- 1State Key Laboratory of Aridland Crop Science, Gansu Agricultural University, Lanzhou, China
- 2College of Agronomy, Gansu Agricultural University, Lanzhou, China
- 3State Key Laboratory of Soil and Sustainable Agriculture, Institute of Soil Science, Chinese Academy of Sciences, Nanjing, China
- 4Department of Water Resources and Sustainable Development, The University of Environment and Sustainable Development, Somanya, Ghana
- 5Institute of Molecular Biology and Biotechnology, The University of Lahore, Lahore, Pakistan
Denitrifying bacteria produce and utilize nitrous oxide (N2O), a potent greenhouse gas. However, there is little information on how organic fertilization treatments affect the denitrifying communities and N2O emissions in the semi-arid Loess Plateau. Here, we evaluated how the denitrifying communities are responsible for potential denitrification activity (PDA) and N2O emissions. A field experiment was conducted with five fertilization treatments, including no fertilization (CK), mineral fertilizer (MF), mineral fertilizer plus commercial organic fertilizer (MOF), commercial organic fertilizer (OFP), and maize straw (MSP). Our result showed that soil pH, soil organic carbon (SOC), and dissolved organic nitrogen (DON) were significantly increased under MSP treatment compared to MF treatment, while nitrate nitrogen (NO3−−N) followed the opposite trend. Organic fertilization treatments (MOF, OFP, and MSP treatments) significantly increased the abundance and diversity of nirS- and nosZ-harboring denitrifiers, and modified the community structure compared to CK treatment. The identified potential keystone taxa within the denitrifying bacterial networks belonged to the distinct genera. Denitrification potentials were significantly positively correlated with the abundance of nirS-harboring denitrifiers, rather than that of nirK- and nosZ-harboring denitrifiers. Random forest modeling and structural equation modeling consistently determined that the abundance, community composition, and network module I of nirS-harboring denitrifiers may contribute significantly to PDA and N2O emissions. Collectively, our findings highlight the ecological importance of the denitrifying communities in mediating denitrification potentials and the stimulatory impact of organic fertilization treatments on nitrogen dynamics in the semi-arid Loess Plateau.
Introduction
Climate change mitigation is essential for carbon-neutral agriculture and food security (Zhang et al., 2020). Nitrous oxide (N2O) is a potential greenhouse gas, contributing to stratospheric ozone layer depletion (Cuello et al., 2015). Agricultural soils account for nearly 55% of global N2O emissions in 2015, and this percentage is projected to increase to 59% by 2030 (Hu et al., 2015). The transformations of nitrogenous compounds by soil denitrifying bacteria with excess fertilizer application is one of the main sources of N2O emissions (Tang et al., 2019).
During denitrification processes, nitrate (NO3−) is converted to nitrite (NO2−), nitric oxide (NO), N2O, and finally to dinitrogen (N2). These conversions are facilitated by nitrate reductase (NAR), nitrite reductase (NIR), nitric oxide reductase (NOR), and nitrous oxide reductase (NOS), respectively (Cui et al., 2016; Azziz et al., 2017). Nitrate reductase reduces NO2 to NO, a rate-limiting step of denitrification encoded by the copper-containing reductase gene (nirK) and cytochrome cd1 nitrite reductase gene (nirS) (Yoshida et al., 2009; Jang et al., 2018). Nitrous oxide reductase is encoded by nosZ gene, and functions in reducing N2O emissions (Yang et al., 2018). These functional genes are appropriate for studying the changes in nirK-, nirS-, and nosZ-harboring denitrifiers under different fertilization regimes (Yu et al., 2018; Chen et al., 2020).
Organic fertilization treatments considerably affect the abundance, diversity, and structure of the denitrifying communities, but their effects are not consistent (Ouyang et al., 2018). For instance, organic fertilizers have no significant effect on the abundance of denitrifiers (Yin et al., 2014), while the combined application of organic and mineral fertilizers increases the abundance of nirS- and nosZ-harboring denitrifiers and reduces N2O emissions (Zhang et al., 2015; Shi et al., 2019). The incorporation of crop straw increases soil organic carbon (SOC) and dissolved organic nitrogen (DON), and improves the abundance of nirS-harboring denitrifiers in alkaline soils (Huang et al., 2019). The changes in soil factors can differentially influence the nirK-, nirS-, and nosZ-harboring denitrifiers, thereby affecting their contributions to N2O emissions (Cui et al., 2016; Xiong et al., 2017). The network-based analytical approach is a powerful approach to investigate microbial associations and identify potential keystone taxa in the complex bacterial community (Barberán et al., 2012). Keystone taxa have highly linked functional features and greatly explain network structure. They are critical for minimizing community fragmentation and maintaining microbial community functioning (Williams et al., 2014; Herren and McMahon, 2018). Although the co-occurrence network of nirK-, nirS- and nosZ-harboring denitrifiers has recently been investigated in natural forest soil and arable black soil (Chen et al., 2019a; Yang et al., 2020b), little is known about the impact of organic fertilization treatments on denitrifying bacterial networks in the semi-arid loess plateau.
A growing body of studies has recently examined the effects of organic amendments on N2O emissions (Pang et al., 2019; Yang et al., 2020a). However, there is still limited knowledge on the mechanisms of the denitrifying communities in driving N2O emission in agroecosystems. It is critical to uncover the associations between the denitrifying bacterial communities and denitrification in the semi-arid loess plateau. For this purpose, we conducted a field experiment with the objectives to (1) evaluate soil properties, and the abundance, structure and co-occurrence network of nirK, nirS, and nosZ-harboring denitrifiers in response to fertilization treatments; (2) investigate the effect of organic fertilization treatments on the co-occurrence network of denitrifying communities; and (3) explore the relationships of soil properties and the denitrifying community with N2O emissions. We hypothesized that organic fertilization treatments would significantly improve the abundance and diversity of denitrifying communities, and alter the structure of denitrifying community by improving SOC and nutrient availability. We expected close links between the denitrifying community and N2O emissions across fertilization treatments.
Materials and methods
Experimental site and description of treatments
The field experiment was performed at the experimental station of Gansu Agricultural University in Dingxi, northwestern China (35°28′N, 104°44′E). The study area has a semi-arid environment with an annual frost-free period of 140 days and an average elevation of 2,000 m. The average annual rainfall in this area is 390 mm, with most rain falling between July and September. Calcaric cambisol is the aeolian soil type at this site, with a sandy loam texture. The tested soil has a pH value of 8.7, SOC content of 8.52 g kg−1, total nitrogen (TN) content of 0.93 g kg−1, and available phosphorus (AP) content of 15.3 mg kg−1. The maximum and minimum temperature of this site were 38°C in July and −22°C in January.
The experiment was started in 2012, including five treatments arranged in a completely randomized design with three replicates. The five fertilization treatments were: (i) no fertilization (CK); (ii) mineral fertilizer (MF) contained 200 kg N ha−1 of urea and 150 kg P2O5 ha−1 of triple superphosphate; (iii) mineral fertilizer plus commercial organic fertilizer (MOF) contained 3.03 t ha−1 of organic commercial fertilizer, 100 kg N ha−1 of urea, and 120 kg P2O5 ha−1 of triple superphosphate; (iv) Organic fertilizer (OFP) contained 6.06 t ha−1 of commercial organic fertilizer, and 90 kg P2O5 ha−1 of triple superphosphate; and (v) maize straw (MSP) contained 28.5 t ha−1 combined with triple superphosphate of 36 kg ha−1. In the spring, all fertilizers were spread evenly on the soil surface. The experimental plots were 13 m long and 3.3 m wide, with alternating narrow (15 cm high × 40 cm wide) and wide (10 cm high × 70 cm wide) ridges (Supplementary Figure S1). All ridges were covered with plastic film to increase soil temperature, reduce evaporative losses, and promote plant productivity. The seeds of maize (cultivar Pioneer 335) were sown at a density of 52,500 plants ha−1 in late April and harvested in October. Weeding was done manually between sowing and harvesting.
Soil sampling and analysis
Soil samples were collected using a soil auger (5 cm diameter) at the flowering stage of maize in 2019. Five soil cores (0–20 cm) were collected along a zigzag line in each plot, and carefully mixed to obtain a composite sample. Soil samples were placed on dry ice and immediately transported to the laboratory. The soil samples were sieved at 2 mm to remove stones and roots. Each soil sample was divided into two halves, one kept at 4°C for chemical analysis and the other at −80°C for molecular analysis.
Soil pH was measured with a glass electrode in a 1:2.5 soil/water solution. SOC was determined by the oxidation of organic C with potassium dichromate (Nelson and Sommers, 1996). TN was measured using the CN elemental analyzer (LECO, Stockport, United Kingdom). Ammonia nitrogen (NH4+ − N) and nitrate nitrogen (NO3−−N) were extracted with 2 M KCl, and determined using a flow injection auto analyzer (FLA star 5000 analyzers, Foss, Denmark; Bremner, 1996). Dissolved organic nitrogen (DON) was extracted by 0.5 M K2SO4 and detected using a Multi N/C 2100 analyzer (Analytik Jena, Germany; Ghani et al., 2003). Available phosphorus (AP) was determined by colorimetric methods and resin extraction with modification (Olsen et al., 1954). Soil water content was measured by drying at 105°C for 24 h (Lamptey et al., 2019).
Measurement of potential denitrification activity
Frozen soil samples were incubated at 25°C for 3 days. Potential denitrification activity (PDA) was determined using the acetylene inhibition method (Philippot et al., 2011), and was expressed as the N rate of N2O production (ng N2O N g−1 dry soil h−1). Briefly, 25 g of soil was placed in 125 ml plasma flask, and 25 ml of solution containing 10 mM KNO3, 10 mM glucose, and 50 mM K2HPO4. Chloramphenicol (0.1 g l−1) was added to suppress new protein formation. The flasks were evacuated to produce anaerobic conditions and purged with a 90:10 He-C2H2 gas combination to reduce N2O reductase activity. Gas samples were obtained after 0, 15, 30, 45, and 60 min after mixing. N2O concentrations was detected by a gas chromatograph (Agilent GC-7890A) with an electron capture detector.
Measurement of N2O fluxes
Static chamber and gas chromatography were used to measure N2O fluxes. Each closed container (38 cm × 35 cm × 36.5 cm) was designed with a completely opaque covered with a corrugated tin foil to limit the influence of radiant heat during gas sampling. N2O gas samples were collected using a plastic syringe during sampling periods (0, 10, and 20 min after chamber closure) and deposited in an airtight aluminum bag for each sampling period (Dalian Delin gas packing, China). N2O gas samples were collected at 15-day intervals from May to September in 2019. Gas chromatography (Agilent 7890A, United States) was used to analyze the collected gas samples with an electron capture detector.
N2O flux (f) was calculated using the protocol described by Jantalia et al. (2008): f = ρ × (V/A) × (C/t) × [273/(273 + T)], where f is the N2O flux (μg m−2 h−1); ρ is the N2O gas density (kg m−3) at standard temperature and pressure; V is the chamber volume (m3); A is the soil area covered by the chamber (m2); T is the temperature in the chamber (°C); ΔC/Δt is the change in N2O concentration inside the chamber during a given time (μl l−1 h−1). The cumulative N2O emissions (kg ha−1) were calculated using the following equation (Yeboah et al., 2021): M = ∑ (FN + 1 + FN) × 0.5 × (TN + 1 − TN) × 24 × 10−2, where M is the cumulative N2O emissions during the measurement period (kg ha−1); F is N2O (in mg m−2 h−1); N and N + 1 are the sampling emissions from the previous and current sampling; T is the number of days since the initial sampling.
DNA extraction and quantitative polymerase chain reaction
Total DNA was extracted and purified from 0.5 g of fresh soil using the HiPure Soil DNA Mini Kit (Magen, Guangzhou, China). The quantity and purity of DNA were determined using a spectrophotometer (Nanodrop, PeqLab, Germany). The quantitative polymerase chain reaction (qPCR) was performed to detect the copy numbers of nirK, nirS, and nosZ genes using an ABI7500 thermocycler equipment (Applied Biosystems, Foster City, CA, United States). The primers to amplify denitrification gene are listed in Supplementary Table S1. The 20-μl reaction mixture contained 10 μl of SYBR Premix Ex Taq (TaKaRa Biotechnology, Tokyo, Japan), 0.5 μl of each primer (10 mM), 1 μl of DNA template (1–10 ng), and 8 μl of double-distilled water. DNA template was replaced with RNase-free ultrapure water as a control. The standard curves were generated to calculate the absolute abundance of nirK, nirS, and nosZ genes. Plasmids extracted from clones containing any of the target genes (nirK, nirS, and nosZ) were diluted to produce a series of standard templates (102–108 copies). The amplification efficiencies and r2 were >90% and 0.99%, respectively.
Sequencing and processing of functional gene amplicons
DNA sequencing was used to investigate the abundance, diversity, and community structure of nirS, nirK, and nosZ-harboring denitrifiers. A unique 7-bp barcode sequence was added to the forward primers. The concentration of purified products was determined using a TBS-380 fluorometer (Turner Biosystems, CA, United States). The diluted PCR were paired-end sequenced on an Illumina MiSeq sequencer (Shanghai Personal Biotechnology, Co., Ltd., Shanghai, China). Raw sequences were quality screened and low-quality sequences were identified using Quantitative Insights Into Microbial Ecology (QIIME; Caporaso et al., 2010). Usearch was used to screen the chimeric assembled sequences (Edgar et al., 2011). The remaining high-quality sequences were then checked for frameshifts using the FrameBot tool of the Ribosomal Database Project (RDP) FunGene Pipeline (Edgar and Flyvbjerg, 2015). Next, the blastn algorithm compared the bacterial nirK, nirS, and nosZ sequences to the non-redundant nucleotide database GenBank (nt) in the National Center for Biotechnology Information (NCBI). Finally, the operational taxonomic units (OTUs) of each sample were determined by clustering the sequences using the cluster database at high identity with tolerance (CD-HIT-EST) algorithm, which requires a minimum sequence identity of 90% (Li and Godzik, 2006). Alpha diversity indices (Shannon index and Chao1 richness) of each functional gene were calculated using R software (version 3.5.3).
Statistical analysis
The analysis of variance (ANOVA) with Tukey’s HSD test at p < 0.05 and Pearson correlation were performed using SPSS 21.0 (SPSS Inc., Chicago, IL, United States). Redundancy analysis (RDA) was used to evaluate the effects of soil physicochemical properties on the denitrifying communities using “vegan” package in R.
Co-occurrence networks were used to identify the significant taxa associations in the nirK-, nirS-, and nosZ-harboring denitrifiers. The OTUs that occurred in all replicates of each treatment were retained for network analysis. Pearson correlations between all nodes were performed, with the correlation coefficient (r) was >0.7 or < −0.7 and p value was <0.05. We then computed permutation and bootstrap distributions to evaluate the valid of edges with 1,000 iterations. The network was laid out using the Fruchterman–Reingold algorithm via Gephi (version 0.9.2). We calculated topological properties of networks, including the number of nodes and edges, average clustering coefficient, average degree, average path length, closeness centrality, network centrality, and modularity. The OTUs with the higher degree and closeness centrality were considered as potential keystone taxa (Berry and Widder, 2014).
Random forest modeling was used to identify the important predictors of N2O emissions, including soil variables and the denitrifying bacterial communities (Liaw and Wiener, 2002). The predictor importance of the model was quantified by the “A3R” package (Fortmannroe, 2015), and the significance of each predictor was determined by the “rfPermute” package (Archer, 2020). Structural equation modeling (SEM) was used to examine the direct and indirect effect of soil properties and the denitrifying communities on PDA and N2O emission using AMOS 21.0. The data distribution was tested for normality before modeling. The chi-square test (χ2, p > 0.05), root mean square error of approximation (RMSEA), and goodness-of-fit index (GFI) were used to determine the model fitness (Sahoo, 2019).
Results
Soil properties, potential denitrification activity, and N2O emission
Our results showed that soil properties significantly (p < 0.05) changed among fertilization treatments (Table 1). Soil pH ranged from 8.32 to 8.66 across fertilization treatments, and was significantly (p < 0.05) lower under MF treatment than under CK and MSP treatments. The MSP and OFP treatments significantly (p < 0.05) increased SOC and DON compared to MF and CK treatments, and significantly increased TN and AP compared to CK treatment (Table 1). NO3−−N concentration ranged from 17.84 to 30.81 mg kg−1, and was significantly (p < 0.05) increased under MF treatment compared to OFP, MSP, and CK treatments. Soil water content (SWC) was significantly (p < 0.05) higher under MF, MOF, OFP, and MSP treatments than under CK treatment (Table 1).
Potential denitrification activity (PDA) was significantly (p < 0.05) increased by 34.6%, 46.4%, 94.1%, and 60.8% under MF, MOF, OFP, and MSP treatments compared to CK treatment, respectively (Figure 1). Overall, N2O emissions were the highest in July and the lowest in September across fertilization treatments (Figure 2A). Furthermore, N2O emissions were significantly (p < 0.05) higher under OFP, MSP, and MF treatments than under MOF treatment. The cumulative N2O emissions under OFP, MSP, MF, and MOF treatments were significantly (p < 0.05) improved by 39.9%, 33.4%, 76.1%, and 63.4% compared to CK treatment, respectively (Figure 2B).
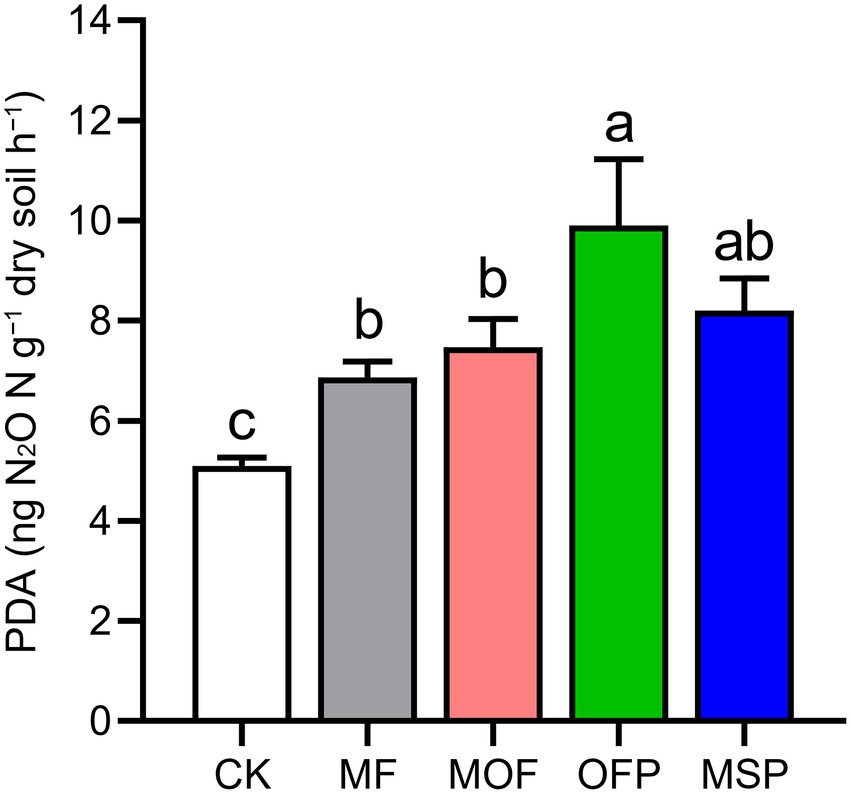
Figure 1. Potential denitrification activity (PDA) under different fertilization treatments. Bars (n = 3) with different lowercase letters indicate significant differences based on Tukey’s HSD test (p < 0.05). CK, No fertilization; MF, mineral fertilizer; MOF, mineral fertilizer plus commercial organic fertilizer; OFP, commercial organic fertilizer; MSP, maize straw.
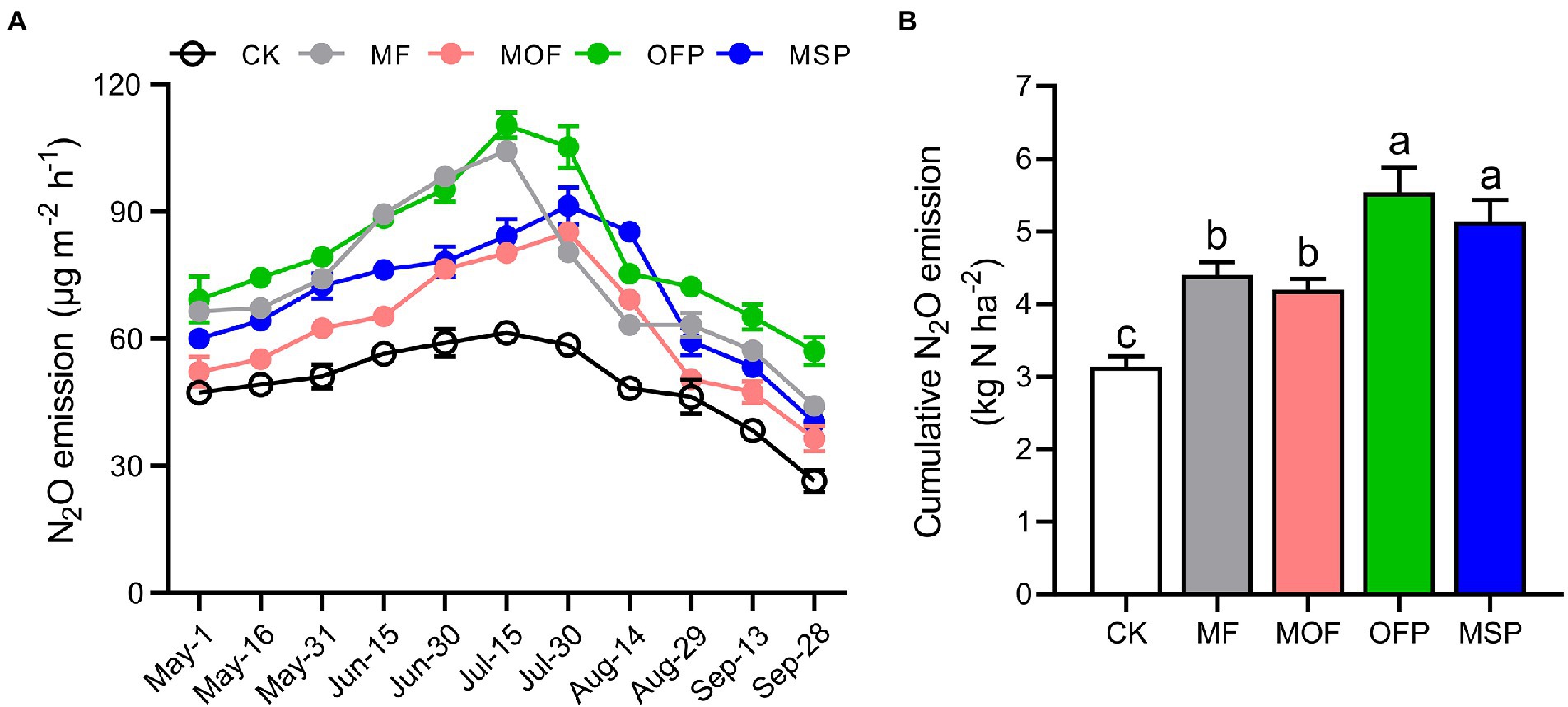
Figure 2. The changes in N2O emission flux (A) and cumulative N2O emission (B) among different fertilization treatments. Bars (n = 3) with different lowercase letters indicate significant differences based on Tukey’s HSD test (p < 0.05). CK, No fertilization; MF, mineral fertilizer; MOF, mineral fertilizer plus commercial organic fertilizer; OFP, commercial organic fertilizer; MSP, maize straw.
Abundance, diversity, and composition of denitrifying communities
The abundance of nirK-harboring denitrifiers indicated by copy number of nirK gene was the highest under MF treatment and the lowest under CK treatment (Figure 3A). The abundance of nirS-harboring denitrifiers were significantly (p < 0.05) higher under OFP and MSP treatments than under CK and MOF treatments (Figure 3B). The abundance of nosZ-harboring denitrifiers under MOF treatment was significantly (p < 0.05) higher than those under CK, MF, OFP, and MSP treatments (Figure 3C). Shannon index and Chao1 richness of the denitrifying bacterial communities were significantly (p < 0.05) altered across fertilization treatments (Table 2), except for Shannon index of nirK-harboring denitrifiers. Shannon index and Chao1 richness of nirS- and nosZ-harboring denitrifiers were significantly (p < 0.05) enhanced under MOF and OFP treatments compared to CK and MF treatments. Shannon index and Chao1 richness of nirK-harboring denitrifiers were significantly (p < 0.05) higher under OFP treatment than under CK treatment.
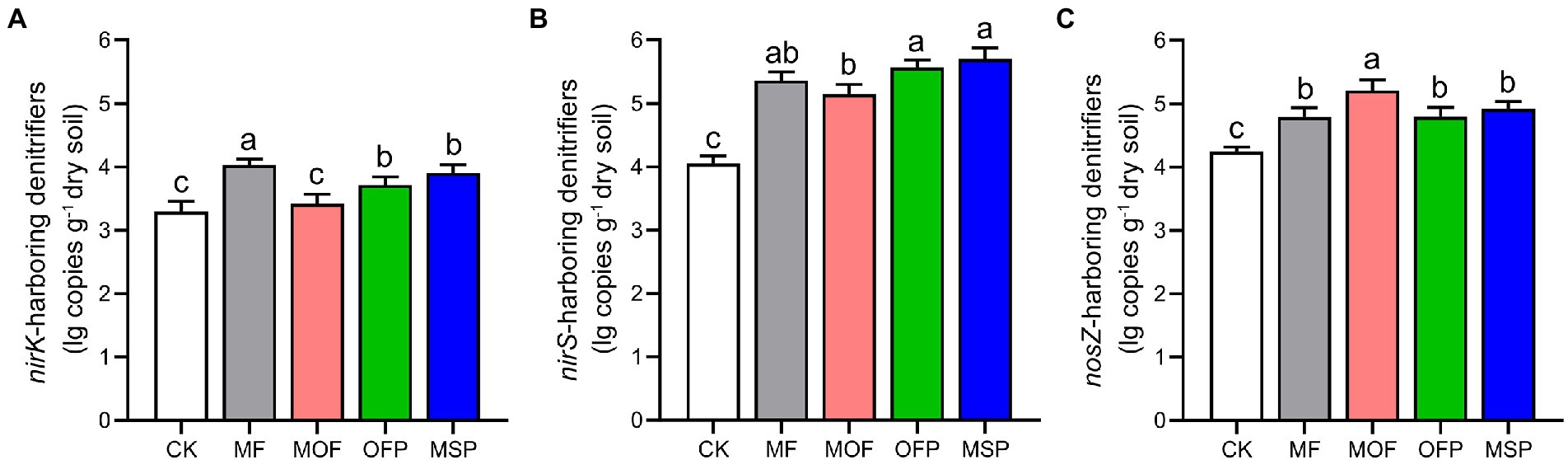
Figure 3. The copy numbers of nirK (A), nirS (B), and nosZ (C) genes under different fertilization treatments. Bars (n = 3) with different lowercase letters indicate significant differences based on Tukey’s HSD test (p < 0.05). CK, No fertilization; MF, mineral fertilizer; MOF, mineral fertilizer plus commercial organic fertilizer; OFP, commercial organic fertilizer; MSP, maize straw.
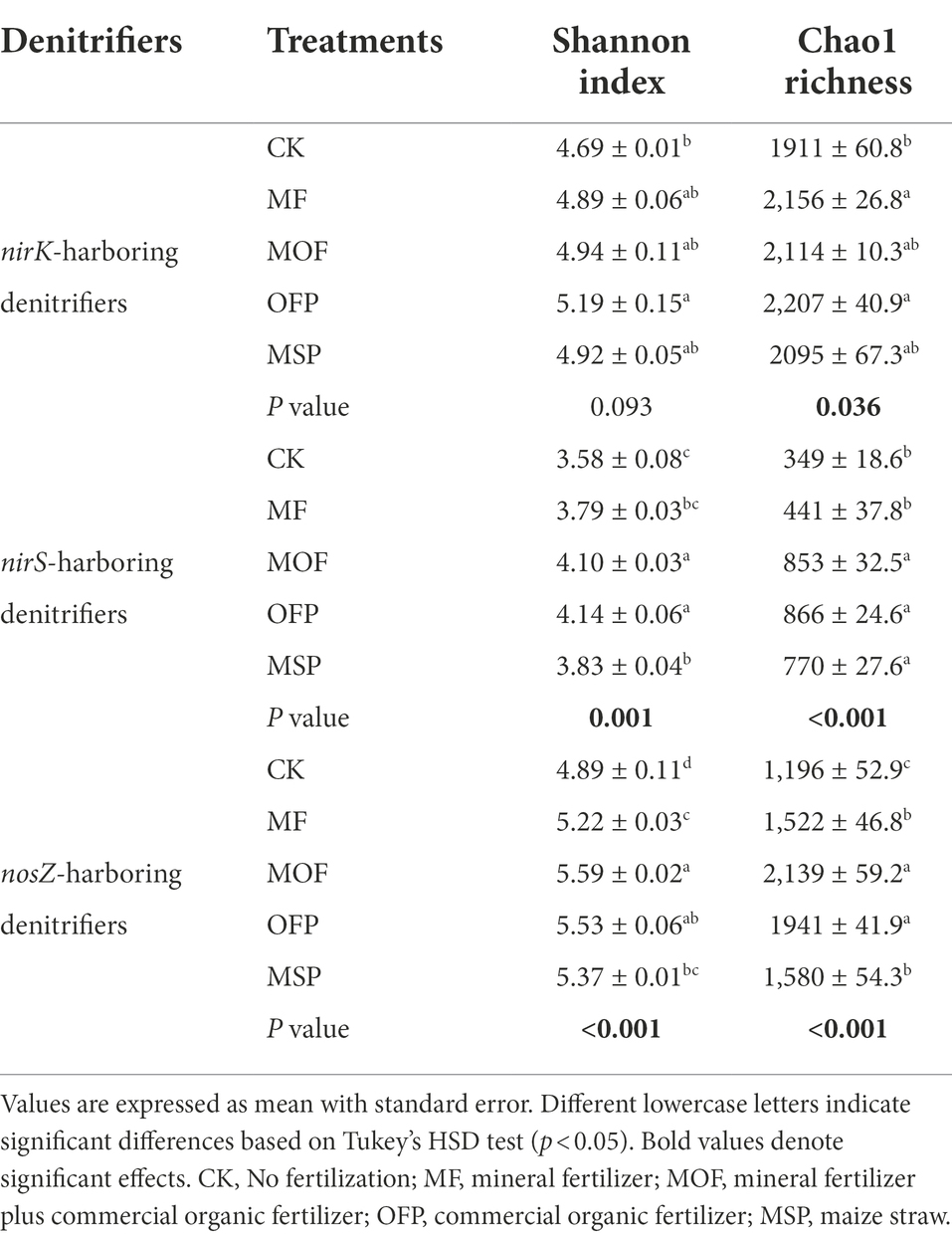
Table 2. The diversity indices of nirK-, nirS-, and nosZ-harboring denitrifies under different fertilization treatments.
The nirK-harboring denitrifiers were dominated by Nitrosospira (36.6%), Rhodobacter (20.4%), Alcaligenes (19.4%), Mesorhizobium (3.6%), and Rubellimicrobium (3.0%; Figure 4A). The relative abundance of Rhodobacter and Nitrosospira was significantly (p < 0.05) higher under MF treatment than under CK and MOF treatments. However, Alcaligenes was significantly (p < 0.05) higher under CK and MSP treatment than under MOF and OFP treatments. Redundancy analysis (RDA) showed that soil pH (14.4%), SOC (13.2%), NO3−−N (13.0%), and TN (12.1%) significantly (p < 0.05) affected the structure of nirK-harboring denitrifier community (Supplementary Figure S2A; Supplementary Table S2). The nirS-harboring denitrifiers were dominated by Cupriavidus (22.5%), Bradyrhizobium (18.0%), Rhodanobacter (12.7%), Azospira (9.4%), Herbaspirillum (7.7%), and Zoogloea (7.1%; Figure 4B). The relative abundance of Bradyrhizobium and Rhodanobacter under MSP treatment was significantly (p < 0.05) higher than that under MOF and OFP treatments, while the relative abundance of Cupriavidus and Zoogloea followed the opposite trend. RDA indicated that NO3−−N (19.1%), SOC (16.6%), and pH (15.7%) significantly (p < 0.05) affected the structure of nirS-harboring denitrifier community (Supplementary Figure S2B; Supplementary Table S2). The nosZ-harboring denitrifiers were mainly comprised of Azospirillum (23.2%), Mesorhizobium (17.2%), Burkholderia (16.0%), and Herbaspirillum (14.0%; Figure 4C). The genera Azospirillum and Mesorhizobium were significantly (p < 0.05) higher under MOF and MSP treatments than under OFP treatment, whereas Burkholderia exhibited the inverse pattern. RDA revealed that SOC (20.5%), TN (18.2%), NO3−−N (15.6%), and pH (13.5%) significantly (p < 0.05) affected the structure of nosZ-harboring denitrifier community (Supplementary Figure S2C; Supplementary Table S2).
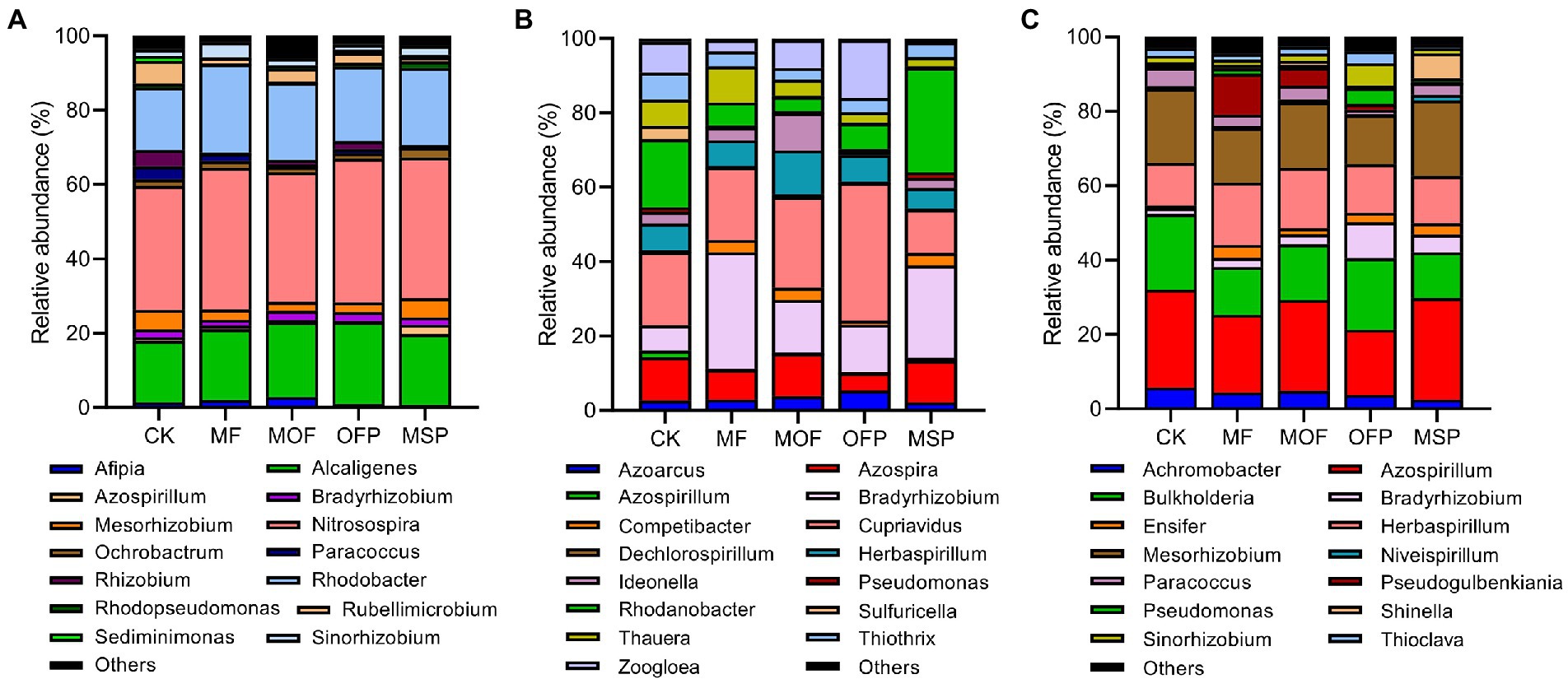
Figure 4. Taxonomic compositions of nirK- (A), nirS- (B), and nosZ-harboring (C) denitrifiers at the genus level under different fertilization treatments. CK, No fertilization; MF, mineral fertilizer; MOF, mineral fertilizer plus commercial organic fertilizer; OFP, commercial organic fertilizer; MSP, maize straw.
Co-occurrence network of the denitrifying communities
The co-occurrence networks were constructed to investigate the critical modules within the denitrifying communities. There were more positive edges than negative edges in the networks of nirK-, nirS-, and nosZ-harboring denitrifiers (Supplementary Figure S3; Supplementary Table S3). The denitrifying bacterial networks were divided into distinct modules that were closely associated functional groups of taxa. Modules I, II, III, and IV of nirK-harboring denitrifier network comprised of 46, 43, 25, and 37 nodes, and 225, 178, 30, and 77 edges, respectively (Supplementary Table S3). Modules I, II, III, and IV of nirS-harboring denitrifier network consisted of 32, 26, 25, and 15 nodes, with 280, 76, 53, and 12 edges, respectively. There were 69, 41, 38, and 53 nodes, and 320, 181, 175, and 294 edges in the modules I, II, III, and IV of nosZ-harboring denitrifier network, respectively.
Within the nirK-harboring denitrifier network, the genera Alcaligenes, Nitrosospira, and Ochrobactrum were identified as the potential keystone taxa in the module I (Supplementary Figure S3A). The module I of nirK-harboring denitrifier network showed significantly positive correlations with NO3−−N (r = 0.77, p < 0.01), AP (r = 0.52, p < 0.05), and community composition (r = 0.80, p < 0.01), but significantly negative correlations with pH (r = −0.74, p < 0.01) and diversity (r = −0.54, p < 0.05; Figure 5). The modules I in the nirS-harboring denitrifier network consisted of three keystone taxa affiliated with Cupriavidus, Rhodanobacter, and Bradyrhizobium (Supplementary Figure S3B). The module I of nirS-harboring denitrifier network exhibited positive relationships with NO3−−N (r = 0.89, p < 0.01), community composition (r = 0.69, p < 0.01), PDA (r = 0.74, p < 0.01), and N2O emissions (r = 0.57, p < 0.05), but negative relationships with pH (r = −0.89, p < 0.01; Figure 5). The module II of nirS-harboring denitrifier network were negatively correlated with NO3−−N (r = −0.64, p < 0.05), abundance (r = −0.77, p < 0.01), and community composition (r = −0.67, p < 0.01), but positively correlated with pH (r = 0.71, p < 0.01). The nosZ-harboring denitrifier network had six keystone taxa, belonging to Azospirillum, Mesorhizobium, Burkholderia, Shinella, Ensifer, and Pseudomonas (Supplementary Figure S3C). The modules III and IV of nosZ-harboring denitrifier network showed negative associations with NO3−−N (r = −0.64, p < 0.05 and r = −0.63, p < 0.05; Figure 5).
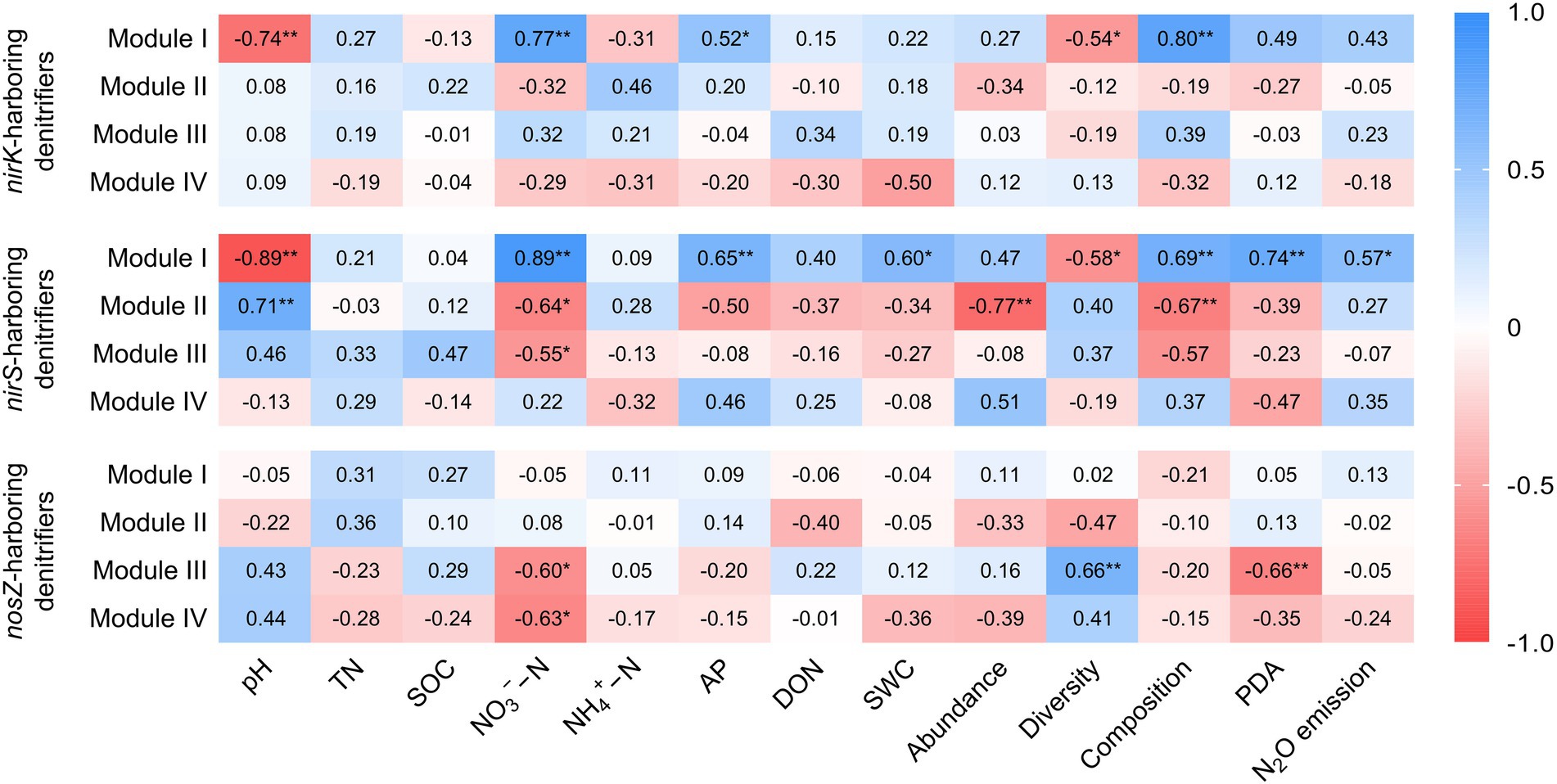
Figure 5. Correlation coefficients between module eigengenes, soil properties, the nirK-, nirS-, and nosZ-harboring denitrifiers, potential denitrification activity (PDA), N2O emission. The denitrifying communities are represented by abundance (the copy numbers of genes), diversity (Chao1 richness), and composition (first principal coordinates, PC1). Blue color indicates positive correlation, and red color indicates negative correlations. TN, total nitrogen; SOC, soil organic carbon; NO3−−N, nitrate nitrogen; NH4+ − N, ammonia nitrogen; AP, available phosphorus; DON, dissolved organic nitrogen; SWC, soil water content. *p < 0.05 and **p < 0.01.
Relationships between soil properties, the denitrifying bacterial communities, and N2O emissions
The abundance of nirK-harboring denitrifiers was positively associated with TN (r = 0.54, p < 0.05) and SOC (r = 0.74, p < 0.01), but negatively associated with N2O emissions (r = −0.62, p < 0.05). The abundance of nirS-harboring denitrifiers was positively correlated with pH (r = 0.53, p < 0.05), NO3−−N (r = 0.67, p < 0.01), SOC (r = 0.65, p < 0.05), DON (r = 0.51, p < 0.05), PDA (r = 0.54, p < 0.05), and N2O emissions (r = 0.57, p < 0.05). The abundance of nosZ-harboring denitrifiers was positively correlated with NO3−−N (r = 0.56, p < 0.05) and SWC (r = 0.68, p < 0.01), but negatively correlated with pH (r = −0.56, p < 0.05), SOC (r = −0.75, p < 0.01), and DON (r = −0.63, p < 0.05).
Random forest modeling indicated that soil pH (6.1%, p < 0.05), SOC (8.9%, p < 0.01), NO3−−N (7.5%, p < 0.05), and DON (11.5%, p < 0.01) were the important abiotic variables predicting N2O emissions (Figure 6A). As for biotic variables, N2O emissions were significantly affected by the abundance (9.6%, p < 0.01), composition (6.9%, p < 0.05) and network module I (6.1%, p < 0.05) of nirS-harboring denitrifiers, and the abundance of nirK-harboring denitrifiers (6.5%, p < 0.05; Figure 6A). However, the nosZ-harboring denitrifiers exhibited no significant impact on N2O emissions. Structural equation modeling further showed that soil properties had significantly positive effects on the nirS- and nirK-harboring denitrifier communities (r = 0.81, p < 0.01 and r = 0.61, p < 0.05), and PDA (r = 0.69, p < 0.05; Figure 6B). Importantly, the nirS-harboring denitrifiers were positively correlated with PDA (r = 0.73, p < 0.05) through the abundance, community composition, and network module I (Figure 6B). However, the abundance of nirK-harboring denitrifiers was negatively associated with PDA (r = −0.49, p < 0.05).
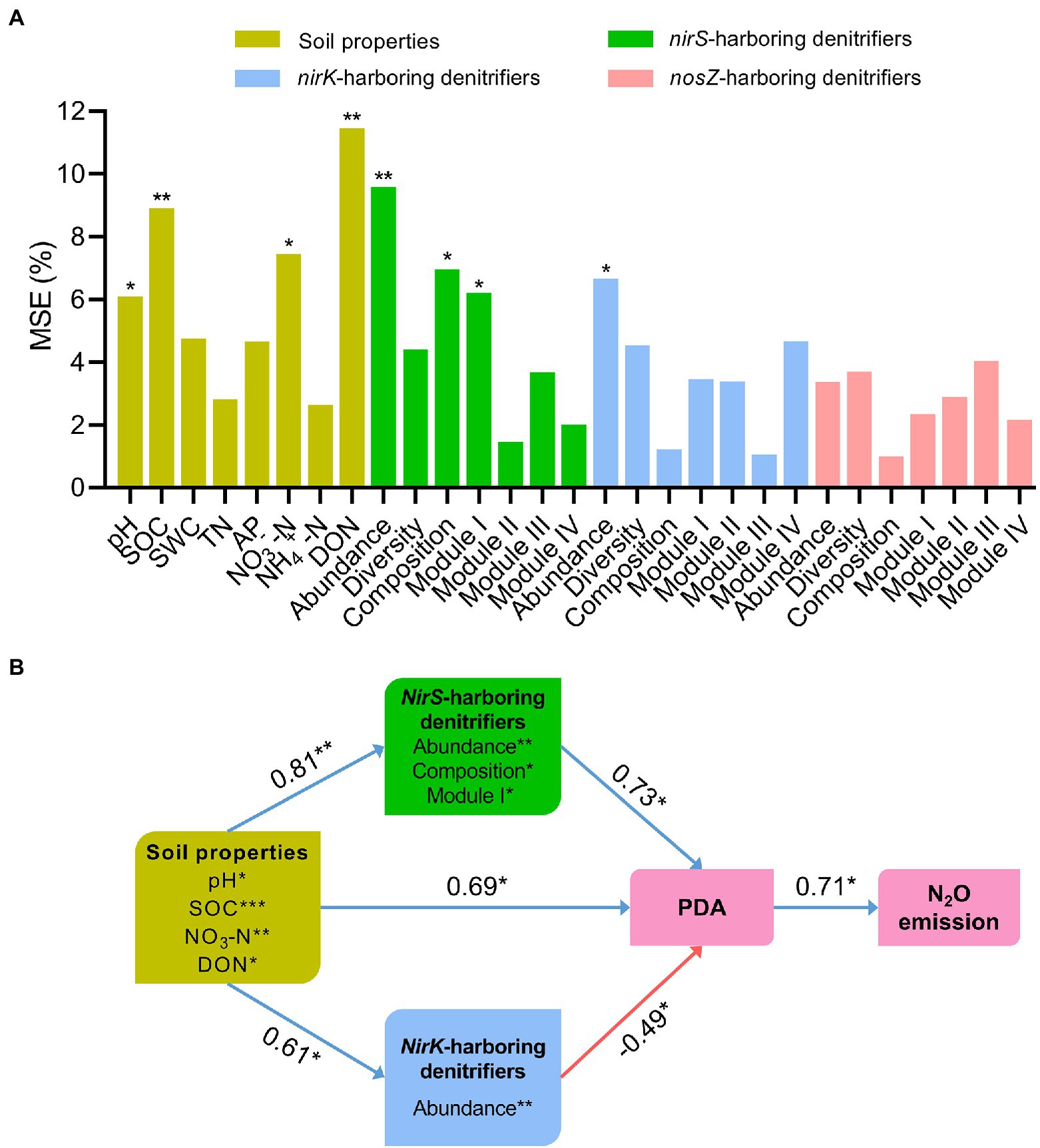
Figure 6. Random forest modeling was used to assess the main predictors of potential denitrification activity (PDA; A). Soil properties is represented by pH, soil organic carbon (SOC), soil water content (SWC), total nitrogen (TN), available phosphorus (AP), nitrate nitrogen (NO3−−N), ammonia nitrogen (NH4+ − N), and dissolved organic nitrogen (DON). The denitrifying communities are represented by abundance (the copy numbers of genes), diversity (Chao1 richness), composition (first principal coordinates, PC1) and network modules (module eigengenes). The significant predictors are further chosen to perform the structural equation modeling (SEM). SEM shows the direct and indirect effects of soil properties and the denitrifying communities on potential denitrification activity (PDA) and N2O emission across fertilization treatments (B). Blue arrows indicate positive correlations, and red arrows indicate negative correlations. The numbers associated with arrows indicate the correlation coefficient. Widths of arrows indicate strength of significant standardized path coefficients. *p < 0.05; **p < 0.01.
Discussion
Soil properties and denitrifying communities in response to fertilization treatments
We found that OFP and MSP treatments considerably affected soil chemical properties compared to MF and CK treatments, including soil pH, SOC, DON, and NO3−−N. Organic fertilization has been widely proposed as alternative approach to solve the problems of excessive mineral fertilizer in sustainable farming systems (Seufert et al., 2012). Organic fertilizers (animal manures or maize straw) generally contains specifically high levels of organic matter content and micronutrients (Lamptey et al., 2019). The replacement of mineral fertilizer with organic fertilizers improves soil quality and physicochemical properties, as well as soil carbon and nitrogen stocks (Qiu et al., 2016). Correspondingly, the increase in SOC can provide readily available C sources for the microbial metabolism to improve DON immobilization (Cusack et al., 2011). However, OFP and MSP treatments significantly increased the potential denitrification activity, which was responsible for NO3−−N reduction relative to MF treatment.
In our study, inorganic fertilizer had a significantly positive effect on the abundance of nirK-harboring denitrifiers, while three organic fertilization treatments enhanced the abundance and diversity of nirS- and nosZ-harboring denitrifiers. Recent studies have reported that the nirK-harboring denitrifiers are more abundant than the nirS- and nosZ-harboring denitrifiers in different soils (Cui et al., 2016; Shi et al., 2019). However, our results showed that the abundance of nirS-harboring denitrifiers was significantly higher than that of nosZ- and nirK-harboring denitrifiers. In general, the variations of nirS-harboring denitrifiers in response to organic fertilization treatments can be largely responsible for the differences in SOC and N availability (Tao et al., 2018). The high level of SOC is considered to support the increasing abundance of nirS- and nosZ-harboring denitrifiers in soils treated with organic materials (Chen et al., 2019a). The nirS-harboring denitrifiers conduct the last step of denitrification, and are dependent on the changes in exogenous carbon and nutrient resource supply. Furthermore, soil pH can directly affect the cell growth and activities of nirS-harboring denitrifiers (Li et al., 2020b). The nosZ-harboring denitrifiers encode nitrous oxide reductase and promote denitrification to be carried out thoroughly. NO3−−N, an electron acceptor in denitrification, is strongly associated with the abundance and diversity of nosZ-harboring denitrifiers (Kastl et al., 2015). The predominance of nosZ-harboring denitrifiers under organic fertilization treatments facilitates the conversion of N2O to N2, thereby reducing N2O emissions (Shi et al., 2019).
Fertilization treatments lead to the changes in the bacterial life-history strategies, and contribute primarily to the variations in community structure (Fierer et al., 2012). Our results determined that the composition of microbial functional groups involved in denitrification responded significantly to fertilization treatments. The organic fertilization treatments presented the high abundance of Cupriavidus and Zoogloea in the nirS-harboring denitrifiers and Mesorhizobium and Azospirillum in the nosZ-harboring denitrifiers. The members of genus Zoogloea plays a key role in endogenous denitrification via nitrogen metabolism, promoting N2O emissions (Shen et al., 2020). In addition, the genera Mesorhizobium and Azospirillum promote diffusive transport of organic substrates, potentially enhancing the availability of organic N substrate for N-cycling microbial communities (Huang et al., 2020). As such, these dominant genera were considered to affect denitrification potentials and mediate N2O emissions under organic fertilization treatments.
Microbial co-occurrence network and potential keystone taxa
Co-occurrence network analysis is often performed to investigate the species associations in the nirK, nirS, and nosZ-harboring denitrifier networks (Zhang et al., 2018). Overall, our results clearly showed that the number of edges and nodes was higher in the nosZ-harboring denitrifying network than in the nirK- and nirS-harboring denitrifier networks. There were more positive associations than negative ones in the networks, implying the intensive species cooperation and exchange events in the nirK-, nirS-, and nosZ-harboring denitrifiers. The high network centrality in the nirS-harboring denitrifier network showed better modularly organized in information transfer between bacteria, indicating their efficient performance within modules. Topological characteristics of the denitrifying bacterial networks can be further applied to statistically identify the modules (strong connecting structures among taxa) and potential keystone taxa based on their connections and central positions in the networks. The modules with higher modularity is likely to be more stable owing to stronger associations within functional groups (Maslov and Sneppen, 2002). The modules in denitrifying bacterial networks exhibited significant correlations with soil pH and NO3−−N, suggesting environmental changes might influence bacterial networks via these specific modules. We found that the potential keystone taxa within the modules of the denitrifying bacterial networks belonged to the distinct genera. The keystone taxa within the denitrifying bacterial networks may directly shape soil microbiome community assemblages with a disproportionate effect due to strong taxa interactions (Faust and Raes, 2012; Chen et al., 2019b). These keystone taxa form a close clustering with other taxa within the microbiome community, and contribute largely to network robustness (Zheng et al., 2022a). The keystone taxa may explain a large part of the network structure, and their removal causes considerable alterations in the stability and functioning of denitrifying bacterial community (Berry and Widder, 2014). However, caution is warranted when inferring the significant effects of keystone taxa on the denitrifying bacterial community. Further targeted culturomic approaches and empirical evidence are urgently needed to verify our findings on the contribution of potential keystone taxa to the entire networks.
Denitrifying bacterial communities mediated N2O emissions
The denitrifying bacterial communities play crucial roles in the biogeochemical cycling of nitrogen through regulating potential denitrification activity (Domeignoz-Horta et al., 2018). We observed that the abundance, diversity and network module I of nirS-harboring denitrifiers were significantly correlated with PDA and N2O emission, rather than those of nirK and nosZ-harboring denitrifiers across five fertilization treatments. This result indicated that the denitrifying communities are not functionally similar under distinct soil environmental conditions. It is broadly accepted that niche differentiation has remarkable influence on the different behaviors and functional activities of denitrifying bacteria (Sun et al., 2017; Chen et al., 2020). Numerous literatures highlight their importance for N2O emissions, indicating by the positive relationship between the abundance of nirS-harboring denitrifiers and PDA (Ullah et al., 2020; Li et al., 2020a). Organic fertilization treatments provided a more balanced and sustainable nutrient resources for the diverse denitrifying bacterial populations, and the higher abundance of nirS-harboring denitrifiers increased N2O emissions. The nirS-harboring denitrifiers were proposed to be the numerical and functional dominance for a high denitrification efficiency over other denitrifying bacterial communities. Additionally, the module I with keystone taxa in the nirS-harboring denitrifier network was positively associated with PDA and N2O emissions. The keystone taxa have been commonly recognized as functional units that are of ecological importance in PDA and N dynamics irrespective of their abundance (Zhang et al., 2018; Zheng et al., 2022b). The species interactions mediated by potential keystone taxa in the nirS-harboring denitrifier network may improve positive abundance-functioning relationships. The large community size induced by keystone taxa contributes to the eventual promotion of denitrification potential and N2O fluxes in the natural field systems (Čuhel et al., 2010). Consequently, we suggested that the networks of the nirS-harboring denitrifiers could facilitate the community performance of denitrification at high levels of abundance.
Conclusion
We observed that organic fertilization treatments significantly enhanced the abundance and diversity of nirS- and nosZ-harboring denitrifiers compared to CK treatment. Importantly, the abundance and the network module with keystone taxa of nirS-harboring denitrifiers exhibited exclusively positive relationships with denitrification potential and N2O emissions. Our study indicated the higher effect of nirS-harboring denitrifiers over nirK- and nosZ-harboring denitrifiers for denitrification and N2O fluxes. Taken together, this study provides insights into the response of the nirS-harboring denitrifiers to agricultural practices and the biotic mechanism behind positive affect on N2O emissions under organic fertilization treatments. As such, the deep understanding of mitigation measures for N2O emissions may pave the way for developing sustainable agroecosystems under a broad range of soil-climate scenarios.
Data availability statement
The data of the nirK, nirS, and nosZ genes were deposited in the Sequence Read Archive of the NCBI database under accession number SRR18251436, SRR18481671, and SRR18481638, respectively.
Author contributions
SF and LL: conceptualization and methodology. SF: validation, writing—original draft preparation, and visualization. JX and LW: resources. SF and LX: data curation. YJ, A-RA, SA, and LL: writing—review and editing. LL and YJ: supervision. JX: project administration. All authors have read and approved the content of the manuscript. All authors contributed to the article and approved the submitted version.
Funding
The research was supported by the Education science and technology innovation project of Gansu Province (GSSYLXM-02), the National Natural Science Foundation of China (31761143004), the Youth Innovation Promotion Association of CAS (Y2021084), Science and technology Project of Jiangsu Province (BE2022394), and the Young Instructor Fund Project of Gansu Agricultural University (GAU-QDFC-2020-03).
Conflict of interest
The authors declare that the research was conducted in the absence of any commercial or financial relationships that could be construed as a potential conflict of interest.
Publisher’s note
All claims expressed in this article are solely those of the authors and do not necessarily represent those of their affiliated organizations, or those of the publisher, the editors and the reviewers. Any product that may be evaluated in this article, or claim that may be made by its manufacturer, is not guaranteed or endorsed by the publisher.
Supplementary material
The Supplementary material for this article can be found online at: https://www.frontiersin.org/articles/10.3389/fmicb.2022.905157/full#supplementary-material
References
Archer, E. (2020). rfPermute: estimate permutation p-values for random forest importance metrics. R package version, 2.1.81.
Azziz, G., Monza, J., Etchebehere, C., and Irisarri, P. (2017). nirS- and nirK-type denitrifier communities are differentially affected by soil type, rice cultivar and water management. Eur. J. Soil Biol. 78, 20–28. doi: 10.1016/j.ejsobi.2016.11.003
Barberán, A., Bates, S. T., Casamayor, E. O., and Fierer, N. (2012). Using network analysis to explore co-occurrence patterns in soil microbial communities. ISME J. 6, 343–351. doi: 10.1038/ismej.2011.119
Berry, D., and Widder, S. (2014). Deciphering microbial interactions and detecting keystone species with co-occurrence networks. Front. Microbiol. 5:219. doi: 10.3389/fmicb.2014.00219
Bremner, J. M. (1996). “Nitrogen-total,” in Methods of Soil Analysis: Part 3 Chemical Methods 5. ed. D. L. Sparks (Madison: American Society of Agronomy Inc), 1085–1121.
Caporaso, J. G., Kuczynski, J., Stombaugh, J., Bittinger, K., Bushman, F. D., Costello, E. K., et al. (2010). QIIME allows analysis of high-throughput community sequencing data. Nat. Methods 7, 335–336. doi: 10.1038/nmeth0510-335
Chen, Q., Ding, J., Li, C., Yan, Z., He, J., and Hu, H. (2020). Microbial functional attributes, rather than taxonomic attributes, drive top soil respiration, nitrification and denitrification processes. Sci. Total Environ. 734:139479. doi: 10.1016/j.scitotenv.2020.139479
Chen, L., Jiang, Y., Liang, C., Luo, Y., Xu, Q., Cheng, H., et al. (2019b). Competitive interaction with keystone taxa induced negative priming under biochar amendments. Microbiome 7:77. doi: 10.1186/s40168-019-0693-7
Chen, J., Li, J., Shen, W., Xu, H., Li, Y., and Luo, T. (2019a). The structure and species co-occurrence networks of soil denitrifying bacterial communities differ between a coniferous and a broadleaved forests. Microorganisms 7:361. doi: 10.3390/microorganisms7090361
Cuello, J. P., Young, H., Gutierrez, J., Yoon, S., and Joo, P. (2015). Impact of plastic film mulching on increasing greenhouse gas emissions in temperate upland soil during maize cultivation. Appl. Soil Ecol. 91, 48–57. doi: 10.1016/j.apsoil.2015.02.007
Čuhel, J., Šimek, M., Laughlin, R. J., Bru, D., Chèneby, D., Watson, C. J., et al. (2010). Insights into the effect of soil pH on N2O and N2 emissions and denitrifier community size and activity. Appl. Environ. Microbiol. 76, 1870–1878. doi: 10.1128/AEM.02484-09
Cui, P., Fan, F., Yin, C., Song, A., Huang, P., Tang, Y., et al. (2016). Long-term organic and inorganic fertilization alters temperature sensitivity of potential N2O emissions and associated microbes. Soil Biol. Biochem. 93, 131–141. doi: 10.1016/j.soilbio.2015.11.005
Cusack, D. F., Silver, W. L., Torn, M. S., Burton, S. D., and Firestone, M. K. (2011). Changes in microbial community characteristics and soil organic matter with nitrogen additions in two tropical forests. Ecology 92, 621–632. doi: 10.1890/10-0459.1
Domeignoz-Horta, L. A., Philippot, L., Peyrard, C., Bru, D., Breuil, M., Bizouard, F., et al. (2018). Peaks of in situ N2O emissions are influenced by N2O-producing and reducing microbial communities across arable soils. Glob. Chang. Biol. 24, 360–370. doi: 10.1111/gcb.13853
Edgar, R. C., and Flyvbjerg, H. (2015). Error filtering, pair assembly and error correction for next-generation sequencing reads. Bioinformatics 31, 3476–3482. doi: 10.1093/bioinformatics/btv401
Edgar, R. C., Haas, B. J., Clemente, J. C., Quince, C., and Knight, R. (2011). UCHIME improves sensitivity and speed of chimera detection. Bioinformatics 27, 2194–2200. doi: 10.1093/bioinformatics/btr381
Faust, K., and Raes, J. (2012). Microbial interactions: from networks to models. Nat. Rev. Microbiol. 10, 538–550. doi: 10.1038/nrmicro2832
Fierer, N., Lauber, C. L., Ramirez, K. S., Zaneveld, J., Bradford, M. A., and Knight, R. (2012). Comparative metagenomic, phylogenetic and physiological analyses of soil microbial communities across nitrogen gradients. ISME J. 6, 1007–1017. doi: 10.1038/ismej.2011.159
Fortmannroe, S. (2015). A3: accurate, adaptable, and accessible error metrics for predictive models. R package version 1.0.0.
Ghani, A., Dexter, M., and Perrott, K. W. (2003). Hot-water extractable carbon in soils: a sensitive measurement for determining impacts of fertilisation, grazing and cultivation. Soil Biol. Biochem. 35, 1231–1243. doi: 10.1016/S0038-0717(03)00186-X
Herren, C. M., and McMahon, K. D. (2018). Keystone taxa predict compositional change in microbial communities. Environ. Microbiol. 20, 2207–2217. doi: 10.1111/1462-2920.14257
Hu, H. W., Chen, D., and He, J. Z. (2015). Microbial regulation of terrestrial nitrous oxide formation: understanding the biological pathways for prediction of emission rates. FEMS Microbiol. Rev. 39, 729–749. doi: 10.1093/femsre/fuv021
Huang, R., Wang, Y., Gao, X., Liu, J., Wang, Z., and Gao, M. (2020). Nitrous oxide emission and the related denitrifier community: a short-term response to organic manure substituting chemical fertilizer. Ecotox. Environ. Safe. 192:110291. doi: 10.1016/j.ecoenv.2020.110291
Huang, R., Wang, Y., Liu, J., Li, J., Xu, G., Luo, M., et al. (2019). Variation in N2O emission and N2O related microbial functional genes in straw- and biochar-amended and non-amended soils. Appl. Soil Ecol. 137, 57–68. doi: 10.1016/j.apsoil.2019.01.010
Jang, J., Ashida, N., Kai, A., Isobe, K., Nishizawa, T., Otsuka, S., et al. (2018). Presence of cu-type (Nirk) and cd1-type (nirs) nitrite reductase genes in the denitrifying bacterium Bradyrhizobium nitroreducens sp. nov. Microbes Environ. 33, 326–331. doi: 10.1264/jsme2.ME18039
Jantalia, C. P., Dos Santos, H. P., Urquiaga, S., Boddey, R. M., and Alves, B. J. R. (2008). Fluxes of nitrous oxide from soil under different crop rotations and tillage systems in the south of Brazil. Nutr. Cycl. Agroecosyst. 82, 161–173. doi: 10.1007/s10705-008-9178-y
Kastl, E.-M., Schloter-Hai, B., Buegger, F., and Schloter, M. (2015). Impact of fertilization on the abundance of nitrifiers and denitrifiers at the root-soil interface of plants with different uptake strategies for nitrogen. Biol. Fertil. Soils 51, 57–64. doi: 10.1007/s00374-014-0948-1
Lamptey, S., Xie, J., Li, L., Coulter, J. A., and Jagadabhi, P. S. (2019). Influence of organic amendment on soil respiration and maize productivity in a semi-arid environment. Agronomy 9:616. doi: 10.3390/agronomy9100611
Li, W., and Godzik, A. (2006). Cd-hit: A fast program for clustering and comparing large sets of protein or nucleotide sequences. Bioinformatics 22, 1658–1659. doi: 10.1093/bioinformatics/btl158
Li, W., Wang, C., Zheng, M., Cai, Z., Wang, B., and Shen, R. (2020b). Fertilization strategies affect soil properties and abundance of N-cycling functional genes in an acidic agricultural soil. Appl. Soil Ecol. 156:103704. doi: 10.1016/j.apsoil.2020.103704
Li, P. P., Zhang, S. Q., Li, F., Zhang, Y. T., and Han, Y. L. (2020a). Long term combined fertilization and soil aggregate size on the denitrification and community of denitrifiers. Appl. Soil Ecol. 156:103718. doi: 10.1016/j.apsoil.2020.103718
Liaw, A., and Wiener, M. (2002). Classification and regression by random forest. R News 2, 18–22. doi: 10.1057/9780230509993
Maslov, S., and Sneppen, K. (2002). Specificity and stability in topology of protein networks. Science 296, 910–913. doi: 10.1126/science.1065103
Nelson, D. W., and Sommers, L. E. (1996). “Total carbon, organic carbon, and organic matter,” in Methods of Soil Analysis: Part 3 Chemical Methods 5. ed. D. L. Sparks (Madison: American Society of Agronomy Inc), 961–1010.
Olsen, S. R., Cole, C., Watanabe, F. S., and Dean, L. (1954). Estimation of Available Phosphorus in Soils by Extraction with sodium Bicarbonate. Circular (No. 939). (Washington DC: USDA Press), 1–19.
Ouyang, Y., Evans, S. E., Friesen, M. L., and Tiemann, L. K. (2018). Effect of nitrogen fertilization on the abundance of nitrogen cycling genes in agricultural soils: a meta-analysis of field studies. Soil Biol. Biochem. 127, 71–78. doi: 10.1016/j.soilbio.2018.08.024
Pang, J., Wang, X., Peng, C., Mu, Y., Ouyang, Z., Lu, F., et al. (2019). Nitrous oxide emissions from soils under traditional cropland and apple orchard in the semi-arid loess plateau of China. Agric. Ecosyst. Environ. 269, 116–124. doi: 10.1016/j.agee.2018.09.028
Philippot, G. C., Andert, J., Jones, C. M., Bru, D., and Hallin, S. (2011). Importance of denitrifiers lacking the genes encoding the nitrous oxide reductase for N2O emissions from soil. Glob. Chang. Biol. 17, 1497–1504. doi: 10.1111/j.1365-2486.2010.02334.x
Qiu, S., Gao, H., Zhu, P., Hou, Y., Zhao, S., Rong, X., et al. (2016). Changes in soil carbon and nitrogen pools in a Mollisol after long-term fallow or application of mineral fertilizers, straw or manures. Soil Tillage Res. 163, 255–265. doi: 10.1016/j.still.2016.07.002
Sahoo, M. (2019). “Structural equation modeling: threshold criteria for assessing model fit,” in Methodological Issues in Management Research: Advances, Challenges, and the Way Ahead. eds. R. N. Subudhi and S. Mishra (Bingley: Emerald Publishing Limited), 269–276.
Seufert, V., Ramankutty, N., and Foley, J. A. (2012). Comparing the yields of organic and conventional agriculture. Nature 485, 229–232. doi: 10.1038/nature11069
Shen, W., Xue, H., Gao, N., Shiratori, Y., Kamiya, T., Fujiwara, T., et al. (2020). Effects of copper on nitrous oxide (N2O) reduction in denitrifiers and N2O emissions from agricultural soils. Biol. Fertil. Soils 56, 39–51. doi: 10.1007/s00374-019-01399-y
Shi, Y., Liu, X., and Zhang, Q. (2019). Effects of combined biochar and organic fertilizer on nitrous oxide fluxes and the related nitrifier and denitrifier communities in a saline-alkali soil. Sci. Total Environ. 686, 199–211. doi: 10.1016/j.scitotenv.2019.05.394
Sun, M., Mao, Y., Liu, K., Schwab, A. P., Liu, M., and Jiao, J. (2017). Dynamic interplay between microbial denitrification and antibiotic resistance under enhanced anoxic denitrification condition in soil. Environ. Pollut. 222, 583–591. doi: 10.1016/j.envpol.2016.10.015
Tang, Y., Yu, G., Zhang, X., Wang, Q., Tian, D., Tian, J., et al. (2019). Environmental variables better explain changes in potential nitrification and denitrification activities than microbial properties in fertilized forest soils. Sci. Total Environ. 647, 653–662. doi: 10.1016/j.scitotenv.2018.07.437
Tao, R., Wakelin, S. A., Liang, Y., Hu, B., and Chu, G. (2018). Nitrous oxide emission and denitrifier communities in drip-irrigated calcareous soil as affected by chemical and organic fertilizers. Sci. Total Environ. 612, 739–749. doi: 10.1016/j.scitotenv.2017.08.258
Ullah, S., Ai, C., Huang, S., Song, D., Abbas, T., Zhang, J., et al. (2020). Substituting ecological intensification of agriculture for conventional agricultural practices increased yield and decreased nitrogen losses in North China. Appl. Soil Ecol. 147:103395. doi: 10.1016/j.apsoil.2019.103395
Williams, R. J., Howe, A., and Hofmockel, K. S. (2014). Demonstrating microbial co-occurrence pattern analyses within and between ecosystems. Front. Microbiol. 5:358. doi: 10.3389/fmicb.2014.00358
Xiong, Z., Guo, L., Zhang, Q., Liu, G., and Liu, W. (2017). Edaphic conditions regulate denitrification directly and indirectly by altering denitrifier abundance in wetlands along the Han River, China. Environ. Sci. Technol. 51, 5483–5491. doi: 10.1021/acs.est.6b06521
Yang, Z., Guan, Y., Bello, A., Wu, Y., Ding, J., Wang, L., et al. (2020b). Dynamics of ammonia oxidizers and denitrifiers in response to compost addition in black soil, Northeast China. PeerJ 8:e8844. doi: 10.7717/peerj.8844
Yang, Y. D., Hu, Y. G., Wang, Z. M., and Zeng, Z. H. (2018). Variations of the nirS-, nirK-, and nosZ-denitrifying bacterial communities in a northern Chinese soil as affected by different long-term irrigation regimes. Environ. Sci. Pollut. Res. 25, 14057–14067. doi: 10.1007/s11356-018-1548-7
Yang, Q., Liu, P., Dong, S., Zhang, J., and Zhao, B. (2020a). Combined application of organic and inorganic fertilizers mitigates ammonia and nitrous oxide emissions in a maize. Nutr. Cycl. Agroecosyst. 117, 13–27. doi: 10.1007/s10705-020-10060-2
Yeboah, S., Jun, W., Liqun, C., Oteng-Darko, P., Tetteh, E. N., and Renzhi, Z. (2021). Nitrous oxide, methane emissions and grain yield in rainfed wheat grown under nitrogen enriched biochar and straw in a semi-arid environment. PeerJ 9, 1–21. doi: 10.7717/peerj.11937
Yin, C., Fan, F., Song, A., Li, Z., Yu, W., and Liang, Y. (2014). Different denitrification potential of aquic brown soil in Northeast China under inorganic and organic fertilization accompanied by distinct changes of nirS- and nirK-denitrifying bacterial community. Eur. J. Soil Biol. 65, 47–56. doi: 10.1016/j.ejsobi.2014.09.003
Yoshida, M., Ishii, S., Otsuka, S., and Senoo, K. (2009). Temporal shifts in diversity and quantity of nirS and nirK in a rice paddy field soil. Soil Biol. Biochem. 41, 2044–2051. doi: 10.1016/j.soilbio.2009.07.012
Yu, Y. X., Jia, H. T., and Zhao, C. Y. (2018). Evaluation of the effects of plastic mulching and nitrapyrin on nitrous oxide emissions and economic parameters in an arid agricultural field. Geoderma 324, 98–108. doi: 10.1016/j.geoderma.2018.03.012
Zhang, X., Fang, Q., Zhang, T., Ma, W., Velthof, G. L., Hou, Y., et al. (2020). Benefits and trade-offs of replacing synthetic fertilizers by animal manures in crop production in China: a meta-analysis. Glob. Chang. Biol. 26, 888–900. doi: 10.1111/gcb.14826
Zhang, K., Gu, J., Wang, X., Yin, Y., Zhang, X., Zhang, R., et al. (2018). Variations in the denitrifying microbial community and functional genes during mesophilic and thermophilic anaerobic digestion of cattle manure. Sci. Total Environ. 634, 501–508. doi: 10.1016/j.scitotenv.2018.03.377
Zhang, L. H., Zeng, G. M., Zhang, J. C., Chen, Y. N., Yu, M., Lu, L. H., et al. (2015). Response of denitrifying genes coding for nitrite (nirK or nirS) and nitrous oxide (nosZ) reductases to different physico-chemical parameters during agricultural waste composting. Appl. Microbiol. Biotechnol. 99, 4059–4070. doi: 10.1007/s00253-014-6293-3
Zheng, J., Dini-Andreote, F., Luan, L., Geisen, S., Xue, J., Li, H., et al. (2022a). Nematode predation and competitive interactions affect microbe-mediated phosphorus dynamics. MBio 13:e0329321. doi: 10.1128/mbio.03293-21
Zheng, J., Tao, L., Dini-Andreote, F., Luan, L., Kong, P., Xue, J., et al. (2022b). Dynamic responses of ammonia-oxidizing archaea and bacteria populations to organic material amendments affect soil nitrification and nitrogen use efficiency. Front. Microbiol. 13:911799. doi: 10.3389/fmicb.2022.911799
Keywords: denitrifiers, functional genes, keystone taxa, nitrous oxide emissions, potential denitrification activity
Citation: Fudjoe SK, Li L, Jiang Y, Alhassan A-RM, Xie J, Anwar S, Wang L and Xie L (2022) Impact of soil amendments on nitrous oxide emissions and the associated denitrifying communities in a semi-arid environment. Front. Microbiol. 13:905157. doi: 10.3389/fmicb.2022.905157
Edited by:
Muhammad Shaaban, Bahauddin Zakariya University, PakistanReviewed by:
Xingjia Xiang, Anhui University, ChinaGuangqiang Long, Yunnan Agricultural University, China
Wei Min, Shihezi University, China
Copyright © 2022 Fudjoe, Li, Jiang, Alhassan, Xie, Anwar, Wang and Xie. This is an open-access article distributed under the terms of the Creative Commons Attribution License (CC BY). The use, distribution or reproduction in other forums is permitted, provided the original author(s) and the copyright owner(s) are credited and that the original publication in this journal is cited, in accordance with accepted academic practice. No use, distribution or reproduction is permitted which does not comply with these terms.
*Correspondence: Lingling Li, bGlsbEBnc2F1LmVkdS5jbg==; Yuji Jiang, eWpqaWFuZ0Bpc3Nhcy5hYy5jbg==