- 1Department of Urology, Renji Hospital, Shanghai Jiao Tong University School of Medicine, Shanghai, China
- 2Department of Urology, Shanghai Tenth People’s Hospital, School of Medicine in Tongji University, Shanghai, China
- 3Department of Laboratory Medicine, Renji Hospital, Shanghai Jiao Tong University School of Medicine, Shanghai, China
Different gut microbiota is implicated in different diseases, including cancer. However, gut microbiota differences between individuals with clear cell renal cell carcinoma (ccRCC) and healthy individuals are unclear. Here, we analyzed gut microbiota composition in 51 ccRCC patients and 40 healthy controls using 16S rRNA sequencing analysis. We observed that Blautia, Streptococcus, [Ruminococcus]_torques_group, Romboutsia, and [Eubacterium]_hallii_group were dominant and positively associated with ccRCC. We isolated and cultured Streptococcus lutetiensis to characterize specific gut microbiota that promotes ccRCC and found that it promoted in vitro ccRCC proliferation, migration, and invasion via the TGF-signaling pathway. Interactions identified between the gut microbiota and ccRCC suggest the gut microbiota could serve as a potential non-invasive tool for predicting ccRCC risk and also function as a cancer therapy target.
Introduction
Globally, renal cell carcinoma (RCC) is the seventh most prevalent cancer (Padala et al., 2020). According to 2020 GLOBOCAN data produced by the International Agency for Research on Cancer (IARC), 431,288 new RCC cases occurred, constituting 2.2% of all cancer diagnoses, with the deaths of 179,368 individuals, and accounting for 1.8% of global cancer deaths. Clear cell renal cell carcinoma (ccRCC) accounts for 70% of RCC and is the most common histological subtype (Kabaria et al., 2016). Approximately two-thirds of RCC cases are localized, but 30–40% tend to progress to metastasis, regardless of complete surgical resection or not (Kim et al., 2017). The 5-year relative survival rate for early renal cancer, characterized as localized tumors in the kidney, is 92% (Kabaria et al., 2016). Although some prognostic markers, such as BAP1 and PBRM1 may predict clinical RCC outcomes (Boorjian, 2014), no definitive markers are yet available to predict the risk of developing RCC.
The gut microbiota is involved in the development of cancer. For example, gut microbiota is correlated with chemo-radiotherapy response rates in colorectal cancer (CRC) (Yi et al., 2021). Moreover, they also impact immunotherapy responses during metastatic RCC (Derosa et al., 2020), which is distant from the gastrointestinal tract. The kidneys interact with the gut microbiota and reflect the “gut-kidney axis” (Giordano et al., 2021). Renal toxins such as trimethylamine oxide (TMAO) originate from microbial metabolites and promote progressive renal tubular-interstitial fibrosis and kidney impairment (Evenepoel et al., 2017). A fecal microbiota transplantation study showed that mice receiving Eggerthella lenta and Fusobacterium nucleatum exhibited more severe renal fibrosis, glomerulosclerosis and oxidative stress, and increased serum levels of uremic toxins, including p-cresol sulfate, phenylacetylglycine, phenyl sulfate, and indoxyl sulfate, while Bifidobacterium animalis decreased the abundance of the aforementioned species and relieving renal impairment severity (Wang et al., 2020). Another study (Denburg et al., 2020), on calcium oxalate kidney stone disease in children showed that oxalate-degrading bacterial taxa, including E. lenta, were decreased in children who had kidney stones, and suggested gut microbiota dysbiosis caused this disease by perturbing the “gut-kidney axis.”
Herein, we investigated differences in gut microbiota diversity between ccRCC and healthy controls (HCs) and identified five biomarkers in a model predicting the ccRCC incidence rate. In addition, we shed light on how gut microbiota affects ccRCC development, which could be used as a potential target to inhibit ccRCC.
Materials and Methods
Patients
In total, 51 ccRCC patients and 40 HCs at Renji Hospital, Shanghai Jiao Tong University School of Medicine, were recruited between March 2021 and June 2021. ccRCC patients were diagnosed according to histology. Inclusion criteria include (1) normal blood glucose and blood pressure, (2) normal liver and kidney function test ranges, and (3) not taking antibiotics or prebiotics three months before fecal sample collection. Fecal samples were collected according to the approved protocol by the Renji hospital Ethics Committees ahead of the procedure of the enrollment, and written informed consent was obtained from both groups. All fecal samples were freshly collected and frozen at −80°C.
DNA Extraction, 16S rRNA Gene Amplicon Sequencing, and Data Processing
Total genomic DNA from fecal samples was extracted using the acetyl trimethylammonium bromide (CTAB) method (Bailey et al., 2022). DNA concentration and purity were monitored using 1% agarose gels. The 16S rRNA V3–V4 region was amplified and sequenced using NovaSeq PE250 (Illumina, CA, United States). Sequencing libraries were generated using the TruSeq® DNA PCR-Free Sample Preparation Kit (Illumina, CA, United States) and index codes were added to the following manufacturer’s recommendations. Library quality was assessed using the Qubit@ 2.0 Fluorometer (Thermo Scientific, MA, United States) and the Agilent Bioanalyzer 2100 system (Agilent Technologies Inc., CA, United States). The library was then sequenced on an Illumina NovaSeq platform, with 250 base pair (bp) paired-end reads generated and assigned to each sample based on barcodes and then merged with FLASH (V1.2.7) (Magoč and Salzberg, 2011). Quality filtering of raw tags was performed under specific filtering conditions to generate high-quality clean tags (Bokulich et al., 2013) according to Quantitative Insights Into Microbial Ecology (QIIME, V1.9.1) (Caporaso et al., 2010) quality control processes. Tags were compared with a reference database (Silva) using the UCHIME algorithm (Edgar et al., 2011) to detect and remove chimeric sequences (Haas et al., 2011), generating effective tags.
Sequence analysis was performed in Uparse software (v7.0.1001) (Edgar, 2013). Sequences with ≥97% similarity were assigned to the same operating taxonomic units (OTUs). For each representative sequence, the Silva database (Quast et al., 2013) was used based on Mothur algorithms to annotate taxonomic information. To study the phylogenetic relationships between different OTUs, and differences in dominant species between different samples (groups), multiple sequence alignments were conducted using MUSCLE software (Version 3.8.311) (Edgar, 2004). OTU abundance was normalized using a standard sequence number corresponding to the sample with the least sequences. We calculated α- and β-diversity in QIIME (Version 1.7.0 and 1.9.1, respectively) and displayed data using R version 4.1.1 (R Foundation for Statistical Computing, Vienna, Austria) and GraphPad Prism 6 software (GraphPad Software, Inc., CA, United States). Tax4Fun function predictions were processed using the nearest neighbor method based on minimum 16S rRNA sequence similarity (Aßhauer et al., 2015).
Bacterial Strains and Culture Conditions
Streptococcus lutetiensis was derived from ccRCC fecal samples and grown at 37°c in brain-heart infusion (BHI) broth, or on Columbia blood agar plates (BKMAM, China). Starter cultures were prepared by growing strains overnight in 3 ml BHI broth. The overnight culture was diluted at 1:100 in fresh BHI broth. Cells were harvested at the exponential growth phase; optical density (OD) = 0.8 at 600 nm, measured by a microplate reader (BioTek, VT, United States).
Cell Lines
Human RCC ACHN and A498 cells were cultured in Modified Eagle Medium (MEM) (Servicebio, China) containing 10% fetal bovine serum (FBS) (Thermo Scientific, MA, United States) and 1% penicillin/streptomycin (Servicebio, China) at 37°C with 5% CO2. Cell lines were obtained from the American Type Culture Collection (Manassas, VA, United States).
5-Ethynyl-2′-Deoxyuridine Assay
An 5-ethynyl-2′-deoxyuridine (EdU) incorporation assay was performed using the Cell-Light™ EdU Apollo®567 in vitro flow cytometry kit (Ribobio, China) according to the manufacturer’s instructions. Briefly, A498 and ACHN cells were cultured in MEM supplemented with 50 μM EdU for 2 h at 37°C and washed three times in cold phosphate-buffered saline (PBS) (Servicebio, China). Cells were fixed in 4% paraformaldehyde (Servicebio, China), resuspended in 100 μl 1 × Apollo reaction buffer (Ribobio, China), and incubated at room temperature for 30 min. Cells were then washed twice in PBS plus 0.5% Triton X-100 (Thermo Scientific, MA, United States), stained in 1 × Hoechst 33342 reaction buffer (Ribobio, China) for 30 min at room temperature, washed twice in PBS, and finally resuspended in 300 μl PBS. Cells were observed using an inverted immunofluorescence microscope at ×10 magnification (Olympus, Japan).
Wound Healing Assay
Upon reaching 90% confluence, cell monolayers were scratched with a 200 μl sterile pipette tip, with detached cells washed away in PBS. Then, cells were cultured at 37°C in MEM plus 2% FBS. Cells migrating into wound sites were analyzed over a 20 h period, with plates imaged at 0 and 20 h using an inverted light microscope (magnification ×100, Olympus). Cell migration was quantified by determining the extent (%) of wound healing using ImageJ (v1.52a) (Wayne Rasband, United States); (0 h scratch area − 24 h scratch area)/0 h scratch area × 100%.
Transwell Invasion Assay
Cell invasion was analyzed using Transwell chambers pre-coated with 20% Matrigel (Corning, NY, United States) for 20 h at 37°C. Briefly, 5 × 104 cells in serum-free MEM were added to the upper chambers of Transwell inserts, while MEM plus 10% FBS (chemoattractant) was added to the lower chambers. Following incubation for 20 h at 37°C, non-invaded cells were removed, and invading cells in the lower chambers were fixed in absolute ethanol for 10 min and stained in 0.5% crystal violet (Servicebio, China) for 10 min at room temperature. Invaded cells were counted in one randomly selected field from each chamber using an inverted light microscope (magnification ×100; Olympus).
Quantitative Real-Time PCR
RNA was extracted using QIAzol lysis reagent (Qiagen, Germany) according to the manufacturer’s instructions. RNA concentrations were measured and samples were stored at −80°C. Reverse transcription was performed according to instructions from the 4 × Reverse Transcription Master Mix kit (EZBioscience, MN, United States).
Streptococcus lutetiensis DNA levels were determined using quantitative real-time PCR (qRT-PCR), which targeted the single-copy gene, superoxide dismutase A (sodA), previously used to distinguish among Streptococcus spp. (Alber et al., 2004). Primer sequences were analyzed using the basic local alignment search tool (BLAST)2 against the GeneBank nucleotide database to confirm primer specificity toward S. lutetiensis. qRT-PCR was performed in a 20 μl reaction volume containing: 2 × Color SYBR Green qPCR Master Mix (Low ROX) (Yeasen, China), 10 μM each primer [S. lutetiensis sodA forward primer; (GGTGGTGGTGCTCTTAACCA) and S. lutetiensis sodA reverse primer (ACGTGTTGTTGCAGCTTTTGT)], and 2 μl DNA from ccRCC fecal samples. The negative control contained DNA from HC fecal samples. Amplification was performed on the QuantStudio 7 Flex Real-Time PCR system (ABI, MA, United States) using the following cycling parameters: denaturation at 95°C for 5 min, and 40 cycles of 95°C for 10 s and 60°C for 30 s. Bacterial loads were determined using standard curves. Samples and standard curves were run in triplicate. Samples with cycle threshold values ≤36 were considered positive for S. lutetiensis.
Western Blotting
Cells were cultured in an appropriate medium in the presence of S. lutetiensis conditioned medium (Sl.CM) or BHI for 6 h and then washed three times in sterile PBS. Cells were then lysed in T-PER Tissue Protein Extraction Reagent (Thermo Scientific, MA, United States), lysates were centrifuged at 12,000 rpm for 10 min, and quantified proteins underwent sodium dodecyl sulfate-gel polyacrylamide gel electrophoresis and Western blotting. The following primary antibodies were used: rabbit GAPDH (1:1,000, Cell Signaling Technology, MA, United States), TGF-β2 (1:1,000, Proteintech, PA, United States), and SMAD2 (1:1,000, Cell Signaling Technology, MA, United States). Membranes were rinsed three times in TBST (Servicebio, China) for 10 min each. Then, membranes were incubated with secondary horse radish peroxidase-conjugated Affinipure Goat Anti-Rabbit IgG antibody (H + L) (1:5,000, Proteintech, PA, United States). Membranes were immersed in super enhanced chemiluminescent plus reagent (Advansta, CA, United States) to develop luminescence.
Statistical Analysis
Significant differences in clinical characteristics were evaluated using an unpaired t-test or Fisher’s exact test. Differences were significant when p < 0.05. The two-tailed Student’s t-test was performed and the p-value adjusted by the Benjamini–Hochberg (BH) correction. The probability level for statistical analysis was set at α = 0.05 and was adjusted by the BH correction to allow for a maximum 5% probability (q = 0.05) of a false positive detection. All data were analyzed in Graph Pad Prism 6 software, R version 4.1.1, and Microsoft Excel (Microsoft Corporation, Seattle, WA, United States).
Results
Clinical Characteristics of Clear Cell Renal Cell Carcinoma Patients and Healthy Controls
All ccRCC patients and HCs were enrolled at Renji Hospital. In total, 98 fecal samples were prospectively collected. After a strict screening process, 51 ccRCC patients and 40 HCs were included for 16S rRNA gene amplicon sequencing (Figure 1). Patient demographics and clinical characteristics are collected from hospital electronic medical records. Clinicopathological variables [gender, age, body mass index (BMI), and tumor-node-metastasis (TNM) stage] (Table 1) from both groups were generally matched.
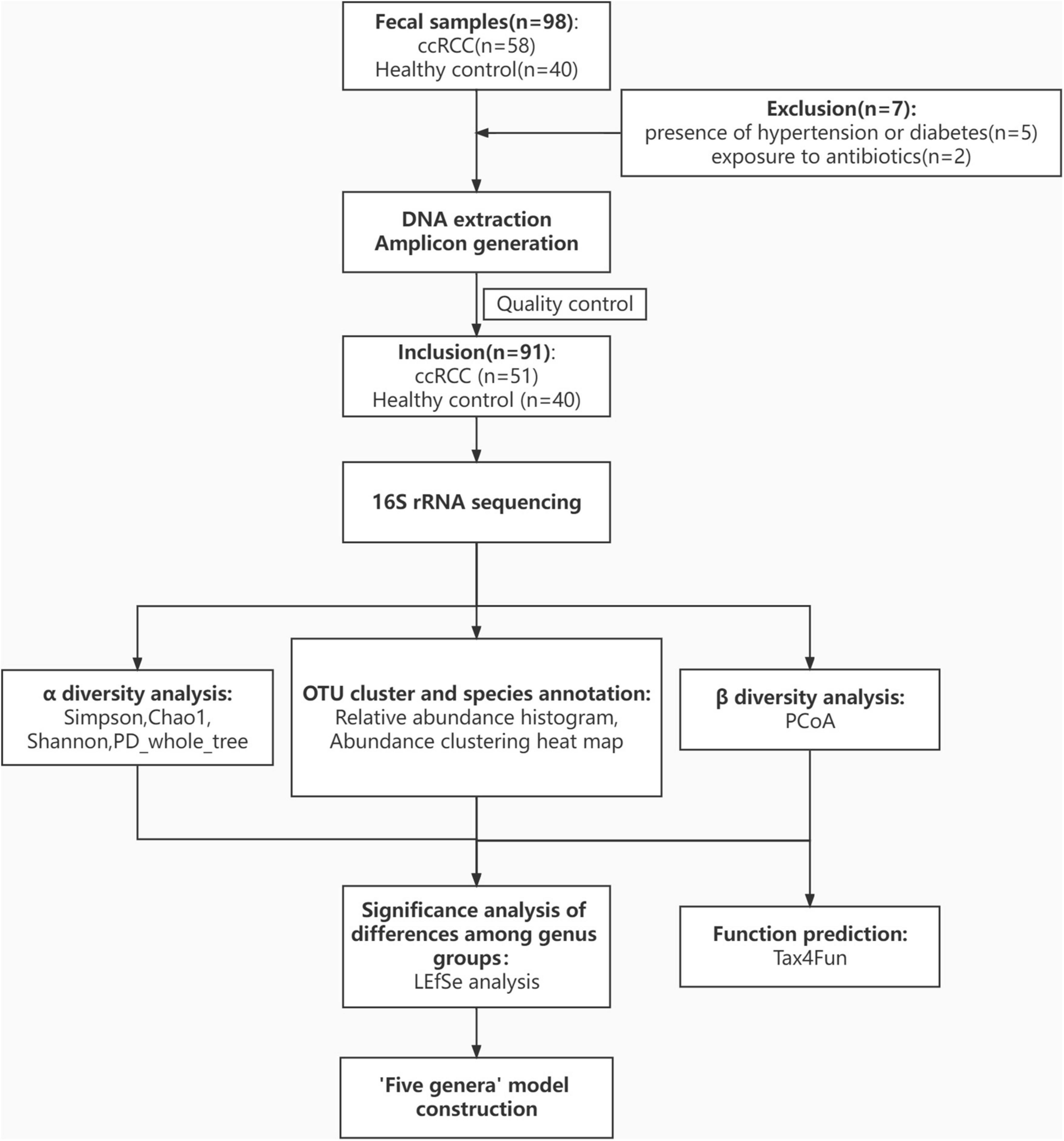
Figure 1. Study design and flow diagram. In total, 98 fecal samples from Renji Hospital were prospectively collected. After excluding 5 patients for the presence of hypertension or diabetes and 2 patients for exposure to antibiotics, 51 patients with ccRCC, and 40 healthy controls were included and their gut microbiota was characterized. ccRCC, clear cell renal cell carcinoma; HC, healthy control.
Increased Gut Microbial Diversity and Microbial Function in Clear Cell Renal Cell Carcinoma Patients
To evaluate differences in bacterial diversity between groups, sequences were aligned to estimate α- and β-diversity. An increased α-diversity was observed in ccRCC group (n = 51) vs. HC group (n = 40), as measured by Simpson, Chao1, Shannon, and PD_whole_tree indices (p = 0.013, 0.0041, 0.014, and 7.68e−05, respectively) using the Wilcoxon rank-sum test) (Figures 2A–D). To indicate microbiome space between groups, β-diversity was calculated using principal coordinate analysis (PCoA) based on unweighted UniFrac values to evaluate variations in community composition. When compared to the HC group, the ccRCC group had a significantly different overall microbiome structure [analysis of similarities (ANOSIM) = 0.1731 and p = 0.001] (Figure 2E). When we examined differentially abundant microbial genes using Tax4Fun, significant differences were identified between groups. These genes involved in transporters, quorum sensing, and cysteine and methionine metabolism were significantly more active in the ccRCC group (Figure 2F).
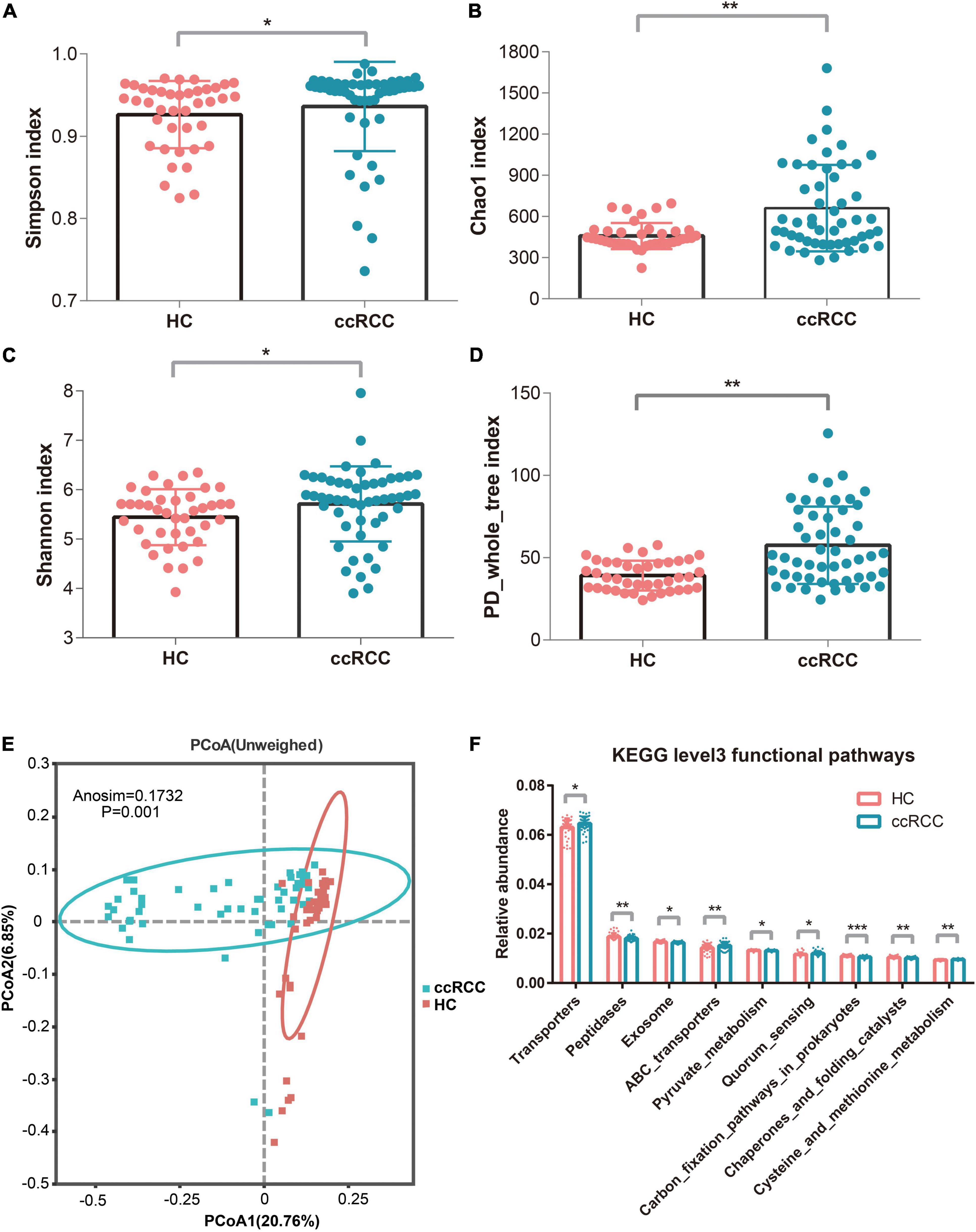
Figure 2. Increased gut microbiota diversity and microbial functions in ccRCC group (n = 51) vs. HC group (n = 40). Alpha diversity, estimated by Simpson (A), Chao1 (B), Shannon (C), and PD_whole_tree index (D) were significantly up-regulated in ccRCC group when compared with HC group (p = 0.013, 0.0041, 0.014, and 7.68e–05, respectively by Wilcoxon rank-sum test). (E) PCoA based on unweighted UniFrac values showed a different taxonomic composition between the ccRCC and HC groups. (F) Tax4Fun analysis identified significant differences in microbial genes between the two groups. Results are presented as the mean ± SD. Statistical evaluations were performed using the Kruskal–Wallis test followed by Dunn’s multiple comparisons test. *p < 0.05, **p < 0.01, *** p < 0.001. ccRCC, clear cell renal cell carcinoma; HC, healthy control; PCoA, principal coordinates analysis; SD, standard deviation.
Characteristic Gut Microbiota in Clear Cell Renal Cell Carcinoma Group
Gut microbiota composition in the ccRCC group at the phylum level was shown (Figure 3A). On average, the combined bacterial phyla of Firmicutes, Bacteroidetes, and Proteobacteria accounted for more than 80% of the sequences and were dominant populations in both groups. At the genus level, the top 35 genera, including Bacteroides, Faecalibacterium, Prevotella, and Escherichia-Shigella are shown (Figure 3B). To compare differences in gut microbiota between groups, linear discriminant analysis effect-size (LEfSe) was used to identify specific bacteria associated with ccRCC (Figure 3C). The five genera, Blautia, Streptococcus, [Ruminococcus]_torques_group, Romboutsia, and [Eubacterium]_hallii_group were all significantly overrepresented [all linear discriminant analysis (LDA) scores (log10) > 3] in fecal samples from ccRCC group, whereas Prevotella, Lachnospira, Lachnoclostridium, and Roseburia were the most abundant microbiota in the HC group [LDA scores (log10) > 3]. To illustrate the predictive value of gut microbiota for ccRCC, we selected five genera positively associated with ccRCC from LEfSe analyses: Blautia, Streptococcus, [Ruminococcus]_torques_group, Romboutsia, and [Eubacterium]_hallii_group (Figure 3D). This “five-genera” analysis accurately predicted ccRCC as demonstrated by a receiver operating characteristic curve (AUC = 93.3%) and suggested predictive models based on genera could serve as biomarkers for ccRCC (Figure 3E).
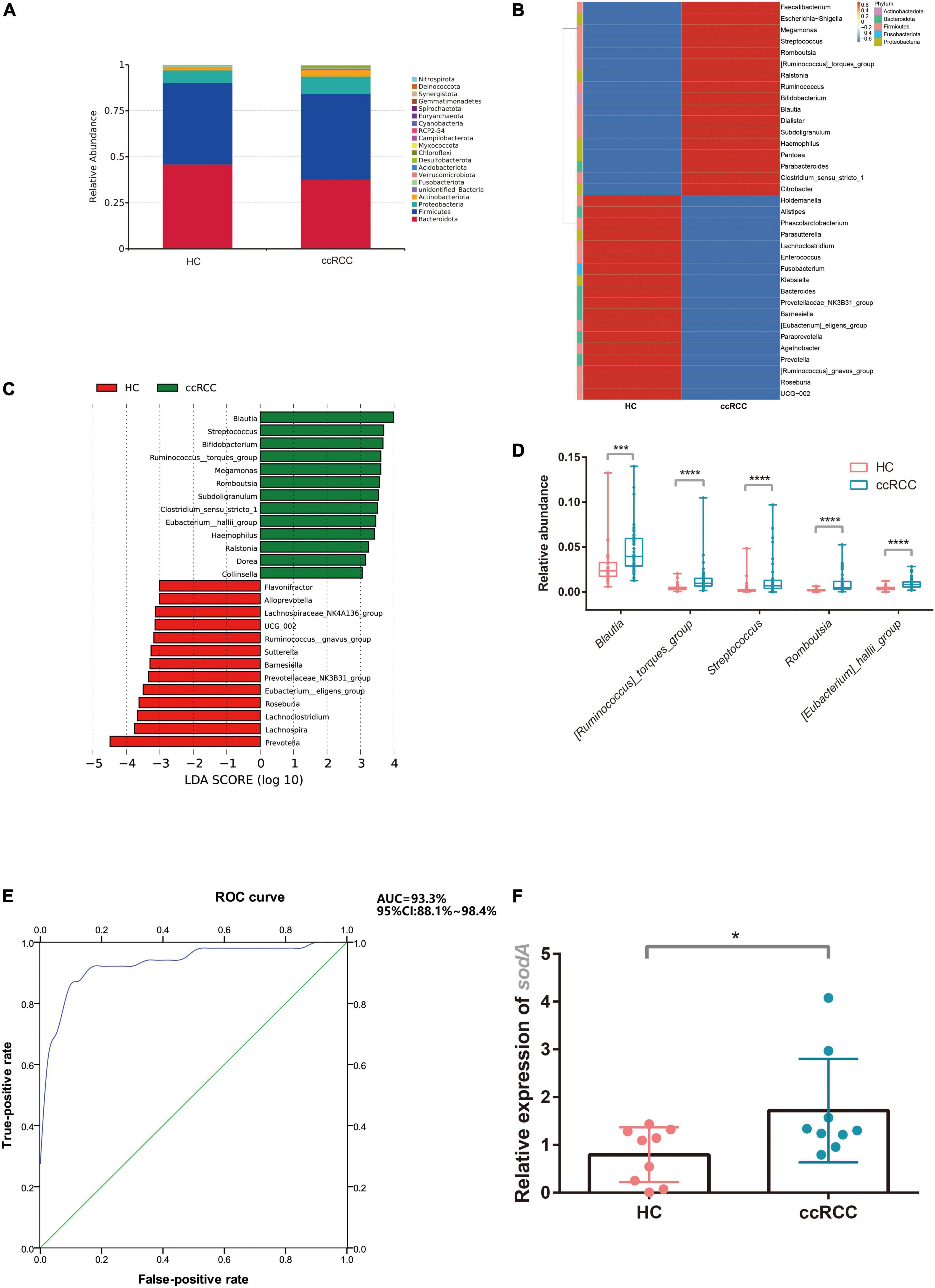
Figure 3. Characteristic gut microbiota in ccRCC and HC groups. The gut microbiota composition at phylum (A) and genus levels (B) between groups was analyzed. (C) LEfSe analysis indicated group-associated microbiota at the genus level. Bars on the right of zero represent microbiota enriched in the ccRCC group, whereas bars left of zero represent microbiota enriched in the HC group (LDA > 3). (D) Five representative genera “Blautia, Streptococcus, [Ruminococcus]_torques_group, Romboutsia, [Eubacterium]_hallii_group” were analyzed (E) to build a receiver operating characteristic curve of a “five-genera” model predicting RCC risk. (F) S. lutetiensis levels were determined using qPCR targeting sodA. Results are presented as the mean ± SD. Statistical evaluations were performed using the Kruskal–Wallis test followed by Dunn’s multiple comparisons test. *p < 0.05, ***p < 0.001, ****p < 0.0001. ccRCC, clear cell renal cell carcinoma; HC, healthy control; LEfSe, linear discriminant analysis effect size; LDA, linear discriminant analysis; PCoA, principal coordinates analysis; sodA, superoxide dismutase A; SD, standard deviation.
To test whether the “five-genera” model indeed contributed to ccRCC progression, we tried to isolate the bacteria from ccRCC fecal samples. Unfortunately, we failed to isolate other strains among the “five-genera” from ccRCC fecal samples except for S. lutetiensis. The abundance of S. lutetiensis was also higher in the ccRCC group when compared with the HC group by testing the expression of sodA from fecal samples (Figure 3F), we hypothesized that S. lutetiensis contributes to the progression of ccRCC. So S. lutetiensis was used for the following experiment.
Streptococcus lutetiensis Conditioned Medium Promotes Clear Cell Renal Cell Carcinoma Cell Proliferation, Migration, and Invasion
To determine if S. lutetiensis promoted ccRCC in vitro, the RCC cell lines, A498 and ACHN, were co-cultured with or without Sl.CM. BHI was used as a blank control. S. lutetiensis was cultured overnight in a BHI medium, bacteria were removed by centrifugation, and the supernatant was filtered through a 0.22-μm membrane to generate the Sl.CM, which was used to treat A498 and ACHN cells at 12.5% (vol/vol) for 6 h. An EdU assay was performed to determine the effect of Sl.CM on cell proliferation. The results demonstrated that Sl.CM increased A498 and ACHN cell proliferation. As shown in Figure 4A, the percentage of EdU-positive cells was significantly higher in the S. lutetiensis group (0.21 ± 0.02) when compared to BHI control (0.04 ± 0.02) (p < 0.001) in A498 cell line. A similar result could also be obtained in the ACHN cell line as the number of EdU-positive cells was higher in S. lutetiensis group (0.35 ± 0.13) when compared to the BHI control (0.20 ± 0.06) (p < 0.05). A wound healing assay was performed to determine the effect of Sl.CM on A498 and ACHN cell migration. The results demonstrated that Sl.CM increased A498 and ACHN cell migration. As shown in Figure 4B, the wound closure rate was higher in S. lutetiensis group (0.14 ± 0.07) when compared to BHI control (0.01 ± 0.01) (p < 0.05) in the A498 cell line. A similar result could also be obtained in the ACHN cell line as the wound closure rate was significantly higher in S. lutetiensis group (0.29 ± 0.08) when compared to the BHI control (0.08 ± 0.05) (p < 0.05). Transwell invasion assay was performed to determine the effect of Sl.CM on A498 and ACHN cell invasion. The results demonstrated that Sl.CM increased A498 and ACHN cell invasion. As shown in Figure 4C, the number of transmembrane cells was significantly higher in S. lutetiensis group (274.30 ± 11.85) when compared to BHI control (86.67 ± 15.37) (p < 0.0001) in the A498 cell line. A similar result could also be obtained in the ACHN cell line as the number of transmembrane cells was significantly higher in S. lutetiensis group (249.00 ± 11.53) when compared to BHI control (75.67 ± 6.03) (p < 0.0001).
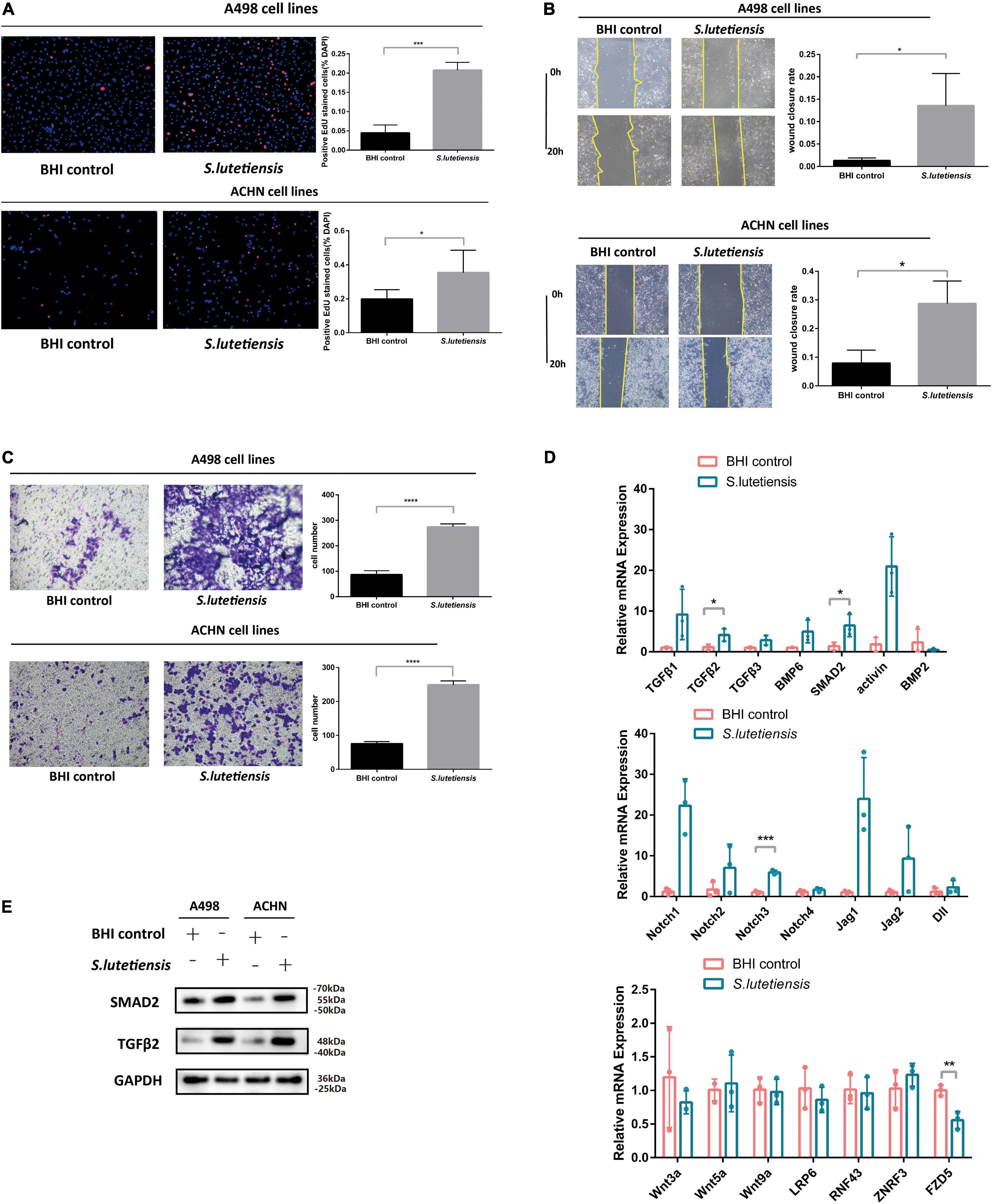
Figure 4. Streptococcus lutetiensis conditioned medium (Sl.CM) promotes A498 and ACHN cell proliferation, migration, and invasion through the TGF-β signaling pathway. (A) EdU assay showed that Sl.CM increased A498 and ACHN cell proliferation. (B) Wound healing assay showed that Sl.CM increased A498 and ACHN cell migration. (C) Transwell invasion assay showed that Sl.CM increased A498 and ACHN cell invasion. (D) qRT-PCR showed that mRNA expression of TGF-β2 and SMAD2 in TGF-β signaling pathway was increased in Sl.CM treated ACHN cells but mRNA expression of Wnt and Notch signaling pathway showed no obvious difference between the two groups. (E) Western blot showed that TGF-β2 and SMAD2 protein expression were increased in Sl.CM treated A498 and ACHN cells. Results are presented as the mean ± SD and statistical significance was determined using the unpaired t-test. *p < 0.05, **p < 0.01, ***p < 0.001, ****p < 0.0001. Sl.CM, S. lutetiensis conditioning medium; EdU, 5-ethynyl-2′-deoxyuridine; SD, standard deviation.
Streptococcus lutetiensis Conditioned Medium Promotes Clear Cell Renal Cell Carcinoma Progression Through TGF-β Signaling Pathway
These observations suggest S. lutetiensis promoted ccRCC development, but the underlying mechanisms were unclear. Previous studies reported that some Streptococcus species activated TGF-β signaling in various diseases, e.g., Streptococcus pneumoniae induced TGF-β1 expression during tympanosclerosis pathogenesis (Qiu et al., 2021), asthma inflammation (Schmit et al., 2020) and pulmonary infection (Peteranderl et al., 2017). So we theorized that S. lutetiensis promoted ccRCC via TGF-β signaling, to explore this possibility, the ACHN cell line was co-cultured with Sl.CM or BHI and the mRNA expression levels of the TGF-β signaling pathway were assessed by qRT-PCR. We also assessed the mRNA expression levels of Wnt and Notch signaling pathways. As shown in Figure 4D, S. lutetiensis increased relative expression levels of the TGF-β2 (4.11 ± 1.52) (p < 0.05) and SMAD2 (6.45 ± 2.73) (p < 0.05) genes in TGF-β signaling pathway while upregulating little markers in the Wnt and Notch signaling pathway. Then we investigated related protein expression levels in A498 and ACHN cell lines. As shown in Figure 4E, TGF-β2 and SMAD2 protein levels in Sl.CM-co cultured RCC cell lines were significantly increased when compared with BHI control, thus verifying our hypothesis.
Discussion
In this study, we investigated gut microbiota specific to ccRCC and successfully constructed a prediction model for disease risk based on five biomarkers with high AUC. We also showed that gut microbiota affected ccRCC progression. Using gut microbiota as a diagnostic tool for tumorigenesis has gained considerable traction in research. In China, a large clinical cohort study involving 419 fecal samples reported that gut microbiota such as Klebsiella and Haemophilus could be used as potential non-invasive tools for early HCC diagnosis (Ren et al., 2019). In colon cancer, 17 CRC-specific metabolites were identified, with polyamines such as cadaverine and putrescine strongly correlated with CRC (Yang et al., 2019). Surprisingly, in cancers distant from the gut, the gut microbiota also accurately predicted cancer. A predictive model of 13 OTU-based markers, including Veillonella and Ruminococcus, accurately predicted lung cancer (Zheng et al., 2020). Also, Bacteroides and Streptococcus in prostate cancer patients were more abundant, with PICRUSt data showing that B vitamin metabolic pathways were down-regulated in these patients, potentially indicating protective effects from prostate cancer risk (Liss et al., 2018), whereas in other work, Prevotella stercorea provided an alternative source of androgen and contributed to endocrine resistance in castration-resistant prostate cancer (Pernigoni et al., 2021).
In RCC, recent studies reported that the oral administration of commensal bacteria, such as Akkermansia muciniphila, was enriched in patients experiencing immunotherapy clinical benefits (Salgia et al., 2020) while Clostridium hathewayi was enriched in non-response groups and related to recent antibiotic-use in metastatic RCC patients. A randomized clinical trial involving metastatic RCC patients with TKI-related diarrhea showed that fecal material from healthy donors greatly relieved their symptoms, while A. muciniphila was shared between healthy donors and patients with improved outcomes after receiving feces from healthy donors (Ianiro et al., 2020).
From our study, Blautia, [Ruminococcus]_torques_group, Streptococcus, Romboutsia, and [Eubacterium]_hallii_group genera were enriched in the ccRCC group. Blautia (Deng et al., 2021), Romboutsia (Deng et al., 2021), and Streptococcus (Deng et al., 2021; Jiang et al., 2021) were reportedly enriched in esophageal cancer (Jiang et al., 2021). Streptococcus was also abundant in CRC (Yang et al., 2021). Streptococcus is a Gram-positive bacteria in humans and is mostly associated with urinary tract infection, sepsis, endocarditis, and CRC. Some studies have shown that Streptococcus induced inflammation and carcinogenesis by activating COX-2 which induced angiogenesis and suppressed apoptosis (Dalal et al., 2021), activating interleukin-8 (IL-8) which is the production of angiogenic factors (Dalal et al., 2021), or activating NF-κB which is a transcriptional factor involved in inflammation (Abdulamir et al., 2009). Streptococcus infection could activate the NLRP3 inflammasome and induce IL-1β secretion (Harder et al., 2009), which was previously shown to promote colon cancer (Wang et al., 2021). Streptococcus was also more abundant in prostate cancer, while PICRUSt data indicated that B vitamin metabolic pathways, which are protective against prostate cancer risk, were down-regulated in prostate cancer patients (Liss et al., 2018). Surprisingly, Streptococcus was more abundant in ccRCC tissue than in normal kidney tissue, which suggested an association with tumorigenesis (Liss et al., 2020). In our study, using Tax4Fun functional predictions, ABC transporter genes were more active in ccRCC. Several studies reported that the ABC transporters, ABCB1 (Watanabe et al., 2017) and ABCG2 (Watanabe et al., 2017; Reustle et al., 2018) were correlated with drug resistance in ccRCC, while ABCG2 (Low et al., 2016) was correlated with drug-induced toxicity. ABCB1 expression may also contribute to the side population cell isolation from the 769P cell line and serve as a mediator in the cancer stem cell pathogenesis of ccRCC (Huang et al., 2016). ABCB8 was also reported as a risk predictor for worse clinical outcomes in ccRCC (Blajan et al., 2021). Our data indicated that quorum sensing was more active in ccRCC. This process is key for microbial biofilm formation, which regulates bacterial active efflux pumps to effectively discharge drugs from systems, thereby promoting multidrug resistance (Chew et al., 2020). Quorum sensing signaling molecules induced the disorder of intestinal goblet cell structure and function, ultimately destroying the intestinal mucus barrier (Xiao et al., 2022). Similarly, cysteine and methionine metabolic functions were also more active in ccRCC. Cysteine promoted colon cancer growth in vitro and in vivo, while it conferred resistance to oxaliplatin and irinotecan chemotherapy (Wu et al., 2021). Conversely, pyruvate metabolism was inactive during ccRCC. Down-regulated pyruvate kinase activity contributes to aerobic glycolysis in ovarian cancer cells and disease progression (Sun et al., 2021).
Streptococcus lutetiensis is Gram-positive (Poyart et al., 2002) and produces several exotoxins (Chen et al., 2021) which may be pathogenic, e.g., diarrhea (Jin et al., 2013) or neonatal meningitis with empyema (Yu et al., 2021). In our study, S. lutetiensis promoted ccRCC via TGF-β signaling, whereas other studies suggested S. lutetiensis virulence genes were also associated with carcinogenesis. The endotoxin encoding genes, SpeG (encoding pyrogenic exotoxin G) and smeZ (encoding mitogenic exotoxin Z), were detected in S. lutetiensis (Chen et al., 2021). Exotoxins increased the levels of C-C motif chemokine ligand 2 (Emmer et al., 2008), which aided tumor-associated macrophages (TAM) and cancer progression (Liu et al., 2020). TAMs have previously been shown to promote the progression of ccRCC (Gu et al., 2021).
The limitation of 16S rRNA sequencing is that it can only determine microbiota composition at the genus level. More precise detection methods like culture or metagenomic sequencing can be applied to identify the exact strains. Furthermore, multi-center studies are necessary for validation. Our ultimate goal was to explore gut microbiota potentially affecting ccRCC pathogenesis. To that end, we developed a “five-genera” model that could detect ccRCC and suggested that S. lutetiensis may promote ccRCC progression via the TGF pathway.
Data Availability Statement
The datasets presented in this study can be found in online repositories. The names of the repository/repositories and accession number(s) can be found below: NCBI BioProject – PRJNA842560.
Ethics Statement
The studies involving human participants were reviewed and approved by the Renji Hospital Ethics Committee, Shanghai Jiao Tong University School of Medicine. The patients/participants provided their written informed consent to participate in this study. Written informed consent was obtained from the individual(s) for the publication of any potentially identifiable images or data included in this article.
Author Contributions
YC designed and finalized the study. YC, JM, and YD performed the experiments. NZ and ZY helped on samples collection and the bioinformatics analysis. YC drew the charts and wrote the manuscript which were revised by WZ, QL, and JZ. QL, WZ, and JZ supervised the project. WZ and JZ funded the project. All authors approved the final version submitted.
Funding
This study was supported by grants from National Natural Science Foundation of China (81972393 and 81972369).
Conflict of Interest
The authors declare that the research was conducted in the absence of any commercial or financial relationships that could be construed as a potential conflict of interest.
Publisher’s Note
All claims expressed in this article are solely those of the authors and do not necessarily represent those of their affiliated organizations, or those of the publisher, the editors and the reviewers. Any product that may be evaluated in this article, or claim that may be made by its manufacturer, is not guaranteed or endorsed by the publisher.
Supplementary Material
The Supplementary Material for this article can be found online at: https://www.frontiersin.org/articles/10.3389/fmicb.2022.913718/full#supplementary-material
Footnotes
References
Abdulamir, A. S., Hafidh, R. R., Mahdi, L. K., Al-jeboori, T., and Abubaker, F. (2009). Investigation into the controversial association of Streptococcus gallolyticus with colorectal cancer and adenoma. BMC Cancer 9:403. doi: 10.1186/1471-2407-9-403
Alber, J., El-Sayed, A., Lämmler, C., Hassan, A. A., Vossen, A., and Siebert, U. (2004). Determination of species-specific sequences of superoxide dismutase A encoding gene sodA and chaperonin 60 encoding gene cpn60 for identification and phylogenetic analysis of Streptococcus phocae. Vet. Microbiol. 101, 117–122. doi: 10.1016/j.vetmic.2004.03.018
Aßhauer, K. P., Wemheuer, B., Daniel, R., and Meinicke, P. (2015). Tax4Fun: predicting functional profiles from metagenomic 16S rRNA data. Bioinformatics 31, 2882–2884. doi: 10.1093/bioinformatics/btv287
Bailey, D. W., Attia, Z., Reinert, S., S Hulke, B., and Kane, N. C. (2022). Effective strategies for isolating DNA from members of Asteraceae with high concentrations of secondary metabolites. Biotechniques 72, 85–89. doi: 10.2144/btn-2021-0050
Blajan, I., Miersch, H., Schmidt, D., Kristiansen, G., Perner, S., Ritter, M., et al. (2021). Comprehensive analysis of the ATP-binding cassette subfamily B across renal cancers identifies ABCB8 overexpression in phenotypically aggressive clear cell renal cell carcinoma. Eur. Urol. Focus 7, 1121–1129. doi: 10.1016/j.euf.2020.09.007
Bokulich, N. A., Subramanian, S., Faith, J. J., Gevers, D., Gordon, J. I., Knight, R., et al. (2013). Quality-filtering vastly improves diversity estimates from Illumina amplicon sequencing. Nat. Methods 10, 57–59. doi: 10.1038/nmeth.2276
Boorjian, S. (2014). Commentary on “Effects on survival of BAP1 and PBRM1 mutations in sporadic clear-cell renal-cell carcinoma: a retrospective analysis with independent validation.” Kapur P, Peña-Llopis S, Christie A, Zhrebker L, Pavía-Jiménez A, Rathmell WK, Xie XJ, Brugarolas J. department of pathology, University of Texas Southwestern Medical Center, Dallas, TX. Lancet Oncol 2013; 14(2):159-67. [Epub 2013 Jan 16]. Urol. Oncol. 32, 934–935. doi: 10.1016/j.urolonc.2014.01.012
Caporaso, J. G., Kuczynski, J., Stombaugh, J., Bittinger, K., Bushman, F. D., Costello, E. K., et al. (2010). QIIME allows analysis of high-throughput community sequencing data. Nat. Methods 7, 335–336. doi: 10.1038/nmeth.f.303
Chen, P., Qiu, Y., Liu, G., Li, X., Cheng, J., Liu, K., et al. (2021). Characterization of Streptococcus lutetiensis isolated from clinical mastitis of dairy cows. J. Dairy Sci. 104, 702–714. doi: 10.3168/jds.2020-18347
Chew, S. S., Tan, L. T., Law, J. W., Pusparajah, P., Goh, B. H., Ab Mutalib, N. S., et al. (2020). Targeting gut microbial biofilms-A key to hinder colon carcinogenesis? Cancers (Basel) 12:2272. doi: 10.3390/cancers12082272
Dalal, N., Jalandra, R., Bayal, N., Yadav, A. K., Harshulika Sharma, M., et al. (2021). Gut microbiota-derived metabolites in CRC progression and causation. J. Cancer Res. Clin. Oncol. 147, 3141–3155. doi: 10.1007/s00432-021-03729-w
Denburg, M. R., Koepsell, K., Lee, J. J., Gerber, J., Bittinger, K., and Tasian, G. E. (2020). Perturbations of the gut microbiome and metabolome in children with calcium oxalate kidney stone disease. J. Am. Soc. Nephrol. 31, 1358–1369. doi: 10.1681/ASN.2019101131
Deng, Y., Tang, D., Hou, P., Shen, W., Li, H., Wang, T., et al. (2021). Dysbiosis of gut microbiota in patients with esophageal cancer. Microb. Pathog. 150:104709. doi: 10.1016/j.micpath.2020.104709
Derosa, L., Routy, B., Fidelle, M., Iebba, V., Alla, L., Pasolli, E., et al. (2020). Gut bacteria composition drives primary resistance to cancer immunotherapy in renal cell carcinoma patients. Eur. Urol. 78, 195–206. doi: 10.1016/j.eururo.2020.04.044
Edgar, R. C. (2004). MUSCLE: multiple sequence alignment with high accuracy and high throughput. Nucleic Acids Res. 32, 1792–1797. doi: 10.1093/nar/gkh340
Edgar, R. C. (2013). UPARSE: highly accurate OTU sequences from microbial amplicon reads. Nat. Methods 10, 996–998. doi: 10.1038/nmeth.2604
Edgar, R. C., Haas, B. J., Clemente, J. C., Quince, C., and Knight, R. (2011). UCHIME improves sensitivity and speed of chimera detection. Bioinformatics 27, 2194–2200. doi: 10.1093/bioinformatics/btr381
Emmer, A., Gerlach, K., Staege, M. S., and Kornhuber, M. E. (2008). Cerebral gene expression of superantigen encephalitis in the lewis rat induced by Staphylococcal enterotoxin a. Scand. J. Immunol. 67, 464–472. doi: 10.1111/j.1365-3083.2008.02094.x
Evenepoel, P., Poesen, R., and Meijers, B. (2017). The gut-kidney axis. Pediatr. Nephrol. 32, 2005–2014. doi: 10.1007/s00467-016-3527-x
Giordano, L., Mihaila, S. M., Eslami Amirabadi, H., and Masereeuw, R. (2021). Microphysiological systems to recapitulate the gut-kidney axis. Trends Biotechnol. 39, 811–823. doi: 10.1016/j.tibtech.2020.12.001
Gu, W., Gong, L., Wu, X., and Yao, X. (2021). Hypoxic TAM-derived exosomal miR-155-5p promotes RCC progression through HuR-dependent IGF1R/AKT/PI3K pathway. Cell Death Discov. 7:147. doi: 10.1038/s41420-021-00525-w
Haas, B. J., Gevers, D., Earl, A. M., Feldgarden, M., Ward, D. V., Giannoukos, G., et al. (2011). Chimeric 16S rRNA sequence formation and detection in Sanger and 454-pyrosequenced PCR amplicons. Genome Res. 21, 494–504. doi: 10.1101/gr.112730.110
Harder, J., Franchi, L., Muñoz-Planillo, R., Park, J. H., Reimer, T., and Núñez, G. (2009). Activation of the Nlrp3 inflammasome by Streptococcus pyogenes requires streptolysin O and NF-kappa B activation but proceeds independently of TLR signaling and P2X7 receptor. J. Immunol. 183, 5823–5829. doi: 10.4049/jimmunol.0900444
Huang, B., Fu, S. J., Fan, W. Z., Wang, Z. H., Chen, Z. B., Guo, S. J., et al. (2016). PKCε inhibits isolation and stemness of side population cells via the suppression of ABCB1 transporter and PI3K/Akt, MAPK/ERK signaling in renal cell carcinoma cell line 769P. Cancer Lett. 376, 148–154. doi: 10.1016/j.canlet.2016.03.041
Ianiro, G., Rossi, E., Thomas, A. M., Schinzari, G., Masucci, L., Quaranta, G., et al. (2020). Faecal microbiota transplantation for the treatment of diarrhoea induced by tyrosine-kinase inhibitors in patients with metastatic renal cell carcinoma. Nat. Commun. 11:4333. doi: 10.1038/s41467-020-18127-y
Jiang, Z., Wang, J., Shen, Z., Zhang, Z., and Wang, S. (2021). Characterization of esophageal microbiota in patients with esophagitis and esophageal squamous cell carcinoma. Front. Cell. Infect. Microbiol. 11:774330. doi: 10.3389/fcimb.2021.774330
Jin, D., Chen, C., Li, L., Lu, S., Li, Z., Zhou, Z., et al. (2013). Dynamics of fecal microbial communities in children with diarrhea of unknown etiology and genomic analysis of associated Streptococcus lutetiensis. BMC Microbiol. 13:141. doi: 10.1186/1471-2180-13-141
Kabaria, R., Klaassen, Z., and Terris, M. K. (2016). Renal cell carcinoma: links and risks. Int. J. Nephrol. Renovasc. Dis. 9, 45–52. doi: 10.2147/IJNRD.S75916
Kim, S. H., Park, W. S., Park, E. Y., Park, B., Joo, J., Joung, J. Y., et al. (2017). The prognostic value of BAP1, PBRM1, pS6, PTEN, TGase2, PD-L1, CA9, PSMA, and Ki-67 tissue markers in localized renal cell carcinoma: a retrospective study of tissue microarrays using immunohistochemistry. PLoS One 12:e0179610. doi: 10.1371/journal.pone.0179610
Liss, M. A., Chen, Y., Rodriguez, R., Pruthi, D., Johnson-Pais, T., Wang, H., et al. (2020). Microbiome within primary tumor tissue from renal cell carcinoma may be associated with PD-L1 expression of the venous tumor thrombus. Adv. Urol. 2020:9068068. doi: 10.1155/2020/9068068
Liss, M. A., White, J. R., Goros, M., Gelfond, J., Leach, R., Johnson-Pais, T., et al. (2018). Metabolic biosynthesis pathways identified from fecal microbiome associated with prostate cancer. Eur. Urol. 74, 575–582. doi: 10.1016/j.eururo.2018.06.033
Liu, Q., Song, J., Pan, Y., Shi, D., Yang, C., Wang, S., et al. (2020). Wnt5a/CaMKII/ERK/CCL2 axis is required for tumor-associated macrophages to promote colorectal cancer progression. Int. J. Biol. Sci. 16, 1023–1034. doi: 10.7150/ijbs.40535
Low, S. K., Fukunaga, K., Takahashi, A., Matsuda, K., Hongo, F., Nakanishi, H., et al. (2016). Association study of a functional variant on ABCG2 gene with sunitinib-induced severe adverse drug reaction. PLoS One 11:e0148177. doi: 10.1371/journal.pone.0148177
Magoč, T., and Salzberg, S. L. (2011). FLASH: fast length adjustment of short reads to improve genome assemblies. Bioinformatics 27, 2957–2963. doi: 10.1093/bioinformatics/btr507
Padala, S. A., Barsouk, A., Thandra, K. C., Saginala, K., Mohammed, A., Vakiti, A., et al. (2020). Epidemiology of renal cell carcinoma. World J. Oncol. 11, 79–87. doi: 10.14740/wjon1279
Pernigoni, N., Zagato, E., Calcinotto, A., Troiani, M., Mestre, R. P., Calì, B., et al. (2021). Commensal bacteria promote endocrine resistance in prostate cancer through androgen biosynthesis. Science 374, 216–224. doi: 10.1126/science.abf8403
Peteranderl, C., Sznajder, J. I., Herold, S., and Lecuona, E. (2017). Inflammatory responses regulating alveolar ion transport during pulmonary infections. Front. Immunol. 8:446. doi: 10.3389/fimmu.2017.00446
Poyart, C., Quesne, G., and Trieu-Cuot, P. (2002). Taxonomic dissection of the Streptococcus bovis group by analysis of manganese-dependent superoxide dismutase gene (sodA) sequences: reclassification of ‘Streptococcus infantarius subsp. coli’ as Streptococcus lutetiensis sp. nov. and of Streptococcus bovis biotype 11.2 as Streptococcus pasteurianus sp. nov. Int. J. Syst. Evol. Microbiol. 52(Pt 4), 1247–1255. doi: 10.1099/00207713-52-4-1247
Qiu, J., Wang, Y., Guo, W., Xu, L., Mou, Y., Cui, L., et al. (2021). Role of TGF-β1-mediated epithelial-mesenchymal transition in the pathogenesis of tympanosclerosis. Exp. Ther. Med. 21:6. doi: 10.3892/etm.2020.9438
Quast, C., Pruesse, E., Yilmaz, P., Gerken, J., Schweer, T., Yarza, P., et al. (2013). The SILVA ribosomal RNA gene database project: improved data processing and web-based tools. Nucleic Acids Res. 41, D590–D596. doi: 10.1093/nar/gks1219
Ren, Z., Li, A., Jiang, J., Zhou, L., Yu, Z., Lu, H., et al. (2019). Gut microbiome analysis as a tool towards targeted non-invasive biomarkers for early hepatocellular carcinoma. Gut 68, 1014–1023. doi: 10.1136/gutjnl-2017-315084
Reustle, A., Fisel, P., Renner, O., Büttner, F., Winter, S., Rausch, S., et al. (2018). Characterization of the breast cancer resistance protein (BCRP/ABCG2) in clear cell renal cell carcinoma. Int. J. Cancer 143, 3181–3193. doi: 10.1002/ijc.31741
Salgia, N. J., Bergerot, P. G., Maia, M. C., Dizman, N., Hsu, J., Gillece, J. D., et al. (2020). Stool microbiome profiling of patients with metastatic renal cell carcinoma receiving Anti-PD-1 immune checkpoint inhibitors. Eur. Urol. 78, 498–502. doi: 10.1016/j.eururo.2020.07.011
Schmit, T., Ghosh, S., Mathur, R. K., Barnhardt, T., Ambigapathy, G., Wu, M., et al. (2020). IL-6 deficiency exacerbates allergic asthma and abrogates the protective effect of allergic inflammation against Streptococcus pneumoniae pathogenesis. J. Immunol. 205, 469–479. doi: 10.4049/jimmunol.1900755
Sun, T., Liu, Z., Bi, F., and Yang, Q. (2021). Deubiquitinase PSMD14 promotes ovarian cancer progression by decreasing enzymatic activity of PKM2. Mol. Oncol. 15, 3639–3658. doi: 10.1002/1878-0261.13076
Wang, X., Jia, Y., Wen, L., Mu, W., Wu, X., Liu, T., et al. (2021). Porphyromonas gingivalis promotes colorectal carcinoma by activating the hematopoietic NLRP3 inflammasome. Cancer Res. 81, 2745–2759. doi: 10.1158/0008-5472.can-20-3827
Wang, X., Yang, S., Li, S., Zhao, L., Hao, Y., Qin, J., et al. (2020). Aberrant gut microbiota alters host metabolome and impacts renal failure in humans and rodents. Gut 69, 2131–2142. doi: 10.1136/gutjnl-2019-319766
Watanabe, A., Yamamoto, K., Ioroi, T., Hirata, S., Harada, K., Miyake, H., et al. (2017). Association of single nucleotide polymorphisms in STAT3, ABCB1, and ABCG2 with stomatitis in patients with metastatic renal cell carcinoma treated with sunitinib: a retrospective analysis in Japanese patients. Biol. Pharm. Bull. 40, 458–464. doi: 10.1248/bpb.b16-00875
Wu, J., Yeung, S. J., Liu, S., Qdaisat, A., Jiang, D., Liu, W., et al. (2021). Cyst(e)ine in nutrition formulation promotes colon cancer growth and chemoresistance by activating mTORC1 and scavenging ROS. Signal Transduct. Target. Ther. 6:188. doi: 10.1038/s41392-021-00581-9
Xiao, Y., Zou, H., Li, J., Song, T., Lv, W., Wang, W., et al. (2022). Impact of quorum sensing signaling molecules in gram-negative bacteria on host cells: current understanding and future perspectives. Gut Microbes 14:2039048. doi: 10.1080/19490976.2022.2039048
Yang, Y., Du, L., Shi, D., Kong, C., Liu, J., Liu, G., et al. (2021). Dysbiosis of human gut microbiome in young-onset colorectal cancer. Nat. Commun. 12:6757. doi: 10.1038/s41467-021-27112-y
Yang, Y., Misra, B. B., Liang, L., Bi, D., Weng, W., Wu, W., et al. (2019). Integrated microbiome and metabolome analysis reveals a novel interplay between commensal bacteria and metabolites in colorectal cancer. Theranostics 9, 4101–4114. doi: 10.7150/thno.35186
Yi, Y., Shen, L., Shi, W., Xia, F., Zhang, H., Wang, Y., et al. (2021). Gut microbiome components predict response to neoadjuvant chemoradiotherapy in patients with locally advanced rectal cancer: a prospective, longitudinal study. Clin. Cancer Res. 27, 1329–1340. doi: 10.1158/1078-0432.ccr-20-3445
Yu, A. T., Shapiro, K., Beneri, C. A., and Wilks-Gallo, L. S. (2021). Streptococcus lutetiensis neonatal meningitis with empyema. Access Microbiol. 3:000264. doi: 10.1099/acmi.0.000264
Keywords: gut microbiota, clear cell renal cell carcinoma, Streptococcus lutetiensis, proliferation, migration, invasion
Citation: Chen Y, Ma J, Dong Y, Yang Z, Zhao N, Liu Q, Zhai W and Zheng J (2022) Characteristics of Gut Microbiota in Patients With Clear Cell Renal Cell Carcinoma. Front. Microbiol. 13:913718. doi: 10.3389/fmicb.2022.913718
Received: 06 April 2022; Accepted: 08 June 2022;
Published: 04 July 2022.
Edited by:
Yang Zhang, Harbin Institute of Technology, ChinaReviewed by:
Alexander Koliada, Institute of Food Biotechnology and Genomics, National Academy of Sciences of Ukraine (NAN Ukraine), UkraineAttayeb Mohsen, National Institutes of Biomedical Innovation, Health and Nutrition, Japan
Copyright © 2022 Chen, Ma, Dong, Yang, Zhao, Liu, Zhai and Zheng. This is an open-access article distributed under the terms of the Creative Commons Attribution License (CC BY). The use, distribution or reproduction in other forums is permitted, provided the original author(s) and the copyright owner(s) are credited and that the original publication in this journal is cited, in accordance with accepted academic practice. No use, distribution or reproduction is permitted which does not comply with these terms.
*Correspondence: Qian Liu, qq2005011@163.com; Wei Zhai, jacky_zw2002@hotmail.com; Junhua Zheng, zhengjh0471@sina.com