- 1School of Physical Science and Engineering, College of Life Sciences and Bioengineering, Beijing Jiaotong University, Beijing, China
- 2Department of Endocrinology and Metabolism, The Third Affiliated Hospital of Southern Medical University, Guangzhou, China
- 3Center for System Biology, Data Sciences, and Reproductive Health, School of Basic Medical Science, Central South University, Changsha, China
- 4Tulane Center for Bioinformatics and Genomics, School of Public Health and Tropical Medicine, Tulane University, New Orleans, LA, United States
- 5Shunde Hospital of Southern Medical University (The First People’s Hospital of Shunde), Foshan, China
Cow milk consumption (CMC) and alterations of gut bacterial composition are proposed to be closely related to human health and disease. Our research aims to investigate the changes in human gut microbial composition in Chinese peri-/postmenopausal women with different CMC habits. A total of 517 subjects were recruited and questionnaires about their CMC status were collected; 394 subjects were included in the final analyses. Fecal samples were used for studying gut bacterial composition. All the subjects were divided into a control group (n = 248) and a CMC group (n = 146) according to their CMC status. Non-parametric tests and LEfSe at different taxonomic levels were used to reveal differentially abundant taxa and functional categories across different CMC groups. Relative abundance (RA) of one phylum (p_Actinobacteria), three genera (g_Bifidobacterium, g_Anaerostipes, and g_Bacteroides), and 28 species diversified significantly across groups. Specifically, taxa g_Anaerostipes (p < 0.01), g_Bacteroides (p < 0.05), s_Anaerostipes_hadrus (p < 0.01), and s_Bifidobacterium_pseudocatenulatum (p < 0.01) were positively correlated with CMC levels, but p_Actinobacteria (p < 0.01) and g_Bifidobacterium (p < 0.01) were negatively associated with CMC levels. KEGG module analysis revealed 48 gut microbiome functional modules significantly (p < 0.05) associated with CMC, including Vibrio cholerae pathogenicity signature, cholera toxins (p = 9.52e-04), and cephamycin C biosynthesis module (p = 0.0057), among others. In conclusion, CMC was associated with changes in gut microbiome patterns including beta diversity and richness of some gut microbiota. The alterations of certain bacteria including g_Anaerostipes and s_Bifidobacterium_pseudocatenulatum in the CMC group should be important for human health. This study further supports the biological value of habitual cow milk consumption.
Introduction
Milk and dairy products are frequently included as important elements in a healthy and balanced diet, which can provide the necessary energy and nutrients to ensure proper growth and development (Pereira, 2014). Epidemiologic studies confirmed the nutritional importance of milk in the human diet and reinforce the possible role of its consumption in preventing several chronic conditions like cardiovascular diseases (CVDs), osteoporosis (Ilesanmi-Oyelere and Kruger, 2020), some forms of cancer, obesity, and diabetes (Pereira, 2014). Milk also provides a multitude of proteins with anti-inflammatory properties, and these bioactive factors may attenuate intestinal inflammation (Chatterton et al., 2013; Thai and Gregory, 2020).
The human gut is populated by trillions of microorganisms, known collectively as the gut microbiota (GM) (Ley et al., 2008). Many human disorders are associated with gut microbiota, such as gastrointestinal disorders like inflammatory bowel disease (IBD) (Odamaki et al., 2016), colorectal cancer (Rao et al., 2006), irritable bowel syndrome, and gastrointestinal cancer (Botteri et al., 2008); and metabolic diseases like diabetes mellitus (Cardinali et al., 2020) and obesity (Cuevas-Sierra et al., 2019). The immune homeostasis of the host is also influenced by gut microbiota (Mason et al., 2015). Gut microbiota can be modulated positively or negatively by different lifestyle and dietary factors (Gilbert et al., 2018).
Several previous studies have assessed the effect of dairy products on human GM, but many have focused on fermented dairy like yogurt (Link-Amster et al., 1994; Odamaki et al., 2012, 2016; Yılmaz et al., 2019; Jie et al., 2021) or special dairy products like casein powder (Beaumont et al., 2017) or whey bars (Reimer et al., 2017). Some recent studies showed that cow milk could influence the alpha (Fernandez-Raudales et al., 2012; Shuai et al., 2021) and beta diversity (Aslam et al., 2021; Shuai et al., 2021) of GM and relative abundance (RA) of some taxa including phyla p_Bacteroidetes and p_Proteobacteria and genus g_Roseburia, g_Lactobacillus, g_Prevotella, g_Bifidobacterium, g_Clostridium, g_Streptococcus, g_Atopobium, g_ Leuconostoc, and g_Veillonella in humans (Fernandez-Raudales et al., 2012; Bendtsen et al., 2018; Swarte et al., 2020; Aslam et al., 2021; de Carvalho et al., 2022). However, very few studies have reported cow milk’s effect on human gut bacterial species (Aslam et al., 2020). In addition, most of the studies were based on animal models or randomized controlled trials. Population-level observational studies which could really evaluate the effects of habitual dairy intake on the gut microbiome were very rare (Aslam et al., 2021). Women in the postmenopausal stage usually suffered from many kinds of diseases, and some of them including osteoporosis and breast cancer were reported to be associated with gut microbiota (Zhu et al., 2018; Gatti and Fassio, 2019; Ozaki et al., 2021; Rettedal et al., 2021). In this study, fecal samples from Chinese peri-/postmenopausal women were collected and metagenomic shotgun sequencing was performed. Metagenomic analyses were conducted to identify the effect of cow milk consumption (CMC) on GM patterns, including some general compositional features and abundance of each taxon at the phylum, genus, and species levels. In particular, KEGG functional modules associated with CMC were also detected. To our knowledge, this is the first gut microbiome-CMC association study based on shotgun sequencing performed in Chinese peri-/postmenopausal women.
Materials and methods
Study subjects and questionnaire
This study was approved by the Third Affiliated Hospital of Southern Medical University (Guangzhou City, China) and performed under the principle of the Helsinki Declaration II. A cohort of 517 unrelated healthy Chinese peri-/postmenopausal women were recruited from June 2014 to January 2018 from local communities. Each subject completed a questionnaire that collected their CMC status and other basic information like age, weight, medication, alcohol drinking, diet, smoking history, and use of nutrition supplements. Specifically, participants selected the CMC from predetermined categories: none (0 ml/day), 250 ml/day, 500 ml/day, and 750 ml or more/day. Subjects were divided into a control group and a CMC group (milk intake ≥ 250 ml/day) according to the questionnaire. The cow milk was pasteurized. Two inclusion criteria were applied in this study: (1) aged 40 years or older and (2) had lived in Guangzhou City for more than 3 months. Exclusion criteria included: (1) Used antibiotics, oestrogens, anticonvulsant, or proton pump inhibitor medications in the past 3 months; (2) Underwent hysterectomy or bilateral ovariectomy; (3) Diabetes mellitus, except for easily controlled, non-insulin-dependent diabetes mellitus; (4) Chronic renal disease manifest by serum creatinine > 1.9 mg/dL; (5) Chronic liver diseases; (6) Significant chronic lung disease; (6) Alcohol abuse; (7) Corticosteroid therapy and anticonvulsant therapy; (8) Other metabolic diseases or inherited bone diseases; (9) major gastrointestinal disease including lactose intolerance; and (10) Any other disease, treatment, or condition that would be an apparent non-genetic factor underlying the variation of BMD, etc. A more detailed exclusion criteria were presented in Supplementary Table 1.
Fecal sampling and DNA extraction
We collected stool samples from all subjects. The fecal samples were frozen at -80°C within 30 min of sample procurement and used for GM DNA extraction with the E.Z.N.A.® Stool DNA Kit (Omega, Norcross, GA, United States). We stored the GM DNA samples at -80°C until further analyses.
Metagenomic shotgun sequencing
A fecal DNA library was constructed with the TruSeq Nano DNA LT Library Preparation Kit (FC-121-4001, Illumina, San Diego, CA, United States). The fecal DNA was fragmented by dsDNA Fragmentase (NEB, M0348S, Massachusetts, United States) and incubated at 37°C for 30 min. Fragmented cDNA was used to construct libraries. Blunt-end DNA fragmentation and size selection were performed with provided sample purification beads. An A-base was added to the blunt ends of each strand for the preparation of ligation to indexed adapters. These adapters also contained sequencing primer hybridization sites for single, paired-end, and indexed reads. The ligated products were amplified with polymerase chain reaction (PCR) under the following conditions: initial denaturation at 95°C for 3 min, followed by 8 cycles of 98°C for 15 s (denaturation), 60°C for 15 s, 72°C for 30 s (extension), and then a final elongation at 72°C for 5 min.
Metagenomic shotgun sequencing was performed by LC-Bio Technologies (Hangzhou) CO., LTD. (Hangzhou, China1) via Hiseq 4000 (Illumina, San Diego, CA, United States) and PE150 strategy. The relative abundance (RA) of unigenes for each sample was estimated by transcripts per kilobase million (TPM, Formula 1, where k was the kth unigene, r was the number of unigene reads, and L indicated unigene length) based on the number of aligned reads and the unigene length by Bowtie2 v2.2.0.
Bioinformatics
Raw data processing
Raw sequencing read data were processed in the following steps: (1) Cutadapt v1.9. was used to remove sequencing adapters from sequencing reads. (2) Low-quality reads were trimmed using Fqtrim v0.94. A sliding window (size = 6 bp) was set to calculate the average quality of the bases within this window, and the 3’ end of reads was trimmed when the average quality value was smaller than 20; we also discarded the reads when the length was less than 100 bp and the percentage of “N” was larger than 5% after trimming. (3) Read alignment to the host genome was performed by Bowtie2 v2.2.0 and host genomic contamination was removed. Once quality-filtered reads were obtained, they were de novo assembled to construct metagenomes for each sample by SPAdes v3.10.0. The coding sequences (CDS) of metagenomic contigs were predicted by MetaGeneMark v3.26. The CDS of all samples were clustered by CD-HIT v4.6.1 to obtain unigenes.
Taxonomic and functional annotation of unigenes
Unigenes of all samples were aligned according to the NCBI NR database2 using DIAMOND software with the lowest common ancestor algorithm. A protein reference based on the KEGG module dataset3 was applied for functional annotation. The sum of RA of unigenes within a module represented the RA of an annotated functional module.
The BLASTP function within DIAMOND was used for unigene alignments. It determines the bit score and expected value (E-value) of the computed alignment, which indicates the alignment quality. We selected the best hit with the highest bit score from all the potential hits (E-values ≤ 1 × 10–5) as the respective KEGG Orthology (KO) for each unigene. KOs were further mapped to GM-associated functional KEGG modules.
Statistical analysis
Differences in covariates like age, weight, body mass index (BMI), and calcium consumption between the two groups were checked with a Chi-square test or two independent sample t-tests according to variable features; p < 0.05 was considered to achieve statistical significance.
Data normalization methods total sum scaling (TSS) or centered-log ratio (CLR) were applied, respectively, in different analyses. Metagenome-Assembled Genome (MAG) counts at the phylum, genus, and species levels in each group were calculated within R by the criteria that one feature is taken into account only if its RA is more than 0.01% after TSS data transformation. For further community composition analysis, the data were filtered by several criteria: (1) relative abundance > 0.1%; (2) detection rate within all samples > 10%; and (3) low variance filter for comparative analysis: inter-quantile range (IQR) > 10%. Dominant microbiotas were calculated within each group at different taxonomic levels via R according to their RA.
Alpha and beta diversity, which, respectively, demonstrate within-sample microbiota richness and between-sample dissimilarities, were computed within R via the Vegan package in each group at the phylum, genus, and species levels. For alpha diversity, two indexes (Shannon and Simpson) were computed and Mann–Whitney test was used to assess the difference within groups; p < 0.05 was considered significant. For beta diversity, dissimilarity distances between samples were assessed by the Bray–Curtis matrix, and Principal Coordinates Analysis (PCoA) and Nonmetric Multidimensional Scaling (NMDS) were used to depict it. Permutational analysis of variance (PERMANOVA) test was used to evaluate the beta diversity difference between two groups, and p < 0.05 was considered significant.
A classical univariate analysis (Mann–Whitney test) was performed to detect differences in RA of each taxon between the CMC group and the control group; p-value < 0.05 was considered statistically significant. In this analysis, we applied both TSS and CLR data normalization methods. TSS is a traditional approach and has been frequently applied in microbiome analysis; however, it is strongly influenced by highly abundant taxa (Badri et al., 2020). CLR transformation could theoretically avoid the compositional effects of microbiome data (McMurdie and Holmes, 2014).
Linear Discriminant Analysis Effect Size (LEfSe) uses the Kruskal–Wallis test, Wilcoxon Rank Sum test, and Linear Discriminant Analysis (LDA) for microbiome biomarker discovery. For this study, LEfSe was performed given two indispensable situations: (1) threshold on the logarithmic LDA score for discriminative features was equivalent to 2.0; and (2) p-value < 0.05 and FDR-adjusted p < 0.1.
R package NetCoMi was applied to depict the profile of the gut microbiota’s association. MAG abundance data and sample data were processed by the phyloseq function in the phyloseq package in R. We selected the 100 taxa with the highest abundance to calculate their association in the gut. CLR data norm method was used and the association was calculated using the SPRING method (Yoon et al., 2019). For the network comparison, the 50 most abundant taxa were chosen. To test the difference in networks between the two groups, the absolute differences in network properties were computed. To depict the overall difference of networks in the two groups, the Jaccard index (j) was computed; the smaller the j was, the more different the two networks were (Peschel et al., 2021).
For the functional module analysis, Mann–Whitney test was applied to assess the richness differences. p < 0.05 was significant.
Validation
An American cohort (n = 260) was used to validate the results of our analysis. These subjects were divided into a control group (n = 130) and a CMC group (n = 130) according to the questionnaire, and diversified GMs between these two groups were identified. The data transformation and statistical analysis methods were the same as for the Chinese cohort.
Results
Basic characteristics of study subjects
After getting rid of subjects with missing information about CMC status, a total of 394 subjects were divided into two groups: the control group (n = 248) and the CMC group (n = 146). All subjects were female and no significant difference was observed in their age distribution (P = 0.79). There was no significant difference in physical characteristics like weight (P = 0.85) or BMI (P = 0.858), or in dietary habits like diet prone (P = 0.59), alcohol drinking (P = 0.787), or pickled or fermented foods (including yogurt) (P = 0.432) across CMC status. However, subjects in the CMC group tend to consume yogurt more compared with the control group (Table 1).
Taxonomic composition of gut microbiota
Number of different taxonomies
After being filtered by the criteria RA > 0.01%, the total number of phyla was 59 in both groups, but the total number of genera and the total number of species was slightly lower in the CMC group: There were 2,097 genera and 3,426 species in the control group, whereas there were 2,090 genera and 3,354 species in the CMC group (Table 2).
Dominated taxa of gut microbiota in subjects
A total of 214 species belonging to 52 genera and 7 phyla remained after the data processing. The five most prevalent phyla were p_Bacteroidetes, p_Firmicutes, p_Proteobacteria, p_Actinobacteria, and p_Fusobacteria. Bacteria in these phyla accounted for 80% of the total bacteria. At the genus level, g_Bacteroides, g_Prevotella, g_Eubacterium, g_Clostridium, and g_Faecalibacterium accounted for 65% of the total bacteria. At the species level, s_Bacteroides_unclassified, s_Bacteroides_vulgatus, s_Faecalibacterium_prausnitzzi, s_Prevotella_corpi, and s_Bacteroidales_unclassified were most abundant, accounting for about 20% of the total species (Table 3).
Alpha and beta diversity
To detect any differences in gut bacterial diversity between the two groups, alpha diversity indexes (Shannon and Simpson) and a beta diversity index (Bray–Curtis distance) were computed. For alpha diversity, no significant difference was observed across the groups at the phylum, genus, or species levels (P > 0.05) (Figure 1 and Supplementary Table 2). For beta diversity, at the phylum level, no significant difference was observed (PPCoA < 0.22 and PNMDS < 0.223) (Figure 2A and Supplementary Table 2); at the genus level, significant p-values were achieved (PPCoA < 0.039 and PNMDS < 0.037) (Figure 2B and Supplementary Table 2); as for the species level, two nearly significant p-values were observed (PPCoA < 0.055 and PNMDS < 0.055) (Figure 2C and Supplementary Table 2).
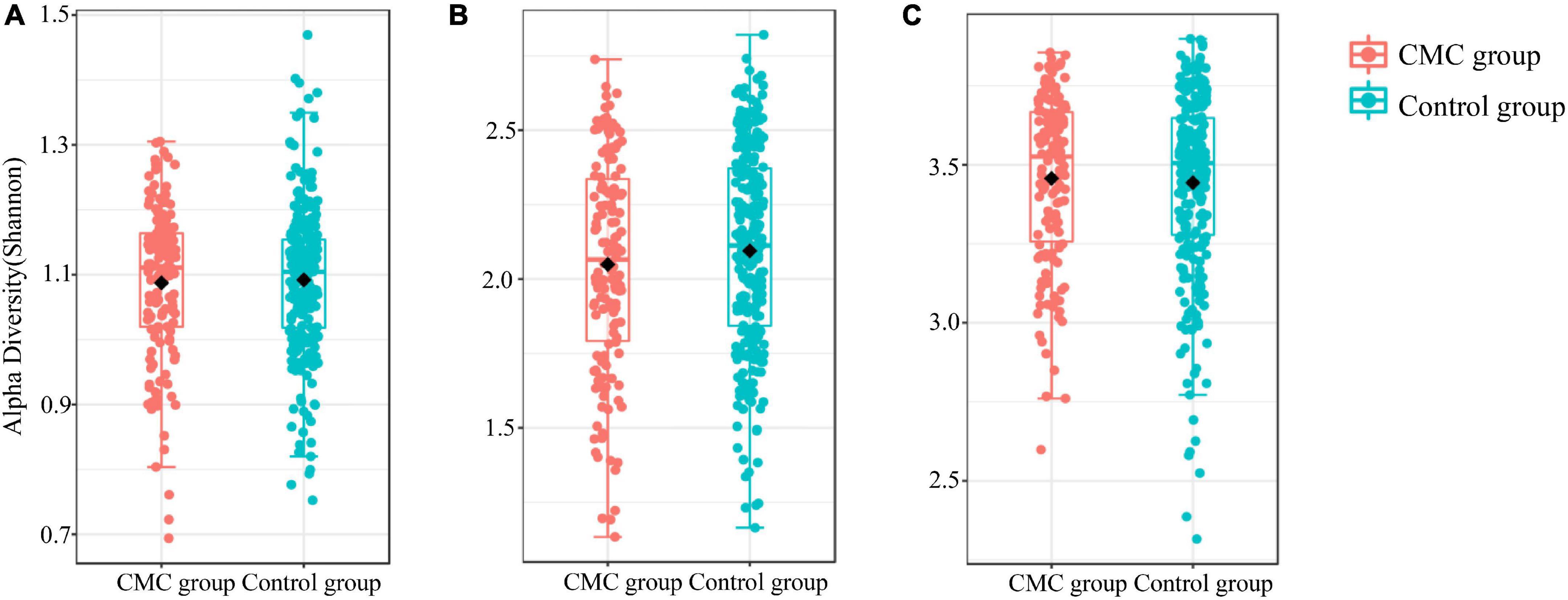
Figure 1. Alpha diversity of subjects grouped by CMC status. The (A–C) refer to the Shannon index of gut microbiota at different MAG levels. (A) Phylum, (B) Genus, (C) Species. Data were normalized via the TSS method.
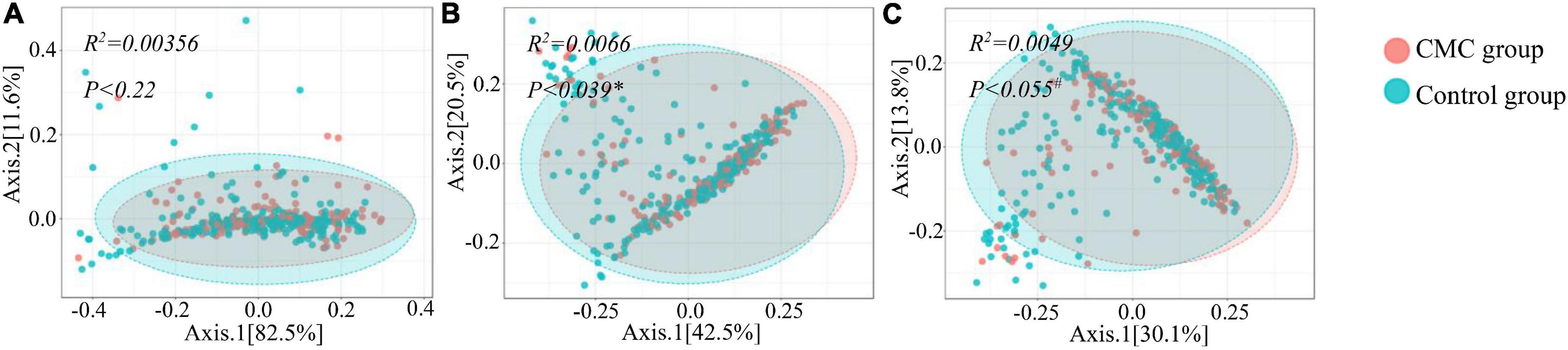
Figure 2. Beta diversity of subjects grouped by CMC status. (A–C) depict beta-diversity of gut microbiota according to Bray–Curtis (BC) distance via principal coordinates analysis (PCoA) method. (A) Phylum, (B) Genus, (C) Species. Data were normalized via the TSS method, PERMANOVA (Permutational ANOVA) test was applied to detect the difference significance in the two groups and #P < 0.1 or *P < 0.05.
Association between gut microbiota and cow milk consumption
We compared the RA of each taxon between two groups via Mann–Whitney test at the phylum, genus, and species levels. Data normalization methods total sum scaling (TSS) and centered-log ratio (CLR) were applied, respectively, to minimize the impact of data normalization on the results. For the TSS transformed data analysis, RA of one phylum (p_Actinobacteria), three genera (g__Bifidobacterium, g__Anaerostipes, g__Bacteroides), and 28 species were altered in the CMC group versus the control group (P < 0.05) and only two (g__Bacteroides, PCLR = 0.084; s__Prevotella_copri_CAG_164, PCLR = 0.057) of these taxa have not been observed to diversify significantly in the CLR transformed data analysis. The five most significantly diversified taxa completely overlapped for these two data normalization methods: p_Actinibacteria (PTSS = 7.02e-4, FDR PTSS = 0.0049; PCLR = 4.44e-4, FDR PCLR = 0.0031), g_Bifidobacterium (PTSS = 7.02e-4, FDR PTSS = 0.019; PCLR = 4.44e-4, FDR PCLR = 0.0115), g__Anaerostipes (PTSS = 7.33e-4, FDR PTSS = 0.019; PCLR = 8.21e-5, FDR PCLR = 0.0043), s__Anaerostipes_hadrus (PTSS = 7.33e-4, FDR PTSS = 0.0932; PCLR = 8.21e-5, FDR PCLR = 0.0176), and s__Bifidobacterium_unclassified (PTSS = 8.71e-4, FDR PTSS = 0.0932; PCLR = 4.49e-4, FDR PCLR = 0.048) (Table 4).
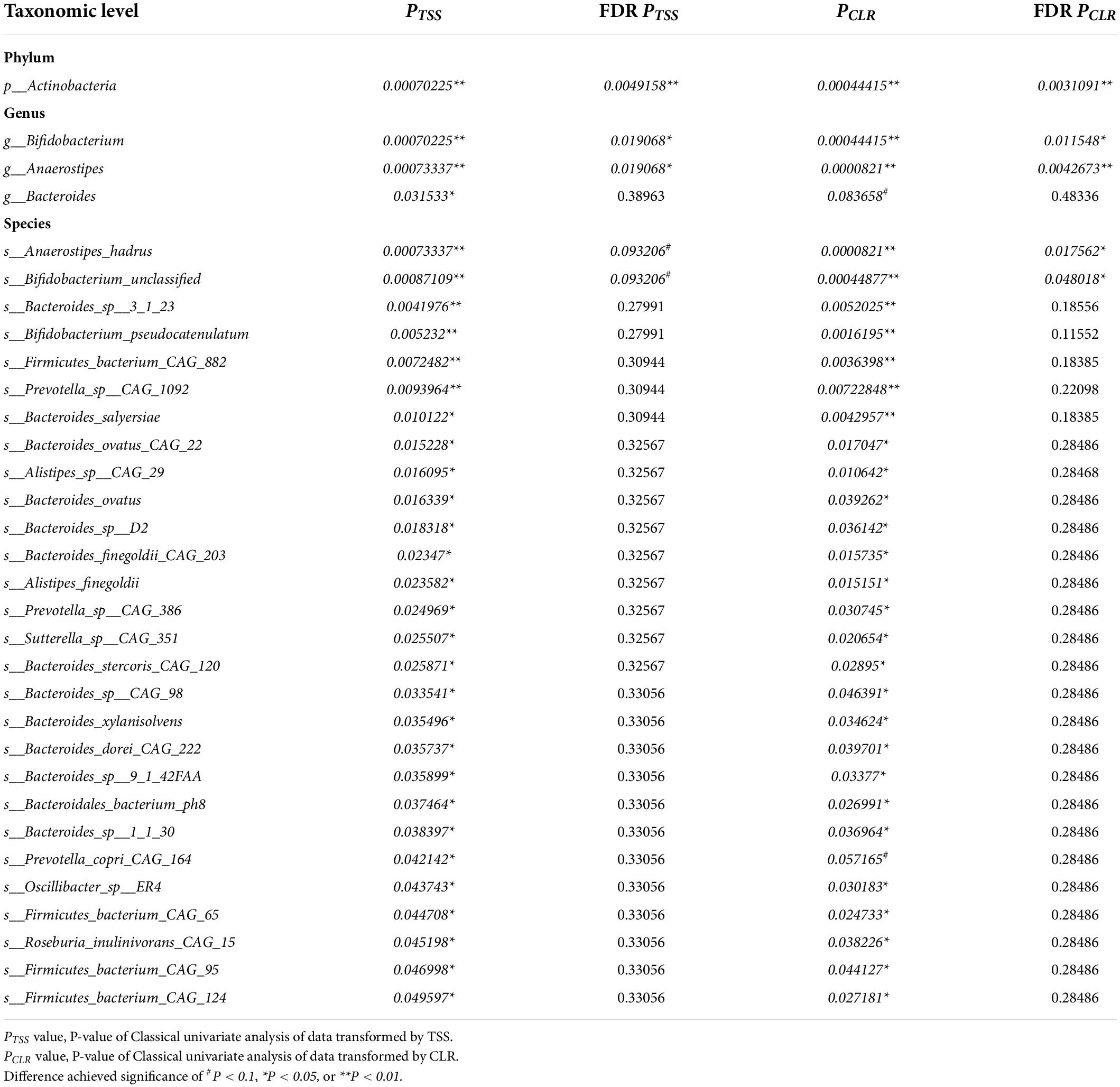
Table 4. Gut microbiota associated with cow milk consumption (CMC) via Mann–Whitney test at phylum, genus, and species levels.
Taxonomic biomarkers associated with cow milk consumption identified by linear discriminant analysis effect size
Linear discriminant analysis effect size (LEfSe) analysis was conducted to identify the correlation between gut microbiota and CMC. An LDA score > 0 means the taxa was positively associated with CMC, while an LDA score < 0 indicates a negative association between taxa and CMC. According to the LEfSe results, a total of 36 taxa correlated with CMC (p < 0.05, | LDA| > 2.0), but only five of these taxa reached FDR < 0.1. Among them, RA of p_Actinobacteria (FDR = 0.0049, LDA score = −2.19), g_Bifidobacterium (FDR = 0.019, LDA score = −2.19), and s_Bifidobacterium_unclassified (FDR = 0.093, LDA score = −3) decreased in the CMC group, but RA of g_Anaerostipes (FDR = 0.019, LDA score = 3.21) and s_Anaerostipes_hadrus (FDR = 0.093, LDA score = 3.21) increased. These taxa were the same as those most significant ones in the Mann–Whitney test. Some other taxa such as g_Bacteroides (p = 0.031, LDA = 5.29) and s_Bifidobacterium_pseudocatenulatum (p = 0.005, LDA = 2.92) were also observed to increase in CMC group, though their FDR > 0.1 (Figures 3, 4 and Supplementary Table 3).
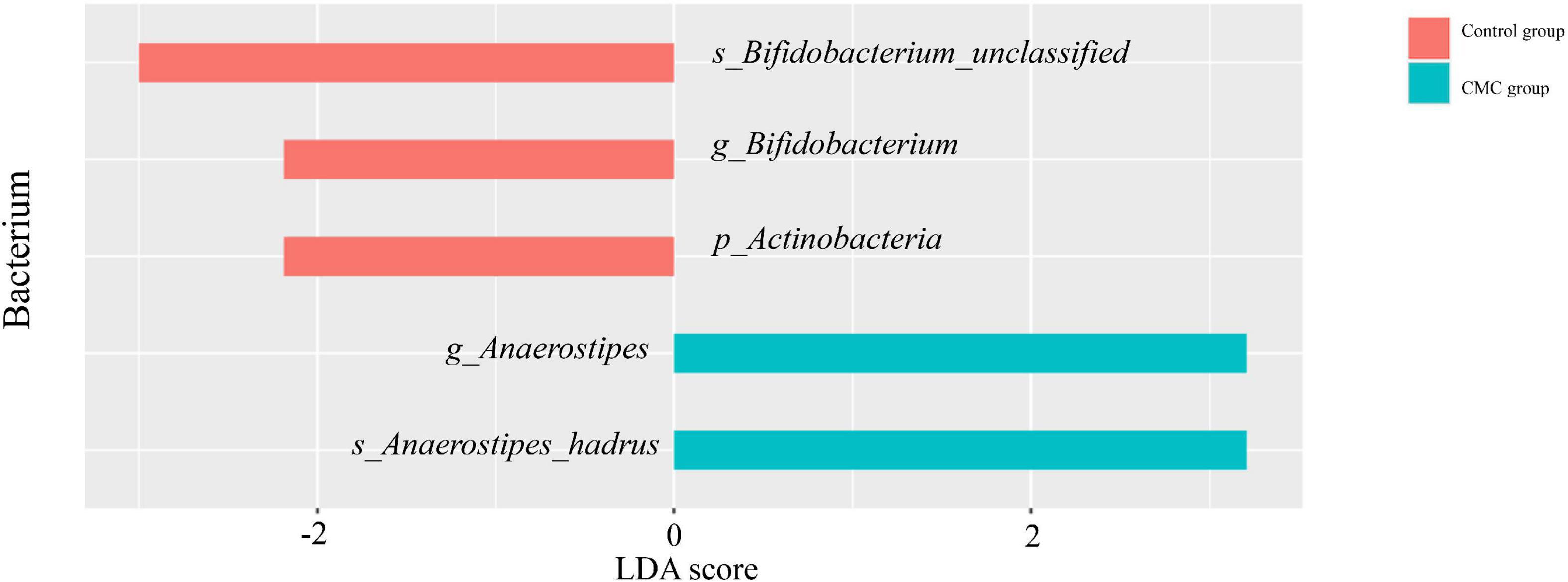
Figure 3. Biomarkers identified by LEfSe. LEfSe indicates differences in the bacterial taxa at different levels (p, phylum; g, genus; s, species), only the taxa having P < 0.05, FDR < 0.1, and LDA value > 2 are shown in the figure.
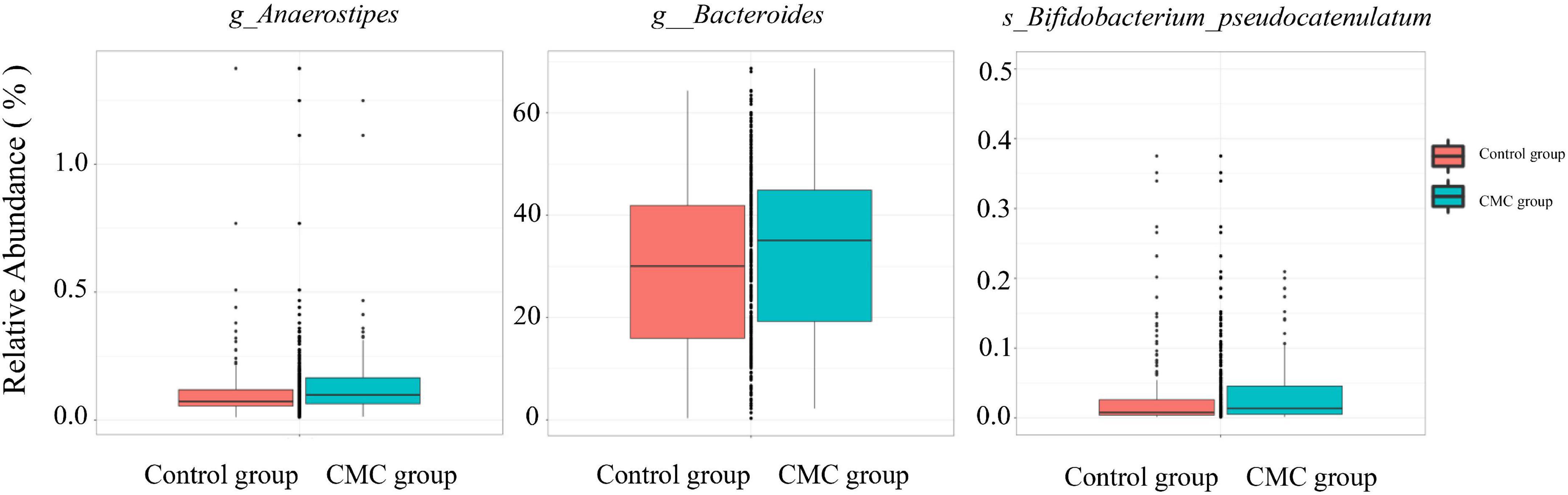
Figure 4. Differences of taxa abundance present in subjects. Data were normalized via total sum scaling (TTS) method and are expressed as relative abundance. Some significantly CMC-associated GM are displayed (g, genus; s, species).
Yogurt consumption was not associated with cow milk consumption-associated gut microbiota
We have observed some differences in yogurt consumption between the two groups, we further explored its possible association with CMC-associated GM via Mann–Whitney test. No significant association was observed and the results were presented in Supplementary Table 4.
Network construction and comparison
Constructing a single microbial interplay network
We constructed a taxa network with all the samples studied, and numerous associations between different taxa were observed. The positive edge percentage was 74.61%, which indicated that most of these taxa had positive associations with others. The five hub taxa with the largest empirical quantiles of centralities were s__Eubacterium_siraeum (X171), s__Firmicutes_bacterium_CAG.103 (X176), s__Bacteroides_unclassified (X2), s__Oscillibacter_sp._ER4 (X36), and s__Roseburia_hominis (X66). X66 had the highest degree of 23, highest closeness centrality of 0.836, and highest eigenvector centrality of 1.000. Nevertheless, taxa X2 had the highest betweenness centrality (Figure 5A and Supplementary Table 5).
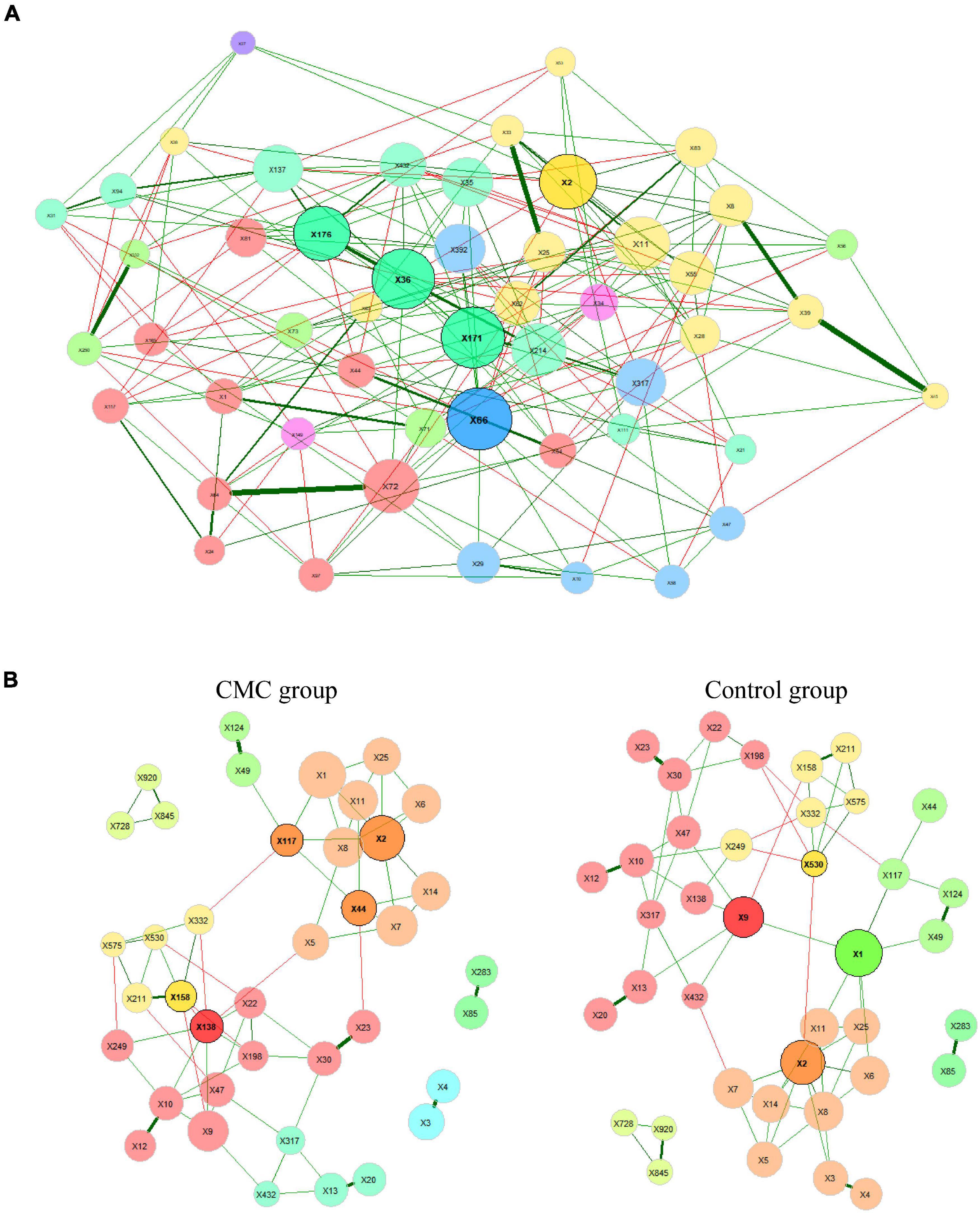
Figure 5. Bacterial associations of samples in two groups. The SPRING method is used as an association measure. The estimated partial correlations are transformed into dissimilarities via the “signed” distance metric and the corresponding (non-negative) similarities are used as edge weights. Green edges correspond to positive estimated associations and red edges to negative ones. Eigenvector centrality is used for defining hubs (nodes with a centrality value above the empirical 95% quantile) and scaling node sizes. Hubs are highlighted by black borders. Node colors represent clusters, which are determined using greedy modularity optimization. The 100 most abundant taxa were analyzed in this part and (A) the complete association network where the 50 nodes with the highest degree are shown. (B) Comparison of bacterial associations in two groups. Centrality and clustering measures are adopted from the complete network. Species represented by the nodes are given in Supplementary Table 5.
Comparing networks between control group and cow milk consumption group
We further constructed the taxa interplay network for the control group and the CMC group and found that the GM’s association diversified across CMC status. First, we compared the hub taxa between the two groups (j = 0.125) (Table 5): s__Ruminococcus_gnavus (X117), s__Bacteroides_unclassified (X2), s__Clostridium_sp._CAG.7 (X44), s__Prevotella_copri (X158), and s__Roseburia_sp._CAG.18 (X138) were the five most significant hub taxa in the CMC group, but s__unclassified (X1), s__Faecalibacterium_prausnitzii (X9), s__Bacteroides_unclassified (X2), and s__Prevotella_sp._CAG.386 (X530) were hub taxa in the control group (Figure 5B). Second, as shown in Table 6, the degree, betweenness centrality, closeness centrality, and eigenvector centrality of certain nodes were different across groups. For example, s__Clostridium_sp._CAG.7 (X44) had a higher degree in the CMC group while s__Prevotella_sp._CAG.386 (X530) had a higher degree in the control group. Third, the overall characteristics of the two networks also diversified, especially the most central nodes defined regarding betweenness centrality (j = 0.077) and closeness centrality (j = 0.000) (Table 5). Finally, the network clustering solution was also dissimilar (adjusted Rand index = 0.0157). As shown in Figure 5B, an apparent dissimilarity of the network clustering across groups is that the cluster comprising s__Bacteroides_stercoris (X3) and s__Bacteroides_stercoris_CAG.120 (X4) only existed in the CMC group. In the control group, these two bacteria were assigned to a large cluster connected by the correlation of s__Bacteroides_stercoris (X3) with s__Bacteroidales_unclassified (X8) and s__Bacteroides_unclassified (X2).
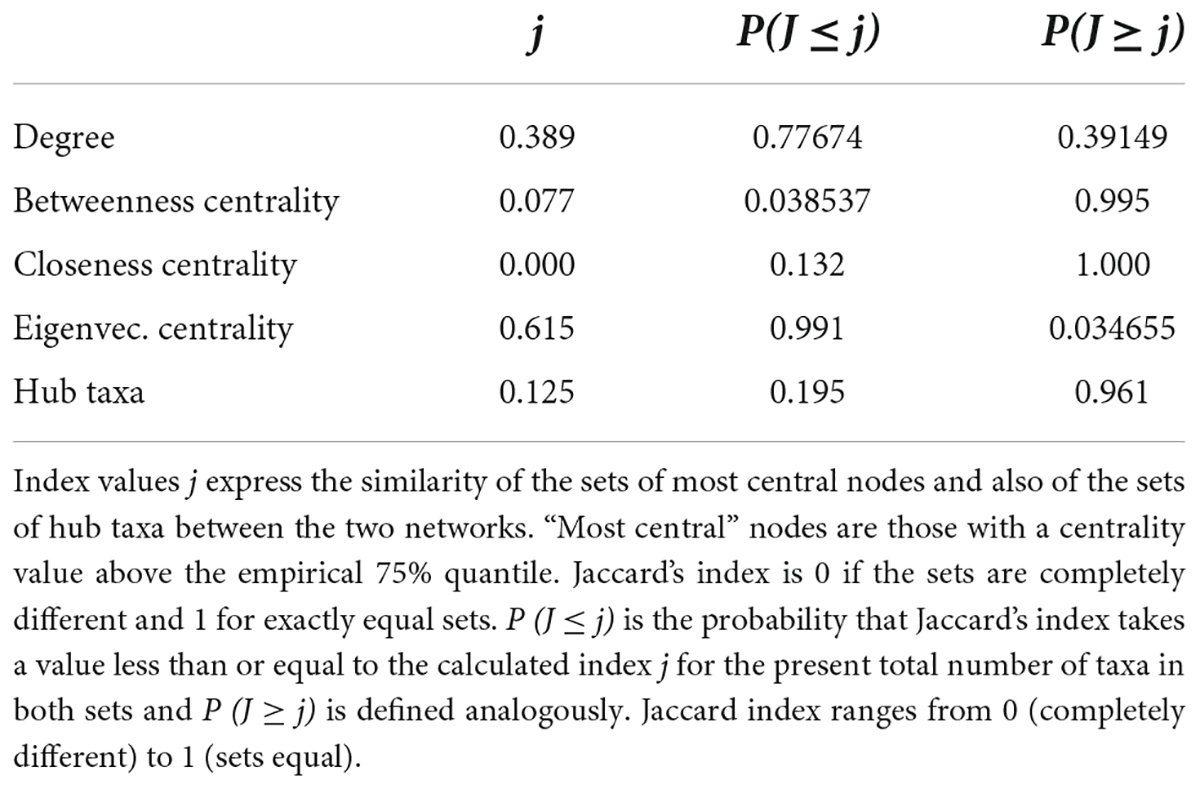
Table 5. Jaccard index values corresponding to the networks shown in Figure 5B.
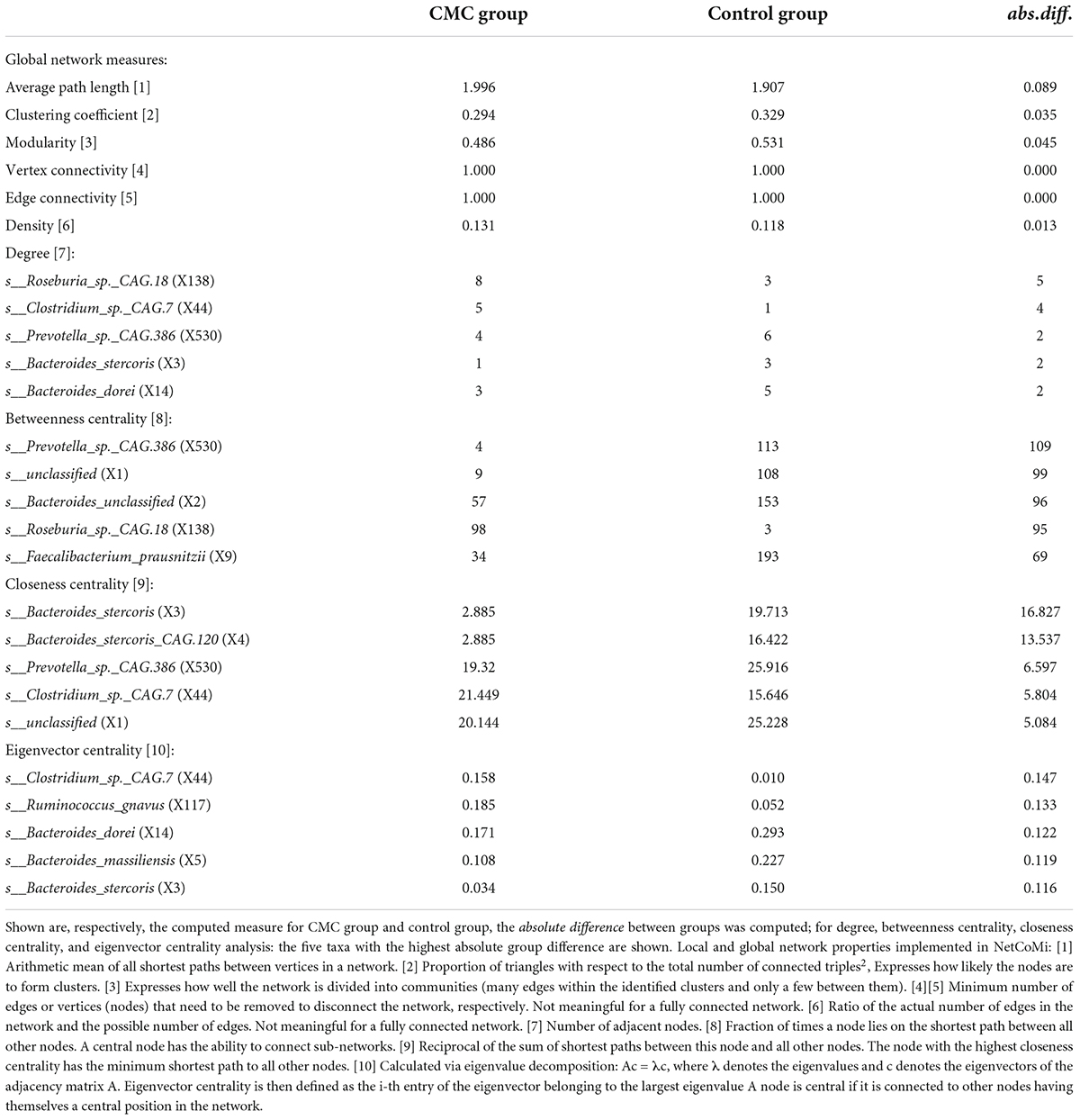
Table 6. Results from testing global network metrics and centrality measures of the networks in Figure 5B.
Cow milk consumption-associated functional modules
A total of 596 KEGG modules were analyzed, and 48 of them diversified significantly (p < 0.05) across the groups as detected by the Mann–Whitney test (Figure 6). Of these, 38 significant modules increased in the CMC group, including dissimilatory sulfate reduction (p = 0.0016); PTS system, lactose-specific II component (p = 0.0021); PTS system, fructose-specific II component (p = 0.0035); pimeloyl-ACP biosynthesis (p = 0.005), and others. Ten modules, including Vibrio cholerae pathogenicity signature, cholera toxins (p = 9.52e-04), and cephamycin C biosynthesis module (p = 0.0057), among others, decreased in the CMC group (Figure 6).
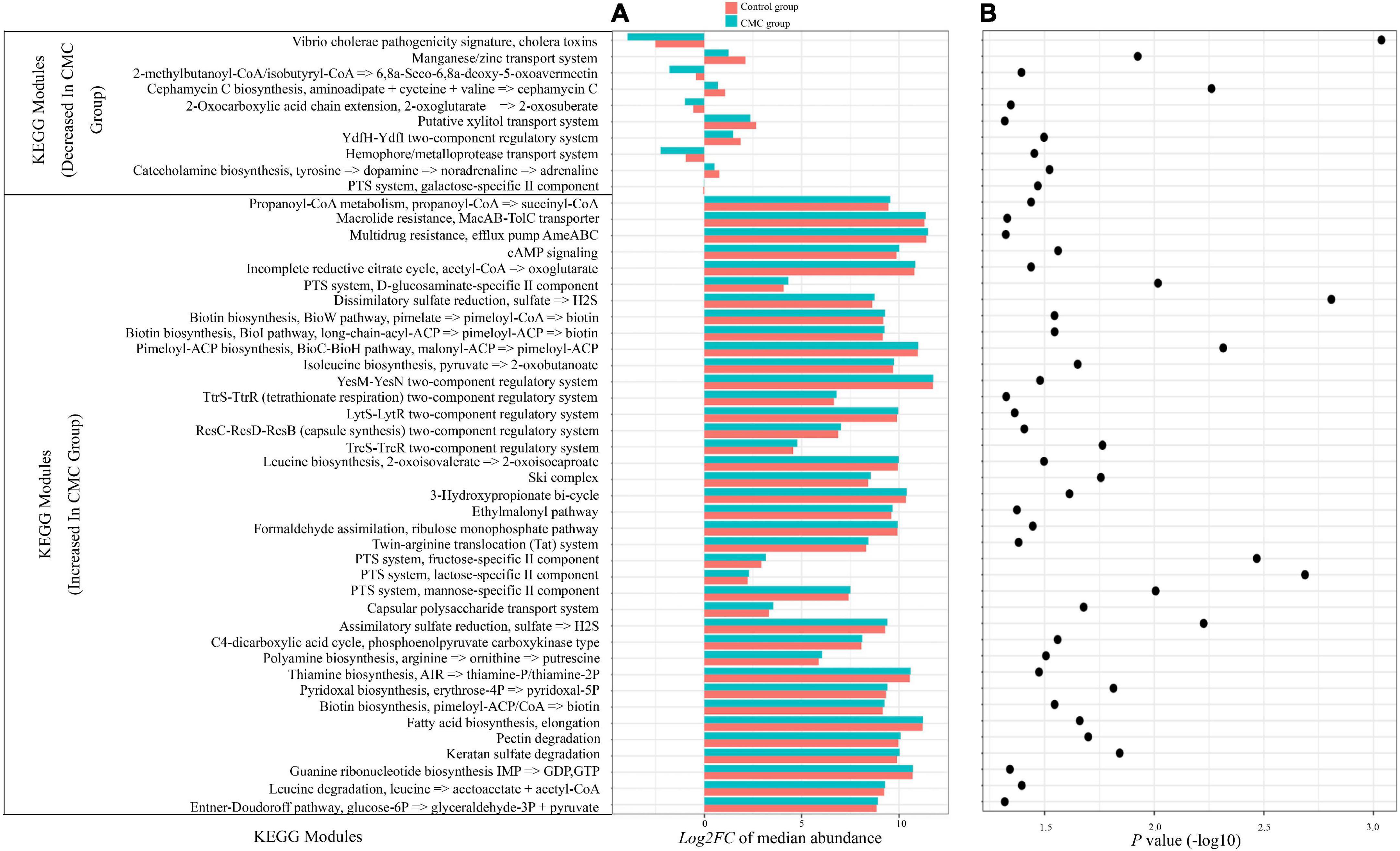
Figure 6. CMC alters the functional potential of the gut microbiome. (A) Microbial genes annotated to Kyoto Encyclopedia of Genes and Genomes (KEGG) orthologs (KOs). The bar chart displays the log2 fold change of the relative abundance median of all individual KOs within a module following the Control group (pink bars) or the CMC group (blue bars), respectively. (B) Dot plot of the negative log10 of the P-value from the Mann–Whitney test of KEGG module abundance of two groups.
Validation
The basic characteristics of the American cohort were shown in Supplementary Table 6. No significant difference in either postmenopausal status (p = 0.494) or BMI (p = 0.484), or exercise (p = 0.281) (Supplementary Table 6) was detected between the CMC group and the control group. Just same as that in the Chinese cohort, the data were normalized via TSS or CLR and LEfSe was used to detect the differential microbiota across groups in the American cohort. As is shown in Table 7, s__Bacteroides_salyersiae (P1 = 0.010, P2 = 0.044) and s__Bifidobacterium_pseudocatenulatum (P1 = 0.002, P2 = 0.016) showed consistent association results in both American cohort and Chinese cohort.
Discussion
Cow’s milk is conventionally considered to be beneficial. In this research, we tried to elucidate the association of CMC with human gut microbiota composition and functional modules, which will be helpful for understanding the possible mechanisms of its effects on human health.
Cow milk consumption associated with gut microbiotas composition features
We first computed the number of taxa and determined the dominating taxa in the control and CMC groups. The dominated taxa in the two groups were the same, which indicated that CMC may not influence human’s most abundant gut microbiota. We also compared the alpha and beta diversity between groups. Several research have studied the association of CMC with GM diversity; however, their results were inconsistent. Fernandez-Raudales et al. (2012) reported that CMC was negatively associated with alpha diversity; Bendtsen et al. (2018) concluded that there’s no association between CMC and alpha/beta diversity); Aslam et al. (2021) reported that beta diversity of gut microbiota differed among milk consumer and non-consumers. In the current study, we figured that the two groups had different beta diversity at levels of genus and species, while no difference in alpha diversity between groups was found. This was partially consistent with Bendtsen and Hajara Aslam’s results.
Cow milk consumption associated with the relative abundance of some taxa
Following the analysis of GM general composition features, we detected the association (by Mann–Whitney test) and correlation (by LEfSe) between RA of taxa and CMC status in two cohorts. It was found that the abundance of certain taxa was significantly different between the control group and the CMC group.
s_Bifidobacterium_pseudocatenulatum, g_Bifidobacterium, and p_Actinobacteria
The abundance of s_Bifidobacterium_pseudocatenulatum was positively associated with CMC in both the Chinese and American cohorts (P < 0.05, and LDA > 2 in both cohorts). Such association has not been reported before. It has been reported that a strain of s_B. pseudocatenulatum could reverse the adverse effects of diet-induced obesity through the gut-bone axis (Fernández-Murga et al., 2020). Some studies also show an advantage of s_B.pseudocatenulatum in treating obesity-associated diseases (Mauricio et al., 2017; Sanchis-Chordà et al., 2019). In addition, s_B.pseudocatenulatum was reported to be beneficial for human health as an inflammation regulator (Moya-Pérez et al., 2015; Sanchis-Chordà et al., 2019). It could also ameliorate gut flora dysbiosis, especially the depletion of the SCFA-producing bacteria Anaerostipes (Muñoz-Tamayo et al., 2011). In our results, s_B.pseudocatenulatum was positively associated with CMC. We hypothesized that CMC is likely to promote human health at least partially through the positive effect of gut microbiota s_B. pseudocatenulatum.
s_B.pseudocatenulatum belongs to genus g__Bifidobacterium (a branch of p_Actinobacteria). Of note, in the Chinese cohort, both p_Actinobacteria and g_Bifidobacterium were detected to be negatively associated with CMC (FDR < 0.1 and LDA < −2). The association of CMC with p_Actinobacteria has not been reported before. An explanation for the decrease of g_Bifidobacterium observed in the CMC group might be that milk is a provider of calcium since Shili et al. (2020) showed that subjects with reduced calcium consumption had a higher abundance of g_Bifidobacterium.
g_Anaerostipes
An increase in abundance of g_Anaerostipes was observed in the CMC group in our research. Although this association had not been replicated in the validation American cohort, it was consistent with Xq. Li.’s conclusion that whole milk consumption significantly increased Anaerostipes (p < 0.01) (Li et al., 2018). The increase of the bacteria g_Anaerostipes in the CMC group may also contribute to intestinal health. Genus g_Anaerostipes is a branch of phylum Firmicutes, which is a dominant bacterial taxon in the human gut. The anaerobic bacteria g_Anaerostipes can produce butyrate from lactic acid (Muñoz-Tamayo et al., 2011; Thomas et al., 2014). Our genomic sequencing data also confirmed the potential butyrate-producing capability of g_Anaerostipes since it encoded genes of the “Reductive acetyl-CoA pathway (Wood-Ljungdahl pathway)”. This is an important pathway involved in the biosynthesis of SCFAs butyrate (Portincasa et al., 2022). Butyrate is an SCFA responsible for regulating mucosal gene expression and maintaining gut barrier integrity. It can regulate the release of insulin and glucagon and provide energy for host cells (Hague et al., 1997). Butyrate also inhibits histone deacetylase-induced apoptosis of colon cells and activates gluconeogenesis through a cAMP-dependent mechanism (Davie, 2003). In another study, the Anaerostipes genus was associated with a higher estimated glomerular filtration rate in the overall population and non-diabetes mellitus subgroup, which also indicated that g_Anaerostipes is beneficial for renal function (Thomas et al., 2014). Species s__Anaerostipes_hadrus (P = 7.32e-4, LDA score = 3.21), which belongs to g_Anaerostipes, were also identified to be positively associated with CMC in our study.
s__Bacteroides_salyersiae and g_Bacteroides
Relative abundance (RA) of s__Bacteroides_salyersiae diversified in both Chinese and American cohorts (p < 0.05). This species belongs to g_Bacteroides, which was observed to increase (p = 0.03, LDA = 5.29) in the CMC group in the Chinese cohort. This is consistent with a report showing that an increase of g_Bacteroides usually results from the long-term intake of protein and animal fat (Wu et al., 2011). g_Bacteroides were reported to be beneficial for human health. Aerobic exercise can increase g_Bacteroides abundance and thus improve cardiopulmonary function (Morita et al., 2019). It is also an inflammation regulator; a decrease in g_Bacteroides level can lead to a decrease in inflammatory cytokines (Fan et al., 2019). Another study has shown that g_Bacteroides can activate T cells in the bodies of infants, thereby promoting their immune system development (Walker and Iyengar, 2015).
Cow milk consumption may alter gut microbiota association network
We further studied the GM association network in each group via the NetCoMi package to better understand the complex interplay of microbial communities. We compared the networks of subjects with different CMC statuses and found that they were essentially different. We observed different hub taxa in the CMC group compared with the control group, which indicated that CMC may at least partially change the interaction of GM. Hub taxa usually act as connector nodes linking multiple clusters/modules in the network, thus their tiny abundance changes may affect the balance of microbe clusters apparently. By comparing the five centrality measures of each taxon and the network clustering between the CMC and control groups, s__Bacteroides_stercoris (X3) attracted our attention. The degree, the closeness centrality, and the eigenvector centrality of s__Bacteroides_stercoris all changed a lot across groups. The correlations of s__Bacteroides_stercoris with s__Bacteroides_unclassified and s__Bacteroidales unclassified disappeared in the CMC group, which directly altered the clustering of the network. In this study, the RA of s__Bacteroides_stercoris was a little bit higher in the CMC group than the control group, but the difference was not statistically significant. Recently, Gaundal et al. (2022) reported that the abundance of human gut Bacteroides Stercoris was associated with higher adherence to Healthy Nordic Food Index (HNFI) and lower diastolic blood pressure. However, the milk intake was not studied in that research. Based on the changes of the five centrality measures (degree, betweenness centrality, closeness centrality, eigenvector centrality, and hub taxa), there were also some other bacteria that should be highlighted. They are s__Ruminococcus_gnavus (X117), s__Faecalibacterium_prausnitzii (X9), s__Prevotella_copri (X158), s__Clostridium_sp._CAG.7 (X44), s__Roseburia_sp._CAG.18 (X138), s__Prevotella_sp._CAG.386 (X530), and s__Bacteroides_dorei (X14). Further studies of them, especially those focused on their interplay with other microbes, should be helpful for elucidating CMC’s effects on GM.
Cow milk consumption associated with richness of functional categories
Following the GM profile analysis, we sought to identify the varied modules between groups and found that many modules diversified greatly. The most striking result is that the KEGG module M00850 (Vibrio cholerae pathogenicity signature, cholera toxins) was decreased in the CMC group (p = 9.52e-04). The reduction of M00850 came from the reduced richness of gut pathogenic bacteria carrying genes ctxB and rtxA, which may induce gastroenteritis in humans (Fang et al., 2019). The reduction of M00850 in the CMC group indicated that CMC habit may be good for maintaining the correct balance between helpful bacteria and harmful bacteria. Cephamycin C biosynthesis module also decreased in the CMC group (p = 0.0057). According to our metagenome sequencing results, Cephamycin C biosynthesis genes were encoded by the s__Clostridiales_bacterium, s__Paenibacillus_chitinolyticus, s__Paenibacillus_sp._G4, and so on. The inhibition of cephamycin C biosynthesis may also be beneficial to gut probiotics, as it is a kind of antibiotic and may affect the balance of intestinal flora.
A total of 38 KEGG biology modules were positively associated with CMC, four of which are correlated to biotin synthesis. Biotin is a B-complex vitamin that acts as an essential coenzyme for five carboxylases. These carboxylases participate in several chemical processes in the cell, including gluconeogenesis, amino acid metabolism, and fatty acid synthesis. Mammals obtain biotin from food, but it can also be produced from gut bacteria (Saleem and Soos, 2022); According to our metagenome sequencing results, biotin synthesis genes were encoded by a lot of microbes such as s__Anaerostipes_hadrus, s__Escherichia_coli, s__Bacteroides_vulgatus, s__Bacteroides_dorei, and so on. Biotin regulates immunological and inflammatory functions. It plays a key role in the function of natural killer (NK) lymphocytes and the generation of cytotoxic T lymphocytes (Kuroishi, 2015; Agrawal et al., 2016). Our results indicated that CMC could improve the gut bacteria’s biotin synthesis and thus should be good for host health.
We also observed alterations in the richness of PTS-related modules. Module galactose-specific component decreased in the CMC group while modules mannose-, lactose-, fructose-, and D-glucosaminate-specific II component increased in the CMC group. It was indicated that CMC may influence the proportional composition of gut microbiota which utilize different PTS-related enzymes.
The strengths of the present study include: (1) We applied shotgun sequencing technology and detected the RA of taxa at the species level; (2) The GM sequencing was done in a relatively large cohort and the statistical power of our study was well guaranteed. We did the power assessment with a recently published R package “Powmic” (Chen, 2020), which enables power analysis for metagenomic sequencing case-control study for identifying differentially abundant microbes. For non-parametric Wilcoxon rank sum test, given a nominal FDR level of 0.1, with our dataset (N1 = 248, N2 = 146, and the taxa filtering criteria used in this study), the overall power evaluation results are as follows: empirical statistical Power “true positive rate” TPR[TPR = TP/(TP+FN)] = 0.81, False Positive Rate FPR[FPR = FP/(FP+TP)] < 0.00001; (3) The data processing and filter were strict.
The weaknesses of our research are as follows: (1) All subjects were women, so it is not known whether CMC has similar effects on men; (2) Our research was cross-sectional. Although we have determined the correlations between GM and CMC, further studies are still needed to elucidate its mechanisms; (3) This study does not allow for controlling all potential confounding factors that might have effect on human gut microbiome, which may lead to some statistical artifacts; (4) In this study, we used an American cohort as the validation cohort. There should be great differences between the Chinese cohort and the American cohort in the genetic background of the host, the lifestyles, or maybe the gastrointestinal microbial community environment. This may be one of the reasons why only two differential taxa are being replicated in the American cohort. We encourage researchers to use a validation cohort that is as similar as possible to the discovery cohort.
Overall, we have identified several gut microbiota taxa and modules significantly associated with CMC in the present study. Some of the positively associated differential taxa or functional modules have been reported to have positive effects on humans health. Women at peri/postmenopausal stage usually suffered from many kinds of diseases, such as osteoporosis, breast cancer, and obesity. Further studies are still needed to elucidate the effects and underlying mechanisms of CMC habit on peri/postmenopausal women’s health that is mediated by the gut microbiome.
Conclusion
The present study has revealed alterations in gut bacterial composition and functional modules associated with CMC. These results suggest that cow milk consumption was associated with the beta diversity and abundance of some beneficial bacterial taxa such as s__B.pseudocatenulatum, g_Anaerostipes, and g_Bacteroides. In addition, cow milk consumption was associated with the abundance of many functional modules such as Vibrio cholerae pathogenicity signature, cholera toxins, and biotin synthesis, which further support the biological value of habitual cow milk consumption.
Data availability statement
The datasets presented in this study can be found in online repositories. The names of the repository/repositories and accession number(s) can be found below: https://www.ebi.ac.uk/ena, PRJEB50761.
Ethics statement
The studies involving human participants were reviewed and approved by The Medical Ethical Committees of Southern Medical University. The patients/participants provided their written informed consent to participate in this study. Written informed consent was obtained from the individual(s) for the publication of any potentially identifiable images or data included in this article.
Author contributions
HS, CQ, ZL, and L-JZ: subject enrollment. XL, H-MX, QY, and XC: conceptualization. J-HY and L-DJ: data curation. BT, J-HY, W-QL, and Z-QW: formal analysis. H-WD: funding acquisition. L-DJ and L-LX: investigation. HX: methodology. JS, L-SZ, and H-WD: supervision. BT: writing – original draft. BT, L-SZ, and H-WD: writing – review and editing. All authors contributed to the article and approved the submitted version.
Funding
H-WD was partially supported by grants from the National Institutes of Health (U19AG05537301, R01AR069055, P20GM109036, R01MH104680, and R01AG061917) and the Edward G. Schlieder Endowment from Tulane University. XC was partially supported by the Research Foundation of the Ministry of Education of China (8091B022138). HX was partially supported by the Beijing Natural Science Foundation (7214242). JS was partially supported by grants from the Science and Technology Program of Guangzhou, China (201604020007), and the National Natural Science Foundation of China (81770878). H-MX was partially supported by the National Key R&D Program of China (2016YFC1201805 and 2017YFC1001100).
Acknowledgments
We thank the National Institutes of Health for financial support and LC-Bio Technologies (Hangzhou) CO., LTD. (Hangzhou, China, www.lc-bio.com) for technical support for our research.
Conflict of interest
The authors declare that the research was conducted in the absence of any commercial or financial relationships that could be construed as a potential conflict of interest.
Publisher’s note
All claims expressed in this article are solely those of the authors and do not necessarily represent those of their affiliated organizations, or those of the publisher, the editors and the reviewers. Any product that may be evaluated in this article, or claim that may be made by its manufacturer, is not guaranteed or endorsed by the publisher.
Supplementary material
The Supplementary Material for this article can be found online at: https://www.frontiersin.org/articles/10.3389/fmicb.2022.957885/full#supplementary-material
Footnotes
- ^ www.lc-bio.com
- ^ ftp://ftp.ncbi.nlm.nih.gov/blast/db/FASTA/nr.gz
- ^ https://www.genome.jp/kegg/module.html
References
Agrawal, S., Agrawal, A., and Said, H. M. (2016). Biotin deficiency enhances the inflammatory response of human dendritic cells. Am. J. Physiol. Cell. Physiol. 311, C386–C391. doi: 10.1152/ajpcell.00141.2016
Aslam, H., Collier, F., Davis, J. A., Quinn, T. P., O’Hely, M., Pasco, J. A., et al. (2021). Gut microbiome diversity and composition are associated with habitual dairy intakes: a cross-sectional study in men. J. Nutr. 151, 3400–3412. doi: 10.1093/jn/nxab252
Aslam, H., Marx, W., Rocks, T., Loughman, A., Chandrasekaran, V., Ruusunen, A., et al. (2020). The effects of dairy and dairy derivatives on the gut microbiota: a systematic literature review. Gut Microbes. 12:1799533. doi: 10.1080/19490976.2020.1799533
Badri, M., Kurtz, Z. D., Bonneau, R., and Müller, C. L. (2020). Shrinkage improves estimation of microbial associations under different normalization methods. NAR Genom. Bioinform 2:lqaa100. doi: 10.1093/nargab/lqaa100
Beaumont, M., Portune, K. J., Steuer, N., Lan, A., Cerrudo, V., Audebert, M., et al. (2017). Quantity and source of dietary protein influence metabolite production by gut microbiota and rectal mucosa gene expression: a randomized, parallel, double-blind trial in overweight humans. Am. J. Clin. Nutr. 106, 1005–1019. doi: 10.3945/ajcn.117.158816
Bendtsen, L. Q., Blædel, T., Holm, J. B., Lorenzen, J. K., Mark, A. B., Kiilerich, P., et al. (2018). High intake of dairy during energy restriction does not affect energy balance or the intestinal microflora compared with low dairy intake in overweight individuals in a randomized controlled trial. Appl. Physiol. Nutr. Metab. 43, 1–10. doi: 10.1139/apnm-2017-0234
Botteri, E., Iodice, S., Bagnardi, V., Raimondi, S., Lowenfels, A. B., and Maisonneuve, P. (2008). Smoking and colorectal cancer: a meta-analysis. JAMA 300, 2765–2778. doi: 10.1001/jama.2008.839
Cardinali, N., Bauman, C., Rodriguez Ayala, F., and Grau, R. (2020). Two cases of type 2 diabetes mellitus successfully treated with probiotics. Clin. Case Rep. 8, 3120–3125. doi: 10.1002/ccr3.3354
Chatterton, D. E., Nguyen, D. N., Bering, S. B., and Sangild, P. T. (2013). Anti-inflammatory mechanisms of bioactive milk proteins in the intestine of newborns. Int. J. Biochem. Cell Biol. 45, 1730–1747. doi: 10.1016/j.biocel.2013.04.028
Chen, L. (2020). powmic: an R package for power assessment in microbiome case-control studies. Bioinformatics 36, 3563–3565. doi: 10.1093/bioinformatics/btaa197
Cuevas-Sierra, A., Ramos-Lopez, O., Riezu-Boj, J. I., Milagro, F. I., and Martinez, J. A. (2019). Diet, gut microbiota, and obesity: links with host genetics and epigenetics and potential applications. Adv. Nutr. 10(Suppl. 1), S17–S30. doi: 10.1093/advances/nmy078
Davie, J. R. (2003). Inhibition of histone deacetylase activity by butyrate. J. Nutr. 133(Suppl. 7), 2485s–2493s. doi: 10.1093/jn/133.7.2485S
de Carvalho, N. M., Oliveira, D. L., Costa, C. M., Pintado, M., and Madureira, A. R. (2022). Can supplemented skim milk (SKM) boost your gut health? Fermentation 8:126. doi: 10.3390/fermentation8030126
Fan, H., Du, J., Liu, X., Zheng, W. W., Zhuang, Z. H., Wang, C. D., et al. (2019). Effects of pentasa-combined probiotics on the microflora structure and prognosis of patients with inflammatory bowel disease. Turk. J. Gastroenterol. 30, 680–685. doi: 10.5152/tjg.2019.18426
Fang, L., Ginn, A. M., Harper, J., Kane, A. S., and Wright, A. C. (2019). Survey and genetic characterization of Vibrio cholerae in Apalachicola Bay, Florida (2012-2014). J. Appl. Microbiol. 126, 1265–1277. doi: 10.1111/jam.14199
Fernández-Murga, M. L., Olivares, M., and Sanz, Y. (2020). Bifidobacterium pseudocatenulatum CECT 7765 reverses the adverse effects of diet-induced obesity through the gut-bone axis. Bone 141:115580. doi: 10.1016/j.bone.2020.115580
Fernandez-Raudales, D., Hoeflinger, J. L., Bringe, N. A., Cox, S. B., Dowd, S. E., Miller, M. J., et al. (2012). Consumption of different soymilk formulations differentially affects the gut microbiomes of overweight and obese men. Gut Microbes 3, 490–500. doi: 10.4161/gmic.21578
Gatti, D., and Fassio, A. (2019). Pharmacological management of osteoporosis in postmenopausal women: the current state of the art. J. Popul. Ther. Clin. Pharmacol. 26, e1–e17. doi: 10.15586/jptcp.v26.i4.646
Gaundal, L., Myhrstad, M. C. W., Rud, I., Gjøvaag, T., Byfuglien, M. G., Retterstøl, K., et al. (2022). Gut microbiota is associated with dietary intake and metabolic markers in healthy individuals. Food Nutr. Res. 66:8580. doi: 10.29219/fnr.v66.8580
Gilbert, J. A., Blaser, M. J., Caporaso, J. G., Jansson, J. K., Lynch, S. V., and Knight, R. (2018). Current understanding of the human microbiome. Nat. Med. 24, 392–400. doi: 10.1038/nm.4517
Hague, A., Singh, B., and Paraskeva, C. (1997). Butyrate acts as a survival factor for colonic epithelial cells: further fuel for the in vivo versus in vitro debate. Gastroenterology 112, 1036–1040. doi: 10.1053/gast.1997.v112.agast971036
Ilesanmi-Oyelere, B. L., and Kruger, M. C. (2020). The role of milk components, Pro-, Pre-, and synbiotic foods in calcium absorption and bone health maintenance. Front. Nutr. 7:578702. doi: 10.3389/fnut.2020.578702
Jie, Z., Liang, S., Ding, Q., Li, F., Sun, X., Lin, Y., et al. (2021). Dairy consumption and physical fitness tests associated with fecal microbiome in a Chinese cohort. Med. Microecol. 9:100038. doi: 10.1016/j.medmic.2021.100038
Kuroishi, T. (2015). Regulation of immunological and inflammatory functions by biotin. Can. J. Physiol. Pharmacol. 93, 1091–1096. doi: 10.1139/cjpp-2014-0460
Ley, R. E., Hamady, M., Lozupone, C., Turnbaugh, P. J., Ramey, R. R., Bircher, J. S., et al. (2008). Evolution of mammals and their gut microbes. Science 320, 1647–1651. doi: 10.1126/science.1155725
Li, X., Yin, J., Zhu, Y., Wang, X., Hu, X., Bao, W., et al. (2018). Effects of whole milk supplementation on gut microbiota and cardiometabolic biomarkers in subjects with and without lactose malabsorption. Nutrients 10:1403. doi: 10.3390/nu10101403
Link-Amster, H., Rochat, F., Saudan, K. Y., Mignot, O., and Aeschlimann, J. M. (1994). Modulation of a specific humoral immune response and changes in intestinal flora mediated through fermented milk intake. FEMS Immunol. Med. Microbiol. 10, 55–63. doi: 10.1111/j.1574-695X.1994.tb00011.x
Mason, M. R., Preshaw, P. M., Nagaraja, H. N., Dabdoub, S. M., Rahman, A., and Kumar, P. S. (2015). The subgingival microbiome of clinically healthy current and never smokers. ISME J. 9, 268–272. doi: 10.1038/ismej.2014.114
Mauricio, M. D., Serna, E., Fernández-Murga, M. L., Portero, J., Aldasoro, M., Valles, S. L., et al. (2017). Bifidobacterium pseudocatenulatum CECT 7765 supplementation restores altered vascular function in an experimental model of obese mice. Int. J. Med. Sci. 14, 444–451. doi: 10.7150/ijms.18354
McMurdie, P. J., and Holmes, S. (2014). Waste not, want not: why rarefying microbiome data is inadmissible. PLoS Comput. Biol. 10:e1003531. doi: 10.1371/journal.pcbi.1003531
Morita, E., Yokoyama, H., Imai, D., Takeda, R., Ota, A., Kawai, E., et al. (2019). Aerobic exercise training with brisk walking increases intestinal bacteroides in healthy elderly women. Nutrients 11:868. doi: 10.3390/nu11040868
Moya-Pérez, A., Neef, A., and Sanz, Y. (2015). Bifidobacterium pseudocatenulatum CECT 7765 reduces obesity-associated inflammation by restoring the lymphocyte-macrophage balance and gut microbiota structure in high-fat diet-fed mice. PLoS One 10:e0126976. doi: 10.1371/journal.pone.0126976
Muñoz-Tamayo, R., Laroche, B., Walter, E., Doré, J., Duncan, S. H., Flint, H. J., et al. (2011). Kinetic modelling of lactate utilization and butyrate production by key human colonic bacterial species. FEMS Microbiol. Ecol. 76, 615–624. doi: 10.1111/j.1574-6941.2011.01085.x
Odamaki, T., Kato, K., Sugahara, H., Xiao, J. Z., Abe, F., and Benno, Y. (2016). Effect of probiotic yoghurt on animal-based diet-induced change in gut microbiota: an open, randomised, parallel-group study. Benef. Microbes 7, 473–484. doi: 10.3920/bm2015.0173
Odamaki, T., Sugahara, H., Yonezawa, S., Yaeshima, T., Iwatsuki, K., Tanabe, S., et al. (2012). Effect of the oral intake of yogurt containing Bifidobacterium longum BB536 on the cell numbers of enterotoxigenic Bacteroides fragilis in microbiota. Anaerobe 18, 14–18. doi: 10.1016/j.anaerobe.2011.11.004
Ozaki, D., Kubota, R., Maeno, T., Abdelhakim, M., and Hitosugi, N. (2021). Association between gut microbiota, bone metabolism, and fracture risk in postmenopausal Japanese women. Osteoporos Int. 32, 145–156. doi: 10.1007/s00198-020-05728-y
Pereira, P. C. (2014). Milk nutritional composition and its role in human health. Nutrition 30, 619–627. doi: 10.1016/j.nut.2013.10.011
Peschel, S., Müller, C. L., von Mutius, E., Boulesteix, A. L., and Depner, M. (2021). NetCoMi: network construction and comparison for microbiome data in R. Brief. Bioinform 22:bbaa290. doi: 10.1093/bib/bbaa290
Portincasa, P., Bonfrate, L., Vacca, M., De Angelis, M., Farella, I., Lanza, E., et al. (2022). Gut microbiota and short chain fatty acids: implications in glucose homeostasis. Int. J. Mol. Sci. 23:1105. doi: 10.3390/ijms23031105
Rao, V. P., Poutahidis, T., Ge, Z., Nambiar, P. R., Horwitz, B. H., Fox, J. G., et al. (2006). Proinflammatory CD4+ CD45RB(hi) lymphocytes promote mammary and intestinal carcinogenesis in Apc(Min/+) mice. Cancer Res. 66, 57–61. doi: 10.1158/0008-5472.Can-05-3445
Reimer, R. A., Willis, H. J., Tunnicliffe, J. M., Park, H., Madsen, K. L., and Soto-Vaca, A. (2017). Inulin-type fructans and whey protein both modulate appetite but only fructans alter gut microbiota in adults with overweight/obesity: a randomized controlled trial. Mol. Nutr. Food Res. 61:1700484. doi: 10.1002/mnfr.201700484
Rettedal, E. A., Ilesanmi-Oyelere, B. L., Roy, N. C., Coad, J., and Kruger, M. C. (2021). The Gut Microbiome Is Altered in Postmenopausal Women With Osteoporosis and Osteopenia. JBMR Plus 5:e10452. doi: 10.1002/jbm4.10452
Saleem, F., and Soos M. P. (2022). Biotin Deficiency. StatPearls [Internet]. Treasure Island (FL): StatPearls Publishing. Available online at: https://www.ncbi.nlm.nih.gov/books/NBK547751/ (accessed Mar 2, 2022).
Sanchis-Chordà, J., Del Pulgar, E. M. G., Carrasco-Luna, J., Benítez-Páez, A., Sanz, Y., and Codoñer-Franch, P. (2019). Bifidobacterium pseudocatenulatum CECT 7765 supplementation improves inflammatory status in insulin-resistant obese children. Eur. J. Nutr. 58, 2789–2800. doi: 10.1007/s00394-018-1828-5
Shili, C. N., Broomhead, J. N., Spring, S. C., Lanahan, M. B., and Pezeshki, A. (2020). A novel corn-expressed phytase improves daily weight gain, protein efficiency ratio and nutrients digestibility and alters fecal microbiota in pigs fed with very low protein diets. Animals (Basel) 10:1926. doi: 10.3390/ani10101926
Shuai, M., Zuo, L. S., Miao, Z., Gou, W., Xu, F., Jiang, Z., et al. (2021). Multi-omics analyses reveal relationships among dairy consumption, gut microbiota and cardiometabolic health. EBioMedicine 66:103284. doi: 10.1016/j.ebiom.2021.103284
Swarte, J. C., Eelderink, C., Douwes, R. M., Said, M. Y., Hu, S., Post, A., et al. (2020). Effect of high versus low dairy consumption on the gut microbiome: results of a randomized, cross-over study. Nutrients 12:2129. doi: 10.3390/nu12072129
Thai, J. D., and Gregory, K. E. (2020). Bioactive factors in human breast milk attenuate intestinal inflammation during early life. Nutrients 12:581. doi: 10.3390/nu12020581
Thomas, L. V., Ockhuizen, T., and Suzuki, K. (2014). Exploring the influence of the gut microbiota and probiotics on health: a symposium report. Br. J. Nutr. 112 (Suppl, 1), S1–S18. doi: 10.1017/s0007114514001275
Walker, W. A., and Iyengar, R. S. (2015). Breast milk, microbiota, and intestinal immune homeostasis. Pediatr. Res. 77, 220–228. doi: 10.1038/pr.2014.160
Wu, G. D., Chen, J., Hoffmann, C., Bittinger, K., Chen, Y. Y., Keilbaugh, S. A., et al. (2011). Linking long-term dietary patterns with gut microbial enterotypes. Science 334, 105–108. doi: 10.1126/science.1208344
Yılmaz, İ, Dolar, M. E., and Özıpnar, H. (2019). Effect of administering kefir on the changes in fecal microbiota and symptoms of inflammatory bowel disease: a randomized controlled trial. Turk. J. Gastroenterol. 30, 242–253. doi: 10.5152/tjg.2018.18227
Yoon, G., Gaynanova, I., and Müller, C. L. (2019). Microbial networks in SPRING - semi-parametric rank-based correlation and partial correlation estimation for quantitative microbiome data. Front. Genet. 10:516. doi: 10.3389/fgene.2019.00516
Keywords: cow milk consumption, metagenomic, gut microbiota, functional module, network
Citation: Tian B, Yao J-H, Lin X, Lv W-Q, Jiang L-D, Wang Z-Q, Shen J, Xiao H-M, Xu H, Xu L-L, Cheng X, Shen H, Qiu C, Luo Z, Zhao L-J, Yan Q, Deng H-W and Zhang L-S (2022) Metagenomic study of the gut microbiota associated with cow milk consumption in Chinese peri-/postmenopausal women. Front. Microbiol. 13:957885. doi: 10.3389/fmicb.2022.957885
Received: 31 May 2022; Accepted: 22 July 2022;
Published: 16 August 2022.
Edited by:
George Tsiamis, University of Patras, GreeceReviewed by:
Shimeng Huang, Jiangsu Academy of Agricultural Sciences, ChinaGiuseppe Jurman, Bruno Kessler Foundation (FBK), Italy
Copyright © 2022 Tian, Yao, Lin, Lv, Jiang, Wang, Shen, Xiao, Xu, Xu, Cheng, Shen, Qiu, Luo, Zhao, Yan, Deng and Zhang. This is an open-access article distributed under the terms of the Creative Commons Attribution License (CC BY). The use, distribution or reproduction in other forums is permitted, provided the original author(s) and the copyright owner(s) are credited and that the original publication in this journal is cited, in accordance with accepted academic practice. No use, distribution or reproduction is permitted which does not comply with these terms.
*Correspondence: Li-Shu Zhang, bHNoemhhbmdAYmp0dS5lZHUuY24=; Hong-Wen Deng, aGRlbmcyQHR1bGFuZS5lZHU=