- State Key Laboratory of Animal Nutrition, College of Animal Science and Technology, China Agricultural University, Beijing, China
The gastrointestinal tract (GIT) contains complex microbial communities and plays an essential role in the overall health of the host. Previous studies of beef cattle feed efficiency have primarily concentrated on the ruminal microbiota because it plays a key role in energy production and nutrient supply in the host. Although the small intestine is the important site of post-ruminal digestion and absorption of nutrients, only a few studies have explored the relationship between the microbial populations in the small intestine and feed efficiency. Moreover, variations in GIT metabolites contribute to differences in feed efficiency. The objective of this study was to investigate relationships among bacterial populations of duodenum, jejunum, ileum; microbial metabolites; and RFI phenotype of beef cattle. We carried out by using Illumina MiSeq sequencing of the 16S rRNA V3-V4 region and liquid chromatography-mass spectrometry (LC–MS). In the duodenum, the relative abundances of Firmicutes ( p < 0.01), Lachnospiraceae, Ruminococcaceae, Family_XIII, Christensenellaceae, Christensenellaceae_R-7_group ( p < 0.05), and Lachnospiraceae_NK3A20_group ( p < 0.05) were higher in the low residual feed intake (LRFI) group compared with the high residual feed intake (HRFI) group, whereas the HRFI group had higher abundances of Proteobacteria and Acinetobacter ( p < 0.01). In the jejunum, the relative abundances of Lachnospiraceae and Lachnospiraceae_NK3A20_group were higher in the LRFI group ( p < 0.05). In the ileum, the relative abundances of Ruminococcaceae ( p < 0.01), Christensenellaceae, Christensenellaceae_R-7_group, and Ruminococcus_2 were also higher in the LRFI group ( p < 0.05). Moreover, the genera Lachnospiraceae_NK3A20_group, Christensenellaceae_R-7_group, and Ruminococcus_2 were negatively associated with RFI, while the genus Acinetobacter was positively associated with RFI. The metabolomics analysis revealed that the LRFI group significantly improved protein digestion and absorption, as well as glycerophospholipid metabolism in the duodenum, jejunum, ileum. The correlation between intestinal microorganisms and metabolites revealed that some microorganisms play an important role in amino acid metabolism, glycerophospholipid metabolism, nutrient digestion and absorption, and antioxidant enhancement. The present study provides a better understanding of the small intestinal microbiota and metabolites of beef cattle with different RFI phenotypes and the relationships among them, which are potentially important for the improvement of beef cattle feed efficiency.
Introduction
In beef cattle production, the feed cost according for 60%–75% of the total cost (Herd et al., 2003), requiring beef cattle producers to focus on the feed efficiency trait (Schnepf, 2020). Residual feed intake (RFI) is a good measure of feed efficiency, which is defined as the difference between the actual dry matter intake (DMI) and the predicted DMI based on body size and growth (Herd et al., 2003; Nkrumah et al., 2006). Additionally, RFI is a negative selection trait (Crowley et al., 2010), low-RFI cattle typically consume less feed than high-RFI cattle, which leads to the conclusion that low-RFI cattle are more profitable than high-RFI cattle; therefore, the lower RFI, the higher feed efficiency (Herd et al., 2003). The gastrointestinal tract (GIT) contains complex microbial communities that play an essential role in metabolic, physiological, immunological processes, and the overall health of the host (Fujimura et al., 2010; Hanning and Diaz-Sanchez, 2015). Therefore, studying the differences in the microbial community across the ruminant GIT might provide the relationship between the RFI phenotypes and the microbial of GIT. Because of rumen plays a key role in energy production to the ruminants, many studies of feed efficiency in beef cattle focused on the ruminal microbiota (Jewell et al., 2015; Myer et al., 2015a). The small intestine (SI) is the important site of post-ruminal digestion and absorption of nutrients in beef cattle; however, only a few studies have explored the link between the microbial populations within the lower GIT and the feed efficiency of beef cattle (Myer et al., 2015b, 2016a,b, 2017; Lopes et al., 2019). Especially, a comparison of the segments of the SI (Perea et al., 2017; Freetly et al., 2020). Additionally, microorganisms in different GIT locations might contribute separately to the RFI phenotype of beef cattle. The GIT metabolites are intermediates or products of metabolic processes, and variations in those metabolites contribute to differences in beef cattle feed efficiency (Saleem et al., 2013; Clemmons et al., 2020). It is well known that 16S rRNA sequencing technology is a mature method to analyze cattle GIT bacteria, which has been used to characterize bacterial phylogeny and taxonomy under various experimental parameters and environmental factors. Increasing numbers of studies have used 16S rRNA sequencing technology as a genetic marker to examine multiple factors associated with the cattle GIT bacteria, such as feed efficiency (Kim et al., 2017a,b; McGovern et al., 2018). Besides, metabolomics analysis produces a snapshot of the GIT environment by profiling comprehensively the metabolite abundance in biological samples (Goldansaz et al., 2017). Liquid chromatography-mass spectrometry (LC–MS) technology has been used widely to identify metabolites that differ in beef cattle with high or low feed efficiency (Clemmons et al., 2017; Artegoitia et al., 2019).
This study investigated relationships among bacterial populations of duodenum, jejunum, ileum; microbial metabolites; and the RFI phenotype of beef cattle. We hypothesized that the changes in the composition of small intestinal bacteria and metabolites are associated with the RFI phenotype of beef cattle. Moreover, we hope to provide a more comprehensive analysis of the biological determinants of SI environment and RFI phenotype in beef cattle through correlation analysis.
Materials and Methods
Animals, Diets, Calculation of RFI, Heifer Selection, and Sampling
The animal care and experimental procedures in this study were carried out according to the guidelines of the Laboratory Animal Welfare and Animal Experiment Ethical Committee of China Agricultural University and with their approval (Protocol No. AW08059102-2). A total of 42 Angus heifers (410 ± 25 kg live weight, aged 15 months) were fed with a diet containing 50% concentrate and 50% forage (Supplementary Table 1) according to National Academies of Sciences Engineering and Medicine (2016), and the trial lasted for 144 days (21 days of adaptation, and 123 days of data collection). During this experiment, all conditions were consistent, heifers had ad libitum access to water and feed. Feeding tank automatic records feed intake of each heifer’s through electronic ear tag (Zhenghong Agriculture and Animal Husbandry Machinery and Equipment Co, Shanghai, China). Weigh at the beginning, the end, as well as 14-days intervals of the experiment. The average daily gain (ADG) was computed as the coefficient of the linear regression of body weight (BW; kg) on time (d) using the PROC REG component of the SAS package (SAS Inst., Inc., Cary, NC, United States). The metabolic body weight (MBW) was computed as the midtest BW0.75 (Nkrumah et al., 2004). The expected DMI of each heifer was modeled and predicted by the MBW, ADG, and actual DMI with PROC REG (Nkrumah et al., 2004). The RFI was defined as the difference between the actual and the expected DMI using the following model (Lancaster et al., 2014):
in which β0 is the y-intercept, β1 is the regression coefficient of MBW, β2 is the regression coefficient of ADG, and is the RFI. RFI SDs above and below the mean were used to divide heifers into high (>0.5 SD) group and low RFI (<0.5 SD) group (Nkrumah et al., 2004).
Finally, for all heifers, the five maximum RFI values and the five minimum RFI values were selected to slaughter. The duodenum, jejunum, and ileum contents were collected in plastic sterile containers (Lopes et al., 2019) at slaughter, and frozen immediately in liquid nitrogen, then stored at −80°C until subsequent microbial DNA extraction and metabolomic analysis. The RFI values and animal performance are shown in Table 1 and Supplementary Tables 2, 3.
DNA Extraction, 16S rRNA Gene Amplification, and Sequencing
One ileum sample was damaged from the HRFI group and one ileum sample was damaged from the LRFI group. Therefore, 16S rRNA and metabolomic analysis were only performed for the remaining 28 SI contents. Microbial DNA was extracted from the duodenum, jejunum, and ileum samples using an E.Z.N.A.® soil DNA Kit (Omega Bio-tek, Norcross, GA, United States) following the manufacturer’s protocols. Subsequently, the amplification and sequencing process of the 16S rRNA gene was described by Liu et al. (2019).
Sequence Processing and Analysis
Raw sequences were quality-filtered using fastp version 0.20.0 (Chen et al., 2008) and merged by FLASH version 1. 2. 7 (Magoč and Salzberg, 2011) with the following criteria: (1) The 300 bp reads were truncated at any site receiving an average quality score of <20 over a 50-bp sliding window, and truncated reads shorter than 50 bp, as well as reads containing ambiguous characters, were discarded; (2) only overlapping sequences longer than 10 bp were assembled according to their overlapped sequence (maximum error ratio = 0.2); and (3) sequences of each sample were separated according to barcodes (exactly matching) and primers (allowing two nucleotide mismatches). Operational taxonomic units (OTUs) at 97% similarity were clustered using UPARSE version 7.1, with a confidence threshold of 0.70, and the taxonomy of each OTU representative sequence was analyzed using the RDP Classifier version 2.2 against the Silva 132/16S_bacteria database (Wang et al., 2007; Quast et al., 2013). Chimeric sequences were identified and removed using a novel “greedy” algorithm (Stackebrandt and Goebel, 1994; Edgar, 2013). The following analyses were performed on the Majorbio I Sanger Cloud Platform.1 Alpha diversity was assessed by MOTHUR version v.1.30.1 (Schloss et al., 2009). Bar graphs were constructed using the “vegan” package in R (Oksanen et al., 2010). Beta-diversity was estimated by computing the Bray–Curtis distance, calculated as similarities (ANOSIM) (999 permutations), and visualized through principal coordinate analysis (PCoA) by the “vegan” package in R (Oksanen et al., 2015). Significantly different bacteria at the phylum, family, and genus levels between the HRFI group and the LRFI group were identified by Student’s t-test and false discovery rate (FDR) multiple check calibration using the “stats” package in R, as well as the “scipy” package in python (Jones et al., 2001; R Core Team, 2013; Parks et al., 2014).
Metabolomic Processing
All SI samples were analyzed using the LC–MS platform (Thermo Ultimate 3000LC, Q Exactive; ThermoFisher Scientific, Waltham, MA, United States). Briefly, 50 mg samples were weighed accurately, then 400 μl of methanol/water (4:1 v/v) was used to extract the metabolites. The mixture was allowed to settle at −20°C and treated by a high throughput tissue grinder (Wonbio-96, Shanghai Wanbo Biotechnology Co., Ltd., Shanghai, China) for 6 min at 50 Hz, followed by vortexing for 30 s and ultrasound disruption at 40 kHz for 30 min at 5°C. The samples were placed at −20°C for 30 min. The samples were then centrifuged at 13,000 × g for 15 min at 4°C, and the supernatant was used for LC–MS/MS analysis. Chromatographic separation of the metabolites was carried out using an ExionLC™AD system (AB Sciex, Framingham, MA, United States) equipped with an ACQUITY UPLC HSS T3 column (100 × 2.1 mm i.d., 1.8 μm particle size; Waters, Milford, MA, United States). Mobile phases consisted of A (0.1% formic acid in water) and B (acetonitrile 50% and isopropyl alcohol 50% with 0.1% formic acid). The gradient of the mobile phase (A:B) consisted of the following: 0–3 min, 95%:5%–80%:20%; 3–9 min, 80%:20%–5%:95%; 9–13 min, 5%:95%–5%:95%; 13.0–13.1 min, 5%:95%–95%:5%; and 13.1–16.0 min, 95%:5%–95%:5% to equilibrate the system. The UPLC system was coupled to a quadrupole time-of-flight mass spectrometer (Triple TOFTM5600+, AB Sciex) equipped with an electrospray ionization (ESI) source. The optimal conditions were as follows: source temperature, 500°C; curtain gas (CUR), 30 psi; both Ion Source GS1 and GS2, 50 psi; ion-spray voltage floating (ISVF), −4,000 V in negative mode and 5,000 V in positive mode, respectively; declustering potential, 80 V; collision energy (CE), 20–60 V rolling for MS/MS. To test the repeatability of the system, quality control (QC) samples prepared by mixing equal volumes of all ruminal liquid were injected at regular intervals.
Metabolomics Data Analysis
After UPLC-TOF/MS analyses, the raw data were first imported into Progenesis QI 2.3 (Nonlinear Dynamics, Waters) for baseline filtering, peak detection, and alignment. A data matrix of retention time, mass charge ratio, and peak intensity was generated by the preprocessing results. At least 50% of the metabolic features of samples were retained. After filtering, the vacancy values were filled (the minimum value in the original matrix), and each metabolic feature was normalized by sum. After the internal standard for data QC (reproducibility) was analyzed and data were discarded if the relative SD (RSD) of QC was >30%, the statistical analysis was performed on log10 transformed data to identify significant differences in metabolite levels between the HRFI and LRFI groups. The mass spectra of these metabolic features were used to search biochemical databases such as the Human metabolome database (HMDB)2 and the Metlin database.3 All data were visualized between the HRFI and LRFI groups using principal component analysis (PCA), followed by orthogonal partial least squares discriminant analysis OPLS-DA with Student’s t-test and the following screening criteria: variable importance in the projection (VIP) values >1.0, difference multiple fold change (FC) > 1.0 or FC < 1.0 and p < 0.05 to obtain significantly differentially abundant metabolites between the LRFI and HRFI groups. Moreover, significantly differentially abundant metabolites were analyzed for abundance pattern clustering using the “gplots” package in R (Warnes et al., 2016). The impact of the RFI phenotype on metabolic pathways and metabolite set enrichment was analyzed using the “stats” package in R and the “scipy” package in python, respectively (Jones et al., 2001; R Core Team, 2013); and the significantly enriched KEGG metabolic pathway was obtained by q value (false discovery rate; FDR) <0.05. The correlations among significantly differentially abundant metabolites, predominant SI bacteria, and RFI phenotype were assessed using Spearman’s correlation analysis in the “pheatmap” package in R (Kolde, 2012).
Results
Animal Performance
As shown in Table 1, at the beginning of the experiment, the HRFI group and the LRFI group were not different in body weight (p = 0.64). However, the LRFI group had a lower DMI (p = 0.04) and a lower RFI value (p < 0.01) compared with the HRFI group.
Sequencing, Alpha Diversity, and Beta Diversity
In total, 448,612; 478,572; and 496,803 raw bacterial sequences were obtained from the duodenum, jejunum, and ileum samples, respectively. After quality control to an equal sequencing depth (286,770; 337,100; or 46,378 reads per sample of the duodenum, jejunum, ileum, respectively) and clustering, we obtained 3,542; 1,589; and 1,030 OTUs at a 97% similarity level, which were assigned to 40, 28, and 22 phyla; 91, 57, and 38 classes; 245, 120, and 83 orders; 415, 208, and 144 families; and 912, 463, and 308 genera in the duodenum, jejunum, and ileum samples, respectively. Good’s coverage after normalization for the duodenum, jejunum, and ileum samples were >99.52%, 99.68%, and 99.78% for the bacterial community, respectively, indicating good sequencing coverage for the samples. Chao1’s richness, Shannon’s diversity, and Simpson’s diversity of Alpha diversity demonstrated that the bacterial community of the duodenum, jejunum, and ileum samples did not vary between the RFI groups (p > 0.05), except for Shannon’s diversity of the ileum (p = 0.04; Supplementary Table 4). Furthermore, we performed a Bray–Curtis dissimilarity analysis of the microbiota for the HRFI and LRFI groups and visualized using PCoA plots as shown in Figure 1. We found that the duodenum and ileum microbiota show clear separation, except for jejunum bacteria, indicating that the RFI phenotype influences the composition of the duodenum and ileum, respectively.
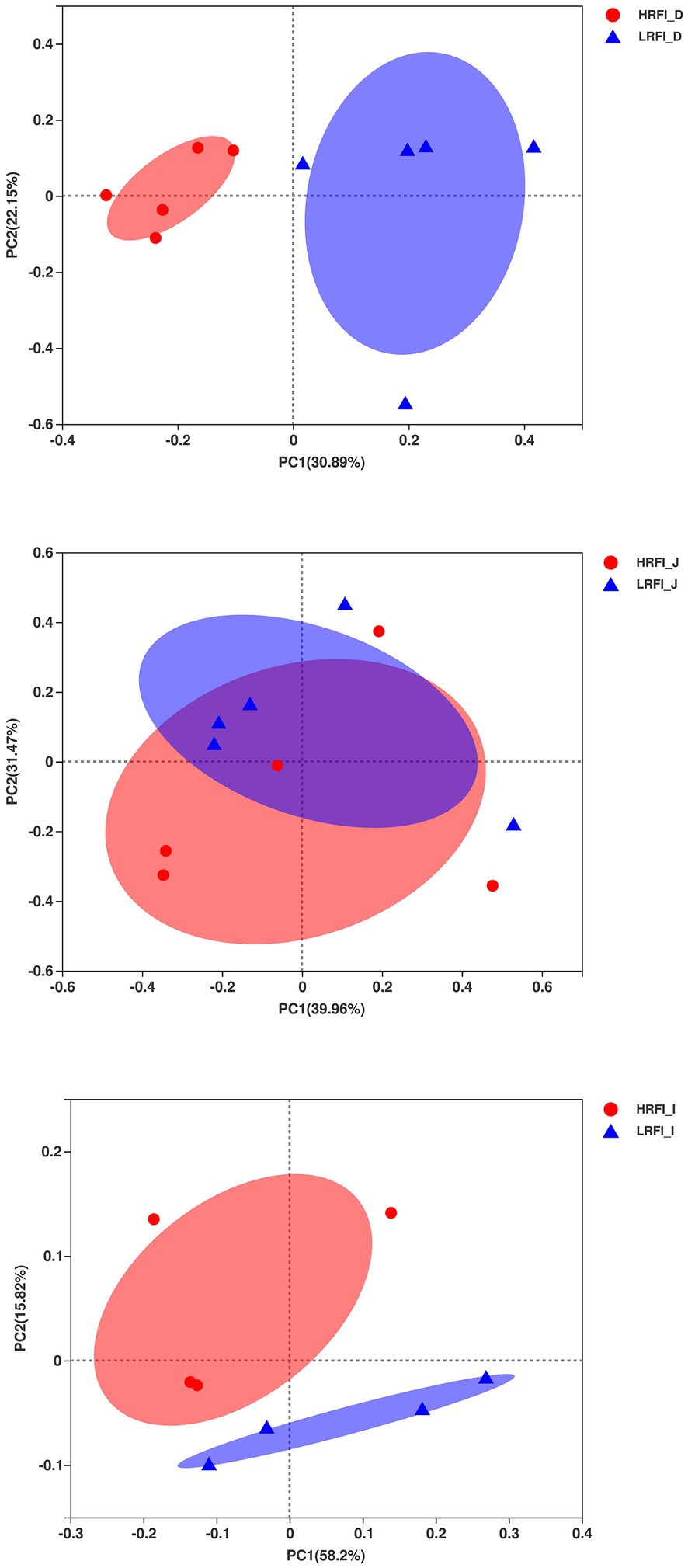
Figure 1. Principal coordinate analysis (PCoA) of the duodenum, jejunum, and ileum bacterial communities.
Bacteria Abundance
In the duodenum, the phyla with relative abundances >10% were Firmicutes (23.22%, 49.16%), Proteobacteria (41.95%, 14.84%), Actinobacteria (16.60%, 14.28%), and Bacteroidetes (10.96%, 14.20%) for the HRFI group and LRFI group, respectively. At the family level, bacterial families with relative abundance >10% were Moraxellaceae (31.68%, 9.70%), Bifidobacteriaceae (14.32,10.26%), Lachnospiraceae (5.36%, 17.73%), and Ruminococcaceae (5.33,11.96%) in the HRFI group and LRFI group, respectively. The genera of bacteria with relative abundances >5% were Acinetobacter (31.64%, 9.68%), norank_f_F082 (5.06%, 7.70%), unclassified_f_Bifidobacteriaceae (7.91%, 4.84%), Bididobacterium (6.27%, 5.19%), and Lachnospiraceae_NK3A20_group (1.57%, 6.45%) in the HRFI group and LRFI group, respectively (Figure 2).
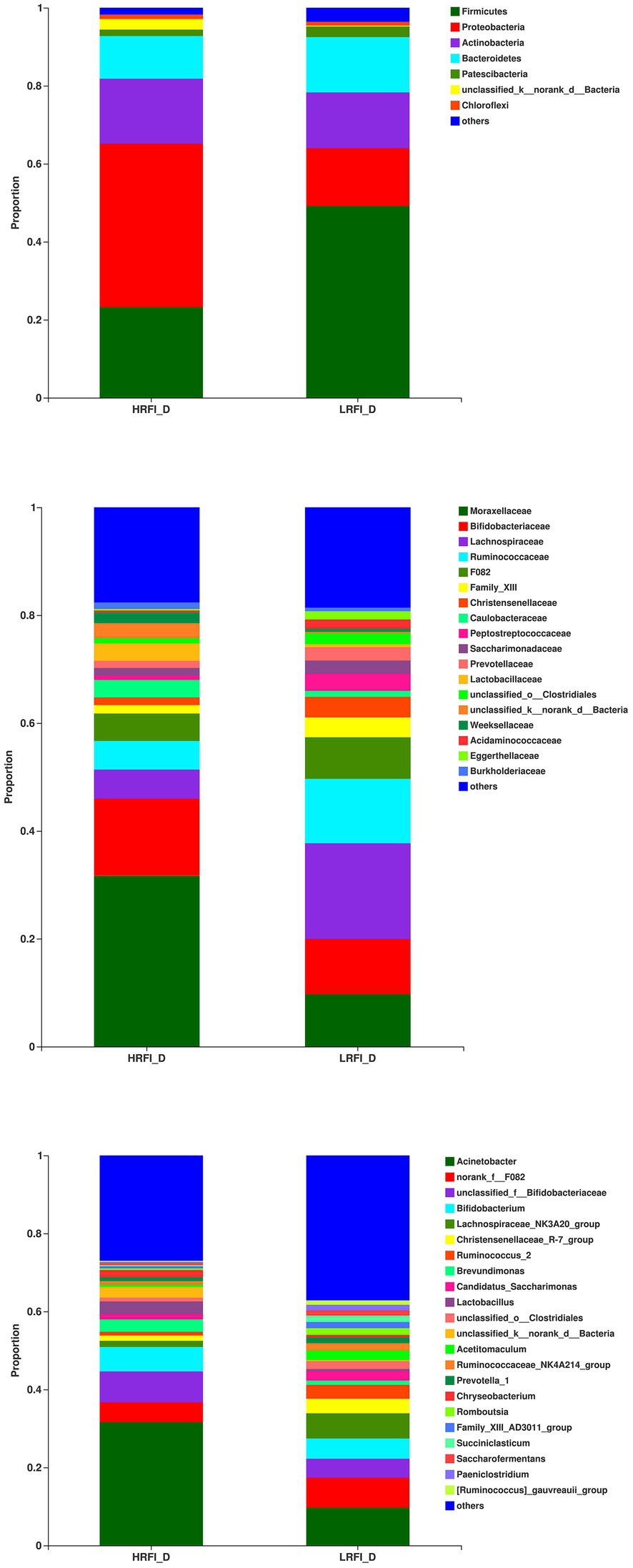
Figure 2. The average proportion of the most dominant duodenum bacteria phyla, families, and genera (relative abundance >1% for all samples).
In the jejunum, the phyla with relative abundances >10% were Firmicutes (44.49%; 58.09%), Proteobacteria (42.27%; 20.21%), and Actinobacteria (7.79%; 15.69%) for the HRFI group and LRFI group, respectively. At the family level, bacterial families with a relative abundance >10% were Pseudomonadacese (38.09%, 18.68%), Peptostreptococcaceae (19.60%, 13.40%), Lachnospiraceae (7.95%, 24.29%), and Bifidobacteriaceae (5.78%, 10.56%) in the HRFI group and LRFI group, respectively. The genera of bacteria with relative abundances >5% were Pseudomonadacese (38.09%, 18.68%), Paeniclostridium (11.05%, 8.42%), Bifidobacterium (5.15%, 10.46%), Lachnospiraceae_NK3A20_group (2.29%, 11.27%), Romboutsia (8.54%, 4.98%), and Christensenellaceae_R-7_group (2.74%, 5.05%) in the HRFI group and LRFI group, respectively (Figure 3).
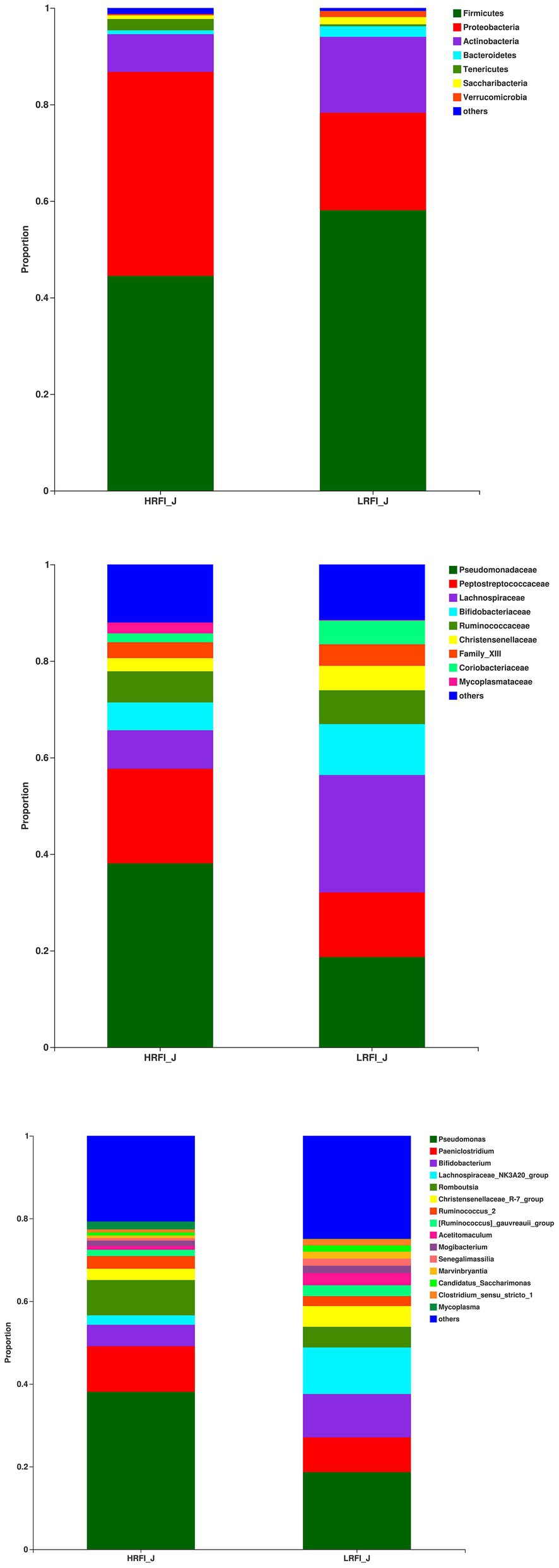
Figure 3. The average proportion of the most dominant jejunum bacteria phyla, families, and genera (relative abundance >1% for all samples).
In the ileum, the phyla with relative abundances >10% were Firmicutes (91.41%; 90.31%), and Actinobacteria (3.93%, 4.06%) for the HRFI group and LRFI group, respectively. At the family level, bacterial families with a relative abundance >10% were Peptostreptococcaceae (60.71%, 44.91%), Lachnospiraceae (8.06%, 13.82%), Clostridiaceae_1 (10.39%, 8.12%) in the HRFI group and LRFI group, respectively. The genera of bacteria with relative abundances >5% were Paeniclostridium (41.12%, 28.72%), Romboutsia (19.52%, 16.12%), Clostridium_sensu_stricto_1 (10.33%, 8.09%), Turicibacter (4.78%, 6.48%), Lachnospiraceae_NK3A20_group (2.27%, 5.43%) in the HRFI group and LRFI group, respectively (Figure 4).
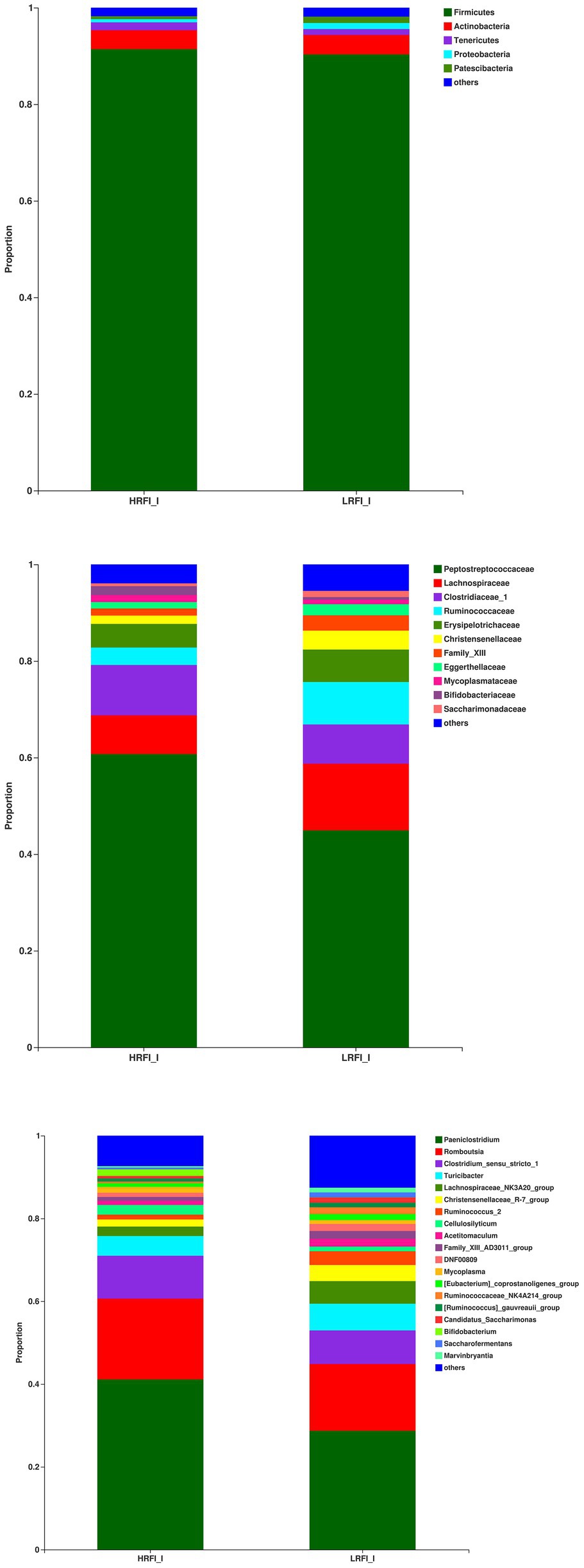
Figure 4. The average proportion of the most dominant ileum bacteria phyla, families, and genera (relative abundance >1% for all samples).
Significantly Differentially Abundant SI Bacteria
As shown in Figure 5, in the duodenum, at the phylum level, the relative abundances of Firmicutes (23.22%, 49.16%) and Proteobacteria (41.95%, 14.84%) was higher in the LRFI group and HRFI group, respectively (p < 0.01). At the family level, the relative abundances of Lachnospiraceae (5.36%, 17.73%), Ruminococcaceae (5.33%, 11.96%), Family_XIII (1.60%, 3.70%), and Christensenellaceae (1.39%, 3.80%) were higher in the LRFI group (p < 0.01). At the genus level, the relative abundances of Lachnospiraceae_NK3A20_group (1.57%, 6.45%; p < 0.05), and Christensenellaceae_R-7_group (1.37%, 3.77%; p < 0.01) were higher in the LRFI group, whereas the HRFI group had a higher abundance of Acinetobacter (32.12%, 11.19%; p < 0.01). As shown in Figure 6, in the jejunum, the relative abundances of family Lachnospiraceae (7.95%, 24.29%) and genus Lachnospiraceae_NK3A20_group (2.29%, 11.27%) were higher in the LRFI group (p < 0.05). In the ileum, the relative abundances of families Ruminococcaceae (3.62%, 8.81%; p < 0.01) and Christensenellaceae (1.74%, 3.93%; p < 0.05) were higher in the LRFI group; the relative abundance of genus Christensenellaceae_R-7_group (1.73%, 3.91%) and Ruminococcus_2 (1.16%, 3.31%) were higher (p < 0.05) in the LRFI group (Figure 7).
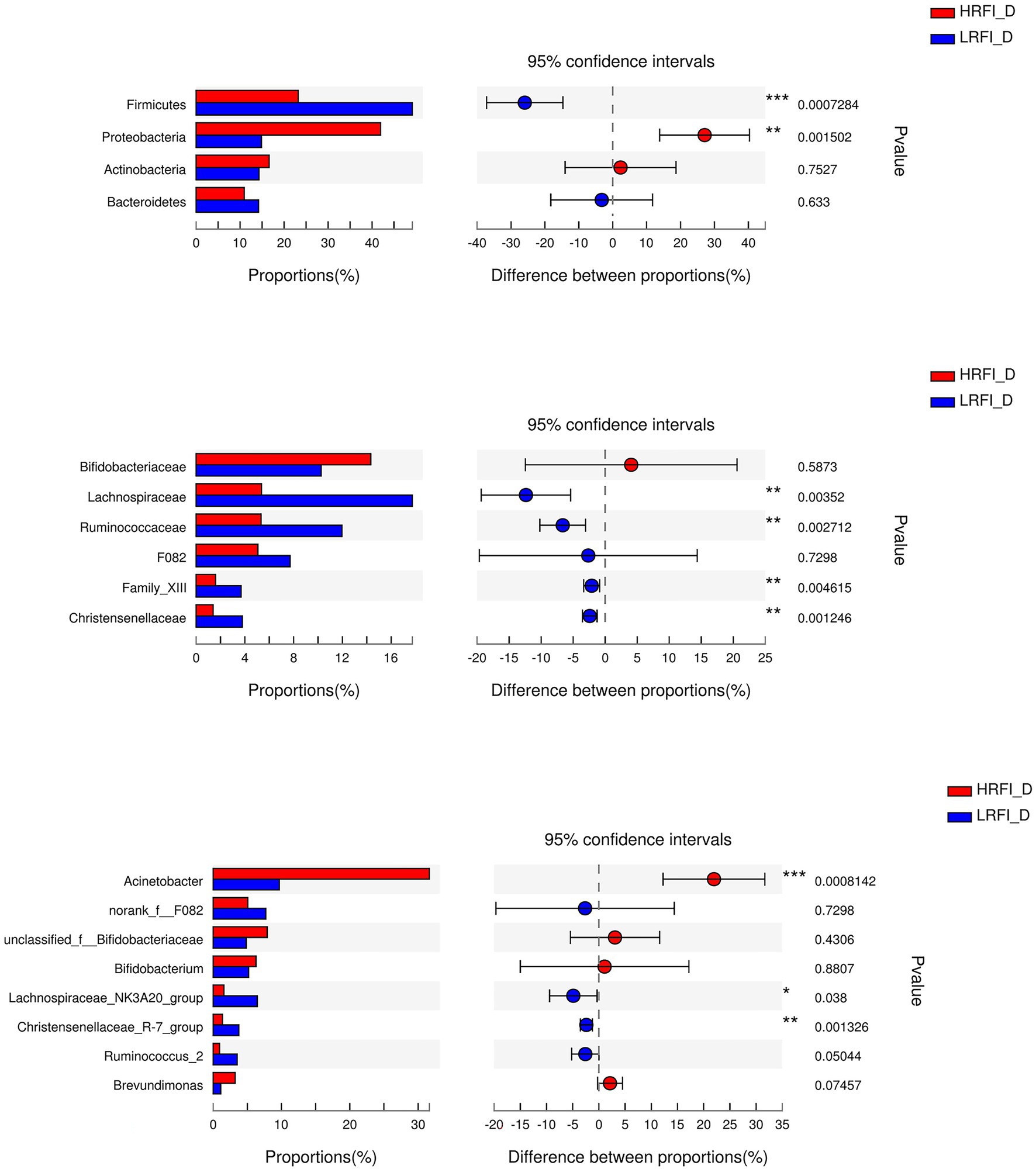
Figure 5. Significantly differential abundant phyla, families, and genera (relative abundance >1%) with the duodenum bacteria. Positive and negative differences indicate a greater abundance in the HRFI group and LRFI group, respectively. *Represents 0.01 < p < 0.05; ** represents 0.001 < p < 0.01; and *** represents p < 0.001.
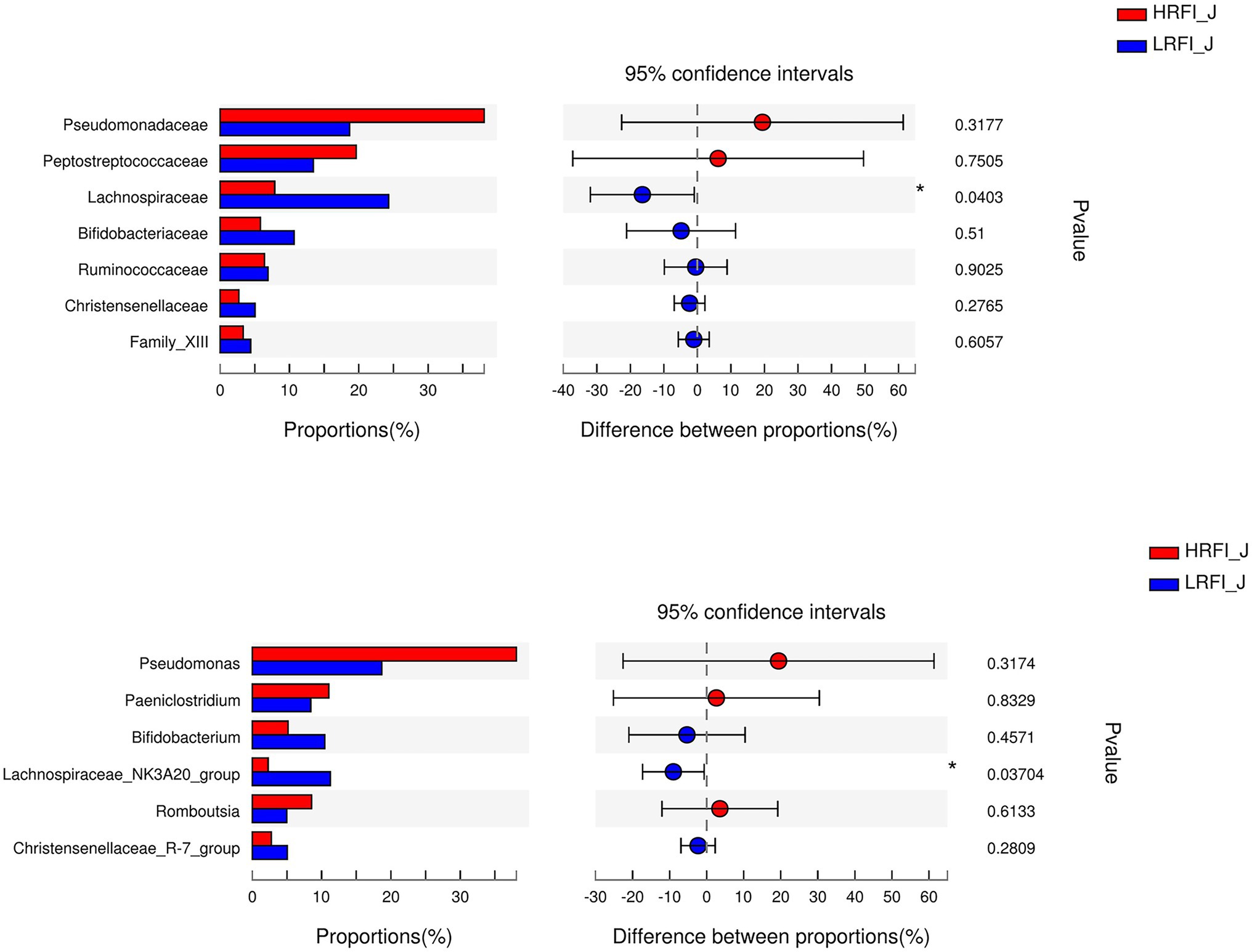
Figure 6. Significantly differential abundant families, and genera (relative abundance >1%) with the jejunum bacteria. Positive and negative differences indicate a greater abundance in the HRFI group and LRFI group, respectively. *Represents 0.01 < p < 0.05.
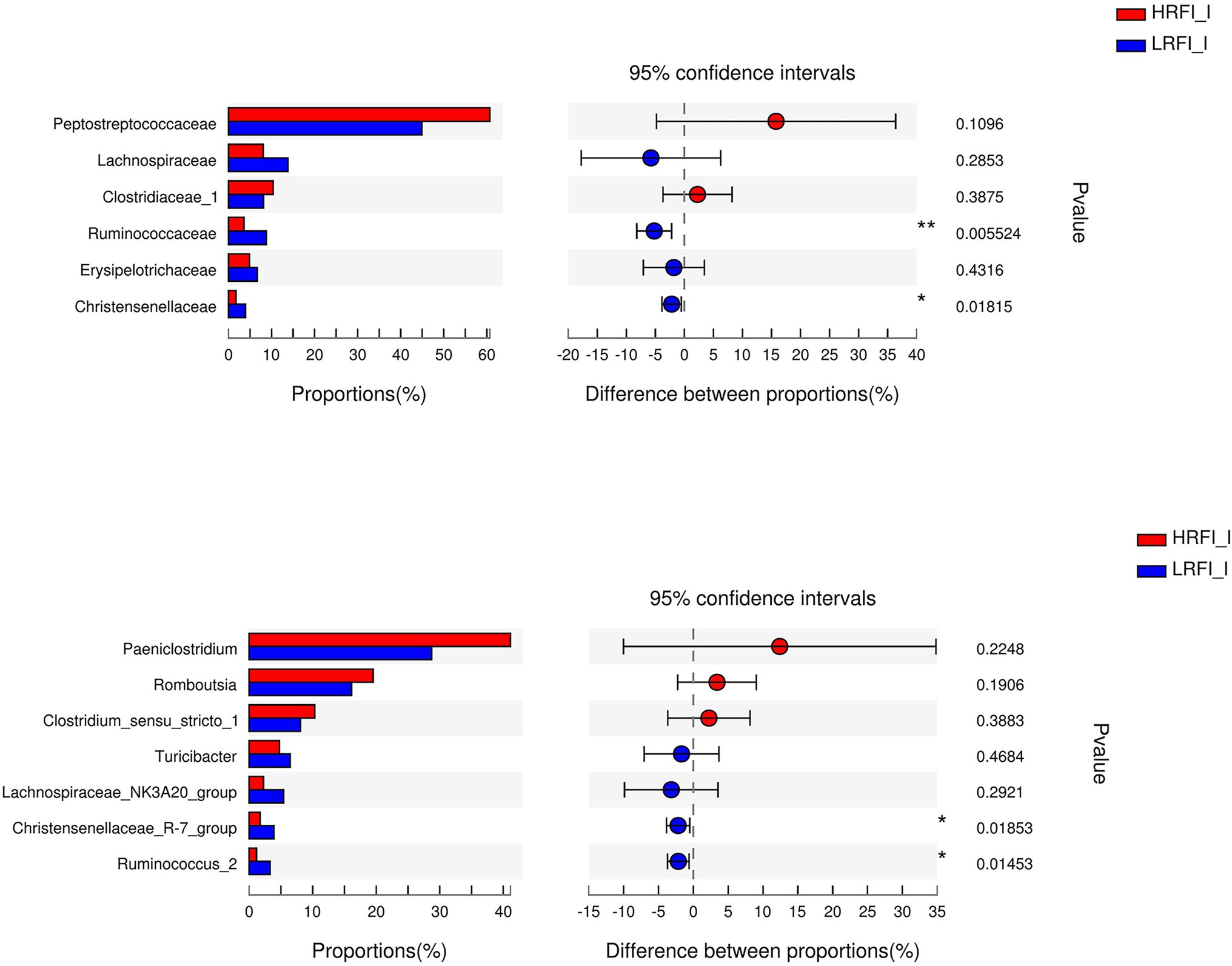
Figure 7. Significantly differential abundant families, and genera (relative abundance >1%) with the ileum bacteria. Positive and negative differences indicate a greater abundance in the HRFI group and LRFI group, respectively. *Represents 0.01 < p < 0.05 and ** represents 0.001 < p < 0.01.
Metabolomic Profiling
Sample Quality Control
The overlap of the total ion chromatogram of the QC samples in the positive (A) and negative (B) ion modes is shown in Supplementary Figure 1, which confirmed the stability and reproducibility of the data. Figure 8 shows the OPLS-DA score plot of duodenum, jejunum, ileum between the HRFI group and LRFI group, all the samples were within the 95% Hotelling T2 ellipse, and the permutation test with a better range of R2-values from 0.920 to 0.973, indicating moderate effectiveness of the model.
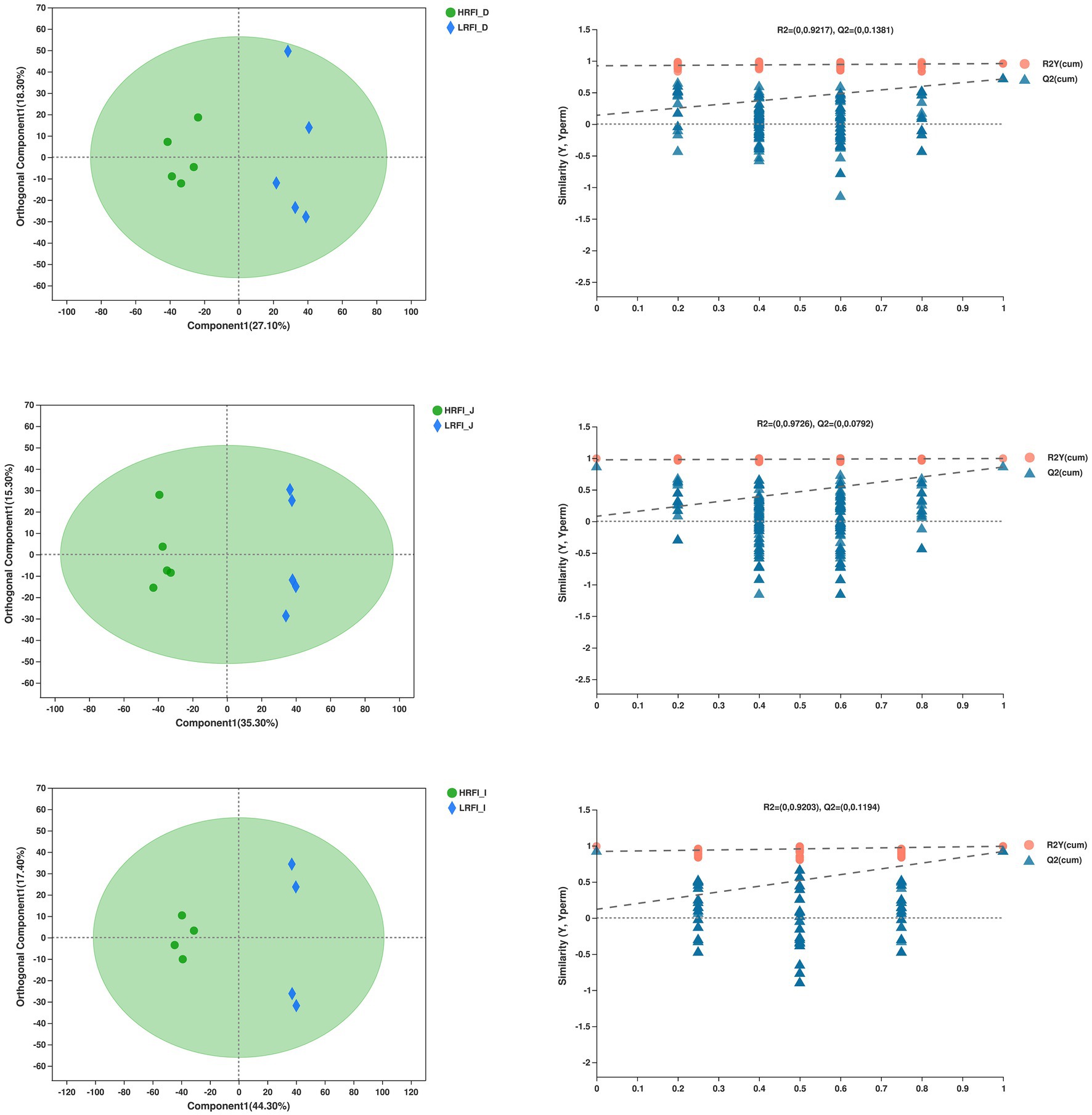
Figure 8. Orthogonal partial least squares discriminant analysis (OPLS-DA) plot of the duodenum, jejunum, ileum bacterial metabolites.
Significantly Differentially Metabolite Analysis
The PCA provided a satisfactory separation of the data between the groups (Supplementary Figure 2). As shown in Table 2, there are 6, 10, and 16 differential metabolites between the LRFI group and HRFI group in the duodenum, jejunum, and ileum, respectively. Moreover, we found that the LRFI group significantly improved protein digestion and absorption, as well as glycerophospholipid metabolism in the duodenum, jejunum, and ileum.
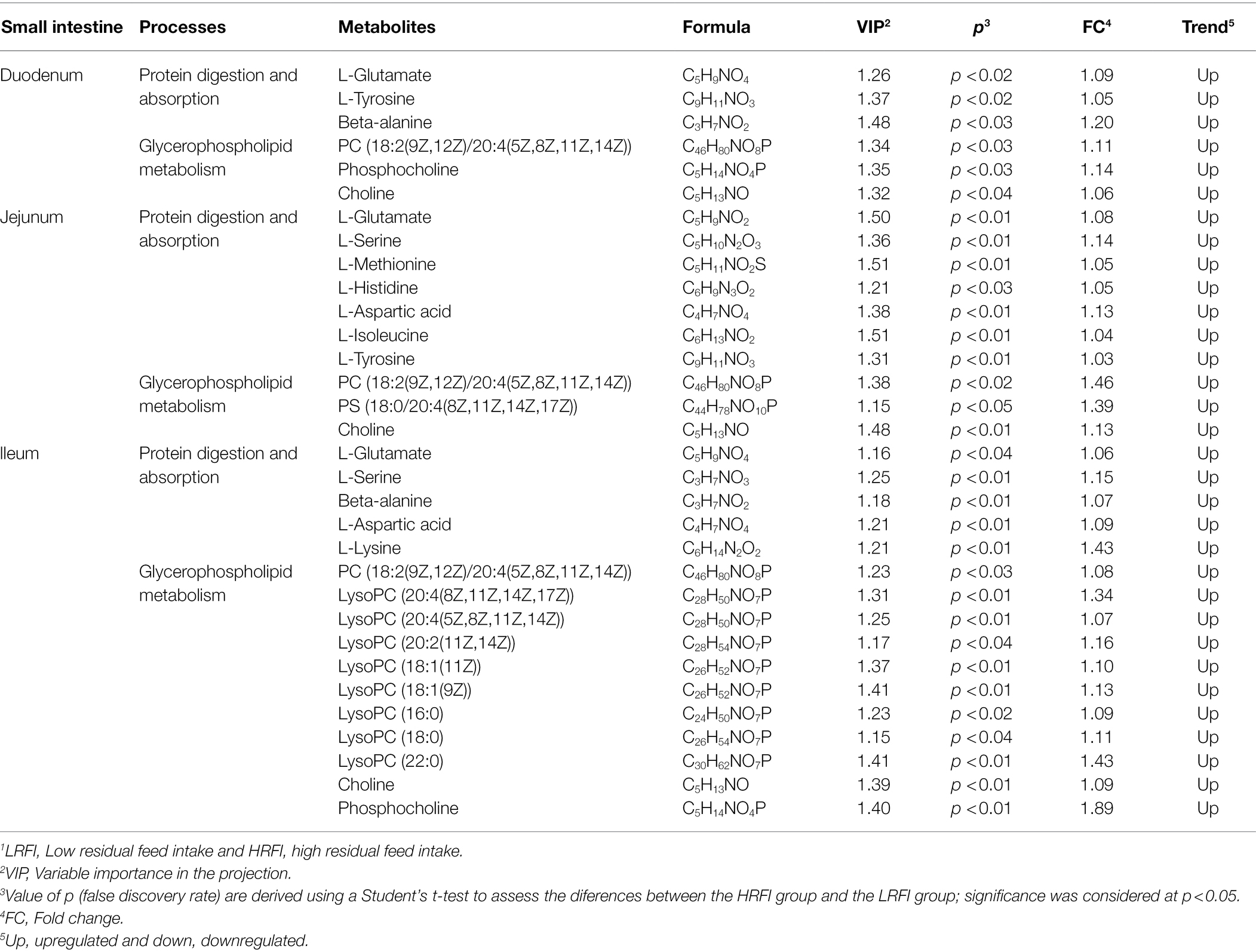
Table 2. Significantly differentially metabolites in small intestine by comparison of the LRFI and HRFI groups.1
Correlation Analysis Among the Predominant Genera Bacteria, Significantly Differentially Metabolites, and the RFI Phenotype
As shown in Figure 9, in the duodenum, the genus Acinetobacter was positively associated with RFI (r = 0.81, p < 0.01), while negatively associated with PC [18:2(9Z,12Z)/20:4(5Z,8Z,11Z,14Z)] (r = −0.70, p < 0.05), L-Glutamate (r = −0.77, p < 0.01), beta-Alanine (r = −0.73, p < 0.05), and L-Tyrosine (r = −0.70, p < 0.05). The genus Lachnospiraceae_NK3A20_group was negatively associated with RFI (r = −0.72, p < 0.05), while positively associated with phosphocholine (r = 0.73, p < 0.05), and beta-Alanine (r = 0.71, p < 0.05). The genus Christensenellaceae_R-7_group was negatively associated with RFI (r = −0.79, p < 0.01), while positively associated with phosphocholine (r = 0.82, p < 0.01), PC [18:2(9Z,12Z)/20:4(5Z,8Z,11Z,14Z)] (r = 0.77, p < 0.01), L-Glutamate (r = 0.72, p < 0.05), beta-Alanine (r = 0.75, p < 0.05), and L-Tyrosine (r = 0.76, p < 0.05). The genus Ruminococcus_2 was negatively associated with RFI (r = −0.69, p < 0.05), while positively associated with phosphocholine (r = 0.71, p < 0.05), and beta-Alanine (r = 0.67, p < 0.05). In the jejunum, the genus Lachnospiraceae_NK3A20_group was negatively associated with RFI (r = −0.92, p < 0.001), while positively associated with L-Serine (r = 0.88, p < 0.001), PS [18:0/20:4(8Z,11Z,14Z,17Z)] (r = 0.86, p < 0.01), L-Glutamate (r = 0.87, p < 0.01), and L-Aspartic Acid (r = 0.92, p < 0.001), PC [18:2(9Z,12Z)/20:4(5Z,8Z,11Z,14Z)] (r = 0.83, p < 0.05), L-Methionine (r = 0.75, p < 0.05), and L-Isoleucine (r = 0.76, p < 0.05). The genus Christensenellaceae_R-7_group was negatively associated with RFI (r = −0.66, p < 0.01). The genus Ruminococcus_2 was positively associated with L-Serine (r = 0.72, p < 0.05), PS [18:0/20:4(8Z,11Z,14Z,17Z)] (r = 0.78, p < 0.01), L-Glutamate (r = 0.64, p < 0.05), and L-Aspartic Acid (r = 0.69, p < 0.05). In the ileum, the genus Paeniclostridium was negatively associated with LysoPC [20:2(11Z,14Z)] (r = −0.79, p < 0.05), PC [18:2(9Z,12Z)/20:4(5Z,8Z,11Z,14Z)] (r = −0.79, p < 0.05), phosphocholine (r = −0.86, p < 0.01), beta-Alanine (r = −0.81, p < 0.05), L-Aspartic Acid (r = −0.74, p < 0.05), L-Glutamate (r = −0.86, p < 0.01), and L-Serine (r = −0.76, p < 0.05). The genus Lachnospiraceae_NK3A20_group was positively associated with L-Glutamate (r = 0.76, p < 0.05). The genus Christensenellaceae_R-7_group was positively associated with LysoPC [18:1(11Z)] (r = 0.71, p < 0.05), LysoPC [20:4(8Z,11Z,14Z,17Z)] (r = 0.79, p < 0.05), LysoPC [20:4(5Z,8Z,11Z,14Z)] (r = 0.79, p < 0.05), LysoPC [18:1(9Z)] (r = 0.91, p < 0.01), LysoPC [20:2(11Z,14Z)] (r = 0.81, p < 0.05), PC [18:2(9Z,12Z)/20:4(5Z,8Z,11Z,14Z)] (r = 0.95, p < 0.001), phosphocholine (r = 0.91, p < 0.01), beta-Alanine (r = 0.91, p < 0.01), L-Aspartic Acid (r = 0.86, p < 0.01), L-Glutamate (r = 0.98, p < 0.001), and L-Serine (r = 0.98, p < 0.001). The genus Ruminococcus_2 was negatively associated with RFI (r = −0.74, p < 0.05), while positively associated with choline (r = 0.79, p < 0.05), LysoPC [18:1(11Z)] (r = 0.76, p < 0.05), LysoPC (16:0) (r = 0.74, p < 0.05), LysoPC [20:4(8Z,11Z,14Z,17Z)] (r = 0.74, p < 0.05), LysoPC [20:4(5Z,8Z,11Z,14Z)] (r = 074, p < 0.05), LysoPC [18:1(9Z)] (r = 0.81, p < 0.05), and beta-Alanine (r = 0.91, p < 0.01).
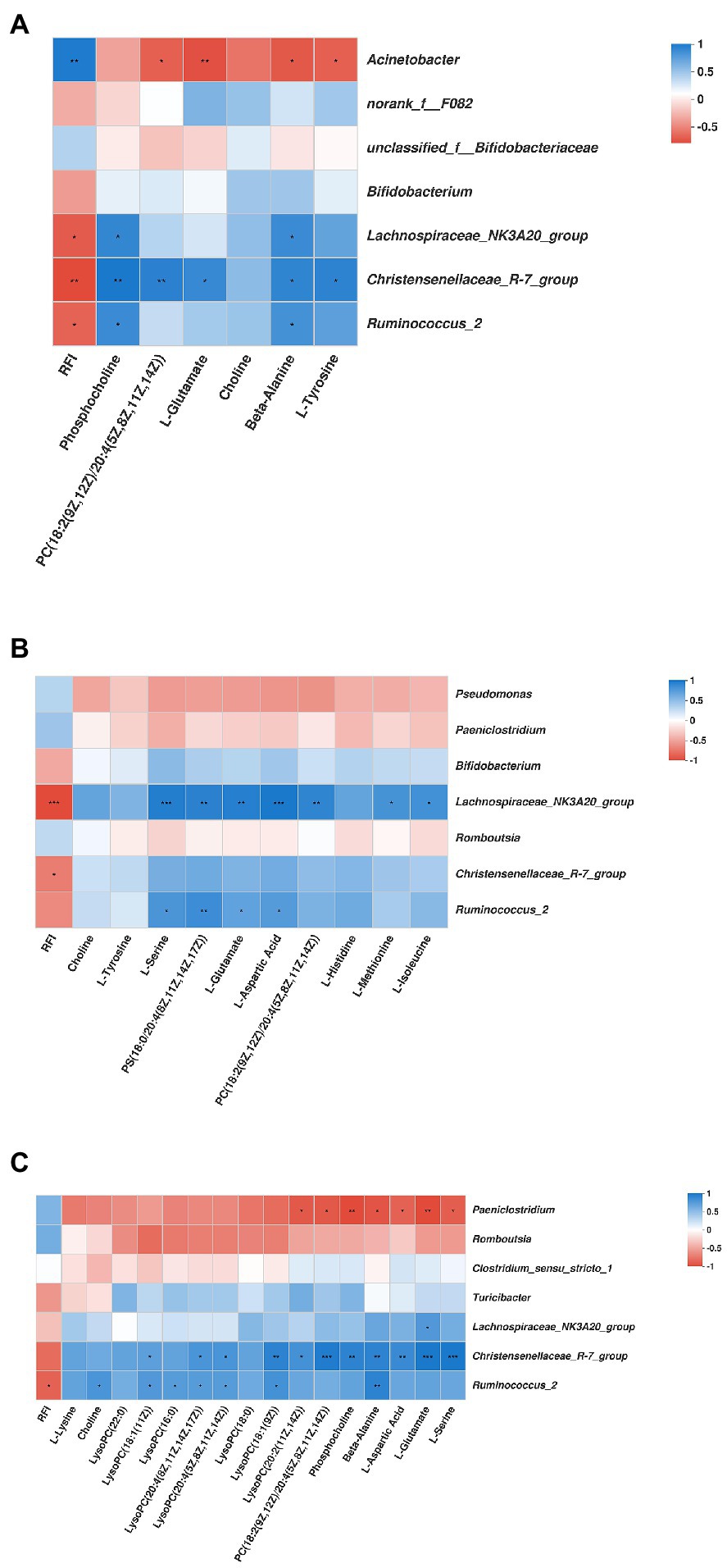
Figure 9. Correlation analysis among the predominant genera duodenum (A), jejunum (B), ileum (C) microbiota bacteria, the significantly differential metabolites, and the RFI. Cells are colored based on Spearman’s correlation coefficient: blue represents a positive correlation; red represents a negative correlation. *Represents 0.01 < p ≤ 0.05; ** represents 0.001 < p ≤ 0.01; and *** represents p ≤ 0.001.
Discussion
Animal Performance
As expected, the LRFI heifers consumed less feed during this experiment, consistent with the result on steers (Welch et al., 2020). Improving feed efficiency by decreasing feed cost means the greatest profitability of the beef cattle production system (Lancaster et al., 2009). Therefore, we should select LRFI cattle to consume less feed without affecting ADG, resulting in maximized profitability for the beef cattle industry (Herd et al., 2003).
Bacterial Diversity
The diverse microorganisms that colonize the digestive tract of beef cattle play a vital role in the host’s digestion and absorption of nutrients, promotion of immunity, regulation of behavior, and maintenance of intestinal homeostasis. In the present study, we found that the diversity of the bacterial communities in the ileum was significantly higher in the LRFI group than in the HRFI group. This might be explained by previous observations that higher intestinal microbial diversity and richness result in a more stable and functionally complete intestinal ecosystem, which means that animals have better adaptability and higher productivity (Firkins and Yu, 2015; Huws et al., 2018). However, we found no differences in diversity and richness of duodenum and jejunum with different RFI phenotypes, previous studies were similar to our result (Li and Guan, 2017; Perea et al., 2017; Paz et al., 2018; Freetly et al., 2020; Lopes et al., 2021). This implies that efficiency status does not depend on a large-scale restructuring of the entire microbial community, but might be dependent on differences in a few key taxa (Perea et al., 2017). Additionally, some studies also observed no differences in rumen microbial diversity of cattle with different RFI phenotypes (Paz et al., 2018; Clemmons et al., 2019; Lopes et al., 2021). These results might indicate that the diversity of the microbial community is not necessarily related to the RFI phenotype of animals. Because of current conflicting results, in the future, more research is needed to confirm these results. Moreover, the structure of the GIT bacterial community has been proven to be host-specific, which might lead to large differences in the diversity and richness of the GIT bacterial community (Huttenhower et al., 2012; Donaldson et al., 2016).
Significantly Differentially Bacteria
In this study, we found that the SI bacterial community structures in duodenum, jejunum, and ileum of heiferes with different RFI phenotypes were significantly different. Additionally, the duodenum, jejunum, and ileum of heifers with different RFI phenotypes might have a similar “core” bacterial microbiota community structure, as well as some specific microbiota (Myer et al., 2016a; Perea et al., 2017; Freetly et al., 2020). Differences were observed for some SI taxa, specifically, the Firmicutes and Proteobacteria phyla were consistent with a previous study of beef cattle (Freetly et al., 2020) and lamb (Perea et al., 2017), respectively. In the present study, Lachnospiraceae was found in greater abundance in the duodenum, and jejunum of the most efficient heifers; as well as Ruminococcaceae in the duodenum, and ileum. Coincident with our results, Shabat et al. (2016) reported that dairy cows with an LRFI phenotype also had greater levels of Lachnospiraceae. Moreover, Gagen et al. (2015) observed that acetogens could be found in both the families Lachnospiraceae and Ruminococcaceae, which might be explained by the presence of more families Lachnospiraceae and Ruminococcaceae in the LRFI group: it is likely that elevated levels of Lachnospiraceae and Ruminococcaceae indicate a more complete fermentation and increased absorbable nutrients available to LRFI animals (Freetly et al., 2020). Additionally, Christensenellaceae has been identified as an indicator of a “healthy digestive system” (Goodrich et al., 2014), and is a known butyrate producer (Morotomi et al., 2012), which verified our observation that higher relative abundance of the family Christensenellaceae in the duodenum and ileum of the LRFI group. Members of the Lachnospiraceae_NK3A20_group were abundant in the SI, being present in the rumen, cecum, and fecal samples (Lopes et al., 2019), and Ruminococcus is found commonly within microbial communities across the GIT (Oliveira et al., 2013; Myer et al., 2016b); however, fewer studies about Family_XIII, Acinetobacter, Lachnospiraceae_NK3A20_group, and Ruminococcus_2, in the future, more studies need to be investigated.
Significantly Differentially Metabolites
Our metabolome data revealed that the RFI significantly altered the concentrations of SI metabolites associated with protein digestion and absorption, glycerophospholipid metabolism. Additionally, correlation analysis showed that there was a certain correlation between SI microorganisms and SI differential metabolites, there is a relationship between the SI microorganisms and the SI differential metabolites. In the gut, amino acids are the degradation products of rumen-protected proteins and microbial proteins, which regulate certain metabolic pathways (Mariz et al., 2018). Notably, we observed increased levels of L-Methionine, L-Lysine, L-Isoleucine, L-Histidine, L-Glutamate, L-Aspartic Acid, Beta-Alanine, L-Serine, and L-Tyrosine in the LRFI group. Greenwood and Titgemeyer (2000) reported that for steers, corn-soybean meal or other feedstuff based diets, the first or second limiting amino acids are basically Lysine and Methionine. Moreover, Isoleucine (Swanepoel et al., 2010a,b) and Histidine (Vanhatalo et al., 1999; Greenwood and Titgemeyer, 2000) might be the third limiting amino acids, but the determination of the third limiting amino acids remains to be further studied. Archibeque et al. (2002) also confirmed methionine as a typically limiting amino acid (AA) for beef steers. Methionine plays important role in protein synthesis, DNA methylation, lipid metabolism, and antioxidant regulation (Löest et al., 2002; Zhou et al., 2016; Martinez et al., 2017). As methyl donors, rumen protected choline has been reported to improve growth performance of cattle (Bindel et al., 2000). Han et al. (2017) also observed the supplementation of methionine improved growing Holstein steers’ performance. Hussein and Berger (1995) also showed that feeding rumen-protected methionine and rumen-protected Lysine to Holstein steers improved average daily gain. Additionally, it has been reported that Lysine deficiency significantly increases anxiety in rats when stimulated by electric shock (Smriga et al., 2000). Histidine is a semi-essential amino acid in animals, and its supply has an obvious linear relationship with protein turnover of the body (Wantanee et al., 2002). Studies have observed that long-term intake of low histidine diets significantly reduces animal body weight and feed intake, reduced activity, lethargy, and even death (Cianciaruso et al., 1981). Studies have shown that histidine could maintain the stability of cell pH, maintain cell homeostasis, reducing stress (Rao et al., 2010) and protecting the heart (Obata et al., 1999). Additionally, histidine has anti-inflammatory and antioxidant effects (Feng et al., 2013). Dong et al. (2005) reported that histidine can inhibit the inflammatory response caused by oxidative stress in human intestinal epithelial cells and improve intestinal health. Meanwhile, histidine contains imidazolidyl, which can combine with Metal ions such as Cu2+ and Zn2+ to promote intestinal absorption of Cu2+ and Zn2+. Metal ions can participate in the composition of various enzymes (SOD etc.) in vivo. Sun et al. (2014) showed that histidine can significantly improve the antioxidant capacity of SOD in mice plasma, reduce MDA content and up-regulate the mRNA expression of CuZnSOD. L-isoleucine is a bioactive molecule involved in nutrient metabolism (Bampidis et al., 2020). Glutamate is the main oxidizing energy supply substance in the intestinal tract (Burrin et al., 2008), and it is important in intestinal antioxidant stress (Duan et al., 2014; Yin et al., 2015). Studies have found that serine is located at key nodes of multiple biological metabolic processes in the body, and plays an indispensable role in promoting cell proliferation (Newman and Maddocks, 2017), antioxidant (He et al., 2020) and immune function (Kitamoto et al., 2020). It is important to maintain animal health and normal physiological functions (Kalhan and Hanson, 2012). Additionally, carnosine, which is composed of histidine and alanine, is an endogenous functional substance and plays an important role in the body’s antioxidant function (Guiotto et al., 2005). Tyrosine is the precursor of melanin synthesis. Under the catalytic action of tyrosinase, tyrosine in animals can synthesize melanin through a series of complex biological processes. Melanin has the function of scavenging free radicals and anti-oxidation (Ye et al., 2014). Wang et al. (2018) reported that increasing the content of tyrosine in feed would lead to the increase of melanin content in the belly and back skin of tilapia. Therefore, the higher feed efficiency of LRFI group may be related to the antioxidant capacity and immune function of the upregulation of L-Methionine, L-Lysine, L-Histidine, L-Isoleucine, L-Glutamate, L-Aspartic Acid, Beta-Alanine, L-Serine, and L-Tyrosine.
It is noteworthy that we also observed alterations in the concentrations of several metabolites associated with glycerophospholipid metabolism. Choline mainly exists as lysophosphatidylcholine (LysoPCs) and phosphocholine in the body, which is important in maintaining the integrity of the cell membrane, and methyl metabolism (Blusztajn, 1998). In ruminants, it has been suggested that rumen-protected choline inhibits fat synthesis in the liver of dairy cattle during the transition period by increasing fatty acid transport and reducing the synthesis of very-low-density lipoprotein (Goselink et al., 2013). Brautigan et al. (2017) found that LysoPCs affect nutrient absorption in the intestinal tract by regulating gene expression of small intestinal epithelial cells, thus having a positive impact on the production performance of livestock. Malanka et al. (2012) also reported that LysoPCs play a regulator of immunological cell functions. Additionally, the function of phosphatidylserine in signal transduction and intercellular information transmission has been demonstrated (Nishizuka, 1984). Therefore, the upregulation of choline, phosphocholine, LysoPCs, PC [18:2(9Z,12Z)/20:4(5Z,8Z,11Z,14Z)], and PS[18:0/20:4(8Z,11Z,14Z,17Z)] might suggest increased maintain integrity of cell membrane, fatty acid transport, nutrient absorption, immune function, and signal transduction in the LRFI group to improve feed efficiency.
Correlation Among the Predominant Bacteria, Significantly Differentially Metabolites, and the RFI Phenotype
Small intestine is the main place to nutrient digestion and absorption, the nutrients such as carbohydrate, protein, lipid after into the intestinal microbes and various enzymes under the effect of extracellular digestion, digestion products by the various transport carrier absorption of intestinal epithelial cells into the blood, and shipped to all parts of the body. Moreover, Arumugam et al. (2011) reported that some microorganisms in the intestinal tract are rich in amylase, protease and other genes to decompose carbohydrates and proteins. Marie et al. (2014) reported that the genus Acinetobacter plays role in opportunistic human infections, studies also have shown that glutamate, alanine, and tyrosine are play important roles in the body’s antioxidant function (Guiotto et al., 2005; Duan et al., 2014; Ye et al., 2014; Yin et al., 2015; Wang et al., 2018), which might explain why Acinetobacter was associated positively with RFI, and negatively associated with L-Glutamate, beta-Alanine, L-Tyrosine, and PC [18:2(9Z,12Z)/20:4(5Z,8Z,11Z,14Z)] in this study. In this study, the genus Christensenellaceae_R-7_group, Lachnospiraceae_NK3A20_group, and Ruminococcus_2 were all associated negatively with RFI. Moreover, the genus Christensenellaceae_R-7_group was positively associated with L-Glutamate, beta-Alanine, L-Tyrosine, L-Aspartic Acid, L-Serine, phosphocholine, LysoPC [18:1(9Z)], LysoPC [18:1(11Z)], LysoPC [20:4(8Z,11Z,14Z,17Z)], LysoPC [20:4(5Z,8Z,11Z,14Z)], LysoPC [20:2(11Z,14Z)], PC [18:2(9Z,12Z)/20:4(5Z,8Z,11Z,14Z)]. The genus Lachnospiraceae_NK3A20_group was positively associated with L-Glutamate, L-Methionine, L-Isoleucine, L-Serine, L-Aspartic Acid, PC [18:2(9Z,12Z)/20:4(5Z,8Z,11Z,14Z)], PS [18:0/20:4(8Z,11Z,14Z,17Z)]. The genus Ruminococcus_2 was positively associated with L-Glutamate, L-Aspartic Acid, L-Serine, beta-Alanine, choline, phosphocholine, PS [18:0/20:4(8Z,11Z,14Z,17Z)], LysoPC [18:1(9Z)], LysoPC [18:1(11Z)], LysoPC (16:0), LysoPC [20:4(8Z,11Z,14Z,17Z)], and LysoPC [20:4(5Z,8Z,11Z,14Z)]. Additionally, Brautigan et al. (2017) and Malanka et al. (2012) reported that LysoPCs affect nutrient absorption in the intestinal tract, and it also plays a regulator of immunological cell functions. Therefore, Christensenellaceae_R-7_group, Lachnospiraceae_NK3A20_group, and Ruminococcus_2 might have a role in the metabolisms of amino acids metabolism and glycerophospholipid metabolism; and these bacterias increased antioxidant capacity and promoted nutrient digestion and absorption, which likely provides evidence supporting the higher feed efficiency in the LRFI group.
Conclusion
In summary, the RFI phenotype significantly altered the relative abundances of certain intestinal bacteria communities, the genera Christensenellaceae_R-7_group, Lachnospiraceae_NK3A20_group, and Ruminococcus_2 were negatively associated with RFI, while the genus Acinetobacter was positively associated with RFI. The RFI phenotype also significantly altered the concentrations of some intestinal metabolites, such as amino acids and glycerophospholipids. Additionally, the correlation between intestinal microorganisms and metabolites revealed that some microorganisms play an important role in amino acid metabolism, glycerophospholipid metabolism, nutrient digestion and absorption, and antioxidant enhancement, which likely provides evidence supporting the higher feed efficiency in the LRFI group. The predominant bacterial communities and the significantly differential metabolites in different SI segments were both common and unique, which suggested that microorganisms in different GIT locations might contribute separately to the RFI phenotype of beef cattle. Future, due to the individual variation of animals, samples from more animals should be analyzed to confirm these findings. Integrative information about the interactions between the SI microbial composition and metabolites in beef cattle with different RFIs could provide a better understanding of the small intestinal microbial and metabolites functions, allowing the development of improved strategies to increase feed efficiency. In addition, the mechanisms of the interactions among SI bacteria, metabolisms, and the RFI deserve further investigation.
Data Availability Statement
The original contributions presented in the study are publicly available. This data can be found at: https://www.ncbi.nlm.nih.gov/, PRJNA796465.
Ethics Statement
The animal study was reviewed and approved by the Laboratory Animal Welfare and Animal Experiment Ethical Committee of China Agricultural University (Protocol No. AW08059102-2).
Author Contributions
QM, ZZ, and HW designed the research. YL and CL conducted the research. YL and CL analyzed the data. YL wrote the manuscript. QM and HW modified the manuscript. ZZ had responsibility for the final content. All authors read and approved the final manuscript.
Funding
This work was supported by grants from the National Natural Science Foundation of China (grant number: 31972593), the Government Purchase Service (grant number: 16200158), and the China Agricultural Research System (grant number: CARS-37).
Conflict of Interest
The authors declare that the research was conducted in the absence of any commercial or financial relationships that could be construed as a potential conflict of interest.
Publisher’s Note
All claims expressed in this article are solely those of the authors and do not necessarily represent those of their affiliated organizations, or those of the publisher, the editors and the reviewers. Any product that may be evaluated in this article, or claim that may be made by its manufacturer, is not guaranteed or endorsed by the publisher.
Acknowledgments
We are grateful to the staff of the Beef Cattle Research Center for their help with the sampling and laboratory analyses.
Supplementary Material
The Supplementary Material for this article can be found online at: https://www.frontiersin.org/articles/10.3389/fmicb.2022.862151/full#supplementary-material
Abbreviations
SI, Small intestine; HRFI, High residual feed intake; LRFI, Low residual feed intake; DMI, Dry matter intake; ADG, Average daily gain; MBW, Metabolic body weight.
Footnotes
References
Archibeque, S. L., Burns, J. C., and Huntington, G. B. (2002). Nitrogen metabolism of beef steers fed endophyte free tall fescue hay: effects of ruminally protected methionine supplementation. J. Anim. Sci. 80, 1344–1351. doi: 10.2527/2002.8051344x
Artegoitia, V. M., Foote, A. P., Lewis, R. M., and Freetly, H. C. (2019). Metabolomics profile and targeted lipidomics in multiple tissues associated with feed efficiency in beef steers. ACS Omega 4, 3973–3982. doi: 10.1021/acsomega.8b02494
Arumugam, M., Raes, J., Pelletier, E., Paslier, D. L., Yamada, T., Mende, D. R., et al. (2011). Enterotypes of the human gut microbiome. Nature 473, 174–180. doi: 10.1038/nature09944
Bampidis, V., Azimonti, G., Bastos, M. D. L., Christensen, H., Dusemund, B., Kouba, M., et al. (2020). Safety and efficacy of L-isoleucine produced by fermentation with Corynebacterium glutamicum KCCM 80189 for all animal species. EFSA J. 18, 6021–6033. doi: 10.2903/j.efsa.2020.6021
Bindel, D. J., Drouillard, J. S., Titgemeyer, E. C., Wessels, R. H., and Löest, C. A. (2000). Effects of ruminally protected choline and dietary fat on performance and blood metabolites of finishing heifers. J. Anim. Sci. 78, 2497–2503. doi: 10.2527/2000.78102497x
Blusztajn, J. K. (1998). Choline, a vital amine. Science 281, 794–795. doi: 10.1126/science.281.5378.794
Brautigan, D. L., Li, R., Kubicka, E., and Turner, S. D. (2017). Lysolecithin as feed additive enhances collagen expression and villus length in the jejunum of broiler chickens. Poult. Sci. 96, 2889–2898. doi: 10.3382/ps/pex078
Burrin, D. G., Janeczko, M. J., and Stoll, B. (2008). Emerging aspects of dietary glutamate metabolism in the developing gut. Asia Pac. J. Clin. Nutr. 17, 368–371. doi: 10.6133/apjcn.2008.17.s1.92
Chen, S., Zhou, Y., Chen, Y., and Gu, J. (2008). fastp: an ultra-fast all-in-one FASTQ preprocessor. Bioinformatics 34, i884–i890. doi: 10.1093/bioinformatics/bty560
Cianciaruso, B., Jones, M. R., and Kopple, J. D. (1981). Histidine, an essential amino acid for adult dogs. J. Nutr. 111, 1074–1084. doi: 10.1093/jn/111.6.1074
Clemmons, B. A., Martino, C., Powers, J. B., Campagna, S. R., and Myer, P. R. (2019). Rumen bacteria and serum metabolites predictive of feed efficiency phenotypes in beef cattle. Sci. Rep. 9:19265. doi: 10.1038/s41598-019-55978-y
Clemmons, B. A., Mihelic, R. I., Beckford, R. C., Powers, J. B., Melchior, E. A., McFarlane, Z. D., et al. (2017). Serum metabolites associated with feed efficiency in black angus steers. Metabolomics 13:147. doi: 10.1007/s11306-017-1282-z
Clemmons, B. A., Powers, J. B., Campagna, S. R., Seay, T. B., Embree, M. M., and Myer, P. R. (2020). Rumen fluid metabolomics of beef steers differing in feed efficiency. Metabolomics 16:23. doi: 10.1007/s11306-020-1643-x
Crowley, J. J., Mcgee, M., Kenny, D. A., Crews, D. H., Evans, R. D., and Berry, D. P. (2010). Phenotypic and genetic parameters for different measures of feed efficiency in different breeds of Irish performance-tested beef bulls. J. Anim. Sci. 88, 885–894. doi: 10.2527/jas.2009-1852
Donaldson, G. P., Lee, S. M., and Mazmanian, S. K. (2016). Gut biogeography of the bacterial microbiota. Nat. Rev. Microbiol. 14, 20–32. doi: 10.1038/nrmicro3552
Dong, O. S., Satsu, H., and Shimizu, M. (2005). Histidine inhibits oxidative stress and TNF-α-induced interleukin-8 secretion in intestinal epithelial cells. FEBS Lett. 579, 4671–4677. doi: 10.1016/j.febslet.2005.07.038
Duan, J. L., Yin, J., Wu, M. M., Liao, P., Deng, D., Liu, G., et al. (2014). Dietary glutamate supplementation ameliorates mycotoxin-induced abnormalities in the intestinal structure and expression of amino acid transporters in young pigs. PLoS One 9:e112357. doi: 10.1371/journal.pone.0112357
Edgar, R. C. (2013). UPARSE: highly accurate OTU sequences from microbial amplicon reads. Nat. Methods 10, 996–998. doi: 10.1038/nmeth.2604
Feng, R. N., Niu, Y. C., Sun, X. W., Li, Q., Zhao, C., Wang, C., et al. (2013). Histidine supplementation improves insulin resistance through suppressed inflammation in obese women with the metabolic syndrome: a randomised controlled trial. Diabetologia 56, 985–994. doi: 10.1007/s00125-013-2839-7
Firkins, J. L., and Yu, Z. (2015). Ruminant nutrition symposium: how to use data on the rumen microbiome to improve our understanding of ruminant nutrition. J. Anim. Sci. 93, 1450–1470. doi: 10.2527/jas.2014-8754
Freetly, H. C., Aaron, D., Lindholm-Perry, A. K., Thallman, R. M., Keele, J. W., Foote, A. P., et al. (2020). Digestive tract microbiota of beef cattle that differed in feed efficiency. J. Anim. Sci. 98, 1–16. doi: 10.1093/jas/skaa008
Fujimura, K. E., Slusher, N. A., Cabana, M. D., and Lynch, S. V. (2010). Role of the gut microbiota in defining human health. Expert Rev. Anti-Infect. Ther. 8, 435–454. doi: 10.1586/eri.10.14
Gagen, E. J., Padmanabha, J., Denman, S. E., and McSweeney, C. S. (2015). Hydrogenotrophic culture enrichment reveals rumen Lachnospiraceae and Ruminococcaceae acetogens and hydrogen-responsive bacteroidetes from pasturefed cattle. FEMS Microbiol. Lett. 362:fnv104. doi: 10.1093/femsle/fnv104
Goldansaz, S. A., Guo, A. C., Sajed, T., Steele, M. A., Plastow, G. S., and Wishart, D. S. (2017). Livestock metabolomics and the livestock metabolome: a systematic review. PLoS One 12:e0177675. doi: 10.1371/journal.pone.0177675
Goodrich, J. K., Waters, J. L., Poole, A. C., Sutter, J. L., Koren, O., Blekhman, R., et al. (2014). Human genetics shape the gut microbiome. Cell 159, 789–799. doi: 10.1016/j.cell.2014.09.053
Goselink, R. M. A., Baal, J. V., Widjaja, H. C. A., Dekker, R. A., Zom, R. L. G., Veth, M. J. D., et al. (2013). Effect of rumen protected choline supplemention on liver and adipose gene expression during the transition period in dairy cattle. J. Dairy Sci. 96, 1102–1116. doi: 10.3168/jds.2012-5396
Greenwood, R. H., and Titgemeyer, E. C. (2000). Limiting amino acids for growing Holstein steer limit-fed soybean hull-based diets. J. Anim. Sci. 78, 1997–2004. doi: 10.2527/2000.7871997x
Guiotto, A., Calderan, A., Ruzza, P., and Borin, G. (2005). Carnosine and carnosine related antioxidants: a review. Curr. Med. Chem. 12:2293. doi: 10.2174/0929867054864796
Han, Z. Y., Yang, B. K., Yang, Z., and Xi, Y. M. (2017). Effects of 2-hydroxy-4-(methylthio) butanoic acid isopropyl ester on growth and blood components in growing Holstein steers. J. Anim. Sci. 88, 286–293. doi: 10.1111/asj.12648
Hanning, I., and Diaz-Sanchez, S. (2015). The functionality of the gastrointestinal microbiome in non-human animals. Microbiome 3:51. doi: 10.1186/s40168-015-0113-6
He, L., Long, J., Zhou, X., Liu, Y., Li, T., and Wu, X. (2020). Serine is required for the maintenance of redox balance and proliferation in the intestine under oxidative stress. FASEB J. 34, 4702–4717. doi: 10.1096/fj.201902690R
Herd, R., Archer, J., and Arthur, P. (2003). Reducing the cost of beef production through genetic improvement in residual feed intake: opportunity and challenges to application. J. Anim. Sci. 13, E9–E17. doi: 10.2527/2003.8113_suppl_1E9x
Hussein, H. S., and Berger, L. L. (1995). Feedlot performance and carcass characteristics of Holstein steers as affected by source of dietary protein and level of ruminally protected lysine and methionine. J. Anim. Sci. 73, 3503–3509. doi: 10.2527/1995.73123503x
Huttenhower, C., Gevers, D., Knight, R., Abubucker, S., and Badger, J. H. (2012). Structure, function and diversity of the healthy human microbiome. Nature 486:207. doi: 10.1038/nature11234
Huws, S. A., Creevey, C. J., Oyama, L. B., Mizrahi, I., Denman, S. E., Popova, M., et al. (2018). Addressing global ruminant agricultural challenges through understanding the rumen microbiome: past, present, and future. Front. Microbiol. 9:2161. doi: 10.3389/fmicb.2018.02161
Jewell, K. A., McCormick, C. A., Odt, C. L., Weimer, P. J., and Suen, G. (2015). Ruminal bacterial community composition in dairy cows is dynamic over the course of two lactations and correlates with feed efficiency. Appl. Environ. Microbiol. 81, 4697–4710. doi: 10.1128/AEM.00720-15
Jones, E., Oliphant, E., and Peterson, P. (2001). SciPy: Open Source Scientific Tools for Python. Available at: http://www.scipy.org (Accessed December 20, 2021).
Kalhan, S. C., and Hanson, R. W. (2012). Resurgence of serine: an often neglected but indispensable amino acid. J. Biol. Chem. 287, 19786–19791. doi: 10.1074/jbc.r112.357194
Kim, M., Park, T., and Yu, Z. (2017a). Metagenomic investigation of gastrointestinal microbiome in cattle. Asian-Australas. J. Anim. Sci. 30, 1515–1528. doi: 10.5713/ajas.17.0544
Kim, B. R., Shin, J., Guevarra, R., Lee, J. H., Kim, D. W., Seol, K. H., et al. (2017b). Deciphering diversity indices for a better understanding of microbial communities. J. Microbiol. Biotechnol. 27, 2089–2093. doi: 10.4014/jmb.1709.09027
Kitamoto, S., Alteri, C. J., Rodrigues, M., Nagao-Kitamoto, H., and Kamada, N. (2020). Dietary l-serine confers a competitive fitness advantage to enterobacteriaceae in the inflamed gut. Nat. Microbiol. 5, 116–125. doi: 10.1038/s41564-019-0591-6
Kolde, R. (2012). Pretty heatmaps. R Package Version. p. 61. Available at: http://CRAN.R-project.org/package=pheatmap (Accessed January 04, 2019).
Lancaster, P. A., Carstens, G. E., Crews, D. H., Welsh, T. H., Forbes, T. D. A., Forrest, D. W., et al. (2009). Phenotypic and genetic relationships of residual feed intake with performance and ultrasound carcass traits in Brangus heifers. J. Anim. Sci. 87, 3887–3896. doi: 10.2527/jas.2009-2041
Lancaster, P. A., Carstens, G. E., Michal, J. J., Brennan, K. M., Johnson, K. A., and Davis, M. E. (2014). Relationships between residual feed intake and hepatic mitochondrial function in growing beef cattle. J. Anim. Sci. 92, 3134–3141. doi: 10.2527/jas.2013-7409
Li, F. Y., and Guan, L. L. (2017). Metatranscriptomic profling reveals linkages between the active rumen microbiome and feed efciency in beef cattle. Appl. Environ. Microbiol. 83, e00061–e00117. doi: 10.1128/aem.00061-17
Liu, C., Wu, H., Liu, S., Chai, S., Qing, X., and Zhou, Z. (2019). Dynamic alterations in yak rumen bacteria community and metabolome characteristics in response to feed type. Front. Microbiol. 10:1116. doi: 10.3389/fmicb.2019.01116
Löest, C. A., Titgemeyer, E. C., St-Jean, G., Metre, D. C. V., and Smith, J. S. (2002). Methionine as a methyl group donor in growing cattle. J. Anim. Sci. 80, 2197–2206. doi: 10.2527/2002.8082197x
Lopes, D. R. G., Duarte, M. D. S., Reau, A. J. L., Chaves, I. Z., and Mantovani, H. C. (2021). Assessing the relationship between the rumen microbiota and feed efficiency in nellore steers. J. Anim. Sci. Biotechnol. 12:79. doi: 10.1186/s40104-021-00599-7
Lopes, D. R. G., Reau, A. J. L., Duarte, M. D. S., Detmann, E., Bento, C. B. P., Mercadante, M. E. Z., et al. (2019). The bacterial and fungal microbiota of Nelore steers is dynamic across the gastrointestinal tract and its fecal-associated microbiota is correlated to feed efficiency. Front. Microbiol. 10, 1263–1278. doi: 10.3389/fmicb.2019.01263
Magoč, T., and Salzberg, S. L. (2011). FLASH: fast length adjustment of short reads to improve genome assemblies. Bioinformatics 27, 2957–2963. doi: 10.1093/bioinformatics/btr507
Malanka, K., Smoleńska-Sym, G., Michur, H., Wróbel, A., Lachert, E., and Brojer, E. (2012). Lysophosphatidylcholines: bioactive lipids generated during storage of blood components. Arch. Immunol. 60, 55–60. doi: 10.1007/s00005-011-0154-x
Marie, T., Jean, C., Eun-Jeong, Y., Lenka, K., Gustavo, C., Cerqueira, C. M., et al. (2014). The genomic diversification of the whole acinetobacter genus: origins, mechanisms, and consequences. Genome Biol. Evol. 6, 2866–2882. doi: 10.1093/gbe/evu225
Mariz, L., Amaral, P. M., Valadares, F., Santos, S. A., Detmann, E., Marcondes, M. I., et al. (2018). Dietary protein reduction on microbial protein, amino acids digestibility and body retention in beef cattle: 2. Amino acid intestinal absorption and their efficiency for whole-body deposition. J. Anim. Sci. 96:670. doi: 10.1093/jas/sky018
Martinez, Y., Li, X., Liu, G., Bin, P., Yan, W., Más, D., et al. (2017). The role of methionine on metabolism, oxidative stress, and diseases. Amino Acids 49, 2091–2098. doi: 10.1007/s00726-017-2494-2
McGovern, E., Kenny, D. A., McCabe, M. S., Fitzsimons, C., McGee, M., Kelly, A. K., et al. (2018). 16S rRNA sequencing reveals relationship between potent cellulolytic genera and feed efficiency in the rumen of bulls. Front. Microbiol. 9:1842. doi: 10.3389/fmicb.2018.01842
Morotomi, M., Nagai, F., and Watanabe, Y. (2012). Description of Christensenella minuta gen. nov., sp. nov., isolated from human faeces, which forms a distinct branch in the order Clostridiales, and proposal of Christensenellaceae fam. Nov. Int. J. Syst. Bacteriol. 62:144. doi: 10.1099/ijs.0.026989-0
Myer, P. R., Freetly, H. C., Wells, J. E., Smith, T. P. L., and Kuehn, L. A. (2017). Analysis of the gut bacterial communities in beef cattle and their association with feed intake, growth, and efficiency. J. Anim. Sci. 95, 3215–3224. doi: 10.2527/jas.2016.1059
Myer, P. R., Smith, T. P. L., Wells, J. E., Kuehn, L. A., and Freetly, H. C. (2015a). Rumen microbiome from steers differing in feed efficiency. PLoS One 10:e0129174. doi: 10.1371/journal.pone.0129174
Myer, P. R., Wells, J. E., Smith, T. P., Kuehn, L. A., and Freetly, H. C. (2015b). Cecum microbial communities from steers differing in feed efficiency. J. Anim. Sci. 93, 5327–5340. doi: 10.2527/jas.2015-9415
Myer, P. R., Wells, J. E., Smith, T. P., Kuehn, L. A., and Freetly, H. C. (2016a). Microbial community profiles of the jejunum from steers differing in feed efficiency. J. Anim. Sci. 94, 327–338. doi: 10.2527/jas.2015-9839
Myer, P. R., Wells, J. E., Smith, T. P. L., Kuehn, L. A., and Freetly, H. C. (2016b). Analysis of the gut bacterial communities in beef cattle and their association with feed intake, growth, and efficiency. J. Anim. Sci. 95, 3215–3224. doi: 10.2527/jas.2016.1059
National Academies of Sciences Engineering and Medicine (2016). Nutrient Requirements of Beef Cattle: Eighth Revised Edition. Washington, DC: The National Academies Press.
Newman, A. C., and Maddocks, O. D. K. (2017). Serine and functional metab olites in cancer. Trends Cell Biol. 27, 645–657. doi: 10.1016/j.tcb.2017.05.001
Nishizuka, Y. (1984). Turn over of inositol phospholipids and signal transduction. Science 225, 1365–1370. doi: 10.1126/science.6147898
Nkrumah, J. D., Basarab, J. A., Price, M. A., Okine, E. K., Ammoura, A., Guercio, S., et al. (2004). Different measures of energetic efficiency and their phenotypic relationships with growth, feed intake, and ultrasound and carcass merit in hybrid cattle. J. Anim. Sci. 82, 2451–2459. doi: 10.2527/2004.8282451x
Nkrumah, J. D., Okine, E. K., Mathison, G. W., Schmid, K., Li, C., Basarab, J. A., et al. (2006). Relationships of feedlot feed efficiency, performance, and feeding behavior with metabolic rate, methane production, and energy partitioning in beef cattle. J. Anim. Sci. 84, 145–153. doi: 10.2527/2006.841145x
Obata, T., Aomine, M., and Yamanaka, Y. (1999). Protective effect of histidine on iron (II)-induced hydroxyl radical generation in rat hearts. J. Physiol. Paris 93, 213–218. doi: 10.1016/s0928-4257(99)80153-3
Oksanen, J., Blanchet, F.G., Kindt, R., Legendre, P., Minchin, P.R., O’Hara, R.B., et al. (2015). Vegan: Community Ecology Package. R Package Version 2.2-1.
Oksanen, J., Blanchet, F.G., Kindt, R., Legendre, P., O’Hara, R., Simpson, G.L., et al. (2010). Vegan: Community Ecology Package. R Package Version 1.17-4.
Oliveira, M. D., Jewell, K. A., Freitas, F. S., Benjamin, L. A., Tótola, M. R., Borges, A. C., et al. (2013). Characterizing the microbiota across the gastrointestinal tract of a Brazilian Nelore steer. Vet. Microbiol. 164, 307–314. doi: 10.1016/j.vetmic.2013.02.013
Parks, D. H., Tyson, G. W., Hugenholtz, P., and Beiko, R. G. (2014). STAMP: statistical analysis of taxonomic and functional profiles. Bioinformatics 30, 3123–3124. doi: 10.1093/bioinformatics/btu494
Paz, H. A., Hales, K. E., Wells, J. E., Kuehn, L. A., Freetly, H. C., Berry, E. D., et al. (2018). Rumen bacterial community structure impacts feed efficiency in beef cattle. J. Anim. Sci. 96, 1045–1058. doi: 10.1093/jas/skx081
Perea, K., Perz, K., Olivo, S. K., Williams, A., and Yeoman, C. J. (2017). Feed efficiency phenotypes in lambs involve changes in ruminal, colonic, and small-intestine-located microbiota. J. Anim. Sci. 95, 2585–2592. doi: 10.2527/jas2016.1222
Quast, C., Pruesse, E., Yilmaz, P., Gerken, J., and Glckner, F. O. (2013). The silva ribosomal RNA gene database project: improved data processing and web-based tools. Nucleic Acids Res. 41, D590–D596. doi: 10.1093/nar/gks1219
R Core Team (2013). R: A Language and Environment for Statistical Computing. Vienna: R Foundation for Statistical Computing. Available at: http://www.R-project.org/ (Accessed March 31, 2021).
Rao, K. V. R., Reddy, P. V. B., Tong, X., and Norenberg, M. D. (2010). Brain edema in acute liver failure: inhibition by L-histidine. Am. J. Pathol. 176, 1400–1408. doi: 10.2353/ajpath.2010.090756
Saleem, F., Bouatra, S., Guo, A. C., Psychogios, N., Mandal, R., Dunn, S. M., et al. (2013). The bovine ruminal fluid metabolome. Metabolomics 9, 360–378. doi: 10.1007/s11306-012-0458-9
Schloss, P. D., Westcott, S. L., Ryabin, T., Hall, J. R., Hartmann, M., Hollister, E. B., et al. (2009). Introducing mothur: open-source, platform-independent, community-supported software for describing and comparing microbial communities. Appl. Environ. Microbiol. 75, 7537–7541. doi: 10.1128/AEM.01541-09
Schnepf, R. (2020). US Farm Income. Washington (DC): Congressional Research Service. Available at: http://digitalcommons.ilr.cornell.edu/key_workplace/1167 (Accessed January 24, 2012).
Shabat, S. K., Sasson, G., Doron-Faigenboim, A., Durman, T., Yaacoby, S., Berg-Miller, M. E., et al. (2016). Specific microbiome-dependent mechanisms underlie the energy harvest efficiency of ruminants. ISME J. 10, 2958–2972. doi: 10.1038/ismej.2016.62
Smriga, M., Mori, M., and Torii, K. (2000). Circadian release of hypothalamic norepinephrine in rats in vivo is depressed during early L-lysine deficiency. J. Nutr. 130, 1641–1643. doi: 10.1046/j.1365-277x.2000.00231.x
Stackebrandt, E., and Goebel, B. M. (1994). Taxonomic note: a place for DNA-DNA reassociation and 16S rRNA sequence analysis in the present species definition in bacteriology. Int. J. Syst. Bacteriol. 44, 846–849. doi: 10.1099/00207713-44-4-846
Sun, X., Feng, R., Li, Y., Lin, S., Zhang, W., Li, Y., et al. (2014). Histidine supplementation alleviates inflammation in the adipose tissue of high-fat diet-induced obese rats via the NF-kB- and PPARγ-involve pathways. Br. J. Nutr. 112, 477–485. doi: 10.1017/s0007114514001056
Swanepoel, N., Robinson, P. H., and Erasmus, L. J. (2010a). Amino acid needs of lactating dairy cows: predicting limiting amino acids in contemporary rations fed to high producing dairy cattle in California using metabolic models. Anim. Feed Sci. Technol. 161, 103–120. doi: 10.1016/j.anifeedsci.2010.08.005
Swanepoel, N., Robinson, P. H., and Erasmus, L. J. (2010b). Amino acid needs of lactating dairy cows: impact of feeding lysine in a ruminally protected form on productivity of lactating dairy cows. Anim. Feed Sci. Technol. 157, 79–94. doi: 10.1016/j.anifeedsci.2010.02.008
Vanhatalo, A., Huhtanen, P., Toivonen, V., and Varvikko, T. (1999). Response of dairy cows fed grass silage diets to abomasal infusions of histidine alone or in combinations with methionine and lysine. J. Dairy Sci. 82, 2674–2685. doi: 10.3168/jds.S0022-0302(99)75524-4
Wang, Q., Garrity, G. M., Tiedje, J. M., and Cole, J. R. (2007). Naive Bayesian classifier for rapid assignment of rRNA sequences into the new bacterial taxonomy. Appl. Environ. Microbiol. 73, 5261–5267. doi: 10.1128/aem.00062-07
Wang, L. M., Zhu, W. B., Yang, J., Miao, L. H., Dong, J. J., Song, F. B., et al. (2018). Effects of dietary cystine and tyrosine on melanogenesis pathways involved in skin color differentiation of Malaysian red tilapia. Aquaculture 490, 149–155. doi: 10.1016/j.aquaculture.2018.02.023
Wantanee, K., Mahroukh, R., Wykes, L. J., Ball, R. O., and Pencharz, P. B. (2002). Long-term effects of histidine depletion on whole-body protein metabolism in health adults. J. Nutr. 132, 3340–3348. doi: 10.1093/jn/132.11.3340
Warnes, G. R., Bolker, B., Bonebakker, L., Gentleman, R., Liaw, W. H. A., Lumley, T., et al. (2016). gplots: Various R Programming Tools for Plotting Data. R Package Version 3.0.1. The Comprehensive R Archive Network. Available at: https://cran.rproject.org/web/packages/gplots/gplots.pdf (Accessed November 28, 2020).
Welch, C. B., Lourenco, J. M., Davis, D. B., Krause, T. R., Carmichael, M. N., Rothrock, M. J., et al. (2020). The impact of feed efficiency selection on the ruminal, cecal, and fecal microbiomes of Angus steers from a commercial feedlot. J. Anim. Sci. 98:skaa230. doi: 10.1093/jas/skaa230
Ye, M., Guo, G. Y., Lu, Y., Song, S., Wang, H. Y., and Yang, L. (2014). Purification, structure and anti-radiation activity of melanin from Lachnum YM404. Int. J. Biol. Macromol. 63, 170–176. doi: 10.1016/j.ijbiomac.2013.10.046
Yin, J., Liu, M., Ren, W., Duan, J., Yang, G., Zhao, Y., et al. (2015). Effects of dietary supplementation with glutamate and aspartate on diquat-induced oxidative stress in piglets. PLoS One 10:e0122893. doi: 10.1371/journal.pone.0122893
Keywords: small intestine, microbiota, metabolomics, residual feed intake, beef cattle
Citation: Liu Y, Liu C, Wu H, Meng Q and Zhou Z (2022) Small Intestine Microbiome and Metabolome of High and Low Residual Feed Intake Angus Heifers. Front. Microbiol. 13:862151. doi: 10.3389/fmicb.2022.862151
Edited by:
Graham Cliff Lamb, Texas A&M University College Station, United StatesReviewed by:
Neeta Agarwal, Indian Veterinary Research Institute (IVRI), IndiaNicolas DiLorenzo, University of Florida, United States
Copyright © 2022 Liu, Liu, Wu, Meng and Zhou. This is an open-access article distributed under the terms of the Creative Commons Attribution License (CC BY). The use, distribution or reproduction in other forums is permitted, provided the original author(s) and the copyright owner(s) are credited and that the original publication in this journal is cited, in accordance with accepted academic practice. No use, distribution or reproduction is permitted which does not comply with these terms.
*Correspondence: Zhenming Zhou, emhvdXptQGNhdS5lZHUuY24=
†These authors have contributed equally to this work