- 1National Research Laboratory of Molecular Microbiology and Toxicology, Department of Agricultural Biotechnology, Seoul National University, Seoul, South Korea
- 2Department of Agricultural Biotechnology, Center for Food and Bioconvergence, Seoul National University, Seoul, South Korea
- 3Department of Agricultural Biotechnology, Research Institute of Agriculture and Life Science, Seoul National University, Seoul, South Korea
The elucidation of the transcriptional regulatory networks (TRNs) of enterohemorrhagic Escherichia coli (EHEC) is critical to understand its pathogenesis and survival in the host. However, the analyses of current TRNs are still limited to comprehensively understand their target genes generally co-regulated under various conditions regardless of the genetic backgrounds. In this study, independent component analysis (ICA), a machine learning-based decomposition method, was used to decompose the large-scale transcriptome data of EHEC into the modulons, which contain the target genes of several TRNs. The locus of enterocyte effacement (LEE) and the Shiga toxin (Stx) modulons mainly consisted of the Ler regulon and the Stx prophage genes, respectively, confirming that ICA properly grouped the co-regulated major virulence genes of EHEC. Further investigation revealed that the LEE modulon contained the hypothetical Z0395 gene as a novel member of the Ler regulon, and the Stx modulon contained the thi and cus locus genes in addition to the Stx prophage genes. Correspondingly, the Stx prophage genes were also regulated by thiamine and copper ions known to control the thi and cus locus genes, respectively. The modulons effectively clustered the genes co-regulated regardless of the growth conditions and the genetic backgrounds of EHEC. The changed activities of the individual modulons successfully explained the differential expressions of the virulence and survival genes during the course of infection in bovines. Altogether, these results suggested that ICA of the large-scale transcriptome data can expand and enhance the current understanding of the TRNs of EHEC.
Introduction
Transcriptional regulatory networks (TRNs) regulate the expression of the target genes for the pathogens to adapt to various environments. The understanding of TRNs and their target genes enables the prediction of molecular mechanisms by which pathogens cause disease and survive under host-specific conditions (Karmali, 2017). Advances in next-generation sequencing technologies facilitate analyzing the large-scale RNA-Seq and comparing the transcriptome of the pathogens grown under specific conditions or lacking a particular transcription factor(s) (TF) (Westermann et al., 2012; DuPai et al., 2020). However, the transcriptome data obtained from the genes expressed under specific experimental conditions or by a certain TF are still limited to comprehensively understand the TRNs and their target genes (Sastry et al., 2019; DuPai et al., 2020). Therefore, to overcome this limitation, studies have been performed to analyze bioinformatically the large-scale transcriptome data of the pathogens and to define the modulons, the independent sets of genes co-regulated under various conditions regardless of their genetic backgrounds (Saelens et al., 2018; Sastry et al., 2019; DuPai et al., 2020; Tan et al., 2020).
Enterohemorrhagic Escherichia coli (EHEC) causes a broad spectrum of human illnesses ranging from mild diarrhea to hemolytic uremic syndrome, often leaving permanent damage to the kidney (Karmali, 2017). The TRNs of Ler and Shiga toxin (Stx) prophage encoding the major virulence factors of EHEC have been studied extensively to understand the molecular pathogenesis of the pathogen. Ler, encoded by ler, regulates the locus of enterocyte effacement (LEE) genes necessary to form attaching and effacing (AE) lesions, the central pathogenesis of EHEC (Kenny et al., 1997; Mellies et al., 1999; Elliott et al., 2000; Tobe et al., 2006). Ler also regulates the genes encoding non-LEE-encoded effector (Nle) proteins crucial for forming AE lesions (Kelly et al., 2006; Li et al., 2006; Tobe et al., 2006), demonstrating that the Ler TRN contains additional non-LEE genes. Additionally, the Stx prophage TRNs include stx1 and stx2 of EHEC, located in the CP-933V and BP-933W prophages, respectively (Xu et al., 2012). The expressions of the Stx genes are regulated by the antiterminator Qs which allows the transcription of the prophage genes by preventing the formation of intrinsic terminators in their promoters (Casjens and Hendrix, 2015; Sy et al., 2020).
Meanwhile, TRNs also coordinate the expressions of the target genes for pathogens to survive under various growth conditions by recognizing the changes in the environmental signals. For example, the copper transport TRNs of EHEC consisting of cusCFBA involved in the detoxification of toxic heavy metals are induced by the high copper ions (Delmar et al., 2015). Other genes induced by environmental signals are generally suppressed by the global regulator H-NS encoded by hns (Atlung and Ingmer, 1997; Lang et al., 2007). Conversely, the target genes of certain TRNs also could be suppressed by the environmental signals. For example, The LEE genes of the Ler TRN are suppressed in the presence of indole, synthesized by the tryptophanase encoded by tna (Kumar and Sperandio, 2019). Similarly, the TRNs containing thiBP and thiCEFGH involved in the thiamine transport and biosynthesis, respectively, are also suppressed in the presence of thiamine (Vander Horn et al., 1993; Webb et al., 1998; Miranda-Rios et al., 2001).
In this study, independent component analysis (ICA), a machine learning method that decomposes a mixture of components into independent components (James and Hesse, 2005; Sastry et al., 2019; Tan et al., 2020), was used to decompose the large-scale transcriptome data of EHEC into the sets of independent modulon, which contains the target genes of several TRNs. The LEE and the Stx modulons mainly consisted of the target genes of the Ler and the Stx prophage TRNs, respectively, indicating that ICA properly grouped the sets of the co-regulated genes of EHEC into the modulons. Further investigation identified the Z0395 gene and the thi and cus locus genes as novel element genes of the LEE and Stx modulons, respectively. Accordingly, the Stx prophage genes were regulated by thiamine and copper ions known to control the thi and cus locus genes, respectively. Changed expressions of the modulons consisting of the inherently co-regulated genes also successfully explained the differential expressions of the virulence and survival genes of EHEC during the course of infection in bovines.
Materials and Methods
Generation of the Trimmed Transcriptome Data of Enterohemorrhagic Escherichia coli
The raw-sequencing reads of available RNA-Seq data of EHEC were retrieved from the Sequence Read Archive (SRA) database at the National Center for Biotechnology Information (NCBI)1 (see Supplementary Data Sheet 1). The reads were mapped to the reference genome of EHEC EDL933 (AE005174.2) using Spliced Transcripts Alignment to a Reference (STAR) (Dobin et al., 2013). The reads aligned to the reference genome were counted using the HTSeq (Anders et al., 2015). The genes with under ten fragments per million-mapped reads across the whole RNA-Seq data were removed before further analyses to ensure the quality of the data. The raw read counts were normalized using the trimmed mean of M values (TMM) method from the R edgeR package (Robinson et al., 2010; Robinson and Oshlack, 2010). The normalized data with R2 < 0.9 between biological replicates were discarded to trim the technical noise (Supplementary Figure 1A). The trimmed transcriptome data were log-transformed (log2 TMM + 1) for further analysis (see Supplementary Data Sheet 2).
Identification of the Modulons by Using Independent Component Analysis
Independent component analysis was conducted to the trimmed transcriptome data as previously described (Sastry et al., 2019). Firstly, the trimmed transcriptome data are centered by using the mean read counts of the transcriptome data of the EHEC EDL933 grown in M9 minimal medium. Then, ICA from the Scikit-learn Python package (Varoquaux et al., 2015), based on the hyperparameters of convergence tolerance of 10–8 and component number of 88 (the size of transcriptome data), was performed on the centered transcriptome data to construct the independent gene components. ICA was executed 256 times with random seeds, and the resulting independent gene components were clustered by using density-based spatial clustering of applications with noise (DBSCAN) to identify robust independent gene components (Ester et al., 1996). DBSCAN from the Scikit-learn Python package was conducted based on the hyperparameters of epsilon of 0.1 and minimum cluster seed size of 128 (50% of the execution times of ICA). In order to select the co-regulated genes of the robust independent components, the D’Agostino K2 test, which measures the skewness and kurtosis of distribution, was performed on the gene coefficients of the element genes in the independent components (D’Agostino et al., 1990). The element gene with the greatest absolute coefficient in each independent gene component was repeatedly removed, and the D’Agostino K2 test statistic was calculated for each removal. If the test statistic dropped below a cut-off, the removed genes were defined as the co-regulated genes of the independent component.
To determine the K2 test statistic cut-off, a two-sided Fisher’s exact test was performed between the previously known regulons of the E. coli regulators and the top 25 element genes of the independent gene components (Gama-Castro et al., 2011; Fang et al., 2017; Sastry et al., 2019). Among the regulators, the regulator with the lowest P-value was linked to each independent gene component. Then, the F1 scores were calculated between the regulons of the component-linked regulators and the co-regulated genes of the independent gene components selected based on the K2 test statistic cut-off varying from 1,500 to 2,500. Because the average of calculated F1 scores showed a maximum value at the K2 test statistic cut-off of 1,800 (Supplementary Figure 1D), the statistic cut-off was used to define the modulons. The independent components with less than 5 co-regulated genes were discarded, and thus the 64 modulons were identified from the 85 independent components. The 64 modulons were named after their related regulator or biological function (e.g., H-NS or LEE). Detailed information of the modulons, such as the related TF or biological function, the co-regulated genes, and the gene coefficients, was available in Supplementary Data Sheet 3.
Calculation of Cumulative Explained Variance for Principal Component Analysis and Independent Component Analysis
The principal component analysis (PCA) of the trimmed transcriptome data was performed with the Scikit-learn Python package (Varoquaux et al., 2015). The cumulative explained variance (CEV) for the PCA results was calculated by sequentially adding the explained variance ratios of the principal components using the Scikit-learn and NumPy Python packages (Varoquaux et al., 2015; Harris et al., 2020). The CEV for the ICA results was calculated as previously described in EEGLAB (Delorme and Makeig, 2004). The Matplotlib Python package was used to visualize the CEV for the PCA and ICA results (Hunter, 2007).
The Correlation Analyses of the Expression Levels of the Genes or the Activities of the Modulons
The expression levels of the genes and the activities of the modulons were obtained from Supplementary Data Sheet 2, 4, respectively. The Pearson correlation analyses between the expression levels of the genes and the activities of the modulons were performed with the SciPy Python package (Virtanen et al., 2020). The Pearson correlations between the expressions of the different genes were performed with the Pandas Python package (McKinney, 2010). The Matplotlib Python package was used to visualize the plots of the correlation analyses (Hunter, 2007).
Searching for the Ler Binding Site of the Z0395 Gene
The binding motif of Ler was discovered from the specific binding sequences of Ler, which were previously reported by Abe et al. (2008), by using the Multiple Expectation maximizations for Motif Elicitation (MEME) (Bailey et al., 2006). The Ler binding site was predicted in silico by searching the upstream sequences of the Z0395 gene by using the Find Individual Motif Occurrences (FIMO) (Grant et al., 2011).
Strains, Plasmids, and Culture Conditions
All the strains and plasmids used in this study are listed in Supplementary Table 1. Unless otherwise noted, the E. coli strains were grown aerobically in Luria-Bertani (LB) medium at 37°C. E. coli DH5α was used as a cloning host, and EHEC EDL933 was used as the wild-type (WT). The pCas and pTargetF plasmids required for mutant construction of E. coli were obtained from Addgene (plasmid #62225 and #62226) (Jiang et al., 2015).
Generation of a ler Deletion Mutant
The ler (Z5140) was inactivated by deletion (207 of 390 bp) of the coding region using the clustered regularly interspaced short palindromic repeats (CRISPR)-Cas9 system as previously described (Jiang et al., 2015). Briefly, two amplicons designed to carry homologous arms with 5′- and 3′-flanking regions of ler were amplified by PCR using LER-F1-F and -R or LER-F2-F and -R pairs of primers (Supplementary Table 2). Both amplicons were fused into donor DNA by overlap extension PCR using the primer pairs of LER-F1-F and LER-F2-R. Replacing the N20 of pTargetF to target ler was performed using the Site-Directed Mutagenesis Kit (NEB, Beverly, MA, United States) according to the manufacturer’s protocols. The N20 replaced pTargetF targeting ler was designated as pTargetF-ler (Supplementary Table 1). The EDL933 electrocompetent cells harboring pCas were prepared as previously described (Sharan et al., 2009). For genome editing, 400 ng of donor DNA and 100 ng of pTargetF-ler were co-electroporated into the EDL933 electrocompetent cells. The construction of the ler deletion mutant was confirmed by PCR.
Quantitative Reverse Transcription-PCR
The total RNA of the EDL933 strains grown under various conditions were isolated to determine the relative transcript levels of genes of interest by quantitative reverse transcription-PCR (qRT-PCR). In detail, to determine the relative transcript levels of the Z0395 gene, the EHEC strains were grown in low-glucose Dulbecco’s modified Eagle’s medium (Merck, Darmstadt, Germany) at 37°C to an A600 of 1.0. To determine the relative transcript levels of thiB, thiC, and stx2a, the EHEC strains were grown in M9 minimal medium with or without thiamine at 37°C to an A600 of 0.75. Finally, to determine the relative transcript levels of cusC and stx2a, the EHEC strains were grown in LB medium with different levels of CuSO4 at 37°C to an A600 of 1.0. The total RNAs of the strains were isolated using the RNeasy mini kit (Qiagen, Valencia, CA, United States). For qRT-PCR, the concentrations of the total RNAs were measured by using a NanoDrop One spectrophotometer (Thermo Scientific, Waltham, MA, United States), and cDNA was synthesized from 100 ng of total RNA by using iScript cDNA synthesis kit (Bio-Rad, Hercules, CA, United States). Real-time PCR amplification of the cDNA was performed by using CFX96 real-time PCR detection system (Bio-Rad) with specific primer pairs (Supplementary Table 2) as described previously (Jang et al., 2017). The relative transcript levels of the genes were calculated by using the transcript levels of the glyceraldehyde-3-phosphate dehydrogenase (GAPDH) as the internal reference for normalization (Kijewski et al., 2020).
Results
The Modulons Containing the Target Genes of Several Transcriptional Regulatory Networks of Enterohemorrhagic Escherichia coli Are Identified by Using Independent Component Analysis
Independent component analysis, a machine learning-based decomposition method, was used to decompose the large-scale transcriptome data of EHEC into the modulons containing the target genes of several TRNs. For this purpose, the trimmed 88 transcriptome data of EHEC (R2 ≥ 0.9 between biological replicates) (Supplementary Figure 1A and Supplementary Data Sheet 1, 2) were decomposed into the 85 independent gene components (Supplementary Data Sheet 3). ICA was also used to calculate the overall expression levels of the decomposed 85 components: the activities of the components in a specific condition. The activities of the 85 independent components (Supplementary Data Sheet 4) successfully explained 83% of the total expression variance of the 88 transcriptome data (Supplementary Figure 1B), validating that ICA properly decomposed the transcriptome data of EHEC into the independent gene components.
The 85 independent components contain the element genes with varied gene coefficients that represent the degree of the regulatory effect on the expressions of the genes. The element genes with a positive or negative gene coefficient indicate that their expressions are proportionally or inversely regulated along with the activities of the independent component, respectively. Unless otherwise noted, gene coefficient signs of element genes in an independent component are positive. Most of the gene coefficients of element genes in an independent component were distributed close to 0 (Supplementary Figure 1C), indicating that the expressions of only a few element genes significantly rely on the activities of an independent component. The distribution of the gene coefficients was reexamined by the statistical analysis, D’Agostino K2 test (D’Agostino et al., 1990; Sastry et al., 2019), to select only the genes with the coefficients far away from 0. As a result, the element genes with the gene coefficients over a cut-off, D’Agostino K2 test statistic 1,800 (Supplementary Figure 1D), were selected as the co-regulated genes of an independent component and defined as the modulons (see section “Materials and Methods” for details on the selection process). Consequently, a total of 64 modulons were identified from the 85 independent components. The 64 modulons with detailed information are presented in Supplementary Data Sheet 3.
The Locus of Enterocyte Effacement and the Shiga Toxin Modulons Contain the Ler Regulon and the Shiga Toxin Prophage Genes, Respectively
The modulons mainly consisting of the LEE and the Stx prophage genes encoding the major virulence factors of EHEC were defined as the LEE and the Stx modulon, respectively, and were further investigated to confirm whether the modulons adequately contain the sets of co-regulated genes. The LEE modulon contains 44 genes, of which 39 were the LEE genes (Figure 1A). Also, the activities of the LEE modulon were strongly correlated with the expression levels of ler (Pearson R = 0.79, P < 10–10) (Figure 1B), indicating that the LEE modulon primarily consisted of the Ler regulon. Furthermore, the LEE modulon contained lpxR, nleA, stcE, and etpC, which were not located in the LEE but known as the Ler regulon (Figure 1A; Grys et al., 2005; Tobe et al., 2006; Roe et al., 2007; Ogawa et al., 2018). The activities of the LEE modulon were also highly correlated with the expression levels of these genes (Pearson R > 0.5, P < 10–5) (Figure 1C), indicating that the modulon properly contained the genes located separately but co-regulated by Ler. Similarly, the Stx modulon contained the CP-933V and BP-933W prophage genes that include stx1 and stx2, respectively (Figure 1D). The activities of the Stx modulon were also highly correlated with the expression levels of the antiterminator Qs (Pearson R > 0.5, P < 10–5) (Figures 1E,F), indicating that the Stx modulons mainly consisted of the Stx prophage genes co-related by the antiterminator Qs. Consequently, these results validated that the modulons, the independent sets of co-regulated genes, were appropriately identified from the large-scale transcriptome data by using ICA.
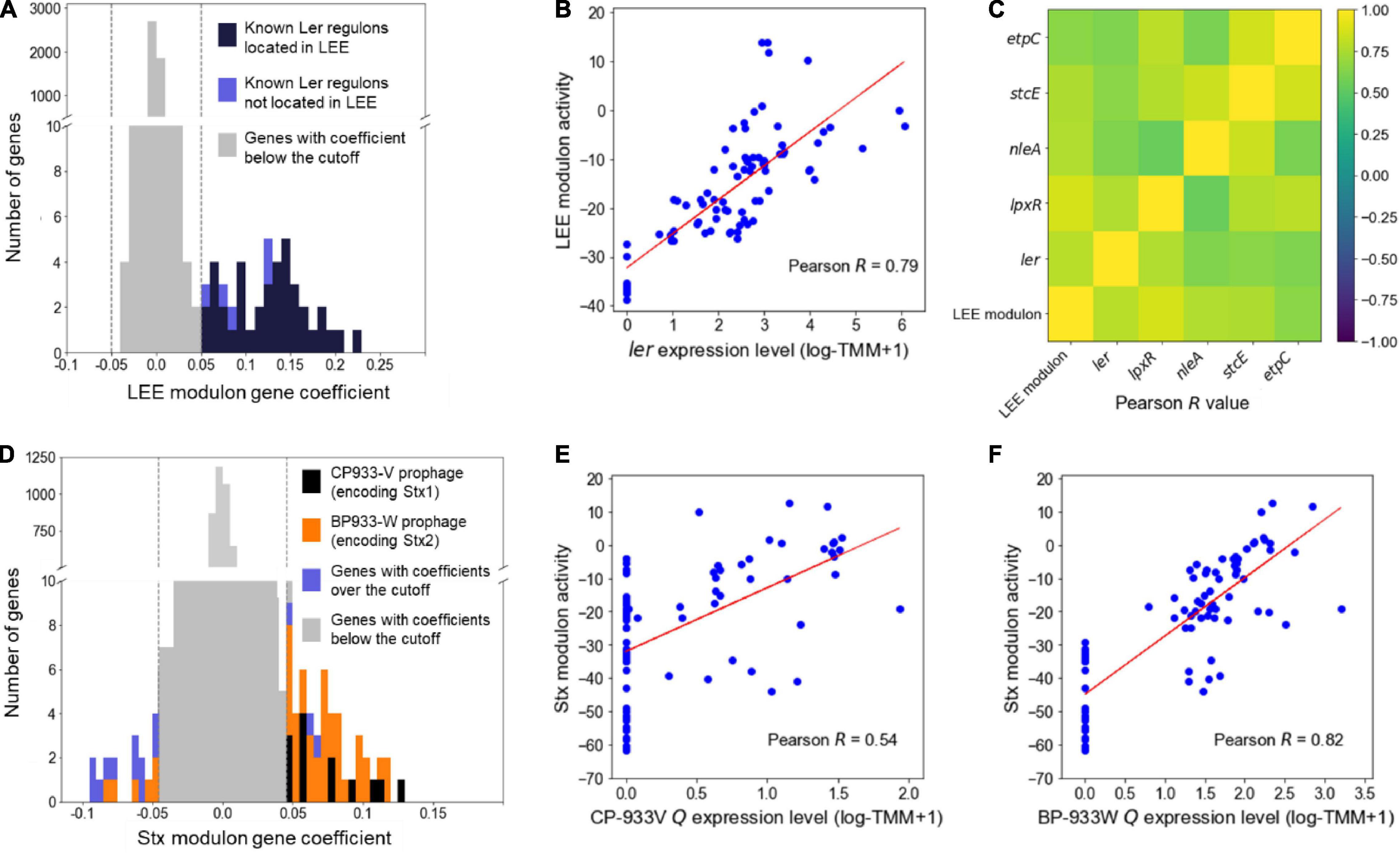
Figure 1. Validation of the LEE and Stx modulons. Histograms of the gene coefficients of the element genes in the LEE (A) and the Stx modulons (D). The dotted lines in the boxes show the cut-off values of the gene coefficients in each modulon. The colors of the bars represent the classifications of the genes as indicated in the plots. The scatter plots of the activities of the LEE modulon and the expression levels of the ler (B), the activities of the Stx modulon and the expression levels of the CP-933V antiterminator Q (E), and the activities of the Stx modulon and the expression levels of the BP-933W antiterminator Q (F). The Pearson R values between the activities of the modulons and the expression levels of their related TF are denoted in the boxes. Each dot of the plots represents a single biological replicate. Red lines represent the regression lines of the plots. (C) Ordered correlation matrix. Colors indicate the Pearson R values between the activities of the LEE modulon and the expression levels of the Ler regulon that are not located in the LEE. Yellow and indigo represent the strongest positive (+1) and negative (–1) correlation, respectively.
The Locus of Enterocyte Effacement Modulon Contains the Z0395 Gene as a Novel Member of the Ler Regulon
The element genes of the LEE modulon were further investigated to analyze the target genes of the Ler TRN encoding the major virulence factor of EHEC. The LEE modulon included a hypothetical gene, the Z0395 gene, which is not located in the LEE (Z5099-5141) and is not known as the Ler regulon. Since most of the genes in the LEE modulon were the Ler regulon (Figure 1A), it was possible that the Z0395 gene is also a member of the Ler regulon. To examine the possibility, the relationship between the expressions of the Z0395 gene and ler was analyzed. As shown in Figure 2A, the expressions of the Z0395 gene and ler were positively correlated (Pearson R = 0.33, P < 0.05). Thus, to further verify the effect of Ler on the expression of the Z0395 gene, the transcript levels of the Z0395 gene in the WT and the ler deletion mutant (Δler) were compared. The transcript level of the Z0395 gene was greatly reduced in Δler (Figure 2B), confirming that Ler activates the Z0395 gene expression at the transcription level. To examine whether Ler directly binds to the probable promoter region of the Z0395 gene, the upstream region of the Z0395 gene was scanned in silico with the binding motif of Ler. The motif-based sequence analysis predicted one Ler binding sequence located in the −212 to −201 region from the open reading frame (ORF) of the Z0395 gene (P < 10–5) (Figures 2C,D). Taken together, these results indicated that Ler regulates the expression of the Z0395 gene by directly binding to its upstream region, supporting that the Z0395 gene in the LEE modulon is a novel member of the Ler regulon.
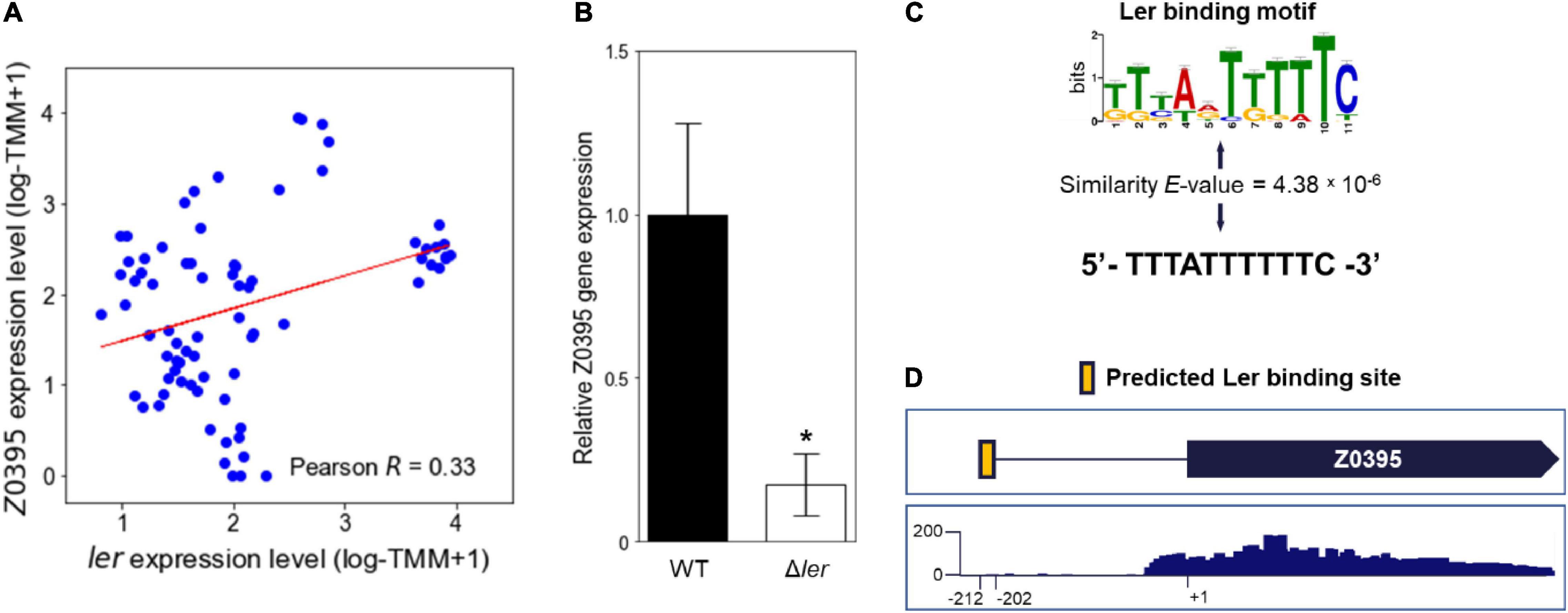
Figure 2. The Z0395 gene is a member of the Ler regulon. (A) The scatter plot of the expression levels of the Z0395 gene and ler. Each dot of the plot represents a single biological replicate. Red line represents the regression line of the plot. The Pearson R value between the expression levels of the Z0395 gene and ler is denoted in the box. (B) The relative expression levels of the Z0395 gene in the WT and ler deletion mutant. The levels of the Z0395 gene transcripts were determined by qRT-PCR, and the Z0395 gene transcript levels in the WT were set to 1. Error bars represent the SD from four independent experiments. Statistical significance was determined by the Student’s t-test (*P < 0.05). WT, EDL933; Δler, ler deletion mutant. (C) The Ler binding motif depicted in the logo and the Ler binding sequence predicted in silico found at the Z0395 gene upstream region. The height of the letters in the logo represents the information contents of the position in bits. The similarity between the Ler binding motif (top) and the predicted binding sequence (bottom) are denoted as E-value. (D) Location of the Ler binding sequence in silico predicted in the Z0395 gene upstream region. The Ler binding sequence is located from –212 to –202 region of the Z0395 ORF, represented as a yellow box. The bellow box represents the coverage plot of the reads mapped to the Z0395 gene. The transcriptome data of EHEC EDL933 grown in M9 minimal medium were used to generate the plot. The y-axis represents the normalized number of reads per base. The average number of reads of the biological triplicates are shown in the plot.
The Shiga Toxin Modulon Contains the thi and cus Locus Genes in Addition to the Shiga Toxin Prophages
The element genes composing the Stx modulon were also further investigated. The Stx modulon contained the thi locus genes thiBP and thiCEFGH and the cus locus genes cusCFBA, which are not located in the Stx prophages (Figure 3A). These genes have negative gene coefficients in the Stx modulon, unlike the Stx prophage genes with positive gene coefficients (Figure 3A), indicating that the expressions of the thi and cus locus genes decrease as the activities of the Stx modulon increase. In accordance with this, the expression levels of thiB, thiC, and cusC were negatively correlated with the activities of the Stx modulon, with Pearson R −0.57 (P < 10–5), −0.72 (P < 10–10), and −0.61 (P < 10–5), respectively (Figures 3B–D). The negative relationship was further verified by the correlation analyses between the expression levels of thiB, thiC, and cusC, and those of stx2a (Pearson R < −0.5, P < 10–8) (Figure 3E), indicating that the expression patterns of the thi and cus locus genes were contrary to those of the Stx prophage genes. Since the expressions of the thi and cus locus genes are regulated by the levels of thiamine and copper ions, respectively (Vander Horn et al., 1993; Webb et al., 1998; Miranda-Rios et al., 2001; Delmar et al., 2015), the effect of the nutrients on the expression of stx2a was examined. Interestingly, the presence of thiamine significantly decreased the transcription of thiB and thiC, but increased that of stx2a (Figure 3F). Copper ions also increased the transcription of cusC, but decreased that of stx2a in a dose-dependent manner (Figure 3G). Consequently, the combined results revealed that the Stx modulon includes the thi and cus locus genes in addition to the Stx prophage genes, which are regulated by the levels of thiamine and copper ions.
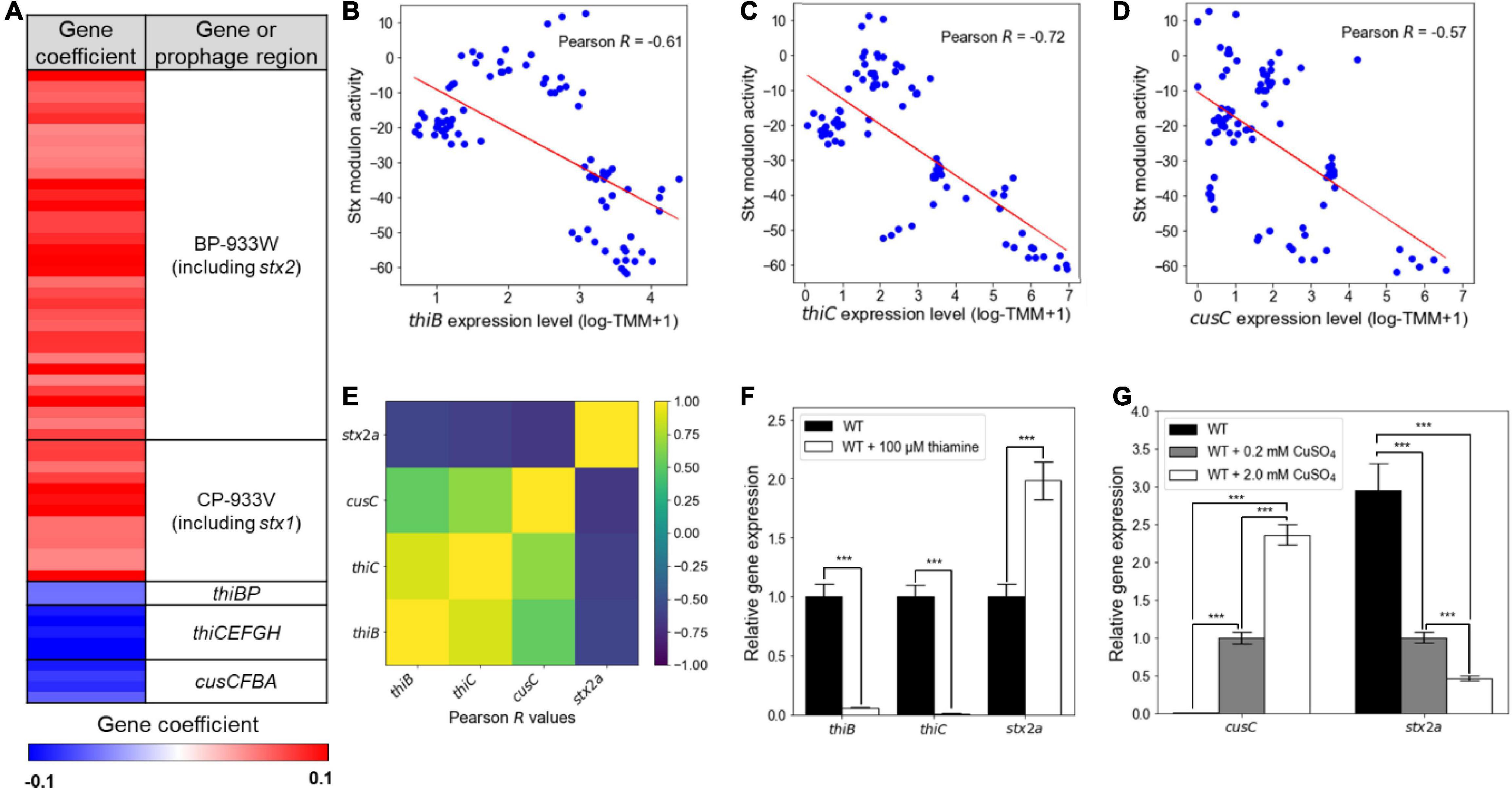
Figure 3. The contrary expression patterns of the thi and cus locus genes to those of the Stx prophage genes. (A) Heatmap for the gene coefficients in the Stx modulon. Red and blue represent the high (+0.1) and low (–0.1) gene coefficient, respectively. The scatter plots of the activities of the Stx modulon and the expression levels of thiB (B), thiC (C), and cusC (D). Each dot of the plots represents a single biological replicate. Red lines represent the regression lines of the plots. The Pearson R values between the activities of the Stx modulon and the expression levels of each gene of the plot are denoted in the boxes. (E) Ordered correlation matrix. Colors indicate the Pearson R values between the expression levels of thiB, thiC, cusC, and stx2a, as indicated. Yellow and indigo represent the strongest positive (+1) and negative (–1) correlation, respectively. (F,G) The relative expression levels of genes of interest in the WT grown under the different levels of thiamine and copper ions. The transcript levels of thiB, thiC, and stx2a in the WT with or without thiamine were determined by qRT-PCR, and the transcript levels of each gene in the WT were set to 1 (F). The transcript levels of cusC and stx2a in the WT with the different levels of CuSO4 were also determined by qRT-PCR, and the transcript levels of each gene in the WT with 0.2 mM CuSO4 were set to 1 (G). Error bars represent the SD from four independent experiments. Statistical significance was determined by the Student’s t-test (***P < 10–3). WT, EDL933.
The Modulons Enhance the Clustering of the Genes Co-regulated Regardless of the Growth Conditions
The element genes of the modulons are expected to be co-regulated under the various growth conditions. To verify this, it was investigated whether the expressions of the element genes in a modulon are altered together. The activities of the modulons were obtained from the transcriptome data of EHEC under different experimental conditions (Supplementary Figure 2). Among them, the significantly changed activities of the LEE modulon were observed from the transcriptome data of the WT and tna deletion mutant (Δtna) in the presence or absence of 500 μM indole. In Δtna imitating EHEC grown without indole, the activities of the LEE modulon increased significantly (P < 10–5) (Figure 4A). Accordingly, the expressions of the LEE genes, such as escE, escJ, cesL, sepL, and tir, increased significantly (Figure 4B). The addition of 500 μM indole significantly decreased the activities of the LEE modulon (P < 10–5) (Figure 4A) and thereby decreased the expressions of the LEE genes (Figure 4B). Interestingly, the changed activities of the LEE modulon altered the expressions of the non-LEE located hypothetical gene Z0395, the novel element gene of the LEE modulon (Figure 2A), in addition to the LEE genes (Figure 4B). These results indicated that the LEE modulon, as an example of the EHEC modulons, indeed enhanced the clustering of the genes co-regulated regardless of the growth conditions.
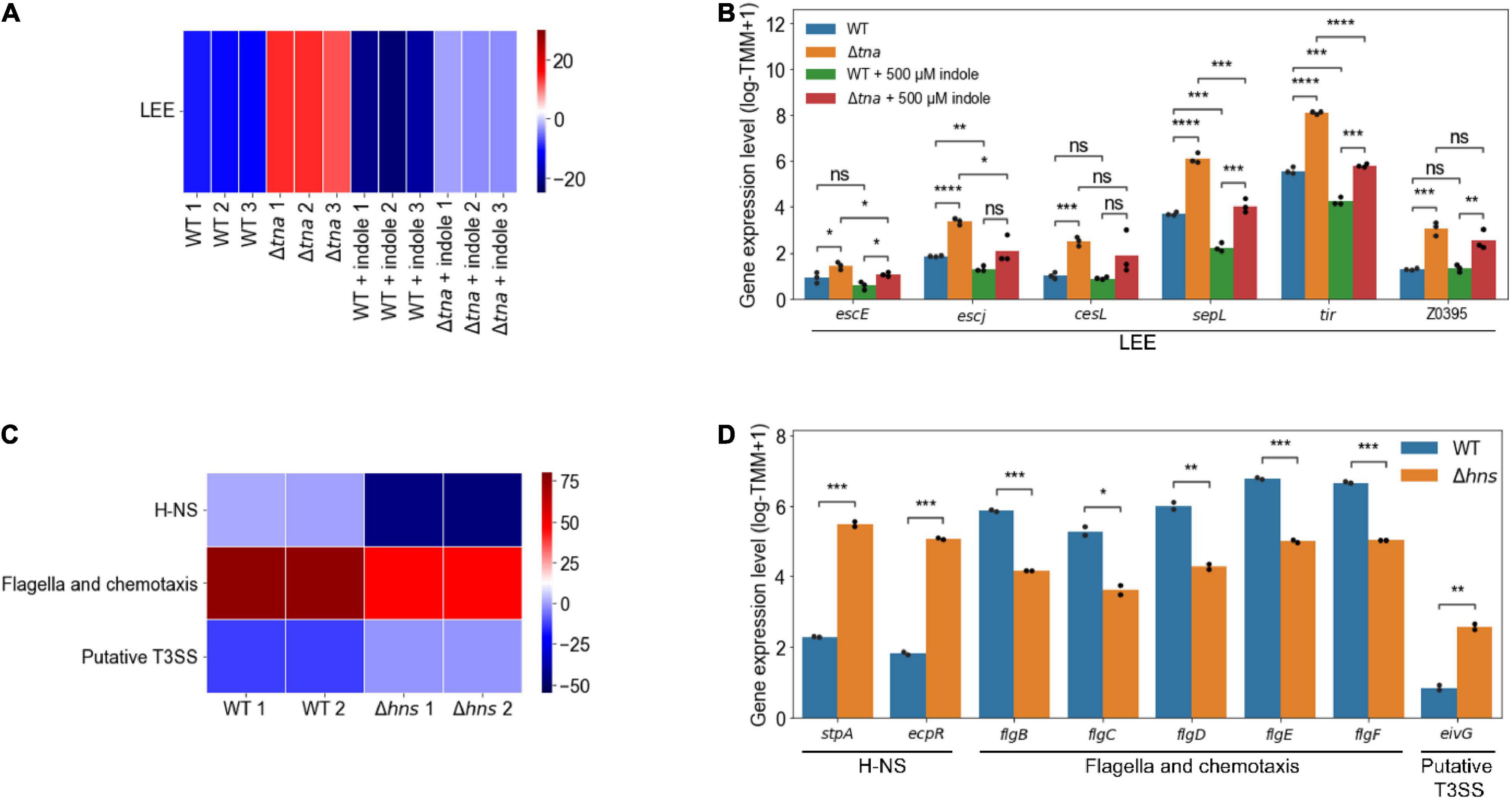
Figure 4. The changed activities of the modulons obtained from the transcriptome data of EHEC EDL933 and its isogenic mutants. Heatmap for the changed activities of the modulons obtained from the transcriptome data of the WT and Δtna in the presence or absence of 500 μM indole (A), and the WT and Δhns (C). The numbers on the bottom labels indicate a distinct single biological replicate. Red and blue represent the high and low activity of the modulon, respectively. WT, EDL933; Δtna, tna deletion mutant; Δhns, hns deletion mutant. The bar plots for the expression levels of the element genes of the modulons obtained from the transcriptome data of the WT and Δtna in the presence or absence of 500 μM indole (B), and the WT and Δhns (D). The modulon names of the element genes are denoted below the plots. The distinct colors of the bars represent the strains and the experimental conditions as indicated in the plots. Each dot on the bars represents a single biological replicate. Statistical significance was determined by the Student’s t-test (ns, not significant; *P < 0.05; **P < 10–2; ***P < 10–3; ****P < 10–4).
The Modulons Improve the Clustering of the Genes Co-regulated Regardless of the Genetic Backgrounds
Significantly changed activities of H-NS (P < 10–2), flagella and chemotaxis (P < 10–2), and putative type III secretion system (T3SS) modulons (P < 10–2) were also observed from the transcriptome data of the WT and hns deletion mutant (Δhns) (Figure 4C). The deletion of hns significantly decreased the activities of the H-NS modulon (P < 10–2) (Figure 4C). Since stpA and ecpR, the element genes of the H-NS modulon, have negative gene coefficients (Supplementary Data Sheet 3), the expressions of the genes increased significantly along with the decreased activities of the modulon in Δhns (Figure 4D). The deletion of hns significantly decreased the activities of the flagella and chemotaxis modulon (P < 10–2) (Figure 4C) and thereby decreased the expressions of the flagella component genes flgBCDEF (Figure 4D). The deletion of hns also significantly increased the activities of the putative T3SS modulon (P < 10–2) (Figure 4C) and thereby increased the expressions of eivG, the putative T3SS component gene (Figure 4D). The stpA and ecpR, flagella component genes, and putative T3SS component genes, known as the H-NS regulon (Lang et al., 2007; Martínez-Santos et al., 2012; Ueda et al., 2013; Wan et al., 2016), were separately classified into the H-NS, flagella and chemotaxis, and putative T3SS modulons, respectively. These results indicated that the modulons successfully clustered the inherently co-regulated genes of EHEC regardless of the genetic backgrounds.
The Modulons Enhance Understanding of the Differential Expressions of the Enterohemorrhagic Escherichia coli Virulence and Survival Genes
The changed activities of the modulons were analyzed from the transcriptome data previously obtained from EHEC in the different sites of the bovine GITs in order to confirm the differential gene expressions of the pathogen in the course of infection. For example, the activities of the RpoS, flagella and chemotaxis, Stx, and LEE modulons significantly changed in the different sites of the bovine GITs (Figure 5A). The activities of the RpoS modulon were significantly higher in the rumen than those in other sites of the bovine GITs (P < 10–2) (Figure 5A). Accordingly, the expressions of the element genes of the RpoS modulon, such as gadABC (Ling et al., 2008), katE (Schellhorn, 1995), hdeA (Dudin et al., 2013), and slp (Kabir et al., 2004), significantly increased in the rumen (Figure 5B). The activities of the flagella and chemotaxis modulon, and thereby the expressions of flgBCDEF, were significantly higher in the small intestine and rectum than in the rumen (P < 0.05) (Figures 5A,B). The activities of the Stx (P < 10–3) and LEE (P < 0.05) modulons, and thereby the expressions of the stx2a, escE, escJ, cesL, sepL, and tir, were significantly higher in the rectum than in other sites of the bovine GITs (Figures 5A,B). Consequently, these results indicated that the activities of the modulons could successfully explain the changed expressions of the virulence and survival genes in the different sites of the bovine GITs, enhancing understanding of the spatially differentiated gene expressions of EHEC during the course of infection.
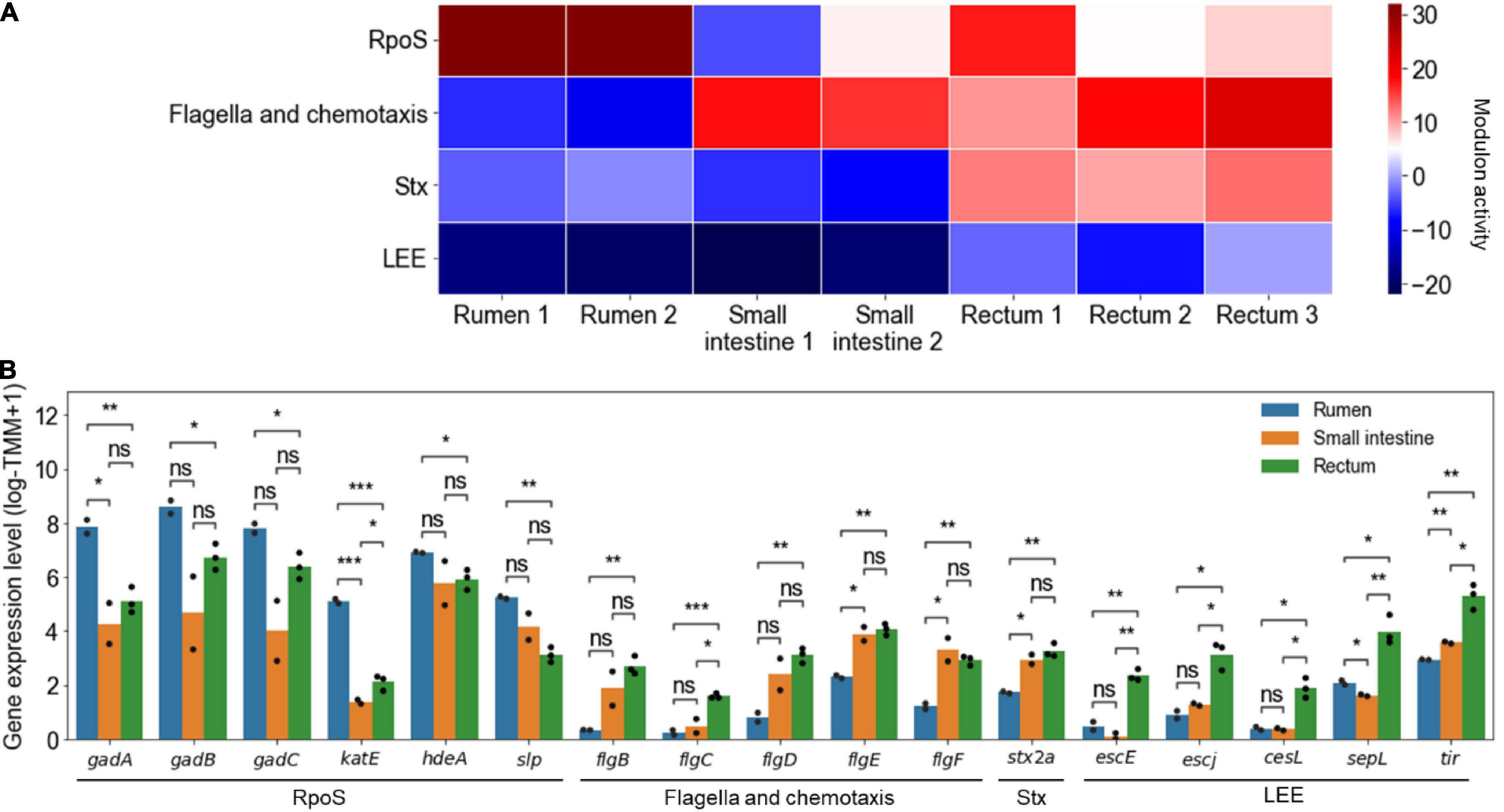
Figure 5. The changed activities of the modulons obtained from the transcriptome data of EHEC EDL933 in the different sites of the bovine GITs. (A) Heatmap for the changed activities of the modulons in the different sites of the bovine GITs. The numbers on the bottom labels indicate a distinct single biological replicate. Red and blue represent the high and low activity of the modulon, respectively. (B) The bar plots for the expression levels of the element genes of the modulons in the different sites of the bovine GITs. The modulon names of the element genes are denoted below the plot. The distinct colors of the bars represent the sites where EHEC was cultured as indicated in the plot. Each dot on the bars represents a single biological replicate. Statistical significance was determined by the Student’s t-test (ns, not significant; *P < 0.05; **P < 10–2; ***P < 10–3).
Discussion
In this study, ICA, a machine learning method that decomposes a mixture of components into independent components, was performed to decompose the large-scale transcriptome data of EHEC into the independent sets of co-regulated genes, the modulons. As a result, the trimmed 88 transcriptome data of EHEC (Supplementary Figure 1A) were decomposed into 64 independent modulons (Supplementary Figures 1B–D), which contain the target genes of the EHEC TRNs. The 64 modulons included the LEE and the Stx modulons mainly consisting of the LEE and the Stx prophage genes that encode the major virulence factors of EHEC, respectively (Figures 1A,D). The activities of the LEE modulon were strongly dependent on the expression level of ler (Figure 1B), and thus the LEE modulon mostly consisted of the Ler regulon. Moreover, the LEE modulon contained additional genes such as lpxR, nleA, stcE, and etpC, which are not located in the LEE but regulated by Ler (Figures 1A,C; Grys et al., 2005; Roe et al., 2007; Ogawa et al., 2018), indicating that ICA can precisely identify the LEE modulon to contain the target genes of the Ler TRN even not located in LEE. The Stx modulon contained the genes of the Stx prophages: CP-933V and BP-933W (Figure 1D). The activities of the Stx modulon were dependent on the expression levels of the antiterminator Qs (Figures 1E,F), indicating that the Stx modulon were adequately grouped with target genes of the Stx prophage TRNs. These results suggested that ICA successfully decomposed the large-scale transcriptome data of EHEC into the modulons.
The LEE modulon included a hypothetical Z0395 gene, which is not located within the LEE (Z5099-5141) and is not known as the Ler regulon. Interestingly, the expression of the Z0395 gene was predicted to increase along with the increased expression of ler (Figure 2A), suggesting that the Z0395 gene is a probable member of the Ler regulon. Experimentally, the deletion of ler significantly decreased the expression of the Z0395 gene (Figure 2B), confirming that the Z0395 gene in the LEE modulon is a new member of the Ler regulon. Furthermore, direct binding of Ler near the Z0395 gene was proposed by a previous ChIP-on-chip assay (Abe et al., 2008), and the Ler binding motif predicted in silico was found at the upstream region of the Z0395 gene (Figures 2C,D; Bailey et al., 2006; Grant et al., 2011). These results indicated that the Z0395 gene is a novel member of the Ler regulon, suggesting that the investigation of the modulons can discover new target genes of the current TRNs of EHEC.
The Stx modulon contained the non-prophage genes, the thi and cus locus genes, in addition to the Stx prophage genes (Figure 3A). The expression patterns of the thi and cus locus genes and those of other element genes in the Stx modulon were contrary (Figures 3B–D), and in detail, the expression levels of the thiB, thiC, and cusC genes have negative correlations with those of stx2a (Figure 3E). Interestingly, the levels of thiamine and copper ions known to control the expressions of the thi and cus locus genes, respectively (Vander Horn et al., 1993; Webb et al., 1998; Miranda-Rios et al., 2001; Delmar et al., 2015), inversely regulated the stx2a prophage gene (Figures 3F,G). Considering that thiamine is mostly produced by the gut microbiota (Said et al., 2001; Bhat and Kapila, 2017; Pan et al., 2017), the presence of thiamine could be an environmental signal for EHEC to suppress the thi locus genes and to induce the Stx virulence factors in the intestinal environments. Meanwhile, copper ions, mostly consumed with foods, are absorbed by the enterocytes in the upper small intestine and then left only in trace amounts in the large intestine (Doguer et al., 2018). Therefore, the relatively low copper ions also could be a signal for EHEC to suppress the cus locus genes and to induce the Stx virulence factors in the large intestine, the major colonization site for the pathogen (Vallance et al., 2002). Consequently, the investigation of the element genes of the Stx modulon could propose novel environmental signals such as the levels of thiamine and copper ions to control expressions of the Stx prophage genes, providing further understanding of the regulation of the TRNs of EHEC virulence factors.
The TRNs of bacteria primarily consist of the genes whose expressions are regulated together by a specific growth condition or the presence of a specific TF(s) (Sastry et al., 2019; DuPai et al., 2020). In contrast, the modulons of bacteria consist of the genes that are identified computationally and are expressed differentially together regardless of their growth conditions and the genetic backgrounds (Saelens et al., 2018; Sastry et al., 2019; Tan et al., 2020). Accordingly, novel gene Z0395, another element gene of the LEE modulon (Figure 2A), is expressed together with the LEE genes in the presence or absence of indole (Figures 4A,B). Additionally, the genes regulated by an identical TF can be classified into different modulons. For example, the flagella component genes and the putative T3SS component genes of the H-NS regulon were separately classified into the flagella and chemotaxis modulon, and putative T3SS modulon, respectively (Figures 4C,D). Altogether, these results indicated that the individual modulon successfully clustered a set of genes that are inherently co-regulated under the various conditions regardless of the genetic backgrounds of EHEC.
The changed activities of the modulons can be obtained from the transcriptome data of EHEC previously observed from the different sites of the bovine GITs. The activities of the RpoS modulon including the acid resistance genes, gadABC, increased significantly in the rumen, the acidic environment (Figures 5A,B; Ogawa et al., 2001; Ling et al., 2008; Chaucheyras-Durand et al., 2010). The activities of the flagella and chemotaxis modulon increased significantly in the small intestine and rectum (Figures 5A,B), which enables EHEC to move to more favorable niches (Naylor et al., 2003; Xu et al., 2012). The activities of the LEE and the Stx modulons increased significantly in the rectum (Figures 5A,B). The LEE genes encode the crucial adherence factors for colonizing the rectum, the primary colonization site of EHEC (Naylor et al., 2003). The Stxs also provide advantages for persistent colonization of EHEC by retarding the adaptive immune system at the bovine intestinal mucosa (Menge, 2020). Altogether, these results indicated that the changed activities of the modulons obtained from the transcriptome data could successfully explain the pathogenesis of EHEC during the course of infection in bovines.
Conclusion
In summary, ICA of the large-scale transcriptome data identified the modulons consisting of the target genes of the EHEC TRNs. Further analysis of the modulons revealed that the Z0395 gene and the thi and cus locus genes are novel element genes of the LEE and Stx modulons, respectively. Concurrently, the Stx prophage genes were also regulated by thiamine and copper ions controlling the thi and cus locus genes, respectively. Changed activities of the modulons consisting of the inherently co-regulated genes enhanced understanding of the differential expressions of the EHEC virulence and survival genes in response to specific intestinal environments. Consequently, ICA can expand and enhance the current understating of the TRNs of EHEC, suggesting that ICA can provide broader insight into the TRNs of other pathogens from their transcriptome data.
Data Availability Statement
The original contributions presented in this study are included in the article/Supplementary Material, further inquiries can be directed to the corresponding authors.
Author Contributions
HI, J-HL, and SC: conceptualization, writing—original draft and review and editing. HI: methodology, validation, formal analysis, investigation, data curation, resources, and visualization. J-HL and SC: supervision, project administration, and funding acquisition. All authors contributed to the article and approved the submitted version.
Funding
This work was supported by the National Research Foundation of Korea (NRF) and funded by the Ministry of Science, Information and Communications Technology, and Future Planning (2017R1E1A1A01074639 and 2021K1A3A1A20001134). This work was also supported by Cooperative Research Program for Agriculture Science and Technology Development (Project No. PJ016298), Rural Development Administration, Republic of Korea.
Conflict of Interest
The authors declare that the research was conducted in the absence of any commercial or financial relationships that could be construed as a potential conflict of interest.
Publisher’s Note
All claims expressed in this article are solely those of the authors and do not necessarily represent those of their affiliated organizations, or those of the publisher, the editors and the reviewers. Any product that may be evaluated in this article, or claim that may be made by its manufacturer, is not guaranteed or endorsed by the publisher.
Supplementary Material
The Supplementary Material for this article can be found online at: https://www.frontiersin.org/articles/10.3389/fmicb.2022.953404/full#supplementary-material
Footnotes
References
Abe, H., Miyahara, A., Oshima, T., Tashiro, K., Ogura, Y., Kuhara, S., et al. (2008). Global regulation by horizontally transferred regulators establishes the pathogenicity of Escherichia coli. DNA Res. 15, 13–23. doi: 10.1093/dnares/dsm028
Anders, S., Pyl, P. T., and Huber, W. (2015). HTSeq-A Python framework to work with high-throughput sequencing data. Bioinformatics 31, 166–169. doi: 10.1093/bioinformatics/btu638
Atlung, T., and Ingmer, H. (1997). H−NS: a modulator of environmentally regulated gene expression. Mol. Microbiol. 24, 7–17. doi: 10.1046/j.1365-2958.1997.3151679.x
Bailey, T. L., Williams, N., Misleh, C., and Li, W. W. (2006). MEME: discovering and analyzing DNA and protein sequence motifs. Nucleic Acids Res. 34, W369–W373. doi: 10.1093/nar/gkl198
Bhat, M. I., and Kapila, R. (2017). Dietary metabolites derived from gut microbiota: critical modulators of epigenetic changes in mammals. Nutr. Rev. 75, 374–389. doi: 10.1093/nutrit/nux001
Casjens, S. R., and Hendrix, R. W. (2015). Bacteriophage lambda: early pioneer and still relevant. Virology 479–480, 310–330. doi: 10.1016/j.virol.2015.02.010
Chaucheyras-Durand, F., Faqir, F., Ameilbonne, A., Rozand, C., and Martin, C. (2010). Fates of Acid-Resistant and Non-Acid-Resistant Shiga Toxin-Producing Escherichia coli Strains in Ruminant Digestive Contents in the Absence and Presence of Probiotics. Appl. Environ. Microbiol. 76, 640–647. doi: 10.1128/AEM.02054-09
D’Agostino, R. B., Belanger, A., and D’Agostino, R. B. Jr. (1990). A Suggestion for Using Powerful and Informative Tests of Normality. Am. Stat. 44, 316–321. doi: 10.1080/00031305.1990.10475751
Delmar, J. A., Su, C. C., and Yu, E. W. (2015). Heavy metal transport by the CusCFBA efflux system. Protein Sci. 24, 1720–1736. doi: 10.1002/pro.2764
Delorme, A., and Makeig, S. (2004). EEGLAB: an open source toolbox for analysis of single-trial EEG dynamics including independent component analysis. J. Neurosci. Methods 134, 9–21. doi: 10.1016/j.jneumeth.2003.10.009
Dobin, A., Davis, C. A. A., Schlesinger, F., Drenkow, J., Zaleski, C., Jha, S., et al. (2013). STAR: ultrafast universal RNA-seq aligner. Bioinformatics 29, 15–21. doi: 10.1093/bioinformatics/bts635
Doguer, C., Ha, J., and Collins, J. F. (2018). Intersection of Iron and Copper Metabolism in the Mammalian Intestine and Liver. Compr. Physiol. 8, 1433–1461. doi: 10.1002/cphy.c170045
Dudin, O., Lacour, S., and Geiselmann, J. (2013). Expression dynamics of RpoS/Crl-dependent genes in Escherichia coli. Res. Microbiol. 164, 838–847. doi: 10.1016/j.resmic.2013.07.002
DuPai, C. D., Wilke, C. O., and Davies, B. W. (2020). A Comprehensive Coexpression Network Analysis in Vibrio cholerae. mSystems 5:e00550–20. doi: 10.1128/mSystems.00550-20
Elliott, S. J., Sperandio, V., Giron, J. A., Shin, S., Mellies, J. L., Wainwright, L., et al. (2000). The locus of enterocyte effacement (LEE)-encoded regulator controls expression of both LEE- and non-LEE-encoded virulence factors in enteropathogenic and enterohemorrhagic Escherichia coli. Infect. Immun. 68, 6115–6126. doi: 10.1128/IAI.68.11.6115-6126.2000
Ester, M., Kriegel, H., Sander, J., and Xu, X. (1996). “A density-based algorithm for discovering clusters in large spatial databases with noise,” in Proceedings of the KDD-96: 2nd International Conference on Knowledge Discovery and Data Mining (Portland, OR: AAAI Press), 226–231.
Fang, X., Sastry, A., Mih, N., Kim, D., Tan, J., Yurkovich, J. T., et al. (2017). Global transcriptional regulatory network for Escherichia coli robustly connects gene expression to transcription factor activities. Proc. Natl. Acad. Sci. U.S.A. 114, 10286–10291. doi: 10.1073/pnas.1702581114
Gama-Castro, S., Salgado, H., Peralta-Gil, M., Santos-Zavaleta, A., Muniz-Rascado, L., Solano-Lira, H., et al. (2011). RegulonDB version 7.0: transcriptional regulation of Escherichia coli K-12 integrated within genetic sensory response units (Gensor Units). Nucleic Acids Res. 39, D98–D105. doi: 10.1093/nar/gkq1110
Grant, C. E., Bailey, T. L., and Noble, W. S. (2011). FIMO: scanning for occurrences of a given motif. Bioinformatics 27, 1017–1018. doi: 10.1093/bioinformatics/btr064
Grys, T. E., Siegel, M. B., Lathem, W. W., and Welch, R. A. (2005). The StcE Protease Contributes to Intimate Adherence of Enterohemorrhagic Escherichia coli O157:H7 to Host Cells. Infect. Immun. 73, 1295–1303. doi: 10.1128/IAI.73.3.1295-1303.2005
Harris, C. R., Millman, K. J., van der Walt, S. J., Gommers, R., Virtanen, P., Cournapeau, D., et al. (2020). Array programming with NumPy. Nature 585, 357–362. doi: 10.1038/s41586-020-2649-2
Hunter, J. D. (2007). Matplotlib: a 2D Graphics Environment. Comput. Sci. Eng. 9, 90–95. doi: 10.1109/MCSE.2007.55
James, C. J., and Hesse, C. W. (2005). Independent component analysis for biomedical signals. Physiol. Meas. 26, R15–39. doi: 10.1088/0967-3334/26/1/R02
Jang, K. K., Lee, Z.-W., Kim, B., Jung, Y. H., Han, H. J., Kim, M. H., et al. (2017). Identification and characterization of Vibrio vulnificus plpA encoding a phospholipase A2 essential for pathogenesis. J. Biol. Chem. 292, 17129–17143. doi: 10.1074/jbc.M117.791657
Jiang, Y., Chen, B., Duan, C., Sun, B., Yang, J., and Yang, S. (2015). Multigene editing in the Escherichia coli genome via the CRISPR-Cas9 system. Appl. Environ. Microbiol. 81, 2506–2514. doi: 10.1128/AEM.04023-14
Kabir, M. S., Sagara, T., Oshima, T., Kawagoe, Y., Mori, H., Tsunedomi, R., et al. (2004). Effects of mutations in the rpoS gene on cell viability and global gene expression under nitrogen starvation in Escherichia coli. Microbiology 150, 2543–2553. doi: 10.1099/mic.0.27012-0
Karmali, M. A. (2017). Emerging Public Health Challenges of Shiga Toxin–Producing Escherichia coli Related to Changes in the Pathogen, the Population, and the Environment. Clin. Infect. Dis. 64, 371–376. doi: 10.1093/cid/ciw708
Kelly, M., Hart, E., Mundy, R., Marchès, O., Wiles, S., Badea, L., et al. (2006). Essential role of the type III secretion system effector NleB in colonization of mice by Citrobacter rodentium. Infect. Immun. 74, 2328–2337. doi: 10.1128/IAI.74.4.2328-2337.2006
Kenny, B., DeVinney, R., Stein, M., Reinscheid, D. J., Frey, E. A., and Finlay, B. B. (1997). Enteropathogenic E. coli (EPEC) transfers its receptor for intimate adherence into mammalian cells. Cell 91, 511–520. doi: 10.1016/S0092-8674(00)80437-7
Kijewski, A., Witsø, I. L., Iversen, H., Rønning, H. T., L’Abée-Lund, T., Wasteson, Y., et al. (2020). Vitamin K Analogs Influence the Growth and Virulence Potential of Enterohemorrhagic Escherichia coli. Appl. Environ. Microbiol. 86:e00583–20. doi: 10.1128/AEM.00583-20
Kumar, A., and Sperandio, V. (2019). Indole Signaling at the Host-Microbiota-Pathogen Interface. MBio 10:e01031–19. doi: 10.1128/mBio.01031-19
Lang, B., Blot, N., Bouffartigues, E., Buckle, M., Geertz, M., Gualerzi, C. O., et al. (2007). High-affinity DNA binding sites for H-NS provide a molecular basis for selective silencing within proteobacterial genomes. Nucleic Acids Res. 35, 6330–6337. doi: 10.1093/nar/gkm712
Li, M., Rosenshine, I., Yu, H. B., Nadler, C., Mills, E., Hew, C. L., et al. (2006). Identification and characterization of NleI, a new non-LEE-encoded effector of enteropathogenic Escherichia coli (EPEC). Microbes Infect. 8, 2890–2898. doi: 10.1016/j.micinf.2006.09.006
Ling, J., Sharma, M., and Bhagwat, A. A. (2008). Role of RNA polymerase sigma-factor (RpoS) in induction of glutamate-dependent acid-resistance of Escherichia albertii under anaerobic conditions. FEMS Microbiol. Lett. 283, 75–82. doi: 10.1111/j.1574-6968.2008.01153.x
Martínez-Santos, V. I., Medrano-López, A., Saldaña, Z., Girón, J. A., and Puente, J. L. (2012). Transcriptional Regulation of the ecp Operon by EcpR, IHF, and H-NS in Attaching and Effacing Escherichia coli. J. Bacteriol. 194, 5020–5033. doi: 10.1128/JB.00915-12
McKinney, W. (2010). “Data Structures for Statistical Computing in Python,” in Proceedings of the 9th Python in Science Conference, eds, S. van der Walt and J. Millman (Austin), 56–61. doi: 10.25080/Majora-92bf1922-00a
Mellies, J. L., Elliott, S. J., Sperandio, V., Donnenberg, M. S., and Kaper, J. B. (1999). The Per regulon of enteropathogenic Escherichia coli: identification of a regulatory cascade and a novel transcriptional activator, the locus of enterocyte effacement (LEE)-encoded regulator (Ler). Mol. Microbiol. 33, 296–306. doi: 10.1046/j.1365-2958.1999.01473.x
Menge, C. (2020). The Role of Escherichia coli Shiga Toxins in STEC Colonization of Cattle. Toxins 12:607. doi: 10.3390/toxins12090607
Miranda-Rios, J., Navarro, M., and Soberon, M. (2001). A conserved RNA structure (thi box) is involved in regulation of thiamin biosynthetic gene expression in bacteria. Proc. Natl. Acad. Sci. U.S.A. 98, 9736–9741. doi: 10.1073/pnas.161168098
Naylor, S. W., Low, J. C., Besser, T. E., Mahajan, A., Gunn, G. J., Pearce, M. C., et al. (2003). Lymphoid Follicle-Dense Mucosa at the Terminal Rectum Is the Principal Site of Colonization of Enterohemorrhagic Escherichia coli O157:H7 in the Bovine Host. Infect. Immun. 71, 1505–1512. doi: 10.1128/IAI.71.3.1505-1512.2003
Ogawa, M., Shimizu, K., Nomoto, K., Tanaka, R., Hamabata, T., Yamasaki, S., et al. (2001). Inhibition of in vitro growth of Shiga toxin-producing Escherichia coli O157:H7 by probiotic Lactobacillus strains due to production of lactic acid. Int. J. Food Microbiol. 68, 135–140. doi: 10.1016/S0168-1605(01)00465-2
Ogawa, R., Yen, H., Kawasaki, K., and Tobe, T. (2018). Activation of lpxR gene through enterohaemorrhagic Escherichia coli virulence regulators mediates lipid A modification to attenuate innate immune response. Cell. Microbiol. 20:e12806. doi: 10.1111/cmi.12806
Pan, X., Xue, F., Nan, X., Tang, Z., Wang, K., Beckers, Y., et al. (2017). Illumina Sequencing Approach to Characterize Thiamine Metabolism Related Bacteria and the Impacts of Thiamine Supplementation on Ruminal Microbiota in Dairy Cows Fed High-Grain Diets. Front. Microbiol. 8:1818. doi: 10.3389/fmicb.2017.01818
Robinson, M. D., McCarthy, D. J., and Smyth, G. K. (2010). edgeR: a Bioconductor package for differential expression analysis of digital gene expression data. Bioinformatics 26, 139–140. doi: 10.1093/bioinformatics/btp616
Robinson, M. D., and Oshlack, A. (2010). A scaling normalization method for differential expression analysis of RNA-seq data. Genome Biol. 11:R25. doi: 10.1186/gb-2010-11-3-r25
Roe, A. J., Tysall, L., Dransfield, T., Wang, D., Fraser-Pitt, D., Mahajan, A., et al. (2007). Analysis of the expression, regulation and export of NleA-E in Escherichia coli O157:H7. Microbiology 153, 1350–1360. doi: 10.1099/mic.0.2006/003707-0
Saelens, W., Cannoodt, R., and Saeys, Y. (2018). A comprehensive evaluation of module detection methods for gene expression data. Nat. Commun. 9:1090. doi: 10.1038/s41467-018-03424-4
Said, H. M., Ortiz, A., Subramanian, V. S., Neufeld, E. J., Moyer, M. P., and Dudeja, P. K. (2001). Mechanism of thiamine uptake by human colonocytes: studies with cultured colonic epithelial cell line NCM460. Am. J. Physiol. Liver Physiol. 281, G144–G150. doi: 10.1152/ajpgi.2001.281.1.G144
Sastry, A. V., Gao, Y., Szubin, R., Hefner, Y., Xu, S., Kim, D., et al. (2019). The Escherichia coli transcriptome mostly consists of independently regulated modules. Nat. Commun. 10:5536. doi: 10.1038/s41467-019-13483-w
Schellhorn, H. E. (1995). Regulation of hydroperoxidase (catalase) expression in Escherichia coli. FEMS Microbiol. Lett. 131, 113–119. doi: 10.1111/j.1574-6968.1995.tb07764.x
Sharan, S. K., Thomason, L. C., Kuznetsov, S. G., and Court, D. L. (2009). Recombineering: a homologous recombination-based method of genetic engineering. Nat. Protoc. 4, 206–223. doi: 10.1038/nprot.2008.227
Sy, B. M., Lan, R., and Tree, J. J. (2020). Early termination of the Shiga toxin transcript generates a regulatory small RNA. Proc. Natl. Acad. Sci. U.S.A. 117, 25055–25065. doi: 10.1073/pnas.2006730117
Tan, J., Sastry, A. V., Fremming, K. S., Bjørn, S. P., Hoffmeyer, A., Seo, S., et al. (2020). Independent component analysis of E. coli’s transcriptome reveals the cellular processes that respond to heterologous gene expression. Metab. Eng. 61, 360–368. doi: 10.1016/j.ymben.2020.07.002
Tobe, T., Beatson, S. A., Taniguchi, H., Abe, H., Bailey, C. M., Fivian, A., et al. (2006). An extensive repetoire of type III secretion effectors in Escherichia coli O157 and the role of lambdoid phages in their dissemination. Proc. Natl. Acad. Sci. U.S.A. 103, 14941–14946. doi: 10.1073/pnas.0604891103
Ueda, T., Takahashi, H., Uyar, E., Ishikawa, S., Ogasawara, N., and Oshima, T. (2013). Functions of the Hha and YdgT Proteins in Transcriptional Silencing by the Nucleoid Proteins, H-NS and StpA, in Escherichia coli. DNA Res. 20, 263–271. doi: 10.1093/dnares/dst008
Vallance, B. A., Chan, C., Robertson, M. L., and Finlay, B. B. (2002). Enteropathogenic and Enterohemorrhagic Escherichia coli Infections: emerging Themes in Pathogenesis and Prevention. Can. J. Gastroenterol. 16, 771–778. doi: 10.1155/2002/410980
Vander Horn, P. B., Backstrom, A. D., Stewart, V., and Begley, T. P. (1993). Structural genes for thiamine biosynthetic enzymes (thiCEFGH) in Escherichia coli K-12. J. Bacteriol. 175, 982–992. doi: 10.1128/jb.175.4.982-992.1993
Varoquaux, G., Buitinck, L., Louppe, G., Grisel, O., Pedregosa, F., and Mueller, A. (2015). Scikit-learn: machine Learning Without Learning the Machinery. GetMobile Mob. Comput. Commun. 19, 29–33. doi: 10.1145/2786984.2786995
Virtanen, P., Gommers, R., Oliphant, T. E., Haberland, M., Reddy, T., Cournapeau, D., et al. (2020). SciPy 1.0: fundamental algorithms for scientific computing in Python. Nat. Methods 17, 261–272. doi: 10.1038/s41592-019-0686-2
Wan, B., Zhang, Q., Tao, J., Zhou, A., Yao, Y., and Ni, J. (2016). Global transcriptional regulation by H-NS and its biological influence on the virulence of enterohemorrhagic Escherichia coli. Gene 588, 115–123. doi: 10.1016/j.gene.2016.05.007
Webb, E., Claas, K., and Downs, D. (1998). thiBPQ Encodes an ABC Transporter Required for Transport of Thiamine and Thiamine Pyrophosphate in Salmonella Typhimurium. J. Biol. Chem. 273, 8946–8950. doi: 10.1074/jbc.273.15.8946
Westermann, A. J., Gorski, S. A., and Vogel, J. (2012). Dual RNA-seq of pathogen and host. Nat. Rev. Microbiol. 10, 618–630. doi: 10.1038/nrmicro2852
Keywords: EHEC, transcriptional regulatory network, machine learning, independent component analysis, transcriptome
Citation: Im H, Lee J-H and Choi SH (2022) Independent Component Analysis Identifies the Modulons Expanding the Transcriptional Regulatory Networks of Enterohemorrhagic Escherichia coli. Front. Microbiol. 13:953404. doi: 10.3389/fmicb.2022.953404
Received: 26 May 2022; Accepted: 06 June 2022;
Published: 24 June 2022.
Edited by:
Kwangcheol Casey Jeong, University of Florida, United StatesReviewed by:
Byeonghwa Jeon, University of Minnesota Twin Cities, United StatesQiyao Wang, East China University of Science and Technology, China
Copyright © 2022 Im, Lee and Choi. This is an open-access article distributed under the terms of the Creative Commons Attribution License (CC BY). The use, distribution or reproduction in other forums is permitted, provided the original author(s) and the copyright owner(s) are credited and that the original publication in this journal is cited, in accordance with accepted academic practice. No use, distribution or reproduction is permitted which does not comply with these terms.
*Correspondence: Ju-Hoon Lee, anVobGVlQHNudS5hYy5rcg==; Sang Ho Choi, Y2hvaXNoQHNudS5hYy5rcg==