- 1CAS Key Laboratory of Mountain Ecological Restoration and Bioresource Utilization and Ecological Restoration Biodiversity Conservation Key Laboratory of Sichuan Province, Chengdu Institute of Biology, Chengdu, China
- 2College of Life Sciences, Nanjing Normal University, Nanjing, China
- 3College of Life Sciences, University of Chinese Academy of Sciences, Beijing, China
- 4Mangkang Ecological Station, Tibet Ecological Safety Monitor Network, Chengdu, China
The molecular basis enabling the adaptation of animals to spatially heterogeneous environments is a critical clue for understanding the variation, formation, and maintenance of biodiversity in the context of global climate change. Mountain dragons (Agamidae: Diploderma) thrive in the Hengduan Mountain Region, a biodiversity hotspot and a typical spatially heterogeneous environment. Here, we compare the liver and muscle metabolome and gut microbiome of 11 geographical populations from three Diploderma species (D. iadinum, D. yulongsense, and D. vela) after 7 days acclimation in the same laboratory conditions. Amino acid metabolism, particularly the products of the glutathione cycle, accounted for major interspecies variations, implying its significance in genetic differentiation among mountain dragons. Notably, the cold-dwelling D. vela and D. yulongense populations tended to have higher glycerophosphate, glycerol-3-phosphocholine, and kinetin levels in their liver, higher carnosine levels in their muscle, and higher Lachnospiraceae levels in their gut. Phylogeny, net primary productivity (NPP), and the temperature had the highest explanation rate to the variations in muscle metabolome, liver metabolome, and gut microbiome, respectively, suggesting heterogeneity of biological systems in response to climatic variations. Therefore, we suggested that the organ heterogeneity in environmental responsiveness might be substantial for mountain dragons to thrive in complicated environments.
Introduction
Almost all animals live in spatially heterogeneous environments (Keller and Seehausen, 2012). Environmental heterogeneity can drive adaptive divergences between populations at both genetic and physiological levels (Rainey and Travisano, 1998; Valladares et al., 2014). These interpopulation variations play a fundamental role in maintaining a species’ genetic or functional diversity and response to climate change (Charmantier et al., 2008; Zhu et al., 2021c). Moreover, adaptive genetic divergence may result in ecological speciation if it causes some form of reproductive isolation (Schluter, 2000; Rundle and Nosil, 2005). Thus, the physiological strategies and underlying molecular basis of environmental adaptation are critical clues for understanding the formation, maintenance, and variation trends of biodiversity in the context of global climate change.
Reptiles have been suggested to be particularly sensitive to climate change due to their poor dispersal capacity (Urban et al., 2013b). These animals have already experienced extensive declines and extinctions worldwide (Sinervo et al., 2010), and climate change has contributed to these threats (Stuart Simon et al., 2004). Correlative climate envelope models even predict that climate change will cause the extinction of 11–49% of endemic reptiles (Thomas et al., 2004), and 20% of lizard species are expected to extinct by 2080 (Sinervo et al., 2010). However, phenotypic plasticity and genetic adaptation are expected to mitigate some of the negative biotic consequences of climate change (Holt, 1990; Urban et al., 2013a). Thus, good knowledge of an environment’s adaptive mechanisms is important for understanding the influences of climate change on these animals and also a precondition for scientific and accurate conservation measures. In this regard, species adapted to typically heterogeneous environments provide a unique opportunity to study how these animals respond to environmental variations. The Hengduan Mountain Region (HMR), located in the southeastern part of the Tibet Plateau, varies spatially in climatic factors (e.g., temperature and precipitation) (Xu et al., 2018), resulting in many different ecosystems (Myers et al., 2000; Lei et al., 2015). It harbors high reptile diversity, including more than ten Diploderma (Squamata: Sauria: Agamidae; mountain dragons) species, which are micro-endemic to the dry and hot valleys of HMR (Wang et al., 2020). Currently, the formation of Diploderma diversity in HMR has not been studied systematically, and vicariant isolation, ecological divergence, and the low migration capacity of lizards (within 10 km; Southwood and Avens, 2010) are all potential drivers. The distribution areas of the Diploderma species are narrow but highly heterogeneous in terms of spatial climatic factors (Zhu et al., 2021c). This makes the Diploderma species an ideal model for investigating environmental adaptation at a micro-geographic scale.
Comparative genetics and genomics are major approaches in the study of the mechanisms of environmental adaptation (Savolainen et al., 2013; Kubota et al., 2015). However, the accumulation of stochastic genetic changes in the genome constitutes an obstacle to the screening of environment-related variations, especially for interspecies studies. Additionally, genetic variations may not always explain adaptation processes intuitively due to the limited understanding of their cellular functions (Chang et al., 2020), especially when adaptive traits are determined by multiple genetic loci or mutations are located in non-coding regions. Moreover, not all environmental adaptive traits are caused by changes in DNA sequences; for example, epigenetics, in response to external or environmental factors, can also shape cellular and physiological phenotypic traits by changing cellular gene expression patterns (Bird, 2007; Vogt, 2017). Despite the variability of genetic materials and the complexity of genetic determination, the expression of genetic information in phenotypic traits always relies on the qualitative and quantitative variations in a set of cellular chemical molecules—metabolites, which are the effectors of cellular regulation networks and the molecular basis of phenotypes (Nicholson and Lindon, 2008; Johnson et al., 2016). The whole set of metabolites in a cell, tissue, or organisms is called metabolome, which is at the frontline of the interactions between organisms and the environment (Bundy et al., 2009). Unlike the sequence mutations of genes, the chemical structures of primary metabolites are not easily varied with phylogeny, and species with close phylogenetic relationships likely share the same set of primary metabolites. This facilitates convenient comparisons on environment-related biological constructions and physiological functions between species or populations in the absence of whole-genome data. For example, comparative metabolomics revealed the critical role of metabolic switch in substrates for the thermal adaptation of a Plateau dwelling insect (Zhu et al., 2016; Zhu et al., 2019a). And combined comparative transcriptomic and metabolomic analysis illuminated the involvement of fatty acid metabolism in the highland adaptation across altitudinal songbirds (Xiong et al., 2021). Accordingly, comparative metabolomics provides an alternative approach for studying the adaptation of animals to spatially heterogeneous environments (Shi et al., 2015).
Additionally, the gut microbiome is considered to be the second genome of animals (Zhu et al., 2010). The host genome is highly conserved, and genetic changes within it occur slowly, whereas the gene pool of microbiota is dynamic and can change rapidly in response to the environment by increasing or reducing the abundance of particular microbes, by acquisition of novel microbes, by horizontal gene transfer, and by mutation (Rosenberg and Zilber-Rosenberg, 2018). Increasing numbers of studies have evidenced the contribution of the commensal microbiome to host environmental adaptation (Chevalier et al., 2015; Zhang et al., 2016); a disturbance in the gut microbiome can also lead to animal maladaptation to climate change (Greenspan et al., 2020). For example, gut microbiota promote cold adaptation of Brandt’s voles by increasing host thermogenesis through the activation of cAMP–PKA–pCREB signaling (Bo et al., 2019). Seasonal shifts in gut microbiota composition is in favor of utilization of nitrogen and energy in yaks at harsh cold winter, implying essential role of symbiotic microbiota in high altitude adaptation of animals (Guo et al., 2021). Thus, variations in the microbiome are important aspects that should be considered in the environmental adaptation of animals.
In this study, environment-related variations in organ metabolism (i.e., liver and hindlimb muscle) and gut microbial community composition were studied in three Diploderma species (D. iadinum, D. yulongense, and D. vela). D. yulongense and D. vela are phylogenetically closer to each other than to D. iadinum (Figure 1A; Wang et al., 2020), while D. iadinum and D. vela are spatially closer to each other (the Lancang River Valley) than to D. yulongense (the Jinsha River Valley) (Figure 1B). The associations between biological traits and climatic factors (e.g., temperature and precipitation) were analyzed at a population level (across species and within species). We raise three scientific questions regarding the contributions of phylogeny and climatic factors to animal metabolic and microbiome variations: (1) What factor is more significant, and whether or not there is any organ heterogeneity? (2) do the metabolome and microbiome vary with climatic factors convergently between species? and (3) which are the biological functions of the environment-related variations and their implications in environmental adaptation?
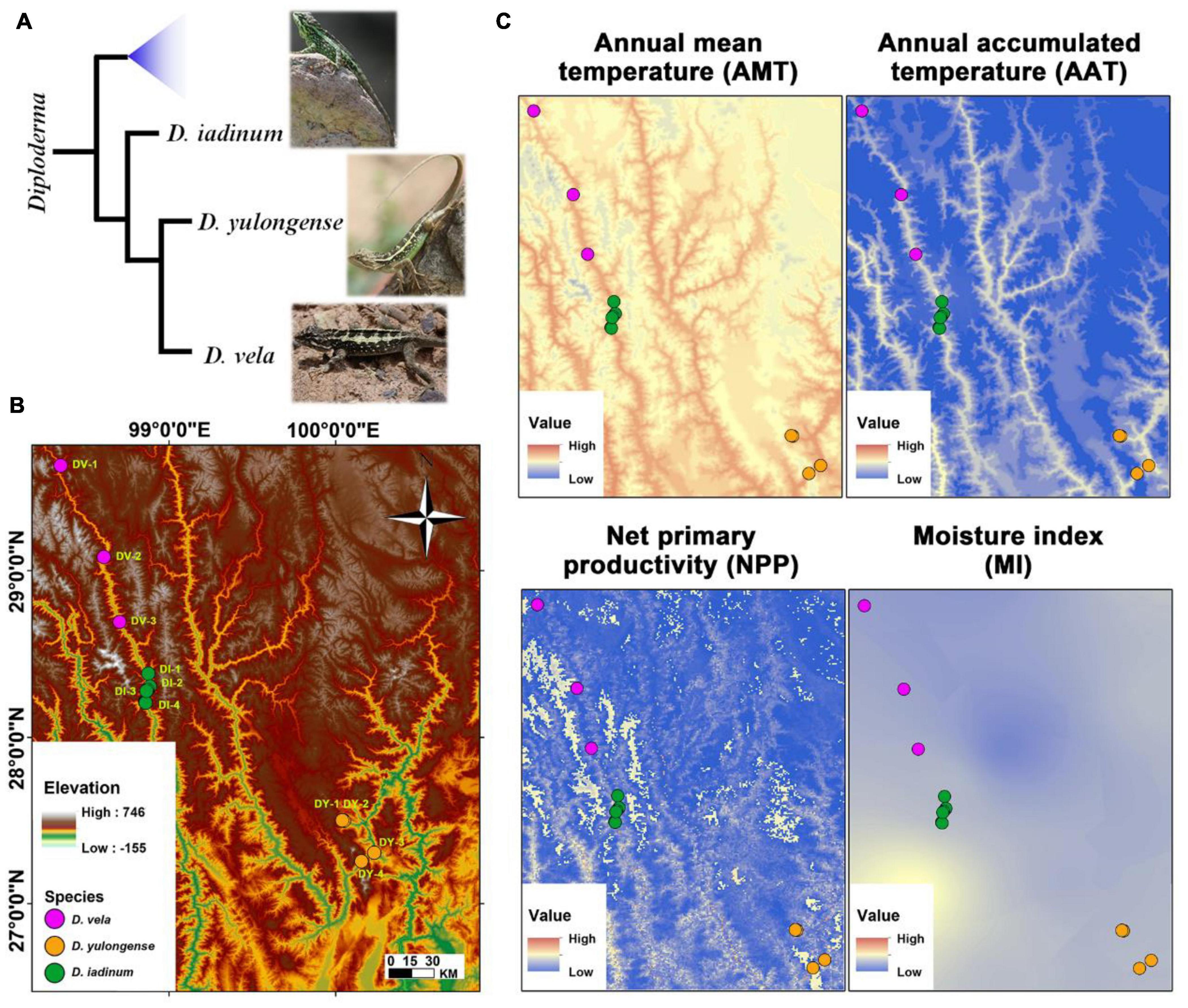
Figure 1. Animal phylogeny, study area, and significant climatic factors. (A) The phylogenic relationships of Diploderma species (D. iadinum, D. yulongense, and D. vela) (Wang et al., 2020). (B) Study area and sample collection sites. (C) Maps illustrating the major climatic factors. The white area in the map of net primary productivity (NPP) denotes that data are unavailable.
Materials and Methods
Habitats and Animals
The D. vela (n = 15) and D. iadinum individuals (n = 14) and D. yulongense individuals (n = 17) were sampled from the dry and hot valley of the Langcang River and the Jinsha River, respectively, in July 2020 (Figure 1B and Supplementary Table 1). The sample information is detailed in Supplementary Table 1. As the study involved invasive experiments, only males were collected. Considering that the animal metabolome and gut microbiome are likely variable to their instant physiological status (e.g., feeding status) and environmental conditions (e.g., the real-time temperature and moisture at the time of collection), the gut content and tissues were not sampled immediately after collected. Instead, all the individuals were acclimated to the same laboratorial condition (24 ± 1°C, L: D = 12: 12) for 7 days before sample collection to diminish the random variations. Our goal was to reveal the inter-populational divergences in animal metabolome and gut microbiome that were potentially associated with phylogeny and climate. Such an acclimation procedure could ensure the authenticity of the differences between geographical populations, despite some information might be lost during this process. During acclimation, each individual was placed in a 29 × 18 × 10 cm plastic container and fed with mealworm (Tenebrio molitor larvae) and tap water daily. The feeding behavior was confirmed by daily observation. Following euthanasia with ether, the liver, hind limb muscle, and gut mucosa (from the intestine to the rectum, pooled) were collected and stored at –80°C. Animal procedures were approved by the Animal Care and Use Committee of the Chengdu Institute of Biology, Chinese Academy of Sciences (permit number: 2020-AR-JJP-01).
Environmental factors (i.e., annual mean temperature/AMT, annual precipitation/AP, annual accumulated temperature (> 10°C)/AAT, moisture index/MI, and net primary productivity/NPP) were extracted from Resource and Environment Science and Data Center.1 This information is detailed in Supplementary Table 1. The gut microbiome and tissue metabolomics data of D. vela have been published previously (Zhu et al., 2021c).
Metabolic Profiling
After grinding in liquid nitrogen, 50 mg tissue powder was transferred into 1.5 ml Eppendorf tubes with 800 μL precooled methanol: acetonitrile = 1:1 (v/v), followed by ultrasonication for 30 min × 2 and incubation at –20°C for 1 h. After centrifugation at 16,000 g for 20 min (4°C), supernates were transferred into new tubes and freeze-dried. Samples were dissolved in 100 μL acetonitrile: water (1: 1, v/v). After centrifugation at 14,000 g for 15 min (4°C), the supernates were ready for analysis. Extracted supernatants were analyzed by LC (1,290 Infinity LC, Agilent) coupled with quadrupole-time-of-flight mass spectrometry (Triple TOF 5,600 +, AB SCIEX). The details in the metabolic profiling followed the methods described by Zhu et al. (2021c). Metabolite data were processed using XCMS software2 and Microsoft Excel (Microsoft, Redmond, WA, United States). Data of impurity peaks from column bleeds were excluded. Metabolites were identified by a combination of molecular weight comparison (molecular ion peak) and MS/MS spectrum comparison to a standard library. The relative abundances/concentrations of metabolites were presented as the ion intensities of their molecular ion peaks.
16S rRNA Gene-Based (Full Length) Microbiome Analyses
The PowerSoil® DNA Isolation kit (MO BIO Laboratories, Solana Beach, CA, United States) was used to extract DNA from the samples at room temperature according to the manufacturer’s protocol. A DNA extraction (blank) control was included during DNA isolation. The integrity of the nucleic acids was determined visually by electrophoresis on a 1.0% agarose gel containing ethidium bromide. The concentration and purity of each DNA extraction was determined using a Qubit dsDNA HS Assay Kit (Life Technologies, Carlsbad, CA, United States). The whole region of the 16S rRNA gene was amplified with 27F (5 = –AGRGTTTGATYNTGGCTCAG-3 =) and 1492R (5 = –TASGGHTACCTTGTTASGACTT-3 =) primers, following the method described by Zhu et al. (2021c). A DNA extraction (blank) control was also included during PCR reaction. We used the following PCR thermocycling conditions: 95°C for 5 min, 30 cycles of 95°C for 30 s, 50°C for 30 s, and 72°C for 60 s, with a final extension step at 72°C for 7 min. The products were purified with MagicPure Size Slection DNA Beads (TransGen Biotech, Beijing, China). High-throughput sequencing was performed using the PacBio platform. Sequencing (including the blank control) was performed by Biomarker Technologies Corporation (Beijing, China). The optimized circular consensus sequences (CCS) were obtained after filtering with the threshold of minPasses ≥ 5, minPredictedAccuracy ≥ 0.9, and length between 1,200 and 1,650 bp (lima v1.7.0 and cutadapt 1.9.1). Amplicon sequence variants (ASVs) were obtained after denoising with dada2 (Callahan et al., 2016). Annotation was conducted by querying against SILVA 132 (Quast et al., 2013), and the taxon summary was shown with QIIME2 2020.6 pipeline (Bolyen et al., 2019). The alpha-diversity (e.g., ACE and Shannon index) was calculated in QIIME 2. The dissimilarity matrices (e.g., unweighted and weighted UniFrac distances) were produced by QIIME2 pipeline.
Statistical Analyses
The influences of phylogeny (presented as genetic distances; Wang et al., 2020) and climatic factors (AMT, ATT, AP, NPP, and MI) on organ metabolome and gut microbiome were analyzed by PERMANOVA (adonis function in the Vegan package) based on R platform (Dixon, 2003). These analyses set metabolites or bacterial taxa abundances as dependent variables, and genetic distances and climatic factors were selected as independent factors. In detail, the beta-diversity of organ metabolomes was presented as Bray-Curtis distance, and the beta-diversity of gut microbiome was presented as Binary Jaccard, Bray-Curtis, Unweighted UniFrac, and Weighted UniFrac distances. The climatic factors and phylogeny (shown as the mean genetic distances to other species) were independent factors. Type I sum of square was used, as our main target was to screen the factors that had the most significant explanation rate to the variations. For each analysis, e.g., influences of phylogeny and climatic factors on beta-diversity of gut microbiota based on Bray-Curtis distances, the model was built by adding independent factors one by one. The sequences of independent factors were determined by ensuring that the factor added at each round could offer the most significant improvement on the total explanation of the total variations of dependent factors. The significance of each independent factors to the model was checked at threshold of p < 0.05. This method could avoid the interference of variable autocorrelation on models and reflect the importance order of factors.
Metabolites and bacterial taxa varied with phylogeny or climatic factors were screened. The metabolites or bacteria associated with phylogeny met the threshold of significant difference between any two species (at p < 0.05, Mann–Whitney U-test). Pearson correlations and Spearman correlations was used simultaneously to screen the metabolites and gut bacteria associated with climatic factors. Valid pairwise correlations met the threshold of q < 0.05 or q < 0.01 (Pearson correlation and BH correction) and p < 0.05 (Spearman correlation). The potential bacterial functions and phenotypes were predicted with PICRUSt2 (Douglas et al., 2020) and BugBase (Ward et al., 2017). However, PiCRUST was developed for human microbiome function and should certainly be used with caution for host groups outside the Mammalia. ANCOVA was conducted to analyze the variations in gut bacterial alpha-diversity and bacterial functions. The intraspecies differences in organ metabolome and gut microbiome between populations were analyzed by PERMANOVA (adonis function in the Vegan package). Principal coordinate analyses (PCoA, based on dissimilarity matrices) were used to visualize the dissimilarity of beta-diversity. Metabolite enrichment analyses were conducted using MetaboAnalyst 5.0.3 The Spearman correlation coefficients were calculated for each metabolite–bacteria pair. To ensure the reliability of the results, the coefficients were calculated across all the samples from different species, as well as limited for the samples from the same species. Valid metabolite–bacteria correlations should meet p < 0.001 across samples and p < 0.01 at least in two species. Correlation networks were constructed on Cytoscape 3.5.0. Other graphs were drawn using Graphpad prism 5 and ggplot2, an R package (Wickham, 2009).
Results
Environmental Heterogeneity of the Diploderma Distribution Range
The climatic factors (i.e., AMT, AAT, NPP, AP, and MI) vary spatially across the distribution range of Diploderma species (Figure 1C and Supplementary Table 1). The AMT and AAT share similar variation pattern, which is different from that of NPP and AP (Supplementary Figure 1). The collection sites for D. vela tended to have lower temperature, MI, and NPP than that of the other two species, and the intraspecies variations in temperature is prominent for D. vela and D. yulongense.
Variations in Organ Metabolome Across Species
D. iadinum, D. yulongense, and D. vela differed significantly in their liver and hindlimb metabolomes (Supplementary Table 2). However, for the liver metabolome, D. yulongense was more similar to D. iadinum than to D. vela (Supplementary Figure 2), despite D. yulongense and D. vela being more adjacent in phylogeny. In fact, while phylogeny contributed significantly to variations in liver metabolome (R2 = 0.072, p = 0.0025), NPP explained the variations better than phylogeny (R2 = 0.126, p = 0.0001) (PERMANOVA; Table 1 and Figure 2A). The similarity between species was consistent with their genetic distances (Supplementary Figure 2). Additionally, phylogeny had the highest explanation rate (R2 = 0.175, p = 0.0001) regarding the variations in muscle metabolome (PERMANOVA, Table 1 and Figure 2A). The other significant contributors included AAT and AP.
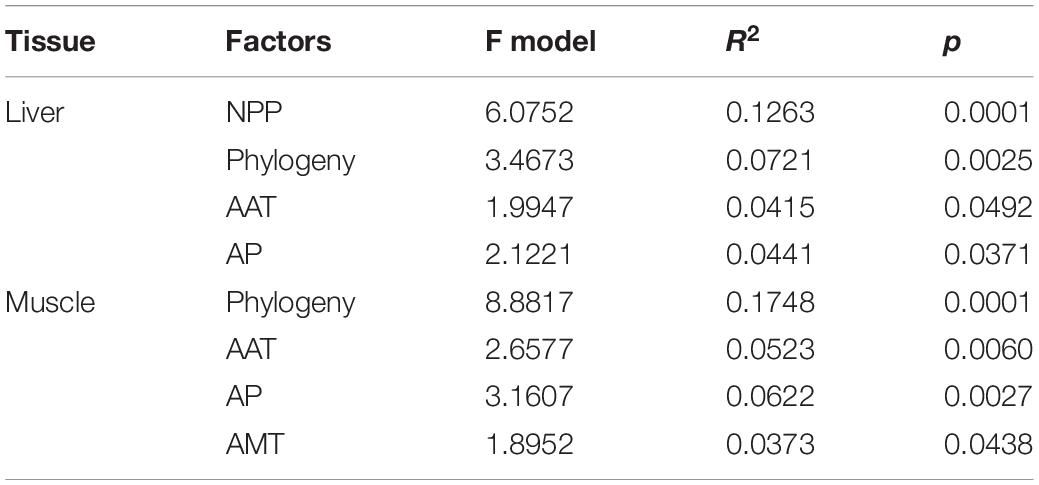
Table 1. The permutation ANOVAs on liver and muscle metabolomes (Bray-Curtis distance, permutations = 9,999) for the best models.
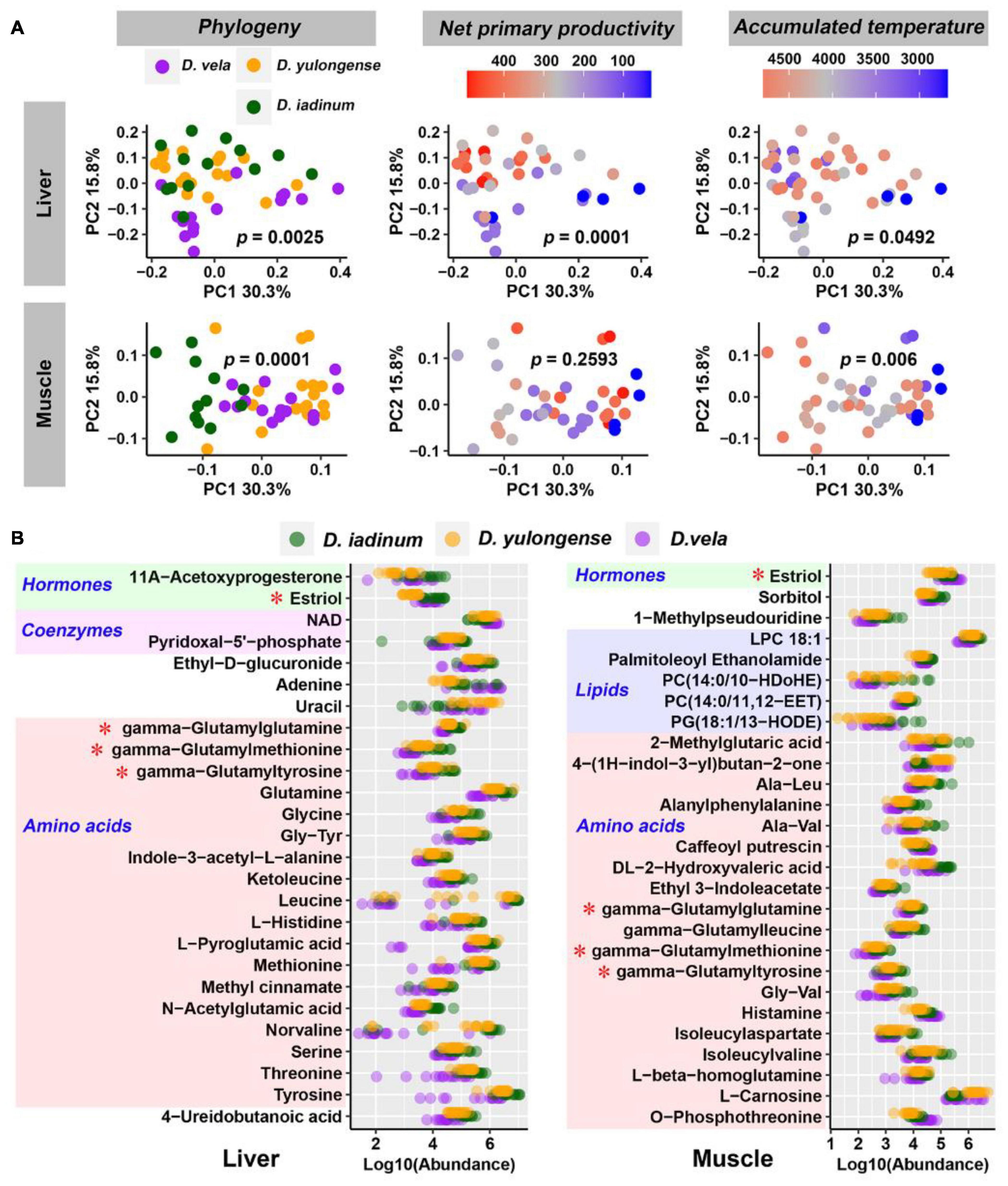
Figure 2. Influences of host phylogeny and climatic factors on the liver and muscle metabolic profiles. (A) PCoA scatter plots presenting the significance of phylogeny and climatic factors on variations in organ metabolome. The p-values of PERMANOVA were labeled. (B) Metabolites varied in abundance between any two Diploderma species (p < 0.05, Mann–Whitney U-test). Red asterisks denote the metabolites shared by the liver and muscle.
Most phylogeny-associated metabolites did not overlap with those associated with climatic factors (Supplementary Figure 3A). In both liver and muscle, phylogeny-associated metabolites were primarily amino acids and their derivates, most of which shared similar variation patterns across species (metabolite abundance: D. iadinum > D. yulongense > D. vela) (Figure 2B). Functional enrichment analyses against the KEGG database highlighted the potential involvement of hepatic purine metabolism, glycine and serine metabolism, ammonia recycling, and methionine metabolism in genetic differentiation of Diploderma species (Supplementary Figure 3B). Notably, three gamma-glutamyl dipeptides (i.e., gamma-glutamylglutamine, gamma-glutamylmethionine, and gamma-glutamyltyrosine) were shared by the liver and muscle.
NPP and AAT were the primary climatic factors associated with the variation in the liver metabolome. Hepatic metabolites showing strong correlations (q < 0.01, Pearson correlation; p < 0.05, Spearman correlation) with AAT included glycerol-3-phosphocholine, glycerophosphate, kinetin, and fructose (Figure 3A). Their levels were higher in individuals that inhabited cooler environments (Figure 3B). Compared to other climatic factors, there were more metabolites associated with NPP in the liver (Supplementary Figure 3A); this finding is consistent with the high explanation rate of NPP for liver metabolome. These metabolites highlighted metabolism, nicotinamide metabolism, ammonia recycling, urea cycle, and numerous amino acid metabolisms (Supplementary Figure 3B). The abundance of nicotinamide, NAD, and AMP, which participated in the same metabolic reaction (interconversion between nicotinamide and NAD), showed correlations with NPP level (Figure 3A). Lower NPP was associated with higher NAD and lower nicotinamide in the liver and vice versa (Figure 3C).
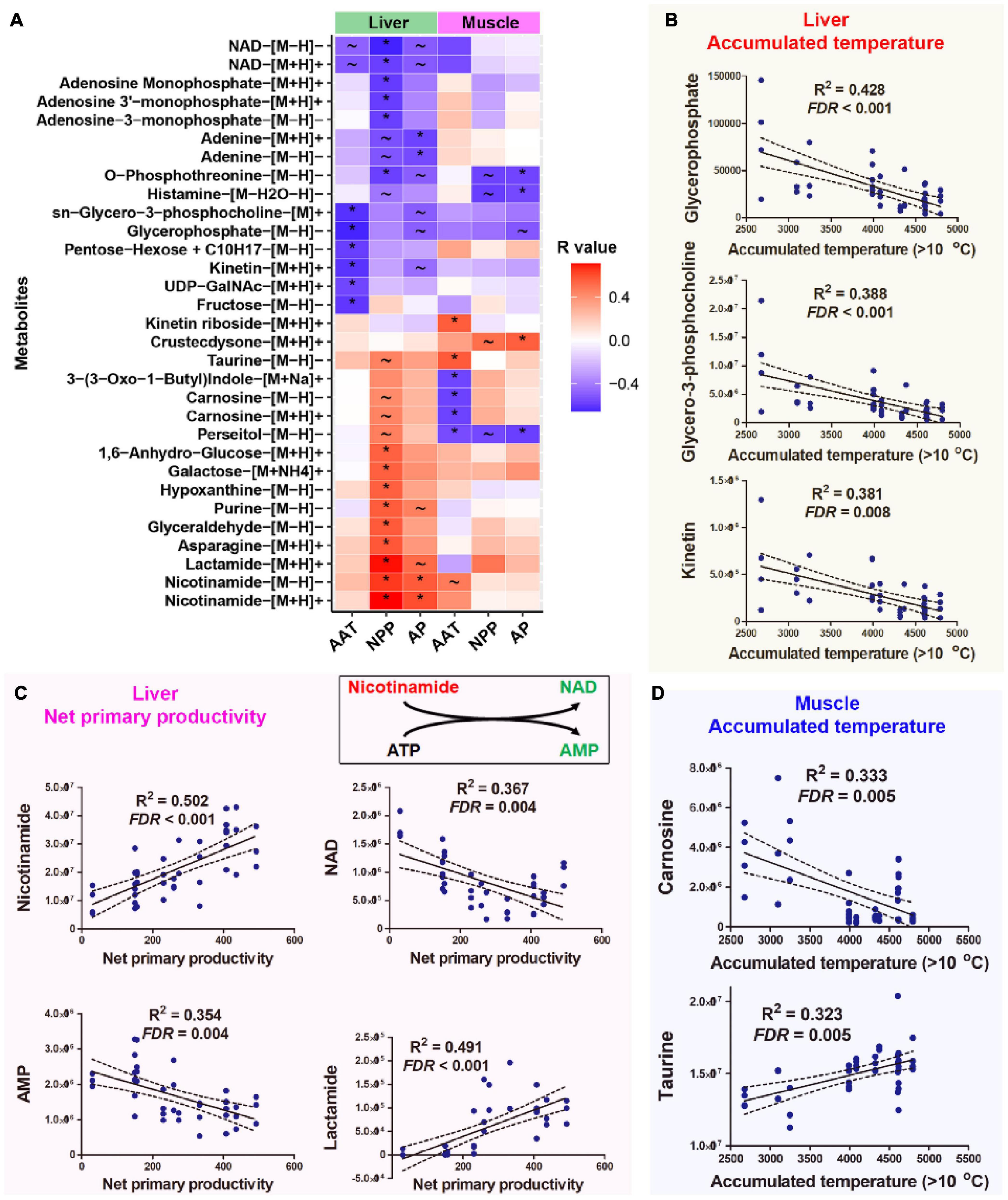
Figure 3. Host metabolites associated with climatic factors. (A) Heatmap presenting the correlations (R-value) between host metabolites and climatic factors. Climatic factors were limited to those contributing significantly to the variations in organ metabolome (p < 0.05, PERMANOVA). The metabolites presented were significantly correlated with (q < 0.01, Pearson correlation; p < 0.05, Spearman correlation) at least one climatic factor. *q < 0.01 for Pearson correlation and p < 0.05 for Spearman correlation; ∼q < 0.05 for Pearson correlation and p < 0.05 for Spearman correlation. (B) Correlations between AAT and liver metabolites. (C) Correlations between NPP and liver metabolites. (D) Correlations between AAT and muscle metabolites. The abundances of metabolites were presented as peak area, which had no unit.
AAT and AP were the major climatic factors associated with variations in muscle metabolome. Enrichment analysis highlighted the potential association between muscle histamine metabolism oxidation of branched-chain fatty acids and environmental AAT (Supplementary Figure 3B). Notably, muscle carnosine and perseitol showed a strong negative correlation with environmental AAT, while taurine and kinetin riboside positively correlated with AAT (Figure 3D). Muscle metabolites associating with AP included persecutor, O-phosphothreonine, and histamine (Figure 3A). Only a few metabolites correlated with environmental AAT and NPP simultaneously (Supplementary Figure 4).
Variations in Gut Microbiome Across Species
Proteobacteria, Firmicutes, and Bacteroidetes dominated the gut microbiome of Diploderma species (Figure 4A), and Enterobacteriaceae, Bacteroidaceae, and Lachnospiraceae were their most abundant bacterial families (Figure 4B). Neither species nor climatic factors had significant influences on the alpha-diversity of the gut microbiota (ANCOVA, Supplementary Table 3). The interspecies similarity of bacterial community structure was not consistent with the genetic distances (unweighted UniFrac distance and weighted UniFrac distance, Figure 4C). When bacterial phylogenetic relations were not considered (Binary Jaccard and Bray-Curtis distances), phylogeny was the primary contributor to the variation in gut microbiota and environmental AMT, NPP, and MI significant contributors (PERMANOVA, Table 2 and Figure 4D). When bacterial phylogenetic relations were considered, phylogeny still had the highest explanation rate (R2 = 0.122) to the gut microbiota community if ASV abundance was ignored (unweighted UniFrac distance). However, when ASV abundance was taken into consideration (weighted UniFrac distance), AMT was the only significant contributor (R2 = 0.125) to the variation in the gut microbiota community (Table 2 and Figure 4D). Additionally, interspecies differences no longer existed (Supplementary Table 2). No bacterial groups or ASVs showed significant variations between species (Kruskal–Wallis test at the threshold of p < 0.05). Instead, many bacterial groups, including Lachnospiraceae, Desulfovibrionaceae, and Veillonellaceae families, negatively correlated with environmental AMT, while Proteobacteria and Gammaproteobacteria positively correlated with it (Figures 5A,B). Notably, Intestinimonas butyriciproducens negatively correlated with environmental AMT, NPP, and MI (q < 0.05 in Pearson correlation and p < 0.05 in Spearman correlation, Figure 5C). There were no significant associations between any environmental factors and bacterial functions predicted by COG4 and KEGG databases.5 However, environmental MI was positively correlated with some bacterial phenotypes (i.e., potentially pathogenic, contains mobile elements, and facultative anaerobic) predicted by BugBase (Supplementary Figure 5).
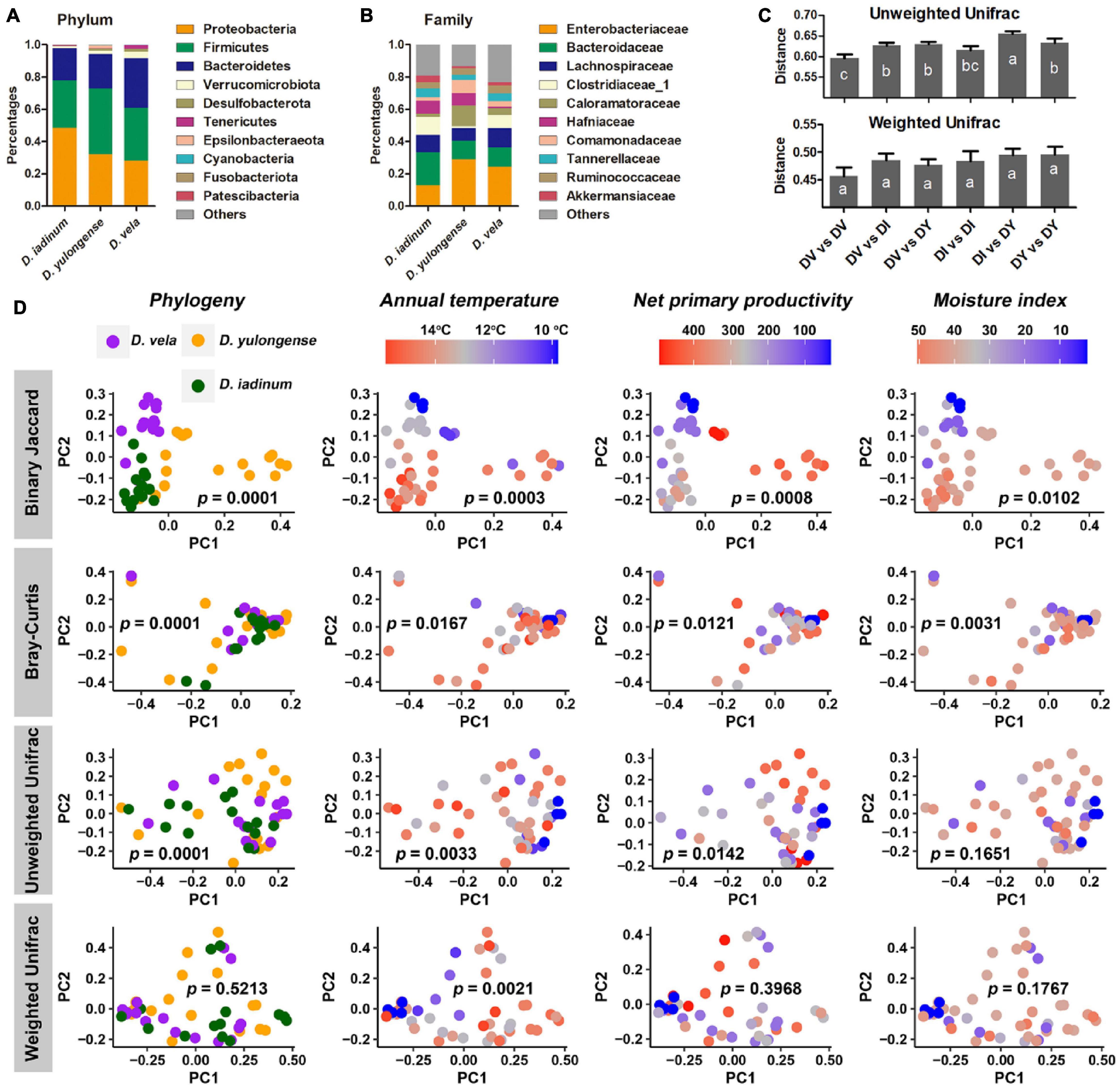
Figure 4. Influences of host phylogeny and climatic factors on the gut bacterial community structure of mountain dragons. (A,B) Composition of the microbiome at phylum (A) and family (B) levels. (C) Interspecies distances of the gut microbiome. (D) PCoA scatter plots presenting the significance of phylogeny and climatic factors on variations in the gut microbiome (interpopulation analyses across species). The p-values of PERMANOVA were labeled.
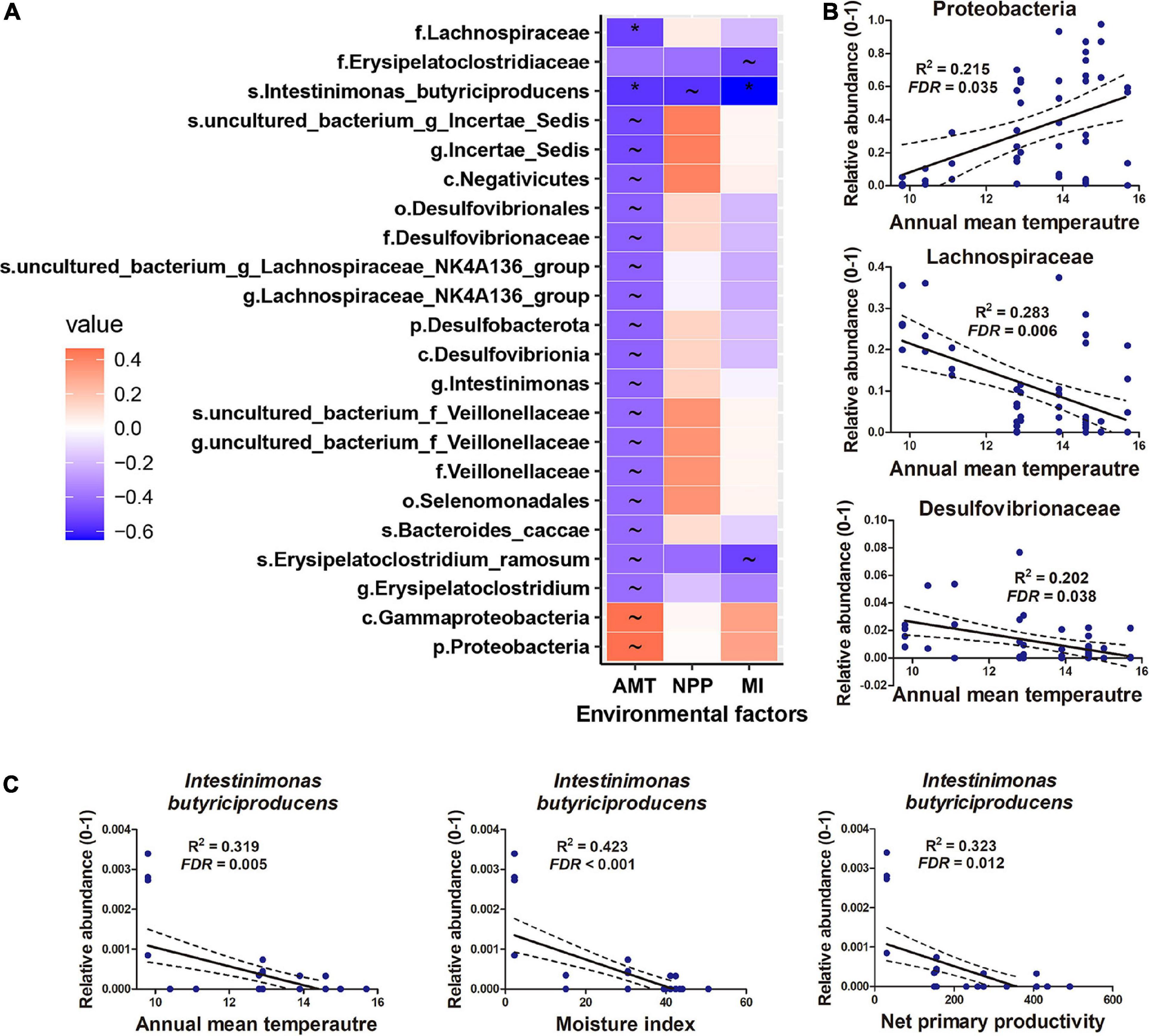
Figure 5. Gut microbiomes associated with climatic factors. (A) Heatmap presenting the correlations (R-value) between gut microbes and climatic factors. Climatic factors were limited to those contributing significantly to the variations in the gut microbiome (p < 0.05, PERMANOVA). *q < 0.01 for Pearson correlation and p < 0.05 for Spearman correlation; ∼q < 0.05 for Pearson correlation and p < 0.05 for Spearman correlation. (B,C) Correlations between microbes and environmental factors.
Intraspecies Variations Associated With Thermal Adaptation
For both D. vela and D. yulongense, there are populations (DV1, DY1, and DY2) inhabiting environments with more than 2°C lower in AMT than other populations from the same species (Figure 6A and Supplementary Figure 6). These populations were classified to be cold-dwelling populations (AMT < 11°C), while the other populations of these two species were classified as warm-dwelling populations (AMT > 12°C). Pairwise distances were calculated between cold-dwelling and warm-dwelling populations of these two species (detailed in Figures 6B,C). For liver metabolome, the distance between two cold-dwelling populations was longer than that between the two warm-dwelling populations (Figure 6B). A topological network suggested that the orientations from warm- to cold-dwelling populations were contradictory between these two species (Figure 6C). The distance between the two cold-dwelling populations was maintained for muscle metabolome compared to that between warm-dwelling populations (Figure 6B). The topological network suggested a paralleled variation orientation of cold adaptation between these two species. The weighted UniFrac distance between two cold-dwelling populations was the shortest (Figure 6B). Its topological network indicated a convergence of gut microbiota in cold-dwelling populations (Figure 6C).
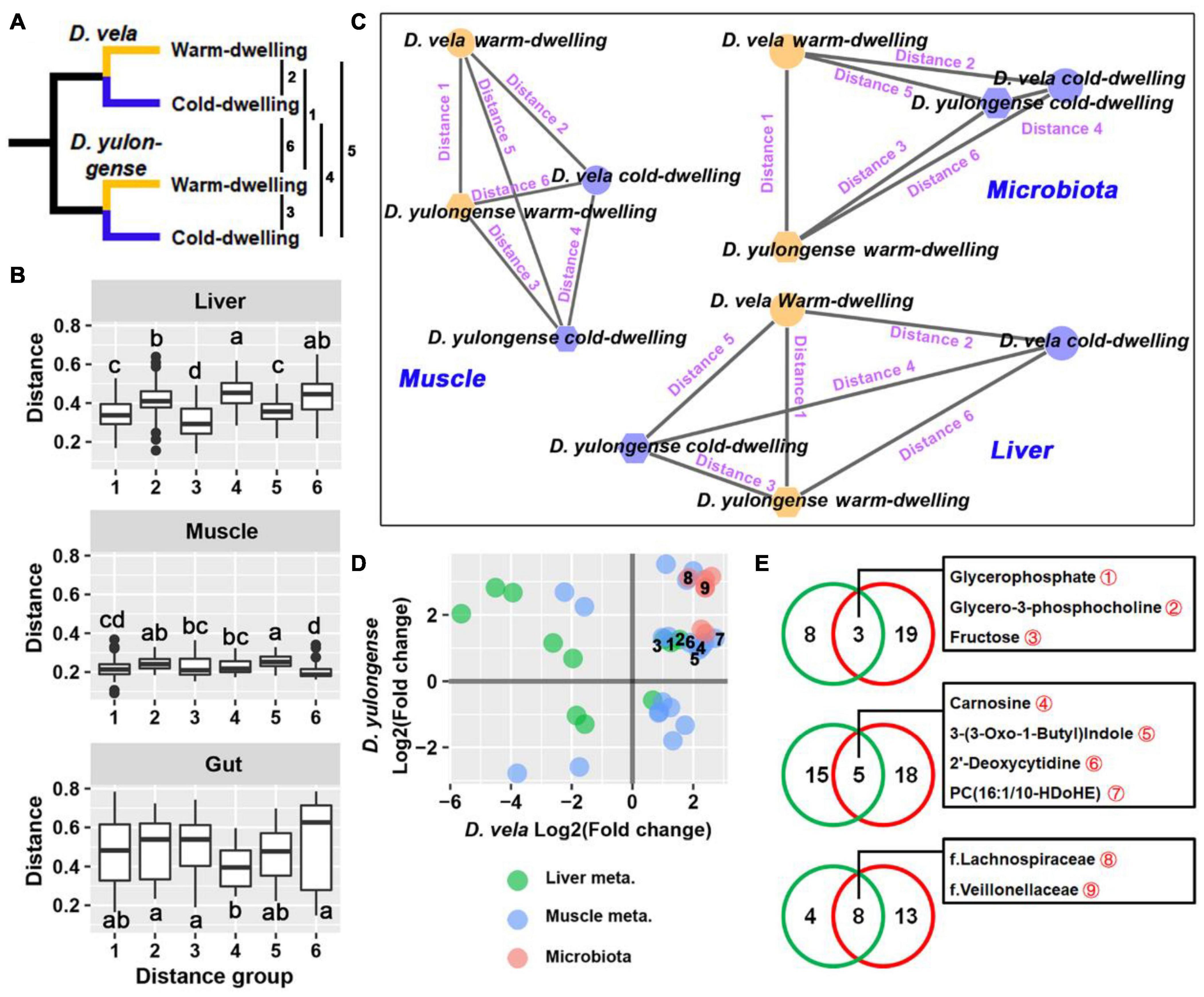
Figure 6. Intraspecies comparisons between cold- and warm-dwelling populations. (A) D. vela and D. yulongense individuals could be divided into cold- (DV-1, DY-1, and DY-2) and warm-dwelling populations (DV-2, DV-3, DY-3, and DY-4) according to the annual temperature. The distances between populations ranged from 1 to 6. (B) Distances between cold- and warm-dwelling species. Metabolome: Bray-Curtis distance; microbiome: weighted UniFrac distance. (C) Topological networks present the relative distances between groups. Note that a cold environment drives the opposite, parallel, and concurrent variations in the liver, muscle, and gut microbiome, respectively, between D. vela and D. yulongense. (D) Point plot presenting the variation patterns of metabolites and microbes, which differed significantly between cold- and warm-dwelling populations in both species. The horizontal and vertical axes denote the fold changes of cold- to warm-dwelling populations in D. vela and D. yulongense, respectively. Points with numeric numbers are annotated in panel (E). (E) Venn plots presenting the number of metabolites associated with the environmental temperature. Green circle, metabolites or microbes screened by correlation analyses across species (q < 0.05 for Pearson correlation and p < 0.05 for Spearman correlation); red circle, metabolites or microbes filtered by intraspecies comparisons between cold- and warm-dwelling populations (p < 0.05 for Mann–Whitney U-test in both species).
The organ metabolites or gut bacteria that differed between cold- and warm-dwelling populations in both species were screened (Figure 6D). For the liver metabolome, most of these metabolites exhibited divergent variation trends between D. vela and D. yulongense (Figure 6D), except for glycerophosphate, glycerol-3-phosphocholine, and fructose (Figure 6E). For the muscle metabolome, however, more metabolites exhibited consensus variation trends between the two species (Figure 6D), including carnosine and 2′-deoxycytidine (Figure 6E). For gut microbiomes, all screened taxa presented consensus variation trends between D. vela and D. yulongense. This included the Lachnospiraceae and Veillonellaceae (Figures 6D,E).
Correlation Networks Analysis
The correlations (q < 0.05 for Pearson correlation and p < 0.05 for Spearman correlation) between organ metabolites, gut bacterial taxa, and environmental factors were analyzed, and ten sub-networks were constructed (Supplementary Figure 7). Liver core metabolites (correlated with more than four bacterial taxa) included glycerophosphate, propionyl carnitine, kinetin, carnitine, creatinine, glutaric acid, 3-phenyllactic acid, and 4-guanidinobutyric acid. Muscle core metabolites included taurine, cholest-4,6-dien-3-one, kinetin-riboside, and beta-homothreonine. Core bacterial taxa were p.Proteobacteria, c.Gammaproteobacteria, o.Enterobacterales, f.Comamonadaceae, g.Comamonas, and o.Burkholderiales. Notably, metabolites associated with environmental AAT and bacteria associated with AMT were involved in the same correlation network.
Discussion
The Primary Factors Shaping the Organ Metabolome and Gut Microbiome
The most significant determinators on muscle, liver, and gut microbiome of Diploderma were phylogeny, NPP, and temperature, respectively. The influence of phylogeny on metabolism is predictable, as species with distant phylogenic relationships accumulate more genetic divergences and thus a more differentiated metabolism (Ma and Zeng, 2004). The metabolic pattern in muscle is associated with locomotive endurance, explosive force, and the locomotor mode of animals and these physiological functions play critical roles in speciation (Hedrick et al., 2020). An interesting finding was that the impact of phylogeny on metabolome exhibited organ heterogeneity. This might be explained by their different sets of organ-specific enzymes (e.g., isoenzymes), which might exhibit different evolutionary rates or varied expression plasticity in response to different environments (Pazzagli et al., 1998; Kryuchkova-Mostacci and Robinson-Rechavi, 2015). Many studies highlight that host phylogeny and diet are the two main factors influencing animal gut microbiota (Ley et al., 2008a,b). Consistently, our study also suggested that the gut bacterial taxa differed between Diploderma species (unweighted UniFrac distance). However, when taxon abundance was considered, host phylogeny was no longer a determinator. These results suggested that host phylogeny and environmental variations were mainly responsible for the gut microbiome’s taxonomic and abundance variations.
NPP was a significant determinator for the liver and muscle metabolomes and gut microbiome of mountain dragons. The relevance between primary productivity and the overall metabolic rate has been well documented in vertebrates (Lovegrove, 2000; Shi et al., 2015), and evidence from field studies suggests that a large fraction of observed variations in animal metabolism is attributable to variations in primary productivity rather than direct temperature (Tieleman et al., 2003). This is consistent with our observations in Diploderma, whose organ metabolome varies with environmental NPP significantly. As all the individuals in our study had been provided with enough food for 7 days, this association was unlikely due to their different nutrition statuses. Alternatively, it implied potential evolutionary adaptation or adaptive plastic response. In the Diploderma lizards, the associations between NPP and metabolome were more significant in the liver than in the muscle. This is reasonable, as the liver plays a central role in metabolic regulation to meet the energy requirements of different organs (Han et al., 2016; Zhu et al., 2021b), and acts a major storage space for resources in fish, amphibians, and reptiles (Derickson, 1976; Zhu et al., 2019b). The associations between environmental NPP and the gut microbiome of mountain dragons might be mediated by food availability and diet compositions, both of which could shape the host’s gut microbiome (Ley et al., 2008a,b).
Like NPP, temperature could also influence the liver and muscle metabolome and gut microbiome of mountain dragons. Environmental temperature imposes selective solid pressure on animals. The metabolome is at the forefront of life in coping with thermal stress. For example, the accumulation of cryoprotectants (e.g., glycerol and proline) and antioxidant metabolites (e.g., glutathione) reinforces the tolerance of thermal-acclimated animals to freezing (Koštál et al., 2012). In Diploderma lizards, the muscle was more responsive to variations in environmental temperatures than the liver. Cold-dwelling D. vela and D. yulongense individuals shared more metabolic variations in the muscle than the liver when compared to their respective warm-dwelling counterparts. The gut bacterial community varied with environmental temperature most significantly in mountain dragons. The influence of temperature on the animal commensal microbiome has been supported by many studies (Fan et al., 2013; Zhu et al., 2021a), and fluctuations in symbiotic microbes have been suggested to play a role in host thermal adaptation (Bo et al., 2019; Guo et al., 2021). Interestingly, we observed convergence in the gut microbiome of cold-dwelling populations from two Diploderma species. Diet-driven convergence of the gut microbiome is common to animals (Muegge et al., 2011), while evidence for environment-related convergences of the commensal microbiome is relatively scarce (Zhang et al., 2016). Whether temperature influenced the gut microbiome of Diploderma directly required further investigations. For example, it is possible that microorganisms adapted to cold conditions are less diverse than those in warm places or because the diet in cold areas is less varied, which determines a more homogeneous microbiota in these conditions.
Collectively, our results suggested that organ systems might differ in their variability, whether genetic or plastic, to climatic factors. Muscle metabolism predominantly reflects the phylogenetic relationships between species and has the lowest environmental variations. By contrast, liver metabolism exhibits a higher correspondence to environmental factors, especially NPP, but phylogeny is still a significant determinator in the liver metabolome. For the gut microbiome, however, the quantitative traits of the microbiome were only associated with environmental temperature (see a graphic summary in Figure 7).
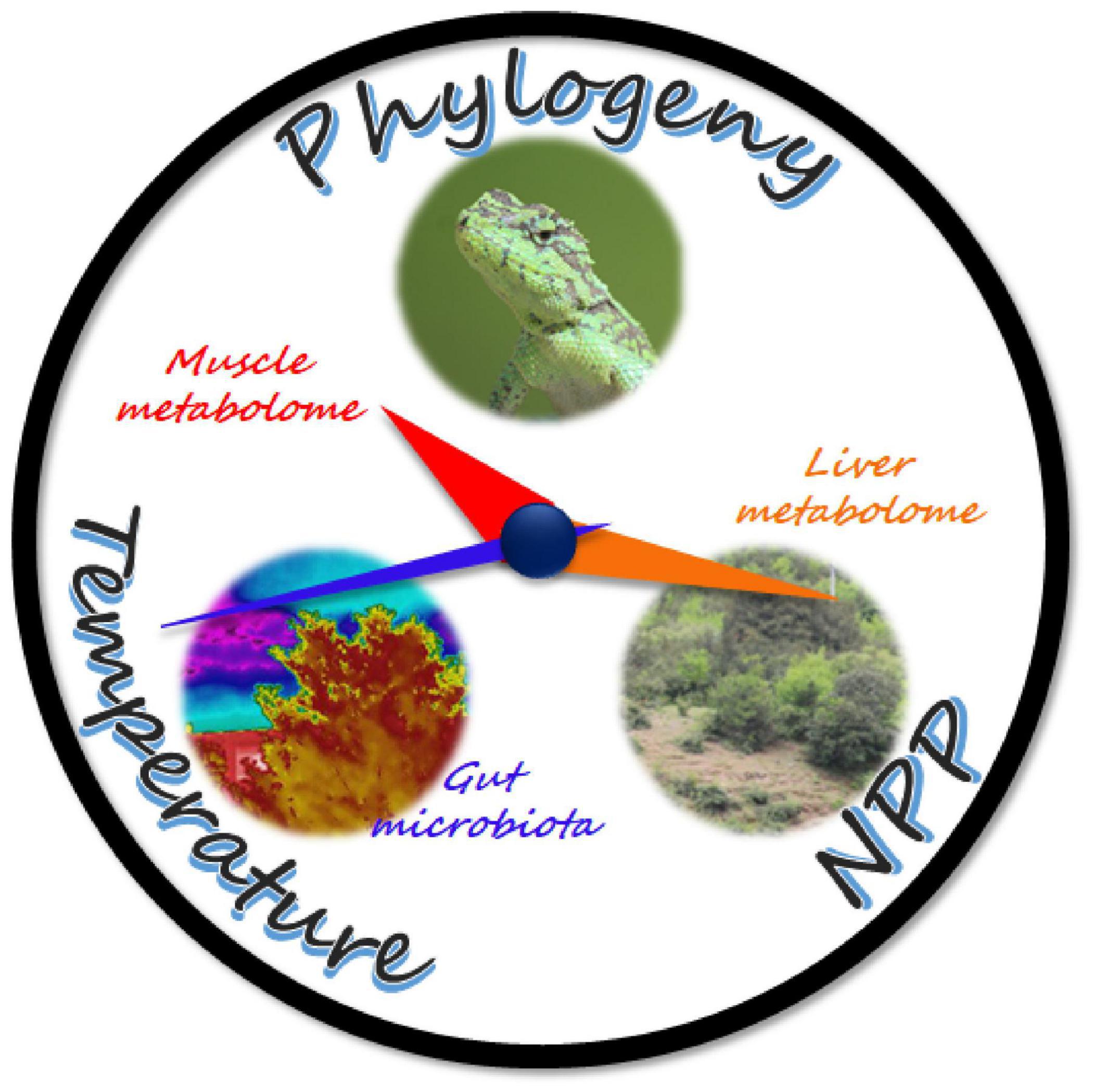
Figure 7. Schematic diagram of the results. The hour, minute, and second hands of the clock symbolize the muscle, liver, and gut microbiomes of the mountain dragons, respectively, and they are pointing to their respective major determinators. The movement speed of the clock hands symbolizes the variability, whether genetic or plastic, of organ metabolome and gut microbiome to the climatic factors. The faster they move, the more variable they are.
Potential Metabolic Adaptation to a Spatially Heterogeneous Environment
Amino acids and dipeptides were highly variable between species, implying that amino acid metabolism underwent remarkable genetic differentiation during the speciation. Notably, three gamma-glutamyl dipeptides (i.e., gamma-glutamylglutamine, gamma-glutamylmethionine, and gamma-glutamyltyrosine) differed between species in both liver and muscle. These metabolites are products of the glutathione cycle, and their levels indicate the oxidative status in tissue (Zierer et al., 2016). Additionally, gamma-glutamylglutamine plays a role in regulating neurobehavioral, e.g., fearfulness (Puurunen et al., 2018). Their variations implied physiological and behavioral divergences during the speciation of mountain dragons. Although their variation patterns between populations were not correlated with current climatic factors, they might be associated with ecological speciation from the perspective of historical climates or presumably involved in topographical adaptations.
The inverse variations in nicotinamide and NAD in the liver were the most significant metabolic variations associated with NPP. Populations with low environmental NPP had higher NAD and lower nicotinamide levels. Nicotinamide is the precursor for NAD biosynthesis (Magni et al., 2004). The cellular NAD level is a critical target for regulating energy expenditure (Cantó et al., 2009). And higher NAD levels are indicative of a metabolic shift from energy storage/anabolism to energy mobilization/catabolism, and vice versa (Revollo et al., 2007). Additionally, NAD is required for the catalytic activity of sirtuins (Imai and Armstrong, 2000; Houtkooper et al., 2010), the deacetylases that promote glucose and lipid metabolism and mediate metabolic transcriptional adaptations linked to nutrition scarcity (Imai and Armstrong, 2000; Canto et al., 2012). Accordingly, the negative correlation between NAD level NPP might be a compensation strategy for the spatial variations in NPP.
The reorganization of phospholipid composition is a common strategy for thermal adaptation in animals (Reynolds et al., 2014). The levels of hepatic glycerol-3-phosphocholine and glycerophosphate, two intermediates in phospholipid metabolism, were negatively correlated with environmental AAT in mountain dragons. These two metabolites were again highlighted in intraspecies comparisons, showing a higher abundance in the cold-dwelling populations. It suggests that phospholipid metabolism might be involved in the adaptation of mountain dragons to spatial variations in temperatures. The hepatic kinetin level was also negatively correlated with environmental AAT. This metabolite was reported to be a cryoprotectant for animal cells with antioxidation function (Zadeh Hashem and Eslami, 2018). Cold-dwelling populations tended to have higher muscle carnosine levels at both interspecies and intraspecies levels. Carnosine is highly concentrated in muscle with biological activities including antioxidation and preventing the formation of advanced glycation end-products (Boldyrev et al., 2013). Notably, carnosine can promote the heat denaturation of glycated protein (Yeargans and Seidler, 2003); thus, we presumed that a higher carnosine level could compensate for the reduced capacity in clearing glycated protein at cold conditions.
Association of Gut Microbes With Spatially Heterogeneous Environments
Gut Proteobacteria and Gammaproteobacteria abundance was positively correlated with environmental AMT. The variation trends of Proteobacteria vary between animal taxa. In insects, temperature increases have been associated with increased relative abundances of Proteobacteria (Moghadam et al., 2018). In the gut microbiome of the Chinese giant salamander, however, the abundance of Proteobacteria decreased with a rise in temperature (Zhu et al., 2021a). These variations’ biological significance or outcomes to the host have not been illuminated. In contrast to Proteobacteria, the families Lachnospiraceae, Desulfovibrionaceae, and Veillonellaceae tended to be more abundant in cold-dwelling populations of mountain dragons. Members of the Lachnospiraceae family are suggested to be beneficial to the host (Meehan and Beiko, 2014) by promoting short-chain fatty acids, converting primary bile acids to secondary ones, and facilitating colonization resistance against intestinal pathogens (Sorbara et al., 2020). Therefore, its enrichment in the gut of cold-dwelling Diploderma populations could be beneficial to the host in adapting to a challenging environment. Our results also suggested that increasing environmental moisture was accompanied by an increase in potential pathogenic and facultative anaerobic bacteria in the gut of mountain dragons (Supplementary Figure 5). Warming and high humidity favor the spread of pathogens in this environment (Bosch et al., 2007). Further studies are required to confirm the association between gut pathogen abundance and environmental moisture in mountain dragons and clarify how this association may influence the existence and distribution of mountain dragons in the HMR. Another noticeable bacterium in mountain dragons was Intestinimonas butyriciproducens due to its properties of butyrate production and host metabolic regulation (Kang, 2018). Its abundance negatively correlates with environmental temperature, moisture, and primary productivity. Further functional studies are required to give a mechanistic insight into the role of symbiotic microbiota in host adaptation to environmental variations.
Our results highlighted robust quantitative correlations between host metabolite levels and gut microbe abundances in Diploderma lizards, suggesting intimate interactions between host metabolism and gut microbes. Many of these metabolites and microbes were also associated with climatic factors, particularly temperature. It implied coordinated variations in host metabolism and gut microbiota with climatic factors. Thus, our results indicated the significance of the concept of holobiont in investigating the influence of climate on biodiversity.
Conclusion
Here, we investigated the relationship between variations in organ metabolism and gut microbiota and climatic factors in mountain dragons. The host metabolomes and gut microbiome displayed distinct variability with environmental variations, and their variations were associated with different climatic characteristics. This organ heterogeneity might be important for mountain dragons to thrive in complicated environments. We also observed convergence in the gut microbiome of cold-dwelling populations between species. Our results using the multi-omics approach provided some details regarding the interaction between holobiont and the environment, which might shed some light on the mechanisms underlying evolutionary adaptation in animals.
Data Availability Statement
The raw data of 16S rRNA gene sequences have been submitted to Genome Sequence Archive (CRA005166) at https://ngdc.cncb.ac.cn/gsub/.
Author Contributions
WZ, JJ, and LZ conceived the project. YQ and XS collected the samples. WZ, YQ, and XS performed the experiments. WZ, XW, LC, and JL analyzed the data. WZ and LZ wrote the manuscript. All authors approved the final version of the manuscript.
Funding
This work was supported by the Second Tibetan Plateau Scientific Expedition and Research Program (STEP, Grant Nos. 2019QZKK05010503 and 2019QZKK04020202) and the National Natural Science Foundation of China (Grant No. 31900327).
Conflict of Interest
The authors declare that the research was conducted in the absence of any commercial or financial relationships that could be construed as a potential conflict of interest.
Publisher’s Note
All claims expressed in this article are solely those of the authors and do not necessarily represent those of their affiliated organizations, or those of the publisher, the editors and the reviewers. Any product that may be evaluated in this article, or claim that may be made by its manufacturer, is not guaranteed or endorsed by the publisher.
Supplementary Material
The Supplementary Material for this article can be found online at: https://www.frontiersin.org/articles/10.3389/fmicb.2022.913700/full#supplementary-material
Footnotes
- ^ http://www.resdc.cn/Default.Aspx
- ^ https://xcmsonline.scripps.edu
- ^ https://www.metaboanalyst.ca/faces/ModuleView.xhtml
- ^ http://www.ncbi.nlm.nih.gov/COG
- ^ https://www.kegg.jp/kegg/
References
Bo, T.-B., Zhang, X.-Y., Wen, J., Deng, K., Qin, X.-W., and Wang, D.-H. (2019). The microbiota–gut–brain interaction in regulating host metabolic adaptation to cold in male Brandt’s voles (Lasiopodomys brandtii). ISME J. 13, 3037–3053. doi: 10.1038/s41396-019-0492-y
Boldyrev, A. A., Aldini, G., and Derave, W. (2013). Physiology and pathophysiology of carnosine. Physiol. Rev. 93, 1803–1845. doi: 10.1152/physrev.00039.2012
Bolyen, E., Rideout, J. R., Dillon, M. R., Bokulich, N. A., Abnet, C. C., Al-Ghalith, G. A., et al. (2019). Reproducible, interactive, scalable and extensible microbiome data science using QIIME 2. Nat. Biotechnol. 37, 852–857.
Bosch, J., Carrascal, L. M., Duran, L., Walker, S., and Fisher, M. C. (2007). Climate change and outbreaks of amphibian chytridiomycosis in a montane area of central Spain; is there a link? Proc. Biol. Sci. 274, 253–260. doi: 10.1098/rspb.2006.3713
Bundy, J. G., Davey, M. P., and Viant, M. R. (2009). Environmental metabolomics: a critical review and future perspectives. Metabolomics 5:3.
Callahan, B. J., Mcmurdie, P. J., Rosen, M. J., Han, A. W., Johnson, A. J. A., and Holmes, S. P. (2016). DADA2: high-resolution sample inference from Illumina amplicon data. Nat. Methods 13, 581–583. doi: 10.1038/nmeth.3869
Cantó, C., Gerhart-Hines, Z., Feige, J. N., Lagouge, M., Noriega, L., Milne, J. C., et al. (2009). AMPK regulates energy expenditure by modulating NAD+ metabolism and SIRT1 activity. Nature 458, 1056–1060. doi: 10.1038/nature07813
Canto, C., Houtkooper, R. H., Pirinen, E., Youn, D. Y., Oosterveer, M. H., Cen, Y., et al. (2012). The NAD(+) precursor nicotinamide riboside enhances oxidative metabolism and protects against high-fat diet-induced obesity. Cell Metab. 15, 838–847. doi: 10.1016/j.cmet.2012.04.022
Chang, L.-M., Zhu, W., Shi, S.-C., Zhang, M.-H., Jiang, J.-P., Li, C., et al. (2020). Plateau grass and greenhouse flower? Distinct genetic basis of closely related toad tadpoles respectively adapted to high altitude and Karst caves. Genes 11:123. doi: 10.3390/genes11020123
Charmantier, A., Mccleery, R. H., Cole, L. R., Perrins, C., Kruuk, L. E. B., and Sheldon, B. C. (2008). Adaptive phenotypic plasticity in response to climate change in a wild bird population. Science 320, 800–803. doi: 10.1126/science.1157174
Chevalier, C., Stojanoviæ, O., Colin, D. J., Suarez-Zamorano, N., Tarallo, V., Veyrat-Durebex, C., et al. (2015). Gut microbiota orchestrates energy homeostasis during cold. Cell 163, 1360–1374. doi: 10.1016/j.cell.2015.11.004
Douglas, G. M., Maffei, V. J., Zaneveld, J. R., Yurgel, S. N., Brown, J. R., Taylor, C. M., et al. (2020). PICRUSt2 for prediction of metagenome functions. Nat. Biotechnol. 38, 685–688. doi: 10.1038/s41587-020-0548-6
Fan, L., Liu, M., Simister, R., Webster, N. S., and Thomas, T. (2013). Marine microbial symbiosis heats up: the phylogenetic and functional response of a sponge holobiont to thermal stress. ISME J. 7, 991–1002. doi: 10.1038/ismej.2012.165
Greenspan, S. E., Migliorini, G. H., Lyra, M. L., Pontes, M. R., Carvalho, T., Ribeiro, L. P., et al. (2020). Warming drives ecological community changes linked to host-associated microbiome dysbiosis. Nat. Clim. Change 10, 1057–1061.
Guo, N., Wu, Q., Shi, F., Niu, J., Zhang, T., Degen, A. A., et al. (2021). Seasonal dynamics of diet-gut microbiota interaction in adaptation of yaks to life at high altitude. NPJ Biofilms Microbiomes 7:38. doi: 10.1038/s41522-021-00207-6
Han, H.-S., Kang, G., Kim, J. S., Choi, B. H., and Koo, S.-H. (2016). Regulation of glucose metabolism from a liver-centric perspective. Exp. Mol. Med. 48:e218. doi: 10.1038/emm.2015.122
Hedrick, B. P., Dickson, B. V., Dumont, E. R., and Pierce, S. E. (2020). The evolutionary diversity of locomotor innovation in rodents is not linked to proximal limb morphology. Sci. Rep. 10:717. doi: 10.1038/s41598-019-57144-w
Holt, R. D. (1990). The microevolutionary consequences of climate change. Trends Ecol. Evol. 5, 311–315. doi: 10.1016/0169-5347(90)90088-U
Houtkooper, R. H., Carles, C., Wanders, R. J., and Johan, A. (2010). The secret life of NAD+: an old metabolite controlling new metabolic signaling pathways. Endocr. Rev. 31, 194–223. doi: 10.1210/er.2009-0026
Imai, S. I., and Armstrong, C. M. (2000). Transcriptional silencing and longevity protein Sir2 is an NAD-dependent histone deacetylase. Nature 403, 795–800. doi: 10.1038/35001622
Johnson, C. H., Ivanisevic, J., and Siuzdak, G. (2016). Metabolomics: beyond biomarkers and towards mechanisms. Nat. Rev. Mol. Cell Biol. 17, 451–459. doi: 10.1038/nrm.2016.25
Kang, Y.-B. (2018). Mechanism of Konjac Glucomannan Treating Obesity by Regulating Gut Microbiota. Doctoral dissertation. Kunming: Kunming University of Science and Technology.
Keller, I., and Seehausen, O. (2012). Thermal adaptation and ecological speciation. Mol. Ecol. 21, 782–799. doi: 10.1111/j.1365-294X.2011.05397.x
Koštál, V., Šimek, P., Zahradníčková, H., Cimlová, J., and Štětina, T. (2012). Conversion of the chill susceptible fruit fly larva (Drosophila melanogaster) to a freeze tolerant organism. Proc. NatL. Acad. Sci. U.S.A. 109, 3270–3274. doi: 10.1073/pnas.1119986109
Kryuchkova-Mostacci, N., and Robinson-Rechavi, M. (2015). Tissue-specific evolution of protein coding genes in human and mouse. PLoS One 10:e0131673. doi: 10.1371/journal.pone.0131673
Kubota, S., Iwasaki, T., Hanada, K., Nagano, A. J., and Morinaga, S. I. (2015). A genome scan for genes underlying microgeographic-scale local adaptation in a wild Arabidopsis species. PLoS Genet. 11:e1005361. doi: 10.1371/journal.pgen.1005361
Lei, F.-M., Qu, Y., Song, G., Alström, P., and Fjeldså, J. (2015). The potential drivers in forming avian biodiversity hotspots in the East Himalaya Mountains of Southwest China. Integr. Zool. 10, 171–181. doi: 10.1111/1749-4877.12121
Ley, R. E., Hamady, M., Lozupone, C., Turnbaugh, P. J., Ramey, R. R., Bircher, J. S., et al. (2008a). Evolution of mammals and their gut microbes. Science 320, 1647–1651.
Ley, R. E., Lozupone, C. A., Hamady, M., Knight, R., and Gordon, J. I. (2008b). Worlds within worlds: evolution of the vertebrate gut microbiota. Nat. Rev. Microbiol. 6, 776–788. doi: 10.1038/nrmicro1978
Lovegrove, B. G. (2000). The zoogeography of mammalian basal metabolic rate. Am. Nat. 156, 201–219. doi: 10.1086/303383
Ma, H.-W., and Zeng, A.-P. (2004). Phylogenetic comparison of metabolic capacities of organisms at genome level. Mol. Phylogenet. Evol. 31, 204–213. doi: 10.1016/j.ympev.2003.08.011
Magni, G., Amici, A., Emanuelli, M., Orsomando, G., Raffaelli, N., and Ruggieri, S. (2004). Enzymology of NAD+ homeostasis in man. Cell. Mol. Life Sci. 61, 19–34. doi: 10.1007/s00018-003-3161-1
Meehan, C. J., and Beiko, R. G. (2014). A phylogenomic view of ecological specialization in the Lachnospiraceae, a family of digestive tract-associated bacteria. Genome Biol. Evol. 6, 703–713. doi: 10.1093/gbe/evu050
Moghadam, N. N., Thorshauge, P. M., Kristensen, T. N., De Jonge, N., Bahrndorff, S., Kjeldal, H., et al. (2018). Strong responses of Drosophila melanogaster microbiota to developmental temperature. Fly 12, 1–12. doi: 10.1080/19336934.2017.1394558
Muegge, B. D., Kuczynski, J., Knights, D., Clemente, J. C., González, A., Fontana, L., et al. (2011). Diet drives convergence in gut microbiome functions across mammalian phylogeny and within humans. Science 332, 970–974. doi: 10.1126/science.1198719
Myers, N., Mittermeier, R. A., Mittermeier, C. G., Da Fonseca, G. A. B., and Kent, J. (2000). Biodiversity hotspots for conservation priorities. Nature 403, 853–858.
Pazzagli, L., Manao, G., Cappugi, G., Caselli, A., Camici, G., Moneti, G., et al. (1998). The amino acid sequences of two acylphosphatase isoforms from fish muscle (Lamna nasus). Biochim. Biophys. Acta 1387, 264–274. doi: 10.1016/s0167-4838(98)00134-4
Puurunen, J., Tiira, K., Vapalahti, K., Lehtonen, M., Hanhineva, K., and Lohi, H. (2018). Fearful dogs have increased plasma glutamine and gamma-glutamyl glutamine. Sci. Rep. 8:15976. doi: 10.1038/s41598-018-34321-x
Quast, C., Pruesse, E., Yilmaz, P., Gerken, J., Schweer, T., Yarza, P., et al. (2013). The SILVA ribosomal RNA gene database project: improved data processing and web-based tools. Nucleic Acids Res. 41, D590–D596. doi: 10.1093/nar/gks1219
Rainey, P. B., and Travisano, M. (1998). Adaptive radiation in a heterogeneous environment. Nature 394, 69–72. doi: 10.1038/27900
Revollo, J. R., Grimm, A. A., and Imai, S. I. (2007). The regulation of nicotinamide adenine dinucleotide biosynthesis by Nampt/PBEF/visfatin in mammals. Curr. Opin. Gastroenterol. 23, 164–170. doi: 10.1097/MOG.0b013e32801b3c8f
Reynolds, A. M., Lee, R. E. Jr., and Costanzo, J. P. (2014). Membrane adaptation in phospholipids and cholesterol in the widely distributed, freeze-tolerant wood frog, Rana sylvatica. J. Comp. Physiol. B 184, 371–383. doi: 10.1007/s00360-014-0805-4
Rosenberg, E., and Zilber-Rosenberg, I. (2018). The hologenome concept of evolution after 10 years. Microbiome 6:78. doi: 10.1186/s40168-018-0457-9
Savolainen, O., Lascoux, M., and Merilä, J. (2013). Ecological genomics of local adaptation. Nat. Rev. Genet. 14, 807–820.
Shi, Y.-L., Chi, Q.-S., Liu, W., Fu, H.-P., and Wang, D.-H. (2015). Environmental metabolomics reveal geographic variation in aerobic metabolism and metabolic substrates in Mongolian gerbils (Meriones unguiculatus). Comp. Biochem. Physiol. D Genomics Proteomics 14, 42–52. doi: 10.1016/j.cbd.2015.03.001
Sinervo, B., Méndez-De-La-Cruz, F., Miles Donald, B., Heulin, B., Bastiaans, E., Villagrán-Santa Cruz, M., et al. (2010). Erosion of lizard diversity by climate change and altered thermal niches. Science 328, 894–899.
Sorbara, M. T., Littmann, E. R., Fontana, E., Moody, T. U., Kohout, C. E., Gjonbalaj, M., et al. (2020). Functional and genomic variation between human-derived isolates of Lachnospiraceae reveals inter- and intra-Species diversity. Cell Host Microbe 28, 134–146.e4. doi: 10.1016/j.chom.2020.05.005
Southwood, A., and Avens, L. (2010). Physiological, behavioral, and ecological aspects of migration in reptiles. J. Comp. Physiol. B 180, 1–23. doi: 10.1007/s00360-009-0415-8
Stuart Simon, N., Chanson Janice, S., Cox Neil, A., Young Bruce, E., Rodrigues Ana, S. L., Fischman Debra, L., et al. (2004). Status and trends of amphibian declines and extinctions worldwide. Science 306, 1783–1786.
Thomas, C. D., Cameron, A., Green, R. E., Bakkenes, M., Beaumont, L. J., Collingham, Y. C., et al. (2004). Extinction risk from climate change. Nature 427, 145–148.
Tieleman, B. I., Williams, J. B., and Bloomer, P. (2003). Adaptation of metabolism and evaporative water loss along an aridity gradient. Proc. R. Soc. B Biol. Sci. 270, 207–214. doi: 10.1098/rspb.2002.2205
Urban, M. C., Zarnetske, P. L., and Skelly, D. K. (2013b). Moving forward: dispersal and species interactions determine biotic responses to climate change. Ann. N. Y. Acad. Sci. 1297, 44–60. doi: 10.1111/nyas.12184
Urban, M. C., Richardson, J. L., and Freidenfelds, N. A. (2013a). Plasticity and genetic adaptation mediate amphibian and reptile responses to climate change. Evol. Appl. 7, 88–103. doi: 10.1111/eva.12114
Valladares, F., Matesanz, S., Guilhaumon, F., Araujo, M. B., Balaguer, L., Benito-Garzon, M., et al. (2014). The effects of phenotypic plasticity and local adaptation on forecasts of species range shifts under climate change. Ecol. Lett. 17, 1351–1364.
Vogt, G. (2017). Facilitation of environmental adaptation and evolution by epigenetic phenotype variation: insights from clonal, invasive, polyploid, and domesticated animals. Environ. Epigenet. 3:dvx002. doi: 10.1093/eep/dvx002
Wang, K., Ren, J., Wu, J., Jiang, K., Jin, J., Hou, S., et al. (2020). Systematic revision of mountain dragons (Reptilia: Agamidae: Diploderma) in China, with descriptions of six new species and discussion on their conservation. J. Zool. Syst. Evol. Res. 59, 222–263.
Ward, T., Larson, J., Meulemans, J., Hillmann, B., Lynch, J., Sidiropoulos, D., et al. (2017). BugBase predicts organism-level microbiome phenotypes. bioRxiv [Preprint] doi: 10.1101/133462
Wickham, H. (2009). Ggplot2: Elegant Graphics for Data Analysis. New York, NY: Springer Publishing Company.
Xiong, Y., Hao, Y., Cheng, Y., Fan, L., Song, G., Li, D., et al. (2021). Comparative transcriptomic and metabolomic analysis reveals pectoralis highland adaptation across altitudinal songbirds. Integr. Zool. [Epub ahead of print]. doi: 10.1111/1749-4877.12620
Xu, F., Jia, Y.-W., Peng, H., Niu, C.-W., and Liu, J.-J. (2018). Temperature and precipitation trends and their links with elevation in the Hengduan Mountain region, China. Clim. Res. 75, 163–180.
Yeargans, G. S., and Seidler, N. W. (2003). Carnosine promotes the heat denaturation of glycated protein. Biochem. Biophys. Res. Commun. 300, 75–80. doi: 10.1016/s0006-291x(02)02796-1
Zadeh Hashem, E., and Eslami, M. (2018). Kinetin improves motility, viability and antioxidative parameters of ram semen during storage at refrigerator temperature. Cell Tissue Bank 19, 97–111. doi: 10.1007/s10561-016-9604-3
Zhang, Z.-G., Xu, D.-M., Wang, L., Hao, J.-J., Wang, J.-F., Zhou, X., et al. (2016). Convergent evolution of rumen microbiomes in high-altitude mammals. Curr. Biol. 26, 1873–1879. doi: 10.1016/j.cub.2016.05.012
Zhu, B.-L., Wang, X., and Li, L.-J. (2010). Human gut microbiome: the second genome of human body. Protein Cell 1, 718–725. doi: 10.1007/s13238-010-0093-z
Zhu, L., Zhu, W., Zhao, T., Chen, H., Zhao, C., Xu, L., et al. (2021a). Environmental temperatures affect the gastrointestinal microbes of the Chinese giant salamander. Front. Microbiol. 12:543767. doi: 10.3389/fmicb.2021.543767
Zhu, W., Chang, L., Shu, G., Wang, B., and Jiang, J. P. (2021b). Fatter or stronger: resource allocation strategy and the underlying metabolic mechanisms in amphibian tadpoles. Comp. Biochem. Physiol. D Genomics Proteomics 38:100825. doi: 10.1016/j.cbd.2021.100825
Zhu, W., Shi, X., Qi, Y., Wang, X., Chang, L., Zhao, C., et al. (2021c). Commensal microbiota and host metabolic divergence are associated with the adaptation of Diploderma vela to spatially heterogeneous environments. Integr. Zool. 17, 346–365.
Zhu, W., Meng, Q., Zhang, H., Wang, M. L., Li, X., Wang, H. T., et al. (2019a). Metabolomics reveals the key role of oxygen metabolism in heat susceptibility of an alpine-dwelling ghost moth, Thitarodes xiaojinensis (Lepidoptera: Hepialidae). Insect Sci. 26, 695–710. doi: 10.1111/1744-7917.12605
Zhu, W., Zhang, M., Chang, L., Zhu, W., Li, C., Xie, F., et al. (2019b). Characterizing the composition, metabolism and physiological functions of the fatty liver in Rana omeimontis tadpoles. Front. Zool. 16:42. doi: 10.1186/s12983-019-0341-x
Zhu, W., Zhang, H., Li, X., Meng, Q., Shu, R. H., Wang, M. L., et al. (2016). Cold adaptation mechanisms in the ghost moth Hepialus xiaojinensis: metabolic regulation and thermal compensation. J. Insect Physiol. 85, 76–85. doi: 10.1016/j.jinsphys.2015.11.008
Keywords: heterogeneous environment, lizard, local adaptation, multi-omics, organ heterogeneity
Citation: Zhu W, Qi Y, Wang X, Shi X, Chang L, Liu J, Zhu L and Jiang J (2022) Multi-Omics Approaches Revealed the Associations of Host Metabolism and Gut Microbiome With Phylogeny and Environmental Adaptation in Mountain Dragons. Front. Microbiol. 13:913700. doi: 10.3389/fmicb.2022.913700
Received: 06 April 2022; Accepted: 25 May 2022;
Published: 28 June 2022.
Edited by:
Tongtong Li, Zhejiang University of Technology, ChinaReviewed by:
Huan Li, Lanzhou University, ChinaQianqian Zhang, State Key Laboratory of Freshwater Ecology and Biotechnology, Institute of Hydrobiology (CAS), China
Copyright © 2022 Zhu, Qi, Wang, Shi, Chang, Liu, Zhu and Jiang. This is an open-access article distributed under the terms of the Creative Commons Attribution License (CC BY). The use, distribution or reproduction in other forums is permitted, provided the original author(s) and the copyright owner(s) are credited and that the original publication in this journal is cited, in accordance with accepted academic practice. No use, distribution or reproduction is permitted which does not comply with these terms.
*Correspondence: Lifeng Zhu, emh1bGYyMDIwQDEyNi5jb20=; Jiangping Jiang, amlhbmdqcEBjaWIuYWMuY24=
†These authors have contributed equally to this work