- 1Department of Animal Science and Technology, Northeast Agricultural University, Harbin, China
- 2Qiqihar Grassland Station, Qiqihar, China
Land use change obviously changes the plant community composition and soil properties of grasslands and thus affects multiple functions and services of grassland ecosystems. However, the response mechanisms of soil microorganisms, key drivers of the nutrient cycle and other soil functions during changes in grassland use type and associated vegetation are not well understood. In this study, Illumina high-throughput sequencing was used to analyze the changes in the soil microbial community structure of four grassland use types: exclosure (EL), mowed land (ML), grazed land (GL), and farmland (FL) in the Songnen Plain of Northeast China. The results showed that the FL and EL had significantly higher soil total nitrogen (TN) and lower soil electrical conductivity (EC) and pH than GL and ML. In contrast, the GL and ML had higher soil bulk density (BD) and organic matter, respectively, than the other land use types. In addition, the values of the Shannon diversity and Pielou’s evenness indexes were highest in the EL of all the land use types. Based on the high-throughput sequencing results, we observed high levels of α diversity in the FL for both bacteria and fungi. A structural equation model (SEM) revealed that pH and EC had a direct and positive effect on the bacterial community structure and composition. In addition, plant taxonomic diversity (according to the Shannon diversity and Pielou’s evenness indexes) indirectly affected the bacterial community composition via soil pH and EC. Notably, fungal composition was directly and positively correlated with soil nutrients and the value of Pielou’s evenness index changed with land use type. In conclusion, soil properties and/or plant diversity might drive the changes in the soil microbial community structure and composition in different grassland use types.
Introduction
Grassland ecosystems are one of the most widely distributed terrestrial ecosystems in the world and cover more than 25% of the Earth’s surface (Wu et al., 2021). Grasslands perform many functions and ecosystem services, including creating a high-yield and high-quality forage supply, storing carbon, purifying water, controlling soil erosion, and maintaining biodiversity (Fuchslueger et al., 2019; Zhang et al., 2021). Many natural and human ecological processes, such as vegetation succession and changes in agricultural management measures, influence changes in grassland ecosystems. The most profound and directive factor is the change in land use. The impact on ecosystem function depends mainly on the type, severity, frequency, and timing of disturbances caused by land use (Wang X. Y. et al., 2020). These factors are related to the participation of soil microorganisms, which respond to changing soil conditions (Fang et al., 2020).
Land use change is currently one of the most important environmental changes and can alter soil environmental factors, nutrient conditions, and biological interactions, thereby affecting microbial communities (Engelhardt et al., 2018; Wang et al., 2019). Previous studies have reported that the conversion of woodland to pasture or farmland could lead to a reduction in the abundance and diversity of some microbial communities; this shift may be related to nutrient cycling because soil microorganisms and their activities play an important role in soil formation, organic matter decomposition, nitrogen fixation and other soil processes (Merloti et al., 2019; Shrestha et al., 2019). Similarly, the bacterial community in cultivated soil under intensive management was different from that in unmanaged soil (Drenovsky et al., 2010). More specifically, management measures, such as fertilization and herbicides, lead to more diverse soil microorganisms with higher functional redundancy, which is an important microbial strategy to overcome environmental interference (Szoboszlay et al., 2017; Santos et al., 2020). Furthermore, changes in plant community composition could alter the input of carbon resources to the soil by producing a variety of litter and root exudates, thereby indirectly changing microbial diversity and activity (Zhong et al., 2020). For example, fungal communities use carbon less efficiently and form less biomass than bacteria (Martí-Roura et al., 2019). However, the microbial communities that perform or participate in these processes in different land use types and their response characteristics to land use change still need to be determined and characterized.
Grazing, mowing, exclosure, and farmland are the main land use types in grasslands (Wang X. Y. et al., 2020). Grazing is a selective disturbance that can reduce the abundance of palatable plants and is one of the main grassland utilization strategies (Chen et al., 2021a). Moderate grazing contributes to improving plant diversity and productivity and may shape soil microbial communities with multiple functions. However, overgrazing reduces the structural and compositional complexity of plant communities and reduces litter and root exudates into the soil, thus affecting the availability of soil substrates (Dassen et al., 2017; Han et al., 2018). Mowing is a nonselective disturbance that changes the material cycle and energy flow of the entire grassland ecosystem, although it does not always produce the expected results (Chen et al., 2021b). Mowing can alter plant species diversity and improve plant community stability (Li et al., 2017). In addition, mowing can change the hydrothermal conditions by removing aboveground biomass, which can have positive or negative effects on soil properties and microbial communities (Yang et al., 2012). Exclosure refers to grassland previously used for grazing and mowing but has been completely conserved with no continuous activities, thus serving as a means of restoring degraded grassland through natural regeneration in northern China (Yan and Lu, 2015). Exclosures can be established to improve soil quality and produce positive feedback to the plant community, i.e., increased plant productivity. In addition, higher plant biomass provides a rich carbon source for soil, which stimulates microbial activities and accelerates nutrient cycling (Szoboszlay et al., 2017). Human population growth causes an increase in food demand and the conversion of more grassland to farmland, resulting in soil degradation. Specifically, these factors lead to a large loss of soil organic matter (SOM), which reduces the stability of soil aggregates, reduces soil fertility, weakens soil structure, and accelerates soil erosion (Li et al., 2021). However, the effects of different grassland use types on microbial community structure remain poorly defined.
The Songnen grassland, the largest meadow grassland in China, is located on the eastern edge of the Eurasian steppe belt and plays an important role in the feedback and regulation of nutrient cycling. Therefore, it is necessary to study the response mechanisms and key microbial processes of the soil microbial community in the Songnen Plain to four grassland use types (exclosure, mowed land, grazed land, and farmland) to provide a theoretical basis for the sustainable management of grassland ecosystems. The objectives of our study were to (1) evaluate the changes in the soil microbial community under different land use types and (2) test the relative importance of environmental factors driving the changes in soil microbial communities in four land use types.
Materials and Methods
Study Sites
This study area was in Qiqihar, Heilongjiang Province, China (123°55′12″E, 47°21′15″N; 140 m a.s.l.) which has a semiarid continental monsoon climate and is located in the mid-temperate zone. The mean annual precipitation is 466 mm, and 80% of this precipitation falls between June and August. The annual average temperature is 3.2°C, with a mean monthly temperature ranging from −25.7°C in January to 22.8°C in July. The soil type of the study area is classified as carbonate meadow soil according to the Chinese Soil Taxonomic Classification (Gong et al., 2011).
Experimental Design
In 2019, we selected four sites of land use in the meadow steppe: (1) The exclosure (EL) covered 50 ha and was fenced in 2016, with no subsequent grazing or mowing. (2) The mowed land (ML) covered 140 ha and was enclosed using iron mesh fence around the EL; mowing to 6 cm occurred every year since 2005 in mid-August to make hay. (3) The grazed land (GL) was located around the EL and ML and was grazed once every calendar month from May to September with 6 sheep/ha, ending when the grassland residual height reached approximately 6 cm. (4) Farmland (FL), which has been cultivated for over 20 years, is also part of this grassland. The main crop is maize, which is sown in May and harvested in October. Crop productivity mainly depends on natural rainfall and materials are not returned to the field after harvest. In the FL, the farmers regularly removed non-target weeds and the composition was monospecific, thus not further investigated here. We selected a large single monitoring area, representative of each land use, in which the altitude, soil texture, and topography (slope direction and gradient) are similar, and all areas use the pseudo-replication procedure (Gilliam et al., 2018; Merloti et al., 2019). The detailed characteristics of the land use history of the study sites are shown in Table 1.
Plant Sampling and Analysis
In mid-August 2019, three non-continuous repeat plots (150 m × 150 m) were established in each site, with a minimum distance of 0.2 km between the plots. Within each plot three 1 m × 1 m randomly selected subplots were used to estimate plant species richness based on the number of species and assess the relative cover of each species by vertically projecting canopy cover (%) for each species. In addition, the individual plant height was recorded in each subplot. Subsequently, the aboveground parts of the plants were clipped, leaving stems with a height of 6 cm from the ground, dried at 65°C for 48 h and weighed. Plant diversity indexes (including Shannon, Simpson’s, and Pielou’s indexes) were calculated according to Tscherko et al. (2004).
Soil Sampling and Analysis
After removing the plant material in the nine subplots mentioned above, 3 individual soil cores within a soil depth of 0–20 cm were randomly collected using a soil corer (4 cm in diameter) from each subplot and manually mixed to form one sample. Then, three composite samples from subplots were mixed as samples of each plot. Finally, a total of 12 samples (4 sites × 3 reps) were collected for this study. The soil was placed in an individual sterile plastic ziplock bag, which was placed inside an ice-cooled container and transported to the laboratory immediately. The samples were sieved (2 mm mesh) to remove plants, roots, and gravel and then partitioned into two subsamples: one was air-dried for physicochemical analysis and the other was stored at −80°C for DNA extraction.
Soil samples (0–20 cm) were collected into aluminum boxes using cutting rings in each plot and dried at 105°C for 24 h to determine the soil bulk density (BD) (Deng et al., 2014). The soil organic matter (SOM) content was determined using the dichromate oxidation method in the presence of H2SO4 and titration with ferrous ammonium sulfate (Sparks et al., 1996). Soil pH and electrical conductivity (EC; important indicators of salinity) were determined with a glass electrode (PHSJ-4A, Shanghai, China) and conductivity meter (DDSJ-308F, Shanghai, China) after shaking the soil (air-dried)/water (1:5, w/v) suspension for 30 min (Bao, 2008). The total nitrogen content (TN) and alkali-hydrolyzable nitrogen (AN) were measured by classical Kjeldahl digestion and distillation azotometry after extraction with 0.02 M sulfuric acid (Hanon K9860, Shanghai, China) (Bremner and Mulvaney, 1982) and using the alkaline hydrolysis diffusion method (Lu, 1999), respectively. Available phosphorus (AP) was extracted using 0.5 M sodium bicarbonate (NaHCO3) (pH 8.5) and then determined using the molybdenum blue method (UV-752 Shanghai, China) (Bao, 2008).
Soil DNA Extraction
For each soil sample, the total DNA was extracted from 0.5 g of fresh soil samples (−80°C) using HiPure Soil DNA Kit (Magen, Guangzhou, China) according to the manufacturer’s protocols. DNA concentration and purity were checked by the absorbance ratios at A260/A280 and A260/A230 using a NanoDrop™ 2000 spectrophotometer (Thermo Fisher Scientific, Wilmington, MA, United States). Amplification of bacterial 16S rRNA was performed for the V3-V4 region, and the primers were 341F (5′-CCTACGGGNGGCWGCAG-3′) and 806R (5′-GGACTACHVGGGTATCTAAT-3′). For the fungal ITS2 region, the primers ITS3_KYO2 (5′-GATGAAGAACGYAGYRAA-3′) and ITS4R (5′-TCCTCCGCTTATTGATATGC-3′) were used for amplification. PCRs were performed in triplicate in a 50 μL mixture containing 5 μL of 10 × KOD Buffer (New England Biolabs, United States), 5 μL dNTPs (2 mM), 3 μL MgSO4 (25 mM), 1.5 μL of each primer (10 μM), 1 μL of KOD polymerase, and 100 ng of template DNA. Amplification was performed as follows: 94°C for 2 min; 30 cycles at 98°C for 10 s, 62°C for 30 s, and 68°C for 30 s; and a final extension at 68°C for 5 min. The PCR products were purified using the AxyPrep DNA Gel Extraction Kit (Axygen Biosciences, Union City, CA, United States) according to the manufacturer’s instructions and quantified using the ABI StepOnePlus Real-Time PCR System (Life Technologies, Foster City, CA, United States). The libraries were sequenced with the Illumina HiSeq 2500 system (Illumina, United States) at Gene Denovo Biotechnology Co., Ltd. (Guangzhou, China).
Sequencing Data Analysis
The fungal and bacterial sequences were analyzed using the Quantitative Insights into Microbial Ecology (QIIME) pipeline (version 1.9.1) (Caporaso et al., 2010). We assembled paired-end clean reads that were merged as raw tags using FLASH (Magoc and Salzberg, 2011), and the sequences with lengths less than 150 bp and/or quality scores lower than 20 were removed for further analysis. The average lengths of sequences for bacteria and fungi were 457 and 368 bp, respectively. To detect and remove chimeric sequences, the tags were compared to the reference database using the UCHIME algorithm (Edgar et al., 2011) and clustered into operational taxonomic units (OTUs) based on a threshold of ≥97% sequence similarity using the UPARSE (version 9.2.64) pipeline. Chimeras and non-target classifications (e.g., chloroplast, mitochondria, archaea) were checked and eliminated during clustering. The taxonomic identities of the bacteria and fungi were determined using RDP software (version 2.2) (Wang et al., 2007) based on comparison with the SILVA database (version 132) (Quast et al., 2013) and UNITE database (version 8.0), respectively.
Statistical Analysis
To reduce the effects of sequencing depth on the downstream analyses, OTU abundance tables were rarefied to the minimum sequence numbers per soil sample (92,000 and 87,000 for bacteria and fungi, respectively), and soil microbial diversity (including the Chao1, Simpson, and Shannon indexes) were calculated in QIIME. One-way ANOVA with Tukey’s HSD test was used to analyze the differences in the biotic and abiotic properties, including plant alpha-diversity, soil alpha-diversity, and relative abundances of dominant phyla across plots. Spearman’s rank correlation coefficients were calculated to determine the relationships of microbial alpha diversity and relative abundances of individual taxa with soil and plant properties. Statistics were performed using SPSS version 19.0 statistical software (SPSS Inc., Chicago, IL, United States). Statistical significance was evaluated at P < 0.05. The results are expressed as the mean values and standard errors (SEs).
We used principal coordinates analysis (PCoA) based on Bray–Curtis distances to analyze the dissimilarity of plant communities and soil microbial composition at the species and OTU levels, respectively. Differences in the microbial communities between land management types were further tested by permutational multivariate analysis of variance (PERMANOVA) (Anderson, 2001) and multivariate homogeneity of groups dispersions (BETADISPER) (Anderson et al., 2006) using the “vegan” package in R (version 2.5.3). Bacterial and fungal taxa with significant differences between sampling groups were identified using the linear discriminant analysis (LDA) effect size (LEfSe) method, and an effect size threshold of 2 was used to identify biomarkers (Segata et al., 2011). LEfSe software (version 1.0) was used to identify organisms that were significantly different using a nonparametric Kruskal–Wallis rank sum test (Krustal and Wallis, 1952). In addition, we performed a redundancy analysis (RDA) using CANOCO 5.0 software (Dang et al., 2010) based on the abundances of OTUs to elucidate the associations among the soil properties, plant communities and microbial groups. Manual forward selection and a Monte Carlo test with 499 permutations were implemented to determine the significance of soil and plant properties on the microbial communities.
A structural equation model (SEM) was created using SPSS Amos 26 (AMOS IBM, United States) to evaluate the direct and indirect effects of land use types on bacterial and fungal communities. To prevent collinearity between variables, we divided the soil properties into two groups: (I) soil nutrients (including SOM and TN) and (II) soil pH and EC. All the indicators were dimensionally reduced by principal component analysis (PCA), and the first principal component (PC1) was extracted to represent each variable group. The PCOA axis 1 scores were used as indicators of bacterial and fungal community composition. The model was fitted by the maximum likelihood estimation method using the chi square test (χ2, P > 0.05), goodness-of-fit index (GFI > 0.9), Akaike information criterion (AIC) and approximate root mean square error (RMSEA < 0.05) to test the goodness of fit of the model (Guo et al., 2021).
Results
Effects of Land Use Types on Soil Properties and Plant Community Parameters
Soil pH, EC, and BD differed significantly among the land use types, with higher pH, EC, and BD recorded in the GL plots than in the ML, EL, and FL plots. The SOM content was highest in the ML and lowest in the GL and differed significantly among all the land use types. Compared with the other land use types, the highest soil TN content was observed in the EL plot (P < 0.05). A significantly higher soil AP content was observed in the FL (11.23 mg kg–1) than in the EL; however, the AP content was similar in the rest of the plots. The soil AN concentration varied from 50 to 107 mg kg–1 and was highest in the ML and EL (Table 2).
Principal coordinates analysis showed that the plant communities in the three land use types were significantly different (Figure 1; P < 0.05), and the plant Shannon diversity and Pielou’s evenness indexes in the EL were higher than those in the GL and ML, whereas Simpson’s dominance index showed the opposite trend (Figure 2). In addition, aboveground biomass was significantly lower in the GL than in the EL and ML (Table 2; P < 0.05).
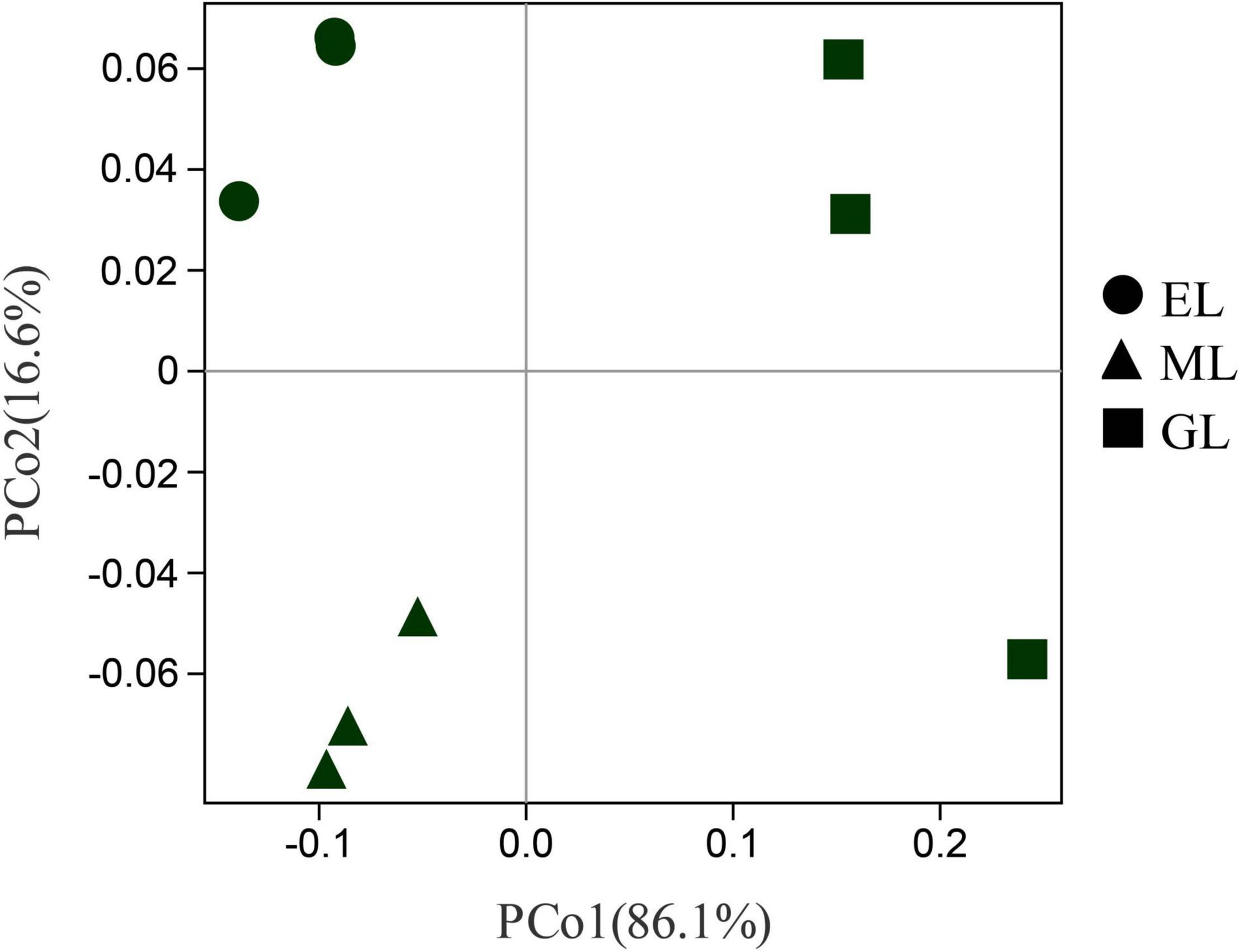
Figure 1. Principal component analysis (PCoA) of plant communities in different land use types. EL, exclosure; ML, mowed land; and GL, grazed land.
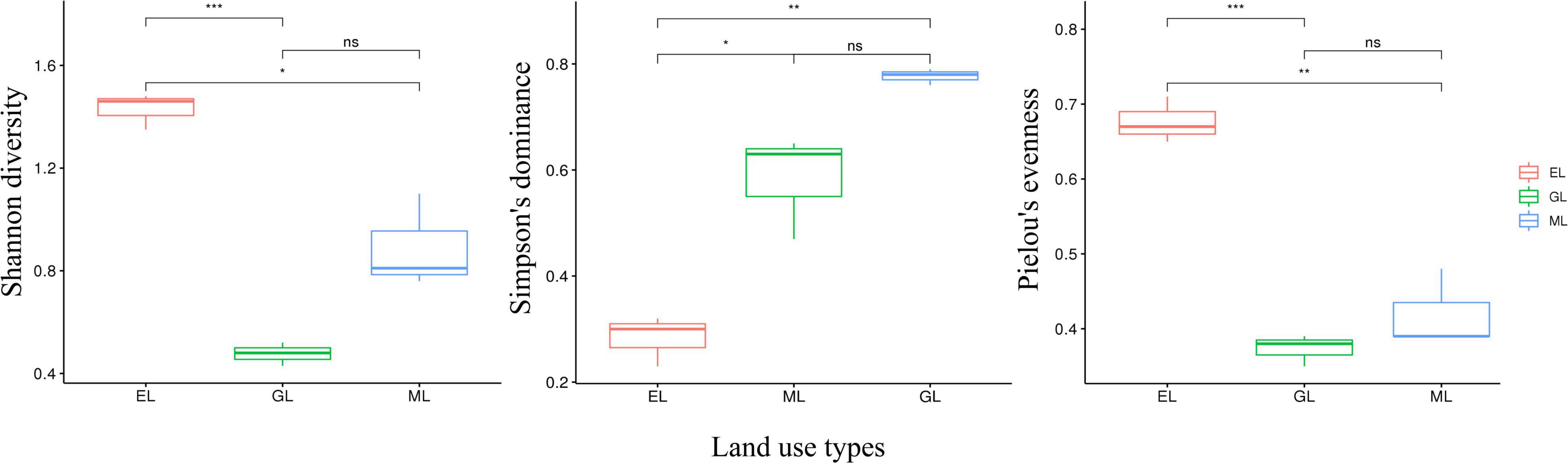
Figure 2. Plant alpha diversity in different land use types. EL, exclosure; ML, mowed land; and GL, grazed land. In the FL, the plant community composition was monospecific, thus it was not included in the analysis. *P < 0.05, **P < 0.01 and ***P < 0.001.
Effects of Land Use Types on Soil Microbial Community Diversity and Composition
A total of 1,223,170 and 1,176,336 high-quality sequences of bacterial and fungal communities were obtained from 12 samples after quality filtering steps. Bacterial sequences ranged from 92,489 to 107,410 per sample (mean = 101,931), whereas fungal sequences ranged from 87,512 to 106,646 per sample (mean = 98,028). The sequences were clustered into 32,269 bacterial and 6,267 fungal OTUs, with a 97% identity threshold. The Good’s coverage index for all plots was higher than 99.18 ± 0.68%, indicating that the sequencing effort was sufficient. For the bacterial community, the EL and FL had significantly higher Chao1 richness and Shannon indexes than the GL and ML, and no differences were found in the Simpson index among the plots. In the fungal community, a significantly higher Shannon index was observed for the FL than for the EL, while there were no significant differences among plots for the Chao1 and Simpson indexes (Table 3). Spearman’s analysis showed that soil pH, EC, and the plant Simpson index were significantly negatively correlated with bacterial richness according to the Chao1 index (r = −0.967, P = 0.033; r = −0.955, P = 0.045; r = −0.979, P = 0.021, respectively), while the fungal Shannon diversity was positively correlated with AP (r = 0.977, P = 0.023) and negatively correlated with the Shannon and Pielou’s indexes for the plants (r = −0.954, P = 0.046; r = −0.981, P = 0.019, respectively) (Supplementary Table 1).
Principal coordinates analysis was performed to visualize the changes in microbial community composition based on the Bray–Curtis distance (Figure 3). The first two axis of the PCoA explained 44.3 and 21.7% of the overall variation in the bacterial composition (Figure 3A). The PERMANOVA results showed that the profiles of the bacterial communities in the four land use types were clearly separated (R2 = 0.7099, P = 0.001), and BETADISPER was not significantly different (P = 0.08) (Supplementary Figure 3A). The bacterial communities in the GL and FL were significantly different from those in the EL and ML, and similar community compositions were observed in the EL and ML. For the fungi, the first two PCoA axis explained 22.6 and 18.5% of the overall variation in the community composition (Figure 3B). The PERMANOVA indicated that there was a significant difference in fungal community composition under different land use types (R2 = 0.4802, P = 0.001), and analyses of dispersion were no significant (P = 0.93) (Supplementary Figure 3B). Moreover, the fungal community in the EL clustered relatively far from those in the GL, FL, and ML along the PCo1 axis, which indicates that the structure of the soil fungal community in the EL differed markedly from those in the other land use types.
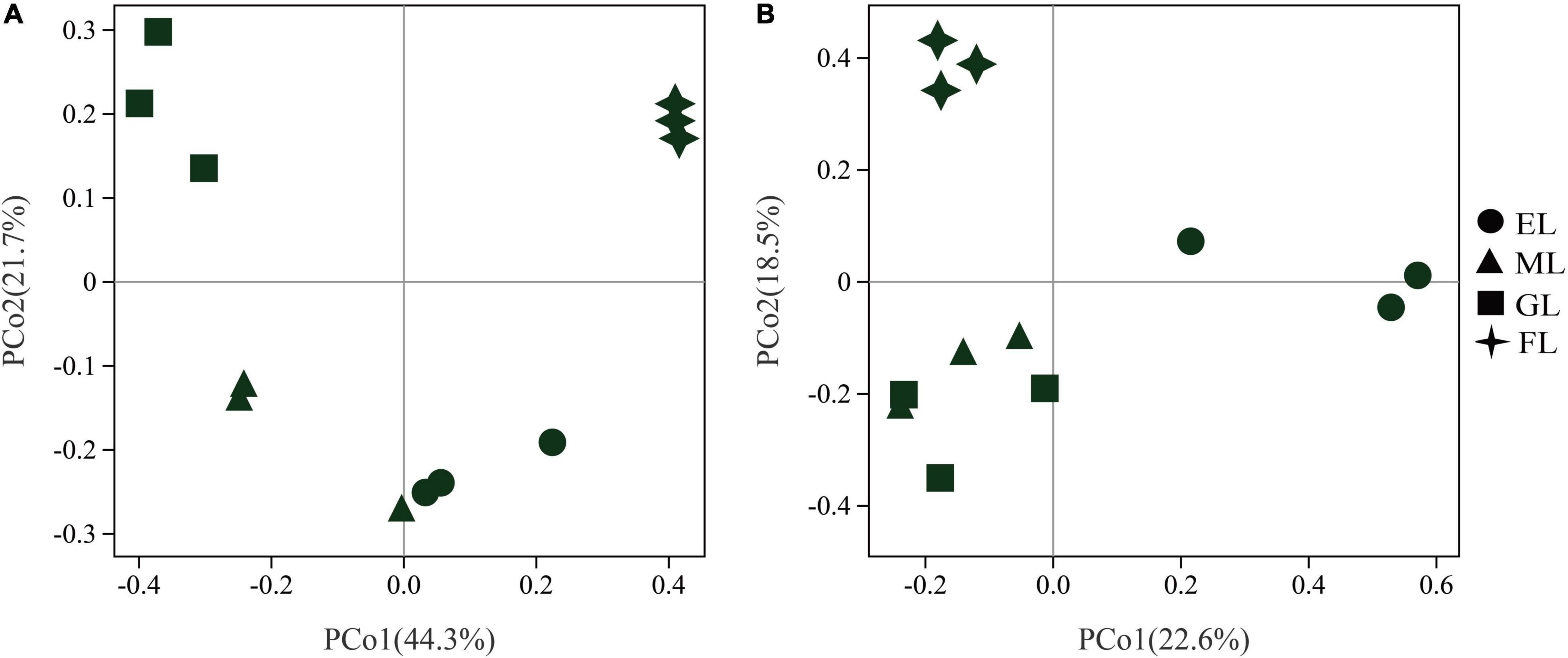
Figure 3. Principal component analysis (PCoA) of bacterial (A) and fungal (B) communities in different land use types. EL, exclosure; ML, mowed land; GL, grazed land; and FL, farmland.
Considering all sequences, the dominant phyla of soil bacteria across all samples (with relative abundance >4%) were Proteobacteria (25.8%), Acidobacteria (19.5%), Gemmatimonadetes (18.2%), Planctomycetes (12.6%), Chloroflexi (6.1%), Actinobacteria (4.3%), and Rokubacteria (4.1%) in the soil profiles (Figure 4A and Supplementary Figure 1A). In detail, compared to the GL and ML, the EL and FL had a significantly higher relative abundance of Proteobacteria and a significantly lower relative abundance of Chloroflexi (no significant in FL). The GL had a higher abundance of Gemmatimonadetes and a lower abundance of Acidobacteria than the other land use types. The highest abundances of Actinobacteria and Rokubacteria were observed in the ML. Moreover, LEfSe analysis with a threshold of 2 showed that Acidobacteria was enriched in FL; Proteobacteria in EL; Gemmatimonadetes in GL; and Actinobacteria in ML (Supplementary Figure 2A). The dominant fungal phyla across all the plots were Ascomycota (55.9%), followed by Basidiomycota (19.5%), Mortierellomycota (6.5%), and Chytridiomycota (0.8%) (Figure 4B and Supplementary Figure 1B). The highest abundance of Ascomycota was found in the GL. Similarly, the relative abundance of Basidiomycota in the EL was considerably different from that in the other land use types. Significantly higher relative abundances of Mortierellomycota and Chytridiomycota were also recorded in the FL than in the other land use types. In addition, the LEfSe analysis revealed that Ascomycota was the fungal indicator group in the ML and GL; Basidiomycota in the EL; and Ascomycota, Basidiomycota, and Chytridiomycota in the FL (Supplementary Figure 2B). In addition, in the analyzed plots, the abundance of Proteobacteria was positively correlated with TN (r = 0.989, P = 0.011), while the abundance of Ascomycota was negatively correlated with TN (r = −0.972, P = 0.028) (Supplementary Tables 2, 3).
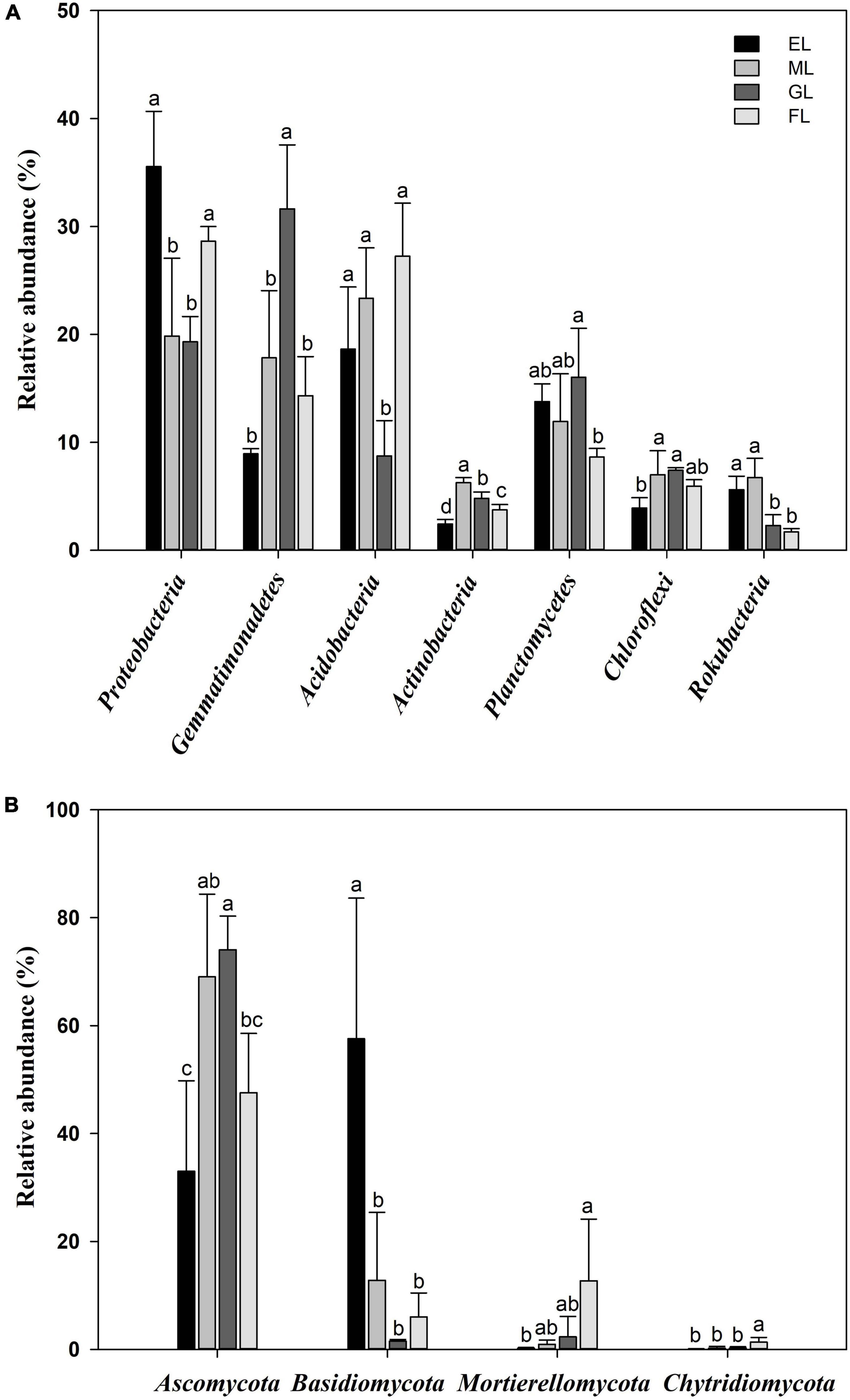
Figure 4. Relative abundances of the dominant bacterial taxa (A) and fungal taxa (B) at the phylum level in different land use types. Different letters indicate a significant difference (P < 0.05). EL, exclosure; ML, mowed land; GL, grazed land; and FL, farmland.
Relationships Between Biotic and Abiotic Properties and Microbial Communities
We performed RDA to illustrate the relationship between microbial community composition and environmental factors. The first two RDA axis explained 58.8 and 57.4% of the total variation in the overall compositions of the bacterial and fungal communities, respectively (Figure 5). The RDA results showed that EC (P = 0.002), SOM (P = 0.004), and Pielou’s evenness index for plants (P = 0.008) significantly influenced the composition of the bacterial community, while TN (P = 0.002) and the Shannon diversity of plants (P = 0.014) significantly affected the composition of the fungal community (Supplementary Table 4).
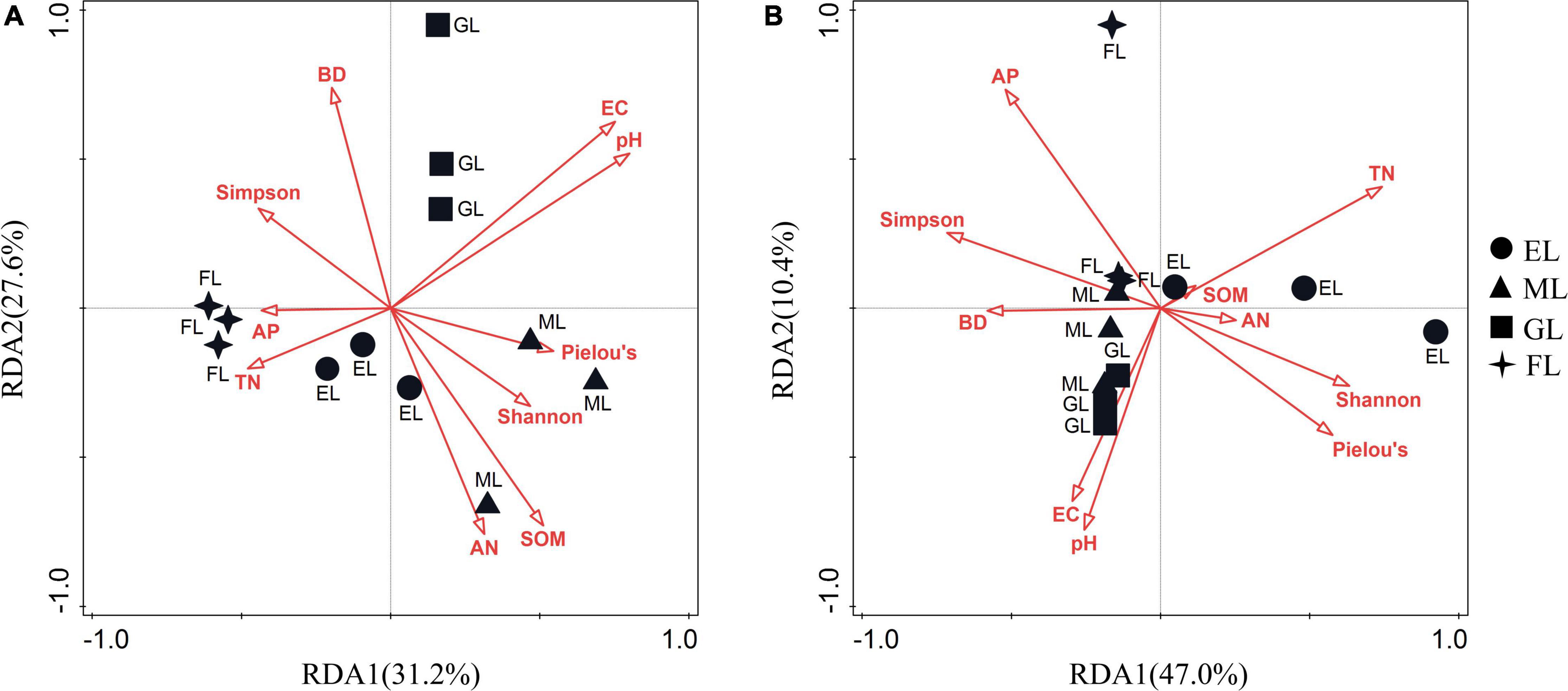
Figure 5. Redundancy analyses (RDA) of pooled data on bacterial (A) and fungal (B) communities with biotic and abiotic variables. The red vectors represent trajectories of environmental parameters as independent variables. EL, exclosure; ML, mowed land; GL, grazed land; and FL, farmland.
The model provided a good fit among vegetation parameters, soil properties, and microbial community composition (P = 0.798, χ2 = 3.843, GFI = 0.915, RMSEA = 0.000, AIC = 45.843) (Figure 6). Our SEM results showed that soil pH and EC exhibited a direct positive correlation with the soil bacterial community (P < 0.001), while the plant Simpson index had a direct negative effect on the soil bacterial community (P < 0.001). Plant taxonomic diversity (Shannon diversity and Pielou’s evenness) affected the bacterial community composition indirectly by changing the soil pH and EC. The value of Pielou’s evenness index of plants directly and positively affected the soil pH and EC and fungal community composition (P < 0.001). In addition, the value of the Shannon diversity index of plants had a significantly negative correlation with soil pH and EC and the fungal community composition (P < 0.001), while the soil nutrient contents had a significantly positive and direct effect on the soil fungal community composition (P < 0.001).
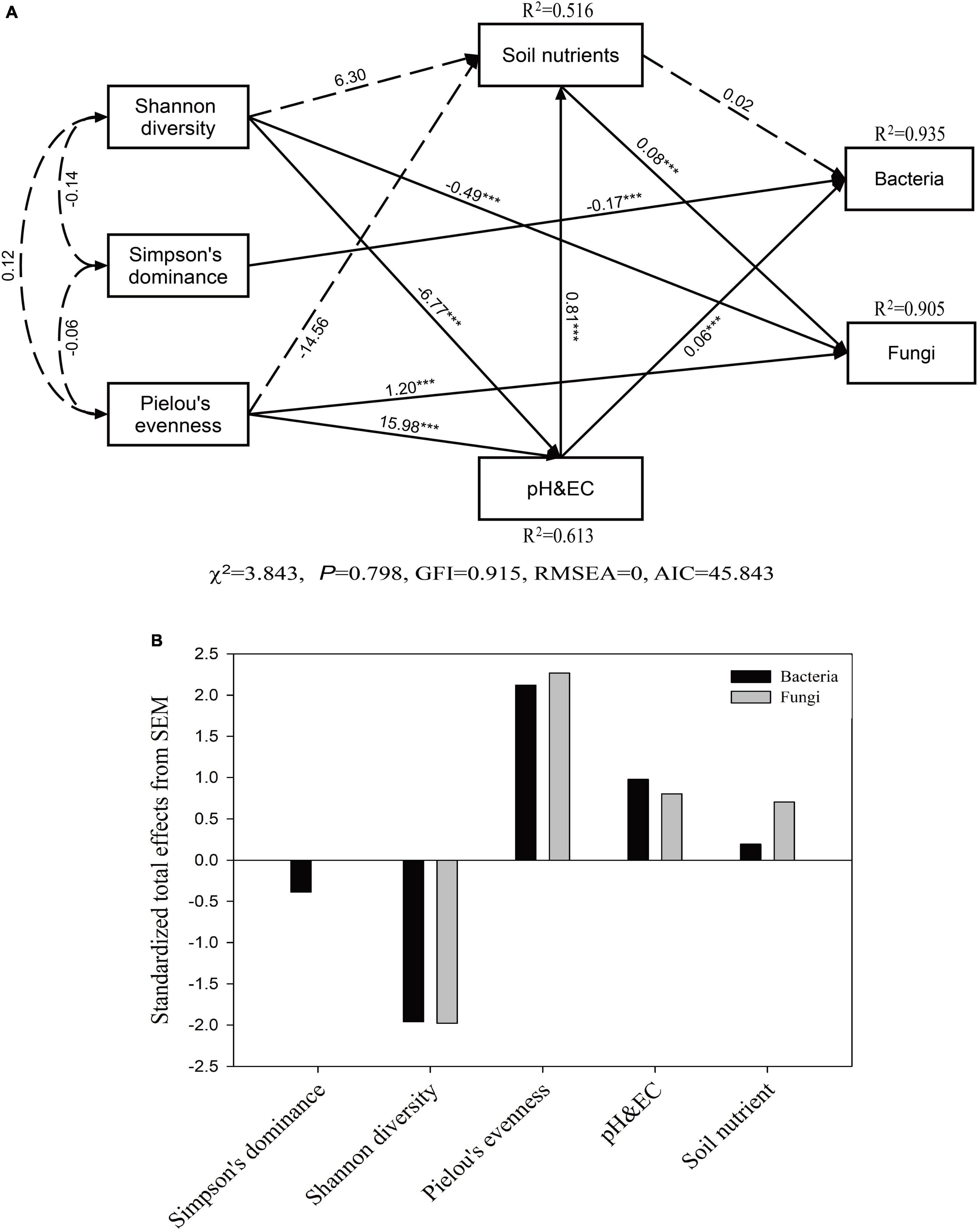
Figure 6. Structural equation model (SEM) based on the effects of plant community composition (Shannon, Simpson’s, and Pielou’s indexes) and soil properties (soil nutrients, pH, and EC) on soil microbial communities (A) and the standardized total effects on plant community composition and soil properties based on the SEM (B). Continuous arrows represent positive or negative relationships, and adjacent numbers in the same direction as the arrows indicate path coefficients. The R2 values indicate the proportion of variance explained by each variable in the model. The significance levels are denoted as follow: ***P < 0.001.
Discussion
Effects of Land Use Types on Soil and Plant Properties
The biotic and abiotic properties represented strong changes that varied with land use type and were correlated with higher microbial activity, more plant litter, and more intense human disturbance (Bargali et al., 2019). The GL had a higher pH and EC due to the return of animal dung and urine to the soil surface. In contrast, our results showed that the FL had a lower soil pH and EC. On the one hand, the amount of fertilizer applied in agricultural production is often greater than the plant absorption capacity, leading to the loss of some fertilizer from the soil through leaching; this process is accompanied by the loss of many alkaline cations, while the H+ produced in the nitrification process remains in the soil, reducing the soil pH (Schirmel et al., 2018). On the other hand, crop harvesting removes biomass (e.g., grains, straw) and OH– from plants, which aggravates the surplus of acid in the soil. Interestingly, we also noticed that a higher content of phosphorus was found in the FL than in the other land use types, which may be explained by the input of chemical fertilizer to offset the loss of nutrients. Many studies have shown that SOC and TN are important components of soil fertility and are closely related to ecosystem stability and environmental sustainability (Shi et al., 2020). Grazing consumed most of the aboveground plant biomass and promoted soil compaction, which significantly decreased plant diversity and richness, leading to a smaller quantity of plant litter inputs to the soil and thus lower C and N contents (Yang et al., 2019; Oggioni et al., 2020). The EL, which was not used for grazing or mowing for 3 years, had relatively high plant biomass and diversity (according to Shannon and Pielou’s indexes, respectively), which contributed to minimizing soil erosion and nutrient leaching; thus, the nutrients released by litter decomposition accumulated in the soil (Huhe et al., 2017). Appropriate annual mowing increased plant richness and diversity by reducing the competitive exclusion of dominant species and accelerated soil nutrient cycling (e.g., N and P cycling) (Wang et al., 2021). In addition, mowing increased the SOM content by increasing root exudates and plant residues during growth, resulting in a continuous flow of carbon into the soil (Chan et al., 2011).
Effects of Land Use Types on Soil Microbial Community Diversity and Composition
Land use type had a strong driving effect on microbial community diversity. In this study, higher bacterial α diversity (measured by the Chao1 richness and Shannon indexes) occurred in the EL and FL. Undisturbed grasslands have rich plant communities, large amounts of biomass and litter, and diverse root exudates, which create a suitable habitat for soil microorganisms, leading to structural changes in the soil bacterial community and a positive relationship between plants and microbial diversity (Zhang et al., 2016). After the grassland was converted to farmland, frequent disturbances caused a decrease in abundances of the dominant OTUs and an increase in diversity, and OTUs were replaced by competitors (George et al., 2019). Grazing or mowing resulted in lower returns of organic carbon, nitrogen, and other nutrients to the soil and reduced available substrate resources for microorganisms, resulting in intensified competition. All these factors affect the soil environment and reduce the soil bacterial diversity (Oggioni et al., 2020). In addition, our study also detected a significantly higher Shannon index among the fungi in the FL across all plots. Human activities, especially agricultural management, have an evident effect on the composition of vegetation, the levels of soil water and heat, and the mineralization of soil organic matter, leading to structural changes in the soil fungal community that can lead to the emergence of diversity and new species (Arévalo-Gardini et al., 2020). Fungi can adapt to phosphorus input levels, giving them a competitive advantage over many bacteria (Yan and Feng, 2020). Therefore, increasing soil nutrients through fertilizer application might also have a positive effect on fungal communities.
Land use change also affected the microbial community composition and reshaped the unique keystone taxa in our study. Among the bacteria, the rate of species replacement in the GL was relatively fast, which might be due to soil nutrient scarcity and intensified competition, leading to intensified species replacement among communities. The abundance of the phylum Gemmatimonadetes was higher in the GL than in the other land use types; members of this phylum contribute to the recycling of essential nutrients and decomposition of cellulose and lignin and are positively correlated with soil bulk density (Xu et al., 2019). In contrast, fertilization results in a large input of nutrients to enhance the metabolic activity of Acidobacteria, which is important for soil carbon and nitrogen cycling (Kielak et al., 2016). The high Proteobacteria abundance in the EL was consistent with the results reported by Ding et al. (2017), who observed a relatively high abundance of Proteobacteria in soils with high nitrogen contents. Moreover, the abundance of Actinobacteria, which contributed to the degradation and mineralization of litter in the soil and may have contributed to the high SOC contents in the plots, was higher in the ML than in the other land use types (Yu et al., 2020). In addition, the bacterial communities in the EL and ML were more similar than those in the other land use types, indicating that the EL and ML had more similar substrate compositions. The accumulation of recalcitrant organic compounds in the EL supports the activities of fungi associated with the decomposition of recalcitrant compounds, i.e., an increase in the number of Basidiomycota, which triggered a change in the soil fungal community structure (Wang J. C. et al., 2020). In addition, Chytridiomycota were recovered in the FL, probably due to their ability to tolerate regular use of pesticides and fertilizers, which are continuously required in agricultural practices (Gleason et al., 2004). Similarly, the abundance of Mortierellomycota, which have utilized simple carbohydrates efficiently, was higher in the FL than in the other land use types.
Relationships Between Biotic and Abiotic Properties and Microbial Communities
Land use change had an obvious impact on the soil bacterial and fungal community compositions in the Songnen grassland ecosystem. Studies have found that soil pH is equally important as salinity in shaping bacterial communities in saline soils (Zhao et al., 2018). Generally, soil pH has long been recognized as a primary driver for bacterial communities (Tripathi et al., 2018). On the other hand, high soil salinity (EC) could limit plant water and nutrient uptake due to low osmotic potential and negatively affect the biomass, activity, and biochemical processes of soil microorganisms (Yang et al., 2020). Our structural model confirms this finding. In our study, soil pH and EC exhibited collinearity and were highly correlated with bacterial diversity, which affected the composition of the bacterial community. Notably, plant diversity may directly affect the soil microbial community structure through resource allocation and nutrient supply, and can also regulate bacterial communities by changing the soil environment (Shen et al., 2021). In this study, frequent grazing or mowing removed a large number of species from the plant community, thus reducing plant diversity and affecting bacterial distribution indirectly by changing soil pH and EC. In addition, the SEM provided evidence that plant and soil properties were highly correlated with the fungal community structure. Not surprisingly, changes in soil nutrients directly affect fungal communities (Huhe et al., 2017). We further found that plant diversity seemed to play a key role in changes in the fungal communities. Plant richness could drive the distribution of soil microorganisms, which may be explained by the fact that higher species richness will modify resource availability and microclimates, creating more niches in the soil and altering the soil fungal composition (Chen et al., 2018a). On the other hand, communities with increased plant richness have a higher chance of including key plant species, and these key species or functional groups will strongly influence the soil fungal composition (Shen et al., 2021). In addition, our study also found that the Shannon diversity of the plant communities had a negative effect on the fungal composition. A study by Chen et al. (2018b) found that soil microbes depend more on the presence of a specific plant than on plant diversity itself.
It is worth mentioning that pseudo-replication is a limitation of this study. The effects of land use types on bacterial and fungal communities were studied within a region rather than across multiple regions, so our different sampling sites should be regarded as geographical pseudo-replications. This limitation should be addressed in future studies. However, since each site within a given region was separated by at least 2 km, we believe that the site effects are almost certainly than any possible random differences, and inferences are drawn from pseudo-replicated designs.
Conclusion
In summary, this study revealed the relationship between the microbial community composition and plant and soil properties in different grassland uses of the Songnen Plain. Our study found that changes in land use types distinctly influenced on the plant community composition, soil properties, and abundances of dominant microbial groups. In addition, we found that plant community evenness, as measured by Pielou’s evenness index and soil nutrient contents might be the key drivers of fungal community formation, whereas the bacterial community was more affected by changes in pH and EC (salinity) during land use. Further research should focus on the links between soil microbial communities and functions to address how land use types affect important ecosystem processes.
Data Availability Statement
The high-throughput sequencing data have been deposited in the Sequence Read Archive (SRA) of the NCBI database under the BioProject ID PRJNA805040: https://www.ncbi.nlm.nih.gov/sra/PRJNA805040.
Author Contributions
GL, ZB, and GC conceived and designed the study. GL drafted the original manuscript. ZB provided constructive suggestions for revisions. WH provided to the study site. ZK, GJ, HG, and DL contributed to the sampling and data analysis. All authors read and approved the final manuscript.
Funding
This project was supported by the National Key R&D Program of China (2016YFC0500607).
Conflict of Interest
The authors declare that the research was conducted in the absence of any commercial or financial relationships that could be construed as a potential conflict of interest.
Publisher’s Note
All claims expressed in this article are solely those of the authors and do not necessarily represent those of their affiliated organizations, or those of the publisher, the editors and the reviewers. Any product that may be evaluated in this article, or claim that may be made by its manufacturer, is not guaranteed or endorsed by the publisher.
Acknowledgments
We gratefully acknowledge students from the Grassland Laboratory at Northeast Agricultural University and the staff of Qiqihar Grassland Station for their help with fieldwork.
Supplementary Material
The Supplementary Material for this article can be found online at: https://www.frontiersin.org/articles/10.3389/fmicb.2022.865184/full#supplementary-material
References
Anderson, M. J. (2001). A new method for non-parametric multivariate analysis of variance. Austral Ecol. 26, 32–46.
Anderson, M. J., Ellingsen, K. E., and McArdle, B. H. (2006). Multivariate dispersion as a measure of beta diversity. Ecol. Lett. 9, 683–693. doi: 10.1111/j.1461-0248.2006.00926.x
Arévalo-Gardini, E., Canto, M., Alegre, J., Arévalo-Hernández, C. O., Loli, O., Julca, A., et al. (2020). Cacao agroforestry management systems effects on soil fungi diversity in the Peruvian Amazon. Ecol. Indic. 115:106404. doi: 10.1016/j.ecolind.2020.106404
Bargali, S. S., Padalia, K., and Bargali, K. (2019). Effects of tree fostering on soil health and microbial biomass under different land use systems in the Central Himalayas. Land Degrad Dev. 30, 1984–1998. doi: 10.1002/ldr.3394
Bremner, J. M., and Mulvaney, C. S. (1982). “Nitrogen-total,” in Methods of Soil Analysis. Part 2. Chemical and Microbiological Properties, eds A. L. Page, R. H. Miller, and D. R. Keeney (Illinois, ILL: ASA, Soil Science Society of America), 595–624.
Caporaso, J. G., Kuczynski, J., Stombaugh, J., Bittinger, K., Bushman, F. D., Costello, E. K., et al. (2010). QIIME allows analysis of high-throughput community sequencing data. Nat. Methods 7, 335–336. doi: 10.1038/nmeth.f.303
Chan, K. Y., Conyers, M. K., Li, G. D., Helyar, K. R., Poile, G., Oates, A., et al. (2011). Soil carbon dynamics under different cropping and pasture management in temperate Australia: results of three long-term experiments. Soil Res. 49, 320–328. doi: 10.1071/Sr10185
Chen, L. L., Baoyin, T., and Minggagud, H. (2021b). Effects of mowing regimes on above- and belowground biota in semi-arid grassland of northern China. J. Environ. Manage. 277:111441. doi: 10.1016/j.jenvman.2020.111441
Chen, L. L., Xu, H. B., Wu, S. Y., and Baoyin, T. (2021a). Plant and soil properties mediate the response of soil microbial communities to moderate grazing in a semiarid grassland of northern China. J. Environ. Manage. 284:112005. doi: 10.1016/j.jenvman.2021.112005
Chen, W. Q., Xu, R., Chen, J., Yuan, X. P., Zhou, L., Tan, T. Y., et al. (2018a). Consistent responses of surface- and subsurface soil fungal diversity to N enrichment are mediated differently by acidification and plant community in a semi-arid grassland. Soil Biol. Biochem. 127, 110–119. doi: 10.1016/j.soilbio.2018.09.020
Chen, W. Q., Xu, R., Wu, Y. T., Chen, J., Zhang, Y. J., Hu, T. M., et al. (2018b). Plant diversity is coupled with beta not alpha diversity of soil fungal communities following N enrichment in a semi-arid grassland. Soil Biol. Biochem. 116, 388–398. doi: 10.1016/j.soilbio.2017.10.039
Dang, H. Y., Li, J., Chen, R. P., Wang, L., Guo, L. Z., Zhang, Z. N., et al. (2010). Diversity, abundance, and spatial distribution of sediment ammonia-oxidizing betaproteobacteria in response to environmental gradients and coastal eutrophication in jiaozhou Bay, China. Appl. Environ. Microb. 76, 4691–4702. doi: 10.1128/Aem.02563-09
Dassen, S., Cortois, R., Martens, H., de Hollander, M., Kowalchuk, G. A., van der Putten, W. H., et al. (2017). Differential responses of soil bacteria, fungi, archaea and protists to plant species richness and plant functional group identity. Mol. Ecol. 26, 4085–4098. doi: 10.1111/mec.14175
Deng, L., Wang, K. B., and Shangguan, Z. P. (2014). Long-term natural succession improves nitrogen storage capacity of soil on the Loess Plateau. China. Soil Res. 52, 262–270. doi: 10.1071/Sr12377
Ding, L. J., Su, J. Q., Li, H., Zhu, Y. G., and Cao, Z. H. (2017). Bacterial succession along a long-term chronosequence of paddy soil in the Yangtze River Delta. China. Soil Biol. Biochem. 104, 59–67. doi: 10.1016/j.soilbio.2016.10.013
Drenovsky, R. E., Steenwerth, K. L., Jackson, L. E., and Scow, K. M. (2010). Land use and climatic factors structure regional patterns in soil microbial communities. Global Ecol Biogeogr. 19, 27–39. doi: 10.1111/j.1466-8238.2009.00486.x
Edgar, R. C., Haas, B. J., Clemente, J. C., Quince, C., and Knight, R. (2011). UCHIME improves sensitivity and speed of chimera detection. Bioinformatics 27, 2194–2200. doi: 10.1093/bioinformatics/btr381
Engelhardt, I. C., Welty, A., Blazewicz, S. J., Bru, D., Rouard, N., Breuil, M. C., et al. (2018). Depth matters: effects of precipitation regime on soil microbial activity upon rewetting of a plant-soil system. ISME J. 12, 1061–1071. doi: 10.1038/s41396-018-0079-z
Fang, C., Ke, W. B., Campioli, M., Pei, J. Y., Yuan, Z. Q., Song, X., et al. (2020). Unaltered soil microbial community composition, but decreased metabolic activity in a semiarid grassland after two years of passive experimental warming. Ecol. Evol. 10, 12327–12340. doi: 10.1002/ece3.6862
Fuchslueger, L., Wild, B., Mooshammer, M., Takriti, M., Kienzl, S., Knoltsch, A., et al. (2019). Microbial carbon and nitrogen cycling responses to drought and temperature in differently managed mountain grasslands. Soil Biol. Biochem. 135, 144–153. doi: 10.1016/j.soilbio.2019.05.002
George, P. B. L., Lallias, D., Creer, S., Seaton, F. M., Kenny, J. G., Eccles, R. M., et al. (2019). Divergent national-scale trends of microbial and animal biodiversity revealed across diverse temperate soil ecosystems. Nat. Commun. 10:1107. doi: 10.1038/s41467-019-09031-1
Gilliam, F. S., Walter, C. A., Adams, M. B., and Peterjohn, W. T. (2018). Nitrogen (N) dynamics in the mineral soil of a central appalachian hardwood forest during a quarter century of whole-watershed N additions. Ecosystems 21, 1489–1504. doi: 10.1007/s10021-018-0234-4
Gleason, F. H., Letcher, P. M., and McGee, P. A. (2004). Some Chytridiomycota in soil recover from drying and high temperatures. Mycol. Res. 108, 583–589. doi: 10.1017/S0953756204009736
Gong, Z., Gu, G., and Zhou, R. (2011). Formation and classification of anthrosols: China’s perspective. Acapulco 6, 120–128.
Guo, Y. Q., Xu, T. Q., Cheng, J. M., Wei, G. H., and Lin, Y. B. (2021). Above- and belowground biodiversity drives soil multifunctionality along a long-term grassland restoration chronosequence. Sci. Total Environ. 772:145010. doi: 10.1016/j.scitotenv.2021.145010
Han, D. M., Wang, G. Q., Xue, B. L., Liu, T. X., Yinglan, A., and Xu, X. Y. (2018). Evaluation of semiarid grassland degradation in North China from multiple perspectives. Ecol. Eng. 112, 41–50. doi: 10.1016/j.ecoleng.2017.12.011
Huhe Chen, X. J., Hou, F. J., Wu, Y., and Cheng, Y. X. (2017). Bacterial and fungal community structures in loess plateau grasslands with different grazing intensities. Front. Microbiol. 8:606. doi: 10.3389/fmicb.2017.00606
Kielak, A. M., Barreto, C. C., Kowalchuk, G. A., van Veen, J. A., and Kuramae, E. E. (2016). The ecology of acidobacteria: moving beyond genes and genomes. Front. Microbiol. 7:744. doi: 10.3389/fmicb.2016.00744
Krustal, W. H., and Wallis, W. A. (1952). Use of ranks in one-criterion variance analysis. J. Am. Stat. Assoc. 47, 583–621.
Li, C. H., Veum, K. S., Goyne, K. W., Nunes, M. R., and Acosta-Martinez, V. (2021). A chronosequence of soil health under tallgrass prairie reconstruction. Appl. Soil Ecol. 164:103939. doi: 10.1016/j.apsoil.2021.103939
Li, J. Y., Zhang, Q. C., Li, Y., Liu, J., Pan, H., Guan, X. M., et al. (2017). Impact of mowing management on nitrogen mineralization rate and fungal and bacterial communities in a semiarid grassland ecosystem. J. Soil Sediment 17, 1715–1726. doi: 10.1007/s11368-016-1620-1
Lu, R. (1999). Soil Agricultural Chemical Analysis Method. Beijing: Chinese Agriculture and Sciences Press.
Magoc, T., and Salzberg, S. L. (2011). FLASH: fast length adjustment of short reads to improve genome assemblies. Bioinformatics 27, 2957–2963. doi: 10.1093/bioinformatics/btr507
Martí-Roura, M., Hagedorn, F., Rovira, P., and Romanyà, J. (2019). Effect of land use and carbonates on organic matter stabilization and microbial communities in Mediterranean soils. Geoderma 351, 103–115. doi: 10.1016/j.geoderma.2019.05.021
Merloti, L. F., Mendes, L. W., Pedrinho, A., de Souza, L. F., Ferrari, B. M., and Tsai, S. M. (2019). Forest-to-agriculture conversion in Amazon drives soil microbial communities and N-cycle. Soil Biol. Biochem. 137:107567. doi: 10.1016/j.soilbio.2019.107567
Oggioni, S. D., Ochoa-Hueso, R., and Peco, B. (2020). Livestock grazing abandonment reduces soil microbial activity and carbon in a Mediterranean Dehesa. Appl. Soil Ecol. 153:103588. doi: 10.1016/j.apsoil.2020.103588
Quast, C., Pruesse, E., Yilmaz, P., Gerken, J., Schweer, T., Yarza, P., et al. (2013). The SILVA ribosomal RNA gene database project: improved data processing and web-based tools. Nucleic Acids Res. 41, 590–596. doi: 10.1093/nar/gks1219
Santos, S. S., Scholer, A., Nielsen, T. K., Hansen, L. H., Schloter, M., and Winding, A. (2020). Land use as a driver for protist community structure in soils under agricultural use across Europe. Sci. Total Environ. 717:137228. doi: 10.1016/j.scitotenv.2020.137228
Schirmel, J., Albert, J., Kurtz, M. P., and Munoz, K. (2018). Plasticulture changes soil invertebrate assemblages of strawberry fields and decreases diversity and soil microbial activity. Appl. Soil Ecol. 124, 379–393. doi: 10.1016/j.apsoil.2017.11.025
Segata, N., Izard, J., Waldron, L., Gevers, D., Miropolsky, L., Garrett, W. S., et al. (2011). Metagenomic biomarker discovery and explanation. Genome Biol. 12:R60. doi: 10.1186/gb-2011-12-6-r60
Shen, C., Wang, J., Jing, Z. W., Qiao, N. H., Xiong, C., and Ge, Y. (2021). Plant diversity enhances soil fungal network stability indirectly through the increase of soil carbon and fungal keystone taxa richness. Sci. Total Environ. 818:151737. doi: 10.1016/j.scitotenv.2021.151737
Shi, A. D., Zhou, X., Yao, S. H., and Zhang, B. (2020). Effects of intensities and cycles of heating on mineralization of organic matter and microbial community composition of a Mollisol under different land use types. Geoderma 357:113941. doi: 10.1016/j.geoderma.2019.113941
Shrestha, P., Gautam, R., and Ashwath, N. (2019). Effects of agronomic treatments on functional diversity of soil microbial community and microbial activity in a revegetated coal mine spoil. Geoderma 338, 40–47. doi: 10.1016/j.geoderma.2018.11.038
Sparks, D. L., Page, A., Helmke, P., Loeppert, R., Soltanpour, P., Tabatabai, M., et al. (1996). Methods of Soil Analysis. Part 3-Chemical Methods. Madison, WI: Soil Science Society of America Inc.
Szoboszlay, M., Dohrmann, A. B., Poeplau, C., Don, A., and Tebbe, C. C. (2017). Impact of land-use change and soil organic carbon quality on microbial diversity in soils across Europe. FEMS Microbiol. Ecol. 93:fix146. doi: 10.1093/femsec/fix146
Tripathi, B. M., Stegen, J. C., Kim, M., Dong, K., Adams, J. M., and Lee, Y. K. (2018). Soil pH mediates the balance between stochastic and deterministic assembly of bacteria. ISME J. 12, 1072–1083. doi: 10.1038/s41396-018-0082-4
Tscherko, D., Hammesfahr, U., Marx, M. C., and Kandeler, E. (2004). Shifts in rhizosphere microbial communities and enzyme activity of Poa alpina across an alpine chronosequence. Soil Biol. Biochem. 36, 1685–1698. doi: 10.1016/j.soilbio.2004.07.004
Wang, D., Huang, X. D., Qiao, N., Geng, Q. L., Liu, Y. Z., Song, H. Q., et al. (2021). Effects of mowing and fertilization on soil quality in a semiarid grassland of North China. Land Degrad. Dev. 32, 1656–1666. doi: 10.1002/ldr.3783
Wang, J., Liu, G. B., Zhang, C., Wang, G. L., Fang, L. C., and Cui, Y. X. (2019). Higher temporal turnover of soil fungi than bacteria during long-term secondary succession in a semiarid abandoned farmland. Soil Till Res. 194:104305. doi: 10.1016/j.still.2019.104305
Wang, J. C., Zou, Y. K., Di Gioia, D., Singh, B. K., and Li, Q. F. (2020). Conversion to agroforestry and monoculture plantation is detrimental to the soil carbon and nitrogen cycles and microbial communities of a rainforest. Soil Biol. Biochem. 147:107849. doi: 10.1016/j.soilbio.2020.107849
Wang, Q., Garrity, G. M., Tiedje, J. M., and Cole, J. R. (2007). Naive Bayesian classifier for rapid assignment of rRNA sequences into the new bacterial taxonomy. Appl. Environ. Microb. 73, 5261–5267. doi: 10.1128/Aem.00062-07
Wang, X. Y., Li, F. Y., Tang, K. Y., Wang, Y. N., Suri, G., Bai, Z., et al. (2020). Land use alters relationships of grassland productivity with plant and arthropod diversity in Inner Mongolian grassland. Ecol. Appl. 30:e02052. doi: 10.1002/eap.2052
Wu, X. F., Yang, J. J., Ruan, H., Wang, S. N., Yang, Y. R., Naeem, I., et al. (2021). The diversity and co-occurrence network of soil bacterial and fungal communities and their implications for a new indicator of grassland degradation. Ecol. Indic. 129:107989. doi: 10.1016/j.ecolind.2021.107989
Xu, L. X., Han, Y. S., Yi, M., Yi, H. L., Guo, E. H., and Zhang, A. Y. (2019). Shift of millet rhizosphere bacterial community during the maturation of parent soil revealed by 16S rDNA high-throughput sequencing. Appl. Soil Ecol. 135, 157–165. doi: 10.1016/j.apsoil.2018.12.004
Yan, R., and Feng, W. (2020). Effect of vegetation on soil bacteria and their potential functions for ecological restoration in the Hulun Buir Sandy Land. China. J. Arid Land. 12, 473–494. doi: 10.1007/s40333-020-0011-z
Yan, Y., and Lu, X. Y. (2015). Is grazing exclusion effective in restoring vegetation in degraded alpine grasslands in Tibet, China? Peerj 3:e1020.
Yang, C., Wang, X. Z., Miao, F. H., Li, Z. Y., Tang, W., and Sun, J. (2020). Assessing the effect of soil salinization on soil microbial respiration and diversities under incubation conditions. Appl. Soil Ecol. 155:103671. doi: 10.1016/j.apsoil.2020.103671
Yang, F., Niu, K. C., Collins, C. G., Yan, X. B., Ji, Y. G., Ling, N., et al. (2019). Grazing practices affect the soil microbial community composition in a Tibetan alpine meadow. Land Degrad. Dev. 30, 49–59. doi: 10.1002/ldr.3189
Yang, H. J., Jiang, L., Li, L. H., Li, A., Wu, M. Y., and Wan, S. Q. (2012). Diversity-dependent stability under mowing and nutrient addition: evidence from a 7-year grassland experiment. Ecol. Lett. 15, 619–626. doi: 10.1111/j.1461-0248.2012.01778.x
Yu, J., Liu, F., Tripathi, B. M., and Steinberger, Y. (2020). Changes in the composition of soil bacterial and fungal communities after revegetation with Caragana microphylla in a desertified semiarid grassland. J. Arid Environ. 182:104262. doi: 10.1016/j.jaridenv.2020.104262
Zhang, C., Liu, G. B., Xue, S., and Wang, G. L. (2016). Soil bacterial community dynamics reflect changes in plant community and soil properties during the secondary succession of abandoned farmland in the Loess Plateau. Soil Biol. Biochem. 97, 40–49. doi: 10.1016/j.soilbio.2016.02.013
Zhang, Q., Liu, K. S., Shao, X. Q., Li, H., He, Y. X., and Sirimuji, et al. (2021). Microbes require a relatively long time to recover in natural succession restoration of degraded grassland ecosystems. Ecol. Indic. 129:107881. doi: 10.1016/j.ecolind.2021.107881
Zhao, S., Liu, J. J., Banerjee, S., Zhou, N., Zhao, Z. Y., Zhang, K., et al. (2018). Soil pH is equally important as salinity in shaping bacterial communities in saline soils under halophytic vegetation. Sci. Rep. 8:4550. doi: 10.1038/s41598-018-22788-7
Zhong, Z. K., Zhang, X. Y., Wang, X., Fu, S. Y., Wu, S. J., Lu, X. Q., et al. (2020). Soil bacteria and fungi respond differently to plant diversity and plant family composition during the secondary succession of abandoned farmland on the Loess Plateau, China. Plant Soil. 448, 183–200. doi: 10.1007/s11104-019-04415-0
Keywords: land use type, bacterial community, fungal community, soil properties, plant community composition
Citation: Liu G, Bai Z, Cui G, He W, Kongling Z, Ji G, Gong H and Li D (2022) Effects of Land Use on the Soil Microbial Community in the Songnen Grassland of Northeast China. Front. Microbiol. 13:865184. doi: 10.3389/fmicb.2022.865184
Received: 01 February 2022; Accepted: 06 June 2022;
Published: 08 July 2022.
Edited by:
Leonardo Erijman, Consejo Nacional de Investigaciones Científicas y Técnicas (CONICET), ArgentinaReviewed by:
Yang Yang, Northwest A&F University, ChinaAri Jumpponen, Kansas State University, United States
Copyright © 2022 Liu, Bai, Cui, He, Kongling, Ji, Gong and Li. This is an open-access article distributed under the terms of the Creative Commons Attribution License (CC BY). The use, distribution or reproduction in other forums is permitted, provided the original author(s) and the copyright owner(s) are credited and that the original publication in this journal is cited, in accordance with accepted academic practice. No use, distribution or reproduction is permitted which does not comply with these terms.
*Correspondence: Guowen Cui, Y2d3NjAzQDE2My5jb20=