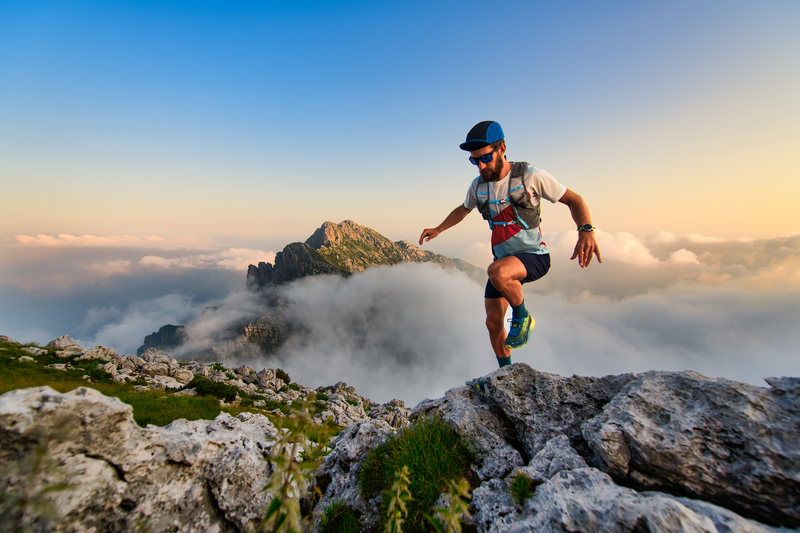
95% of researchers rate our articles as excellent or good
Learn more about the work of our research integrity team to safeguard the quality of each article we publish.
Find out more
ORIGINAL RESEARCH article
Front. Microbiol. , 03 October 2022
Sec. Antimicrobials, Resistance and Chemotherapy
Volume 13 - 2022 | https://doi.org/10.3389/fmicb.2022.939390
This article is part of the Research Topic Advances in the discovery of Natural Molecules and their Analogues Against Microbial Infection-Related Biofilms View all 14 articles
The development of biofilm on the biotic and abiotic surfaces is the greatest challenge for health care sectors. At present times, oral infection is a common concern among people with an unhealthy lifestyle and most of these biofilms-associated infections are resistant to antibiotics. This has increased a search for the development of alternate therapeutics for eradicating biofilm-associated infection. Nanobiotechnology being an effective way to combat such oral infections may encourage the use of herbal compounds, such as bio-reducing and capping agents. Green-synthesis of ZnO nanoparticles (ZnO NP) by the use of the floral extract of Clitoria ternatea, a traditionally used medicinal plant, showed stability for a longer period of time. The NPs as depicted by the TEM image with a size of 10 nm showed excitation spectra at 360 nm and were found to remain stable for a considerable period of time. It was observed that the NPs were effective in the eradication of the oral biofilm formed by the major tooth attacking bacterial strains namely Porphyromonsas gingivalis and Alcaligenes faecalis, by bringing a considerable reduction in the extracellular polymeric substances (EPS). It was observed that the viability of the Porphyromonsas gingivalis and Alcaligenes faecalis was reduced by NP treatment to 87.89 ± 0.25% in comparison to that of amoxicillin. The results went in agreement with the findings of modeling performed by the use of response surface methodology (RSM) and artificial neural network (ANN). The microscopic studies and FT-IR analysis revealed that there was a considerable reduction in the biofilm after NP treatment. The in silico studies further confirmed that the ZnO NPs showed considerable interactions with the biofilm-forming proteins. Hence, this study showed that ZnO NPs derived from Clitoria ternatea can be used as an effective alternative therapeutic for the treatment of biofilm associated oral infection.
The oral cavity provides an ideal environment for the growth of a large number of microbial communities that are responsible for the development of oral biofilm (Caputo et al., 2018; Morse et al., 2018; Quintieri et al., 2020). The development of oral biofilm takes place under the influence of the salivary glycoproteins, which act as adhering material for the sessile communities (Lahiri et al., 2021a). The sessile communities remain entangled with the help of a self-secreted polymeric substance (EPS) that acts as a natural glue. The EPS not only provides nourishment to the indwelling cells but also prevents the penetration of drug-like molecules resulting in the development of antimicrobial resistance (Khatoon et al., 2018). Thus, the use of alternative therapeutics has become an important way of combating biofilm-associated oral infections. Bio-nanotechnology is an upcoming and booming area in the field of Biotechnology and is coming up as an important part of clinical dentistry and dental practices (Thrall, 2004). Nano-particles are more effective than conventional antimicrobial agents and are used in mouth-wash, toothpaste, mouth-freshener, etc., and also in many oral clinics as a healthcare technology for reducing the risk factor of any kind of surgery (Thrall, 2004). Nano-particle technology or rather bio-nano-particle technology is especially used in the processes of dental filling, enamel polishing for prevention of caries, implantation technology as nano implant particles, which has more effectiveness than the regular type of nanoparticles, etc (Sahoo and Labhasetwar, 2003). A significant part of the nano-strategy lies in their role in the prevention of bacterial growth and bacterial biofilm formation. This nanoparticle technology is getting more and more popular among people as this technology is cost-effective, time-saving, and its application may avoid major surgery (Vasir and Labhasetwar, 2005). Among various types of metallic and non-metallic nanoparticles used, there are many added values of using the zinc oxide nanoparticles as the type selection of the nanoparticle. In comparison to the other types of nanoparticles like silver oxide nanoparticles or copper oxide nanoparticles that are organic or bulk oxide, it is seen that the zinc-oxide nanoparticles are robust, chemically more stable with marked thermal resistance, and have a long shelf life (Vasir et al., 2005). Also, many studies have confirmed that the selective toxicity of ZnO nanoparticles is almost negligible in comparison to the other kinds of nanoparticles, and also while comparing them with other nanoparticles, they show the minimal effect of toxicity on human cells (Maeda et al., 2000). Zinc oxide nanoparticles also show microbicidal properties against Gram-positive and Gram-negative bacteria and fungal spores that are usually resistant to a higher temperature and higher pressure (Allaker, 2011). The advancement of modern nano-strategy due to its effectiveness and preciseness can solve many dental disturbances. The efficiency of nanoparticles can be further increased once they are mixed with some bioactive compounds derived from natural phytoextracts having high medicinal properties and are used traditionally for cleaning and whitening teeth enamel (Allen and Cullis, 2004).
Nanobiotechnology based on a phytocompound-induced synthesis of nanoparticles is becoming a rising tendency in green chemistry due to its simple, non-toxic, and inexpensive nature (Bala et al., 2015). Amongst the preferred nanoparticles, the use of biogenically synthesized ZnO nanoparticles having diverse biomedical applications (Jan et al., 2020) is considered an environment-friendly and convenient technique.
In this study, the flowers of Clitoria ternatea, known for their medicinal values, were used as the biogenic source for ZnO nanoparticle production. Traditionally this plant is known mainly for its antistress, antidepressant, and anticonvulsant activities. The ZnO nanoparticles, biogenically formed from this plant extract, are evaluated for their antibiofilm activities against dental biofilm-forming bacterial strains and their superiority will be confirmed over conventional antibiotics. Such appraisal was confirmed by simulation-based studies with some statistical and computational model-based analysis. The in silico study helped in understanding the optimum condition for the production of nanoparticles and also helped in understanding the interaction of the ZnO NP with the targeted biofilm-forming proteins.
Porphyromonsas gingivalis and Alcaligenes faecalis were used in this study. The bacterial cells were cultured in Luria Bertani Broth (LB broth) at 37°C for 24 h.
The dried petals of the flower C. ternatea were pulverized in water and incubated for a period of 24 h. This was followed by filtering using the gauge filter and the filtrate was stored at 0–4°C for further use (Lahiri et al., 2021c).
Synthesis of ZnO NPs was performed by dissolving 5 mM zinc nitrate in 50 mL Mili Q water and was kept in a stirrer for a period of 1 h (Jamdagni et al., 2018). This was followed by the addition of 25 mL of sodium hydroxide. There was an observed change in the coloration of the solution after incubation for 1 h with plant extract at a volume of 25 mL. The solution was kept under the stirring condition at least for a period of 3 h. The precipitate was separated from the mixture by centrifugation at 8,000 g for 15 min. The pellets were dried using a hot air oven at 80°C for 2 h.
The Box–Behnken design (BBD) was considered to model the production of ZnO NP. The pH, volume of extract (mL), reaction time (min), temperature (°C), and concentration of zinc nitrate (mM) were the input parameters, while the absorption characteristics of the synthesized NP were optimized with Design-Expert Version 7.0.0 (Statease Inc; Minneapolis, USA) (Sarkar et al., 2021b).
The multilayer perception (MLP) neural network along with error backpropagation was considered in this study using MATLAB R2014b (Math Works Inc., USA) (Sarkar et al., 2020; Lahiri et al., 2021c). The neural network model was built of 5 (input layer)−3 (hidden layer)−1 (output layer) architecture (Figure 3), learning range varied from 0.5 to 0.9. For non-linear activation functions, the Levenberg–Marquardt ANN tool was used, along with the ANN backpropagation technique for feed-forward neural networks.
The environment of classroom learning and improvement of the student's performance has been simulated in the teaching–learning based optimization (TLBO). The input variables, fitness value, and the initial solution have been assumed as the subject, marks obtained by the students, and the size of the student batch, respectively. In this study, the temperature, pH, time, concentration of ZnNO3, and volume of the plant extract were considered as the subject. The values of those parameters were assumed by the student. The evaluation criteria to measure the performance of each student was maximization. In the teacher phase, the teaching factor was introduced to improve the performance of the students. Two random students with unequal performance were selected in the learner phase, in this phase higher performance was attained by learning. Learning from their own interactions as well from the teacher was considered as the iteration. The best-performing student was identified in each iteration. The process continued till the attainment of a maximum number of iterations selected or attainment of the constant best value for the last 50 generations (Morse et al., 2018; Stalin et al., 2019). Here, the absorbance value was assumed as the student's performance and maximization of the absorbance value. The best-performing student was selected from the batch of the absorbance value was considered as the TLBO objective. The TLBO parameters considered for the study are provided in Table 1.
The proposed ANN-TLBO methodology to find the value of the optimum input parameters for maximum absorbance is illustrated in Figure 1. The ANN model was developed with the dataset of the input parameter–response combined with the aim of maximizing the coefficient of correlation (R) value. The TLBO was employed considering the size of the student batch of 40, and the student's performance was boosted in the teacher and learner phase till the attainment of the stopping criteria.
Aliquots were taken for analyzing the UV–vis spectra at time intervals of 24 h. The UV–vis spectra were analyzed within the range of 200–1,000 nm. Transmission electron microscopy was performed for analyzing the dimension and size of the ZnO NPs (Tailor et al., 2019; Bharadwaj et al., 2021). An X-ray diffractometer is used for the purpose of analyzing crystalline phase and purity between 20° and 80°.
The antibiofilm effect of the aqueous extract, biogenically synthesized ZnO NPs from C. ternatea on Porphyromonsas gingivalis and Alcaligenes faecalis, was analyzed using the microdilution method (Balouiri et al., 2016). The bacterial cells were grown within the wells of a 96-well plate at a concentration of 1 × 106 CFU/ml followed by incubation at 37°C for a period of 72 h to allow the development of biofilm (Lahiri et al., 2021b,c). After the development of biofilm, it was followed by the addition of ZnO NPs, plant extract, and amoxicillin as standard antibiotics. This was followed by the addition of 3-[4,5-dimethyl-2-thiazolyl]-2, 5-diphenyl-2H-tetrazolium bromide (MTT dye) to quantify the viability of the bacterial cells in the presence of the challenge using the ELISA plate reader (2018 GEN-NET).
The density of the sessile population associated with the biofilm is dependent on the total amount of carbohydrates and proteins that are associated with the EPS (Limoli et al., 2015). The process of extracting the carbohydrates and proteins from the biofilm comprises cell incubation in accordance with the biofilm assay. After the incubation period, the biofilm was washed gently using the PBS and boiled for a period of 30 min within 0.5 N NaOH for the purpose of extracting the surface protein. This was followed by centrifugation at 10,000 g for a period of 5 min followed by the collection of clear solution. The protein concentration was determined using the Lowry method. The carbohydrate concentration was analyzed by taking the sample of EPS within the test tube followed by the addition of 900 μl of distilled water and 5 mL of 98% of sulphuric acid. The color intensity was analyzed spectrophotometrically at 490 nm.
The three-dimensional X-ray crystallographic structures of proteins responsible for biofilm formation, quorum sensing, and motility-related proteins in the EPS, with an approximate resolution of 1.5–2Å, were collected from the Protein Data Bank (https://www.rcsb.org/). The structure of receptor protein PDB ID: 5OLJ is associated with dental biofilm forming bacterial species Porphyromonas gingivalis. Another dental biofilm-forming protein was obtained from the isolated bacterial species Alcaligenes faecalis, using the phyre2 web server (http://www.sbg.bio.ic.ac.uk/phyre2/) in the.pdb format.
The working strain grown on 0.1% chitin flakes (w/v) for a period of 72 h was washed with 0.1% (w/v) normal saline to eliminate planktonic groups of cells. After treating the sessile cells with plant extracts and bioactive compounds, the growth was determined spectrophotometrically at 590 nm at varying intervals of time (Baishya et al., 2016).
The prediction of the ADME characteristics of the selected ligands was achieved through the SwissADME web server (http://www.swissadme.ch/). Swiss ADME is an open-source free web tool that computes the drug-likeness of compounds through their physicochemical and pharmacokinetic properties of small molecules (Daina et al., 2017). The input format consisted of the canonical SMILES of the selected compounds and the output from SwissADME containing the descriptors was obtained in.csv file format.
In this study, VESTA (Momma and Izumi, 2008) was used for drawing the three-dimensional structure of Zinc oxide nanoparticles (ZnO) in space-filling representation. Visualization for Electronic Structural Analysis or VESTA is a three-dimensional visualization programme for structural models, volumetric data, such as electron or nuclear densities, and crystal morphologies. The software is managed and controlled by the Windows workstations via WPKG. ZnO nanoparticle has the lattice parameters a = b = 3.24940 and c = 5.20380 with alpha = beta = 90 and gamma = 120. Atomic position of Zn in unit cell is [0.33333, 0.66667, 0.00000] and O in unit cell situated at [0.33333, 0.66667, 0.38210].
The macromolecule preparation for the pre-processing of proteins before docking was achieved by AutoDockTools (ADT), which is a part of MGLTools, from the Molecular Graphics Laboratory at The Scripps Research Institute (Morris et al., 2008). Using ADT, the bound ligands were manually visualized using the Python Molecule Viewer (PMV). Removal of water molecules and addition of hydrogen were also carried out using the same. Finally, Gasteiger charges were added to the cleaned-up protein. Energy minimization of the ligands was achieved using the Auto Optimize tool of the Avogadro molecule editor and visualizer (Hanwell et al., 2012). Auto optimization continuously optimizes molecular geometry through molecular mechanics (Hanwell et al., 2012). The UFF or Universal Force Field was used along with a default value of 4 for “Steps per Update” and the energy minimization was achieved with a dE value of 0. The optimized ligands were written in.pdb format and were subjected to automatic preparation by AutoDock Tools. ADT checked for and merged non-polar hydrogens with the heavier atoms to which they are attached and added Gasteiger charges.
To understand how the nanoparticles affected the interactions between the biofilm-associated proteins and the phytocompounds, a double-docking approach was followed, wherein, docking was first performed between the protein and ligand, and the resultant protein–ligand complex with the best cluster and lowest energy rank was further re-docked with zinc-oxide nanoparticles (ZnO-NPs). The whole procedure was carried out using the AutoDockTools software package (Morris et al., 2009). AutoDock is a molecular modeling simulation software specialized for effective protein–ligand docking, which is available under the GNU General Public License and is one of the most cited software used for docking applications in the research community.
AutoGrid was used to pre-calculate the three-dimensional grid of interaction energy based on macromolecular coordinates. AutoGrid constructs a three-dimensional grid surrounding the coordinates for the protein target and calculates the interaction energy of each grid point within it, thus creating a three-dimensional “array” of interaction energies called a “grid map” (Morris et al., 2008). This is done for the rapid evaluation of interaction energies as the completed grid of energies provided a quick lookup table (Goodsell et al., 1996). For both the docking studies, the entire macromolecule or the protein complex was enclosed within the grid box for the programme to create a larger amount of grid maps and search for the best interaction residues instead of limiting it to selective space.
The actual docking simulation was achieved with AutoDock4 for both rigid docking of protein and phytochemical ligand, and the resultant complex with the nanoparticle. A total of 1,000 runs of genetic algorithm (GA) with default parameters were used as search parameters and the output was set to the Lamarckian genetic algorithm (LGA) docking, also known as a hybrid genetic algorithm-local search (GA-LS).
The biofilm was developed on the surface of the glass slip and was rinsed with phosphate buffer saline maintained at pH 7.4. Green synthesized ZnO NP was used for treating the biofilm being casted on the surface of the glass slide. Both treated and untreated samples of biofilm were scanned by the use of AFM (NT-MDT, Russia) at a speed of 1 Hz.
The parameters that were used for the purpose of optimizing the synthesis of ZnO NPs include pH, the volume of the extract of the plant used, and the concentration of zinc nitrate, which plays an essential role in the mechanism of synthesizing ZnO NPs. Thus, these three important parameters were taken into consideration for further study with RSM. RSM was used to optimize the use of three operational variables: pH, the volume of the extract, and the concentration of zinc nitrate for the purpose of better production of the ZnO NPs (Table 2). The values at Y showed that the parameters had a significant effect on the production of ZnO NPs (Table 3). The Box-Behnken design was used for the purpose of optimizing the three variables comprising 46 runs with five replicates of the central point (Figure 2). The optimization of the parameters was conducted one-factor-at-time and the results were in ignorance of the interactions between the process variables. The model helped in predicting the maximum absorbance of the biogenic ZnO NPs that showed an F-value of 15.97 and a p-value of <0.01 that greatly implies that the model was used for the purpose of optimizing the conditions of NPs synthesis is significant (Figure 2, Table 2).
Figure 2. (A–J) Optimization of parameters for the synthesis of green-synthesized ZnO by C. ternatea.
The training of the neural network by a subset of various data matrices along with validation and testing regulates the performance of ANN-TLBO (training: testing: validation = 70:15:15). Thus, the ANN-TLBO model is implemented on the investigational data obtained from the executed BBD, the data set was split into three subsets (32:7:7) for the purpose of training, testing and validating the model. The mean square obtained from the analysis is considered as an indicator of performance, and R2 (Correlation coefficient) is considered the precision index (Sarkar et al., 2020, 2022). The regression plots of the neural networks help in the representation of the training set, testing set, and validation set. The ANN-TLBO model for determining the biogenic ZnO nanoparticle synthesis comprises five input neuron layer that represents the process parameters, three hidden, and one output layer with the correlation coefficient value of 0.99939. On the other hand, Staphylococcus aureus comprises one input and four hidden neuron layers with a correlation coefficient value of 0.95709. It was further observed that the predicted values by ANN-TLBO were in close proximity with the actual run and the higher R2 value in comparison to that of the RSM model illustrated that it was able to build a more robust model in comparison to that of the RSM technique (Figure 3, Table 3).
Figure 3. ANN-TLBO approach for ZnO NP synthesis. (A) Model architecture, (B) error histogram of the proposed model, (C) performance plot of the proposed model, and (D) regression plot for training, testing, and validation.
The green synthesis of ZnO nanoparticles from C. ternatea extract includes plant secondary metabolites those act as reducing as well as stabilizing agents of Zn ions in solutions of zinc oxide. The green synthesis of ZnO NPs from C. ternatea showed a broad peak at 335 nm (Figure 4) when scanned in the wavelength range of 300–450 nm. For ZnO nanoparticles, the absorbance peak is reported between 310 and 360 nm of wavelength (Song and Yang, 2016; Jayachandran and Nair, 2021) (Figure 4). The XRD patterns obtained by XRD (Figure 5) showed the synthesis of ZnO NP. The pattern of the crystalline peaks corresponding to (002), (101), (102), (110), (103), and (112) was almost similar to the work performed by previously published work (Alahmdi, 2022).
The green synthesized ZnO NPs thus formed were observed to be spherical in shape within the size range of 10–20 nm when observed with TEM (Figure 6).
The green-synthesized ZnO NPs were able to bring about the degradation of the biofilm with the enfeeblement of the structural components of the EPS constituting the biofilm (Hsueh et al., 2015). It was observed that the green-synthesized ZnO NPs were able to reduce the biofilm formed by A. faecalis and P. gingivalis by 92.27 ± 1.22% and 95.27 ± 1.28%, respectively. This was similar to the work done by Husain et al. (2022), which showed that the biosynthesized ZnO NPs were able to bring about degradation of the biofilm formed by E. coli, S. aureus, and P. aeruginosa (Husain et al., 2022). The destabilization of biofilm architecture can be possible with the degradation of the EPS matrix (Pinto et al., 2020). The EPS has two architectural components, carbohydrates and proteins, which provide strength to the biofilm structure (Flemming et al., 2007). It was observed that the green-synthesized ZnO NPs were able to bring a marked reduction in the carbohydrate and protein content of the EPS. It was observed that the carbohydrate content within the EPS of A. faecalis and P. gingivalis was markedly reduced by 84.26 ± 1.09 % and 90.12 ± 1.09%, respectively, on the action of green-synthesized ZnO NPs. The NPs were also responsible for a marked reduction in the protein content of the EPS by 81.26 ± 1.09% and 88.23 ± 1.89%, respectively. This observation greatly portrayed the biogenic ZnO NPs were effective in the enfeeblement of biofilm by bringing about the destruction of the EPS (Lahiri et al., 2021d) (Figure 7).
Figure 7. (A) Reduction of biofilm formed by A. faecalis and P. gingivalis in the presence of green-synthesized ZnO NPs. The ZnO NPs were also responsible for a marked reduction in the EPS with the degradation of (B) the carbohydrate and (C) protein content.
The biogenic ZnO NPs possess the ability to penetrate through the biofilm and act on the cells by bringing degradation in the genomic DNA and RNA content of the cell (Kamli et al., 2021). The biogenic ZnO NPs brought a marked reduction in the genomic DNA content of A. faecalis and P. gingivalis by 94.26 ± 1.09% and 96.23 ± 1.89%, respectively. It was also observed that the NPs were further responsible to reduce the RNA content of the sessile cells in both A. faecalis and P. gingivalis by 95.26 ± 1.01% and 98.23 ± 1.83%, respectively (Figure 8).
Figure 8. Biogenic ZnO NPs were responsible for a marked reduction in the DNA (A) and RNA (B) content of the sessile microbial colonies of A. faecalis and P. gingivalis.
The morphological and numerical alterations of biofilm-producing cells of the A. faecalis and P. gingivalis imparted by the green-synthesized ZnO NP were reflected by the scanning electron micrographs (Figure 9). The ZnO NP brought about a significant reduction in the number of biofilm-producing cells but also brought about a notable shrinkage in the cellular morphology and fewer colonization areas were visible compared to the control. Bacterial cells were found to form different layers of extracellular polymeric substance (EPS) in the control set which upon treatment with ZnO NP was reduced to single layers of cells showing a visible loss of the EPS and release of the cytoplasmic content. This shows that the NP brings about a substantial reduction in the biofilm along with considerable elimination of the sessile microcolonies.
Figure 9. SEM images of P. gingivalis (A,B) control and treated with biogenic ZnO NPs and A. faecalis control and treated (C,D) with biogenic ZnO NPs.
The loss of biofilm production ability by the green synthesized ZnO-challenged cells of A. faecalis and P. gingivalis was checked by the fluorescence microscopic study (Figure 10). It showed a thick coating of biofilm in untreated conditions, whereas the treatment with ZnO NP resulted in a scattered appearance with a much lesser number of cells and a significantly reduced amount of biofilm. The application of the ZnO NP resulted in the killing of the cells as they appeared reddish in color in the presence of propidium iodide.
Figure 10. Fluorescence images of untreated and treated sessile cells by ZnO NPs of P. gingivalis (A,B) and A. faecalis (C,D).
In this study, all the selected ligands were observed to have <10 hydrogen donors; however, azadirachtin was observed to have 16 hydrogen acceptors that resulted in a Lipinski violation. Apart from that, azadirachtin also had a molecular weight >500. Except for azadirachtin and nimbin, all the compounds had zero violations of Lipinski's rule of drug-likeness. However, azadirachtin was found to have the highest synthetic accessibility. All the compounds except the same had high gastrointestinal absorption, and none of the compounds are permeable to the blood brain barrier (Table 4).
AutoDock uses a “hybrid” force field that employs a “full” desolvation model, and also considers directionality in hydrogen bonds (Sarkar et al., 2021a). To estimate the interaction energy, it calculates the energy of ligand and protein in the unbound state first and then calculates the energy of the protein-ligand complex. Finally, these values are collectively used to compute the lowest and mean binding energy values. The lowest the energy value, the better is the interaction score of docking. In this study, nimbolide was found to have the best interaction with both 5OLJ and DMR19 proteins. Each of the compounds is observed to have inhibition constants in the micromolar range (uM). The inhibition concentration is a measure of the potency of an inhibitor. Mathematically, it is the concentration required to produce half the maximum inhibition.
The binding energy values of all complexes significantly decreased by ~3 units. Double-docking with the ZnO nanoparticle was also found to enhance the number of cluster formations in all the complexes. In nimbolide, the number of clusters was found to be enhanced by a factor of 21 on docking with nanoparticles. ZnO nanoparticles also had a considerably lower inhibition constant (Ki), which was in the picoMolar and nanoMolar concentrations for biofilm forming proteins P. gingivalis and A. faecalis, respectively. This implies that a very low concentration of ZnO nanoparticles can be used to get the desired strong inhibition of the biofilm formation (Figure 11, Table 5).
Figure 11. Docked poses and three-dimensional interactions of biofilm producing bacterial protein P. gingivalis and A. faecalis with phytochemical: (1A,C) Azadirachtin, (1B,D) Quercetin and the respective phytocompounds along with nanoparticles (2A,C) and (2B,D).
AFM revealed that the tested strains was able to form biofilm and had the potency to adhere to a glass surface (Figure 12A). On the other hand, green synthesized ZnO NP decreased significantly the adherence to glass (Figure 12B). In addition, the combination of green synthesized ZnO NP decreased significantly the biofilm formation. AFM clearly showed the disrupted surface topology and height distribution profile of the biofilm developed in the presence of NP compared to the control biofilm.
Figure 12. Detection of attenuation of biofilm by Atomic force microscope (A) tested strains was able to form a biofilm (B) green synthesized ZnO NP decreased significantly the adherence to glass.
This study is focused on the efficiency of the green-synthesized ZnO NPs in the elimination of biofilm formed by A. faecalis and P. gingivalis. This was further ratified by the in silico studies. The recent study focused on the bioaugmented production of ZnO-NPs using floral extract of C. ternatea revealed the fact that it has a great possibility for making a nano-based herbal mouthwash with antibiofilm activities, which may be used for checking oral biofilm-induced diseases like periodontitis and tooth loss.
The original contributions presented in the study are included in the article/supplementary material, further inquiries can be directed to the corresponding author/s.
Conceptualization and writing—review and editing: DL, MN, SPat, TS, and RR. Methodology: DL, MN, TS, SPan, SG, and MM. Formal analysis: DL, MN, MM, and RR. Investigation: DL, MN, and RR. Writing—original draft preparation: DL, MN, ZA, HE, SPat, TS, and RR. All authors have read and agreed to the published version of the manuscript.
The authors would like to acknowledge the University of Engineering and Management, Kolkata and Universiti Sains Malaysia for funding the work carried out in this manuscript.
Author SPat was employed by NatNov Bioscience Private Ltd. and VU was employed by AMH Energy Pvt. Ltd.
The remaining authors declare that the research was conducted in the absence of any commercial or financial relationships that could be construed as a potential conflict of interest.
All claims expressed in this article are solely those of the authors and do not necessarily represent those of their affiliated organizations, or those of the publisher, the editors and the reviewers. Any product that may be evaluated in this article, or claim that may be made by its manufacturer, is not guaranteed or endorsed by the publisher.
Alahmdi, M. I. (2022). In Vitro Anticancer and Antibacterial Activity of Green Synthesized ZnO NPs Using Clitorea Ternatea Flower Extract : Inhibits MCF-7 Cell Proliferation Via Intrinsic Apoptotic Pathway. 1–21. doi: 10.21203/rs.3.rs-1269775/v1
Allaker, R. P. (2011). “The use of antimicrobial nanoparticles to control oral infections,” in Nano-Antimicrobials eds N. Cioffi, and M. Rai (Berlin, Heidelberg: Springer), 395–425. doi: 10.1007/978-3-642-24428-5_14
Allen, T. M., and Cullis, P. R. (2004). Drug delivery systems: entering the mainstream. Science 303, 1818–1822. doi: 10.1126/science.1095833
Baishya, R., Bhattacharya, A., Mukherjee, M., Lahiri, D., and Banerjee, S. (2016). Establishment of a simple reproducible model for antibiotic sensitivity pattern study of biofilm forming staphylococcus aureus. Mater. Today Proc. 3, 3461–3466. doi: 10.1016/j.matpr.2016.10.028
Bala, N., Saha, S., Chakraborty, M., Maiti, M., Das, S., Basu, R., et al. (2015). Green synthesis of zinc oxide nanoparticles using Hibiscus subdariffa leaf extract: effect of temperature on synthesis, anti-bacterial activity and anti-diabetic activity. RSC Adv. 5, 4993–5003. doi: 10.1039/C4RA12784F
Balouiri, M., Sadiki, M., and Ibnsouda, S. K. (2016). Methods for in vitro evaluating antimicrobial activity: a review. J. Pharm. Anal. 6, 71–79. doi: 10.1016/j.jpha.2015.11.005
Bharadwaj, K. K., Rabha, B., Pati, S., Choudhury, B. K., Sarkar, T., Gogoi, S. K., et al. (2021). Green synthesis of silver nanoparticles using diospyros malabarica fruit extract and assessments of their antimicrobial, anticancer and catalytic reduction of 4-nitrophenol (4-NP). Nanomaterials 11, 1999. doi: 10.3390/nano11081999
Caputo, L., Quintieri, L., Cavalluzzi, M. M., Lentini, G., and Habtemariam, S. (2018). Antimicrobial and antibiofilm activities of citrus water-extracts obtained by microwave-assisted and conventional methods. Biomedicine 6, 70. doi: 10.3390/biomedicines6020070
Daina, A., Michielin, O., and Zoete, V. (2017). SwissADME: a free web tool to evaluate pharmacokinetics, drug-likeness and medicinal chemistry friendliness of small molecules. Sci. Rep. 7, 42717. doi: 10.1038/srep42717
Flemming, H.-C., Neu, T. R., and Wozniak, D. J. (2007). The EPS matrix: the “house of biofilm cells”. J. Bacteriol. 189, 7945–7947. doi: 10.1128/JB.00858-07
Goodsell, D. S., Morris, G. M., and Olson, A. J. (1996). Automated docking of flexible ligands: applications of AutoDock. J. Mol. Recognit. 9, 1–5.3. doi: 10.1002/(SICI)1099-1352(199601)9:<1::AID-JMR241>23.0.CO;2-6
Hanwell, M. D., Curtis, D. E., Lonie, D. C., Vandermeersch, T., Zurek, E., and Hutchison, G. R. (2012). Avogadro: an advanced semantic chemical editor, visualization, and analysis platform. J. Cheminform. 4, 17. doi: 10.1186/1758-2946-4-17
Hsueh, Y.-H., Ke, W.-J., Hsieh, C.-T., Lin, K.-S., Tzou, D.-Y., and Chiang, C.-L. (2015). ZnO nanoparticles affect bacillus subtilis cell growth and biofilm formation. PLoS ONE 10, e0128457. doi: 10.1371/journal.pone.0128457
Husain, F. M., Qais, F. A., Ahmad, I., Hakeem, M. J., Baig, M. H., Masood Khan, J., et al. (2022). Biosynthesized zinc oxide nanoparticles disrupt established biofilms of pathogenic bacteria. Appl. Sci. 12, 710. doi: 10.3390/app12020710
Jamdagni, P., Khatri, P., and Rana, J. S. (2018). Green synthesis of zinc oxide nanoparticles using flower extract of Nyctanthes arbor-tristis and their antifungal activity. J. King Saud Univ. Sci. 30, 168–175. doi: 10.1016/j.jksus.2016.10.002
Jan, H., Shah, M., Usman, H., Khan, M. A., Zia, M., Hano, C., et al. (2020). Biogenic Synthesis and characterization of antimicrobial and antiparasitic zinc oxide (ZnO) nanoparticles using aqueous extracts of the himalayan columbine (Aquilegia pubiflora). Front. Mater. 7, 249. doi: 10.3389/fmats.2020.00249
Jayachandran, A. T R A, and Nair, A. S. (2021). Green synthesis and characterization of zinc oxide nanoparticles using Cayratia pedata leaf extract. Biochem. Biophys. Rep. 26, 100995. doi: 10.1016/j.bbrep.2021.100995
Kamli, M. R., Malik, M. A., Srivastava, V., Sabir, J. S. M., Mattar, E. H., and Ahmad, A. (2021). Biogenic ZnO nanoparticles synthesized from origanum vulgare abrogates quorum sensing and biofilm formation in opportunistic pathogen Chromobacterium violaceum. Pharmaceutics 13, 1743. doi: 10.3390/pharmaceutics13111743
Khatoon, Z., McTiernan, C. D., Suuronen, E. J., Mah, T. F., and Alarcon, E. I. (2018). Bacterial biofilm formation on implantable devices and approaches to its treatment and prevention. Heliyon 4, e01067. doi: 10.1016/j.heliyon.2018.e01067
Lahiri, D., Nag, M., Banerjee, R., Mukherjee, D., Garai, S., Sarkar, T., et al. (2021a). Amylases: biofilm inducer or biofilm inhibitor? Front. Cell. Infect. Microbiol. 11, 660048. doi: 10.3389/fcimb.2021.660048
Lahiri, D., Nag, M., Dutta, B., Sarkar, T., and Ray, R. R. (2021b). Artificial Neural Network and Response Surface Methodology-Mediated Optimization of Bacteriocin Production by Rhizobium leguminosarum. Iran. J. Sci. Technol. Trans. A Sci. 45, 1509–1517. doi: 10.1007/s40995-021-01157-6
Lahiri, D., Nag, M., Sarkar, T., Dutta, B., and Ray, R. R. (2021c). Antibiofilm activity of α-amylase from bacillus subtilis and prediction of the optimized conditions for biofilm removal by Response Surface Methodology (RSM) and Artificial Neural Network (ANN). Appl. Biochem. Biotechnol. 193, 1853–1872. doi: 10.1007/s12010-021-03509-9
Lahiri, D., Nag, M., Sheikh, H. I., Sarkar, T., Edinur, H., atan Siddhartha, P., et al. (2021d). Microbiologically synthesized nanoparticles and their role in silencing the biofilm signaling cascade. Front. Microbiol. 12, 636588. doi: 10.3389/fmicb.2021.636588
Limoli, D. H., Jones, C. J., and Wozniak, D. J. (2015). Bacterial extracellular polysaccharides in biofilm formation and function. Microbiol. Spectr. 3, 1–30. doi: 10.1128/9781555817466.ch11
Maeda, H., Wu, J., Sawa, T., Matsumura, Y., and Hori, K. (2000). Tumor vascular permeability and the EPR effect in macromolecular therapeutics: a review. J. Control. Release 65, 271–284. doi: 10.1016/S0168-3659(99)00248-5
Momma, K., and Izumi, F. (2008). VESTA: a three-dimensional visualization system for electronic and structural analysis. J. Appl. Crystallogr. 41, 653–658. doi: 10.1107/S0021889808012016
Morris, G. M., Huey, R., Lindstrom, W., Sanner, M. F., Belew, R. K., Goodsell, D. S., et al. (2009). AutoDock4 and AutoDockTools4: automated docking with selective receptor flexibility. J. Comput. Chem. 30, 2785–2791. doi: 10.1002/jcc.21256
Morris, G. M., Huey, R., and Olson, A. J. (2008). Using AutoDock for ligand-receptor docking. Curr. Protoc. Bioinforma. Chapter 8, 14. doi: 10.1002/0471250953.bi0814s24
Morse, D. J., Wilson, M. J., Wei, X., Lewis, M. A. O., Bradshaw, D. J., Murdoch, C., et al. (2018). Denture-associated biofilm infection in three-dimensional oral mucosal tissue models. J. Med. Microbiol. 67, 364–375. doi: 10.1099/jmm.0.000677
Pinto, R. M., Soares, F. A., Reis, S., Nunes, C., and Van Dijck, P. (2020). Innovative strategies toward the disassembly of the EPS matrix in bacterial biofilms. Front. Microbiol. 11, 952. doi: 10.3389/fmicb.2020.00952
Quintieri, L., Fanelli, F., Zühlke, D., Caputo, L., Logrieco, A. F., Albrecht, D., et al. (2020). Biofilm and pathogenesis-related proteins in the foodborne P. fluorescens ITEM 17298 with distinctive phenotypes during cold storage. Front. Microbiol. 11, 991. doi: 10.3389/fmicb.2020.00991
Sahoo, S. K., and Labhasetwar, V. (2003). Nanotech approaches to drug delivery and imaging. Drug Discov. Today 8, 1112–1120. doi: 10.1016/S1359-6446(03)02903-9
Sarkar, T., Bharadwaj, K. K., Salauddin, M., Pati, S., and Chakraborty, R. (2021a). Phytochemical characterization, antioxidant, anti-inflammatory, anti-diabetic properties, molecular docking, pharmacokinetic profiling, and network pharmacology analysis of the major phytoconstituents of raw and differently dried Mangifera indica (Himsaga). Appl. Biochem. Biotechnol. 194, 950–987. doi: 10.1007/s12010-021-03669-8
Sarkar, T., Salauddin, M., Choudhury, T., Um, J. S., Pati, S., Hazra, S. K., et al. (2021b). Spatial optimisation of mango leather production and colour estimation through conventional and novel digital image analysis technique. Spat. Inf. Res. 29, 439–453. doi: 10.1007/s41324-020-00377-z
Sarkar, T., Salauddin, M., Hazra, S., and Chakraborty, R. (2020). Artificial neural network modelling approach of drying kinetics evolution for hot air oven, microwave, microwave convective and freeze dried pineapple. SN Appl. Sci. 2, 1621. doi: 10.1007/s42452-020-03455-x
Sarkar, T., Salauddin, M., Mukherjee, A., Shariati, M. A., Rebezov, M., Tretyak, L., et al. (2022). Application of bio-inspired optimization algorithms in food processing. Curr. Res. Food Sci. 5, 432–450. doi: 10.1016/j.crfs.2022.02.006
Song, Y., and Yang, J. (2016). Preparation and in-vitro cytotoxicity of zinc oxide nanoparticles against osteoarthritic chondrocytes. Trop. J. Pharm. Res. 15, 2321. doi: 10.4314/tjpr.v15i11.4
Stalin, B., Ramesh Kumar, P., Ravichandran, M., Siva Kumar, M., and Meignanamoorthy, M. (2019). Optimization of wear parameters using Taguchi grey relational analysis and ANN-TLBO algorithm for silicon nitride filled AA6063 matrix composites. Mater. Res. Express 6, 106590. doi: 10.1088/2053-1591/ab3d90
Tailor, G., Chaudhay, J., Verma, D., and Kr. Sarma, B. (2019). Microscopic study of zinc nanoparticles synthesised using thermosetting polymer. Appl. Microsc. 49, 20. doi: 10.1186/s42649-019-0018-0
Thrall, J. H. (2004). Nanotechnology and medicine. Radiology 230, 315–318. doi: 10.1148/radiol.2302031698
Vasir, J. K., and Labhasetwar, V. (2005). Targeted drug delivery in cancer therapy. Technol. Cancer Res. Treat. 4, 363–374. doi: 10.1177/153303460500400405
Keywords: antibiofilm, dental biofilm, Clitoria ternatea, response surface methodology, artificial neural network
Citation: Lahiri D, Ray RR, Sarkar T, Upadhye VJ, Ghosh S, Pandit S, Pati S, Edinur HA, Abdul Kari Z, Nag M and Ahmad Mohd Zain MR (2022) Anti-biofilm efficacy of green-synthesized ZnO nanoparticles on oral biofilm: In vitro and in silico study. Front. Microbiol. 13:939390. doi: 10.3389/fmicb.2022.939390
Received: 09 May 2022; Accepted: 11 August 2022;
Published: 03 October 2022.
Edited by:
Laura Quintieri, Italian National Research Council, ItalyReviewed by:
Jun Mei, Shanghai Ocean University, ChinaCopyright © 2022 Lahiri, Ray, Sarkar, Upadhye, Ghosh, Pandit, Pati, Edinur, Abdul Kari, Nag and Ahmad Mohd Zain. This is an open-access article distributed under the terms of the Creative Commons Attribution License (CC BY). The use, distribution or reproduction in other forums is permitted, provided the original author(s) and the copyright owner(s) are credited and that the original publication in this journal is cited, in accordance with accepted academic practice. No use, distribution or reproduction is permitted which does not comply with these terms.
*Correspondence: Moupriya Nag, bW91cHJpeWEubmFnQHVlbS5lZHUuaW4=; Muhammad Rajaei Ahmad Mohd Zain, cmFqYWVpQHVzbS5teQ==
†These authors have contributed equally to this work
‡ORCID: Tanmay Sarkar orcid.org/0000-0003-3869-1604
Disclaimer: All claims expressed in this article are solely those of the authors and do not necessarily represent those of their affiliated organizations, or those of the publisher, the editors and the reviewers. Any product that may be evaluated in this article or claim that may be made by its manufacturer is not guaranteed or endorsed by the publisher.
Research integrity at Frontiers
Learn more about the work of our research integrity team to safeguard the quality of each article we publish.