- Guangxi Key Laboratory of Forest Ecology and Conservation, College of Forestry, Guangxi University, Nanning, China
The complex gut bacterial communities have a major impact on organismal health. However, knowledge of the effects of habitat change on the gut microbiota of wild birds is limited. In this study, we characterized the gut microbiota of two different subspecies of the Silver-eared Mesia (Leiothrix argentauris), the native subspecies (L. a. rubrogularis) and immigrant subspecies (L. a. vernayi), using 16S rRNA gene high-throughput sequencing. These two subspecies live in a trace metal-contaminated area, and L. a. vernayi was trafficked. They are an excellent system for studying how the gut microbiome of wild animal changes when they move to new habitats. We hypothesized that the immigrant subspecies would develop the same adaptations as the native subspecies in response to habitat changes. The results showed that there were no significant differences in the composition, diversity, or functional metabolism of gut microbiota between native and immigrant subspecies under the combined action of similar influencing factors (the p values of all analyses of variance >0.05). In addition, the composition and functional metabolism of gut microbiota in two subspecies showed adaptation against trace metal damage. Linear discriminant analysis effect size (LEfSe) analysis revealed that Massilia in the intestinal microbiota of immigrant subspecies was significantly higher than that of native subspecies, suggesting that immigrant subspecies suffered habitat change. Finally, we found that these two subspecies living in the mining area had an extremely high proportion of pathogenic bacteria in their gut microbiota (about 90%), much higher than in other species (about 50%) living in wild environment. Our results revealed the adaptation of intestinal microbiota of immigrant Silver-eared Mesias under heavy metals stress, which would provide guidance for biodiversity conservation and pollution management in mining area.
Introduction
Vertebrates exhibit a highly complex symbiotic relationship with their gut microbiota (McFall-Ngai et al., 2013). The gut microbiota is conducive to metabolism, immunity, behavior, and development of the organisms, with positive implications on host health (McFall-Ngai et al., 2013; Youngblut et al., 2019). For instance, the gut microbiome can regulate nutrient absorption efficiency, influence external temperature, and control metabolic rate (Jumpertz et al., 2011; Chevalier et al., 2015). Besides, some specific microorganisms can enhance the resistance of the host to toxicity (Kohl et al., 2014). In turn, the hosts provide a nutrient-rich, stable habitat for the gut microbiome (Bodawatta et al., 2022a). Hosts and their gut microbiota have shaped a complex symbiotic relationship.
The animal’s gut microbiome is derived primarily from the environment (Candela et al., 2012). As a result, the gut microbiome is an unstable element, constantly varying in response to external environment changes (Candela et al., 2012). For example, animals can acquire new gut microbes from the environment (Hehemann et al., 2010). The relative abundance of gut microbes can be reconfigured according to various environmental factors (Candela et al., 2012). The hosts’ metabolism (Fan and Pedersen, 2021), nutrition (Moszak et al., 2020), immunology (Grond et al., 2018), behavior (Morais et al., 2021), morphology (Broderick et al., 2014) and development (Gilbert et al., 2015) would be affected by gut microbiome changes. Understanding how environmental change dictates the microbiota in the intestine will facilitate the conservation and management of wildlife (Alberdi et al., 2016; Rosenberg and Zilber-Rosenberg, 2018).
Many factors cause animals to alter their habitat. These include natural migrations, such as the migration of birds and fish, as well as forced migrations, such as captivity, global warming, and animal trade. Generally, intestinal microbiota changes caused by natural migration are usually weak - most bacterial taxa are significantly unaffected (Risely et al., 2017, 2018). Conversely, wildlife will lose their native microbiome and reduce the alpha diversity of gut microbiome when moving to captivity (Alberdi et al., 2021; Dallas and Warne, 2022). Forced migrations caused by climate warming often result in gut microbiota variations due to diet changes (Chen et al., 2022a,b). The illegal trade of animals usually ends in captivities or the release of wildlife to new habitats (Ni et al., 2020). However, previous research always focused on the gut microbiota of animals in captivities (Alberdi et al., 2021; Dallas and Warne, 2022). The gut microbiome of illegally traded wildlife released into new natural habitats is poorly known.
The Silver-eared Mesia (Leiothrix argentauris), of least concern threat-status (IUCN), is a resident bird and distributed mainly in forests around China and India, as well as in Sumatra1. This bird does not show distinct sexual dimorphism. Its feathers are brightly colored, varying in different subspecies. Silver-eared Mesia is ubiquitous in international live-bird trade because of its unique characteristics (Li and Jiang, 2014; Eaton et al., 2015). This eventually leads to the traded Silver-eared Mesias being raised in captivity or introduced into natural habitats where other native populations live. In addition, the immigrant and native population of Silver-eared Mesia may live in the same flock because of their gregarious habits. Hence, Silver-eared Mesia is an excellent model to study how the gut microbiota of wildlife would change after moving to a new habitat by international trade.
In this study, we reported the composition of gut microbiota in the two subspecies of Silver-eared Mesia, including native (L. a. rubrogularis) and immigrant subspecies (L. a. vernayi), at a mine area in Southern China. The overall goal was to compare the composition, diversity, and functional characteristics of the gut microbiota of both native (L. a. rubrogularis) and immigrant (L. a. vernayi) populations. In addition, we also analyzed the intestinal microbiome under trace element stress to explore whether the immigrant population enhanced adaptability to the new environment for the host.
Materials and methods
Ethical guidelines
All samples of feathers and cloacal swabs of Silver-eared Mesias were obtained under the permission of Chongzuo Forestry Department (2018. 1), and Animal Ethics Committee, Guangxi University (GXU2018-039), China, and procedures followed the laws of the People’s Republic of China.
Study area overview
We conducted this study in a mine tailing (22°58′15″N, 107°17′28″E) in the Daxin County of Chongzuo Prefecture in Guangxi, Zhuang Autonomous Region, Southern China. The French began mining here more than 100 years ago. However, due to environmental pollution and the depletion of mineral resources, the mine was closed in 2001 (He et al., 2020). The region has a subtropical Marine monsoon climate, with an average annual precipitation of 1348.8 mm. Due to water leaching and surface runoff, the downstream rivers and soil are seriously polluted by trace elements. Cadmium levels in the soil were 11.3 times higher than the recommended limit set in 2000 (Pan et al., 2020).
Study species
There are two subspecies of the Silver-eared Mesia in this area. One is a native subspecies, L. a. rubrogularis, which is naturally distributed in the studied area (Jiang A. et al., 2021). Another subspecies, L. a. vernayi, which is distributed initially mainly in Myanmar and Southwestern China, has recently been observed in field monitoring. The plumage characteristics of the two subspecies are similar. The only apparent difference is that L. a. rubrogularis has a red collar and base coverings of upper tail, while L. a. vernayi is orange. The diet of Silver-eared Mesia is uniformly omnivorous, feeding on insects and their larvae, fruits, and seeds.
Sample collection
We captured the two subspecies living in the forests around the tailing pond using mist nets in January 2022. We used passive methods (no birdsong playback), placing nets from dawn to dusk and patrolling them at least once per hour. We eventually captured 19 Silver-eared Mesias (5 L. a. rubrogularis and 14 L. a. vernayi). Once birds are captured, we cleaned the outside of the cloaca with an alcohol pad, inserted a sterile flocking swab (Puritan 25-3,316-U Ultra Flocked Swab, United States) fully into the cloaca, turned for 3–5 s, and preserved the swab in RNAlater (Qiagen, Hilden, Germany). Then, samples were immediately placed in sterile vials, kept in a cool box in the field, and later stored at −20°C. Upon return to the laboratory, all samples were stored at −80°C until processed. Before color-mapping and release, we measured the body index of each bird (including body weight, body length, length of wing and length of tarsometatarsus). Besides, we collected each bird’s primary, secondary, chest and tail feathers to measure trace element levels. The measurement method was described in detail in the Supplementary materal.
DNA extraction, PCR amplification, and amplicon sequencing
Total genome DNA from samples was extracted using cetyltrimethylammonium bromide (CTAB) method. DNA concentration and purity were monitored on 1% agarose gel. According to the concentration, DNA was diluted to 1 ng/μL using sterile water.
PCR amplification of the V3–V4 hypervariable region of bacterial 16S rRNA genes was performed using the bacterial-specific forward primer 341F (5′-CCTAYGGGRBGCASCAG-3′) and reverse primer 805R (5′-GGACTACNNGGGTATCTAAT-3′) with the barcode. All PCR reactions were carried out with 15 μl of Phusion® High-Fidelity PCR Master Mix (New England Biolabs); 2 μM of forward and reverse primers, and about 10 ng template DNA. Thermal cycling consisted of initial denaturation at 98°C for 1 min, followed by 30 cycles of denaturation at 98°C for 10 s, annealing at 50°C for 30 s, and elongation at 72°C for 30 s, followed by a final extension of 5 min at 72°C. Mix same volume of 1X TAE buffer with PCR products and operate electrophoresis on 2% agarose gel for detection. PCR products was mixed in equidensity ratios. Then, mixture PCR products was purified with Qiagen Gel Extraction Kit (Qiagen, Germany).
Sequencing libraries were generated using TruSeq® DNA PCR-Free Sample Preparation Kit (Illumina, United States) following manufacturer’s recommendations and index codes were added. The library quality was assessed on the Qubit@ 2.0 Fluorometer (Thermo Scientific). At last, the library was sequenced on an Illumina NovaSeq platform and 250 bp paired-end reads were generated (a total of 19 samples, 5 L. a. rubrogularis, and 14 L. a. vernayi). Raw sequences obtained in this study are available through the National Center for Biotechnology Information (NCBI) database (accession number PRJNA853520).
16S rRNA gene amplicon sequencing analysis and processing
Microbiome bioinformatics analysis were performed with the QIIME 2 (2022.2) process (Bolyen et al., 2019). With reference to the review by Knight et al. (2018), we made slightly modified based on the official tutorial2. In short, paired-end reads were merged using ‘vsearch join-pairs’ and quality filtered using ‘quality-filter q-score-joined’ (Bokulich et al., 2013) within QIIME2 after trimming the barcode and primer sequences from the reads. Next, sequences were quality filtered and denoised using the Deblur workflow (Amir et al., 2017). All amplicon sequence variants (ASVs) were aligned with mafft (Katoh et al., 2002). Taxonomy was assigned to ASVs using ‘feature-classifier classify-sklearn’ plugin against the pre-trained Naive Bayes classifier (Bokulich et al., 2018) [based on SILVA v138 database (Quast et al., 2012)]. Then, the ASVs containing less than 0.1% of total sequences across all samples, any contaminating mitochondrial and chloroplast sequences and sequences not assigned to phylum were filtered out from the denoising results. Next, ‘fragment-insertion sepp’ (Mirarab et al., 2012) is used to generate our phylogenetic tree by inserting short molecular sequences into an existing phylogenetic tree and aligning each short molecular sequence to the aligment of the full-length sequences, then that alignment is used to find the optimal location in the phylogenetic tree for querying sequences. Finally, to minimize the difference of sequencing depth across samples, data was rarefied to a sampling depth of 17,400 reads per sample for the downstream diversity analysis.
Metagenomic pathway prediction by PICRUSt2
The 16S rRNA gene sequence of the sample was mapped to KEGG3 database for annotation, and the abundance of metabolic pathways was predicted based on Phylogenetic Investigation of Communities by Reconstruction of Unobserved States (PICRUSt2) (Douglas et al., 2020). R software (v4.1.2) was utilized for statistical analyses and visualization of the identified pathways. Correlations between samples were visualized using the pheatmap package. Linear discriminant analysis effect size (LEfSe) analysis was used to detect the differentially abundant metabolic pathways between L. a. rubrogularis and L. a. vernayi.
Statistical analysis
Statistical analysis was completed in R v4.1.2 (R Core Team, 2021). Alpha diversity analysis was carried out using the “picante” package (v1.8.2) (Kembel et al., 2010). Differences in Alpha diversity between L. a. rubrogularis and L. a. vernayi were evaluated by one-way ANOVA (Jobson, 1991), and all p values were corrected via the false discovery rate (FDR) correction. Rarefaction curves were generated by “ggplot2” package (v3.3.5) (Wickham, 2016). The ASV-level ranked abundance curves were generated to compare the richness and evenness of ASVs among samples. Venn diagram was generated to visualize the shared and unique ASVs among groups using the R package “VennDiagram” (v1.7.3) (Hanbo, 2022), based on the occurrence of ASVs across samples/groups regardless of their relative abundance. Beta diversity analysis was performed to investigate the structural variation in microbial communities across samples using weighted and unweighted UniFrac distance metrics (Lozupone et al., 2011) and visualized via principal coordinate analysis (PCoA) (Ramette, 2007). Differences in the UniFrac distances for pairwise comparisons among groups were determined by Anosim test with 999 permutations using “vegan” package (v2.5–7) (Jari et al., 2020). The taxonomic composition of each sample was visualized as a stacked bar plot at the phylum, family and genus level with the “ggplot2” package (v3.3.5) (Wickham, 2016). The circles plot of L. a. rubrogularis and L. a. vernayi were generated by Circos4 (Krzywinski et al., 2009). Correlations between samples were visualized using the pheatmap package (v1.0.12) (Raivo, 2019). Linear discriminant analysis effect size (LEfSe) was performed to detect differentially abundant taxa across groups using the default parameters (Segata et al., 2011).
Results
Sequencing quality and ASVs distribution
After strict quality filtering, a total of 482,768 16S rRNA gene reads were obtained, with an average of 25,409 ± 3,882 sequences per sample (median: 25785), from which 501 ASVs were identified to be from gut microbiotas of 19 Silver-eared Mesias (L. a. rubrogularis = 462, L. a. vernayi = 501). The rank abundance and rarefaction curves supported the adequacy of the sequencing depth, indicating that almost all the bacterial species were found in intestinal samples (Figures 1A–D). A total of 92.22% of the ASVs were shared among the two groups, with unique ASVs entirely being present in the L. a. vernayi (7.78%). All ASVs can be detected in L. a. vernayi (Supplementary Figure S1).
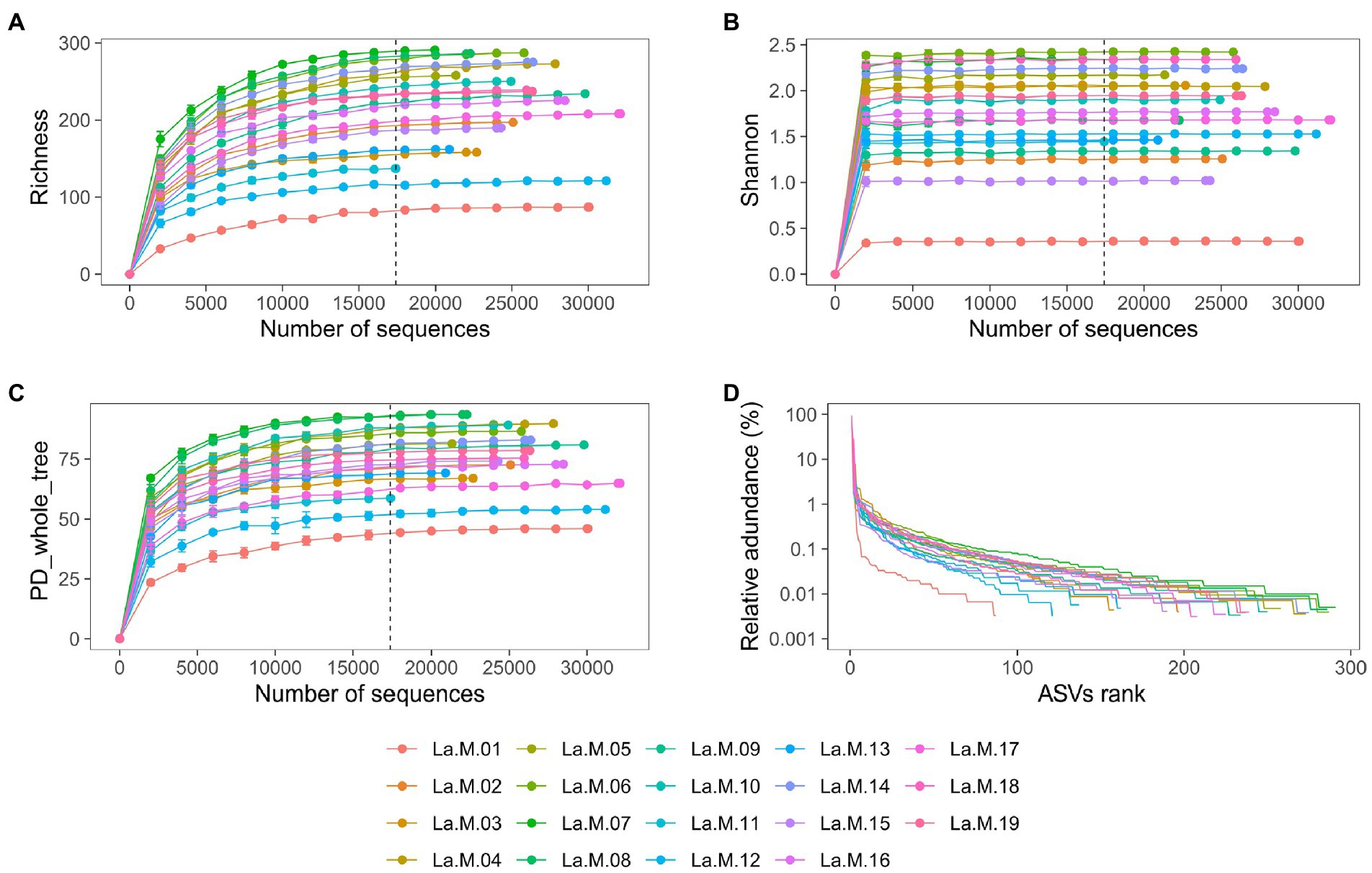
Figure 1. Sequencing depth analysis and ASVs distribution of gut microbiota in L. a. rubrogularis and L. a. vernayi. Rarefaction curves of gut microbiota of all samples based on Illumina MiSeq sequencing. Horizontal axis: the amount of effective sequencing data; vertical axis: (A) the observed number of operational taxonomic units (richness index); (B) Shannon index; (C) and PD_whole_tree. (D) Rank abundance curve of gut microbiota of Leiothrix argentauris. Horizontal axis: the number of ASVs according to the abundance, from high to low. Vertical axis: the abundance of ASVs. The larger the span curve on the horizontal axis, the higher the species richness. The smoother the curve on the vertical axis, the more even the species distribution.
Characteristics of gut microbial diversity of L. a. rubrogularis and L. a. vernayi
To evaluate the differences in community richness and diversity among the subspecies, the effective sequences were aligned to calculate the Good’s coverage, Richness, Shannon, and PD_whole_tree indices. Good’s coverage estimates of each sample ranged from 99.82 to 99.97%, suggesting excellent coverage (Figure 2A). Measurements of gut community alpha diversity did not vary significantly between L. a. rubrogularis and L. a. vernayi (based on one-way ANOVA, Richness index, value of p = 0.4350; Shannon index, value of p = 0.876; PD_whole_tree, value of p = 0.329). Thus, the diversity of intestinal microbiota between L. a. rubrogularis and L. a. vernayi was similar (Figures 2B–D).
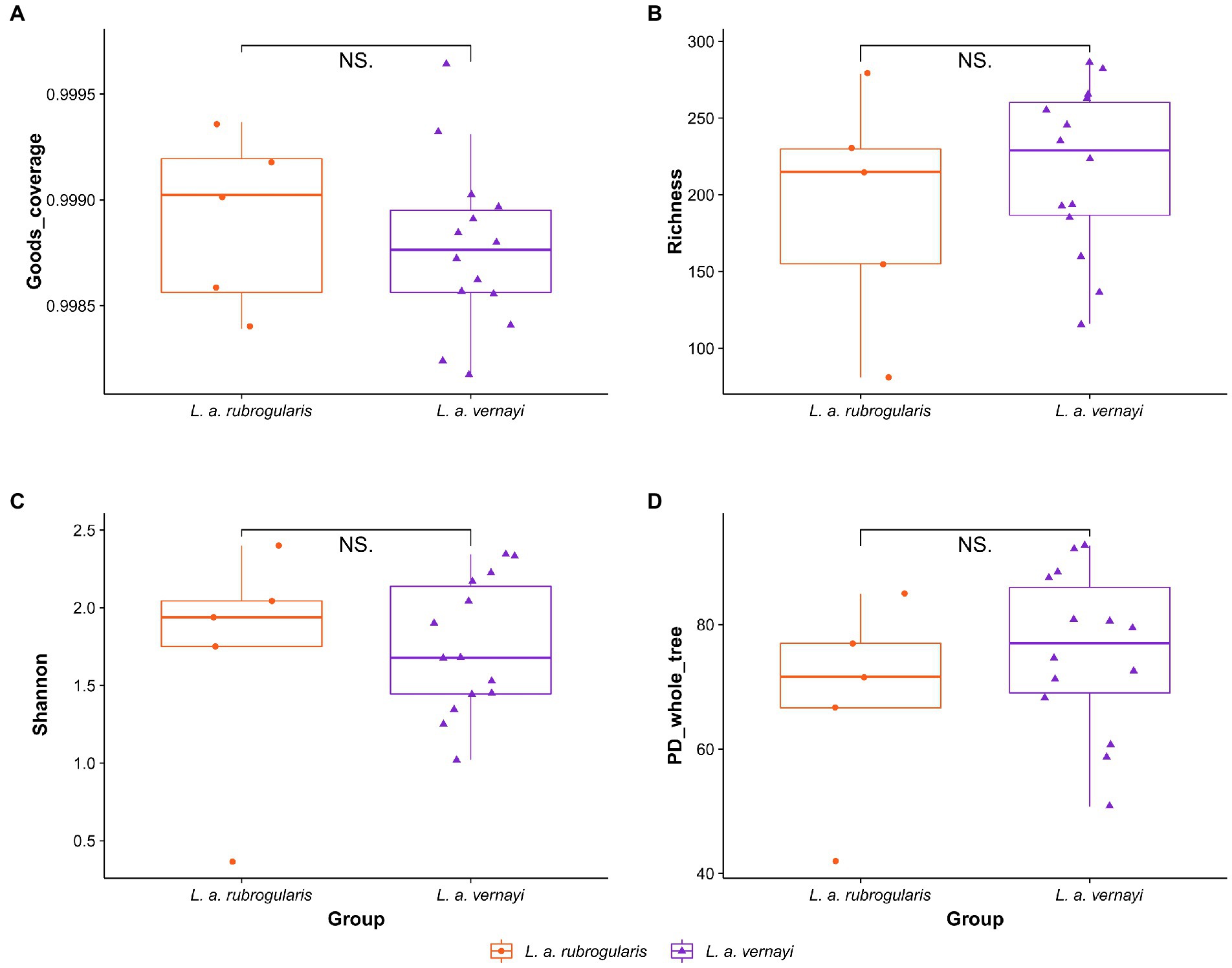
Figure 2. Alpha diversity of gut microbiota in the L. a. rubrogularis and L. a. vernayi. (A) Good’s coverage; (B) Richness index; (C) Shannon index; (D) PD_whole_tree. “NS.” showed no significant difference between the two groups (p > 0.05).
Weighted and unweighted Unifrac distances are used to generate PCoA plots reflecting the gut microbial beta-diversity between individuals. No significant difference between L. a. rubrogularis and L. a. vernayi was detected no matter what weighted Unifrac distance or unweighted Unifrac distance was considered (Figures 3A,B, Anosim test: value of p = 0.251 and 0.217, R = 0.0945 and 0.1084, respectively).
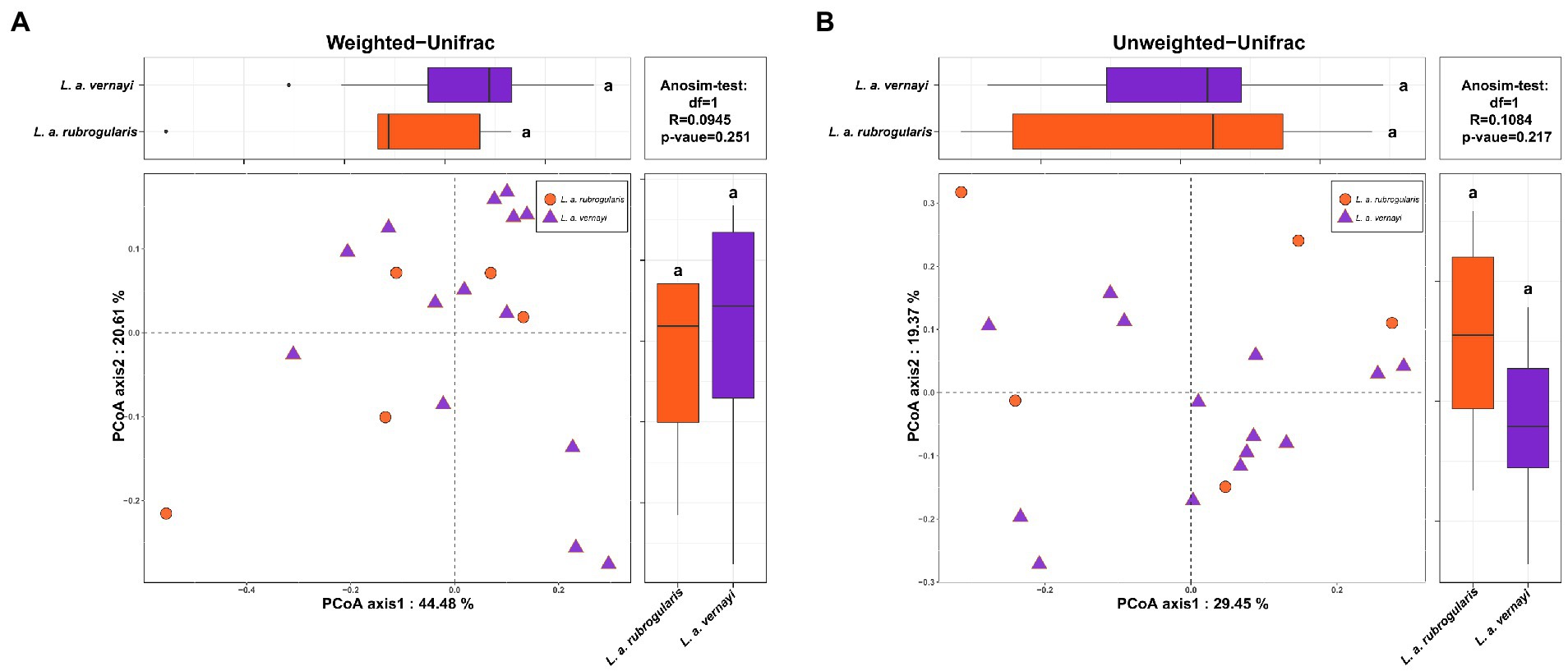
Figure 3. Beta diversity of gut microbiota in the L. a. rubrogularis and L. a. vernayi. (A) Principal coordinates analysis (PCoA) based on weighted UniFrac distance (B) and unweighted UniFrac distance. Wilcoxon rank-sum test of two subspecies on the first axis is shown on the upper left; Wilcoxon rank-sum test of two subspecies on the second axis is at the lower right; Anosim-test results based on bray-Curits distance between two subspecies are shown in the upper right.
Characteristics of gut microbiota composition of L. a. rubrogularis and L. a. vernayi
Nearly all reads were assignable to 13 phyla, 17 classes, 54 orders, 97 families, and 162 genera. At the phylum level, Proteobacteria were the most abundant bacterial phylum (88.94, 93.46%) among L. a. rubrogularis and L. a. vernayi gut communities followed by Firmicutes (6.05, 3.12%), Bacteroidetes (2.51, 1.24%), and Actinobacteria (1.65, 1.61%) (Figure 4A). The relative abundance of the remaining others phyla was low. All the phyla of gut microbiota in L. a. rubrogularis and L. a. vernayi were shown in Figure 4B. The relative abundance of each phylum across samples fluctuated little.
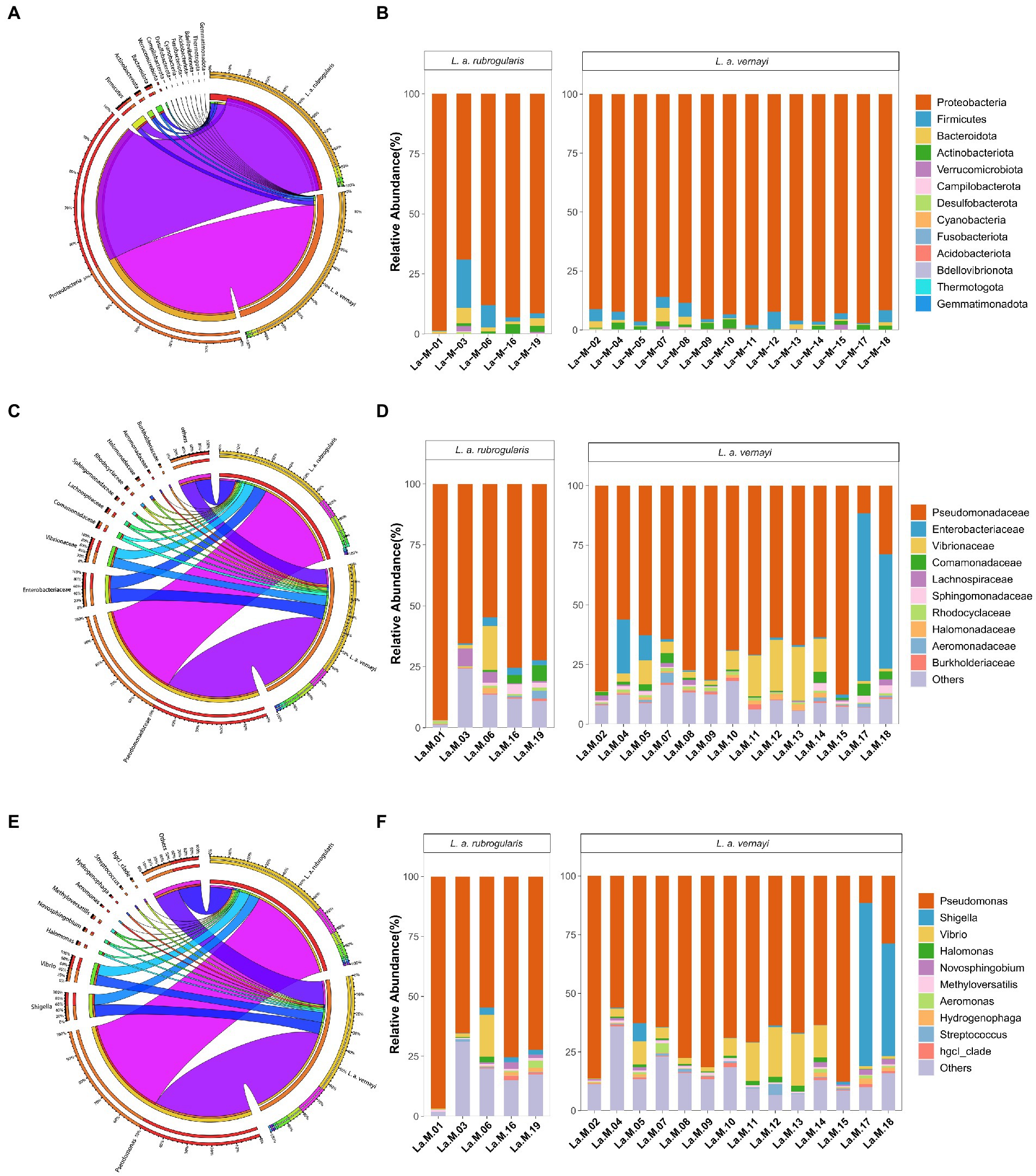
Figure 4. Relative abundance of the dominant taxa in the L. a. rubrogularis and L. a. vernayi. (A) Phylum; (C) family; (E) genus. Relative contribution of the dominant taxa in all samples. (B) Phylum; (D) family; (F) genus.
At the family level, Pseudomonadaceae predominated (66.04, 63.87%) among L. a. rubrogularis and L. a. vernayi gut communities followed by Enterobacteriaceae (8.80, 9.72%), Vibrionaceae (6.71, 7.48%), Comamonadaceae (1.96, 1.80%), Lachnospiraceae (1.27, 1.38%), Sphingomonadaceae (1.22, 1.25%), Rhodocyclaceae (0.87, 0.84%), Halomonadaceae (0.85, 0.94%), Aeromonadaceae (0.73, 0.63%) and Burkholderiaceae (0.66, 0.66%) (Figure 4C). The top 10 families of gut microbiota in L. a. rubrogularis and L. a. vernayi were shown in Figure 4D. We observed that the abundance of Enterobacteriaceae in two samples of L. a. vernayi was higher than that in other samples, and even exceeded that of Pseudomonadaceae.
At the genus level, Pseudomonas predominated (66.04, 63.87%) among L. a. rubrogularis and L. a. vernayi gut communities followed by Shigella (7.18, 7.90%), Vibrio (6.56, 7.31%), Halomonas (0.85, 0.94), Novosphingobium (0.84, 0.86), Methyloversatilis (0.81, 0.78%), Aeromonas (0.71, 0.61), Hydrogenophaga (0.65, 0.63%), Streptococcus (0.64, 0.70%), and hgcl_clade (0.63, 0.65%) (Figure 4E). The top 10 genera of gut microbiota in L. a. rubrogularis and L. a. vernayi were shown in Figure 4F. The abundance of Shigella was higher in two samples of L. a. vernayi than in the other samples.
Difference analysis of the gut microflora between L. a. rubrogularis and L. a. vernayi
To further investigate whether there were differences in intestinal microbial community composition between L. a. rubrogularis and L. a. vernayi. The top 40 genera with average abundance were used to construct the heatmap of the genus-level hierarchical clustering. Although the clustering was divided into two categories, L. a. rubrogularis and L. a. vernayi were not, respectively, assigned to the two categories, and the distribution of the two groups was very dispersed (Figure 5A). LEfSe analysis was used to determine the microbial communities with significant differences in abundance between the two groups across the phylum, class, order, family, and genus levels. Interestingly, we identified only one genus taxon, Massilia, as having a significantly higher abundance in L. a. vernayi than in L. a. rubrogularis (LDA > 2, value of p < 0.05) (Figure 5B). Lefse analysis confirmed the results of genus clustering and showed that the composition of intestinal flora in L. a. rubrogularis and L. a. vernayi was basically consistent.
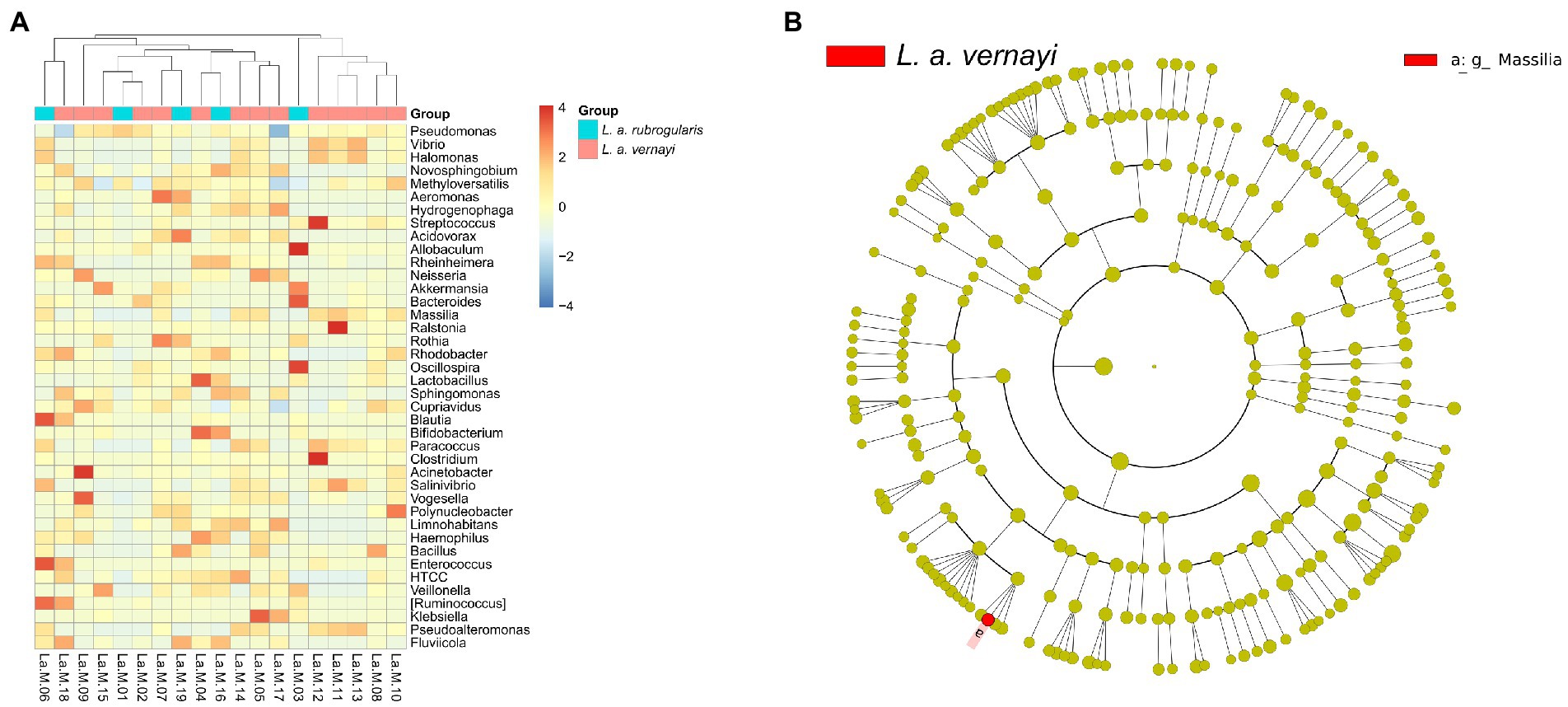
Figure 5. (A) Heatmap of the genus-level hierarchical clustering of the microbial community in L. a. rubrogularis and L. a. vernayi. (B) Differential microbial phylogenetic distribution was visualized by the cladogram. The taxonomic branch diagram shows the taxonomic hierarchy of the main taxa from phylum to genus (from inner ring to outer ring) in the sample community.
Prediction and comparisons in the gut microbial metabolism pathways
According to the prediction, the KEGG Pathway Database classified metabolic pathways into six categories, including cellular processes, environmental information processing, genetic information processing, human diseases, metabolism, and organismal systems. Each metabolic pathway was further divided into several levels. The second level included 31 metabolic pathway sub-functions, and a total of 159 the third level functional pathways was predicted. Figure 6A demonstrates the average relative abundance of bacteria in all individuals of Silver-eared Mesia mapped to the secondary functional pathway of the KEGG database. The KEGG pathways showed that ‘metabolism’ was the most important pathway of gut microbiota.
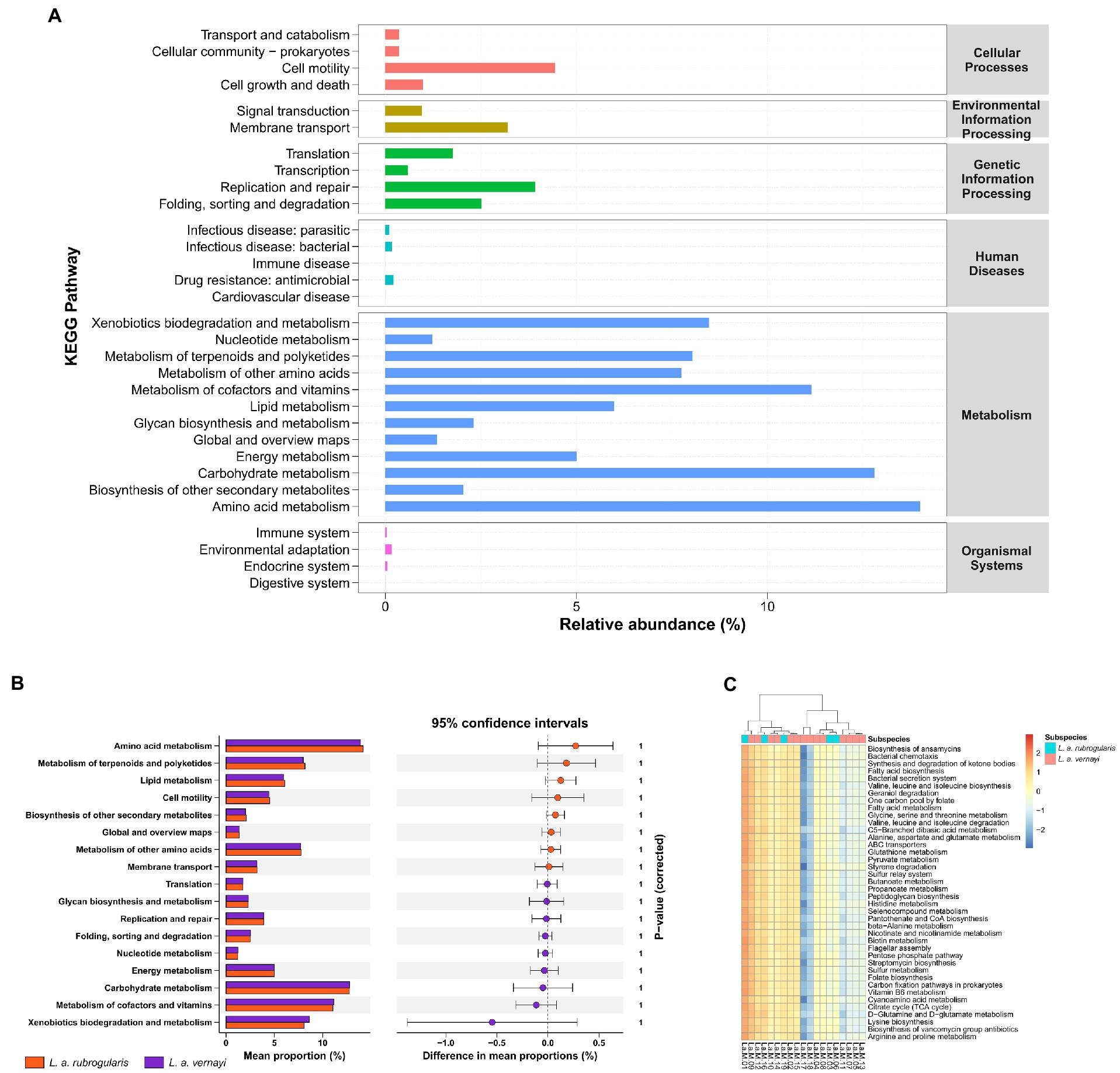
Figure 6. The prediction of metabolic function within the microbiome in L. a. rubrogularis and L. a. vernayi (n = 19). (A) KEGG analysis of the relative abundance of metabolic pathways between L. a. rubrogularis and L. a. vernayi. The abscissa represents the relative abundance of functional pathways, the ordinate represents the functional pathways of the second classification level of KEGG, the rightmost box represents the first level to which the pathways belong. (B) Extended error bar plot to determine the significant difference in the predicted gene function between L. a. rubrogularis and L. a. vernayi. The middle value represents the mean differences between the groups (upper bar value – lower bar), and the error bar represents the 95% confidence intervals. The right side of the zero-point indicates the larger effect on the gene function in the L. a. rubrogularis, and the left side of the zero-point indicates the larger effect of that in the L. a. vernayi. p value at the side indicates the significance levels between the upper and lower bars. (C) Heatmap of the genus-level hierarchical clustering of the third level functional pathways in L. a. rubrogularis and L. a. vernayi.
Wilcoxon rank-sum test showed no significant difference in secondary metabolic pathways between L. a. rubrogularis and L. a. vernayi (Figure 6B) (secondary metabolic pathways with relative abundance of less than 1% in all samples were excluded and the p-values were corrected by Bonferroni). Clustering heat maps of the abundances of the top 40 third level functional pathways showed differences in functional abundances across samples, but the individuals of L. a. rubrogularis and L. a. vernayi were not clustered into two categories, respectively (Figure 6C). The results demonstrated no significant difference in the metabolic function of the intestinal microbiota between L. a. rubrogularis and L. a. vernayi. The LEfSe analysis was applied to all levels of KEGG metabolic pathways and also did not detect any variation between L. a. rubrogularis and L. a. vernayi (Supplementary Figure S2).
Discussion
This is the first study to show the gut microbiota of Silver-eared Mesia whose habitat has been altered by trade. Previous studies have focused on comparing native habitats with captivity (Wienemann et al., 2011; Sun et al., 2019; Oliveira et al., 2020; San Juan et al., 2021; Grieves et al., 2022; Zhang et al., 2022). However, under captive conditions, the host’s living environment and diet are artificially controlled, so it is difficult to reflect the impact of the real field environment on the intestinal microbiota of immigrant species. In addition, under natural conditions, the host’s habitat environment and food sources are variable, such as the significant differences in diet composition between individuals within a species (Bodawatta et al., 2022b), which is difficult to overcome for cross-species and cross-regional comparison. In this study, native (L. a. rubrogularis) and immigrant (L. a. vernayi) subspecies lived in the same group, so their diet, environment, and other external factors were the same, and there were only slight phylogenetic differences, which can supply a unique opportunity to compare the gut microbiota of immigrant and native subspecies. Wildlife trade is a common route for introducing invasive species (Cardador et al., 2019). Traded migrant species may adversely affect native ecosystems, economic activity, and human well-being (Simberloff et al., 2013). Our findings can help to assess the risk of invasion of this non-native species for better understanding and management.
The gut microbiota of immigrant subspecies coincides with the native subspecies
On the whole, our results demonstrated no significant differences in the composition, diversity, or functional metabolism of the gut microbiota between native and immigrant subspecies, which might be related to a similar diet, environment, and other external factors. The convergent evolution of gut microbiome, driven by similar dietary and environmental factors, has been found in other vertebrates as well. For example, the bamboo-eating Ailuropoda melanoleuca and Ailurus styani share more similarities in their gut microbiota structure and function with each other than their carnivorous relatives (Huang et al., 2021). Similarly, this phenomenon has been observed in studies of immigrants of different races. The gut microbiota of immigrants in the United States would become more and more similar to that of native people over time (Vangay et al., 2018; Peters et al., 2020; Copeland et al., 2021). To sum up, the intestinal microbiota of immigrant populations developed the same adaptive characteristics as that of native populations, and the generation of these adaptive characteristics may help immigrant populations adapt quickly to habitat changes (Alberdi et al., 2016).
Characteristics of the gut microbiota of the Silver-eared Mesia living in mining area
Trace metals in the soil can be concentrated in the plants, which insects then feed on and carry trace elements into their bodies (Zhang et al., 2017). Thus, the extensive diet of Silver-eared Mesia will enrich a mass of trace elements into the body, making its intestinal microbiota under trace element stress environment. As a result, we also investigated the characteristics of its intestinal microbiota under trace elements stress. From the community composition of the gut microbes of native and immigrant subspecies, it was found that at the phylum level, Proteobacteria dominated the intestinal flora of the two subspecies (about 90%), followed by Firmicutes, Bacteroidetes, and Actinobacteria. Previous studies have found that Firmicutes are the main microbiome in the guts of mammals, chickens and wild birds (Waite and Taylor, 2014; Grond et al., 2018). Members of Firmicutes play an important role in the metabolism, digestion and absorption of proteins and other substances nutrients, and participate in the synthesis of digestive enzymes to assist the host in the digestion and absorption of nutrients (Grond et al., 2018). Proteobacteria, however, are mostly pathogenic bacteria, usually associated with intestinal ecological imbalances, metabolic and immune disorders (Colston and Jackson, 2016). Some studies of other wild birds have found Firmicutes to be the dominant species in the gut microbiota (Wang et al., 2017; Oliveira et al., 2020; Wu et al., 2021). In several other studies on wild birds, nevertheless, Proteobacteria were found to have a higher abundance than Firmicutes (Zhou et al., 2020; Zhang et al., 2022). Even so, compared with our study in which Proteobacteria accounted for almost 90% of the intestinal flora, Proteobacteria in their study were only slightly higher than Firmicutes. Given that the samples in these studies came from captivity or normal wild environments, it may be suggested that adaptation of intestinal flora to trace element stress may lead to Proteobacteria dominance. This is consistent with the characteristics of intestinal microbiota in other species under trace element stress (Šrut et al., 2019; Kakade et al., 2020; Wu et al., 2020).
The role of intestinal microbiota in reducing heavy metal toxicity
Previous studies have shown that the gut microbiome is essential in protecting the host from the toxic effects of heavy metal exposure (Halttunen et al., 2008; Breton et al., 2013; Ninkov et al., 2015). In our study, we also found that the dominant group in the intestinal microbiome showed strong resistance to heavy metal toxicity. For instance, the Pseudomonas was dominant among almost all samples. Pseudomonas can degrade cellulose (Jiménez et al., 2014) and produce multitudinous antibiotic compounds, which can effectively stem the diseases caused by pathogenic bacteria and fungi (Haas and Défago, 2005). Particularly important, Pseudomonas showed a sky-high degree of resistance to heavy metals, such as Cd, Pb and As (Al-Ansari et al., 2021; Liu et al., 2021; Pramanik et al., 2021; Sun et al., 2021). In addition, several genera with high abundance, such as Shigella, Vibrio and Halomonas, also have high trace element resistance (Mukherjee et al., 2019; Jo et al., 2020; Bombaywala et al., 2021). These bacteria may play an important role in resisting trace element stress and maintaining normal growth, development and life activities of Silver-eared Mesia.
We also predicted the metabolic function of the gut microbiota of the Silver-eared Mesia. The metabolic pathways predicted by PICRUSt2 were consistent with those predicted by other wild species (Figure 6A; Zhou et al., 2020; Jiang F. et al., 2021; Zhang et al., 2022). Microbial metabolic pathways (such as xenobiotics biodegradation metabolism, carbohydrate metabolism, amino acid metabolism, and metabolism of cofactors and vitamins) accounted for more than 60% of the 31 predicted most abundant pathways. This suggests that the gut microbiome of Silver-eared Mesia may be involved in high levels of metabolic activity that may help to resist damage caused by trace elements.
Speculation about when L. a. vernayi immigrated
We cannot evaluate the specific time of immigration of immigranted L. a. vernayi. According to our field survey results and some data (Jiang A. et al., 2021) in recent years, we can infer that its earliest migration time was around 2019, because it has not been found in the field survey before 2019. Interestingly, the Lefse analysis of our taxa may support this inference. Among all taxa of L. a. rubrogularis and L. a. vernayi, lefse analysis detected a genus, Massilia, with a significantly higher relative abundance in L. a. vernayi than that in L. a. rubrogularis. The Massilia are important resistant microbes in trace element stress environments, as it has been identified in mining soil, farmland soils, beach, and sludge polluted by trace elements (Feng et al., 2016; Krishnamoorthy et al., 2016; Lee et al., 2017; Wang et al., 2020). The Massilia can secrete a large amount of cyclodextrin, whose special physical structure can contain trace element ions (Santos and Barbosa-Tessmann, 2019), thus reducing the content of trace element in the intestinal environment and reducing its toxicity to Silver-eared Mesia (Badruddoza et al., 2013; Tajuddin Sikder et al., 2014). Another study on earthworms (Eisenia fetida) also found that Massilia showed a higher abundance after cadmium contamination, and that the abundance of Massilia decreased in the middle stage of cadmium pollution and increased to a stable level in the later stage (Zhou et al., 2021). This finding suggests that L. a. vernayi did not migrate for very long. We speculate that in the future, the abundance of Massilia in the intestinal tract of L. a. vernayi will fluctuate until it becomes similar to that of L. a. rubrogularis.
Limitations
It should be noted that this study has several limitations. First, we do not understand the microbiome of the immigrant form, prior to our sampling. Secondly, we did not observe it in non-mining areas perhaps due to its rarity in China (second class national protected animal), which led to the inability to observe the adaptation process of the intestinal microbiota of Silver-eared Mesia under trace element stress. In addition, there are some differences in the sample numbers of L. a. rubrogularis and L. a. vernayi. Although the intestinal microbiota of L. a. rubrogularis and L. a. vernayi are very similar in our study, the differences in sample numbers may also cause some deviations in the results. From another perspective, however, the different sample numbers of the two subspecies may reflect their proportions in the wild-living taxa. There are far more immigrant subspecies than native ones, which could be detrimental to our biodiversity conservation. We suggest that some controlled experiments in non-mining areas and the habitat of L. a. vernayi should be carried out in future studies, which will be of significance for the protection of wild animals.
Conclusion
In conclusion, the composition, diversity, and function of the intestinal microbiota of immigrant subspecies (L. a. vernayi) were basically the same as those of the native subspecies (L. a. rubrogularis). The gut microbiota of the Silver-eared Mesia living in the mining area developed adaptive characteristics to trace element stress. The significance of this study lies in: (1) the results shed some light on the adaptation of the gut microbiota of immigrant species to cope with habitat changes after “migration”. It supplies new insights into the adaptation mechanism of gut microbiota in response to environmental changes in wild animals, thus providing important implications for wildlife conservation and biodiversity conservation. (2) The results also showed the adaptation mechanism of the intestinal microbiota of birds under trace element environmental stress (how to change the composition and function of the microbiota to resist the toxic effects of trace elements). It is helpful to understand the toxicity of trace elements to birds and the strategies of birds to adapt to trace element pollution, which has a guiding role in pollution control and biodiversity protection in mining areas.
Data availability statement
The datasets presented in this study can be found in online repositories. The names of the repository/repositories and accession number(s) can be found at: https://www.ncbi.nlm.nih.gov/, PRJNA853520.
Ethics statement
The animal study was reviewed and approved by Chongzuo Forestry Bureau and Ethics Committee of Guangxi University.
Author contributions
TZ, SL, and AJ contributed intellectual input and assistance to this study. TZ and AJ designed the research. TZ and SL did the sampling and laboratory work. TZ conducted data analysis and wrote the first draft of the manuscript. AJ contributed substantially to revisions. All authors contributed to the article and approved the submitted version.
Funding
This work was funded by the National Natural Science Foundation of China (31870370) and the Key Grant of Guangxi Nature and Science Foundation (2018GXNSFDA281016).
Acknowledgments
We thank Madhava Meegaskumbura for his help in the manuscript revision process. We are grateful to the Chongzuo Forestry Bureau for granting permission for this study. We thank Wekemo Tech Group Co., Ltd. Shenzhen China for its sequencing services.
Conflict of interest
The authors declare that the research was conducted in the absence of any commercial or financial relationships that could be construed as a potential conflict of interest.
Publisher’s note
All claims expressed in this article are solely those of the authors and do not necessarily represent those of their affiliated organizations, or those of the publisher, the editors and the reviewers. Any product that may be evaluated in this article, or claim that may be made by its manufacturer, is not guaranteed or endorsed by the publisher.
Supplementary material
The Supplementary material for this article can be found online at: https://www.frontiersin.org/articles/10.3389/fmicb.2023.1076523/full#supplementary-material
Footnotes
References
Al-Ansari, M. M., Benabdelkamel, H., AlMalki, R. H., Abdel Rahman, A. M., Alnahmi, E., Masood, A., et al. (2021). Effective removal of heavy metals from industrial effluent wastewater by a multi metal and drug resistant Pseudomonas aeruginosa strain RA-14 using integrated sequencing batch reactor. Environ. Res. 199:111240. doi: 10.1016/j.envres.2021.111240
Alberdi, A., Aizpurua, O., Bohmann, K., Zepeda-Mendoza, M. L., and Gilbert, M. T. P. (2016). Do vertebrate gut metagenomes confer rapid ecological adaptation? Trends Ecol. Evol. 31, 689–699. doi: 10.1016/j.tree.2016.06.008
Alberdi, A., Martin Bideguren, G., and Aizpurua, O. (2021). Diversity and compositional changes in the gut microbiota of wild and captive vertebrates: a meta-analysis. Sci. Rep. 11:22660. doi: 10.1038/s41598-021-02015-6
Amir, A., McDonald, D., Navas-Molina, J. A., Kopylova, E., Morton, J. T., Xu, Z. Z., et al. (2017). Deblur rapidly resolves single-nucleotide community sequence patterns. mSystems 2:e00191-16. doi: 10.1128/mSystems.00191-16
Badruddoza, A. Z. M., Shawon, Z. B. Z., Tay, W. J. D., Hidajat, K., and Uddin, M. S. (2013). Fe3O4/cyclodextrin polymer nanocomposites for selective heavy metals removal from industrial wastewater. Carbohydr. Polym. 91, 322–332. doi: 10.1016/j.carbpol.2012.08.030
Bodawatta, K. H., Hird, S. M., Grond, K., Poulsen, M., and Jønsson, K. A. (2022a). Avian gut microbiomes taking flight. Trends Microbiol. 30, 268–280. doi: 10.1016/j.tim.2021.07.003
Bodawatta, K. H., Klečková, I., Klečka, J., Pužejová, K., Koane, B., Poulsen, M., et al. (2022b). Specific gut bacterial responses to natural diets of tropical birds. Sci. Rep. 12:713. doi: 10.1038/s41598-022-04808-9
Bokulich, N. A., Kaehler, B. D., Rideout, J. R., Dillon, M., Bolyen, E., Knight, R., et al. (2018). Optimizing taxonomic classification of marker-gene amplicon sequences with QIIME 2’s q2-feature-classifier plugin. Microbiome 6:90. doi: 10.1186/s40168-018-0470-z
Bokulich, N. A., Subramanian, S., Faith, J. J., Gevers, D., Gordon, J. I., Knight, R., et al. (2013). Quality-filtering vastly improves diversity estimates from Illumina amplicon sequencing. Nat. Methods 10, 57–59. doi: 10.1038/nmeth.2276
Bolyen, E., Rideout, J. R., Dillon, M. R., Bokulich, N. A., Abnet, C. C., Al-Ghalith, G. A., et al. (2019). Reproducible, interactive, scalable and extensible microbiome data science using QIIME 2. Nat. Biotechnol. 37, 852–857. doi: 10.1038/s41587-019-0209-9
Bombaywala, S., Purohit, H. J., and Dafale, N. A. (2021). Mobility of antibiotic resistance and its co-occurrence with metal resistance in pathogens under oxidative stress. J. Environ. Manag. 297:113315. doi: 10.1016/j.jenvman.2021.113315
Breton, J., Daniel, C., Dewulf, J., Pothion, S., Froux, N., Sauty, M., et al. (2013). Gut microbiota limits heavy metals burden caused by chronic oral exposure. Toxicol. Lett. 222, 132–138. doi: 10.1016/j.toxlet.2013.07.021
Broderick, N. A., Buchon, N., Lemaitre, B., and McFall-Ngai, M. J. (2014). Microbiota-induced changes in Drosophila melanogaster host gene expression and gut morphology. mBio 5, e01117–e01114. doi: 10.1128/mBio.01117-14
Candela, M., Biagi, E., Maccaferri, S., Turroni, S., and Brigidi, P. (2012). Intestinal microbiota is a plastic factor responding to environmental changes. Trends Microbiol. 20, 385–391. doi: 10.1016/j.tim.2012.05.003
Cardador, L., Tella, J. L., Anadón, J. D., Abellán, P., and Carrete, M. (2019). The European trade ban on wild birds reduced invasion risks. Conserv. Lett. 12:e12631. doi: 10.1111/conl.12631
Chen, S., Holyoak, M., Liu, H., Bao, H., Ma, Y., Dou, H., et al. (2022a). Effects of spatially heterogeneous warming on gut microbiota, nutrition and gene flow of a heat-sensitive ungulate population. Sci. Total Environ. 806:150537. doi: 10.1016/j.scitotenv.2021.150537
Chen, S., Holyoak, M., Liu, H., Bao, H., Ma, Y., Dou, H., et al. (2022b). Global warming responses of gut microbiota in moose (Alces alces) populations with different dispersal patterns. J. Zool. 318, 63–73. doi: 10.1111/jzo.12998
Chevalier, C., Stojanović, O., Colin, D. J., Suarez-Zamorano, N., Tarallo, V., Veyrat-Durebex, C., et al. (2015). Gut microbiota orchestrates energy homeostasis during cold. Cells 163, 1360–1374. doi: 10.1016/j.cell.2015.11.004
Colston, T. J., and Jackson, C. R. (2016). Microbiome evolution along divergent branches of the vertebrate tree of life: what is known and unknown. Mol. Ecol. 25, 3776–3800. doi: 10.1111/mec.13730
Copeland, J. K., Chao, G., Vanderhout, S., Acton, E., Wang, P. W., Benchimol, E. I., et al. (2021). The impact of migration on the gut metagenome of south Asian Canadians. Gut Microbes 13, 1–29. doi: 10.1080/19490976.2021.1902705
Dallas, J. W., and Warne, R. W. (2022). Captivity and animal microbiomes: potential roles of microbiota for influencing animal conservation. Microb. Ecol. doi: 10.1007/s00248-022-01991-0
Douglas, G. M., Maffei, V. J., Zaneveld, J. R., Yurgel, S. N., Brown, J. R., Taylor, C. M., et al. (2020). PICRUSt2 for prediction of metagenome functions. Nat. Biotechnol. 38, 685–688. doi: 10.1038/s41587-020-0548-6
Eaton, J., Shepherd, C., Rheindt, F., Harris, J. B. C., van Balen, B., Wilcove, D., et al. (2015). Trade-driven extinctions and near-extinctions of avian taxa in Sundaic Indonesia. Forktail 31, 1–12.
Fan, Y., and Pedersen, O. (2021). Gut microbiota in human metabolic health and disease. Nat. Rev. Microbiol. 19, 55–71. doi: 10.1038/s41579-020-0433-9
Feng, G.-D., Yang, S.-Z., Li, H.-P., and Zhu, H.-H. (2016). Massilia putida sp. nov., a dimethyl disulfide-producing bacterium isolated from wolfram mine tailing. Int. J. Syst. Evol. Microbiol. 66, 50–55. doi: 10.1099/ijsem.0.000670
Gilbert, S. F., Bosch, T. C. G., and Ledón-Rettig, C. (2015). Eco-Evo-devo: developmental symbiosis and developmental plasticity as evolutionary agents. Nat. Rev. Genet. 16, 611–622. doi: 10.1038/nrg3982
Grieves, L. A., Bottini, C. L. J., Gloor, G. B., and MacDougall-Shackleton, E. A. (2022). Uropygial gland microbiota differ between free-living and captive songbirds. Sci. Rep. 12:18283. doi: 10.1038/s41598-022-22425-4
Grond, K., Sandercock, B. K., Jumpponen, A., and Zeglin, L. H. (2018). The avian gut microbiota: community, physiology and function in wild birds. J. Avian Biol. 49:e01788. doi: 10.1111/jav.01788
Haas, D., and Défago, G. (2005). Biological control of soil-borne pathogens by fluorescent pseudomonads. Nat. Rev. Microbiol. 3, 307–319. doi: 10.1038/nrmicro1129
Halttunen, T., Collado, M. C., El-Nezami, H., Meriluoto, J., and Salminen, S. (2008). Combining strains of lactic acid bacteria may reduce their toxin and heavy metal removal efficiency from aqueous solution. Lett. Appl. Microbiol. 46, 160–165. doi: 10.1111/j.1472-765X.2007.02276.x
Hanbo, C. (2022). VennDiagram: Generate High-Resolution Venn and Euler Plots. R package version 1.7.3. URL: https://CRAN.R-project.org/package=VennDiagram
He, C., Su, T., Liu, S., Jiang, A., Goodale, E., and Qiu, G. (2020). Heavy metal, arsenic, and selenium concentrations in bird feathers from a region in southern China impacted by intensive mining of nonferrous metals. Environ. Toxicol. Chem. 39, 371–380. doi: 10.1002/etc.4622
Hehemann, J.-H., Correc, G., Barbeyron, T., Helbert, W., Czjzek, M., and Michel, G. (2010). Transfer of carbohydrate-active enzymes from marine bacteria to Japanese gut microbiota. Nature 464, 908–912. doi: 10.1038/nature08937
Huang, G., Wang, X., Hu, Y., Wu, Q., Nie, Y., Dong, J., et al. (2021). Diet drives convergent evolution of gut microbiomes in bamboo-eating species. Sci. China Life Sci. 64, 88–95. doi: 10.1007/s11427-020-1750-7
Jari, O., Blanchet, F. G., Michael, F., Roeland, K., Pierre, L., Dan, M., et al. (2020). Vegan: Community Ecology Package. R package version 2.5-7. URL: https://CRAN.R-project.org/package=vegan
Jiang, F., Gao, H., Qin, W., Song, P., Wang, H., Zhang, J., et al. (2021). Marked seasonal variation in structure and function of gut microbiota in Forest and alpine musk deer. Front. Microbiol. 12:699797. doi: 10.3389/fmicb.2021.699797
Jiang, A., Qin, C., and Huang, L. (2021). Field Guide to the Birds of Guangxi. Guangxi: Guangxi Science and Technology Press.
Jiménez, D. J., Dini-Andreote, F., and van Elsas, J. D. (2014). Metataxonomic profiling and prediction of functional behaviour of wheat straw degrading microbial consortia. Biotechnol. Biofuels Bioprod. 7:92. doi: 10.1186/1754-6834-7-92
Jo, S., Shin, C., Shin, Y., Kim, P. H., Park, J. I., Kim, M., et al. (2020). Heavy metal and antibiotic co-resistance in Vibrio parahaemolyticus isolated from shellfish. Mar. Pollut. Bull. 156:111246. doi: 10.1016/j.marpolbul.2020.111246
Jobson, J. D. (1991). “Analysis of variance and experimental design” in Applied Multivariate Data Analysis: Regression and Experimental Design. ed. J. D. Jobson (New York, NY: Springer New York), 399–544.
Jumpertz, R., Le, D. S., Turnbaugh, P. J., Trinidad, C., Bogardus, C., Gordon, J. I., et al. (2011). Energy-balance studies reveal associations between gut microbes, caloric load, and nutrient absorption in humans. Am. J. Clin. Nutr. 94, 58–65. doi: 10.3945/ajcn.110.010132
Kakade, A., Salama, E.-S., Pengya, F., Liu, P., and Li, X. (2020). Long-term exposure of high concentration heavy metals induced toxicity, fatality, and gut microbial dysbiosis in common carp, Cyprinus carpio. Environ. Pollut 266:115293. doi: 10.1016/j.envpol.2020.115293
Katoh, K., Misawa, K., Kuma, K. I., and Miyata, T. (2002). MAFFT: a novel method for rapid multiple sequence alignment based on fast Fourier transform. Nucleic Acids Res. 30, 3059–3066. doi: 10.1093/nar/gkf436
Kembel, S. W., Cowan, P. D., Helmus, M. R., Cornwell, W. K., Morlon, H., Ackerly, D. D., et al. (2010). Picante: R tools for integrating phylogenies and ecology. Bioinformatics 26, 1463–1464. doi: 10.1093/bioinformatics/btq166
Knight, R., Vrbanac, A., Taylor, B. C., Aksenov, A., Callewaert, C., Debelius, J., et al. (2018). Best practices for analysing microbiomes. Nat. Rev. Microbiol. 16, 410–422. doi: 10.1038/s41579-018-0029-9
Kohl, K. D., Weiss, R. B., Cox, J., Dale, C., and Denise Dearing, M. (2014). Gut microbes of mammalian herbivores facilitate intake of plant toxins. Ecol. Lett. 17, 1238–1246. doi: 10.1111/ele.12329
Krishnamoorthy, R., Kim, K., Subramanian, P., Senthilkumar, M., Anandham, R., and Sa, T. (2016). Arbuscular mycorrhizal fungi and associated bacteria isolated from salt-affected soil enhances the tolerance of maize to salinity in coastal reclamation soil. Agric. Ecosyst. Environ. 231, 233–239. doi: 10.1016/j.agee.2016.05.037
Krzywinski, M. I., Schein, J. E., Birol, I., Connors, J., Gascoyne, R., Horsman, D., et al. (2009). Circos: an information aesthetic for comparative genomics. Genome Res. 19, 1639–1645. doi: 10.1101/gr.092759.109
Lee, H., Kim, D.-U., Park, S., Yoon, J.-H., and Ka, J.-O. (2017). Massilia chloroacetimidivorans sp. nov., a chloroacetamide herbicide-degrading bacterium isolated from soil. Antonie Van Leeuwenhoek 110, 751–758. doi: 10.1007/s10482-017-0845-3
Li, L., and Jiang, Z. (2014). International trade of CITES listed bird species in China. PLoS One 9:e85012. doi: 10.1371/journal.pone.0085012
Liu, C., Lin, H., Li, B., Dong, Y., and Gueret Yadiberet Menzembere, E. R. (2021). Endophyte pseudomonas putida enhanced Trifolium repens L. growth and heavy metal uptake: a promising in-situ non-soil cover phytoremediation method of nonferrous metallic tailing. Chemosphere 272:129816. doi: 10.1016/j.chemosphere.2021.129816
Lozupone, C., Lladser, M. E., Knights, D., Stombaugh, J., and Knight, R. (2011). UniFrac: an effective distance metric for microbial community comparison. ISME J. 5, 169–172. doi: 10.1038/ismej.2010.133
McFall-Ngai, M., Hadfield, M. G., Bosch, T. C. G., Carey, H. V., Domazet-Lošo, T., Douglas, A. E., et al. (2013). Animals in a bacterial world, a new imperative for the life sciences. Proc. Nat1. Acad. Sci. U.S.A. 110, 3229–3236. doi: 10.1073/pnas.1218525110
Mirarab, S., Nguyen, N., and Warnow, T. (2012). SEPP: SATé-enabled phylogenetic placement. Pac Symp Biocomput, 247–258. doi: 10.1142/9789814366496_0024
Morais, L. H., Schreiber, H. L., and Mazmanian, S. K. (2021). The gut microbiota–brain axis in behaviour and brain disorders. Nat. Rev. Microbiol. 19, 241–255. doi: 10.1038/s41579-020-00460-0
Moszak, M., Szulińska, M., and Bogdański, P. (2020). You are what you eat—the relationship between diet, microbiota, and metabolic disorders—a review. Nutrients 12:1096. doi: 10.3390/nu12041096
Mukherjee, P., Mitra, A., and Roy, M. (2019). Halomonas Rhizobacteria of Avicennia marina of Indian Sundarbans promote rice growth under saline and heavy metal stresses through exopolysaccharide production. Front. Microbiol. 10:1207. doi: 10.3389/fmicb.2019.01207
Ni, Q., He, X., Zeng, B., Meng, X., Xu, H., Li, Y., et al. (2020). Variation in gut microbiota of captive Bengal slow Lorises. Curr. Microbiol. 77, 2623–2632. doi: 10.1007/s00284-020-02035-x
Ninkov, M., Popov Aleksandrov, A., Demenesku, J., Mirkov, I., Mileusnic, D., Petrovic, A., et al. (2015). Toxicity of oral cadmium intake: impact on gut immunity. Toxicol. Lett. 237, 89–99. doi: 10.1016/j.toxlet.2015.06.002
Oliveira, B. C. M., Murray, M., Tseng, F., and Widmer, G. (2020). The fecal microbiota of wild and captive raptors. Anim Microbiome. 2:15. doi: 10.1186/s42523-020-00035-7
Pan, J. Q., Luo, Q. W., Huang, Z. J., Qian, P. R., Huang, J. L., Wei, Q. S., et al. (2020). Analysis of cadmium pollution in Xijiang River basin of Guangxi (in Chinese with English abstract). Technol. Innov. Appl. 2, 61–63.
Peters, B. A., Yi, S. S., Beasley, J. M., Cobbs, E. N., Choi, H. S., Beggs, D. B., et al. (2020). US nativity and dietary acculturation impact the gut microbiome in a diverse US population. ISME J. 14, 1639–1650. doi: 10.1038/s41396-020-0630-6
Pramanik, K., Mandal, S., Banerjee, S., Ghosh, A., Maiti, T. K., and Mandal, N. C. (2021). Unraveling the heavy metal resistance and biocontrol potential of pseudomonas sp. K32 strain facilitating rice seedling growth under cd stress. Chemosphere 274:129819. doi: 10.1016/j.chemosphere.2021.129819
Quast, C., Pruesse, E., Yilmaz, P., Gerken, J., Schweer, T., Yarza, P., et al. (2012). The SILVA ribosomal RNA gene database project: improved data processing and web-based tools. Nucleic Acids Res. 41, D590–D596. doi: 10.1093/nar/gks1219
R Core Team (2021). R: A Language and Environment for Statistical Computing. R Foundation for Statistical Computing, Vienna, Austria. https://www.R-project.org/
Raivo, K. (2019). Pheatmap: Pretty Heatmaps. R package version 1.0.12. https://CRAN.R-project.org/package=pheatmap
Ramette, A. (2007). Multivariate analyses in microbial ecology. FEMS Microbiol. Ecol. 62, 142–160. doi: 10.1111/j.1574-6941.2007.00375.x
Risely, A., Waite, D. W., Ujvari, B., Hoye, B. J., and Klaassen, M. (2018). Active migration is associated with specific and consistent changes to gut microbiota in Calidris shorebirds. J. Anim. Ecol. 87, 428–437. doi: 10.1111/1365-2656.12784
Risely, A., Waite, D., Ujvari, B., Klaassen, M., and Hoye, B. (2017). Gut microbiota of a long-distance migrant demonstrates resistance against environmental microbe incursions. Mol. Ecol. 26, 5842–5854. doi: 10.1111/mec.14326
Rosenberg, E., and Zilber-Rosenberg, I. (2018). The hologenome concept of evolution after 10 years. Microbiome 6:78. doi: 10.1186/s40168-018-0457-9
San Juan, P. A., Castro, I., and Dhami, M. K. (2021). Captivity reduces diversity and shifts composition of the Brown kiwi microbiome. Anim Microbiome. 3:48. doi: 10.1186/s42523-021-00109-0
Santos, F. C. D., and Barbosa-Tessmann, I. P. (2019). Recombinant expression, purification, and characterization of a cyclodextrinase from Massilia timonae. Protein Expr. Purif. 154, 74–84. doi: 10.1016/j.pep.2018.08.013
Segata, N., Izard, J., Waldron, L., Gevers, D., Miropolsky, L., Garrett, W. S., et al. (2011). Metagenomic biomarker discovery and explanation. Genome Biol. 12:R60. doi: 10.1186/gb-2011-12-6-r60
Simberloff, D., Martin, J.-L., Genovesi, P., Maris, V., Wardle, D. A., Aronson, J., et al. (2013). Impacts of biological invasions: what's what and the way forward. Trends Ecol. Evol. 28, 58–66. doi: 10.1016/j.tree.2012.07.013
Šrut, M., Menke, S., Höckner, M., and Sommer, S. (2019). Earthworms and cadmium – heavy metal resistant gut bacteria as indicators for heavy metal pollution in soils? Ecotoxicol. Environ. Saf. 171, 843–853. doi: 10.1016/j.ecoenv.2018.12.102
Sun, C.-H., Liu, H.-Y., Zhang, Y., and Lu, C.-H. (2019). Comparative analysis of the gut microbiota of hornbill and toucan in captivity. MicrobiologyOpen 8:e00786:e786. doi: 10.1002/mbo3.786
Sun, W., Zhu, B., Yang, F., Dai, M., Sehar, S., Peng, C., et al. (2021). Optimization of biosurfactant production from pseudomonas sp. CQ2 and its application for remediation of heavy metal contaminated soil. Chemosphere 265:129090. doi: 10.1016/j.chemosphere.2020.129090
Tajuddin Sikder, M., Tanaka, S., Saito, T., and Kurasaki, M. (2014). Application of zerovalent iron impregnated chitosan-caboxymethyl-β-cyclodextrin composite beads as arsenic sorbent. J. Environ. Chem. Eng. 2, 370–376. doi: 10.1016/j.jece.2014.01.009
Vangay, P., Johnson, A. J., Ward, T. L., Al-Ghalith, G. A., Shields-Cutler, R. R., Hillmann, B. M., et al. (2018). US immigration westernizes the human gut microbiome. Cells 175, 962–972.e10. doi: 10.1016/j.cell.2018.10.029
Waite, D. W., and Taylor, M. W. (2014). Characterizing the avian gut microbiota: membership, driving influences, and potential function. Front. Microbiol. 5:223. doi: 10.3389/fmicb.2014.00223
Wang, L., Wang, L. A., Zhan, X., Huang, Y., Wang, J., and Wang, X. (2020). Response mechanism of microbial community to the environmental stress caused by the different mercury concentration in soils. Ecotoxicol. Environ. Saf. 188:109906. doi: 10.1016/j.ecoenv.2019.109906
Wang, W., Zheng, S., Sharshov, K., Sun, H., Yang, F., Wang, X., et al. (2017). Metagenomic profiling of gut microbial communities in both wild and artificially reared bar-headed goose (Anser indicus). MicrobiologyOpen 6:e00429. doi: 10.1002/mbo3.429
Wickham, H. (2016). ggplot2: Elegant Graphics for Data Analysis. New York, NY: Springer. https://ggplot2.tidyverse.org
Wienemann, T., Schmitt-Wagner, D., Meuser, K., Segelbacher, G., Schink, B., Brune, A., et al. (2011). The bacterial microbiota in the ceca of Capercaillie (Tetrao urogallus) differs between wild and captive birds. Syst. Appl. Microbiol. 34, 542–551. doi: 10.1016/j.syapm.2011.06.003
Wu, N., Wang, X., Xu, X., Cai, R., and Xie, S. (2020). Effects of heavy metals on the bioaccumulation, excretion and gut microbiome of black soldier fly larvae (Hermetia illucens). Ecotoxicol. Environ. Saf. 192:110323. doi: 10.1016/j.ecoenv.2020.110323
Wu, H., Wu, F.-T., Zhou, Q.-H., and Zhao, D.-P. (2021). Comparative analysis of gut microbiota in captive and wild oriental white storks: implications for conservation biology. Front. Microbiol. 12:649466. doi: 10.3389/fmicb.2021.649466
Youngblut, N. D., Reischer, G. H., Walters, W., Schuster, N., Walzer, C., Stalder, G., et al. (2019). Host diet and evolutionary history explain different aspects of gut microbiome diversity among vertebrate clades. Nat. Commun. 10:2200. doi: 10.1038/s41467-019-10191-3
Zhang, C., Wang, X., Ashraf, U., Qiu, B., and Ali, S. (2017). Transfer of lead (Pb) in the soil-plant-mealybug-ladybird beetle food chain, a comparison between two host plants. Ecotoxicol. Environ. Saf. 143, 289–295. doi: 10.1016/j.ecoenv.2017.05.032
Zhang, K., Wang, X., Gong, X., and Sui, J. (2022). Gut microbiome differences in rescued common kestrels (Falco tinnunculus) before and after captivity. Front. Microbiol. 13:858592. doi: 10.3389/fmicb.2022.858592
Zhou, L., Huo, X., Liu, B., Wu, H., and Feng, J. (2020). Comparative analysis of the gut microbial communities of the Eurasian kestrel (Falco tinnunculus) at different developmental stages. Front. Microbiol. 11:592539. doi: 10.3389/fmicb.2020.592539
Keywords: gut microbiota, Leiothrix argentauris, trace element, immigrant subspecies, native subspecies
Citation: Zhou T, Liu S and Jiang A (2023) Comparison of gut microbiota between immigrant and native populations of the Silver-eared Mesia (Leiothrix argentauris) living in mining area. Front. Microbiol. 14:1076523. doi: 10.3389/fmicb.2023.1076523
Edited by:
Mohamed T. El-Saadony, Zagazig University, EgyptReviewed by:
Mohsen Almutary, Imam Abdulrahman Bin Faisal University, Saudi ArabiaAhmed M. Saad, Zagazig University, Egypt
Copyright © 2023 Zhou, Liu and Jiang. This is an open-access article distributed under the terms of the Creative Commons Attribution License (CC BY). The use, distribution or reproduction in other forums is permitted, provided the original author(s) and the copyright owner(s) are credited and that the original publication in this journal is cited, in accordance with accepted academic practice. No use, distribution or reproduction is permitted which does not comply with these terms.
*Correspondence: Aiwu Jiang, aiwuu@gxu.edu.cn