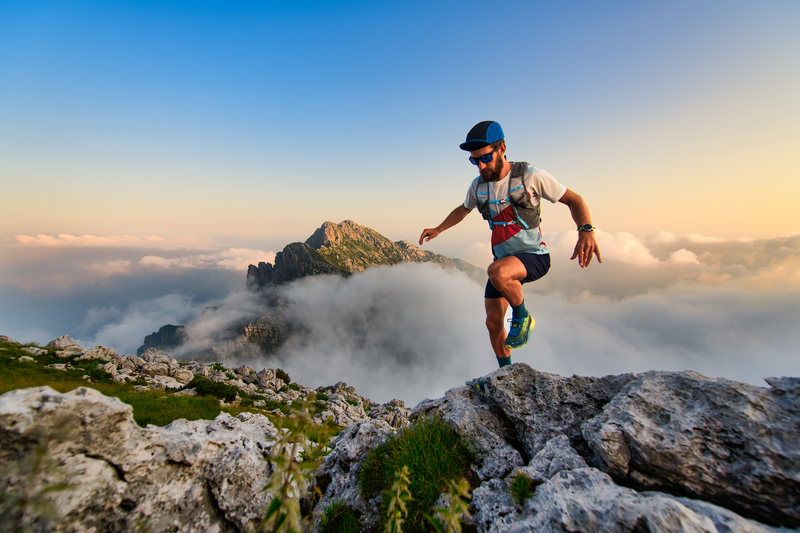
94% of researchers rate our articles as excellent or good
Learn more about the work of our research integrity team to safeguard the quality of each article we publish.
Find out more
ORIGINAL RESEARCH article
Front. Microbiol. , 25 July 2022
Sec. Terrestrial Microbiology
Volume 13 - 2022 | https://doi.org/10.3389/fmicb.2022.911116
This article is part of the Research Topic Rising Stars in Terrestrial Microbiology: 2022 View all 9 articles
Soil microeukaryotes play a pivotal role in soil nutrient cycling and crop growth in agroecosystems. However, knowledge of microeukaryotic community distribution patterns, assembly processes, and co-existence networks is greatly limited. Here, microbial eukaryotes in bulk and rhizosphere soils of the North China Plain were investigated. The results showed that soil pH was the driving factor for the microeukaryotic community composition in the bulk and rhizosphere soils. The soil microeukaryotic community could significantly differ between alkaline and acidic soils. The results indicated that the soil pH had a stronger effect than niche differences on community composition. Partial Mantel tests showed that soil pH and spatial distance had similar effects on the microeukaryotic community composition in the bulk soil. However, in the rhizosphere soil, spatial distance had a stronger effect than soil pH. Infer Community Assembly Mechanisms by Phylogenetic bin-based null model (iCAMP) analysis revealed that drift was the most important process driving microeukaryotic community assembly, with an average relative importance of 37.4–71.1%. Dispersal limitation displayed slightly greater importance in alkaline rhizosphere than in alkaline bulk soils. Meanwhile, the opposite trend was observed in acidic soils. In addition, the contribution of each assembly process to each iCAMP lineage “bin” varied according to the acidic or alkaline conditions of the soil and the niche environment. High proportions of positive links were found within the four ecological networks. Alkaline soil networks, especially the alkaline bulk soil network, showed greater complexity than the acidic soil networks. Natural connectivity analysis revealed that the rhizosphere community had a greater stability than the bulk soil community in alkaline soil. This study provides a foundation for understanding the potential roles of microbial eukaryotes in agricultural soil ecosystem functioning.
Soil microbial eukaryotes (microeukaryotes) including fungi and protists are key soil residents that play a pivotal role in terrestrial ecosystem functioning (Fierer, 2017; Delgado-Baquerizo et al., 2018, 2020). Fungi, for example, are the central players in soil nutrient cycling, dead plant decomposition and disease mediation (Tedersoo et al., 2014; Soudzilovskaia et al., 2019; Liu et al., 2021; Zhang et al., 2022). Meanwhile, protists, which comprise an important component of the soil microbiome, play a critical role in top-down interactions and soil food webs (Oliverio et al., 2020; Xiong et al., 2020; Aslani et al., 2021). Due to the critical role of microbial eukaryotes in ecological service, it is necessary to understand the diversity, assembly process, and co-existence patterns of microeukaryotic communities in various ecosystems (Crowther et al., 2019).
Understanding the factors driving microbial diversity and distribution is a core area of research in microbial ecology (Fierer and Jackson, 2006; Martiny et al., 2006). Soil pH, which regulates the soil capacity for storing and supplying nutrients (Slessarev et al., 2016), has a fundamental influence on microbial distribution patterns (Fierer, 2017; Jiao and Lu, 2020). However, there are few available reports on how pH affects soil microeukaryotes. Recently, Aslani et al. (2021) found that soil pH was the primary determinant of eukaryotic microbial community distribution on a global scale. However, most studies are from natural ecosystems (Fierer, 2017), such as forest (Yang et al., 2019) and grassland ecosystem (Oliverio et al., 2020), the effect of soil pH on microeukaryotes in agroecosystems is understudied (Shi et al., 2019a). Moreover, rhizosphere, a hotspot for beneficial interactions between plant roots and microbes (de Vries et al., 2020; Li et al., 2021), also harbors various microeukaryotes. However, our understanding of distribution patterns of microeukaryotes in rhizosphere is still limited in agricultural ecosystem (Pineda et al., 2017).
Several studies have reported that both deterministic (e.g., soil) and stochastic processes (e.g., drift) play important roles in soil microbial distribution (Martiny et al., 2006; Stegen et al., 2013; Dini-Andreote et al., 2015; Shi et al., 2018). These deterministic and stochastic processes entail five main scenarios. Dispersal limitation (DL), drift (DR), and homogenous dispersal (HD) are defined as stochastic process, while heterogeneous selection (HeS) and homogenous selection (HoS) are deterministic processes. These five scenarios have been carefully described by Shi et al. (2019a). Briefly, HeS refers to environments that are highly spatially heterogeneous (Vellend, 2010); HoS refers to situations with spatially homogeneous environments (Shi et al., 2019a); HD describes high rates of dispersal between communities (Shi et al., 2019a); DL refers to spatial isolation (Whitaker et al., 2003; Zhou et al., 2008); and DR describes situations of ecological drift (Dini-Andreote et al., 2015; Feng Y. et al., 2018).
The five scenarios have been well-described in various habitats (Zhou et al., 2014; Feng M. M. et al., 2018; Jiao and Lu, 2020). For example, Feng Y. et al. (2018) revealed the relative role of these five processes in shaping soil microbial communities in long term fertilization fields. More recently, Aslani et al. (2021) suggested that drift is a dominant ecological process shaping soil eukaryotic community assembly on the global scale. However, their findings should be confirmed by further studies. Based on these findings, in this study, it was hypothesized that drift may be the dominant factor driving microeukaryotic community assembly in agricultural ecosystems (Orrock and Watling, 2010; Powell et al., 2015; Giner et al., 2018; Fodelianakis et al., 2021).
The tremendous numbers of microorganisms living in the soil are not independent, but form ecological networks involving mutualism, commensalism, amensalism, competitive parasitism, and predative relationships (Faust and Raes, 2012; Cardinale et al., 2015; Zhang B. et al., 2018; Ma et al., 2020; Shi et al., 2020). Microbial co-existence patterns involving prokaryotes, protists, and fungi have been well-described in marine (Lima-Mendez et al., 2015), forest (Ma et al., 2016), grassland (Shi et al., 2019b), and crop ecosystems (Xiong et al., 2019). Faust et al. (2015) revealed microbial association networks from 20 different cross-biome 16S rDNA sequencing datasets and observed that the tundra network contained a node representing pH. In the North China Plain, Shi et al. (2020) built a large-scale co- existence network of fungal and bacterial taxa using 243 soil samples, and they found the importance of the abundance of network hubs for soil functioning in wheat field systems.
Previous studies have revealed that microbial associations within biological community networks are critical for their stability (Neutel et al., 2002; Coux et al., 2016). Co-existence network approaches have been increasingly applied to reveal the stability of the association relationships among microbial individuals (Fan et al., 2018; Wu et al., 2021). Network robustness, calculated by the degree of natural connectivity through “attacking” (randomly removing) the edges and nodes within the network (Albert et al., 2000; Peng and Wu, 2016), is a method that is frequently being used to reflect network stability (Fan et al., 2018; Shi et al., 2019b; Wu et al., 2021). A greater network robustness indicates a more stable community, while a lower robustness reflects an unstable community. It is generally considered that soil microeukaryotes also form ecological associations. However, far fewer studies have specifically investigated co-existence patterns for these critical functioning players and their stability in agroecosystems (Zhang W. J. et al., 2018).
The North China Plain is the most important food-producing area in Asia, providing over 50% of China’s total cereal production (Piao et al., 2010; Jeong et al., 2014). Previously, researchers revealed the distribution patterns of soil bacteria and fungi in this region (Shi et al., 2018, 2020), and found the driving effect of soil pH. Additionally, microbial assembly processes and co-existence patterns were investigated across the North China Plain (Shi et al., 2018, 2020). In particular, deterministic processes were found to dominate at a broad scale (Shi et al., 2018), and the abundance of keystone species within the soil microbial networks showed high soil functional potential in this region (Shi et al., 2020). Moreover, the wheat rhizosphere was found to display a less complex but more stable microbial association network than the bulk soil (Fan et al., 2018).
In the present study, to investigate soil microeukaryotic community distribution patterns, assembly processes and co-existence networks, 20 bulk soil samples and 20 rhizosphere soil samples were collected across four sites in the North China Plain. It was hypothesized that soil pH could be the main driver shaping the bulk and rhizosphere soil microeukaryotic communities, and that drift would play an important role in determining microeukaryotic community assembly.
To survey the assembly processes and co-existence patterns of the microeukaryotic communities, 20 bulk soil and 20 rhizosphere soil samples in wheat fields were collected across four sites in the North China Plain in late April 2018. To ensure that all quadrats in each site have similar soil pH values in this study, we chose four typical sites which could represent acidic and alkaline soils according to Shi et al.’s (2018) study. For example, in a site, all the rhizosphere and bulk soils are alkaline in the five plots, while in another site, all the soils are acidic. The four sites were in Daming county (DM), Sheqi county (SQ), Taihe county (TH), and Tengzhou county (TZ). Each site had dimensions of 10 km by 10 km (100 km2) (Supplementary Table 1). Within each site, there were five plots (four plots are from the four corners and one plot in the center; Supplementary Figure 1), with the plots being at least 6 km apart from any other plot. The topography of sampling area is flat, and the altitude of sampling sites is below 50 m above sea level. The sampling region has a warm temperate monsoon climate, with an average annual temperature of 8–15°C and the average annual precipitation of 500–1,000 mm. The soils of sampling sites were classified as Ochric Aquic Cambosols (Chinese soil taxonomy) in our study (Zhu et al., 2005).
At each plot, groups of wheat plants (with 6–8 plants in each group) were removed to collect the rhizosphere soil (Donn et al., 2015; Fan et al., 2017). To obtain the rhizosphere soil, the plants were first lightly shaken and then the tightly bonded soil that remained attached the root surface was collected. Next to each group of plants (∼25 cm), from an area without plants, 3–5 cores of topsoil (0–15 cm) were collected and mixed by drill as bulk soil. Finally, five bulk soil and five rhizosphere soil samples were obtained for each site (a total of four sites were surveyed). All collected soil samples were immediately shipped to the laboratory in a cooler at 4°C. To remove the visible roots, residues and stones, the soils were sieved using 2 mm mesh. The sample was then divided into two parts: one part was stored at 4°C for physicochemical analysis and the other was stored at 20°C for DNA extraction.
To measure soil pH, fresh soil with a soil-to-water ratio of 1:5 was tested using a pH monitor (Thermo Orion-868, Boston, MA, United States). The soil moisture content of each sample was determined gravimetrically after oven-drying at 105°C for 16 h. A total of 0.5 g of fresh soil was used for DNA extraction. The soil DNA was extracted using a Power Soil DNA kit (MO BIO, Carlsbad, CA, United States) and purified with an Ultra Clean 15 DNA purification kit (MO BIO) following the manufacturer’s instructions. The soil DNA was then stored at −40°C.
The primers SSU0817F (5′-TTAGCATGGAATAATRRAATAG GA-3′) and 1196R (5′-TCTGGACCTGGTGAGTTTCC-3′) (Rousk et al., 2010) were used to target and amplify the microbial eukaryotic 18S rRNA V5–V7 region in each sample. The polymerase chain reaction (PCR) products were obtained under the following conditions: 94°C for 5 min, followed by 35 cycles of 94°C for 30 s, 50°C for 30 s, and 72°C for 30 s. The PCR products were sequenced using the Illumina MiSeq PE 250 platform. High-throughput data from this analysis were submitted to the National Center for Biotechnology Information (NCBI) Sequence Read Archive (SRA) under accession number SRP347607.
Raw data sequences were processed and analysed using QIIME 2 (version: 2019.7) following the workflow at https://qiime2.org (Bolyen et al., 2019). Briefly, to obtain the amplified sequence variants (ASVs), Deblur was used to perform the quality control of the raw sequencing data (Amir et al., 2017). Low quality regions of the sequences were removed according to the sequence quality plot (each sequence was truncated at 120 bp). To identify and filter chimeras, vsearch was used to perform de novo chimera filtering. Based on the Sklearn-based taxonomy classifier, taxonomy assignment was performed using the dynamic Unite database from 10.10.2017.1 To rarefy the sequence number, 10,539 high-quality sequences were randomly selected for each sample.
To reveal the soil microeukaryotic community assembly processes, iCAMP was selected (Ning et al., 2020). Using this approach (which can quantitatively explain community assembly mechanisms through phylogenetic bin based null model analysis), five assembly processes could be examined: DR, HD, DL, HeS, and HoS. In brief, the five processes could be observed via three major steps. The first step is phylogenetic binning. The second step is bin-based null model analysis, which partitions deterministic and stochastic processes into HoS, HeS, HD, DL, and DR. The final step is then to integrate the results for different bins to assess the relative importance of each process to them (Ning et al., 2020). In this study, 43 microeukaryotic bins were obtained. The confidence index was used for null model significance testing.
The Sparse Correlations for Compositional data (SparCC) package was used to construct the microeukaryotic co-existence network following the procedure carefully described by Weiss et al. (2016). Before network construction, the ASVs table was filtered to improve the reliability of the networks. Four networks were constructed corresponding to the acidic rhizosphere soil network (AcRN), acidic bulk soil network (AcBN), alkaline rhizosphere soil network (AlRN), and alkaline bulk soil network (AlBN). Firstly, singletons were removed and only ASVs with an abundance of more than 0.01% of all samples in each group were retained. Finally, 295, 297, 301, and 379 ASVs were retained in AcRN, AcBN, AlRN, and AlBN, respectively. Then, the filtered ASVs tables were selected to construct the networks. All network topological features were quantified using the R ‘‘igraph’’ package2 and network visualizations were generated using Gephi.3
To investigate the microeukaryotic community stability, the robustness test was selected. To test the robustness of the networks, the natural connectivity was estimated by “attacking” the nodes (May, 1973) or edges (Jordan, 2009) of the SparCC network. To identify the network hubs, module hubs and connectors of each network, the z and c scores of each node within each network were calculated. Based on the threshold values of the z score (within-module degree) and the c score (participation coefficient) of nodes: nodes with a z score > 2.5 and c score > 0.6 were classified as network hubs; nodes with a z score > 2.5 and c score < 0.6 were classified as module hubs; nodes with a z score < 2.5 and c score < 0.6 were classified as connectors; and nodes with a z score < 2.5 and c score < 0.6 were classified as peripherals. The role of the network hubs, module hubs and connectors within networks have been carefully described by Shi et al. (2019b).
After high-throughput sequencing, between 11,318 and 23,839 high-quality microeukaryotic sequences were obtained per sample. Of these, 99.9% were classified into a total of 1,034 distinct ASVs, including mostly fungi (95.3%), followed by other microbial eukaryotes such as Ciliophora (2.79%), Aphelidea (0.14%), and very few Incertae_Sedis, Amoebozoa, and Cercozoa (less than 0.01% in total). Sordariomycetes, Dothideomycetes, and Tremellomycetes dominated the assigned microeukaryotic classes (mainly fungi) and accounted for approximately 30.3, 13.6, and 5.82% of all ASVs sequences, respectively (Figure 1). The relative abundance of each microeukaryotic group varied among soil groups. For example, Sordariomycetes was highly abundant in alkaline and bulk soils (Figures 1B,C). Meanwhile, Dothideomycetes was less abundant in bulk soils than in rhizosphere soils (Figure 1C). Tremellomycetes was more abundant in acidic soils than in alkaline soils. The alpha diversity, which was represented by the observed species, was higher in alkaline soils than in acidic soils. Meanwhile, in bulk and rhizosphere soils, there was no significant difference in alpha diversity (Supplementary Figure 1).
Figure 1. Relative abundance of the dominant soil microeukaryotic groups in alkaline (Alk), acidic (Aci), bulk (B), and rhizosphere (R) soils from four sites in the North China Plain (Daming [DM], Sheqi [SQ], Taihe [TH], and Tengzhou [TZ]). The relative abundances of the different groups are compared across (A) site, soil type (alkaline or acidic) and niche environment (rhizosphere or Bulk soil), (B) soil type, and (C) niche environment. The relative abundances are based on the frequencies of DNA sequences that could be classified to the class level. “Other” represents sequences that were unclassified and sequences that were present in amounts of less than 1% of the total.
The non-metric multidimensional scaling (NMDS) ordination plots of the soil microeukaryotic communities displayed clear patterns (Figure 2). The results showed that the microeukaryotic communities could significantly differ between alkaline and acidic soils (Adonis test, F = 4.17, R2 = 0.1, P < 0.001), and between bulk and rhizosphere soils (Adonis test, F = 1.70, R2 = 0.04, P < 0.001). In particular, the acidity and alkalinity of the soil had a stronger effect than the niche difference on the community composition (Figure 2).
Figure 2. Non-metric multidimensional scaling (NMDS) ordinations showing the microeukaryotic community compositional dissimilarities among the four sampling sites. Triangles, Daming [DM]; squares, Taihe [TH]; stars, Sheqi [SQ]; circles, Tengzhou [TZ]; red, alkaline rhizosphere soil; blue, alkaline bulk soil; light blue, acidic rhizosphere soil; pink, acidic bulk soil.
To elucidate the relative roles of the environment and spatial distance on the microeukaryotic community, Mantel and partial Mantel tests were conducted. The results showed that both the soil pH (bulk: r = 0.49, P = 0.001; rhizosphere: r = 0.29, P = 0.001) and soil moisture (bulk: r = 0.28, P = 0.001; rhizosphere: r = 0.17, P = 0.026) significantly correlated with the microeukaryotic community composition in the bulk and rhizosphere soils. The soil pH had a stronger effect than the soil moisture (Table 1). The partial Mantel tests showed that soil pH and spatial distance had similar effects on the soil microeukaryotic community composition in the bulk soil. However, in the rhizosphere soil, spatial distance had a stronger effect than the soil pH.
Table 1. Mantel test results showing relationships between soil pH, soil moisture (SM), and eukaryotic community composition in rhizosphere and bulk soil.
The iCAMP analysis revealed that DR was the most important among the five processes, with an average relative importance of 37.4–71.1% (Figure 3 and Supplementary Table 2). DL also provided a strong contribution to community assembly, with an average relative importance of 12.3–45.1% (Figure 3 and Supplementary Table 2). In particular, DL appeared to be slightly more important in the rhizosphere than in the bulk soil in alkaline soils (Figure 3). Meanwhile in acidic soils, the opposite trend was observed. Additionally, HoS was of slightly greater importance in bulk soils than in rhizosphere soils in DM, TH, and SQ, while it had less importance in TZ (Figure 3).
Figure 3. Relative importance of five ecological processes for the assembly of the microeukaryotic communities in the rhizosphere and bulk soils across the four sampling sites [(A): Daming [DM], (B): Taihe [TH], (C): Sheqi [SQ], and (D): Tengzhou [TZ]]. Ecological processes include: Dispersal limitation-DL, drift-DR, homogenous dispersal-HD, heterogeneous selection-HeS, and homogenous selection-HoS.
The next step was to investigate the contribution of different assembly processes to individual lineages (i.e., bins). In this study, the observed 1,034 ASVs were divisible into 43 phylogenetic bins. The relative importance of a given assembly process was independent of the relative abundance in the bins (Figure 4). In addition, the contribution of each assembly process to a given bin varied according to the acidic or alkaline conditions of the soil and the niche environment. For example, for Bin1, DL provided a large contribution in the rhizosphere soil in SQ, but HoS was of greater importance in the rhizosphere soil in DM. For Bin2, DR contributed strongly in bulk soil in SQ, while DL showed greater importance in bulk soil in DM.
Figure 4. Relative importance of each ecological process for each iCAMP microeukaryotic lineage bin across the four sampling sites: (A) Sheqi (SQ), (B) Tengzhou (TZ), (C) Daming (DM), and (D) Taihe (TH). Only the five most abundant bins are shown. Source data can be found in Supplementary Table 2. For abbreviations, please see Figure 3.
Using SparCC correlation analysis, four association networks were constructed: acidic bulk soil, acidic rhizosphere soil, alkaline bulk soil, and alkaline rhizosphere soil (Figure 5). The topological features are shown in Table 2. Briefly, high proportions of positive links were found in the four ecological networks. Alkaline soil networks, especially the alkaline bulk soil network, showed greater complexity than acidic soil networks (Table 2).
Figure 5. Co-existence networks for microeukaryotic communities in acidic bulk, acidic rhizosphere, alkaline bulk, and alkaline rhizosphere soil. The co-existence networks were constructed using the SparCC method to correlate amplified sequence variants (ASVs). For the node colors, yellow represents network hubs, light blue represents module hubs, purple represents connectors and dark gray represents peripherals.
Table 2. Topological features of the microeukaryotic co-existence networks in acidic bulk, acidic rhizosphere, alkaline bulk, and alkaline rhizosphere soils.
To identify the relative roles of the nodes in the networks, z and c scores were calculated for them for each soil type. Module hubs and connectors were frequently found in the four networks. However, a network hub was only found in the acidic bulk soil. Additionally, the acidic rhizosphere soil network harbored fewer connectors than the other three networks.
Natural connectivity analysis is a powerful method of investigating network robustness, which can, in turn, reflect network stability. Accordingly, the robustness of the four ecological networks was tested by altering the amplitude of natural connectivity via the deletion of nodes and edges (Figure 6). The results revealed that the rhizosphere community was more stable than the bulk soil community in alkaline soil. Meanwhile, the opposite trend was observed in acidic soil. This indicated that the adaptation mechanism of the microeukaryotes varied according to the soil pH conditions.
Figure 6. Robustness analysis of the rhizosphere and bulk soil microeukaryotic community networks in acidic and alkaline soils. Robustness is depicted as the relationship between natural connectivity and the proportion of excluded (A) nodes and (B) edges.
To uncover the importance of the lineage bins in the networks, the bins were correlated to nodes in the network through ASVs ID. Finally, it was found that the five bins that were most influenced by each assembly process did not display important roles in the network (Table 3). For example, Bin1 was identified as peripheral in acidic bulk, acidic rhizosphere and alkaline rhizosphere soil, and only displayed connector function in alkaline bulk soil. However, the bins that were less influenced by the assembly processes than the top five bins, occupied important positions in the network such as module hubs and connectors. This suggested that the bins most tightly linked to the assembly processes may not play critical roles in the ecological network.
Table 3. The roles of different iCAMP microeukaryotic lineage bins within the microeukaryotic co-existence networks.
The spatial distribution, assembly processes, and co-existence networks of microeukaryotic community were revealed in the earing and flowering stage of wheat (late April), which is crucial for microbes (Roesti et al., 2006). Generally, fungi was the dominant microeukaryotic group in the soil, and it was also evident in many studies (Anderson et al., 2003; Tedersoo et al., 2014; Shi et al., 2019a). Our findings revealed that the pH of the soil displayed a stronger effect on the soil microeukaryotic community composition than niche differences (bulk vs. rhizosphere). This indicated the powerful regulation function of soil pH in nutrients storing and supplying (Slessarev et al., 2016), which in turn presented a strong effect on microbes. Therefore, the analyses of assembly processes and co-existence networks were firstly grouped by acidity and alkalinity, and then divided by bulk and rhizosphere. Generally, DR was the dominant process driving microeukaryotic community assembly across all soils. Additionally, the contribution of the different assembly processes in each bin varied according to the soil pH and niche environment, reflecting the importance of microbial species corresponding to environment or soil pH conditions. Alkaline soil networks showed greater complexity than acidic soil networks, and the rhizosphere community was more stable than the bulk soil community in alkaline soil. Consistent with our first hypothesis, the soil pH played an important role in driving microeukaryotic community distribution. Using a global set of samples, Aslani et al. (2021) also found that soil pH was a main driver determining soil microeukaryotic community structure. Numerous studies have reported the primary role of soil pH in shaping soil bacterial and fungal distribution patterns (Fierer, 2017). For example, Shen et al. (2013) found that soil pH could drive the spatial distribution of soil bacterial communities with respect to elevation on Changbai Mountain. Lauber et al. (2009) reported that soil pH was a predictor of soil bacterial community structure at the continental scale. More recently, Shi et al. (2018, 2021) revealed that soil bacterial and fungal community structures could both be determined by soil pH in the North China Plain. Using a natural system, significant correlations were found between protist ß diversity and phosphorous (Logares et al., 2018). While, across a broad geographic range, soil protists were found marginally influenced by pH (Bates et al., 2013). Given that soil pH is critical in soil nutrient cycling (Fierer, 2017), both the prokaryotes and eukaryotes are involved in the cycle all the time, therefore the important role of pH on the soil microbial eukaryotes is obvious.
Our results confirmed that drift played a dominant role in controlling microeukaryotic community assembly. These results are consistent with the global-scale investigation of soil microeukaryotes (Aslani et al., 2021). Drift reflects the influence of random demographic variability, including birth, death and migration rates, on the microbial community (Martiny et al., 2006). Drift results in a high dispersal rate, which can homogenize the community and thus form weaker distance—decay patterns (Vellend, 2010). Some studies found drift process was important for microeukaryotes (Orrock and Watling, 2010; Powell et al., 2015; Logares et al., 2018; Fodelianakis et al., 2021), but not for prokaryotes. Here, we speculated that compared to bacteria, fungi or protist species have larger body size, which is positively correlated with the proportion of the drift (Aslani et al., 2021). On the other hand, larger body size species which present higher birth or death rate will be shown as higher proportion of drift in ecological process (Logares et al., 2018). Different from prokaryotes such as bacteria with lighter mass and smaller body size (Smith et al., 2013), for the fungi and protists, dispersal limitation presents stronger effect on their assembly process due to their low mobility. In our study, contrasting microeukaryotic community assembly patterns were found in alkaline soil and acidic soils. Possibly, the reason is that the filtering effect of plant roots in alkaline soils is stronger than that in acidic soils (Fan et al., 2017; Nuccio et al., 2020). This suggested that the niche environment and soil pH condition jointly affected soil microeukaryotic community assembly in the agricultural ecosystem, indicating the importance of habitats and environments in mediating assembly process of soil microbes.
The results of the present study showed that the microeukaryotic networks of the acidic soils were less complex than those in the alkaline soils, indicating the importance of soil pH in determining the microbial association network. Furthermore, the stability, which was represented by the robustness, was greater in alkaline soil than in acidic soils. These findings suggest that the capacity of a soil microeukaryotic community to maintain stability was not independent of its complexity, but also related to the soil pH conditions. Under permafrost conditions, Wu et al. (2021) also found that microbial (including bacteria and fungi) network complexity was associated with community stability. However, they found an opposing trend; the greater the complexity, the lower the stability. An explanation for these conflicting results could be that the larger body size of the microeukaryotes means that they respond differently from soil bacteria and fungi to their environments (Aslani et al., 2021). Indeed, wheat rhizosphere soil has previously been found to harbor a less complex community and more stable microbial association network in North China Plain soils (Fan et al., 2018). This could be due to the stronger filtering effect of rhizosphere which has stronger ability in recruiting beneficial microbes, and thus builds more stable environment (Thebault and Fontaine, 2010; de Vries et al., 2020). In this study, although we found high abundance of fungal sequences in the soil and few other microbial eukaryotic species, all microbial eukaryotic sequences were analysed for diversity, community characteristics and network associations. Compare to other microeukaryotes, higher abundance fungi contributes a great deal to the ecological functions (e.g., litter decomposition, carbon and nitrogen cycling) (Tedersoo et al., 2014). Our findings provide the first insight into microeukaryotic community (mainly fungi) complexity and stability, and the relationship between them, in agricultural ecosystems.
Our results indicated the critical role of soil pH in determining community distribution patterns, assembly processes and co-existence networks of soil microeukaryotes in wheat fields of the North China Plain. Furthermore, we identified the dominant role of drift in controlling microeukaryotic community assembly. Finally, we found greater microeukaryotic community complexity was associated with a more stable community in crop rhizosphere. These findings broaden our understanding about the important influence of soil pH in soil microbes from procaryotic to eukaryotic microbes, which has implications for microbial functioning under various agricultural practices.
The datasets presented in this study can be found in online repositories. The names of the repository/repositories and accession number(s) can be found below: www.ncbi.nlm.nih.gov/sra/, SRP347607.
HC designed the study. YS, MX, YZ, and LC performed the research and analyzed the data. YS and HC wrote, edited, and finalized the manuscript. All authors contributed to the article and approved the submitted version.
This work was supported by the National Natural Science Foundation of China (42077053), the National Key Research and Development Program of China (2021YFD1900100), and the Natural Science Foundation of Henan (222300420035 and 2017YFD0200604).
The authors declare that the research was conducted in the absence of any commercial or financial relationships that could be construed as a potential conflict of interest.
All claims expressed in this article are solely those of the authors and do not necessarily represent those of their affiliated organizations, or those of the publisher, the editors and the reviewers. Any product that may be evaluated in this article, or claim that may be made by its manufacturer, is not guaranteed or endorsed by the publisher.
We thank Xiao Fu for her assistance in soil sampling and laboratory analyses.
The Supplementary Material for this article can be found online at: https://www.frontiersin.org/articles/10.3389/fmicb.2022.911116/full#supplementary-material
Albert, R., Jeong, H., and Barabasi, A. L. (2000). Error and attack tolerance of complex networks. Nature 406, 378–382. doi: 10.1038/35019019
Amir, A., McDonald, D., Navas-Molina, J. A., Kopylova, E., Morton, J. T., and Zech Xu, Z. (2017). Deblur rapidly resolves single-nucleotide community sequence patterns. mSystems 2, e00191–16. doi: 10.1128/mSystems.00191-16
Anderson, I. C., Campbell, C. D., and Prosser, J. I. (2003). Potential bias of fungal 18S rDNA and internal transcribed spacer polymerase chain reaction primers for estimating fungal biodiversity in soil. Environ. Microbiol. 5, 36–47. doi: 10.1046/j.1462-2920.2003.00383.x
Aslani, F., Geisen, S., Ning, D., Tedersoo, L., Bahram, M., and de Vries, F. (2021). Towards revealing the global diversity and community assembly of soil eukaryotes. Ecol. Lett. 25, 65–76. doi: 10.1111/ele.13904
Bates, S. T., Clemente, J. C., Flores, G. E., Walters, W. A., Parfrey, L. W., Knight, R., et al. (2013). Global biogeography of highly diverse protistan communities in soil. ISME J. 7, 652–659. doi: 10.1038/ismej.2012.147
Bolyen, E., Rideout, J. R., and Dillon, M. R. (2019). Reproducible, interactive, scalable and extensible microbiome data science using QIIME 2. Nat. Biotechnol. 37, 1091–1091. doi: 10.1038/s41587-019-0209-9
Cardinale, M., Grube, M., Erlacher, A., Quehenberger, J., and Berg, G. (2015). Bacterial networks and co-occurrence relationships in the lettuce root microbiota. Environ. Microbiol 17, 239–252. doi: 10.1111/1462-2920.12686
Coux, C., Rader, R., Bartomeus, I., and Tylianakis, J. M. (2016). Linking species functional roles to their network roles. Ecol. Lett. 19, 762–770. doi: 10.1111/ele.12612
Crowther, T. W., van den Hoogen, J., Wan, J., Mayes, M. A., Keiser, A. D., Mo, L., et al. (2019). The global soil community and its influence on biogeochemistry. Science 365:772. doi: 10.1126/science.aav0550
de Vries, F. T., Griffiths, R. I., Knight, C. G., Nicolitch, O., and Williams, A. (2020). Harnessing rhizosphere microbiomes for drought-resilient crop production. Science 368, 270–274. doi: 10.1126/science.aaz5192
Delgado-Baquerizo, M., Oliverio, A. M., Brewer, T. E., Benavent-Gonzalez, A., Eldridge, D. J., Bardgett, R. D., et al. (2018). A global atlas of the dominant bacteria found in soil. Science 359:320. doi: 10.1126/science.aap9516
Delgado-Baquerizo, M., Reich, P. B., Trivedi, C., Eldridge, D. J., Abades, S., Alfaro, F. D., et al. (2020). Multiple elements of soil biodiversity drive ecosystem functions across biomes. Nat. Ecol. Evol. 4, 210–220. doi: 10.1038/s41559-019-1084-y
Dini-Andreote, F., Stegen, J. C., van Elsas, J. D., and Salles, J. F. (2015). Disentangling mechanisms that mediate the balance between stochastic and deterministic processes in microbial succession. Proc. Natl. Acad. Sci. U.S.A. 112, E1326–E1332. doi: 10.1073/pnas.1414261112
Donn, S., Kirkegaard, J. A., Perera, G., Richardson, A. E., and Watt, M. (2015). Evolution of bacterial communities in the wheat crop rhizosphere. Environ. Microbiol 17, 610–621. doi: 10.1111/1462-2920.12452
Fan, K. K., Cardona, C., Li, Y. T., Shi, Y., Xiang, X. J., and Shen, C. C. (2017). Rhizosphere-associated bacterial network structure and spatial distribution differ significantly from bulk soil in wheat crop fields. Soil Biol. Biochem. 113, 275–284. doi: 10.1016/j.soilbio.2017.06.020
Fan, K. K., Weisenhorn, P., Gilbert, J. A., and Chu, H. Y. (2018). Wheat rhizosphere harbors a less complex and more stable microbial co-occurrence pattern than bulk soil. Soil Biol. Biochem. 125, 251–260. doi: 10.1016/j.soilbio.2018.07.022
Faust, K., and Raes, J. (2012). Microbial interactions: from networks to models. Nat. Rev. Microbiol. 10, 538–550. doi: 10.1038/nrmicro2832
Faust, K., Lima-Mendez, G., Lerat, J. S., Sathirapongsasuti, J. F., Knight, R., Huttenhower, C., et al. (2015). Cross-biome comparison of microbial association networks. Front. Microbiol. 6:1200. doi: 10.3389/fmicb.2015.01200
Feng, M. M., Adams, J. M., Fan, K. K., Shi, Y., Sun, R. B., Wang, D. Z., et al. (2018). Long-term fertilization influences community assembly processes of soil diazotrophs. Soil Biol. Biochem. 126, 151–158. doi: 10.1016/j.soilbio.2018.08.021
Feng, Y., Chen, R., Stegen, J. C., Guo, Z., Zhang, J., Li, Z., et al. (2018). Two key features influencing community assembly processes at regional scale: initial state and degree of change in environmental conditions. Mol. Ecol. Resour. 27, 5238–5251. doi: 10.1111/mec.14914
Fierer, N. (2017). Embracing the unknown: disentangling the complexities of the soil microbiome. Nat. Rev. Microbiol. 15, 579–590. doi: 10.1038/nrmicro.2017.87
Fierer, N., and Jackson, R. B. (2006). The diversity and biogeography of soil bacterial communities. Proc. Natl. Acad. Sci. U.S.A. 103, 626–631. doi: 10.1073/pnas.0507535103
Fodelianakis, S., Valenzuela-Cuevas, A., Barozzi, A., and Daffonchio, D. (2021). Direct quantification of ecological drift at the population level in synthetic bacterial communities. ISME J. 15, 55–66. doi: 10.1038/s41396-020-00754-4
Giner, C. R., Balague, V., Krabberod, A. K., Ferrera, I., Rene, A., Garces, E., et al. (2018). Quantifying long-term recurrence in planktonic microbial eukaryotes. Mol. Ecol. 28, 923–935. doi: 10.1111/mec.14929
Jeong, S. J., Ho, C. H., Piao, S. L., Kim, J., Ciais, P., Lee, Y. B., et al. (2014). Effects of double cropping on summer climate of the North China Plain and neighbouring regions. Nat. Clim. Chang. 4, 615–619. doi: 10.1038/NCLIMATE2266
Jiao, S., and Lu, Y. H. (2020). Soil pH and temperature regulate assembly processes of abundant and rare bacterial communities in agricultural ecosystems. Environ. Microbiol. 22, 1052–1065. doi: 10.1111/1462-2920.14815
Jordan, F. (2009). Keystone species and food webs. Philos Trans. R. Soc. B Biol. Sci. 364, 1733–1741. doi: 10.1098/rstb.2008.0335
Lauber, C. L., Hamady, M., Knight, R., and Fierer, N. (2009). Pyrosequencing-based assessment of soil pH as a predictor of soil bacterial community structure at the continental scale. Appl. Environ. Microbiol. 75, 5111–5120. doi: 10.1128/AEM.00335-09
Li, E., de Jonge, R., Liu, C., Jiang, H., Friman, V.-P., Pieterse, C. M. J., et al. (2021). Rapid evolution of bacterial mutualism in the plant rhizosphere. Nat. Commun 12:3829. doi: 10.1038/s41467-021-24005-y
Lima-Mendez, G., Faust, K., Henry, N., Decelle, J., Colin, S., Carcillo, F., et al. (2015). Determinants of community structure in the global plankton interactome. Science 348:6237. doi: 10.1126/science.1262073
Liu, Y. R., Eldridge, D. J., Zeng, X. M., Wang, J., Singh, B. K., and Delgado-Baquerizo, M. (2021). Global diversity and ecological drivers of lichenised soil fungi. New Phytol. 231, 1210–1219. doi: 10.1111/nph.17433
Logares, R., Tesson, S. V., Canback, B., Pontarp, M., Hedlund, K., and Rengefors, K. (2018). Contrasting prevalence of selection and drift in the community structuring of bacteria and microbial eukaryotes. Environ. Microbiol. 20, 2231–2240. doi: 10.1111/1462-2920.14265
Ma, B., Wang, Y. L., Ye, S. D., Liu, S., Stirling, E., and Gilbert, J. A., et al. (2020). Earth microbial co-occurrence network reveals interconnection pattern across microbiomes. Microbiome 8:82. doi: 10.1186/s40168-020-00857-2
Ma, B., Wang, H., Dsouza, M., Lou, J., He, Y., Dai, Z., et al. (2016). Geographic patterns of co-occurrence network topological features for soil microbiota at continental scale in eastern China. ISME J. 10, 1891–1901. doi: 10.1038/ismej.2015.261
Martiny, J. B. H., Bohannan, B. J. M., Brown, J. H., Colwell, R. K., Fuhrman, J. A., and Green, J. L. (2006). Microbial biogeography: putting microorganisms on the map. Nat. Rev. Microbiol. 4, 102–112. doi: 10.1038/nrmicro1341
May, M.R. (1973). Stability and Complexity in Model Ecosystems. Princeton, NJ: Princeton University Press.
Neutel, A. M., Heesterbeek, J. A. P., and de Ruiter, P. C. (2002). Stability in real food webs: weak links in long loops. Science 296, 1120–1123. doi: 10.1126/science.1068326
Ning, D., Yuan, M., Wu, L., Zhang, Y., Guo, X., and Zhou, X. (2020). A quantitative framework reveals ecological drivers of grassland microbial community assembly in response to warming. Nat. Commun. 11:4717. doi: 10.1038/s41467-020-18560-z
Nuccio, E. E., Starr, E., Karaoz, U., Brodie, E. L., Zhou, J., Tringe, S., et al. (2020). Niche differentiation is spatially and temporally regulated 1 in the rhizosphere. ISME J. 14, 999–1014. doi: 10.1038/s41396-019-0582-x
Oliverio, A. M., Geisen, S., Delgado-Baquerizo, M., Maestre, F. T., Turner, B. L., and Fierer, N. (2020). The global-scale distributions of soil protists and their contributions to belowground systems. Sci. Adv. 6:eaax8787. doi: 10.1126/sciadv.aax8787
Orrock, J. L., and Watling, J. I. (2010). Local community size mediates ecological drift and competition in metacommunities. Proc. Biol. Sci 277, 2185–2191. doi: 10.1098/rspb.2009.234
Peng, G. S., and Wu, J. (2016). Optimal network topology for structural robustness based on natural connectivity. Phys. A Stat. Mech. Appl. 443, 212–220. doi: 10.1016/j.physa.2015.09.023
Piao, S., Ciais, P., Huang, Y., Shen, Z., Peng, S., Li, J., et al. (2010). The impacts of climate change on water resources and agriculture in China. Nature 467, 43–51. doi: 10.1038/nature09364
Pineda, A., Kaplan, I., and Bezemer, T. M. (2017). Steering soil microbiomes to suppress aboveground insect pests. Trends Plant. Sci. 22, 770–778. doi: 10.1016/j.tplants.2017.07.002
Powell, J. R., Karunaratne, S., Campbell, C. D., Yao, H., Robinson, L., and Singh, B. K. (2015). Deterministic processes vary during community assembly for ecologically dissimilar taxa. Nat. Commun 6:8444. doi: 10.1038/ncomms9444
Roesti, D., Gaur, R., Johri, B. N., Imfeld, G., Sharma, S., Kawaljeet, K., et al. (2006). Plant growth stage, fertiliser management and bio-inoculation of arbuscular mycorrhizal fungi and plant growth promoting rhizobacteria affect the rhizobacterial community structure in rain-fed wheat fields. Soil Biol. Biochem. 38, 1111–1120. doi: 10.1016/j.soilbio.2005.09.010
Rousk, J., Baath, E., Brookes, P. C., Lauber, C. L., Lozupone, C., Caporaso, J. G., et al. (2010). Soil bacterial and fungal communities across a pH gradient in an arable soil. ISME J. 4, 1340–1351. doi: 10.1038/ismej.2010.58
Shen, C. C., Xiong, J. B., Zhang, H. Y., Feng, Y. Z., Lin, X. G., Li, X. Y., et al. (2013). Soil pH drives the spatial distribution of bacterial communities along elevation on Changbai Mountain. Soil Biol. Biochem. 57, 204–211. doi: 10.1016/j.soilbio.2012.07.013
Shi, Y., Dang, K. K., Dong, Y. H., Feng, M. M., Wang, B. R., Li, J. G., et al. (2019a). Soil fungal community assembly processes under long-term fertilization. Eur. J. Soil. Sci 71, 716–726. doi: 10.1111/ejss.12902
Shi, Y., Fan, K., Li, Y., Yang, T., He, J.-S., and Chu, H. (2019b). Archaea enhance the robustness of microbial co-occurrence networks in tibetan plateau soils. Soil. Sci. Soc. Am. J. 83, 1093–1099. doi: 10.2136/sssaj2018.11.0426
Shi, Y., Delgado-Baquerizo, M., Li, Y., Yang, Y., Zhu, Y.-G., Peñuelas, J., et al. (2020). Abundance of kinless hubs within soil microbial networks are associated with high functional potential in agricultural ecosystems. Environ. Int. 142:105869. doi: 10.1016/j.envint.2020.105869
Shi, Y., Li, Y. T., Xiang, X. J., Sun, R. B., Yang, T., He, D., et al. (2018). Spatial scale affects the relative role of stochasticity versus determinism in soil bacterial communities in wheat fields across the North China Plain. Microbiome 6:27. doi: 10.1186/s40168-018-0409-4
Shi, Y., Li, Y., Yang, T., and Chu, H. (2021). Threshold effects of soil pH on microbial co-occurrence structure in acidic and alkaline arable lands. Sci. Total Environ. 800:149592. doi: 10.1016/j.scitotenv.2021.149592
Slessarev, E. W., Lin, Y., Bingham, N. L., Johnson, J. E., Dai, Y., Schimel, J. P., et al. (2016). Water balance creates a threshold in soil pH at the global scale. Nature 540, 567–569. doi: 10.1038/nature20139
Smith, D. J., Timonen, H. J., Jaffe, D. A., Griffin, D. W., Birmele, M. N., Perry, K. D., et al. (2013). Intercontinental dispersal of bacteria and archaea by transpacific winds. Appl. Environ. Microbiol. 79, 1134–1139. doi: 10.1128/AEM.03029-12
Soudzilovskaia, N. A., van Bodegom, P. M., Terrer, C., Zelfde, M. V., McCallum, I., Luke McCormack, M., et al. (2019). Global mycorrhizal plant distribution linked to terrestrial carbon stocks. Nat. Commun. 10:5077. doi: 10.1038/s41467-019-13019-2
Stegen, J. C., Freestone, A. L., Crist, T. O., Anderson, M. J., Chase, J. M., Comita, L. S., et al. (2013). Stochastic and deterministic drivers of spatial and temporal turnover in breeding bird communities. Glob. Ecol. Biogeogr. 22, 202–212. doi: 10.1111/j.1466-8238.2012.00780.x
Tedersoo, L., Bahram, M., Polme, S., Koljalg, U., Yorou, N. S., Wijesundera, R., et al. (2014). Global diversity and geography of soil fungi. Science 346:1078. doi: 10.1126/science.1256688
Thebault, E., and Fontaine, C. (2010). Stability of ecological communities and the architecture of mutualistic and trophic networks. Science 329, 853–856. doi: 10.1126/science.1188321
Vellend, M. (2010). Conceptual synthesis in community ecology. Q. Rev. Biol. 85, 183–206. doi: 10.1086/652373
Weiss, S., Van Treuren, W., Lozupone, C., Faust, K., Friedman, J., and Deng, Y. (2016). Correlation detection strategies in microbial data sets vary widely in sensitivity and precision. ISME J. 10, 1669–1681. doi: 10.1038/ismej.2015.235
Whitaker, R. J., Grogan, D. W., and Taylor, J. W. (2003). Geographic barriers isolate endemic populations of hyperthermophilic archaea. Science 301, 976–978. doi: 10.1126/science.1086909
Wu, M. H., Chen, S. Y., Chen, J. W., Xue, K., Chen, S. L., Wang, X. M., et al. (2021). Reduced microbial stability in the active layer is associated with carbon loss under alpine permafrost degradation. Proc. Natl. Acad. Sci. U.S.A. 118:e2025321118. doi: 10.1073/pnas.2025321118
Xiong, W., Li, R., Guo, S., Karlsson, I., Jiao, Z. X., Xun, W. B., et al. (2019). Microbial amendments alter protist communities within the soil microbiome. Soil Biol. Biochem. 135, 379–382. doi: 10.1016/j.soilbio.2019.05.025
Xiong, W., Song, Y., Yang, K., Gu, Y., Wei, Z., and Kowalchuk, G. A. (2020). Rhizosphere protists are key determinants of plant health. Microbiome 8:27. doi: 10.1186/s40168-020-00799-9
Yang, T., Tedersoo, L., Soltis, P. S., Soltis, D. E., Gilbert, J. A., Sun, M., et al. (2019). Phylogenetic imprint of woody plants on the soil mycobiome in natural mountain forests of eastern China. ISME J. 13, 686–697. doi: 10.1038/s41396-018-0303-x
Zhang, B., Zhang, J., Liu, Y., Shi, P., and Wei, G. (2018). Co-occurrence patterns of soybean rhizosphere microbiome at a continental scale. Soil Biol. Biochem. 118, 178–186. doi: 10.1016/j.soilbio.2017.12.011
Zhang, L., Zhou, J., George, T. S., Limpens, E., and Feng, G. (2022). Arbuscular mycorrhizal fungi conducting the hyphosphere bacterial orchestra. Trends Plant. Sci. 27, 402–411. doi: 10.1016/j.tplants.2021.10.008
Zhang, W. J., Pan, Y. B., Yang, J., Chen, H. H., Holohan, B., Vaudrey, J., et al. (2018). The diversity and biogeography of abundant and rare intertidal marine microeukaryotes explained by environment and dispersal limitation. Environ. Microbiol 20, 462–476. doi: 10.1111/1462-2920.13916
Zhou, J. Z., Deng, Y., Zhang, P., Xue, K., Liang, Y. T., Van Nostrand, J. D., et al. (2014). Stochasticity, succession, and environmental perturbations in a fluidic ecosystem. Proc. Natl. Acad. Sci.U.S.A. 111, E836–E845. doi: 10.1073/pnas.1324044111
Zhou, J., Kang, S., Schadt, C. W., and Garten, C. T. (2008). Spatial scaling of functional gene diversity across various microbial taxa. Proc. Natl. Acad. Sci. U.S.A. 105, 7768–7773. doi: 10.1073/pnas.0709016105
Keywords: microeukaryote, soil pH, community assembly, co-occurrence network, North China Plain, rhizosphere
Citation: Shi Y, Xu M, Zhao Y, Cheng L and Chu H (2022) Soil pH Determines the Spatial Distribution, Assembly Processes, and Co-existence Networks of Microeukaryotic Community in Wheat Fields of the North China Plain. Front. Microbiol. 13:911116. doi: 10.3389/fmicb.2022.911116
Received: 02 April 2022; Accepted: 30 May 2022;
Published: 25 July 2022.
Edited by:
Saowaluck Tibpromma, Qujing Normal University, ChinaReviewed by:
Juntao Wang, Western Sydney University, AustraliaCopyright © 2022 Shi, Xu, Zhao, Cheng and Chu. This is an open-access article distributed under the terms of the Creative Commons Attribution License (CC BY). The use, distribution or reproduction in other forums is permitted, provided the original author(s) and the copyright owner(s) are credited and that the original publication in this journal is cited, in accordance with accepted academic practice. No use, distribution or reproduction is permitted which does not comply with these terms.
*Correspondence: Haiyan Chu, aHljaHVAaXNzYXMuYWMuY24=
Disclaimer: All claims expressed in this article are solely those of the authors and do not necessarily represent those of their affiliated organizations, or those of the publisher, the editors and the reviewers. Any product that may be evaluated in this article or claim that may be made by its manufacturer is not guaranteed or endorsed by the publisher.
Research integrity at Frontiers
Learn more about the work of our research integrity team to safeguard the quality of each article we publish.