Long-duration spaceflight and lunar missions are essential projects for the astronauts in the present and future (Williams et al., 2009). Microgravity, radiation, isolation, and immobilization are some of the factors that profoundly influence astronauts’ physiology during spaceflight (LaPelusa et al., 2021). Multiple shreds of evidence demonstrate that spaceflight has an impact the human immune system, central nervous system, bone and muscle support system, cardiovascular system, and microbiome (Crucian et al., 2016; Van Ombergen et al., 2017). The spaceflight-associated neuro-ocular syndrome has been documented in astronauts during long-duration spaceflights (Lee et al., 2020). During space flight, increased virulence and immune dysregulation may contribute to elevate risk of infectious diseases (Wilson et al., 2007). Therefore, it becomes increasingly important to investigate the impact of specific environmental factors on long-term health in spaceflight.
Gut microbiota play a pivotal role in health and disease. Dysbiosis of gut microbiota is associated with several disorders, including inflammatory bowel disease, obesity, metabolomics, cardio cancer, and even neurodegenerative disorders (Weingarden and Vaughn, 2017; Lim, 2022; Paik et al., 2022; Park et al., 2022; SantaCruz-Calvo et al., 2022; Sorboni et al., 2022). Recent studies demonstrate that the human gut microbiota is sensitive to spaceflight. Previous analysis of the microbiome composition of crewmembers and participants in analog missions suggested that the composition and function of the gut flora were substantially affected by spaceflight and analog missions, including changes to alpha and beta diversity and microbial and host gene expression (Liu et al., 2020). In a long ground-based spaceflight project, MARS500, the human gut microbiota of six astronauts was inherently dynamic, capable of shifting between different steady states and some key microbial clusters showed conserved temporal dynamics (Turroni et al., 2017).
Microgravity is one of the major environmental risk factors for astronauts in spaceflight. In a 45-day head-down bed rest experiment, the urinary excretion of metabolites related to the deconditioning of bone, muscle, and gut flora changed dramatically (Chen et al., 2016). The model of hindlimb unloading in mice or rats, a well-established ground-based simulated microgravity model, was commonly used to mimic specific physiological effects (Chelyshev et al., 2014; Kulikova et al., 2017; Marzuca-Nassr et al., 2019). A recent study illustrated that simulated microgravity suppresses the p38 mitogen-activated protein kinase (MAPK) pathway-mediated innate immune response to bacterial infection and induces gut microbiota dysbiosis. While probiotics VSL#3 alleviated gut microbiota dysbiosis and led to the inhibition of innate immunity (Wang et al., 2020). These human and animal studies indicate the impact of short-term simulated microgravity on the intestinal flora.
Gut microbiota derived-metabolites play critical roles in maintaining health (Wang and Zhao, 2018). After 21 days of simulated weightlessness, the intestinal metabolic profile in rats is significantly altered, mainly participating in pyrimidine metabolism and pentose and glucuronate interconversions metabolism (Jin et al., 2019). This project also indicated that the differential metabolites were associated with the changes of the intestinal microbiota and disruption of immunological characteristics (Jin et al., 2019). The interaction between host physiology and microbial metabolites is complex and the nonconformity is attributed to microbial, environmental and host sources (Krautkramer et al., 2021). Therefore, short-term microgravity probably disturbs the intestinal flora, further leading to alteration of the metabolites. As the remarkably increased space missions and the essential role of gut homeostasis in astronaut physiology, it is necessary to reveal the influence of long-term weightlessness on microbiota and metabolites. However, studies focused on the adverse effects of long-term microgravity on intestinal microbiota, metabolome, and their associations are limited. Here, we established a hindlimb unloading mouse model of microgravity and performed multi-omics analysis of 16S rRNA sequencing and untargeted metabonomic technology to access the impact of 8 weeks of SMG on the intestinal microbiota, metabolites, and participating pathways. Then, we further analyze and their associations in detail. Our work will provide a better understanding of the impact of long-term microgravity on intestinal homeostasis, which provides clues for further revealing the mechanisms of long-term SMG involved diseases.
Materials and methods
Animals and SMG model construction
Sixteen C57BL/6 mice (female, 6–8 weeks) of SPF grade were purchased from SiPeiFu Biotechnology Co. Ltd., Beijing, China. All mice were kept in separate cages in a temperature-controlled environment. The light–dark cycle was 12/12 h, and they had free access to food and water. After 1 week of adaptive feeding, mice were randomly divided into two groups, the control group (Control) and the simulated microgravity group (SMG), each group contained eight mice. Hindlimb suspension of mice by the tail model was followed to create a ground-based model of microgravity (Globus and Morey-Holton, 2016). The mice were suspended by tail with hindlimbs suspended at 15–30° horizontally, ensuring that the hindlimbs do not touch the cage floor. Mice in the control group were raised in identical cages without hindlimb unloading. Thirty-six fecal samples from 6 mice of each group were collected at the end of week 2, 4, and 8, and stored at −80°C until used for 16S rRNA gene sequencing. Mice were sacrificed at the end of week 8. Then, cecal contents from each mouse were obtained and saved at −80°C until used for metabolic analysis. All efforts were made to minimize the discomfort of the animals. The whole experimental design is shown in revised Supplementary Figure S1.
DNA extraction and 16S rRNA gene sequencing
Total bacteria DNA was extracted from approximately 220 mg of stool samples using the CTAB method following the standard protocol and then sequencing libraries were created. Briefly, the V3-V4 region of the 16S rRNA genes was amplified using specific primer (341F, 5ˊ-CCTAYGGGRBGCASCAG-3ˊ, 806R, 5ˊ-GGACTACNNGGGTAT CTAAT-3ˊ) with the barcode. The sequencing libraries were generated using TruSeq® DNA PCR-Free Sample Preparation Kit (Illumina, USA) according to the manufacturer’s instructions, and index codes were added. The library quality was assessed on the Qubit@ 2.0 Fluorometer (Thermo Scientific). Finally, the library was sequenced on an Illumina NovaSeq platform and 250 bp paired-end reads were generated.
Sequence analysis
The analysis was conducted by following the “Atacama soil microbiome tutorial” of Qiime2 along with customized program scripts.1 Briefly, raw data FASTQ files were imported into the format which could be operated by the QIIME2 system. Demultiplexed sequences were quality filtered, trimmed, de-noised, and merged. Then the chimeric sequences were identified and removed using the QIIME2 dada2 plugin to obtain the amplicon sequence variant feature table (ASV) (Callahan et al., 2016). To generate the taxonomy table, the QIIME2 feature-classifier plugin was then used to align ASV sequences to a pre-trained GREENGENES 13_8 99% database (Bokulich et al., 2018). Kruskal–Wallis and LEfSe were employed to identify the bacteria with different abundance among samples and groups. Alpha diversity indices were calculated using qiime2 diversity, with the observed OTU to calculate species richness and Shannon index to calculate species diversity. Beta diversity distance measurements were performed to investigate the structural variation of microbial communities across samples and then visualized via nonmetric multidimensional scaling (NMDS). Co-occurrence analysis was performed by calculating Spearman’s rank correlations between predominant taxa and the network plot was used to display the associations among taxa.
Metabolomics profiling
Fecal samples (100 mg) were individually grinded with liquid nitrogen and the homogenates were resuspended with prechilled 80% methanol by vigorous vortexing. The samples were incubated on ice for 5 min and then centrifuged at 15,000 g for 20 min at 4°C. Some of the supernatant was diluted to a final concentration of 53% methanol by LC–MS grade water. The samples were transferred to a new Eppendorf tubes and centrifuged at 15,000 g, 4°C for 20 min. Finally, the supernatant was injected into the LC–MS/MS system for analysis (Want et al., 2013).
UHPLC–MS/MS analysis was performed using a Vanquish UHPLC system (Thermo Fisher, Germany) coupled with an Orbitrap Q ExactiveTM HF mass spectrometer (Thermo Fisher, Germany) in Novogene Co., Ltd. (Beijing, China). Samples were injected onto a Hypesil Gold column (100 × 2.1 mm, 1.9 μm) using a 17-min linear gradient at a flow rate of 0.2 ml/min. The eluents for the positive polarity mode were eluent A (0.1% FA in Water) and eluent B (Methanol).The eluents for the negative polarity mode were eluent A (5 mM ammonium acetate, pH 9.0) and eluent B (Methanol). Q ExactiveTM HF mass spectrometer was operated in positive/negative polarity mode with spray voltage of 3.5 kV.
Metabolomic data processing and analysis
The raw data files generated by UHPLC–MS/MS were processed using the Compound Discoverer 3.1 (CD3.1, Thermo Fisher) to perform peak alignment, peak picking, and quantitation for each metabolite. The normalized data were used to predict the molecular formula based on additive ions, molecular ion peaks, and fragment ions. And then peaks were matched with the mzCloud,2 mzVaultand MassListdatabase to obtain the accurate qualitative and relative quantitative results. Statistical analyses were performed using the statistical software (Rohart et al., 2017). When data were not normally distributed, normal transformations were attempted by using area normalization method. Random Forest machine learning analysis was constructed through tools of bioincloud.tech and Mean Decrease Accuracy is used to measure the importance of metabolites in distinguishing groups in random forests. These metabolites were annotated using the KEGG database, HMDB database, and LIPIDMaps database. Furthermore, MetOrigin database3 was used to discriminate the origins of identified metabolites.
Statistical analysis
Statistical analysis of the results was performed by using the statistical software R (R version R-3.4.3) and GraphPad Prism 7.0 software. Statistical significance between SMG and control groups was evaluated by Mann–Whitney test and Kruskal–Wallis test. Differences between the groups were considered statistically significant at the 5% level (p < 0.05). All p -values for the statistical tests of metabolite variations in the two groups were corrected for multiple testing using Benjamini-Hochberg false discovery rates (FDR) method. Significant differences were indicated by *p < 0.05, **p < 0.01, ***p < 0.001. Correlations between the relative abundance of differential bacteria (p < 0.05, average relative adundance˃0.01%) and the concentration of major metabolites (FDR < 0.05) involving in major differential metabolic pathways were computed with Spearman’s test using Oebiotech tools4 and MetaboAnalyst 5.0.5
Results
Dynamic changes in community composition under the long-term simulated microgravity
We performed the V3-V4 region of the 16S rRNA gene sequencing to assess whether the long-term SMG altered the intestinal flora at different time points after 8 weeks of SMG. The 36 fecal samples yielded a total of 3,070,422 raw reads corresponding to 7,719 OTUs. The data were normalized by established a depth of 63,210 reads per sample., Alpha diversity analysis showed there were no significant differences in the indices of observed OTU and Shannon index (p > 0.05, Figure 1A) compared to the control mice at weeks 2, 4 and 8. These results suggested that SMG did not affect the richness and diversity of gut microbiota during the 8 weeks of SMG. Beta diversity reveals that SMG had a statistically significant effect on community composition and structure at weeks 2, 4, and 8 (p < 0.01, Figures 1B,C). Non-metric multidimensional scaling (NMDS) based on the Bray–Curtis distance revealed a significant differences in microbial structure between the control group and the SMG group (p < 0.01, Figure 1B). Better separation of SMG and control mice was observed at the genus level throughout the 8 weeks of SMG (Figure 1C). In addition, the co-network analysis showed that the microbial associations differed between the SMG and the control groups at different time points (Supplementary Figure S3). For example, the relative abundance of Akkermansia is positively related to the beneficial bacteria Lactobacillus and negatively associated with Bacillus in the control mice at week 2. However, the relative abundance of Akkermansia is negatively relative with Helicobacter in SMG mice. Moreover, the difference between the two groups seemed more apparent in week 8.
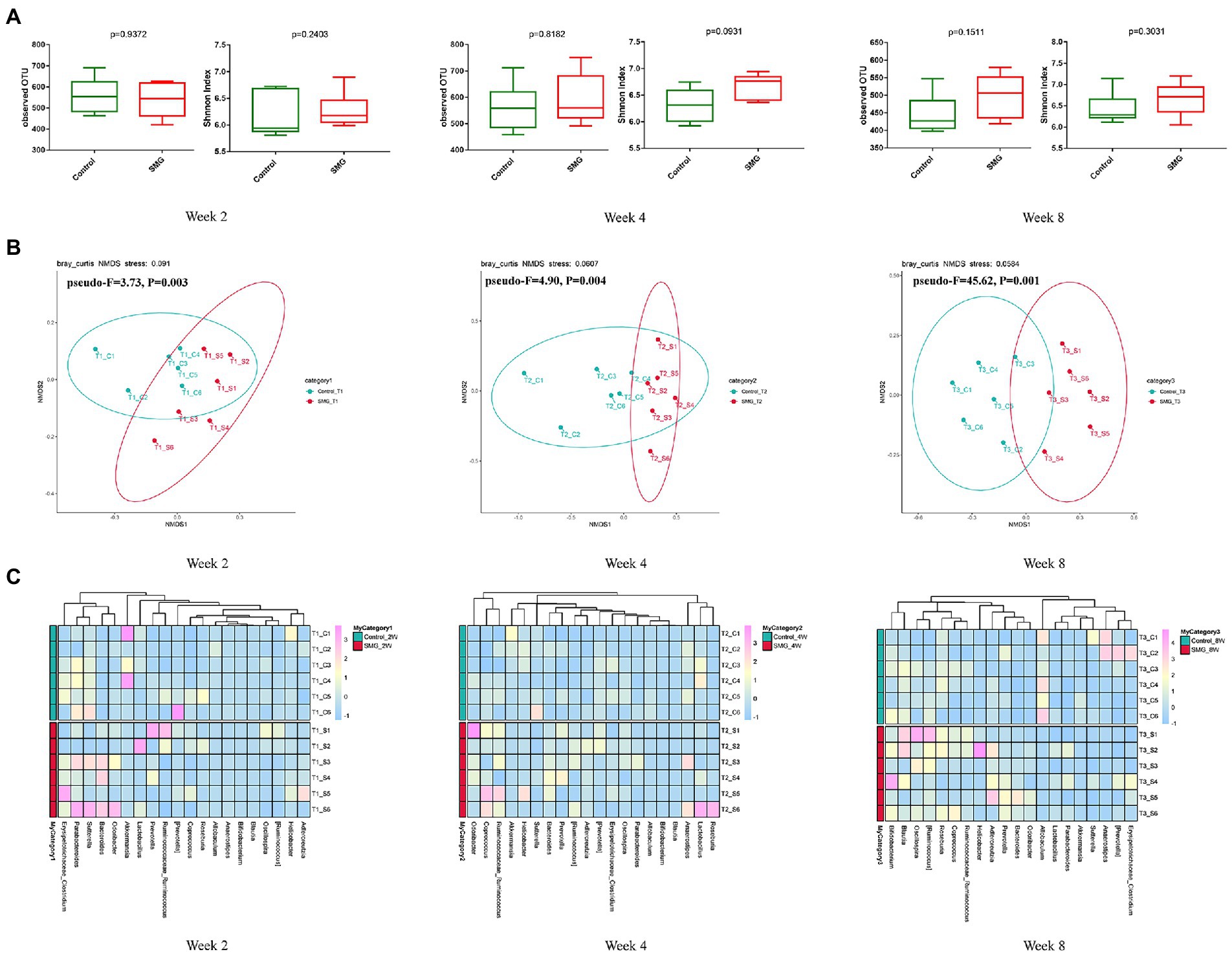
Figure 1. Bacterial diversity analysis of the gut microbiota in two groups during SMG treatment. (A) Alpha diversity metrics (observed OTU and Shannon indices) with respect to SMG and control at week 2, 4 and 8. (B) Beta diversity of the gut microbiota in two groups at week 2, 4 and 8. (A) The NMDS plot of gut microbiota from the control and SMG group at three time point intervals. (C) Heatmap of the relative abundance of top 20 bacterial genera at three time points.
The most affected bacterial populations at different time points
We also performed multivariate analysis to identify differential abundant taxa in SMG mice as compared to the control group at three-time points. At phylum level, fecal microbiota in both groups dominated by bacterial phyla Firmicutes, Bacteroidetes, and Proteobacteria (Figure 2A). The proportions of Bacteroidetes and Firmicutes significantly declined in the control group at week 8 (Supplementary Figure S2B). However, there were no significantly differences in abundance of Bacteroidetes and Firmicutes in the SMG groups (Supplementary Figure S2C). These results imply that long-term SMG may lead to spices belonging to Bacteroidetes prone to growth. SMG significantly decreased the relative abundance of Verrucomicrobia at a different time points, and Actinobacteria and Deferribacteres increased significantly at week 8 (Figure 2B). At the genus level, we observed that SMG showed a significant decrease in the relative abundance of Allobaculum and increased Bacteroides throughout the 8 weeks of SMG (Figures 2B,C, 3). At the species level, Desulfovibrio_c21_c20, Akkermansia_muciniphila, and Ruminococcus_flavefaciens were decreased considerably in SMG mice at week 2. At week 4, only Akkermansia_muciniphila were significantly decreased. At week 8, Bacteroides_uniformis and Faecalibacterium_prausnitzii were declined, while Ruminococcus_gnavus and Mucispirillum_schaedleri were significantly increased (Figures 2D). By performing LEFSe at the genus level, we identified two taxa (Bacteroides and Gracilibacter) characteristic of the SMG mice at week 2 and 8, while taxa such as Akkermansia and Allobaculum were enriched in the control group (Figure 3A). At week 4, we identified five taxa (Bacteroides, Odoribacter, Sporosarcina, Gracilibacter, and Clostridium) enriched in the SMG group (Figure 3B). Nine taxa were identified in SMG group at week 8, including Bacteroides, Prevotella, Odoribacter, Rothia, Mucispirillum, Anaerotruncus, Clostridium, Adlercreutzia, and Bilophila (Figure 3C). Together, these results suggested that Bacteroides, Allobaculum, and Akkermansia were the most affected genera throughout the simulated microgravity process. In line with previous studies (Wang et al., 2020; Sun et al., 2021), SMG could significantly reduce the relative abundance of beneficial bacteria, such as Akkermansia and Allobaculum. Our findings confirm that SMG induces a marked disturbance in the intestinal flora at different times, and longer SMG has a greater effect.
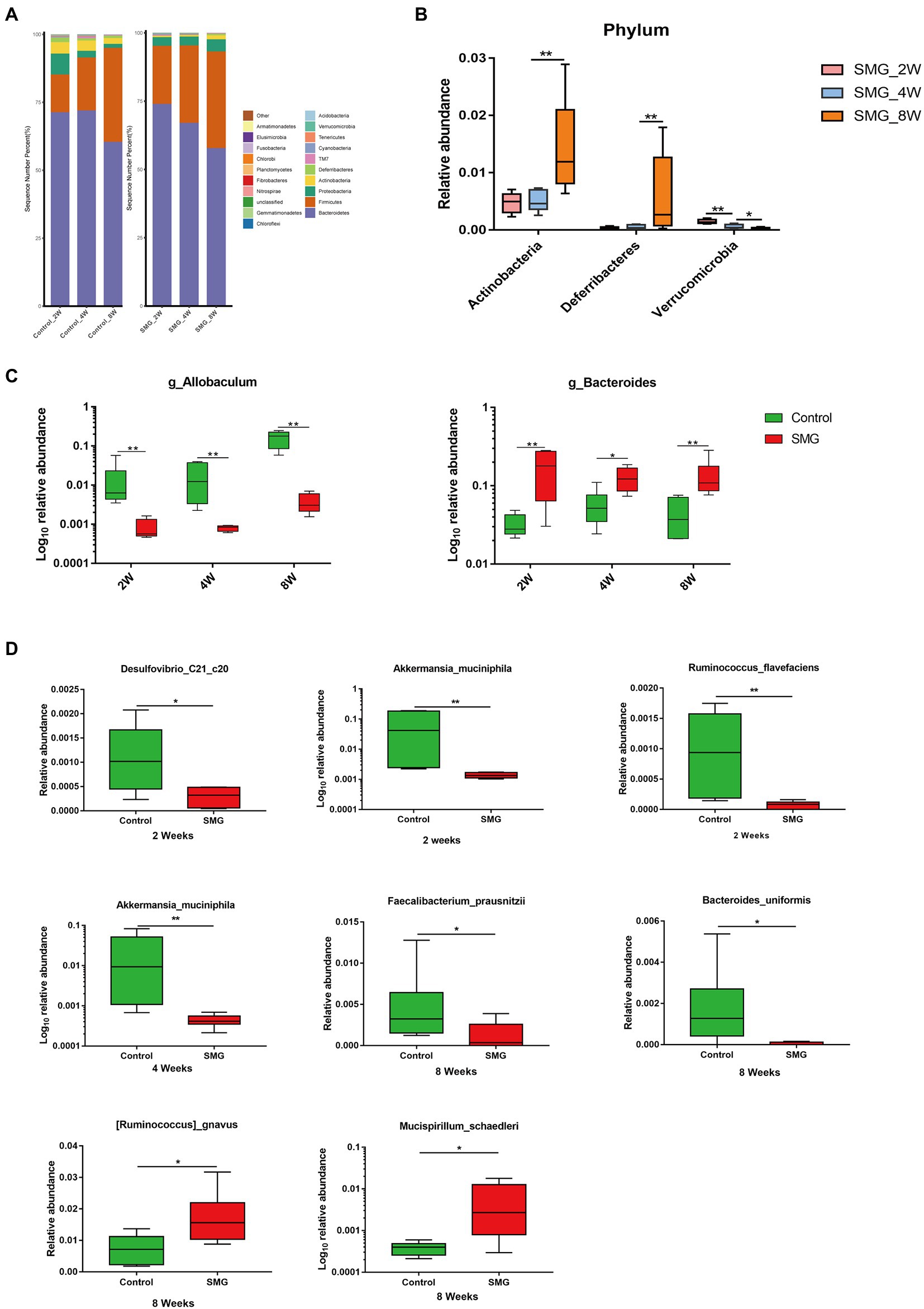
Figure 2. Taxonomic characterization of the gut microbiota in SMG mice at week 2, 4 and 8. (A) The column chart of the relative abundances of species in two groups the at phylum level. Differential bacteria in feces at phylum (B), genus (C), and species level (D). *p < 0.05, **p < 0.01.
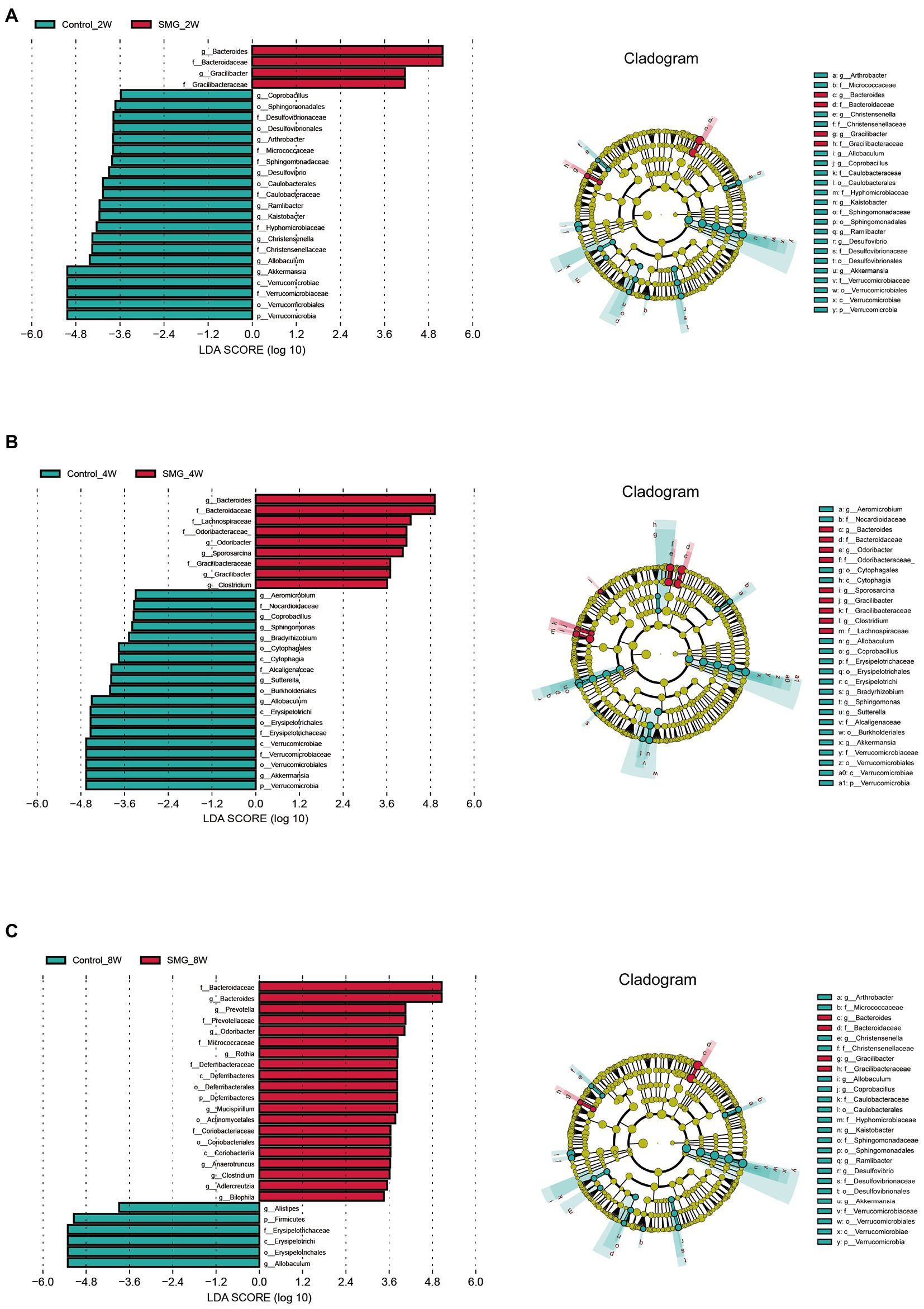
Figure 3. Microbial biomarkers associated with the SMG at week 2 (A), 4 (B), 8 (C). Linear discriminant analysis (LDA) effect size (LEfSe) methods were used to identify the most deferentially abundant taxa between two groups at three time point. The cladogram of bacteria with larger effect size in the SMG group is shown in red, and bacteria with larger effect size in the control group are shown in green.
Long-term SMG changed the intestinal metabolism and metabolic pathways
SMG treatment significantly altered the intestinal microbiota, raising our interest in investigating whether and how it could affect microbial metabolites, which could modulate circulating metabolites. We evaluated the intestinal metabolomics profiles from 15 samples of intestinal contents after 8 weeks of SMG. PCA and PLS-DA scores plots revealed clear significant differences in the composition of intestinal microbiota between the SMG and control groups (Figures 4A,B). A total of 1,193 metabolic features were identified across 15 samples. The volcano plots showed 275 features (23.05%) significantly changed in SMG (p<0.05) mice. Among them, 129 metabolites were upregulated and 146 downregulated (Figure 4C). On the super class level, the relative abundance of organic acids, peptide and carbohydrate significantly decreased after 8 weeks of SMG (Supplementary Table S1).Overall, these data indicate that long-term SMG significantly alter the intestinal metabolites, which may mainly contribute to disturbance in circulatory metabolism. We then used Random Forest machine learning analysis to determine the most discriminatory metabolites from SMG and control groups. The top 30 different metabolites including but not limited to 19(R)-Hydroxy-prostaglandin E2, DL-Tryptophan, 2,6-Dihydroxybenzoic acid, Tetrahydroaldosterone, Suberic acid, N-Carbamyl-L-glutamic acid, 5-Hydroxyindole, Prostaglandin D3, and 11-keto Testosterone (CRM), which were primarily from the amino acid, lipid, and organic acids (Figure 4D).
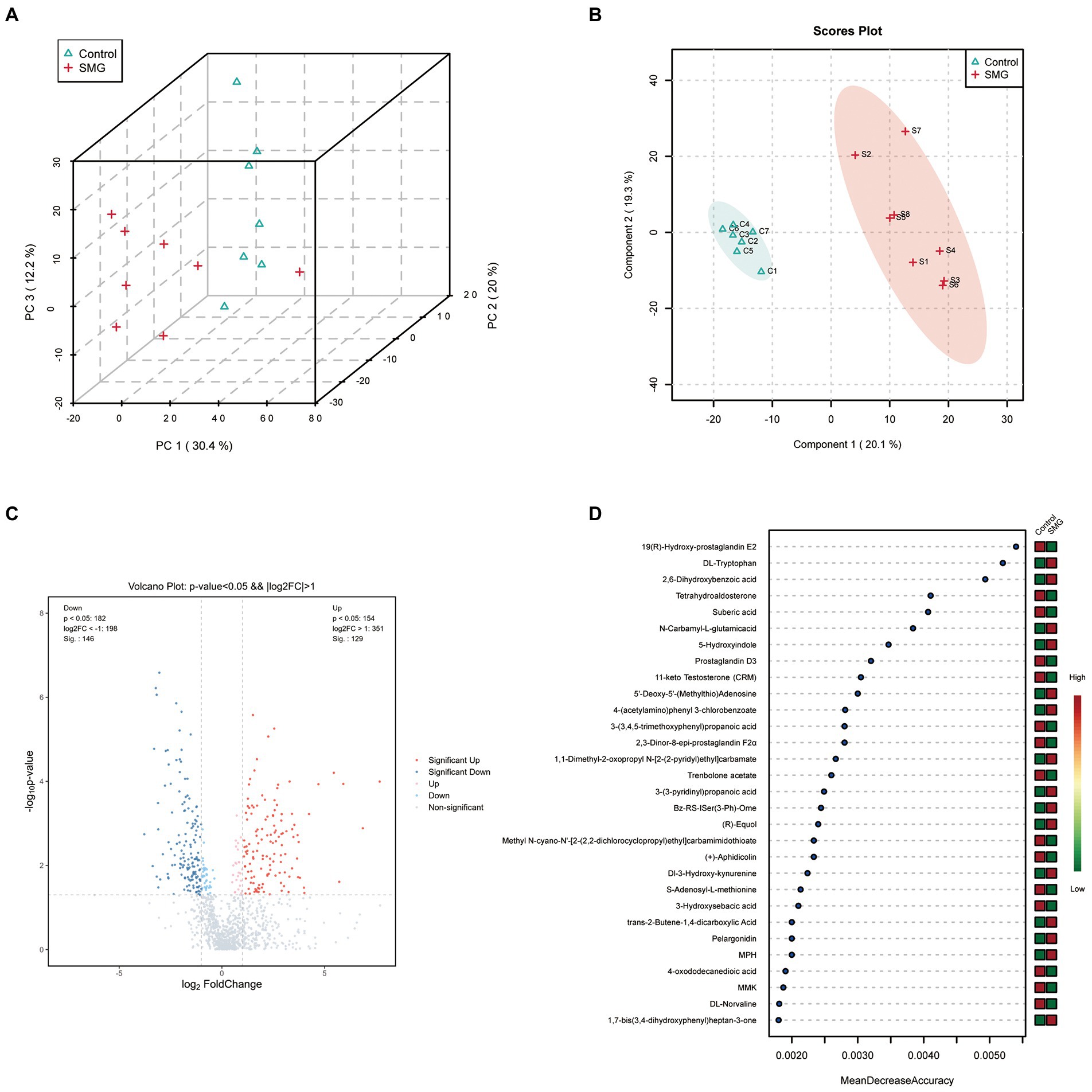
Figure 4. Long-term SMG alters intestinal metabolome profile. (A) PCA scores plots of intestinal metabolites based on the Bray–Curtis distance between SMG and control groups. (B) PLS-DA scores plots of intestinal metabolites between two groups. (C) Volcano plot of the differential metabolites between SMG and control groups. Each dot represents a specific metabolite. The red point means up-regulation, blue point means downregulation. (D) The top 30 named metabolites identified in random forests. The higher value of mean decrease accuracy, the higher the importance of the variable.
Further, to investigate the potential role of SMG-related metabolites, we annotated metabolic features by using the Kyoto Encyclopedia of Genes and Genomes (KEGG) database. The SMG mice exhibited distinct patterns of metabolites involved in 36 metabolic pathways, including tryptophan metabolism, Tyrosine metabolism, alanine, aspartate and glutamate metabolism, and caffeine metabolism (Supplementary Table S2). Metabolites that were increased in abundance in SMG reflected pathways mainly involved in tryptophan metabolism (L-tryptophan, 2-ketoadipic acid, and 5-hydroxytryptophan), histidine metabolism (carnosine, histamine and 1-methylhistidine), cysteine and methionine metabolism (S-adenosylmethionine, L-cystathionine and 5′-deoxy-5′(methylthio) adenosine), and arginine and proline metabolism (S-adenosylmethionine, oxaceprol, and 4-acetamidobutyric acid) (p < 0.05) (Figures 5A,C). Metabolites whose abundance was decreased in SMG reflected pathways involved in steroid hormone biosynthesis (etiocholanolone, hydrocortisone, and cortisone) (p < 0.05) (Figures 5B,C). Overall, these results revealed that long-term SMG significantly affects the intestinal metabolites and metabolic pathways.
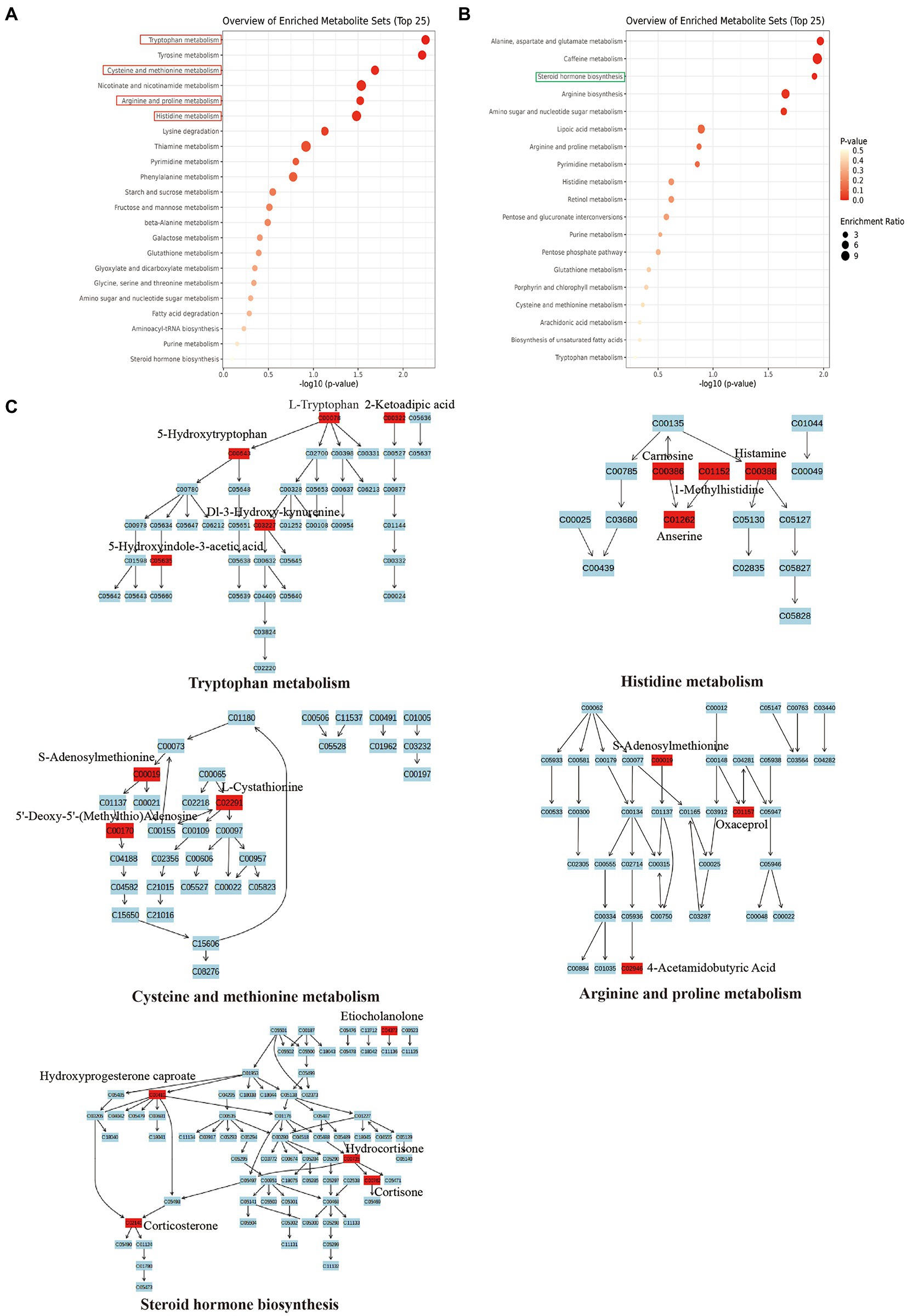
Figure 5. Major metabolic pathway involved in the differentially enriched metabolites of SMG mice compared to controls. (A) The major metabolic pathways involved in increased metabolites. (B) The major metabolic pathways involved in decreased metabolites. (C) Reaction steps for 5 major metabolic pathways both involved in increased and decreased metabolites.
Discriminate the origins of metabolites significantly changed
We further sought to determine the potential origin of the metabolites that were highly correlated with gut bacteria. Total of 106 co-metabolism metabolites, 36 microbiota metabolites, and 21 host metabolites were identified (Supplementary Figure S4A,B). We performed functional enrichment analysis of these metabolites and observed that these metabolites were mainly enriched in steroid hormone biosynthesis, histidine metabolism, tryptophan metabolism, tyrosine metabolism, Galactose metabolism, arginine biosynthesis, arginine and proline metabolism, beta-Alanine metabolism, and glutathione metabolism (Supplementary Figure S4C). We found that metabolites related to 9 pathways were originated from microbiota, including tryptophan metabolism, tyrosine metabolism, Galactose metabolism, arginine and proline metabolism, pyrimidine metabolism, folate biosynthesis, aminobenzoate degradation, and carotenoid biosynthesis. Interestingly, only the pathway of steroid hormone biosynthesis was identified to be related to host metabolism and 27 pathways involved in co-metabolism, such as histidine metabolism, tryptophan metabolism, tyrosine metabolism, arginine biosynthesis, arginine and proline metabolism, beta-alanine metabolism, and glutathione metabolism. Collectively, the differential metabolites we identified here were mostly produced or influenced by the gut microbiota. Our results suggested that the altered intestinal metabolism may have a strong correlation with the changed gut microbiota induced by SMG.
Associations of differential intestinal metabolites with the major bacterial genera
To further investigate the potential dependencies between the perturbed intestinal metabolites and gut microbiota, the Spearman’s correlation coefficient between differential metabolites and the abundance of differential gut bacteria was determined. Strong connections were identified between the perturbed intestinal metabolites and gut microbiota (Table 1). Our results showed that the level of several differential metabolites were significantly correlated with the relative abundance of biomarker microbial genera (ρ > 0.6, p < 0.05). Notably, Allobaculum, Akkermansia, Phascolarctobacterium, and Faecalibacterium who have been reported to produce SCFAs, exhibited a negative association with metabolites involved in tryptophan metabolism (5-hydroxyindole-3-acetic acid, 2-ketoadipic acid, Dl-3-hydroxy-kynurenine, L-tryptophan, and oxoadipic Acid), histidine metabolism (1-methylhistidine, carnosine, anserine, and histamine), arginine and proline metabolism (S-adenosylmethionine, oxaceprol, and 4-acetamidobutyric Acid), but positive with hydroxyprogesterone caproate and etiocholanolone involving in steroid hormone biosynthesis. Moreover, genera of Bacteroides, Lachnospiraceae_Clostridium, Rothia, Bilophila, and Coprococcus were positively correlated with arginine and proline metabolism (4-acetamidobutyric acid and oxaceprol), tryptophan metabolism (5-hydroxyindole-3-acetic acid), Dl-3-hydroxy-kynurenine, L-tryptophan), and histidine metabolism (anserine, carnosine, and histamine), but negatively associated with steroid hormone biosynthesis (etiocholanolone, hydroxyprogesterone caproate, and cortisol). The relative abundance of Alistipes showed significant positive correlation with histidine metabolism (urocanic acid) and steroid hormone biosynthesis (etiocholanolone and cortisol). Our results suggested that the alteration of intestinal metabolites and pathways under long-term of SMG have a strong association with the imbalance of gut microbiota.
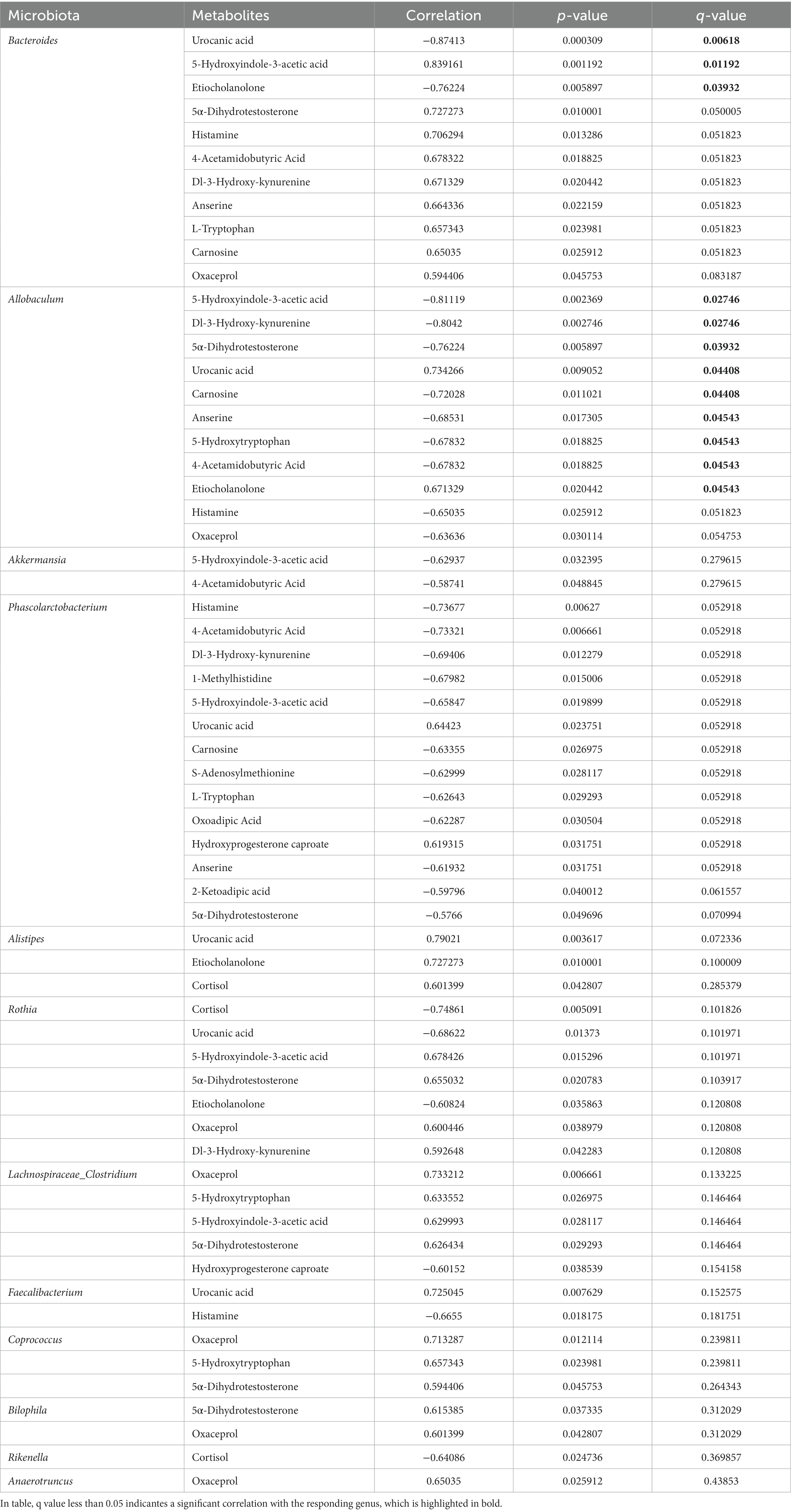
Table 1. The Spearman’s correlation significantly changed between intestinal differential metabolites and bacteria genera.
Discussion
Accumulating evidence showed that microgravity affects bone, muscle and the gut microbiota, yet the effect of long-term microgravity on intestinal microbiome and metabolism remains unclear. Hence, we dynamically compared the intestinal microbiome and metabolome between SMG and control mice during the period of eight weeks, and we found that SMG induced no significant difference in diversity and richness of intestinal flora, but altered the composition of the intestinal microbiome at week 2, 4 and 8. This observation was inconsistence with an earlier work, which reported that 3 weeks of SMG reduce the diversity of colonic microbiota (Wang et al., 2020). These results indicated that SMG have distinct effects on bacterial flora in different period of time. Meanwhile, the intestinal metabolites and metabolic pathways changed characteristically under long-term SMG. Together, the current study contributes to identifying microbes, metabolites, and metabolic pathways that are mostly affected by a long-term SMG, and may be associated with the pathogenesis of SMG-associated diseases.
In line with previous reports, this research suggested that SMG altered the structural composition of gut microbiota. A previous study found that 3 weeks of SMG increased the relative abundance of Proteobacteria (Wang et al., 2020). However, in our study, the phylum of Verrucomicrobia significantly declined from weeks 2 to 8, yet the relative abundance of Actinobacteria and Deferribacteres raised remarkably at week 8. These findings indicated that the influence of SMG on gut microbiota is different across different time intervals. Moreover, at the genus level, Allobaculum and Bacteroides showed the most remarkable discrepancy between SMG and control groups during the whole 8 weeks period. Allobaculum is a kind of glucose utilizers and producers of lactate and butyrate, and its decline has been reported to be correlated with cognitive impairments, aging, high-fat diets, inflammatory disease and fatty acid metabolism (Bárcena et al., 2019; Pujo et al., 2021; Hu et al., 2022; Wang et al., 2022). While, the genus Bacteroides considered as friendly commensal bacteria, overgrowth of which may produce different disease conditions by competed nutrition with other gut commensals or transfer virulence genes, was enriched in the SMG group (Zafar and Saier, 2021). Further, Cambell et al. have reported increased levels of Bacteroides spp. in cancer patients (Parida and Sharma, 2019). In another study, they have reported that Bacteroides spp. may trigger autoimmunity accompanied by cardiomyopathy (Gil-Cruz et al., 2019). Hence, this study identifies Allobaculum and Bacteroides as biomarker bacteria mostly affected by longer-term microgravity in a simulated microgravity mouse model.
We also found that SMG continuously decreased several beneficial bacterial species such as Bacteroides_uniformis, Akkermansia_muciniphila, and Faecalibacterium_prausnitzii at different time points. A previous study reported that supplementation with Bacteroides_uniformis could improve ASD-like behaviors by decreasing serum glutamine levels and intestinal amino acid transport (Yu et al., 2022). In addition, Akkermansia_muciniphila is a well-known mucin-degrading bacterium, playing a critical role in the thickening of mucus layers and intestinal barrier improvement. Loss of Akkermansia_muciniphila correlated with inflammation, impairment of insulin secretion and glucose homeostasis, metabolic disease, cancer immunotherapy, and homeostatic immunity (Yoon et al., 2021; Zhang et al., 2021; Bae et al., 2022; Schneider et al., 2022). Faecalibacterium_prausnitzii, an important butyrate-producer of the phylum of Firmicutes, has been suggested as a biosensor and a major player of the intestinal health. The reduction of Faecalibacterium_prausnitzii has been reported to be associated with inflammation, metabolic disease and type-2 diabetes (Verhoog et al., 2019). Interestingly, two potential pathogenic bacterial species Ruminococcus gnavus and Mucispirillum schaedleri were increased significantly at week 8 of SMG. Multiple studies associate the mucin-glycan foraging Ruminococcus gnavus with Crohn’s disease, IBD, allergic disease and neurological disorder (Hall et al., 2017; Chua et al., 2018; Henke et al., 2019; Coletto et al., 2022). Recently, Mucispirillum schaedleri, belonging to the phylum Deferribacteres, was also linked to inflammatory conditions in the intestine (Herp et al., 2021). Taken together, our results verify the gut bacterial biomarkers dynamically caused by SMG, probably leading to microgravity-associated systemic imbalance.
Changes in the gut microbiota have been closely linked to intestinal and host metabolism. We firstly performed a comprehensive profiling of the fecal metabolites from control and SMG mice at week 8. Our results suggested that specific individual metabolites and metabolic pathways were significantly altered, involving in tryptophan metabolism, histidine metabolism, cysteine and methionine metabolism, arginine and proline metabolism, and steroid hormone biosynthesis, which show a strong association with different microbes. Next, we applied a MetOrigin database to distinguish origination of metabolites, and our results verified that the disturbed metabolites mostly resulted from co-metabolism or originated from microbiota. In the last few decades, role of the gut microbiota has been suggested in many diseases, such as IBD, metabolic syndrome and associated complications, and neuropsychiatric disorders which is partially mediated by impaired Trp metabolism (Agus et al., 2018). Previous literature depicts that gut microbiota altered proline metabolism in depression (Mayneris-Perxachs et al., 2022), implying its essential role in depression by regulating metabolism. Gut microbes also impacts intestinal motility through histamine secretion and activation of histamine receptors, and bacterial histidine decarboxylases are enriched in patients with Crohn’s disease (Chen et al., 2019). Histidine and arginine metabolism have been proven as potential immunomodulatory pathways mediated by Akkermansia muciniphila and Bifidobacterium longum (Stražar et al., 2021). In this study, Akkermansia muciniphila and some other specific bacteria showed a negative correlation with the metabolites involved in histidine, arginine and proline metabolism, indicating that long-term SMG-induced intestinal microbiota imbalance may affect host inflammatory response and neuropsychiatric disorders by regulating intestinal metabolism.
Gut microbiota dysbiosis affects steroid hormone metabolism contributing to several diseases, such as cancer development, hypertension, obesity, and neurological disorders (Daisley et al., 2020; Yan et al., 2020; Mayneris-Perxachs et al., 2020a; Li et al., 2022). Yan et.al showed that intestinal microbiota modulated blood pressure by affecting corticosterone level (Yan et al., 2020). Gut microbiota participate in steroid hormone biosynthesis and degradation. The gut microbiota of pre-menopausal women enriched in genes from the steroid biosynthesis and degradation, and women harbor microbial community type with lower abundance of Ruminococcaceae, Faecalibacterium, and Alistipes, but higher abundance of Bacteroides and Prevotella in another study (Ding and Schloss, 2014; Mayneris-Perxachs et al., 2020b). Here, our results demonstrated that intestinal metabolites involved in steroid hormone biosynthesis were significantly reduced by 8 weeks of SMG, and strongly associated with special microbes, such as Bacteroides, Alistipes, Allobaculum, Faecalibacterium, and Phascolarctobacterium. Collectively, these results indicated that long-term SMG-induced gut microbiota leads to intestinal metabolic disturbance directly or indirectly, which may affect host health. Furthermore, systematic research is required to explore the potential role of altered intestinal flora in microgravity-associated host dysfunction.
Conclusion
To the best of our knowledge, this is the first research of integrated intestinal microbiome and metabolomics in the simulated microgravity mice model under longer time of SMG. Different gut microbial biomarkers were verified at different time points of SMG. Allobaculum and Bacteroides were identified as biomarkers for the whole process of SMG. Bacteroides_uniformis, Faecalibacterium_prausnitzii, Ruminococcus_flavefaciens, and Mucispirillum_schaedleri were most affected species under 8 weeks of SMG. This long-term SMG-induced intestinal dysbiosis has a strong association with differential gut metabolites participating in metabolic pathways including tryptophan metabolism, histidine metabolism, arginine and proline metabolism, and steroid hormone biosynthesis. Our results indicated that long-term SMG has a more significant impact on intestinal microbiota, which further influence the intestinal metabolism. Our work will provide a better understanding of the long-term microgravity impact on intestinal homeostasis, and urges further deep explorations of the relationships between gut microbial dysbiosis, metabolism and microgravity-induced host dysfunction.
Data availability statement
The datasets presented in this study can be found in online repositories. The names of the repository/repositories and accession number(s) can be found below: MTBLS6526 PRJNA905934.
Ethics statement
The animal study was reviewed and approved by the Institutional Experimental Animal Care and Use Committee of the PLA Strategic Support Force Medical Center.
Author contributions
LY and RZ designed the research and drafted the manuscript. XL and SS co-supervised the project and revised the manuscript. LY, RZ, XM, LZ, QP, and MW performed the experiments. LX, CG, and XH helped analyzing the data. SH revised the manuscript. All authors read and approved the final manuscript.
Funding
This work was supported by the scientific research fund project for doctoral graduates by the PLA Strategic Support Force Medical Center (21ZXO8).
Acknowledgments
We thank the staff at the Wekemo Tech Group Co., Ltd. for sequencing service. We also thanks to all members of Si lab for sample collection and stuff preparation.
Conflict of interest
The authors declare that the research was conducted in the absence of any commercial or financial relationships that could be construed as a potential conflict of interest.
Publisher’s note
All claims expressed in this article are solely those of the authors and do not necessarily represent those of their affiliated organizations, or those of the publisher, the editors and the reviewers. Any product that may be evaluated in this article, or claim that may be made by its manufacturer, is not guaranteed or endorsed by the publisher.
Supplementary material
The Supplementary material for this article can be found online at: https://www.frontiersin.org/articles/10.3389/fmicb.2023.1100747/full#supplementary-material
Footnotes
1. ^https://docs.qiime2.org/2019.1/
3. ^http://metorigin.met-bioinformatics.cn/
References
Agus, A., Planchais, J., Sokol, H. J., and Microbe, C. H. (2018). Gut microbiota regulation of tryptophan metabolism in health and disease. Cell Host Microbe 23, 716–724. doi: 10.1016/j.chom.2018.05.003
Bae, M., Cassilly, C. D., Liu, X., Park, S. M., Tusi, B. K., Chen, X., et al. (2022). Akkermansia muciniphila phospholipid induces homeostatic immune responses. Nature 608, 168–173. doi: 10.1038/s41586-022-04985-7
Bárcena, C., Valdés-Mas, R., Mayoral, P., Garabaya, C., Durand, S., Rodríguez, F., et al. (2019). Healthspan and lifespan extension by fecal microbiota transplantation into progeroid mice. Nat Med 25, 1234–1242. doi: 10.1038/s41591-019-0504-5
Bokulich, N. A., Dillon, M. R., Bolyen, E., Kaehler, B. D., Huttley, G. A., and Caporals, J. G. (2018). Q2-sample-classifier: machine-learning tools for microbiome classification and regression. J Open Res Softw. 3:934. doi: 10.21105/joss.00934
Callahan, B. J., McMurdie, P. J., Rosen, M. J., Han, A. W., Johnson, A. J., and Holmes, S. P. (2016). Dada2: high-resolution sample inference from illumina amplicon data. Nat. Methods 13, 581–583. doi: 10.1038/nmeth.3869
Chelyshev, Y. A., Muhamedshina, Y. O., Povysheva, T. V., Shaymardanova, G. F., Rizvanov, A. A., Nigmetzyanova, M. V., et al. (2014). Characterization of spinal cord glial cells in a model of hindlimb unloading in mice. Neuroscience 280, 328–339. doi: 10.1016/j.neuroscience.2014.09.004
Chen, H., Nwe, P., Yang, Y., Rosen, C., Bielecka, A., Kuchroo, M., et al. (2019). A forward chemical genetic screen reveals gut microbiota metabolites that modulate host physiology 177, 1217–1231.e18. doi: 10.1016/j.cell.2019.03.036
Chen, P., Yu, Y.-b., Tan, C., Liu, H., Wu, F., Li, H., et al. (2016). Human metabolic responses to microgravity simulated in a 45-day 6° head-down tilt bed rest (hdbr). Exp. Dermatol. 8, 4334–4344. doi: 10.1039/C6AY00644B
Chua, H. H., Chou, H. C., Tung, Y. L., Chiang, B. L., Liao, C. C., Liu, H. H., et al. (2018). Intestinal dysbiosis featuring abundance of ruminococcus gnavus associates with allergic diseases in infants. Gastroenterology 154, 154–167. doi: 10.1053/j.gastro.2017.09.006
Coletto, E., Latousakis, D., Pontifex, M. G., Crost, E. H., Vaux, L., Perez Santamarina, E., et al. (2022). The role of the mucin-glycan foraging ruminococcus gnavus in the communication between the gut and the brain. Gut Microbes 14:2073784. doi: 10.1080/19490976.2022.2073784
Crucian, B., Babiak-Vazquez, A., Johnston, S., Pierson, D. L., Ott, C. M., and Sams, C. (2016). Incidence of clinical symptoms during long-duration orbital spaceflight. Int. J. Gen. Med 9, 383–391. doi: 10.2147/IJGM.S114188
Daisley, B., Chanyi, R., Abdur-Rashid, K., Al, K., Gibbons, S., Chmiel, J., et al. (2020). Abiraterone acetate preferentially enriches for the gut commensal akkermansia muciniphila in castrate-resistant prostate cancer patients 11, 4822. doi: 10.1038/s41467-020-18649-5,
Ding, T., and Schloss, P. D. (2014). Dynamics and associations of microbial community types across the human body. Nature 509, 357–360. doi: 10.1038/nature13178
Gil-Cruz, C., Perez-Shibayama, C., De Martin, A., Ronchi, F., van der Borght, K., Niederer, R., et al. (2019). Microbiota-derived peptide mimics drive lethal inflammatory cardiomyopathy. Science (New York, N.Y.) 366, 881–886. doi: 10.1126/science.aav3487
Globus, R. K., and Morey-Holton, E. (2016). Hindlimb unloading: rodent analog for microgravity. J. Appl. Physiol. 120, 1196–1206. doi: 10.1152/japplphysiol.00997.2015
Hall, A. B., Yassour, M., Sauk, J., Garner, A., Jiang, X., Arthur, T., et al. (2017). A novel ruminococcus gnavus clade enriched in inflammatory bowel disease patients. Genome Med. 9:103. doi: 10.1186/s13073-017-0490-5
Henke, M. T., Kenny, D. J., Cassilly, C. D., Vlamakis, H., Xavier, R. J., and Clardy, J. (2019). Ruminococcus gnavus, a member of the human gut microbiome associated with crohn's disease, produces an inflammatory polysaccharide. Proc. Natl. Acad. Sci. U. S. A. 116, 12672–12677. doi: 10.1073/pnas.1904099116
Herp, S., Durai Raj, A. C., Salvado Silva, M., Woelfel, S., and Stecher, B. (2021). The human symbiont mucispirillum schaedleri: causality in health and disease. Med. Microbiol. Immunol. 210, 173–179. doi: 10.1007/s00430-021-00702-9
Hu, Y., Xu, J., Sheng, Y., Liu, J., Li, H., Guo, M., et al. (2022). Pleurotus ostreatus ameliorates obesity by modulating the gut microbiota in obese mice induced by high-fat diet. Nutrients. 14:1868. doi: 10.3390/nu14091868
Jin, M., Wang, J., Zhang, H., Zhou, H., and Zhao, K. (2019). Simulated weightlessness perturbs the intestinal metabolomic profile of rats. Front. Physiol. 10:1279. doi: 10.3389/fphys.2019.01279
Krautkramer, K. A., Fan, J., and Bäckhed, F. (2021). Gut microbial metabolites as multi-kingdom intermediates. Nat. Rev. Microbiol. 19, 77–94. doi: 10.1038/s41579-020-0438-4
Kulikova, E. A., Kulikov, V. A., Sinyakova, N. A., Kulikov, A. V., and Popova, N. K. (2017). The effect of long-term hindlimb unloading on the expression of risk neurogenes encoding elements of serotonin-, dopaminergic systems and apoptosis; comparison with the effect of actual spaceflight on mouse brain. Neurosci. Lett. 640, 88–92. doi: 10.1016/j.neulet.2017.01.023
LaPelusa, M., Donoviel, D., Branzini, S. E., Carlson, P. E. Jr., Culler, S., Cheema, A. K., et al. (2021). Microbiome for mars: surveying microbiome connections to healthcare with implications for long-duration human spaceflight, virtual workshop, july 13, 2020. Microbiome 9:2. doi: 10.1186/s40168-020-00951-5
Lee, A. G., Mader, T. H., Gibson, C. R., Tarver, W., Rabiei, P., Riascos, R. F., et al. (2020). Spaceflight associated neuro-ocular syndrome (sans) and the neuro-ophthalmologic effects of microgravity: a review and an update. NPJ Microgravity 6:7. doi: 10.1038/s41526-020-0097-9
Li, D., Liu, R., Wang, M., Peng, R., Fu, S., Fu, A., et al. (2022). 3β-hydroxysteroid dehydrogenase expressed by gut microbes degrades testosterone and is linked to depression in males 30, 329–339.e5. doi: 10.1016/j.chom.2022.01.001,
Lim, G. J. C. (2022). Changes to the gut microbiota drive the progression of cardiometabolic disease. Nat Rev Cardiol. 19:283. doi: 10.1038/s41569-022-00686-w
Liu, Z., Luo, G., Du, R., Sun, W., Li, J., Lan, H., et al. (2020). Effects of spaceflight on the composition and function of the human gut microbiota. Gut Microbes 11, 807–819. doi: 10.1080/19490976.2019.1710091
Marzuca-Nassr, G. N., Vitzel, K. F., Murata, G. M., Márquez, J. L., and Curi, R. (2019). Experimental model of hindlimb suspension-induced skeletal muscle atrophy in rodents. Methods Mol. Biol. 1916, 167–176. doi: 10.1007/978-1-4939-8994-2_16
Mayneris-Perxachs, J., Arnoriaga-Rodríguez, M., Luque-Córdoba, D., Priego-Capote, F., Pérez-Brocal, V., Moya, A., et al. (2020a). Changes to the gut microbiota drive the progression of cardiometabolic diseaseChanges to the gut microbiota drive the progression of cardiometabolic disease, Gut microbiota steroid sexual dimorphism and its impact on gonadal steroids: influences of obesity and menopausal status 8, 136. doi: 10.1186/s40168-020-00913-x,
Mayneris-Perxachs, J., Arnoriaga-Rodríguez, M., Luque-Córdoba, D., Priego-Capote, F., Pérez-Brocal, V., Moya, A., et al. (2020b). Gut microbiota steroid sexual dimorphism and its impact on gonadal steroids: influences of obesity and menopausal status. Microbiome 8:136. doi: 10.1186/s40168-020-00913-x
Mayneris-Perxachs, J., Castells-Nobau, A., Arnoriaga-Rodríguez, M., Martin, M., de la Vega-Correa, L., Burokas, A., et al. (2022). Microbiota alterations in proline metabolism impact depression 34, 681–701.e10. doi: 10.1016/j.cmet.2022.04.001,
Paik, D., Yao, L., Zhang, Y., Bae, S., D'Agostino, G., Zhang, M., et al. (2022). Human gut bacteria produce τ17-modulating bile acid metabolites. Nature 603, 907–912. doi: 10.1038/s41586-022-04480-z
Parida, S., and Sharma, D. (2019). The microbiome-estrogen connection and breast cancer risk. Cells 8:1642. doi: 10.3390/cells8121642
Park, E., Chelvanambi, M., Bhutiani, N., Kroemer, G., and Zitvogel, L. (2022). Wargo JJNm. Target. grant tUmor microb cancer. 28, 690–703. doi: 10.1038/s41591-022-01779-2
Pujo, J., Petitfils, C., Le Faouder, P., Eeckhaut, V., Payros, G., Maurel, S., et al. (2021). Bacteria-derived long chain fatty acid exhibits anti-inflammatory properties in colitis. Gut 70, 1088–1097. doi: 10.1136/gutjnl-2020-321173
Rohart, F., Gautier, B., Singh, A., and Ka, L. C. (2017). MixomicCs: an r package for 'omics feature selection and multiple data integration. PLoS Comput. Biol. 13:e1005752
SantaCruz-Calvo, S., Bharath, L., Pugh, G., SantaCruz-Calvo, L., Lenin, R. R., Lutshumba, J., et al. (2022). Adaptive immune cells shape obesity-associated type 2 diabetes mellitus and less prominent comorbidities. Nat. Rev. Endocrinol. 18, 23–42. doi: 10.1038/s41574-021-00575-1
Schneider, K. M., Mohs, A., Gui, W., Galvez, E. J. C., Candels, L. S., Hoenicke, L., et al. (2022). Imbalanced gut microbiota fuels hepatocellular carcinoma development by shaping the hepatic inflammatory microenvironment. Nat. Commun. 13:3964. doi: 10.1038/s41467-022-31312-5
Sorboni, S., Moghaddam, H., Jafarzadeh-Esfehani, R., and Soleimanpour, S. J. C. (2022). A comprehensive review on the role of the gut microbiome in human neurological disorders. Clin. Microbiol. Rev. 35:e0033820. doi: 10.1128/CMR.00338-20
Stražar, M., Temba, G., Vlamakis, H., Kullaya, V., Lyamuya, F., Mmbaga, B., et al. (2021). Gut microbiome-mediated metabolism effects on immunity in rural and urban african populations. Nat Commun 12:4845. doi: 10.1038/s41467-021-25213-2
Sun, P., Yang, J., Wang, B., Ma, H., Zhang, Y., Guo, J., et al. (2021). The effects of combined environmental factors on the intestinal flora of mice based on ground simulation experiments. Sci. Rep. 11:11373. doi: 10.1038/s41598-021-91077-7
Turroni, S., Rampelli, S., Biagi, E., Consolandi, C., Severgnini, M., Peano, C., et al. (2017). Temporal dynamics of the gut microbiota in people sharing a confined environment, a 520-day ground-based space simulation, mars500. Microbiome 5:39. doi: 10.1186/s40168-017-0256-8
Van Ombergen, A., Demertzi, A., Tomilovskaya, E., Jeurissen, B., Sijbers, J., Kozlovskaya, I. B., et al. (2017). The effect of spaceflight and microgravity on the human brain. J. Neurol. 264, 18–22. doi: 10.1007/s00415-017-8427-x
Verhoog, S., Taneri, P. E., Roa Díaz, Z. M., Marques-Vidal, P., Troup, J. P., et al. (2019). Dietary factors and modulation of bacteria strains of akkermansia muciniphila and faecalibacterium prausnitzii: a systematic review. Nutrients 11:1565. doi: 10.3390/nu11071565
Wang, M., Amakye, W., Gong, C., Ren, Z., Yuan, E., JJFrb, R., et al. (2022). Effect of oral and intraperitoneal administration of walnut-derived pentapeptide pw5 on cognitive impairments in app/ps1 mice. Free Radic. Biol. Med. 180, 191–197. doi: 10.1016/j.freeradbiomed.2022.01.003
Wang, J., Han, C., Lu, Z., Ge, P., Cui, Y., Zhao, D., et al. (2020). Simulated microgravity suppresses mapk pathway-mediated innate immune response to bacterial infection and induces gut microbiota dysbiosis. FASEB J. 34, 14631–14644. doi: 10.1096/fj.202001428R
Wang, Z., and Zhao, Y. (2018). Gut microbiota derived metabolites in cardiovascular health and disease. Protein Cell 9, 416–431. doi: 10.1007/s13238-018-0549-0
Want, E. J., Masson, P., Michopoulos, F., Wilson, I. D., Theodoridis, G., Plumb, R. S., et al. (2013). Global metabolic profiling of animal and human tissues via uplc-ms. Nat. Protoc. 8, 17–32. doi: 10.1038/nprot.2012.135,
Weingarden, A. R., and Vaughn, B. P. (2017). Intestinal microbiota, fecal microbiota transplantation, and inflammatory bowel disease. Gut Microbes 8, 238–252. doi: 10.1080/19490976.2017.1290757
Williams, D., Kuipers, A., Mukai, C., and Thirsk, R. (2009). Acclimation during space flight: effects on human physiology. CMAJ 180, 1317–1323. doi: 10.1503/cmaj.090628
Wilson, J. W., Ott, C. M., Höner Zu Bentrup, K., Ramamurthy, R., Quick, L., Porwollik, S., et al. (2007). Space flight alters bacterial gene expression and virulence and reveals a role for global regulator hfq. Proc. Natl. Acad. Sci. U. S. A. 104, 16299–16304. doi: 10.1073/pnas.0707155104
Yan, X., Jin, J., Su, X., Yin, X., Gao, J., Wang, X., et al. (2020). Intestinal flora modulates blood pressure by regulating the synthesis of intestinal-derived corticosterone in high salt-induced hypertension. Circ. Res. 126, 839–853. doi: 10.1161/CIRCRESAHA.119.316394
Yoon, H. S., Cho, C. H., Yun, M. S., Jang, S. J., You, H. J., Kim, J. H., et al. (2021). Akkermansia muciniphila secretes a glucagon-like peptide-1-inducing protein that improves glucose homeostasis and ameliorates metabolic disease in mice. Nat. Microbiol. 6, 563–573. doi: 10.1038/s41564-021-00880-5
Yu, Y., Zhang, B., Ji, P., Zuo, Z., Huang, Y., Wang, N., et al. (2022). Changes to gut amino acid transporters and microbiome associated with increased e/i ratio in chd8 mouse model of asd-like behavior. Nat. Commun. 13, 1151. doi: 10.1038/s41467-022-28746-2,
Zafar, H., and Saier, M. H. (2021). Gut bacteroides species in health and disease. Gut Microbes 13, 1–20. doi: 10.1080/19490976.2020.1848158
Keywords: associations, metabolic pathways, intestinal metabolites, intestinal microbiota, simulated microgravity, long-term
Citation: Yuan L, Zhang R, Li X, Gao C, Hu X, Hussain S, Zhang L, Wang M, Ma X, Pan Q, Lou X and Si S (2023) Long-term simulated microgravity alters gut microbiota and metabolome in mice. Front. Microbiol. 14:1100747. doi: 10.3389/fmicb.2023.1100747
Edited by:
Asker Daniel Brejnrod, Technical University of Denmark, DenmarkReviewed by:
Qing Fang, University of Copenhagen, DenmarkJosé Luis Márquez, University of Santiago, Chile
Copyright © 2023 Yuan, Zhang, Li, Gao, Hu, Hussain, Zhang, Wang, Ma, Pan, Lou and Si. This is an open-access article distributed under the terms of the Creative Commons Attribution License (CC BY). The use, distribution or reproduction in other forums is permitted, provided the original author(s) and the copyright owner(s) are credited and that the original publication in this journal is cited, in accordance with accepted academic practice. No use, distribution or reproduction is permitted which does not comply with these terms.
*Correspondence: Xiaotong Lou, TG91eHRAc2luYS5jb20=; Shaoyan Si, c2lzaHkzMDZAMTI2LmNvbQ==
†These authors have contributed equally to this work and shared first authorship