- 1Department of Periodontology, National Engineering Laboratory for Digital and Material Technology of Stomatology, NHC Research Center of Engineering and Technology for Computerized Dentistry, National Clinical Research Center for Oral Diseases, Peking University School and Hospital of Stomatology, Beijing, China
- 2Beijing Tiantan Hospital, Capital Medical University, Beijing, China
- 3Laboratory of Environmental Microbiology, Department of Energy and Resources Engineering, College of Engineering, Peking University, Beijing, China
This pilot study was designed to identify the salivary microbial community and metabolic characteristics in patients with generalized periodontitis. A total of 36 saliva samples were collected from 13 patients with aggressive periodontitis (AgP), 13 patients with chronic periodontitis (ChP), and 10 subjects with periodontal health (PH). The microbiome was evaluated using 16S rRNA gene high-throughput sequencing, and the metabolome was accessed using gas chromatography-mass spectrometry. The correlation between microbiomes and metabolomics was analyzed by Spearman’s correlation method. Our results revealed that the salivary microbial community and metabolite composition differed significantly between patients with periodontitis and healthy controls. Striking differences were found in the composition of salivary metabolites between AgP and ChP. The genera Treponema, Peptococcus, Catonella, Desulfobulbus, Peptostreptococcaceae_[XI] ([G-2], [G-3] [G-4], [G-6], and [G-9]), Bacteroidetes_[G-5], TM7_[G-5], Dialister, Eikenella, Fretibacterium, and Filifactor were present in higher levels in patients with periodontitis than in the healthy participants. The biochemical pathways that were significantly different between ChP and AgP included pyrimidine metabolism; alanine, aspartate, and glutamate metabolism; beta-alanine metabolism; citrate cycle; and arginine and proline metabolism. The differential metabolites between ChP and AgP groups, such as urea, beta-alanine, 3-aminoisobutyric acid, and thymine, showed the most significant correlations with the genera. These differential microorganisms and metabolites may be used as potential biomarkers to monitor the occurrence and development of periodontitis through the utilization of non-invasive and convenient saliva samples. This study reveals the integration of salivary microbial data and metabolomic data, which provides a foundation to further explore the potential mechanism of periodontitis.
Introduction
Periodontitis, which causes alveolar bone resorption, is inflammation of periodontal tissue that results from the host immune response to bacterial infection (Marchesan et al., 2020). Periodontitis is the most common cause of tooth loss and can subsequently impair the quality of life (Petersen, 2003). Aggressive periodontitis (AgP) refers to rapidly progressing periodontitis that usually occurs in younger patients and differs from chronic periodontitis (ChP) according to the 1999 Classification of Periodontal Diseases and Condition (Armitage, 1999; Armitage, 2000). AgP constitutes a disease that causes considerable morbidity because of its pronounced destruction, which may lead to edentulism early in life. Some studies reported that AgP differs from ChP due to the age of the onset of the disease, rapid progression, the severity of disease, structure of subgingival flora, differences in host response, changes in response to treatment, and familial transmission characteristics of the disease (Emingil et al., 2007; Armitage and Cullinan, 2010).
Saliva is particularly promising as it contains locally produced proteins, as well as other components from the systemic circulation (Buduneli and Kinane, 2011). Moreover, saliva samples can be collected in an easy, non-invasive, repeated, minimal time-consuming manner. Salivary diagnostics has proved to be a promising substrate for the early detection of oral and systemic diseases (Buzalaf et al., 2020; Martina et al., 2020; Hyvarinen et al., 2021; Khurshid et al., 2021). Even so, various challenges persist regarding the use of saliva as a potential diagnostic media for periodontitis, mainly because of the lack of specific markers of the disease.
The microorganisms are believed to be involved in the pathogenesis of periodontitis. Moreover, it is now clear that some important factors that connect microbiota to periodontitis are microbial metabolites (Lu et al., 2013; Duran-Pinedo and Frias-Lopez, 2015). A few studies have used next-generation sequencing technologies to characterize the salivary microbiota in patients with AgP or ChP (Damgaard et al., 2019; Lundmark et al., 2019). These results revealed distinct and disease-specific patterns of salivary microbial composition between patients with periodontitis and healthy controls. Furthermore, several interventional studies have demonstrated the impact of non-surgical periodontal treatment on the composition of the salivary microbiome (Belstrom et al., 2018; Chen et al., 2018; Greenwood et al., 2020). It was reported that the salivary levels of periopathogens might be used as a biomarker of periodontitis (Yoshizawa et al., 2013; Kageyama et al., 2017).
The salivary metabolome is a rapidly evolving discipline aiming to reflect the real-time molecular phenotype of oral health (Liebsch et al., 2019; Gardner et al., 2020). The metabolic profile of saliva can give valuable information on the complex pathogenic mechanisms of periodontitis (Rzeznik et al., 2017; Romano et al., 2018; Kim et al., 2021). Several studies have shown its potential to reflect periodontal inflammation severity (Kuboniwa et al., 2016; Sakanaka et al., 2017). Also, non-surgical periodontal therapy may produce significant changes in the metabolic profile (Romano et al., 2019; Citterio et al., 2020).
Although increasing evidence is emerging on the variation in the salivary microbiota and metabolic characteristics of periodontitis, respectively, the relationship between host bacterial interactions and biochemical metabolism has not been identified. Thus, this pilot study was designed to identify the salivary microbial community and metabolic profiles in patients with generalized AgP and ChP, and subjects with periodontal health (PH) using 16S rRNA gene high-throughput sequencing and gas chromatography-mass spectrometry (GC-MS). The integration of microbiome and metabolomics can effectively provide a new sight regarding the influence of periodontitis.
Materials and methods
Study population and clinical examination
Ethics approval for this study was granted by the Ethics Committee of the Peking University Health Science Center (approval number: PKUSSIRB-201631135). All participants were individually informed and signed informed consent forms in accordance with the Declaration of Helsinki.
From January 2017 to August 2017, 13 patients with AgP and 13 patients with ChP were recruited from Peking University School and Hospital of Stomatology, and 10 PH subjects were selected as controls. The clinical inclusion criteria for PH and the diagnostic criteria for ChP and AgP have been previously described in detail (Shi et al., 2018). Exclusion criteria included systemic diseases, use of antibiotics or any other drugs that were known to affect periodontal conditions within the past 3 months, periodontal treatment in the past 6 months, a remaining tooth with caries, pregnancy or lactation, and smoking.
Full-mouth periodontal indices, including probing depth (PD), attachment loss (AL), and bleeding index (BI) (Mazza et al., 1981), were recorded at six sites per tooth by one practitioner (WH). His reliability was calibrated as described by Shi et al. (2018). All patients fulfilled the categorization of generalized Stage III or IV, Grade C periodontitis, according to the new periodontitis classification system (Tonetti et al., 2018).
Sample collection
One week after the full-mouth periodontal examination, saliva samples were collected in the morning (around 8 a.m. to 9 a.m.). All subjects were restrained from food for 8 h and oral hygiene for 12 h before sampling. Each subject was instructed to allow saliva to be collected in the mouth, and let the saliva drain into a sterile graduated tube for 10 min. About 3 ml of unstimulated whole saliva was collected. After centrifugation, the saliva was immediately frozen at –80°C.
Microbial DNA extraction and 16S rRNA gene sequencing
The bacterial DNA of saliva samples was extracted using the QIAamp DNA Mini Kit (QIAGEN Sciences, USA). Polymerase chain reaction amplification was performed using specific primers, 338F (5′, ACTCCTACGGGAGGCAGCAG, 3′) and 806R (5′, GGACTACNNGGGTATCTAAT, 3′), targeting the V4–V5 region of the bacterial 16S rRNA gene. Thermal cycling conditions were as follows: initial denaturation at 95°C for 3 min, followed by 24 cycles of denaturation at 95°C for 30 s, annealing at 56°C for 30 s, elongation at 72°C for 45 s, and finally at 72°C for 10 min. Purified amplicons were pooled in equimolar and paired-end sequenced using the Illumina MiSeq PE300 platform (Illumina, San Diego, USA). The datasets generated and analyzed during the current study are available from the NCBI Sequence Read Archive database (Accession Number: SRP173111; SRP228020).
Gas chromatography-mass spectrometry metabolomics processing
Gas chromatography-mass spectrometry (GC-MS) was performed as described in a previous study (Shi et al., 2020). Briefly, 300 μL of methanol was used for extraction, following the addition of 20 μL of 2-chloro-l-phenylalanine (0.3 mg/mL) as an internal standard. All samples were analyzed with an Agilent Gas Chromatography Mass Spectrometer (7890A/5975C GC-MS System, Agilent, CA, USA). The GC system employed a chromatographic column (HP-5MS) (30 m × 0.25 mm × 0.25 μm, Agilent J & W Scientific, Folsom, CA, USA).
Bioinformatic analysis, statistical analysis, and visualization
The sequences were analyzed using the Quantitative Insights Into Microbial Ecology (QIIME) software package, and alpha and beta diversity values were determined. After the OTU table was rarified, alpha diversity and beta diversity calculations were performed. Analysis of similarity (ANOSIM), permutational multivariate analysis of variance (Adonis), and multi-response permutation procedure (MRPP) were used to examine the differences in the microbial community structure between the groups. The composition and abundance depicted in the taxonomy profile were visualized by GraphPad PRISM® software (version 4.0). Normality tests for each group of data were conducted. The Kruskal–Wallis test, Mann–Whitney test, and Student’s t-tests were performed using SPSS 20.0.
The raw data obtained in .D format from the platform were converted to .CDF format by using ChemStation analysis software (version E.02.02.1431, Agilent, CA, USA), and then imported into Chroma TOF software (version 4.34, LECO, St Joseph) for preprocessing. The remaining steps were carried out as described in the literature (Shi et al., 2020). Unsupervised principal component analysis (PCA) and (orthogonal) partial least-squares analysis [(O) PLS-DA] were then applied to determine the differences in metabolic profiles between the groups. In the (O)PLS-DA analysis, variable importance in the projection (VIP) value greater than 1 and p-values (two-tailed Student’s t-test) less than 0.05 were considered to be different variables. Metabolites were then identified by searching in a self-built database of the Majorbio I-Sanger Cloud Platform,1 which integrated the data of the Human Metabolome Database, KEGG Compound Database, and Lipid Maps Structure Database.
The correlation between microbiomes and metabolomics was analyzed by the Spearman method. A heat map of Spearman’s rank correlation coefficient was used to illustrate the relationship between microbial communities and metabolites.
Results
Microbial signatures of saliva samples
The demographic characteristics and clinical parameters are presented in Table 1. A total of 36 subjects, including 13 patients with AgP, 13 patients with ChP, and 10 PH, were included in this study. PD, AL, and BI values were significantly higher in the group with periodontitis (AgP and ChP) than in the PH subjects (p < 0.05). The mean age in the ChP group was significantly higher than in the other two groups.
Sequences were clustered to 888 OTUs based on 97% sequence similarity. A total of 12 phyla, 30 classes, 54 orders, 92 families, and 173 genera were detected. The rarefaction curves suggested that the sequencing depth was adequate (Supplementary Figure 1).
In the AgP, ChP, and PH groups, the five most abundant phyla were Firmicutes, Bacteroidetes, Proteobacteria, Fusobacteria, and Actinobacteria, which represented more than 97% of the total sequences (Figure 1A). At the genus level, Streptococcus, Prevotella, Neisseria, Fusobacterium, Haemophilus, Veillonella, Actinomyces, Rothia, Porphyromonas, Gemella, Peptostreptococcus, Granulicatella, Leptotrichia, Parvimonas, and Capnocytophaga accounted for more than 85% of the sequencing results in all saliva samples, though there were some differences in the precise proportions among the AgP, ChP, and PH groups (Figure 1B).
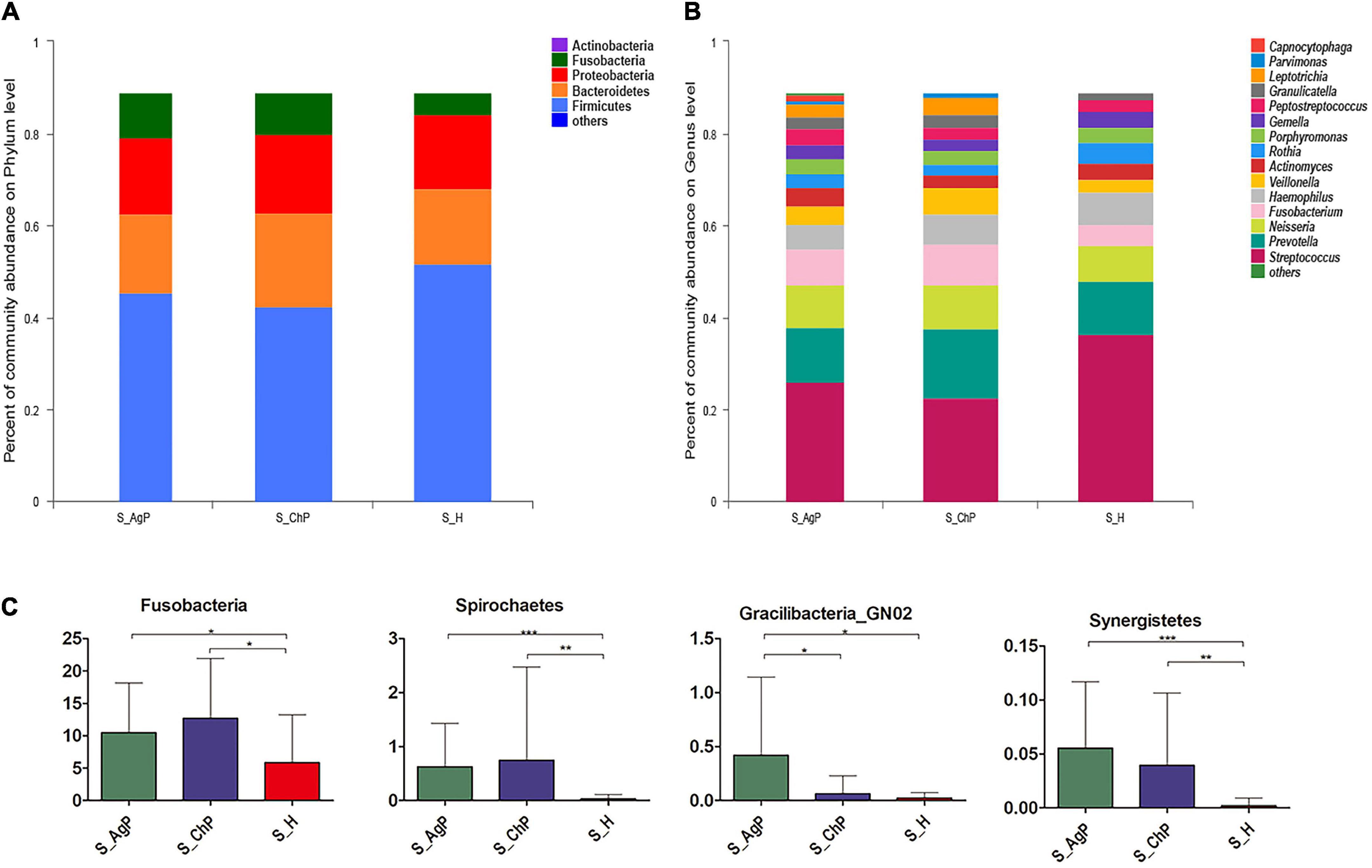
Figure 1. Bacterial composition of three groups. Microbial community composition at the phylum level (A) and genus level (B) of salivary samples in the aggressive periodontitis group (shown as S_AgP), chronic periodontitis group (shown as S_ChP), and healthy group (shown as S_PH). The relative abundance of bacterial taxa at the phylum level shows significant differences among groups (C). *p < 0.05; **p < 0.01; ***p < 0.001.
Then we compared the relative abundance of microbiota between samples from periodontitis and healthy subjects. The abundance of Fusobacteria, Spirochetes, and Synergistetes increased significantly in the periodontitis group. The abundance of Gracilibacteria_GN02 was significantly higher in AgP than in ChP and PH (Figure 1C). At the genus level, 43, 31, and 5 genera were distributed differently between AgP vs. PH, ChP vs. PH, and AgP vs. ChP, respectively (Supplementary Tables 1–3). Most significant differences were observed in the low-abundance genera. Leptotrichia, Treponema, Peptococcus, Catonella, Desulfobulbus, Peptostreptococcaceae_[XI] ([G-2], [G-3] [G-4], [G-6], and [G-9]), Bacteroidetes_[G-5], TM7_[G-5], Dialister, Eikenella, Filifactor, Fretibacterium, Lachnospiraceae_[G-8], Mollicutes_[G-1], and Slackia increased significantly both in the ChP and AgP groups when compared to the PH group. Agrobacterium, GN02_[G-2], GN02_[G-1], Mobiluncus, and Peptostreptococcaceae_[XI][G-6] showed significant differences between the AgP and ChP groups.
To illustrate the richness, evenness, and species diversity of the microbial community, α diversity indices, including the Chao index, ACE index, Shannon index, and Simpson index, were calculated. The results showed that the alpha diversity of subjects with periodontitis was greater than that observed in healthy individuals (Figure 2). The β diversity calculations based on Bray-Curtis, Jaccard, weighted, and unweighted UniFrac distances showed significant compositional shifts between periodontitis and healthy groups (Figure 3). ANOSIM, Adonis, and MRPP were used to further confirm that there was no significant difference between the AgP and ChP groups (Table 2).

Figure 2. Alpha diversity indexes of microbial communities in three groups. The alpha diversity of microbiota as calculated by ACE index, Chao index, Shannon index, and Simpson index of saliva samples in aggressive periodontitis group (S_AgP), chronic periodontitis group (S_ChP), and healthy group (S_PH). The error bars indicate mean with standard error. *p < 0.05; ***p < 0.001.
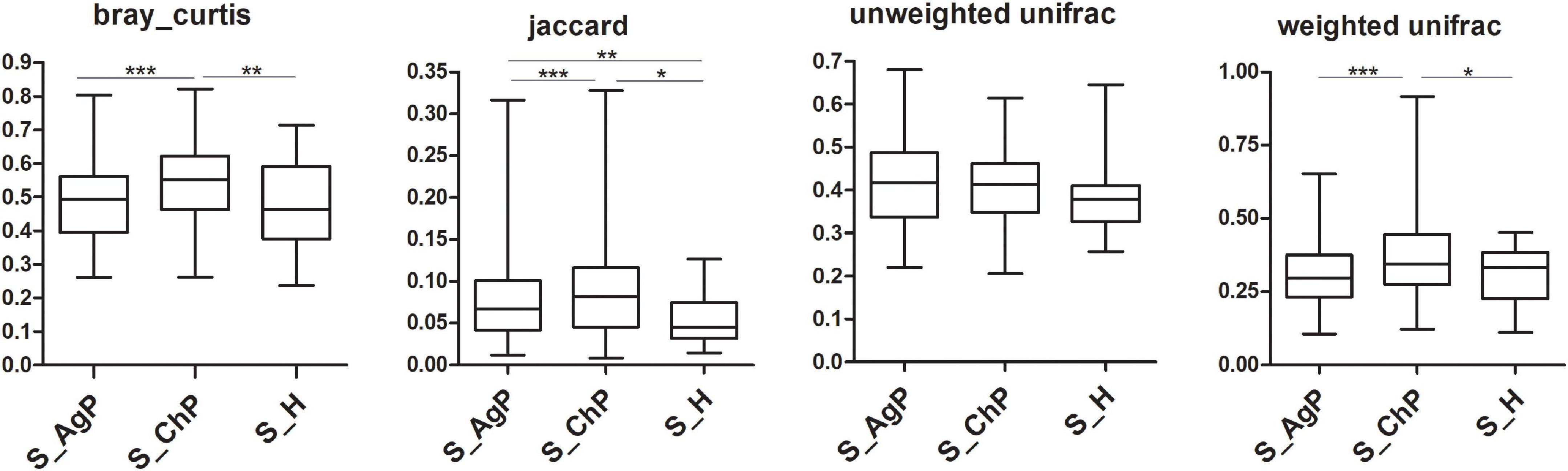
Figure 3. Beta diversity of saliva samples in three groups based on Bray-Curtis, Jaccard, weighted, and unweighted UniFrac distances. *p < 0.05; **p < 0.01; ***p < 0.001.
Metabolic signatures of saliva samples
Multivariate analyses between different groups were performed in PCA, PLS-DA, and OPLS-DA. In the plot of PCA scores, we noted a trend of divergence in the saliva sample among the three groups. The PLS-DA model demonstrated satisfactory modeling and predictive abilities. The OPLS-DA model demonstrated a distinct separation between the metabolite profiles of these groups (Figures 4–6). The dissimilarity tests, including ANOSIM, Adonis, and MRPP, indicated significant differences in metabolites among the three groups (Table 3).
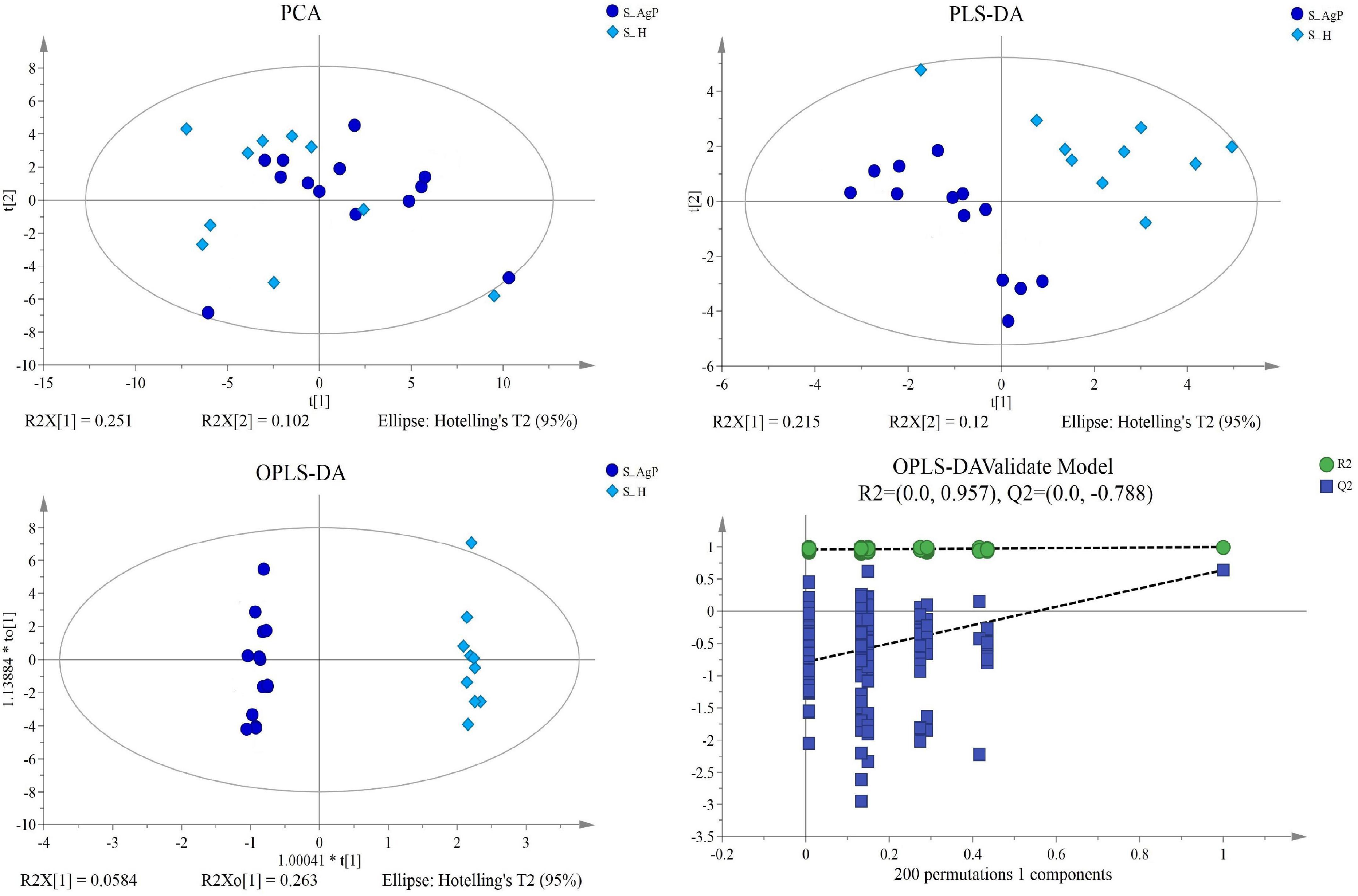
Figure 4. Dissimilarity in salivary metabolic signatures between AgP and PH. PCA analysis (A), PLS-DA analysis (B), OPLS-DA analysis (C), and response permutation testing of OPLS-DA (D). PCA, principal components analysis; PLS-DA, partial least-squares discriminant analysis; OPLS-DA, orthogonal partial least-squares discriminant analysis.
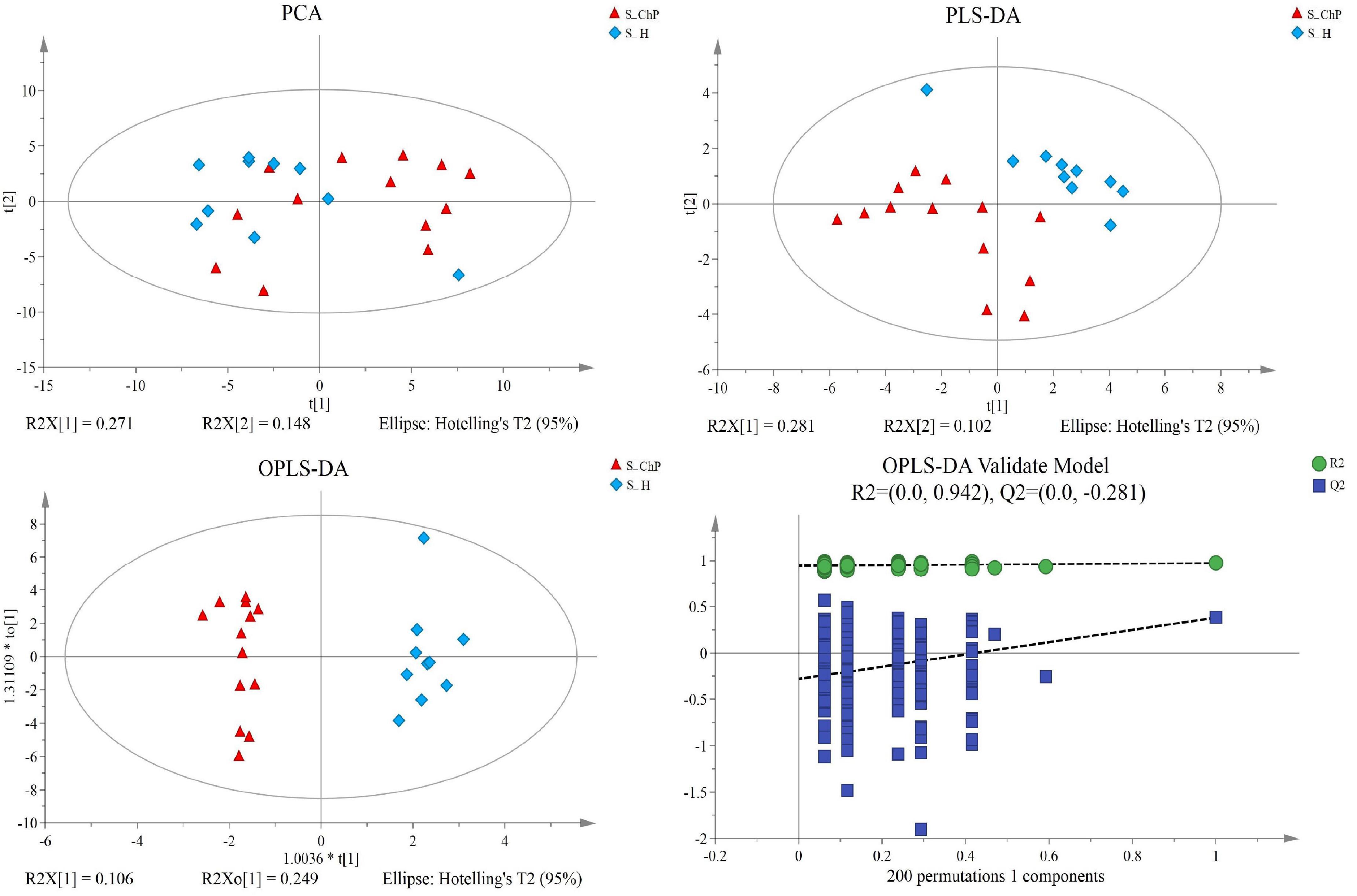
Figure 5. Dissimilarity of salivary metabolic signatures between ChP and PH. PCA analysis (A), PLS-DA analysis (B), OPLS-DA analysis (C), and response permutation testing of OPLS-DA (D).
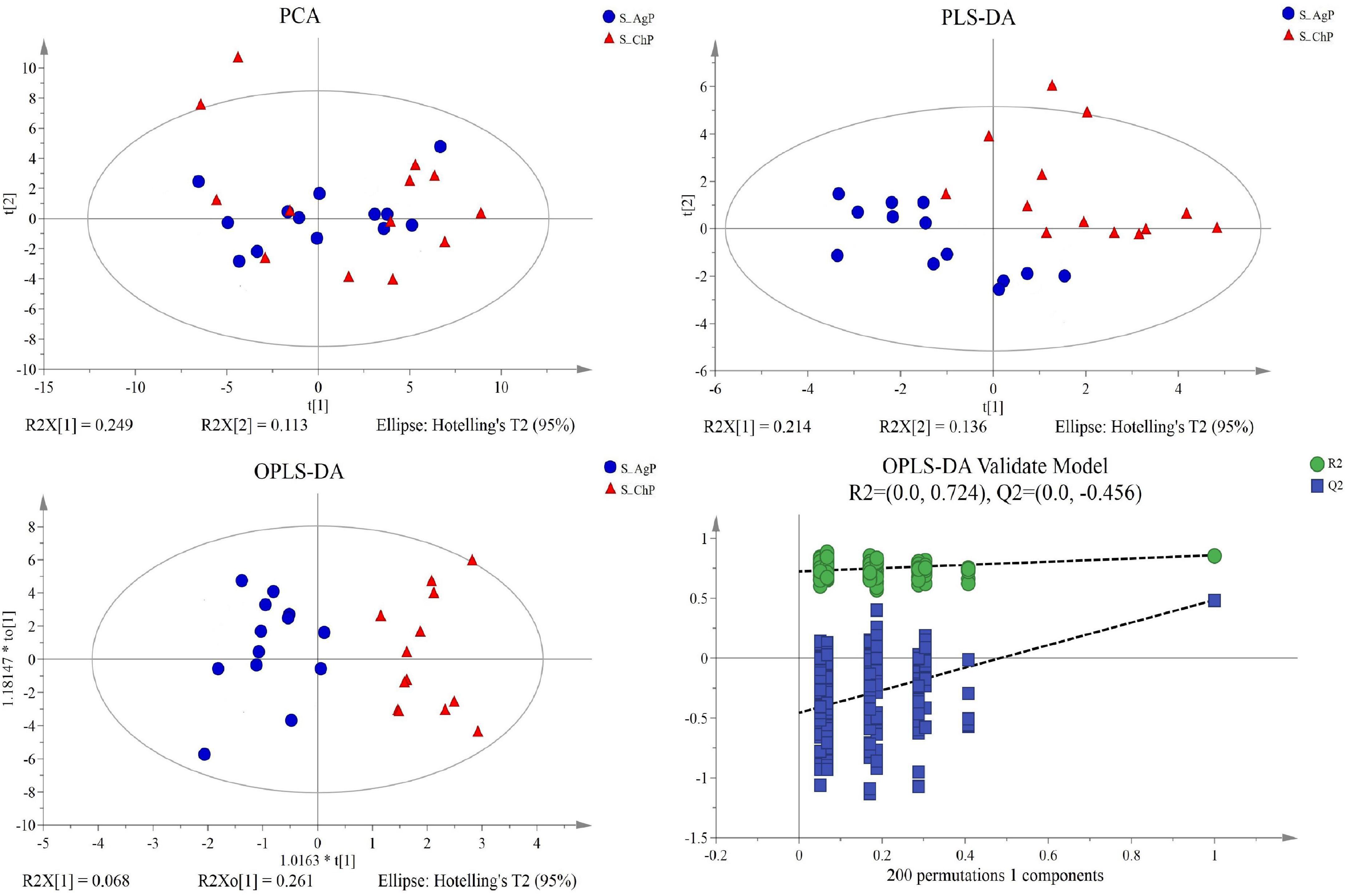
Figure 6. Dissimilarity in salivary metabolic signatures between AgP and ChP. PCA analysis (A), PLS-DA analysis (B), OPLS-DA analysis (C), and response permutation testing of OPLS-DA (D).
There were 13, 14, and 15 metabolites that were distributed differently between AgP vs. PH, ChP vs. PH, and AgP vs. ChP, respectively (Figure 7). Among these compounds, the levels of isoleucine, serotonin, hydrocinnamic acid, 4-hydroxycinnamic acid, serine, proline, stearic acid, palmitic acid, aspartate, and glycolic acid were significantly higher in AgP, while the levels of hypoxanthine, beta-alanine, and oleic acid were significantly higher in PH. Compared with PH, subjects with ChP are characterized by lower levels of urea, aminomalonate, and phosphoethanolamine, and higher levels of allantoin, isoleucine, hydrocinnamic acid, serotonin, threonine, 3-(4-hydroxyphenyl)propionic acid, 4-hydroxycinnamic acid, phenylacetic acid, 4-aminobutyric acid, serine, and lysine. Compared with ChP, the levels of biphenyl, stearic acid, palmitic acid, urea, and conduritol-beta-expoxide increased significantly in AgP, while the levels of thymine, fumaric acid, allantoin, beta-alanine, 2-hydroxyhexanoic acid, uracil, 3-aminoisobutyric acid, 4-aminobutyric acid, lysine, and citric acid were significantly decreased.
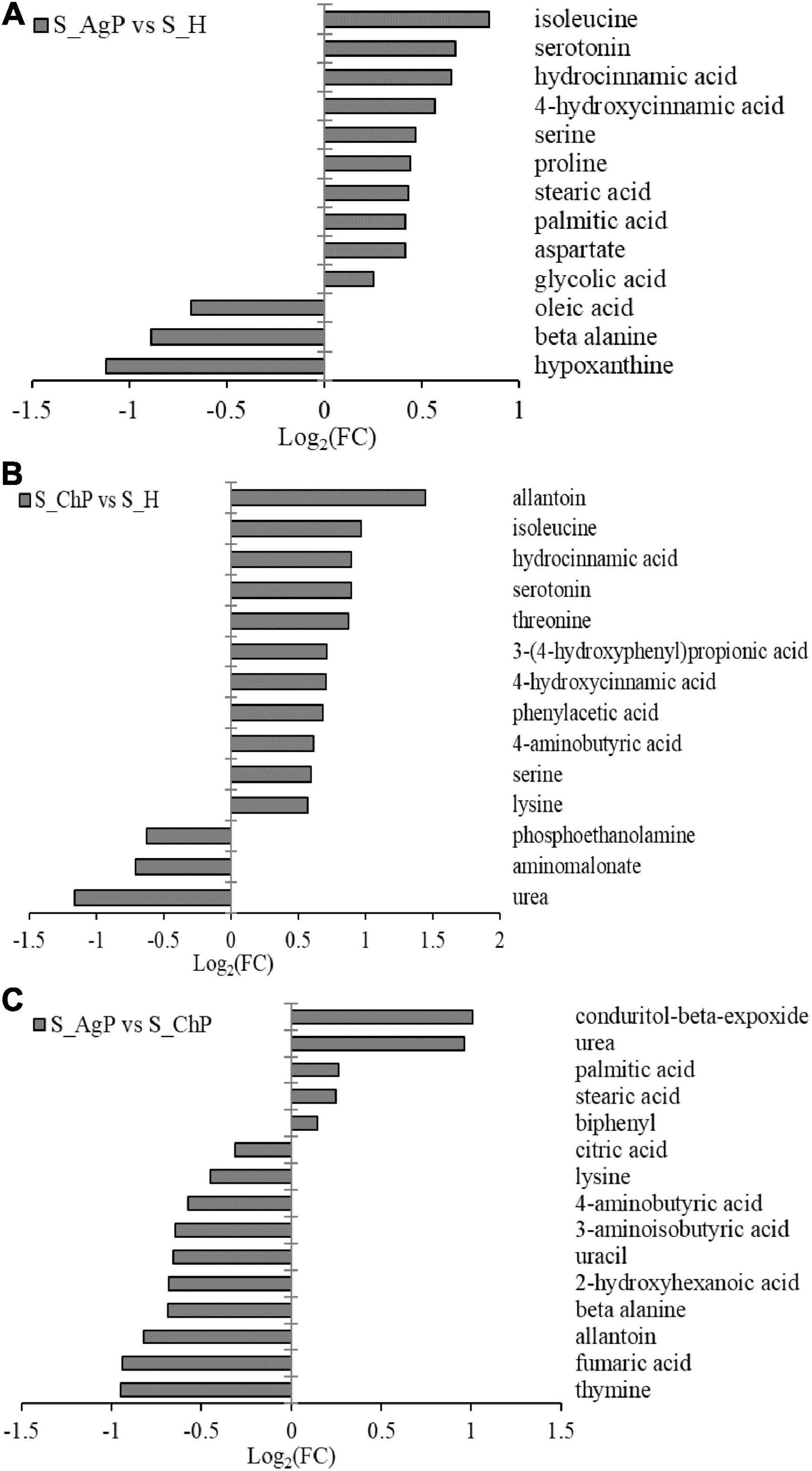
Figure 7. Significantly different metabolites between groups. Differential metabolites between AgP and PH. A positive value indicates a relatively higher concentration present in AgP, while a negative value means a relatively higher concentration in the PH (A), based on differential metabolites between ChP and PH. A positive value indicates a relatively higher concentration present in ChP, while a negative value means a relatively higher concentration in the PH (B), based on differential metabolites between AgP and ChP. A positive value indicates a relatively higher concentration present in AgP, while a negative value means a relatively higher concentration in the ChP (C).
Pathways and enrichment
Following the enrichment analysis of the significantly different metabolites, the key pathways with the highest correlation between metabolite differences were identified based on both raw p and impact values.
When the AgP and PH groups were compared, several pathways containing more types of differential metabolites were detected, including protein digestion and absorption (including beta-alanine, proline, isoleucine, aspartate, and serine), biosynthesis of antibiotics (including serine, proline, isoleucine, glycolic acid, and aspartate), biosynthesis of amino acids (including serine, proline, isoleucine, and aspartate), aminoacyl-tRNA biosynthesis (including serine, proline, isoleucine, and aspartate), and fatty acid biosynthesis (including palmitic acid, stearic acid, and oleic acid) (Figure 8A).
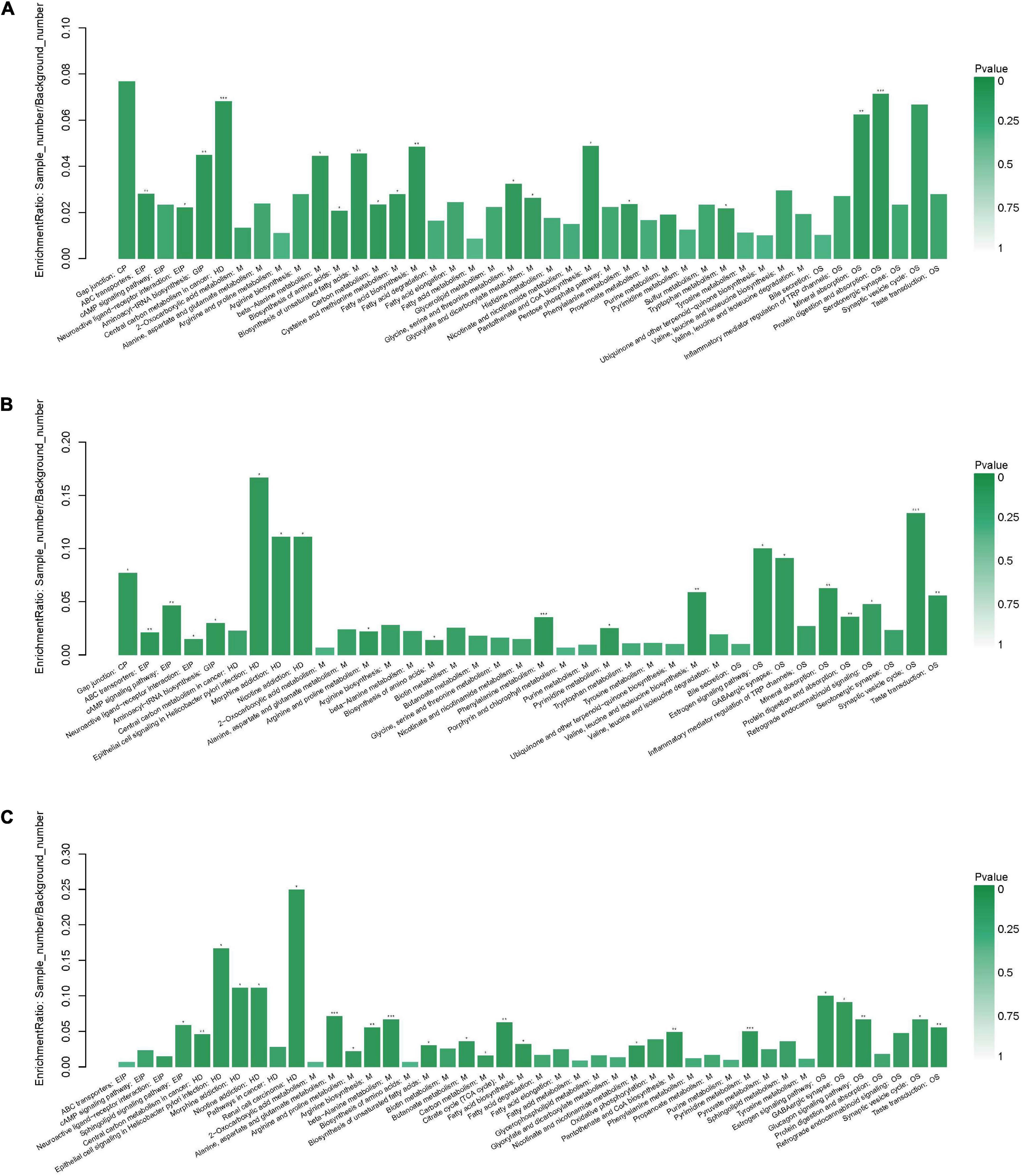
Figure 8. Functional enrichment analysis of differential metabolites between groups. Pathway analysis of salivary metabolites with significant difference between AgP and PH (A), between ChP and PH (B), and between AgP and ChP (C). The height of the column represents the enrichment rate. *p < 0.05, **p < 0.01, ***p < 0.001.
When the ChP and PH groups were compared, several pathways containing more types of differential metabolites were detected, including aminoacyl-tRNA biosynthesis (including serine, isoleucine, lysine, and threonine), biosynthesis of amino acids (including serine, isoleucine, lysine, and threonine), and biosynthesis of valine, leucine, and isoleucine (including isoleucine and threonine) (Figure 8B).
When the ChP and AgP groups were compared, several pathways containing more types of differential metabolites were identified, including pyrimidine metabolism (including uracil, urea, thymine, and beta-alanine); alanine, aspartate, and glutamate metabolism (including citric acid, fumaric acid, and 4-aminobutyric acid); beta-alanine metabolism (including uracil, beta-alanine, and 4-aminobutyric acid); citrate cycle (including citric acid and fumaric acid); and arginine and proline metabolism (including urea and 4-aminobutyric acid) (Figure 8C).
Association between microbial community and metabolome characteristics
Spearman correlation analysis was performed between the differential metabolites, the microbial diversity was analyzed at the phylum and genus levels, and the related network was constructed (| correlation r| > 0.25, p < 0.05).
At the phylum level, by comparing AgP and PH groups, we found that Actinobacteria and Fusobacteria were the most closely related to the metabolites (Supplementary Figure 2). At the genus level, Rothia, Streptococcus, Corynebacterium, Enterococcus, Lautropia, and Solobacterium were the genera with the most correlations with differential metabolites, which had significant correlations with 7, 6, 5, 5, 5, and 5 types of metabolites, respectively (Figure 9). Analysis from the perspective of metabolites showed that serotonin, oleic acid, proline, and hydrocinnamic acid had the most significant correlations with microorganisms, which were significantly correlated with 24, 20, 19, and 17 types of bacterial genera, respectively.
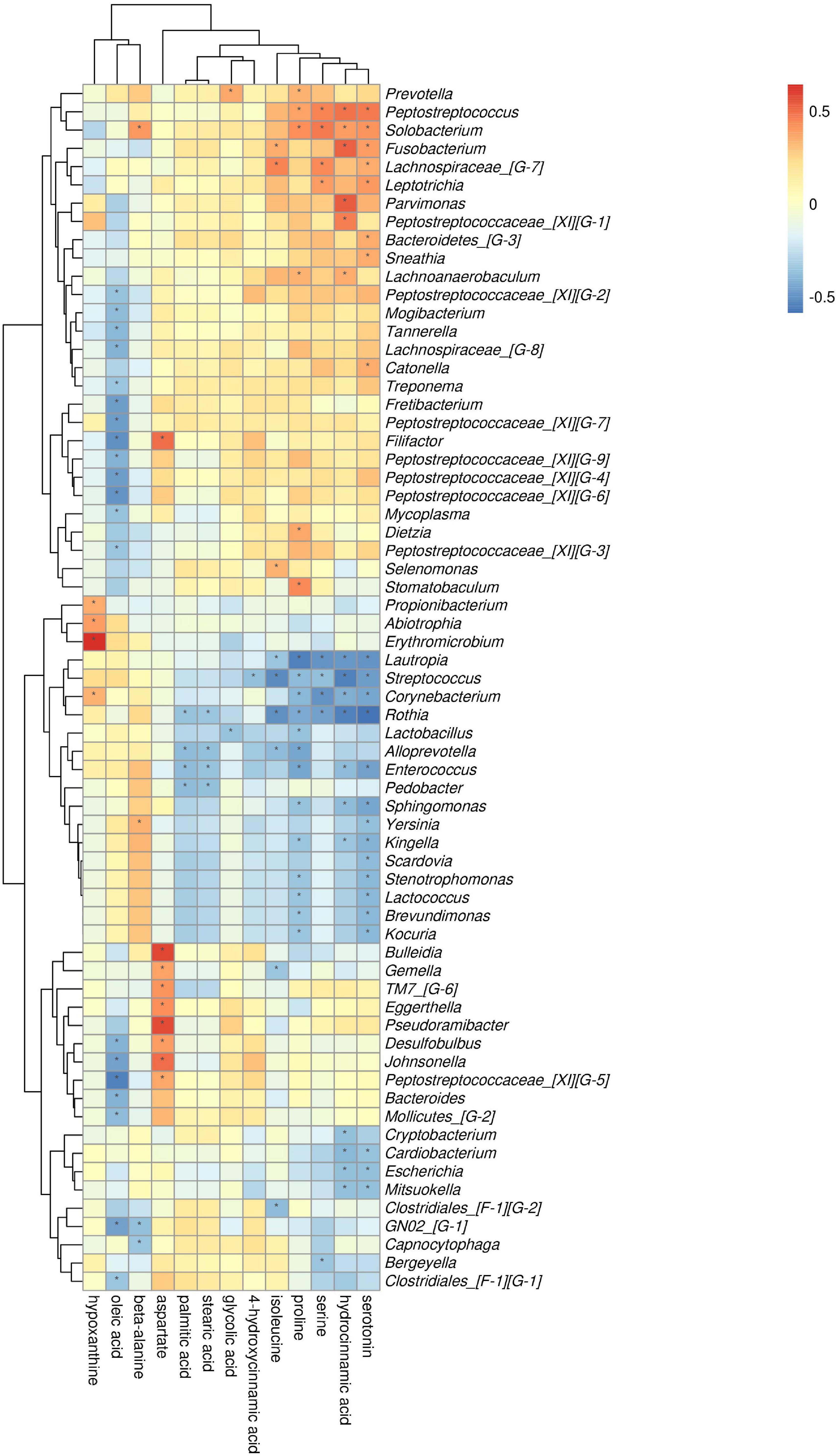
Figure 9. Association of microbial genera with differential metabolites in AgP and PH. Color intensity represents the magnitude of correlation. Red, positive correlations; blue, negative correlations. *Significant correlation between the genus and metabolite (p < 0.05).
The comparison of ChP and PH groups revealed that Firmicutes, Actinobacteria, and Fusobacteria were most closely related to the metabolites (Supplementary Figure 3). At the genus level, Streptococcus, Fusobacterium, and Gemella were the genera with the most correlations with differential metabolites, which had significant correlations with 13, 8, and 8 types of metabolites, respectively (Figure 10). Lysine, aminobutyric acid, hydrocinnamic acid, and phenylacetic acid had the most significant correlations with microorganisms, which were significantly correlated with 25, 17, 15, and 13 types of bacterial genera, respectively.
The comparison of ChP and AgP groups showed that Actinobacteria, Synergistetes, Fusobacteria, and Proteobacteria were the most closely related to the metabolites (Supplementary Figure 4). At the genus level, Rothia, Enterococcus, Rhizobium, and Haemophilus are the genera with the most correlations with differential metabolites, which had significant correlations with 8, 7, 6, and 6 types of metabolites, respectively (Figure 11). Urea, beta-alanine, 3-aminoisobutyric acid, and thymine had the most significant correlations with microorganisms, which were significantly correlated with 23, 22, 21, and 20 types of bacterial genera, respectively.
Discussion
Periodontitis is a biofilm-associated inflammatory condition that is associated with the activation of the host inflammatory response to bacterial challenge, which mainly includes chronic periodontitis and aggressive periodontitis. This pilot study provided a comprehensive and comparative analysis of the microbiome and metabolome of salivary samples from individuals with AgP, ChP, and PH.
As the present study was conducted between January 2017 and August 2017, the included patients were diagnosed with AgP or ChP, according to the 1999 classification system. When the new classification system was presented in 2018, the authors reclassified the groups according to the new classification system and revealed that all patients fully met the criteria for generalized Stage III or IV and Grade C periodontitis features. However, the terms “AgP” and “ChP” were retained, as the results of the present study were also compared with the previous studies on AgP and ChP.
Saliva is the most easily accessible and readily obtained biofluid, which is enriched with microbiota from the totality of the oral cavity, including the subgingival region where periodontitis occurs (Acharya et al., 2017; Krishnan et al., 2017). Despite the progressive development of high-throughput sequencing techniques, there are still a limited number of studies investigating the salivary microbial composition of periodontitis.
Our results reported that the alpha diversity of subjects with periodontitis was greater than that observed in healthy individuals, which is in agreement with the previous studies (Ko et al., 2020; Sun et al., 2020). In line with a previous study, there were significant differences in β diversity values between periodontitis and control, while there was no significant difference in the salivary microbial data between AgP and ChP (Damgaard et al., 2019). Nibali et al. (2021) also indicated that limited microbial differences existed in the subgingival microbial communities between AgP and ChP. Salivary bacteria have been characterized in healthy individuals, and the predominant phyla were found to be Firmicutes, Bacteroidetes, Proteobacteria, Fusobacteria, and Actinobacteria (Segata et al., 2012). In this study, the phyla Spirochaetes and Synergistetes and the genera Treponema, Peptococcus, Catonella, Desulfobulbus, Peptostreptococcaceae_[XI] ([G-2], [G-3] [G-4], [G-6], and [G-9]), Bacteroidetes_[G-5], TM7_[G-5], Dialister, Eikenella, Fretibacterium, and Filifactor were present at higher levels in patients with periodontitis than in healthy participants. The association between these taxa in saliva and periodontitis has been well demonstrated (Lundmark et al., 2019; Ko et al., 2020; Sun et al., 2020). In addition, these genera have also previously been associated with periodontitis in subgingival plaque samples. Treponema, Desulfobulbus, Catonella, Bacteroides, Peptostreptococcus, and Eikenella have been reported as periodontitis biomarkers (Cai et al., 2021). Filifactor, Fretibacterium, Peptostreptococcaceae_[XI][G-5], Peptostreptococcaceae_[XI][G-6], Peptostreptococcus, and Treponema were significantly higher in the AgP group (Shi et al., 2020).
Metabolomics is a newly emerging technique that has been increasingly used to discover biomarkers for the diagnosis and prognosis of periodontitis. However, “chronic” and “aggressive” are now grouped as “Periodontitis” according to the current classification system (Papapanou et al., 2018). Significant differences in the composition of salivary metabolites among the three groups were found in the present study. In previous studies, AgP and ChP metabolomic profiles were not unequivocally discriminated through the NMR-based spectroscopic analysis of saliva (Rzeznik et al., 2017; Romano et al., 2018). The reason for this inconsistency might be based on different testing methods. The metabolites could be released due to bacterial metabolism or host-induced inflammatory processes, which suggested host–bacteria interactions in AgP may be different when compared with ChP. AgP displays an inadequate host response to periodontopathogenic bacteria, which can be attributed to increased expression of a wide variety of immunological and genetic risk factors (Takahashi et al., 2001; Yoshida et al., 2021).
Significantly higher levels of serine, serotonin, 4-hydroxycinnamic acid, hydrocinnamic acid, and isoleucine were identified in both the AgP and ChP groups when compared with the healthy controls in this study. The reports of previous studies on metabolite changes of saliva due to periodontitis match our findings, including isoleucine and proline (Romano et al., 2018; Citterio et al., 2020), threonine (Sugimoto et al., 2010; Rzeznik et al., 2017), and 4-aminobutyric acid (Sakanaka et al., 2017). Isoleucine reflects the host immune response to the oral microbiome and has already been associated with active periodontal disease (Zhang et al., 2017). The higher level of proline in AgP could be explained by the up-regulation of protease activity found in periodontitis (Wishart, 2008). The biochemical pathways that are increased in patients with periodontitis compared with healthy subjects include protein digestion and absorption, biosynthesis of amino acids, aminoacyl-tRNA biosynthesis, and glycine, serine, and threonine metabolism. Aminoacyl-tRNA determines how the genetic code is translated into amino acids. The biochemical pathways that are significantly different between the ChP and AgP groups include pyrimidine metabolism, beta-alanine metabolism, alanine, aspartate and glutamate metabolism, citrate cycle, and arginine and proline metabolism. Pyrimidine metabolism has been reported to be associated with AgP (Pei et al., 2020; Shi et al., 2020). Arginine and proline metabolism has been demonstrated to be involved in periodontitis mechanism (Kouznetsova et al., 2021). For example, degradation of arginine and other amino acids occurs in the periodontal pockets by asaccharolytic anaerobic Gram-positive rods (Uematsu et al., 2003). Arginine metabolism to nitric oxide also plays a role in periodontitis (Ozer et al., 2011).
To our knowledge, we reported the combined interactions between salivary microbiota and metabolites for the first time. In the association analysis of differential metabolites and microorganisms, the results showed that genera with the same change in trend between groups tended to have a similar correlation with some certain metabolites, either positively or negatively. The abundance of Streptococcus was significantly higher in PH than in AgP and ChP, which was positively correlated with the majority of healthy control-enriched metabolites and negatively correlated with the majority of periodontitis-enriched metabolites. Hydrocinnamic acid had significant related genera with 17 types in AgP and 15 types in ChP, respectively. The differential metabolites between the ChP and AgP groups, such as urea, beta-alanine, 3-aminoisobutyric acid, and thymine, had the most significant correlations with genera. However, the specific mechanism remains to be further explored and confirmed.
In conclusion, distinct differences were observed in salivary microbiome and metabolomics between periodontitis and healthy control. Striking differences were found in the composition of salivary metabolites between the AgP and ChP groups. The integration of microbial data and metabolomic data may help us understand the potential mechanism of periodontitis and offer potential biomarkers. A larger sample size is needed to validate the findings. Another limitation of our study is the differences in age distribution among the three groups that might potentially affect the results. In addition, it has already been demonstrated that salivary metabolites are not affected by gender, body mass index, and dietary intake (Walsh et al., 2006; Bertram et al., 2009). In addition, Kim et al. (2021) indicated that age differences did not affect the metabolite profile of saliva. A further longitudinal study in a larger cohort study, including dynamic changes in the salivary microbiome and metabolome after the initial treatment, would provide a better understanding of periodontal host–microbial interactions.
Data availability statement
The datasets presented in this study can be found in online repositories. The names of the repository/repositories and accession number(s) can be found in the article/Supplementary material.
Ethics statement
The studies involving human participants were reviewed and approved by Ethics Committee of the Peking University Health Science Center. The patients/participants provided their written informed consent to participate in this study.
Author contributions
WH and XW: conception and design. YW, MS, FS, and WJ: recruitment of patients and collection of oral samples. MS, YW, and YN: analysis and interpretation of data. YW and MS: manuscript preparation. MS, YW, YN, CW, and WH: manuscript revision. All authors contributed to the article and approved the submitted version.
Funding
This work was supported by the National Natural Science Foundation of China (No. 81672077), Peking University Clinical Scientist Program (BMU2019LCKXJ010), and the Natural Science Foundation of Beijing (No. 7214273).
Conflict of interest
The authors declare that the research was conducted in the absence of any commercial or financial relationships that could be construed as a potential conflict of interest.
Publisher’s note
All claims expressed in this article are solely those of the authors and do not necessarily represent those of their affiliated organizations, or those of the publisher, the editors and the reviewers. Any product that may be evaluated in this article, or claim that may be made by its manufacturer, is not guaranteed or endorsed by the publisher.
Supplementary material
The Supplementary Material for this article can be found online at: https://www.frontiersin.org/articles/10.3389/fmicb.2022.959416/full#supplementary-material
Supplementary Figure 1. Rarefaction curves.
Supplementary Figure 2. Correlations between salivary plaque bacteria (phylum level) with differential metabolites between AgP and PH. Each row in the graph represents a phylum, each column represents a metabolite, and each lattice represents a correlation coefficient between a component and a metabolite. Red represents a positive correlation, while blue represents a negative correlation. *Significant correlation between the phyla and metabolites (p < 0.05).
Supplementary Figure 3. Correlations between salivary plaque bacteria (phylum level) with differential metabolites between ChP and PH.
Supplementary Figure 4. Correlations between salivary plaque bacteria (phylum level) with differential metabolites between AgP and ChP.
Footnotes
References
Acharya, A., Chan, Y., Kheur, S., Jin, L. J., Watt, R. M., and Mattheos, N. (2017). Salivary microbiome in non-oral disease: A summary of evidence and commentary. Arch. Oral Biol. 83, 169–173. doi: 10.1016/j.archoralbio.2017.07.019
Armitage, G. C. (1999). Development of a classification system for periodontal diseases and conditions. Ann. Periodontol. 4, 1–6.
Armitage, G. C. (2000). Development of a classification system for periodontal diseases and conditions. Northwest. Dent. 79, 31–35.
Armitage, G. C., and Cullinan, M. P. (2010). Comparison of the clinical features of chronic and aggressive periodontitis. Periodontol. 2000 53, 12–27. doi: 10.1111/j.1600-0757.2010.00353.x
Belstrom, D., Grande, M. A., Sembler-Moller, M. L., Kirkby, N., Cotton, S. L., Paster, B. J., et al. (2018). Influence of periodontal treatment on subgingival and salivary microbiotas. J. Periodontol. 89, 531–539. doi: 10.1002/JPER.17-0377
Bertram, H. C., Eggers, N., and Eller, N. (2009). Potential of human saliva for nuclear magnetic resonance-based metabolomics and for health-related biomarker identification. Anal. Chem. 81, 9188–9193. doi: 10.1021/ac9020598
Buduneli, N., and Kinane, D. F. (2011). Host-derived diagnostic markers related to soft tissue destruction and bone degradation in periodontitis. J. Clin. Periodontol. 38, (Suppl. 11), 85–105. doi: 10.1111/j.1600-051X.2010.01670.x
Buzalaf, M. A. R., Ortiz, A. C., Carvalho, T. S., Fideles, S. O. M., Araujo, T. T., Moraes, S. M., et al. (2020). Saliva as a diagnostic tool for dental caries, periodontal disease and cancer: Is there a need for more biomarkers? Expert. Rev. Mol. Diagn. 20, 543–555. doi: 10.1080/14737159.2020.1743686
Cai, Z., Lin, S., Hu, S., and Zhao, L. (2021). Structure and function of oral microbial community in periodontitis based on integrated data. Front. Cell Infect. Microbiol. 11:663756. doi: 10.3389/fcimb.2021.663756
Chen, C., Hemme, C., Beleno, J., Shi, Z. J., Ning, D., Qin, Y., et al. (2018). Oral microbiota of periodontal health and disease and their changes after nonsurgical periodontal therapy. ISME J. 12, 1210–1224. doi: 10.1038/s41396-017-0037-1
Citterio, F., Romano, F., Meoni, G., Iaderosa, G., Grossi, S., Sobrero, A., et al. (2020). Changes in the salivary metabolic profile of generalized periodontitis patients after non-surgical periodontal therapy: A metabolomic analysis using nuclear magnetic resonance spectroscopy. J. Clin. Med. 9:3977. doi: 10.3390/jcm9123977
Damgaard, C., Danielsen, A. K., Enevold, C., Massarenti, L., Nielsen, C. H., Holmstrup, P., et al. (2019). Porphyromonas gingivalis in saliva associates with chronic and aggressive periodontitis. J. Oral Microbiol. 11:1653123. doi: 10.1080/20002297.2019.1653123
Duran-Pinedo, A. E., and Frias-Lopez, J. (2015). Beyond microbial community composition: Functional activities of the oral microbiome in health and disease. Microb. Infect. 17, 505–516. doi: 10.1016/j.micinf.2015.03.014
Emingil, G., Berdeli, A., Baylas, H., Saygan, B. H., Gurkan, A., Kose, T., et al. (2007). Toll-like receptor 2 and 4 gene polymorphisms in generalized aggressive periodontitis. J. Periodontol. 78, 1968–1977. doi: 10.1902/jop.2007.060360
Gardner, A., Carpenter, G., and So, P. W. (2020). Salivary metabolomics: From diagnostic biomarker discovery to investigating biological function. Metabolites 10:47. doi: 10.3390/metabo10020047
Greenwood, D., Afacan, B., Emingil, G., Bostanci, N., and Belibasakis, G. N. (2020). Salivary microbiome shifts in response to periodontal treatment outcome. Proteomics Clin. Appl. 14:e2000011. doi: 10.1002/prca.202000011
Hyvarinen, E., Savolainen, M., Mikkonen, J. J. W., and Kullaa, A. M. (2021). Salivary metabolomics for diagnosis and monitoring diseases: Challenges and possibilities. Metabolites 11:587. doi: 10.3390/metabo11090587
Kageyama, S., Takeshita, T., Asakawa, M., Shibata, Y., Takeuchi, K., Yamanaka, W., et al. (2017). Relative abundance of total subgingival plaque-specific bacteria in salivary microbiota reflects the overall periodontal condition in patients with periodontitis. PLoS One 12:e0174782. doi: 10.1371/journal.pone.0174782
Khurshid, Z., Warsi, I., Moin, S. F., Slowey, P. D., Latif, M., Zohaib, S., et al. (2021). Biochemical analysis of oral fluids for disease detection. Adv. Clin. Chem. 100, 205–253. doi: 10.1016/bs.acc.2020.04.005
Kim, S., Kim, H. J., Song, Y., Lee, H. A., Kim, S., and Chung, J. (2021). Metabolic phenotyping of saliva to identify possible biomarkers of periodontitis using proton nuclear magnetic resonance. J. Clin. Periodontol. 48, 1240–1249. doi: 10.1111/jcpe.13516
Ko, Y., Lee, E. M., Park, J. C., Gu, M. B., Bak, S., and Ji, S. (2020). Salivary microbiota in periodontal health and disease and their changes following nonsurgical periodontal treatment. J. Periodontal. Implant Sci. 50, 171–182. doi: 10.5051/jpis.2020.50.3.171
Kouznetsova, V. L., Li, J., Romm, E., and Tsigelny, I. F. (2021). Finding distinctions between oral cancer and periodontitis using saliva metabolites and machine learning. Oral Dis. 27, 484–493. doi: 10.1111/odi.13591
Krishnan, K., Chen, T., and Paster, B. J. (2017). A practical guide to the oral microbiome and its relation to health and disease. Oral Dis. 23, 276–286. doi: 10.1111/odi.12509
Kuboniwa, M., Sakanaka, A., Hashino, E., Bamba, T., Fukusaki, E., and Amano, A. (2016). Prediction of periodontal inflammation via metabolic profiling of saliva. J. Dent. Res. 95, 1381–1386. doi: 10.1177/0022034516661142
Liebsch, C., Pitchika, V., Pink, C., Samietz, S., Kastenmuller, G., Artati, A., et al. (2019). The Saliva Metabolome in association to oral health status. J. Dent. Res. 98, 642–651. doi: 10.1177/0022034519842853
Lu, R. F., Feng, L., Gao, X. J., Meng, H. X., and Feng, X. H. (2013). [Relationship between volatile fatty acids and Porphyromonas gingivalis and Treponema denticola in gingival crevicular fluids of patients with aggressive periodontitis]. Beijing Da Xue Xue Bao Yi Xue Ban 45, 12–16.
Lundmark, A., Hu, Y. O. O., Huss, M., Johannsen, G., Andersson, A. F., and Yucel-Lindberg, T. (2019). Identification of salivary microbiota and its association with host inflammatory mediators in periodontitis. Front. Cell Infect Microbiol. 9:216. doi: 10.3389/fcimb.2019.00216
Marchesan, J. T., Girnary, M. S., Moss, K., Monaghan, E. T., Egnatz, G. J., Jiao, Y., et al. (2020). Role of inflammasomes in the pathogenesis of periodontal disease and therapeutics. Periodontol. 2000 82, 93–114. doi: 10.1111/prd.12269
Martina, E., Campanati, A., Diotallevi, F., and Offidani, A. (2020). Saliva and oral diseases. J. Clin. Med. 9:466. doi: 10.3390/jcm9020466
Mazza, J. E., Newman, M. G., and Sims, T. N. (1981). Clinical and antimicrobial effect of stannous fluoride on periodontitis. J. Clin. Periodontol. 8, 203–212.
Nibali, L., Sousa, V., Davrandi, M., Liu, L. S., Spratt, D., and Donos, N. (2021). Patterns of subgingival microbiota in different periodontal phenotypes. J. Dent. 117:103912. doi: 10.1016/j.jdent.2021.103912
Ozer, L., Elgun, S., Ozdemir, B., Pervane, B., and Ozmeric, N. (2011). Arginine-nitric oxide-polyamine metabolism in periodontal disease. J. Periodontol. 82, 320–328. doi: 10.1902/jop.2010.100199
Papapanou, P. N., Sanz, M., Buduneli, N., Dietrich, T., Feres, M., Fine, D. H., et al. (2018). Periodontitis: Consensus report of workgroup 2 of the 2017 world workshop on the classification of periodontal and peri-implant diseases and conditions. J. Periodontol. 89, (Suppl. 1), S173–S182. doi: 10.1002/JPER.17-0721
Pei, J., Li, F., Xie, Y., Liu, J., Yu, T., and Feng, X. (2020). Microbial and metabolomic analysis of gingival crevicular fluid in general chronic periodontitis patients: Lessons for a predictive, preventive, and personalized medical approach. EPMA J. 11, 197–215. doi: 10.1007/s13167-020-00202-5
Petersen, P. E. (2003). The world oral health report 2003: Continuous improvement of oral health in the 21st century–the approach of the WHO Global Oral Health Programme. Community Dent. Oral Epidemiol. 31, (Suppl. 1), 3–23. doi: 10.1046/j.2003.com122.x
Romano, F., Meoni, G., Manavella, V., Baima, G., Mariani, G. M., Cacciatore, S., et al. (2019). Effect of non-surgical periodontal therapy on salivary metabolic fingerprint of generalized chronic periodontitis using nuclear magnetic resonance spectroscopy. Arch. Oral Biol. 97, 208–214. doi: 10.1016/j.archoralbio.2018.10.023
Romano, F., Meoni, G., Manavella, V., Baima, G., Tenori, L., Cacciatore, S., et al. (2018). Analysis of salivary phenotypes of generalized aggressive and chronic periodontitis through nuclear magnetic resonance-based metabolomics. J. Periodontol. 89, 1452–1460. doi: 10.1002/JPER.18-0097
Rzeznik, M., Triba, M. N., Levy, P., Jungo, S., Botosoa, E., Duchemann, B., et al. (2017). Identification of a discriminative metabolomic fingerprint of potential clinical relevance in saliva of patients with periodontitis using 1H nuclear magnetic resonance (NMR) spectroscopy. PLoS One 12:e0182767. doi: 10.1371/journal.pone.0182767
Sakanaka, A., Kuboniwa, M., Hashino, E., Bamba, T., Fukusaki, E., and Amano, A. (2017). Distinct signatures of dental plaque metabolic byproducts dictated by periodontal inflammatory status. Sci. Rep. 7:42818. doi: 10.1038/srep42818
Segata, N., Haake, S. K., Mannon, P., Lemon, K. P., Waldron, L., Gevers, D., et al. (2012). Composition of the adult digestive tract bacterial microbiome based on seven mouth surfaces, tonsils, throat and stool samples. Genome Biol. 13:R42. doi: 10.1186/gb-2012-13-6-r42
Shi, M., Wei, Y., Hu, W., Nie, Y., Wu, X., and Lu, R. (2018). The subgingival microbiome of periodontal pockets with different probing depths in chronic and aggressive periodontitis: A pilot study. Front. Cell Infect Microbiol. 8:124. doi: 10.3389/fcimb.2018.00124
Shi, M., Wei, Y., Nie, Y., Wang, C., Sun, F., Jiang, W., et al. (2020). Alterations and correlations in microbial community and metabolome characteristics in generalized aggressive periodontitis. Front. Microbiol. 11:573196. doi: 10.3389/fmicb.2020.573196
Sugimoto, M., Wong, D. T., Hirayama, A., Soga, T., and Tomita, M. (2010). Capillary electrophoresis mass spectrometry-based saliva metabolomics identified oral, breast and pancreatic cancer-specific profiles. Metabolomics 6, 78–95. doi: 10.1007/s11306-009-0178-y
Sun, X., Li, M., Xia, L., Fang, Z., Yu, S., Gao, J., et al. (2020). Alteration of salivary microbiome in periodontitis with or without type-2 diabetes mellitus and metformin treatment. Sci. Rep. 10:15363. doi: 10.1038/s41598-020-72035-1
Takahashi, K., Ohyama, H., Kitanaka, M., Sawa, T., Mineshiba, J., Nishimura, F., et al. (2001). Heterogeneity of host immunological risk factors in patients with aggressive periodontitis. J. Periodontol. 72, 425–437. doi: 10.1902/jop.2001.72.4.425
Tonetti, M. S., Greenwell, H., and Kornman, K. S. (2018). Staging and grading of periodontitis: Framework and proposal of a new classification and case definition. J. Clin. Periodontol. 45, (Suppl. 20), S149–S161. doi: 10.1111/jcpe.12945
Uematsu, H., Sato, N., Hossain, M. Z., Ikeda, T., and Hoshino, E. (2003). Degradation of arginine and other amino acids by butyrate-producing asaccharolytic anaerobic Gram-positive rods in periodontal pockets. Arch. Oral Biol. 48, 423–429. doi: 10.1016/s0003-9969(03)00031-1
Walsh, M. C., Brennan, L., Malthouse, J. P., Roche, H. M., and Gibney, M. J. (2006). Effect of acute dietary standardization on the urinary, plasma, and salivary metabolomic profiles of healthy humans. Am. J. Clin. Nutr. 84, 531–539.
Wishart, D. S. (2008). Quantitative metabolomics using NMR. Trac-Trends Anal. Chem. 27, 228–237. doi: 10.1016/j.trac.2007.12.001
Yoshida, A., Bouziane, A., Erraji, S., Lakhdar, L., Rhissassi, M., Miyazaki, H., et al. (2021). Etiology of aggressive periodontitis in individuals of African descent. Jpn. Dent. Sci. Rev. 57, 20–26. doi: 10.1016/j.jdsr.2020.12.001
Yoshizawa, J. M., Schafer, C. A., Schafer, J. J., Farrell, J. J., Paster, B. J., and Wong, D. T. (2013). Salivary biomarkers: Toward future clinical and diagnostic utilities. Clin. Microbiol. Rev. 26, 781–791. doi: 10.1128/CMR.00021-13
Keywords: periodontitis, salivary microbiome, metabolome, high-throughput nucleotide sequencing, gas chromatography-mass spectrometry
Citation: Wei Y, Shi M, Nie Y, Wang C, Sun F, Jiang W, Hu W and Wu X (2022) Integrated analysis of the salivary microbiome and metabolome in chronic and aggressive periodontitis: A pilot study. Front. Microbiol. 13:959416. doi: 10.3389/fmicb.2022.959416
Received: 01 June 2022; Accepted: 06 September 2022;
Published: 26 September 2022.
Edited by:
Biswarup Sen, Tianjin University, ChinaReviewed by:
Renato Correa Viana Casarin, Universidade Estadual de Campinas, BrazilMin Gou, Sichuan University, China
Copyright © 2022 Wei, Shi, Nie, Wang, Sun, Jiang, Hu and Wu. This is an open-access article distributed under the terms of the Creative Commons Attribution License (CC BY). The use, distribution or reproduction in other forums is permitted, provided the original author(s) and the copyright owner(s) are credited and that the original publication in this journal is cited, in accordance with accepted academic practice. No use, distribution or reproduction is permitted which does not comply with these terms.
*Correspondence: Wenjie Hu, aHV3ZW5qaWVAcGt1c3MuYmptdS5lZHUuY24=
†These authors have contributed equally to this work