- 1Institute of Resource, Ecosystem and Environment of Agriculture, Nanjing Agricultural University, Nanjing, China
- 2Department of Soil Science, Nanjing Agricultural University, Nanjing, China
- 3Jiangsu Collaborative Innovation Center for Solid Organic Waste Resource Utilization, Nanjing Agricultural University, Nanjing, China
- 4Department of Civil and Environmental Engineering, Massachusetts Institute of Technology, Cambridge, MA, United States
- 5Center of Agro-Technology Extension, Suzhou, China
Microbial communities of soil aggregate-size fractions were explored with molecular and networking assays for topsoil samples from a clayey rice paddy under long-term fertilization treatments. The treatments included no fertilizer (NF) as control, chemical fertilizer only (CF), chemical fertilizer with swine manure (CFM), and chemical fertilizer with rice straw return (CFS). Following a wet-sieving protocol, water-stable aggregates were separated into size fractions of large macroaggregates (L-MacA, >2,000 μm), macroaggregates (MacA, 2,000–250 μm), microaggregates (MicA, 250–53 μm), fine microaggregates (F-MicA, 53–2 μm), and fine clay (F-Clay, <2 μm). Mass proportion was 32.3–38.2% for F-MicA, 23.0–31.5% for MacA, 19.0–23.1% for MicA, 9.1–12.0% for L-MacA, and 4.9–7.5% for F-Clay, respectively. The proportion of MacA was increased, but F-Clay was reduced by fertilization, whereas the mean weight diameter was increased by 8.0–16.2% from 534.8 μm under NF to 621.5 μm under CFM. Fertilization affected bacterial 16S rRNA and fungal 18S rRNA gene abundance in F-MicA and F-Clay but not in aggregates in size larger than 53 μm. However, bacterial and fungal community α-diversities and community structures were quite more divergent among the fertilization treatments in all size fractions. Organic carbon and gene abundance of bacteria and fungi were enriched in both L-MacA and MacA but depleted in F-Clay, whereas microbial Shannon diversity was rarely changed by fraction size under the four treatments. L-MacA and MacA contained more bacteria of r-strategists and copiotrophs, whereas F-MicA and F-Clay were demonstrated with a higher abundance of K-strategists and oligotrophs. Guilds of parasitic and litter saprotrophic fungi were enriched in F-MicA but depleted in L-MacA. Furthermore, most of bacterial and fungal operational taxonomic units were strongly interacted in L-MacA and MacA rather than in MicA and F-Clay. Thus, MacA acted as micro-hotspots enriched with functional and networked microbial communities, which were enhanced with organic/inorganic fertilization in the rice paddy.
Introduction
Soil structure is known to be essential for soil porosity, water movement, solute transport, gas exchanges, carbon sequestration (Six et al., 2002; Pituello et al., 2016), and biological components (Tecon and Or, 2017). Soil aggregation, a key soil formation process, can greatly affect soil biophysical entity, resulting in distinct microbial communities (Wilpiszeski et al., 2019) and consequent biogeochemical processes (Lehmann and Rillig, 2015). Meanwhile, soil microbial biota in turn can create protected microcosms within soil and mediate microscale ecological interactions (Vos et al., 2013). Microbial populations are spatially isolated and stabilized within soil aggregates, often regarded as “concurrent incubators” for the evolution of microbial communities inside (Rillig et al., 2017). Therefore, variations in composition and function of microbial communities across aggregate-size fractions are increasingly addressed as microscale mechanisms in soil biogeochemical processes, owing to the controls on overall performance and the mediation of element cycling in soil (Six et al., 2000; Rillig et al., 2017; Li et al., 2019c; Wilpiszeski et al., 2019).
Soil organic matter (SOM) plays a vital role in maintaining global carbon balance, supporting food production and sustaining soil ecosystem services (Lal, 2004; Powlson et al., 2011; Schmidt et al., 2011; Wiesmeier et al., 2019). Soil microorganisms, the key driver of SOM turnover (Gleixner, 2013; Kallenbach et al., 2016), can also be largely shaped by SOM in reverse in community structure (Roller and Schmidt, 2015). SOM accumulation is often associated with distinct microbial communities with higher fungal abundances and efficient microbial biomass production (Kallenbach et al., 2016). Furthermore, soil microhabitats with higher labile organic content and microbial population higher in carbon metabolism efficiency are suggested as microbial hotspots for C biogeochemical cycling in soil, compared with bulk soil (Kuzyakov and Blagodatskaya, 2015). The distribution, decomposability, and accessibility of SOM, at both spatial and temporal scales, substantially determine the microbial and functional diversity among aggregate-size fractions (Marx et al., 2005; Wallenius et al., 2011; Lagomarsino et al., 2012). In response to SOM accumulation, changes in microbial activity can cope with stabilizing organic carbon and soil aggregates for ecological functioning (Tecon and Or, 2017). For example, microbial metabolites can contribute notably to aggregate stabilization by binding mineral particles and/or microaggregates to form macroaggregates (MacA) (Wander, 2004), within which particulate organic matter (POM) is preserved and physically protected by spatially excluding from microbes and enzymes (Six et al., 2000; Martens et al., 2004; Lützow et al., 2006; Six and Paustian, 2014). As a major C sequestration mechanism, the extent of microbes accessing to SOM under physical protection can be conferred by localization of microbial communities, extracellular enzyme activities, and carbon substrates among soil aggregates (Dungait et al., 2012; Navarro-Garcia et al., 2012; Trivedi et al., 2017). Therefore, in agricultural management, organic fertilization is the most effective way to improve soil fertility by promoting SOM content, soil aggregation, and microbial activity (Zhang et al., 2007, 2015; Wang et al., 2017; Li et al., 2019c).
Traditionally, we often research soil functions especially related to C and N cycling by determining the specific extracellular enzyme activities. For example, our previous work has addressed that carbon-degrading enzymes and N-acetylglucosaminidase activities are enriched in >53-μm aggregate fractions under different fertilization, whereas β-glucosidase and cellobiohydrolase activities are enhanced under CFS (Li et al., 2019c). However, we cannot achieve a general view of soil functioning mediated by microbial community in detail in such manner. Recently, the tools of PICRUSt (Phylogenetic Investigation of Communities by Reconstruction of Unobserved States) and FUNGuild (Fungi functional guild) have been increasingly used for researching the functional profiles of bacterial (Hou et al., 2019; Wang et al., 2019; Liu et al., 2020; Lu et al., 2020; Ma et al., 2020) and fungal community (Schlatter et al., 2018; Li et al., 2019b; Lu et al., 2020), respectively, based on amplicon sequencing in soil science. By analyzing functional results, the general metabolic functions of soil microbial community can also be linked to microbial trophic and ecological strategy (Koch, 2001; Lauro et al., 2009; Song et al., 2017). For instance, Song et al. (2017) addressed that a high (copiotrophic) or low (oligotrophic) nutrient strategy was rich in genes, respectively, related to cell division and cell cycle or related to carbohydrate metabolism and virulence, disease, and defense, whereas an r- or K-strategy was rich in genes, respectively, related to regulation and cell signaling or related to motility and chemotaxis, whereas these tools have been rarely applied to studying microbial functional profiles of communities across soil aggregate-size fractions. At the macroscale, soil microbial interactions are known to be altered by abiotic or biotic factors, or both, which can also result in specific community structure and metabolic functional profile (Prosser et al., 2007; Barberan et al., 2012; Ma et al., 2016; Schlatter et al., 2018; Schmid et al., 2018; Hou et al., 2019). Using high-throughput sequencing data sets, network analysis of significant taxon co-occurrence patterns of soil microbiota can help to disentangle community assembly patterns and interactions among microbial individuals within microbial consortia (Horner-Devine et al., 2007; Fuhrman, 2009). Meanwhile, based on the analysis of co-occurrence network, bacterial functional complexity and community interactions also have been used for demonstrating geographical variation of soil microbiome (Ma et al., 2016) and for assessing the associations between microbial interaction and soil quality (Schmid et al., 2018).
Paddy soil, as a unique type of anthropogenic soil formed due to long-term hydragric management for rice production under prolonged surface flooding (Gong et al., 2007), has higher SOC storage and sequestration potential compared with dry croplands (Wu et al., 2003; Pan et al., 2004; Haefele et al., 2014). Long-term rice cultivation leads to an accumulation of SOM and in turn soil microbial abundance and activity, with the latter positively correlated to POM accumulation in MacA (Wang et al., 2015; Liu et al., 2016). Optimized management practices including combined organic/inorganic fertilization can enhance SOM accumulation and microbial activity, thus improving soil fertility of paddy soils (Li et al., 2007; Pan et al., 2009). With long-term field experiments, soil macroaggregation of rice paddy has been seen significantly enhanced under organic/inorganic fertilization compared with mineral fertilization (Zhang et al., 2014; Wang et al., 2018). Under combined application of rice straw and balanced chemical fertilizers (Ding et al., 2018), gene abundance and diversity of microbes involved in carbon and nitrogen cycling are increased, and the overall community shifts toward better nutrient biotransformation and plant productivity.
However, the comprehensive and deep study to disentangle how soil performs ecosystem service functions through microbial functioning in different-size fractions of aggregates under different fertilization is still limited. The knowledge of microbial community interactions and functions at the aggregate scale is still insufficient for understanding soil processes governed by soil microbes (Wilpiszeski et al., 2019). Moreover, the distribution of microbial community and functional groups and how they coexist or network within aggregates sustaining carbon and nitrogen cycling in rice paddy soil under long-term fertilization remain yet unclear.
Therefore, it was hypothesized that microbial abundance, diversity, and functional association concerning C cycling could be enhanced in MacA of fertilized paddy soil, due to a physically protected labile C pool and diverse mineral-associated stable C pool in microaggregates within MacA. We also hypothesized that long-term organic/inorganic fertilization could promote the microbial diversity and co-occurrence networks among the water-stable aggregates of rice paddy, by increasing soil macroaggregation. Taking a paddy soil under long-term conventional fertilization as a case, this study aims to provide novel knowledge of microbial communities, interactions, and functions among aggregate-size fractions for understanding SOM accumulation and ecosystem functioning improvement under rational soil management to sustain rice agriculture.
Materials and Methods
Experiment Site and Soil
In this study, topsoil samples from a rice paddy were used under a long-term different fertilizer application trial initiated in 1987. The experiment site was located in Jinjiaba Township (31°12′15″ N, 120°15′15″ E), Suzhou Municipality, Jiangsu Province, China. Lying in the central Tai Lake plain, the area was controlled by a humid subtropical monsoon climate, with a mean annual temperature of 15.9°C and precipitation of 1,110 mm, during 1993–2013. Derived from paleo-alluvial/lacustrine deposit, because of the long history of hydroagric management for rice production, the soil had developed as an Entic Halpudept (Soil Survey Staff, 2014) or a Ferric-accumulic Stagnic Anthrosol according to Chinese Soil Taxonomy (Gong et al., 2007). The soil had been conventionally cultivated with summer rice (Oryza sativa L.) and winter rape (Brassica campestris L.) in an annual rotation for the last decades. The soil texture was clayey loam with 30.3% of clay (<2 μm). The basic properties of topsoil before the experiment were soil pH (H2O) of 5.6, bulk density of 1.2 g cm–3, cation exchange capacity of 20.5 cmol (+) kg–1, soil organic carbon (SOC) of 14.3 g kg–1, and total N of 1.7 g kg–1.
Fertilization Treatment
The fertilizer application treatments included no fertilizer (NF) as a control, chemical fertilizer only (CF), chemical fertilizer plus rice straw return (CFS), and chemical fertilizer plus pig manure (CFM), as described elsewhere (Pan et al., 2009). As per the conventional rates by local farmers, chemical fertilizers were applied at in total 427.5 kg N ha–1, 45 kg P2O5 ha–1 and 84 kg K ha–1 every year, with 60% allocated for rice and the other 40% for rape. Meanwhile, rice straw was applied at 4.5 t ha–1 for CFS and swine manure at 16.8 t ha–1 (fresh weight) for CFM, every year. Swine manure was collected from a household pig farm, and fresh rice straw after harvest was chopped before use. Both chopped rice straw and swine manure were spread onto topsoil and merged into a depth of 0–20 cm with manual operation before rape seedling transplantation. Triplicated subplots (each 66.7 m2 in area) for each treatment were arranged in a completely randomized block design. All the farming practices including irrigation and plant protection were consistent across the treatments. The basic properties of topsoil under the treatments are listed in Table 1.
Soil Sampling
Field moist topsoil (0–15 cm) samples were collected at rice harvest in early November 2013. In each plot, five undisturbed bulk soil cores were randomly taken with a soil core sampler and pooled and homogenized as a composite sample of approximately 1 kg. All samples were sealed in stainless steel cans, stored in an icebox, and shipped to the laboratory within 24 h following sampling. After removal of plant debris, a sample was gently crushed into small clods of about 10 mm in size and further homogenized. Subsequently, the sample was divided into four portions by quadrats. One portion was frozen-dried and then stored at −70°C prior to DNA extraction. Another portion was sieved through a 2-mm mesh and air-dried prior to treatment for basic physicochemical determination. The remnant two portions were pooled and directly stored in a stainless steel jar at 4°C before aggregate-size fractionation and microbial biomass determination for bulk soil.
Aggregate-Size Fractionation
Size fractionation of soil-stable aggregates was performed with a wet-sieving protocol using a series of nylon sieves, respectively, in sizes of 2,000, 250, and 53 μm in distilled water, based on Stemmer et al. (1998) with modifications after Fansler et al. (2005) and Lobe et al. (2011). In detail, a 300-g equivalent moist bulk sample was gently and evenly put on the top of a nylon sieve of 2-mm mesh size and submerged for 10 min in water at room temperature. The sieves were gently moved up and down by 3 cm to the water table for 250 times. Three fractions of water-stable soil aggregates remaining on each sieve were, respectively, collected as large MacA (L-MacA) in size of >2,000 μm, MacA in size of 250–2,000 μm, and microaggregates (MicA) in size of 250–53 μm. Subsequently, the soil suspension passed through the 53-μm sieve was centrifuged at 600 revolutions per minute [rpm] (86 relative centrifugal force [rcf] × g) for 4 min in 500-mL plastic centrifuge bottles to collect fine microaggregates (F-MicA) in size of 53–2 μm. The subsequent suspension was again centrifuged at 4,200 rpm (4,219 rcf × g) for 36 min in 50-mL centrifuge tubes to obtain the fine clay (F-Clay) particles in size <2 μm. Finally, each obtained size fraction sample was frozen-dried and stored in polyethylene bags at −70°C prior to physicochemical determination and DNA extraction.
Soil Properties Analysis
Analysis of basic properties of bulk soil and aggregate fraction samples was done following the methods described by Lu (2000). Soil moisture content was measured by oven-drying at 105°C for 12 h. SOC content was determined with wet digestion and dichromate oxidation, whereas total nitrogen (TN) with Kjeldahl method. Soil pH was determined in suspension with the soil-to-water ratio of 1:2.5 (m/v) using a Mettler Toledo pH meter (SevenEasy S20K, Mettler Toledo Inc, 2008). Microbial biomass carbon (MBC) and nitrogen (MBN) contents were determined using the fumigation–extraction protocol (Brookes et al., 1985). Differences in carbon and nitrogen contents between fumigated and unfumigated samples were calculated and converted to contents of MBC and MBN, respectively, with a coefficient of 0.38 and 0.45.
DNA Extraction and Quantification of Bacteria and Fungi Population
Total DNA of soil aggregate-size fraction samples was extracted from 0.25 g equivalent dry soil using the PowerSoil® DNA Isolation Kit (MO BIO Laboratories Inc., Carlsbad, CA, United States). The extracted DNA was qualified by gel electrophoresis and quantified using a NanoDrop 2000 spectrophotometer (Thermo Scientific, MA, United States) and then stored at −70°C prior to molecular assays. Quantification of bacterial 16S rDNA and fungal 18S rDNA was performed on a 7500 real-time polymerase chain reaction (PCR) system (Applied Biosystems, United States) using SYBR® Premix Ex Taq™ II kit (TAKARA). Specifically, universal primer pairs of 338F (ACTCCTACGGGAGGCAGCAG)/518R(ATTACCGCGGCTG CTGG) (Fierer et al., 2005) and NS1F (GTAGTCATATGCT TGTCTC)/FungR (ATTCCCCGTTACCCGTTG) (May et al., 2001) were used to target bacterial 16S and fungal 18S rRNA genes, respectively. The quantitative PCR conditions included an initial denaturation at 95°C for 5 min, followed by denaturation at 95°C for 45 s for 35 cycles, and annealing at 56°C for bacteria and 57°C for fungi for 30 s, respectively. Standard curves were generated with triplicated 10-fold dilution series of plasmids with the corresponding target genes. The R2 of each standard curve exceeded 0.99, and each PCR efficiency was between 90 and 110%.
Amplicon Sequencing of Bacterial and Fungal House-Keeping Genes
The amplicon sequencing library was prepared by targeting the V4–V5 hypervariable region of bacterial 16S rRNA gene and/or the ITS1 region of fungal ITS. Primer pairs of 515F (GTGCCAGCMGCCGCGG)/907R(CCGTCAATTCMTTTRAG TTT) were chosen to flank the bacterial target region, whereas the fungal ITS region was captured by primer pairs of ITS1F (CTTGGTCATTTAGAGGAAGTAA)/ITS2(GCTGCGTTCTTC ATCGATGC). A 20-μL mixture was prepared for each PCR reaction, including 1× reaction buffer (TAKARA), 2 mM Mg2+, 0.2 mM dNTP, 0.1 μM of each primer, 1 U HotStar Taq polymerase (TAKARA), and 2 μL template DNA, followed by 95°C for 2 min; 35 cycles of 94°C for 20 s, 55°C for 40 s, 72°C for 1 min, and 72°C for 2 min. The PCR products were then subject to adapter annealing where a unique 6-bp barcode was added by a second PCR reaction. Each reaction contained 1 × reaction buffer (NEB Q5™), 0.3 mM dNTP, 0.25 μM of F primer, 0.25 μM of index primer, 1 U Q5™ DNA polymerase, and 1 μL diluted template. The PCR conditions included an initial denaturation at 98°C for 30 s followed by 11 cycles of (i) denaturation (98°C for 10 s), and (ii) annealing (65°C for 30 s), and extension (72°C for 30 s). The PCR ended with a final extension at 72°C for 5 min. Paired-end sequence data were assembled by FLASH (Magoc and Salzberg, 2011) after masking low-quality end bases (<Q15) with the FASTX-Toolkit1. Barcodes and primers were trimmed with cutadapt (Martin, 2011). Sequences shorter than 200 bp and with a quality score <20 were discarded, and chimeras and singletons were filtered with the UPARSE algorithm (Edgar, 2013). The remaining reads were then clustered into operational taxonomic units (OTUs) at 97% similarity cutoff via UPARSE with default settings. Taxonomy was assigned to bacterial OTUs by RDP2 or to fungal OTUs by UNITE3. Each sample was rarefied before downstream analyses. Shannon (H) and Good’s coverage index were calculated at the OTU level using Mothur (Kozich et al., 2013). Sequence data from this study have been deposited to the NCBI Sequence Read Archive (SRA) under the accession numbers of PRJNA591295 and PRJNA591298 for bacterial and fungal communities, respectively.
Microbial Composition and Community Structure Assessment
Relative abundances were determined by dividing the number of sequences assigned to each category classified at the phylum level (phylum Proteobacteria was classified at class level) by the total number of sequences within each sample. Average proportions less than 1% were affiliated to category others. Non-metric multidimensional scaling (NMDS) (Faith et al., 1987) was generated in the R package vegan (Oksanen et al., 2007) via the metaMDS function to compare and visualize the microbial composition across samples. To quantitatively evaluate the impacts of fertilization treatments and aggregate-size fractions on bacterial and fungal community structures, a forward permutational multivariate analysis of variance (PERMANOVA; 999 permutations) (Anderson, 2001) was performed via the adonis function in the vegan package. Soil chemical properties (SOC, TN, C/N ratio) were fitted onto the NMDS ordinations by the envfit function.
Analysis of Microbial Co-occurrence Network and Community Functional Prediction
Microbial co-occurrence in each size fraction was examined by network analysis. To reduce network complexity, only the OTUs with more than five reads and presented in more than two-thirds samples were kept to generate Spearman rank correlation coefficient matrices using the WGCNA package (Langfelder and Horvath, 2012). Corresponding p values of the correlation coefficient matrices were adjusted into q values by Benjamini–Hochberg’s false discovery rate (FDR) in the fdrtool package to minimize false-positive rates. Co-occurrence networks were constructed based on correlation coefficient matrices with q < 0.01 or | ρ| > 0.6 (Barberan et al., 2012; Ma et al., 2016) in the igraph package (Csardi and Nepusz, 2006) and visualized using Gephi (version 0.9.2) (Bastian et al., 2009). Unconnected nodes along with loops that indicate OTUs correlated with themselves were, respectively, removed using the delete.vertices and simplify functions. The topological features used to describe network properties were assessed by following parameters using the corresponding functions in igraph (Newman, 2003, 2006). Specifically, the number of nodes denoted the number of OTUs with at least one correlation, and the number of edges denoted the number of connections among the nodes obtained, whereas the average degree indicated the average number of connections through each node. Average path length denoted the average network distance among the nodes, whereas the diameter denoted the longest distance among the nodes. Furthermore, clustering coefficient was the degree to which nodes in a network tended to cluster together. Finally, a parameter of modularity was used to represent the network structure indicating the strength of division of a network into modules. At last, the resulting networks were exported in GRAPHML format for further optimization and visualization in Gephi version 0.9.2 (Bastian et al., 2009). The network graphs were eventually generated using the Fruchterman-Reingold layout by Gephi and resized according to the parameter of diameter by Adobe Illustrator.
Soil bacterial functional profile was predicted using PICRUSt (Phylogenetic Investigation of Communities by Reconstruction of Unobserved States) from the 16S rRNA marker gene sequence data (Langille et al., 2013). Such work was based on the databases of Cluster of Orthologous Groups of proteins (COGs) (Tatusov et al., 2000) and Kyoto Encyclopedia of Genes and Genomes (KEGG) (Kanehisa and Goto, 2000) to infer gene content and then to predict the bacterial metagenome functional content annotated into COG and KEGG pathway categories, respectively, whereas an open annotation tool, FUNGuild (Fungi functional guild), was used to taxonomically parse fungal community OTUs into ecological functional guild with the python script Guilds v1.1, which run against the FUNGuild database (Nguyen et al., 2016). The annotations with confidence ranking of “Highly Probable” and “Probable” were used for the prediction of the community functional guilds. Differences of the functional groups between each size fraction of aggregate samples and all the other samples were compared with two-sided Welch t test with 0.95 confidence interval (CI) and Benjamini–Hochberg FDR for multiple tests and then plotted with features at q < 0.05 by the software STAMP 2.1.3 (Parks et al., 2014; Douglas et al., 2018).
Data Processing and Statistics
Data processing was performed with Microsoft Excel 2016. All data of a sample were expressed as mean plus/minus a standard deviation (n = 3). Statistical analyses were conducted by GraphPad Prism 7 and SPSS 24. Figures were plotted with Excel 2016 and R 3.5.0. Differences among different fertilization treatments and aggregate-size fractions were analyzed with one-way or two-way analysis of variance (ANOVA). Subsequently, multiple comparisons were conducted using Tukey test to determine the significance defined at p < 0.05.
Results
Basic Properties of Aggregate-Size Fractions
Compared with NF, contents of SOC and TN were all significantly increased, whereas pH decreased, although no change was observed in C/N ratio under the long-term application of fertilizers (Table 1). Meanwhile, MBC was significantly increased under CFS but MBN was unchanged with the treatments. The separation protocol yielded an overall recovery in the range of 94.23–99.45%. Across the samples, water-stable aggregates were dominated by F-MicA (32.3–38.2%) and MacA (23.0–31.5%), followed by MicA (19.5–23.1%) and L-MacA (9.1–12.0%), with the least contribution by F-Clay (4.9–7.5%) (Table 2). Except for the F-Clay fraction, the mass proportion of an aggregate-size fraction varied greatly across the treatments. With fertilization compared with no fertilization, the mass proportion of MacA was significantly increased by 19.2–37.3%, but that of F-MicA was reduced by 14.0–15.5%, and that of the other fractions was insignificantly unchanged. Consequently, the mean weight diameter (MWD) of water-stable soil aggregates (Table 1) was increased from 534.8 μm under NF to 577.5–621.5 μm by 8.0–16.2% under fertilizer treatments. Thus, the mass proportion of soil aggregate fractions varied greatly with size but significantly affected, mainly for MacA and F-MicA fractions, with fertilization practice.
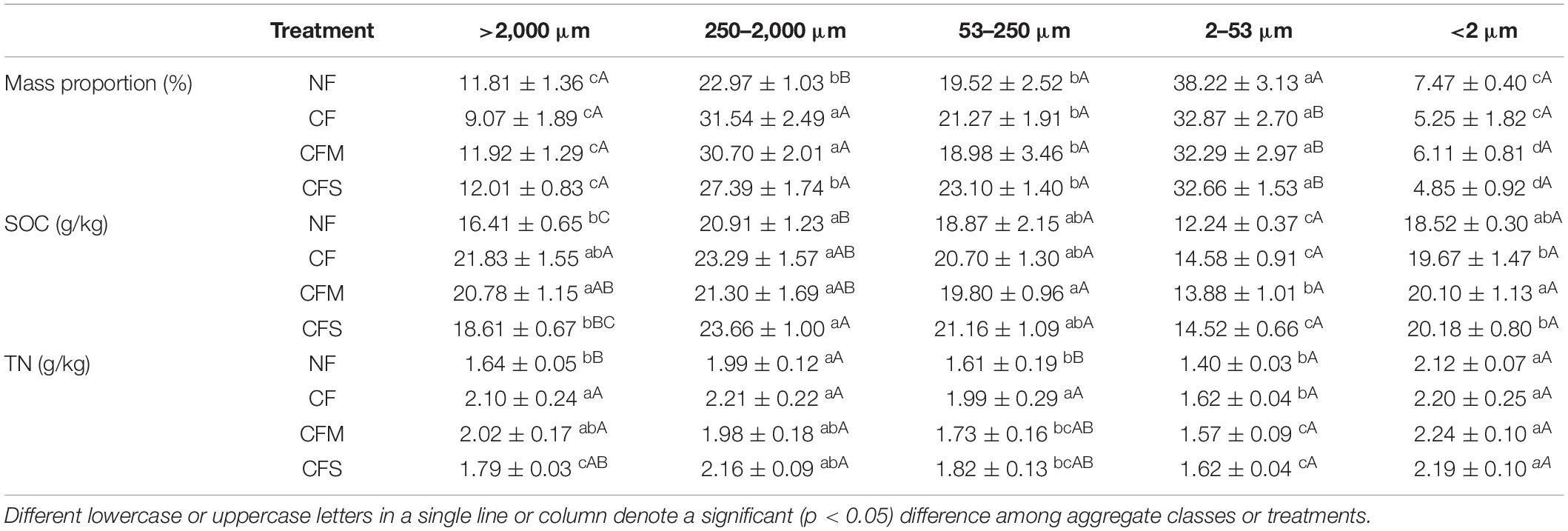
Table 2. Mass proportion and basic properties (mean ± SD, n = 3) of aggregate-size fractions under different fertilization treatments.
The contents of SOC and total N of soil aggregates varied moderately (CV 12–20%) across the size fractions. Generally, the SOC content peaked in MacA (20.91–23.66 g kg–1) but depleted in F-MicA (12.24–14.58 g kg–1) with no significant difference among the fractions of MicA, L-MacA and F-Clay. The contents of TN followed a similar trend, although enriched in the F-Clay fraction. However, the change in these contents with the treatments was insignificant across the size fractions of MicA, F-MicA and F-Clay despite marked (CV > 10%) changes found only in L-MacA. Compared with NF, the SOC content of MacA was increased by 13.2% under CFS, and that of L-MacA by 33.0% under CF and by 26.6% under CFM. Unlike the SOC content, the total N (TN) content of L-MacA was increased by 9.1% under CFS and by 23.2–28.0% under CFM and CF, whereas that of MicA was increased by 22.3% under CF only. Consequently, the C/N ratio showed a slight (CV ∼10%) variation across the size fractions but a weak variation with fertilization treatments. Generally, the C/N ratio was highest (10.4–11.7) in MicA, followed by MacA and L-MacA (10.0–10.9), and lowest in the F-MicA and F-Clay fractions (8.7–9.2). Under CF, particularly, the C/N ratio was significantly lower in MicA compared with other fertilizer applications (Table 2). Overall, the aggregate size factor caused a greater contribution (by 62.6–93.8%) than the treatment factor with a slight contribution (by 0.0–12.0%) to the overall variation of aggregate properties, as shown in Supplementary Table 1.
Microbial Population Abundance and Community Structure
The population sizes of both bacteria and fungi, quantified by 16S and 18S rRNA gene copy numbers, respectively (Table 3), demonstrated a broad range of variation across aggregate-size fractions (CV 51–98%), decreasing generally with the sizes, and among fertilizer treatments (CV 3–123%) (Table 3). In a range of ≤0.5 × 109 to 5.10 × 109 copies g–1 dw and of ≤0.2 × 107 to 10.2 × 107 copies g–1 dw, the abundance of both bacterial 16S and fungal 18S rRNA gene was lowest in F-Clay but highest in MacA (for bacteria) or in L-MacA (for fungi). Despite no change was found among treatments in L-MacA, MacA, and MicA, both bacterial 16S and fungal 18S rRNA gene copy numbers were highly increased by fertilizer application in F-MicA (2- to 3-fold for bacteria, 4- to 5-fold for fungi), while increased under CF but decreased under CFM and CFS by folds in F-Clay (Table 3). In addition, the B/F ratio, ranging from 45.97 to 611.23, was lowest in L-MacA or MacA and increased with the decreasing size of the aggregate fractions. Meanwhile, the ratio was seen to decrease under fertilizer application only in F-MicA fraction, without treatment induced changes in those >53-μm fractions. Based on the two-way ANOVA, the aggregate size factor exerted a greater effect (p < 0.0001) than the treatment factor (p < 0.01), on the bacterial abundance, fungal abundance, and B/F ratio, which were, respectively, contributed by 83.3, 73.5, and 64.1% by size factor, whereas 4.0, 6.5, and 7.4% by treatment factor to the total variation (Supplementary Table 2).
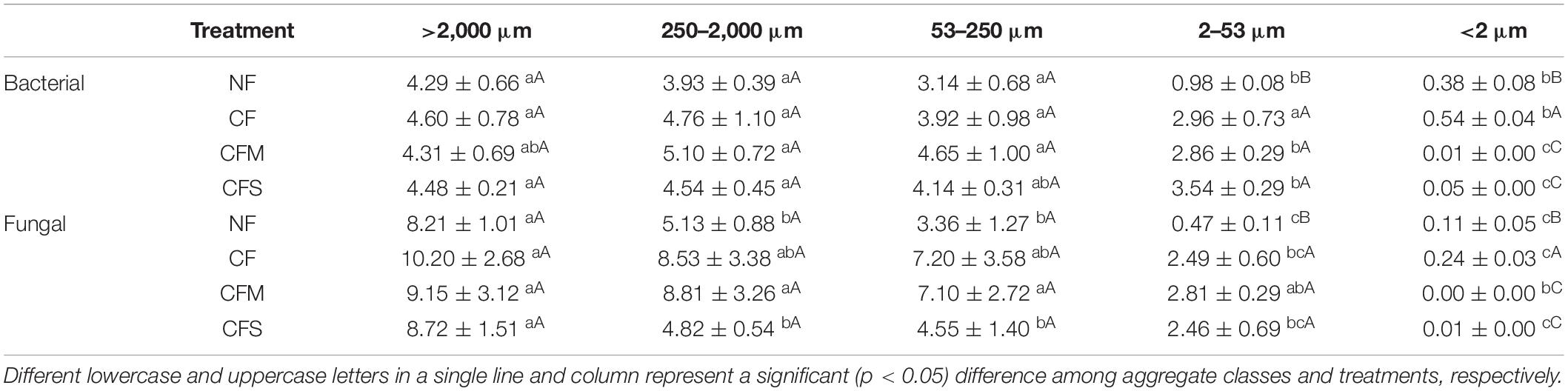
Table 3. Bacterial 16S (109 copies g–1 dw) and fungal 18S (107 copies g–1 dw) gene abundances (mean ± SD, n = 3) of aggregate-size fractions under different treatments.
Moreover, the Shannon diversity indices were generally greater for bacteria population than fungi for a given aggregate fraction (Figure 1). Regardless of fertilization treatment, the Shannon indices of fungi of an aggregate fraction showed an insignificantly increasing trend with decreasing size, whereas that of bacterial was similar across the size fractions. In addition, the diversity index for both bacteria and fungi was lower under CFM compared with the other fertilization treatments, across the size fractions (Figure 1). Overall, microbial community diversity among aggregate fractions was rather impacted by fertilization than by aggregates size, and there was no significant interaction between size and treatment (Supplementary Table 3).
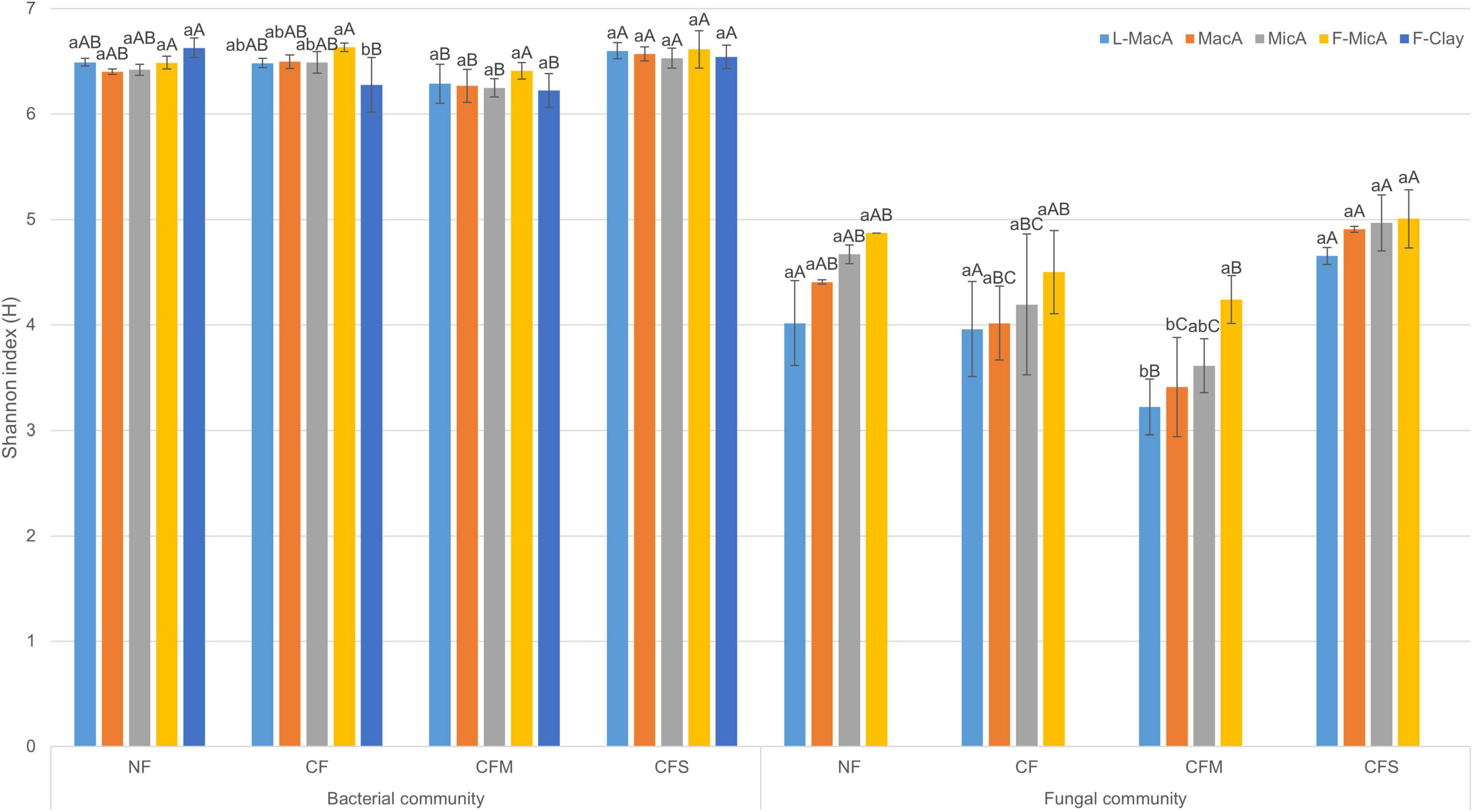
Figure 1. Shannon index (H) (mean ± SD, n = 3) of bacterial and fungal communities in aggregate-size fractions under treatments. Different lowercase and uppercase letters represent significant (p < 0.05) difference among aggregate-size fractions and treatments, respectively. NF, no fertilizer applied; CF, compound inorganic fertilizer; CFM, inorganic fertilizer combined with swine manure; CFS, inorganic fertilizer combined with residue return. L-MacA, >2,000 μm; MacA, 2,000–250 μm; MicA, 250–53 μm; F-MicA, 53–2 μm; F-Clay, <2 μm.
The bacterial population detected in soil aggregates across size fractions was dominated by Chloroflexi (3.5–30.8%), Betaproteobacteria (11.2–20.6%), Acidobacteria (12.0–17.9%), and Deltaproteobacteria (6.3–10.6%) (Figure 2A). For bacteria assembly, the population of Chloroflexi was smaller, but that of gamma-proteobacteria was larger in the F-Clay fraction than in the MicA and MacA fractions. For fertilizer treatment, the population of Chloroflexi was larger, and that of Betaproteobacteria was smaller under the NF and CFS treatments than under the CF and CFM treatments (Figure 2A). The fungal community was predominated by Ascomycota (39.8–64.9%) and Basidiomycota (35.6–59.7%) across the aggregate samples (Figure 2B). Hereby, the relative abundance of fungal communities in a fraction was much variable for the larger standard deviation compared with bacterial communities. Specifically, Basidiomycota in all the aggregate fractions, except for the F-Clay fraction, showed a significantly higher relative abundance under CF than under the other treatments. Differently, the abundance of Ascomycota was significantly lower in L-MacA under CF and CFM, and in F-MicA under CFM, respectively, compared with under NF or CFS. However, the relative abundance of Zygomycota, a minor fungal phylum, appeared several folds higher in L-MacA and F-MicA under CFM over the other fertilizer treatments (Figure 2B). Besides, there was a generally lower relative abundance of unspecified fungal phyla under fertilizer applications compared with NF.
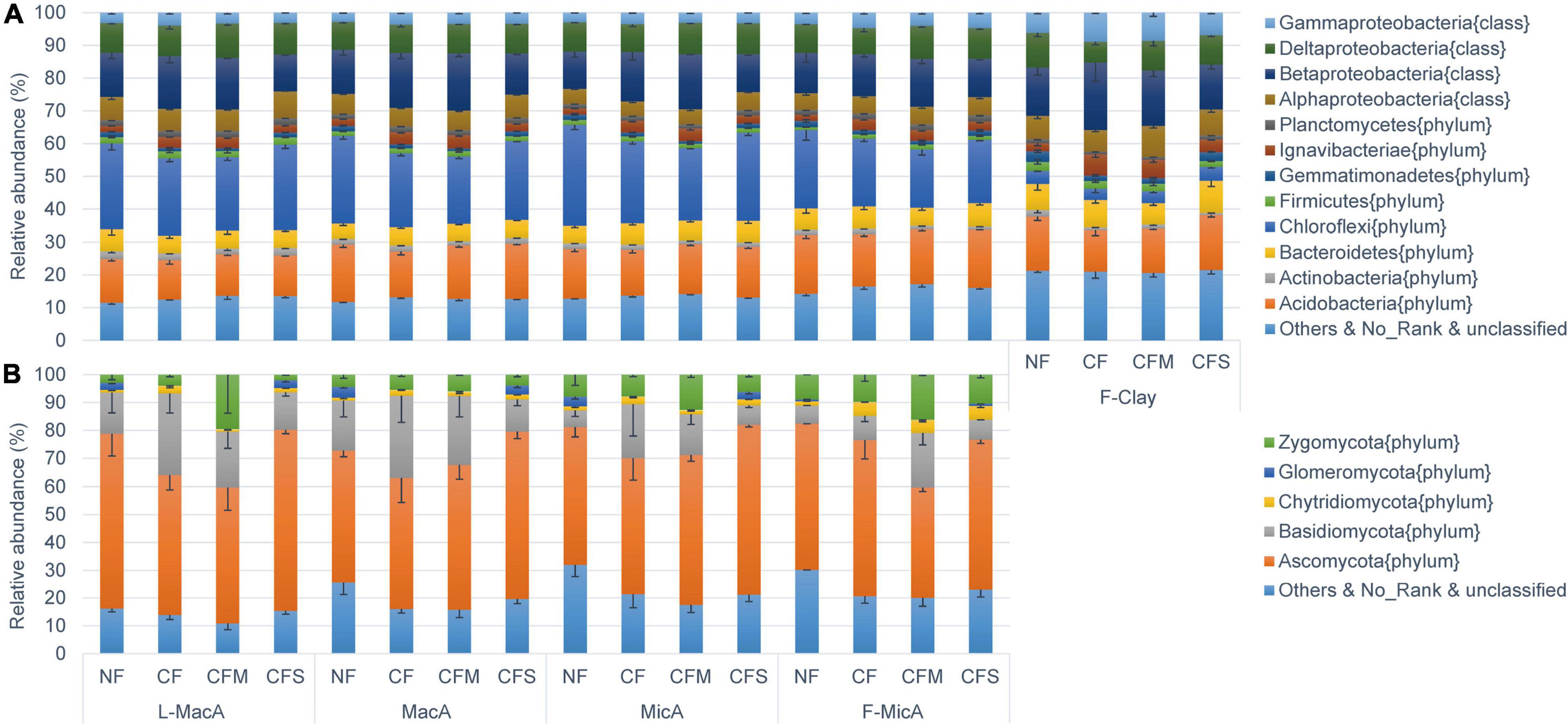
Figure 2. Average relative abundance distribution of V4–V5 16S rDNA sequence classified at phylum level (class for Proteobacteria) for bacteria (A) and ITS1-ITS2 sequence classified at phylum level for fungi (B). Error bars represent the standard errors. Average proportions of showed bacterial phyla (class for Proteobacteria) and fungal phyla all exceed 1%. NF, no fertilizer applied; CF, compound inorganic fertilizer; CFM, inorganic fertilizer combined with swine manure; CFS, inorganic fertilizer combined with residue return. L-MacA, >2,000 μm; MacA, 2,000–250 μm; MicA, 250–53 μm; F-MicA, 53–2 μm; F-Clay, < 2 μm.
Based on NMDS, the bacterial community assembly of aggregate-size fractions was clearly influenced by both soil aggregate size and fertilization treatment. Especially, the bacterial communities in F-MicA and F-Clay were distinct from larger-sized aggregate fractions hardly altered with the treatments (Figure 3A). Moreover, the bacterial community structures of the L-MacA, MacA, and MicA fractions were similar in NMDS1, whereas F-MicA and/or F-Clay housed bacteria communities whose structures were similar to each other than to those in larger aggregates (Figure 3A). However, the bacterial community structures were altered with fertilization by NMDS2. Thereby the similarity of bacterial community structure with the treatments was in an order of NF, CFS, CF, and CFM. In contrast, size factor showed minor influence on fungal community structures of soil aggregates, relative to fertilization treatment (Figure 3B). The fungal community structures were altered with fertilization by NMDS1, whereas the treatment effect was greater under CF and CFM than under CFS, compared with NF. Meanwhile, the fungal community structures were altered more or less with the size factor by NMSD2, showing the MicA and F-MicA fractions were relatively separated from the L-MacA and MacA fractions (Figure 3B). Based on PERMANOVA, the bacterial community structure was significantly attributed to C/N ratio (p < 0.001), SOC (p < 0.05), and TN (p < 0.05), whereas the fungal community similarity was statistically correlated to SOC (p < 0.01) (Figure 3).
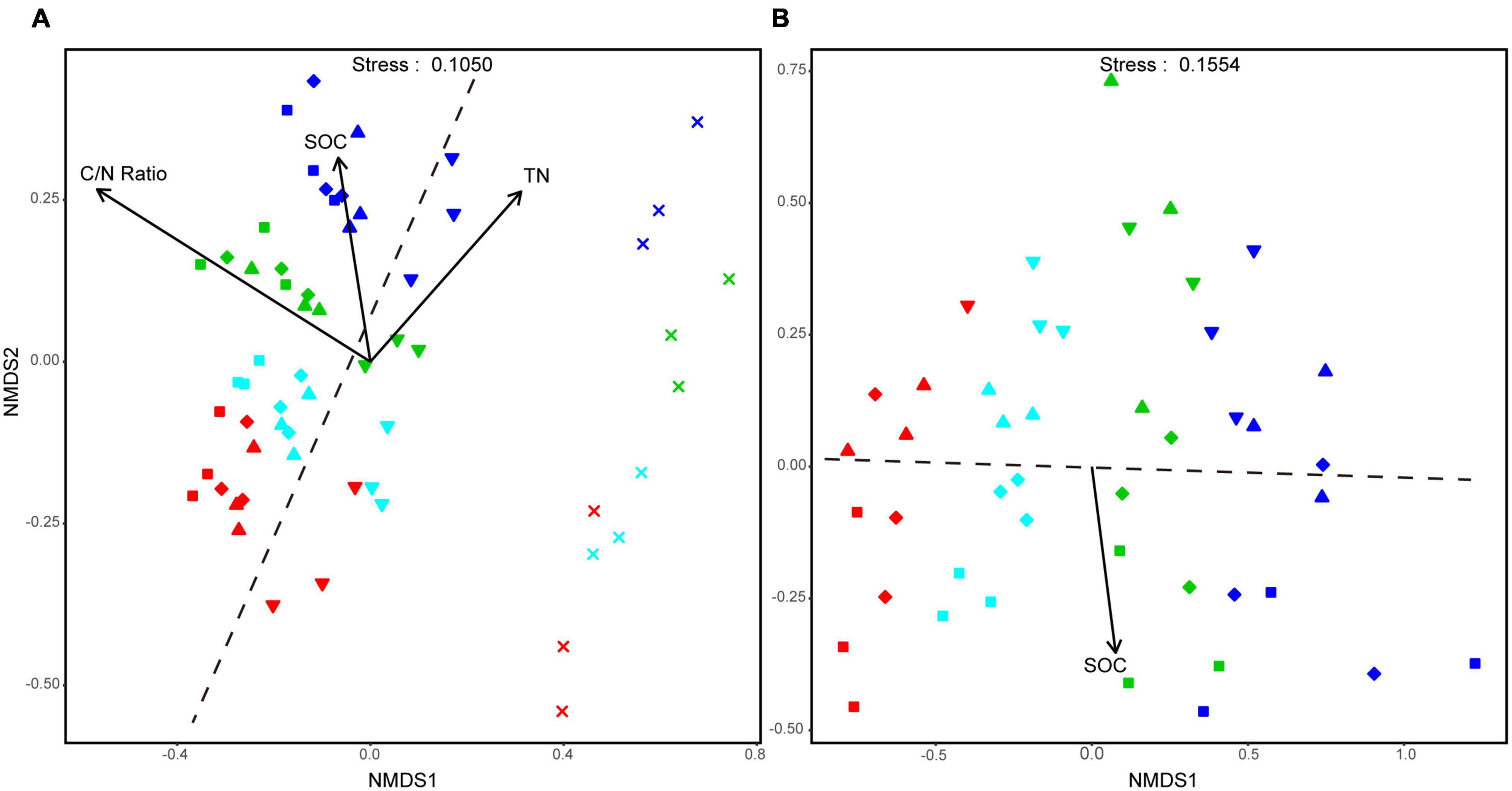
Figure 3. Non-metric multidimensional scaling (NMDS) plots of all the detected samples at OTU level of the aggregate-size fractions, respectively, for the bacterial (A) and fungal community (B). Symbols denote size fractions as follows: ■, >2,000 μm; ◆, 2,000–250 μm; ▲, 250–53 μm; ▼, 53–2 μm; ×, <2 μm. Colors denote treatments as follows: red, NF; green, CF; blue, CFM; black, CFS. Dotted lines, respectively, indicate the boundary between MicA and F-MicA in A while between MacA and MicA in panel B.
Microbial Co-occurrence Networks Among Aggregate-Size Fractions
Both SOC and microbial abundance of soil aggregate fractions were shaped predominantly by size (see Basic properties of aggregate-size fractions and Microbial population abundance and community structure), thereby the microbial OTUs of a single fraction of individual samples under different treatments were pooled as a single sample for analysis of co-occurrence networks. After filtering all the OTU data, in total 639–763 bacterial OTUs and 229–305 fungal OTUs were obtained, respectively, for network construction of bacterial and fungal community for the aggregate-size fractions, based on the significant (p < 0.01) and strong (ρ > 0.6 or ρ < −0.6) correlations as per Barberan et al. (2012). The bacterial co-occurrence networks were dominated by OTUs affiliated with the phyla of Proteobacteria, Chloroflexi, Acidobacteria, Bacteroidetes, and Ignavibacteriae (Figure 4), whereas the fungal networks were centered around Ascomycota, Chytridiomycota, Glomeromycota, Basidiomycota, and Zygomycota (Figure 4). The bacterial–fungal co-occurrence networks were attributed to 52.9–69.2% of bacterial and 30.8–47.1% of fungal communities across the size fractions (Figure 4). Generally, the microbial co-occurrence networks exhibited disparate patterns among size fractions, and both the positive and negative co-occurrence networks were much more complicated for the bacterial communities than the fungal communities (Figure 4). Both positive and negative correlations in bacterial, fungal, and bacterial–fungal networks were observed in all the aggregate fractions other than F-Clay with fungal and bacterial–fungal networks and significantly in MicA, MacA and L-MacA. As indicated by the edge thickness, the positive networking was relatively stronger than negative networking across the size fractions, particularly in MacA and L-MacA. The numbers of nodes, edges, and the average degree of the networks were all higher in MacA and MicA, compared with other fractions (Supplementary Tables 6, 7). However, more intricate networks led to lower modularity in MacA and MicA with a similar number of clusters to the other fractions. For MacA and MicA compared with the other fractions, the average path length of bacterial network was lower, whereas that of fungal and bacterial–fungal networks was higher. The opposite trend of density and clustering coefficient across size fractions showed more compact bacterial networks but looser fungal and bacterial–fungal networks in MacA and MicA compared with the other size fractions. Overall, microbial communities exerted higher networking performance in MacA than MicA and for bacterial than fungal and bacterial–fungal networks.
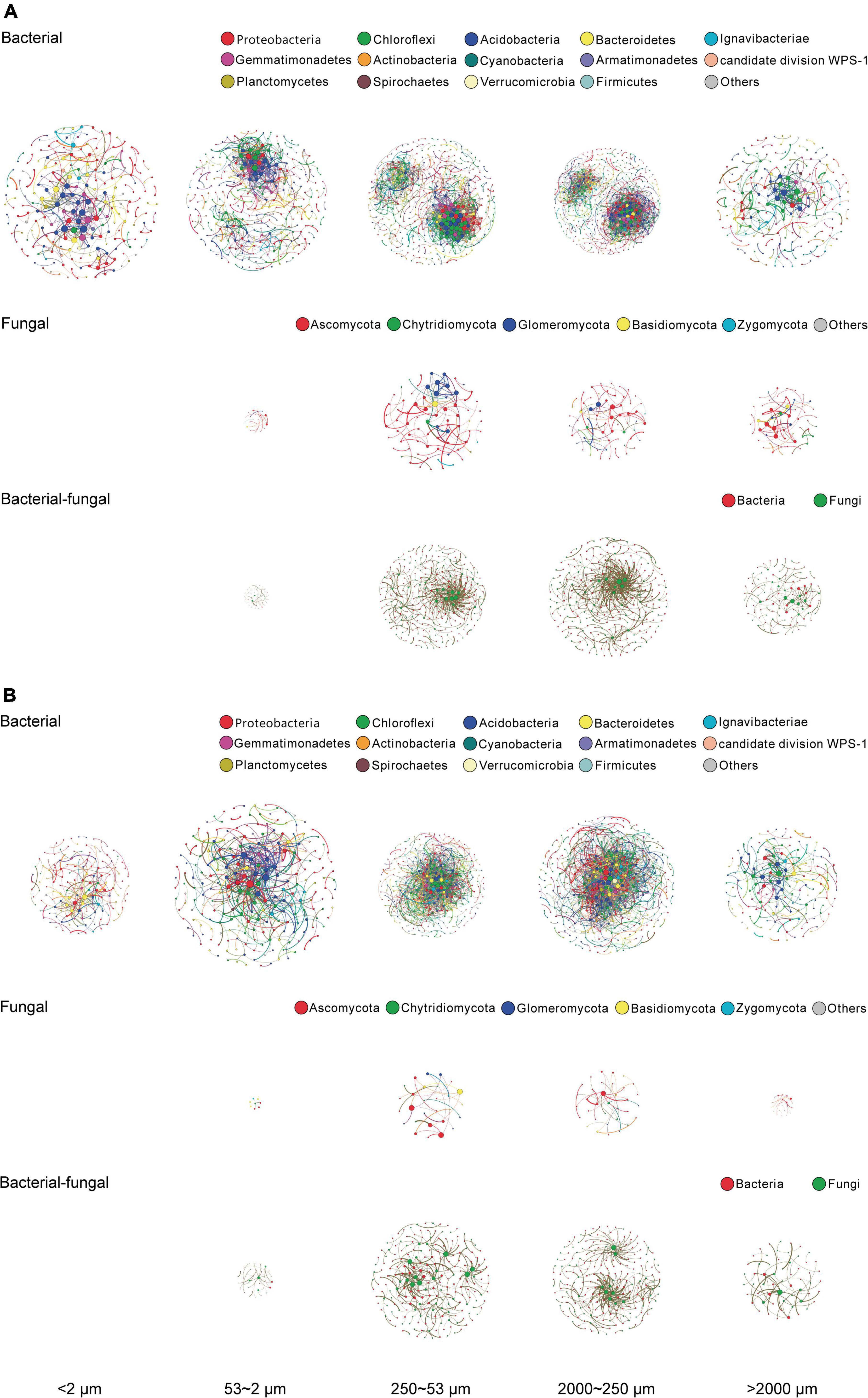
Figure 4. Positive (A) and negative (B) co-occurrence networks of bacterial, fungal, and bacterial–fungal consortia of the size fractions of aggregates based on Spearman rank correlation coefficient matrices. Colored nodes indicate OTUs represented by phyla. An edge stands for a strong positive (Spearman ρ > 0.6) and significant (p < 0.01) correlation between the two nodes. For each network, the size of each node is proportional to the number of edges (degree). The size of a network for a size fraction is proportional to the size of the corresponding networking scope. The thickness of each edge is proportional to the correlation coefficient.
Microbial Community Functional Profiles Among Aggregate-Size Fractions
Summarized from the statistical results in Supplementary Figures 1, 2, the difference in the functional profiles of COGs and KEGG metabolic pathways for bacterial communities among the aggregate-size fractions is shown in Tables 4, 5, respectively. L-MacA, MacA, and MicA all possessed more bacterial populations with functional genes encoding enzymes involving transport and metabolism of inorganic ion and various types of carbohydrate, transport proteins, and RNA processing and modification, but were less abundant of genes related to bacterial chemotaxis, cell motility, flagellar assembly, protein biosynthesis, signal transduction, intracellular trafficking, secretion and vesicular transport, DNA repair and recombination, and so on. However, F-MicA possessed a higher abundance of functional genes encoding enzymes involving defense mechanisms and metabolism of certain sugars and amino acids, while possessed a lower abundance of genes with functions of inorganic ion transport and metabolism and cell chemotaxis, and so on. Bacterial populations with functional genes associated with bacterial chemotaxis, cell motility, flagellar assembly, and protein biogenesis were predominantly detected in F-Clay. Meanwhile, this fraction possessed a lower abundance of genes functioning in transport and metabolism of carbohydrate and amino acid, defense mechanisms, RNA processing and modification, transport proteins, and so on.
For fungal communities across aggregate-size fractions, F-MicA contained more functional fungal guilds associated with fungal parasite, litter saprotroph, animal pathogen, endophyte, and epiphyte but less of plant pathogen, arbuscular mycorrhizal, dung saprotroph, and wood saprotroph, whereas larger MacA tended to contain less fungal functional guilds of fungal parasite and litter saprotroph (Table 6 and Supplementary Figure 3).
Discussion
Microbial Abundance in Relation to Soil Organic Matter in MacA
In this study, variation of carbon and microbial abundance among soil aggregate fractions were shaped predominantly by size, although microbial diversity was less variable across the size fractions of aggregates. This is in general agreement with many previous studies of agricultural soils (Whalen and Chang, 2002; Li et al., 2007; Zhang et al., 2007; Hao et al., 2008; Dorodnikov et al., 2009; Lagomarsino et al., 2012). Unlike dryland soils containing significant amounts of coarse sand-sized MacA (Whalen and Chang, 2002; Dorodnikov et al., 2009), soils in this study derived from clayed sediments and were dominated by silt-sized MicA and fine-sand-sized MacA (Table 2). This is attributed to potential stabilization of MicA with water dispersion under long-term hydroagric tillage (Denef et al., 2001; Zhang et al., 2016; Sun et al., 2017). Different to upland soils, where SOC content was enhanced only in fractions with size >63 μm (Marx et al., 2005), consistently high content of SOC was observed in MacA in this study. Similar patterns have been reported in Chinese rice paddies (Li et al., 2007; Zhang et al., 2015; Liu et al., 2016; Lu et al., 2020). Bacterial and fungal populations were more abundant in MacA and L-MacA, compared with those in F-Clay or F-MicA, which is in agreement with the reported microbial populations of rice soils (Zhang et al., 2007; Liu et al., 2016). Therefore, fungal community can be promoted in larger-sized MacA due to physical protection of relatively younger organic matter derived by plant roots with a high C/N ratio (Six et al., 2000; Lützow et al., 2006; Grandy and Robertson, 2007).
Interestingly, when scaled by organic carbon content of aggregate fractions (Table 2), bacterial and fungal abundances were positively correlated with the size of aggregate fractions (Figure 5A), indicating a potential linkage between soil microbial biomass and organic carbon in MacA. With prolonged rice cultivation, wetland converted rice paddy can enhance both the microbial abundance and activity concerning organic carbon accumulation (Jin et al., 2012). In a previous study (Liu et al., 2016), soil microbial abundance and activity were promoted by mainly POM accumulation in MacA, which is triggered via SOC accumulation under rice paddy management over the centuries. Consequently, as argued by Ranjard et al. (2000), up to 90% soil bacteria are associated with MacA in soil. On the other hand, MicA and F-Clay particles have been well known for their enriched mineral-associated organic matter (Cotrufo et al., 2013), which are inaccessible to microbes, particularly fungi. Considering the mass distribution, SOC, and microbial abundance by size fractions of soil aggregates, the relative proportion by MacA of > 250 μm in size was significantly higher than its mass proportion for the abiotic property of SOC and total N but greatly higher for the biotic property of bacterial and fungal abundance (Figure 5B).
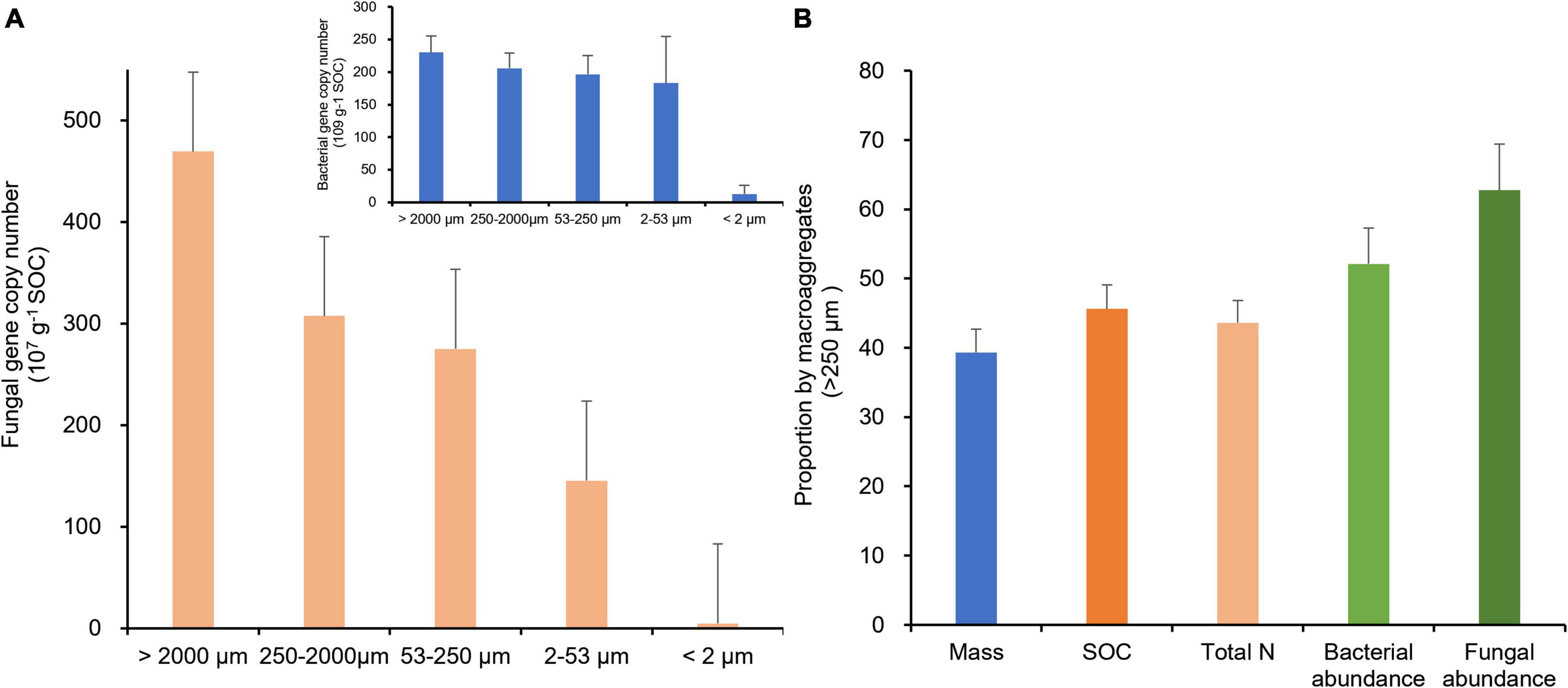
Figure 5. Gene abundance of fungi (A, 107 copies g–1 SOC) and bacterial (embedded in A, 109 copies g–1 SOC) in different-size fractions and proportion (%) possessed by macroaggregates > 250 μm (B), of water-stable aggregates of the rice paddy.
Thus, we see the existence of biotic “hotspot” for MacA at the microscale of soil matrix, first proposed by Bundt et al. (2001) for enriched younger OM in pores within MacA, where active biogeochemical reactions are demonstrated as a result of abundant extracellular enzymes (Sinsabaugh et al., 2008; Kuzyakov and Blagodatskaya, 2015; Li et al., 2019c). Therefore, the linkage between such biotic hotspots and microbial community composition and functioning will be crucial for soil and ecosystem health.
Microbial Community Composition, Networked Interaction, and Functional Association Enriched in MacA
In the study, the Shannon diversity indices of both bacteria and fungi showed no consistent trend across the aggregate-size fractions or between bacterial and fungal communities, although relatively higher in F-MicA (Table 5). Upton et al. (2019) recently also reported higher Shannon diversity of fungi in MicA than in L-MacA in different bioenergy management systems, although it was subjected to farming management in agricultural soils (Liao et al., 2018; Lu et al., 2018; Li and Yang, 2019). For bacterial community diversity of agricultural soil nonetheless, MicA could be either higher (Zhang et al., 2013; Bach et al., 2018) or lower (Lupwayi et al., 2001). Hereby, the dominant bacterial phyla were generally consistent, whereas fungal phylum composition varied across the size fractions except for the F-Clay fraction. However, based on PERMANOVA (Supplementary Table 4), size of aggregates exerted a significant (p < 0.001) but independent impact on both the bacterial and fungal communities of aggregate fractions (Figure 3).
While significantly related to SOC, TN, and C/N ratio (Supplementary Table 5), community composition structures of bacteria in the F-MicA and F-Clay fractions were clearly separated from the L-MacA, MacA, and MicA fractions by NMDS1, whereas that of fungi in L-MacA and MacA were separated by NMDS2, although divergent by NMDS1. In another long-term fertilization study, Li et al. (2019a) also reported a distinct change in fungal and bacterial communities, most closely related to soil carbon and nitrogen contents, in aggregates due to fertilization. This could again suggest that microbial community structure, particularly of fungi, was evidently different in MacA from MicA, particularly in F-MicA, as a dominant pool of soil (Table 2). Again, in this study, the interaction patterns (Figure 4) of bacterial and fungal OTUs, and across bacterial and fungal OTUs, were variable among aggregate-size fractions. While the trends of the positive interactions were roughly similar to the negative interactions among the aggregate-size fractions, bacterial interactions in both positive and negative co-occurrence networks were much more complex and intensive than fungal interactions in each single size fraction. Clearly, all the bacterial, fungal, and bacterial–fungal interactions in MacA (and MicA) were remarkably stronger than in other size fractions, although weak but divergent interactions were detected in the F-MicA and F-Clay fractions. Moreover, the level of co-occurrence network complexity was highest for MacA compared with other fractions. The bacterial communities showed a more compact topology during assembly in MacA and MicA than other fractions, whereas fungal communities showed a looser network, indicating bacterial communities assembled more non-randomly in MacA and MicA whereas fungal communities assembled more randomly (Jiang et al., 2018). Considering that positive correlations indicate synergistic or symbiotic interaction, whereas negative correlations indicate competitive or predatorial interaction between species (Barberan et al., 2012; Faust and Raes, 2012; Lupatini et al., 2014), microbial interactive activity was enhanced in MacA (and MicA), whereas bacterial activity was much higher than fugal activity. Seemingly, the relationships among microbial individuals and the patterns of microbial interaction networks were quite divergent across aggregate-size fractions and were regulated not only by the microbial abundance (Figure 5) and community structure (Figure 4) but also by the specific microenvironment of different soil aggregates, which were raised as concurrent evolutionary incubators for microbial community by Rillig et al. (2017). Being shaped by size of aggregate fractions, the bacterial, fungal, and bacterial–fungal interaction patterns are indeed shifted with macroaggregation. This indicates enhanced microbial community co-occurrence and interaction in MacA rather than in MicA.
As a result of micropore structure of soil with different microenvironments (Tecon and Or, 2017; Juyal et al., 2019; Liang et al., 2019), microbes can interact with each other differently at the microscale (Raynaud and Nunan, 2014). Accordingly, an appropriate distribution of soil pore spaces can regulate the microbial dispersion and functional distribution and even facilitate the interaction within microbiota (Ebrahimi and Or, 2014; Negassa et al., 2015; Juyal et al., 2018; Tecon et al., 2018). Obviously, MicA are mostly occlusive as closely associated with mineral surface and thus can isolate associated microorganisms by spatial exclusion (Six et al., 2000; Lützow et al., 2006; Six and Paustian, 2014). However, the formation of MacA, with MicA occluded and particular organic matter physically protected, increases the complexity in spatial arrangement of soil pores and thus the habitat heterogeneity (Baldock and Skjemstad, 2000). Importantly, microbial interactions, synergistic or competitive, between phyla/species can mediate metabolic functions, affecting the geochemical cycling processes (Wilpiszeski et al., 2019). While Lehmann et al. (2017) noted microbial species interactions across major taxonomic groups in relation to soil aggregation, this study suggests a microscale hotspot of MacA with enhanced microbial community networking and interactions. This further supports our previous finding of enhanced soil enzyme activities in MacA as biochemically functional hotspots in soil matrix (Li et al., 2019c).
Soil aggregation can stabilize microbial composition and strengthen microbial interaction and likely alter microbial community function (Wilpiszeski et al., 2019). In this study, the predictive microbial functional profiles (Tables 5, 6) were altered with the soil aggregate-size fractions of the paddy. Bacterial communities within larger-sized aggregates possessed intense activities in carbon and nitrogen cycling but weak activities involving bacterial migration, DNA metabolism, and biochemical processes for surviving. However, bacterial communities within the smaller-sized fractions, especially F-Clay, were relatively more active in cell motility, DNA metabolism, and intracellular biochemical processes potential for surviving, but less active in assimilation of carbon and nitrogen sources. MacA could be more likely active in functioning of C and N cycling, for ecosystem performance, but those in finer-sized aggregates tended to perform their activity for surviving. This could be related to higher microbial metabolic activities, particularly of C-degrading enzyme activities, found in MacA (Kuzyakov and Blagodatskaya, 2015; Li et al., 2019c) and to the resource limitation in F-MicA due to mineral association and limited pores (Baldock and Skjemstad, 2000), whereas the FUNGuild result (Table 6) indicates that L-MacA tended to be less abundant in guilds of fungal parasite and litter saprotroph than the other fractions except for F-MicA, which was lowest in fungi abundance, but contained more guilds related to disease or fungal parasitic but less of mycorrhizal and dung or saprotrophic fungi for OM decay. Lu et al. (2020) also noted a decrease in some fungal parasite guilds with biochar promoting macroaggregation in a rice soil in an adjacent area. Therefore, it suggests a better microenvironment for soil health and carbon cycling in MacA than smaller-sized aggregates.
Microorganisms adapted to rapid growth depending on easily assimilable substrate and slow growth using relatively recalcitrant substrate are defined, respectively, as r-strategists and K-strategists (Fontaine et al., 2003). r-Strategy is associated with a greater abundance of genes related to regulation and cell signaling, whereas K-strategy is rich in motility and chemotaxis-related genes (Song et al., 2017). Accordingly, the functional profiling here can be translated as that the aggregate fractions in larger size tended to possess both abundant r-strategists and K-strategists. whereas those in smaller size tended to be dominated by K-strategists. In F-MicA and F-Clay particles, diffusion transport of microbial signal molecules can be severely limited at the micrometer scale (Vincent et al., 2010). Instead, larger-sized MacA, MacA, and, to some extent, MicA can provide a balanced combination of substrate availability, diffusion passages, and vicinity to other microbes, allowing active microbial growing and functioning (Nannipieri et al., 2003; Ekschmitt et al., 2005) in addition to microbes with K-strategy in (fine) MicA survived within MacA. Considering their trophic type, microorganisms can also be divided into oligotrophs and copiotrophs associated with, respectively, available and limited resources (Koch, 2001). A copiotrophic strategy is associated with a greater abundance of genes related to cell division and cell cycle, whereas an oligotrophic strategy has a greater abundance of genes related to carbohydrate metabolism and virulence, disease, and defense (Song et al., 2017). Indeed, larger-sized MacA possessed less fungal guilds of fungal parasite and litter saprotroph but higher relative abundance of Basidiomycetes (Figure 2B). As pointed out by Davinic et al. (2012), copiotrophs outcompeted slower growing oligotrophs in L-MacA for abundant labile organic matter in MacA. This is coincident with the higher abundance of functions associated with cell division and cell cycle for rapid growth observed in MacA (Table 5). All these further support MacA as biogeochemically functional hotspots, which was raised in previous studies (Kuzyakov and Blagodatskaya, 2015; Li et al., 2019c).
Overall, this study suggests a coevolution of SOC accumulation and soil bioactivity in rice paddy through the promotion of microbial abundance and activity with the enhancement of physically protected OC in larger-sized MacA under fertilization (Pan et al., 2014, 2015). Likewise, King et al. (2019) commented that reduced MacA turnover can promote SOC accumulation via the stabilization of C into occluded fractions. More importantly, this study further demonstrates the sand-sized MacA as micro-hotspots (Kuzyakov and Blagodatskaya, 2015) with enriched abundance in relation to SOC accumulation and networked interactions for functioning in carbon cycling and also possibly for soil health (Lucas et al., 2014; Vogel et al., 2014). Yet, the linkage of MacA dynamics to plant growth performance and ecosystem health deserves further field studies.
Microbial Abundance, Community Composition, and Diversity Affected by Fertilization Rather in MacA
In this study, the total variation of microbial abundance (Table 4) and community structure of soil aggregates by NMDS analysis (Figure 3) was shaped by size with a minor impact by fertilization treatment. Using a sonication-wet sieving protocol, Sessitsch et al. (2001) first noted that bacterial populations and community structure were affected rather by the particle size fraction than by the very contrasting organic amendments, in a long-term field experiment from Sweden. Using an “optimal moisture” approach of aggregate isolation, Bach et al. (2018) reported that bacterial and fungal community structure and diversity varied among soil aggregates, regardless of land management of prairie soils. Using a wet-sieving protocol like this study, Davinic et al. (2012) addressed distinct microbial community abundance and structure related to carbon composition between MacA and MicA other than among types of agroecosystems.
However, this study did show microbial community changes among aggregates fractions rather impacted by fertilization than by aggregates size, being generally lower in diversity under CFM compared with NF (Table 5, detailed in Supplementary Table 3). Particularly, fungal diversity exerted clear change with fertilization despite of a generally increasing trend with decreasing size of aggregate fractions. Compared with NF, there was a significant decrease under CFM, an insignificant decrease under CF, and an insignificant increase under CFS, in fungal diversity of aggregates in size larger than 53 μm (Table 5). This decrease in fungal diversity in these larger-sized aggregates under CFM and CF could be attributed to the sensitivity of fungi to heavy metal accumulation of Pb and Cu, particularly in the available pool (Li et al., 2003). A decline in culturable fungal population size and in the ratio of fungal to bacterial PLFAs was observed consistently across the sites of metal contaminated rice paddies across South China (Liu et al., 2012). Again, there was an evident change with treatments in the fungal community composition of MacA compared with MicA in size < 250 μm (Figure 1). In detail, the relative abundance of Ascomycota was decreased in L-MacA, whereas that of Basidiomycota and Zygomycota increased, and that of Glomeromycota and unspecified fungal communities markedly decreased both in L-MacA and MacA, under CF and CFM, as compared with NF. In contrast to this study, Yan et al. (2018) reported a decreased relative abundance of Basidiomycota and Zygomycota but increased that of Ascomycota, Chytridiomycota, and Glomeromycota for the whole paddy soil with field contamination of metals. Overall, although diversity cannot be a sensitive indicator for microbial communities among size fractions of aggregates (Lauber et al., 2009), particularly bacterial community herein, diversity and composition with the relative proportion of microbial individuals in MacA are found altered by management practices (Davinic et al., 2012), such as fertilization in this study.
In addition, the proportions by different-size fractions of aggregates among the fertilization treatments are shown in Figure 6. Hereby, compared with mass proportion (Figure 6A), the proportion by MacA became increasingly dominant for SOC (Figure 6B), bacterial (Figure 6C), and fungal abundance (Figure 6D). However, compared with the SOC proportion by L-MacA unchanged with fertilization, the fungal proportion by L-MacA decreased with CF and CFM treatment, although the bacterial proportion was similar among the fertilized plots. This can infer that the enriched fungal population in L-MacA is not favoring macroaggregation possibly due to the stress by metal contamination mentioned previously. Again, MacA functioned as hotspots supporting a dominant portion of microbes, which can be modified to a lesser extent by fertilization. As Nie et al. (2014) advocated, the distribution of aggregates within soils can be affected by both physical and biological processes and in turn affected microbial function in response to climate change. Therefore, fertilization can alter the soil microbial distribution pattern mainly by modifying the relative proportion of different-size fractions, thus affecting the extent of microbial interaction among aggregates shaped by size.
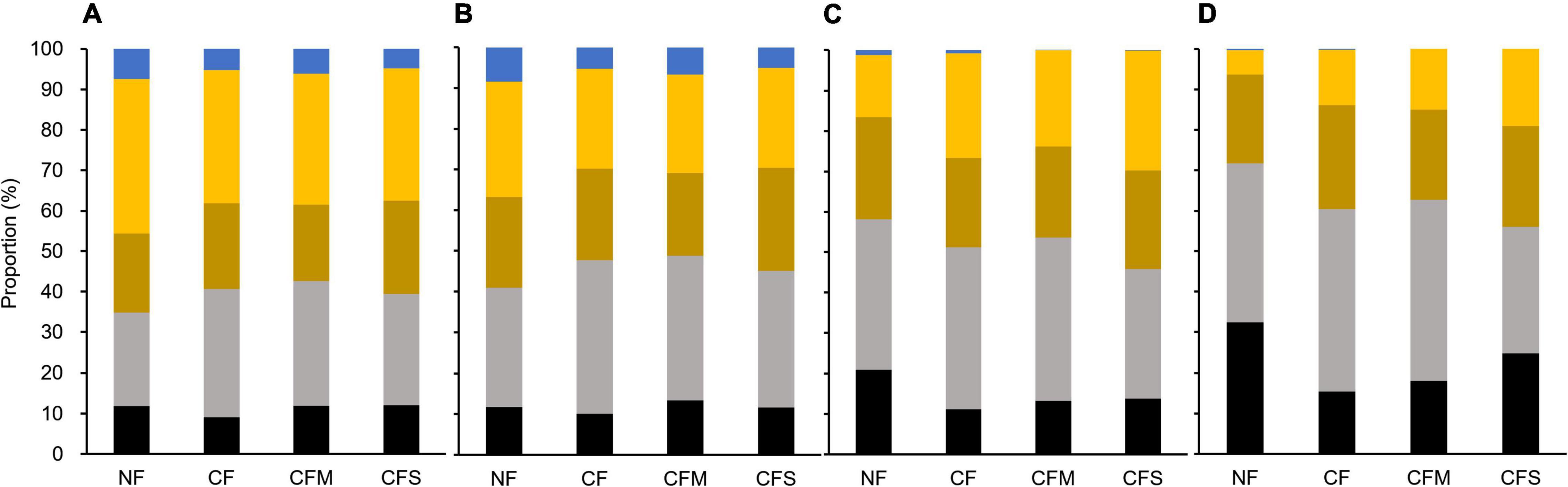
Figure 6. Proportion of mass (A), SOC (B), bacterial abundance (C), and fungal abundance (D) by different-size fractions of soil aggregates under the fertilization treatments. Black, L-MacA; gray, MacA; Brown, MicA; yellow, F-MicA; blue, F-Clay. NF, no fertilizer applied; CF, compound inorganic fertilizer; CFM, inorganic fertilizer combined with swine manure; CFS, inorganic fertilizer combined with residue return.
Conclusion
This study provides general insight into microbial community structure, individual interaction, and functional profile across aggregate-size fractions of a paddy soil under long-term conventional fertilization regimens from the Taihu Plan, China. Our results revealed that SOC and microbial abundance were both enriched in L-MacA and MacA but depleted in F-Clay particles across the treatments. Meanwhile, compared with MicA, microbial abundance concerning SOC accumulation was increased, and microbial networking and interaction were enhanced in MacA, with higher abundances of r-strategists and copiotrophs with more functional genes related to carbon and nitrogen metabolism. Also, soil MacA tended to contain fewer guilds of fungal parasite and litter saprotroph, suggesting better soil health with macroaggregation promoted under combined organic/inorganic fertilization. Thereby, this study confirms MacA as micro-hotspots for microbial community networking, microbial functioning, and potential plant defense. Of course, fertilization can modify the aggregate mass proportion and in turn microbial composition by macroaggregation. Consequently, our study suggests that spatial distribution or isolation of microbes in differently sized soil aggregates can underspin the soil fertility and health changes with fertilization in rice paddy. Moreover, the dynamics of microbial distribution, interaction, and function within aggregate-size fractions can matter soil functions for plant production and ecosystem health in rice paddy under the pressure of food production and climate change.
Data Availability Statement
The datasets presented in this study can be found in online repositories. The names of the repository/repositories and accession number(s) can be found in the article/Supplementary Material.
Author Contributions
ZR, GP, ZCL, DZ, and SS designed the research. ZR, ZCL, and GP performed the data analysis. ZR wrote the manuscript. GP, XDL, ZL, HL, XYL, JZ, MD, KC, RB, XZ, and LL revised and commented on the draft. All authors contributed to the article and approved the submitted version.
Funding
This study was partially supported by the National Natural Science Foundation of China under the grant number of 41370528. Funding for advanced soil research was also provided with the project “Two First-Class Discipline Construction Plan” by the Ministry of Education of China.
Conflict of Interest
The authors declare that the research was conducted in the absence of any commercial or financial relationships that could be construed as a potential conflict of interest.
Publisher’s Note
All claims expressed in this article are solely those of the authors and do not necessarily represent those of their affiliated organizations, or those of the publisher, the editors and the reviewers. Any product that may be evaluated in this article, or claim that may be made by its manufacturer, is not guaranteed or endorsed by the publisher.
Supplementary Material
The Supplementary Material for this article can be found online at: https://www.frontiersin.org/articles/10.3389/fmicb.2022.831746/full#supplementary-material
Footnotes
References
Anderson, M. J. (2001). A new method for non-parametric multivariate analysis of variance. Aust. Ecol. 26, 32–46. doi: 10.1111/j.1442-9993.2001.01070.pp.x
Bach, E. M., Williams, R. J., Hargreaves, S. K., Yang, F., and Hofmockel, K. S. (2018). Greatest soil microbial diversity found in micro-habitats. Soil Biol. Biochem. 118, 217–226. doi: 10.1016/j.soilbio.2017.12.018
Baldock, J. A., and Skjemstad, J. O. (2000). Role of the soil matrix and minerals in protecting natural organic materials against biological attack. Org. Geochem. 31, 697–710. doi: 10.1016/s0146-6380(00)00049-8
Barberan, A., Bates, S. T., Casamayor, E. O., and Fierer, N. (2012). Using network analysis to explore co-occurrence patterns in soil microbial communities. ISME J. 6, 343–351. doi: 10.1038/ismej.2011.119
Bastian, M., Heymann, S., and Jacomy, M. (2009). “Gephi: an open source software for exploring and manipulating networks,” in Proceedings of the 3rd International AAAI Conference on Weblogs and Social Media, San Jose, CA.
Brookes, P. C., Landman, A., Pruden, G., and Jenkinson, D. S. (1985). Chloroform fumigation and the release of soil nitrogen: a rapid direct extraction method to measure microbial biomass nitrogen in soil. Soil Biol. Biochem. 17, 837–842. doi: 10.1016/0038-0717(85)90144-0
Bundt, M., Widmer, F., Pesaro, M., Zeyer, J., and Blaser, P. (2001). Preferential flow paths: biological ‘hot spots’ in soils. Soil Biol. Biochem. 33, 729–738. doi: 10.1016/S0038-0717(00)00218-2
Cotrufo, M. F., Wallenstein, M. D., Boot, C. M., Denef, K., and Paul, E. (2013). The Microbial Efficiency-Matrix Stabilization (MEMS) framework integrates plant litter decomposition with soil organic matter stabilization: do labile plant inputs form stable soil organic matter? Glob. Change Biol. 19, 988–995. doi: 10.1111/gcb.12113
Csardi, G., and Nepusz, T. (2006). The igraph software package for complex network research. Int. J. Complex Syst. 1695, 1–9. doi: 10.1186/1471-2105-12-455
Davinic, M., Fultz, L. M., Acosta-Martinez, V., Calderon, F. J., Cox, S. B., Dowd, S. E., et al. (2012). Pyrosequencing and mid-infrared spectroscopy reveal distinct aggregate stratification of soil bacterial communities and organic matter composition. Soil Biol. Biochem. 46, 63–72. doi: 10.1016/j.soilbio.2011.11.012
Denef, K., Six, J., Paustian, K., and Merckx, R. (2001). Importance of macroaggregate dynamics in controlling soil carbon stabilization: short-term effects of physical disturbance induced by dry-wet cycles. Soil Biol. Biochem. 33, 2145–2153. doi: 10.1016/s0038-0717(01)00153-5
Ding, L. J., Su, J. Q., Sun, G. X., Wu, J. S., and Wei, W. X. (2018). Increased microbial functional diversity under long-term organic and integrated fertilization in a paddy soil. Appl. Microbiol. Biotechnol. 102, 1969–1982. doi: 10.1007/s00253-017-8704-8
Dorodnikov, M., Blagodatskaya, E., Blagodatsky, S., Marhan, S., Fangmeier, A., and Kuzyakov, Y. (2009). Stimulation of microbial extracellular enzyme activities by elevated CO2 depends on soil aggregate size. Glob. Change Biol. 15, 1603–1614. doi: 10.1111/j.1365-2486.2009.01844.x
Douglas, G. M., Beiko, R. G., and Langille, M. G. I. (2018). “Predicting the functional potential of the microbiome from marker genes using PICRUSt,” in Microbiome Analysis: Methods and Protocols, eds R. G. Beiko, W. Hsiao, and J. Parkinson (New York, NY: Springer New York), 169–177. doi: 10.1007/978-1-4939-8728-3_11
Dungait, J. A. J., Hopkins, D. W., Gregory, A. S., and Whitmore, A. P. (2012). Soil organic matter turnover is governed by accessibility not recalcitrance. Glob. Change Biol. 18, 1781–1796. doi: 10.1111/j.1365-2486.2012.02665.x
Ebrahimi, A. N., and Or, D. (2014). Microbial dispersal in unsaturated porous media: characteristics of motile bacterial cell motions in unsaturated angular pore networks. Water Resour. Res. 50, 7406–7429. doi: 10.1002/2014wr015897
Edgar, R. C. (2013). UPARSE: highly accurate OTU sequences from microbial amplicon reads. Nat. Methods 10, 996–998. doi: 10.1038/nmeth.2604
Ekschmitt, K., Liu, M., Vetter, S., Fox, O., and Wolters, V. (2005). Strategies used by soil biota to overcome soil organic matter stability — why is dead organic matter left over in the soil? Geoderma 128, 167–176. doi: 10.1016/j.geoderma.2004.12.024
Faith, D. P., Minchin, P. R., and Belbin, L. (1987). Compositional dissimilarity as a robust measure of ecological distance. Vegetatio 69, 57–68. doi: 10.1007/bf00038687
Fansler, S. J., Smith, J. L., Bolton, H., and Bailey, V. L. (2005). Distribution of two C cycle enzymes in soil aggregates of a prairie chronosequence. Biol. Fertil. Soils 42, 17–23. doi: 10.1007/s00374-005-0867-2
Faust, K., and Raes, J. (2012). Microbial interactions: from networks to models. Nat. Rev. Microbiol. 10, 538–550. doi: 10.1038/nrmicro2832
Fierer, N., Jackson, J. A., Vilgalys, R., and Jackson, R. B. (2005). Assessment of soil microbial community structure by use of taxon-specific quantitative PCR assays. Appl. Environ. Microbiol. 71, 4117–4120. doi: 10.1128/AEM.71.7.4117-4120.2005
Fontaine, S., Mariotti, A., and Abbadie, L. (2003). The priming effect of organic matter: a question of microbial competition? Soil Biol. Biochem. 35, 837–843. doi: 10.1016/s0038-0717(03)00123-8
Fuhrman, J. A. (2009). Microbial community structure and its functional implications. Nature 459, 193–199. doi: 10.1038/nature08058
Gleixner, G. (2013). Soil organic matter dynamics: a biological perspective derived from the use of compound-specific isotopes studies. Ecol. Res. 28, 683–695. doi: 10.1007/s11284-012-1022-9
Gong, Z. T., Zhang, G. L., and Chen, Z. C. (2007). Pedogenesis and Soil Taxonomy. Beijing: Science Press, 335–338.
Grandy, A. S., and Robertson, G. P. (2007). Land-use intensity effects on soil organic carbon accumulation rates and mechanisms. Ecosystems 10, 58–73. doi: 10.1007/s10021-006-9010-y
Haefele, S. M., Nelson, A., and Hijmans, R. J. (2014). Soil quality and constraints in global rice production. Geoderma 235, 250–259. doi: 10.1016/j.geoderma.2014.07.019
Hao, R. J., Li, Z. P., Che, Y. P., and Fang, H. L. (2008). Organic carbon mineralization in various size aggregates of paddy soil under aerobic and submerged conditions. Chin. J. Appl. Ecol. 19, 1944–1950. doi: 10.3724/SP.J.1005.2008.01083
Horner-Devine, M. C., Silver, J. M., Leibold, M. A., Bohannan, B. J., Colwell, R. K., Fuhrman, J. A., et al. (2007). A comparison of taxon co-occurrence patterns for macro- and microorganisms. Ecology 88, 1345–1353. doi: 10.1890/06-0286
Hou, D., Zhang, P., Zhang, J., Zhou, Y., Yang, Y., Mao, Q., et al. (2019). Spatial variation of sediment bacterial community in an acid mine drainage contaminated area and surrounding river basin. J. Environ. Manage. 251:109542. doi: 10.1016/j.jenvman.2019.109542
Jiang, Y. L., Lei, Y. B., Yang, Y., Korpelainen, H., Niinemets, U., and Li, C. Y. (2018). Divergent assemblage patterns and driving forces for bacterial and fungal communities along a glacier forefield chronosequence. Soil Biol. Biochem. 118, 207–216. doi: 10.1016/j.soilbio.2017.12.019
Jin, Z. J., Tai, J. C., Pan, G. X., Li, L. Q., Song, X. Y., Xie, T., et al. (2012). Comparison of soil organic carbon, microbial diversity and enzyme activity of wetlands and rice paddies in Jingjiang area of Hubei, China. Sci. Agric. Sin. 45, 3773–3781. doi: 10.3864/j.issn.0578-1752.2012.18.010
Juyal, A., Eickhorst, T., Falconer, R., Baveye, P. C., Spiers, A., and Otten, W. (2018). Control of pore geometry in soil microcosms and its effect on the growth and spread of Pseudomonas and Bacillus sp. Front. Environ. Sci. 6:73. doi: 10.3389/fenvs.2018.00073
Juyal, A., Otten, W., Falconer, R., Hapca, S., Schmidt, H., Baveye, P. C., et al. (2019). Combination of techniques to quantify the distribution of bacteria in their soil microhabitats at different spatial scales. Geoderma 334, 165–174. doi: 10.1016/j.geoderma.2018.07.031
Kallenbach, C. M., Frey, S. D., and Grandy, A. S. (2016). Direct evidence for microbial-derived soil organic matter formation and its ecophysiological controls. Nat. Commun. 7:13630. doi: 10.1038/ncomms13630
Kanehisa, M., and Goto, S. (2000). KEGG: Kyoto encyclopedia of genes and genomes. Nucleic Acids Res. 28, 27–30. doi: 10.1093/nar/28.1.27
King, A. E., Congreves, K. A., Deen, B., Dunfield, K. E., Voroney, R. P., and Wagner-Riddle, C. (2019). Quantifying the relationships between soil fraction mass, fraction carbon, and total soil carbon to assess mechanisms of physical protection. Soil Biol. Biochem. 135, 95–107. doi: 10.1016/j.soilbio.2019.04.019
Kozich, J. J., Westcott, S. L., Baxter, N. T., Highlander, S. K., and Schloss, P. D. (2013). Development of a dual-index sequencing strategy and curation pipeline for analyzing amplicon sequence data on the MiSeq Illumina sequencing platform. Appl. Environ. Microbiol. 79, 5112–5120. doi: 10.1128/AEM.01043-13
Kuzyakov, Y., and Blagodatskaya, E. (2015). Microbial hotspots and hot moments in soil: concept & review. Soil Biol. Biochem. 83, 184–199. doi: 10.1016/j.soilbio.2015.01.025
Lagomarsino, A., Grego, S., and Kandeler, E. (2012). Soil organic carbon distribution drives microbial activity and functional diversity in particle and aggregate-size fractions. Pedobiologia 55, 101–110. doi: 10.1016/j.pedobi.2011.12.002
Lal, R. (2004). Soil carbon sequestration impacts on global climate change and food security. Science 304, 1623–1627. doi: 10.1126/science.1097396
Langfelder, P., and Horvath, S. (2012). Fast R functions for robust correlations and hierarchical clustering. J. Stat. Softw. 46, 1–17.
Langille, M. G., Zaneveld, J., Caporaso, J. G., McDonald, D., Knights, D., Reyes, J. A., et al. (2013). Predictive functional profiling of microbial communities using 16S rRNA marker gene sequences. Nat. Biotechnol. 31, 814–821. doi: 10.1038/nbt.2676
Lauber, C. L., Hamady, M., Knight, R., and Fierer, N. (2009). Pyrosequencing-based assessment of soil pH as a predictor of soil bacterial community structure at the continental scale. Appl. Environ. Microbiol. 75, 5111–5120. doi: 10.1128/aem.00335-09
Lauro, F. M., McDougald, D., Thomas, T., Williams, T. J., Egan, S., Rice, S., et al. (2009). The genomic basis of trophic strategy in marine bacteria. Proc. Natl. Acad. Sci. U.S.A. 106, 15527–15533. doi: 10.1073/pnas.0903507106
Lehmann, A., and Rillig, M. C. (2015). Understanding mechanisms of soil biota involvement in soil aggregation: a way forward with saprobic fungi? Soil Biol. Biochem. 88, 298–302. doi: 10.1016/j.soilbio.2015.06.006
Lehmann, A., Zheng, W., and Rillig, M. C. (2017). Soil biota contributions to soil aggregation. Nat. Ecol. Evol. 1, 1828–1835. doi: 10.1038/s41559-017-0344-y
Li, J., and Yang, C. (2019). Inconsistent response of soil bacterial and fungal communities in aggregates to litter decomposition during short-term incubation. PeerJ 7:e8078. doi: 10.7717/peerj.8078
Li, L. Q., Zhang, X. H., Zhang, P. J., Zheng, J. F., and Pan, G. X. (2007). Variation of organic carbon and nitrogen in aggregate size fractions of a paddy soil under fertilisation practices from Tai Lake Region, China. J. Sci. Food Agric. 87, 1052–1058. doi: 10.1002/jsfa.2806
Li, L. Q., Zheng, J. W., Pan, G. X., Tian, F. Q., Qiu, D. S., Xu, X. D., et al. (2003). Change of available pool of heavy metals in paddy soils under human land use impacts from the Taihu Lake region, Jiangsu Province, China. Chin. J. Environ. Sci. 24, 101–104. doi: 10.13227/j.hjkx.2003.03.020
Li, Z. C., Rui, Z. P., Zhang, D. X., Feng, X., Lu, H. F., Shen, S. Y., et al. (2019c). Macroaggregates as biochemically functional hotspots in soil matrix: evidence from a rice paddy under long-term fertilization treatments in the Taihu Lake Plain, eastern China. Appl. Soil Ecol. 138, 262–273. doi: 10.1016/j.apsoil.2019.01.013
Li, Y., Nie, C., Liu, Y., Du, W., and He, P. (2019b). Soil microbial community composition closely associates with specific enzyme activities and soil carbon chemistry in a long-term nitrogen fertilized grassland. Sci. Total Environ. 654, 264–274. doi: 10.1016/j.scitotenv.2018.11.031
Li, F., Qiu, P., Shen, B., and Shen, Q. (2019a). Soil aggregate size modifies the impacts of fertilization on microbial communities. Geoderma 343, 205–214. doi: 10.1016/j.geoderma.2019.02.039
Liang, A., Zhang, Y., Zhang, X., Yang, X., McLaughlin, N., Chen, X., et al. (2019). Investigations of relationships among aggregate pore structure, microbial biomass, and soil organic carbon in a Mollisol using combined non-destructive measurements and phospholipid fatty acid analysis. Soil Tillage Res. 185, 94–101. doi: 10.1016/j.still.2018.09.003
Liao, H., Zhang, Y. C., Zuo, Q. Y., Du, B. B., Chen, W. L., Wei, D., et al. (2018). Contrasting responses of bacterial and fungal communities to aggregate-size fractions and long-term fertilizations in soils of northeastern China. Sci. Total Environ. 635, 784–792. doi: 10.1016/j.scitotenv.2018.04.168
Liu, C., Li, L., Xie, J., Coulter, J. A., Zhang, R., Luo, Z., et al. (2020). Soil bacterial diversity and potential functions are regulated by long-term conservation tillage and straw mulching. Microorganisms 8:836. doi: 10.3390/microorganisms8060836
Liu, Y., Zhou, T., Crowley, D., Li, L., Liu, D., Zheng, J., et al. (2012). Decline in topsoil microbial quotient, fungal abundance and C utilization efficiency of rice paddies under heavy metal pollution across South China. PLoS One 7:e38858. doi: 10.1371/journal.pone.0038858
Liu, Y. L., Wang, P., Ding, Y. J., Lu, H. F., Li, L. Q., Cheng, K., et al. (2016). Microbial activity promoted with organic carbon accumulation in macroaggregates of paddy soils under long-term rice cultivation. Biogeosciences 13, 6565–6586. doi: 10.5194/bg-13-6565-2016
Lobe, I., Sandhage-Hofmann, A., Brodowski, S., du Preez, C. C., and Amelung, W. (2011). Aggregate dynamics and associated soil organic matter contents as influenced by prolonged arable cropping in the South African Highveld. Geoderma 162, 251–259. doi: 10.1016/j.geoderma.2011.02.001
Lu, H., Bian, R., Xia, X., Cheng, K., Liu, X., Liu, Y., et al. (2020). Legacy of soil health improvement with carbon increase following one time amendment of biochar in a paddy soil – a rice farm trial. Geoderma 376:114567. doi: 10.1016/j.geoderma.2020.114567
Lu, R. (2000). Methods of Soil and Agro-Chemical Analysis. Beijing: China Agricultural Science and Technology Press, 127–332.
Lu, X., Lu, X., and Liao, Y. (2018). Effect of tillage treatment on the diversity of soil arbuscular mycorrhizal fungal and soil aggregate-associated carbon content. Front. Microbiol. 9:2986. doi: 10.3389/fmicb.2018.02986
Lucas, S. T., D’Angelo, E. M., and Williams, M. A. (2014). Improving soil structure by promoting fungal abundance with organic soil amendments. Appl. Soil Ecol. 75, 13–23. doi: 10.1016/j.apsoil.2013.10.002
Lupatini, M., Suleiman, A. K. A., Jacques, R. J. S., Antoniolli, Z. I., de Siqueira Ferreira, A. O., Kuramae, E. E., et al. (2014). Network topology reveals high connectance levels and few key microbial genera within soils. Front. Environ. Sci. 2:10. doi: 10.3389/fenvs.2014.00010
Lupwayi, N. Z., Arshad, M. A., Rice, W. A., and Clayton, G. W. (2001). Bacterial diversity in water-stable aggregates of soils under conventional and zero tillage management. Appl. Soil Ecol. 16, 251–261. doi: 10.1016/s0929-1393(00)00123-2
Lützow, M. V., Kögel-Knabner, I., Ekschmitt, K., Matzner, E., Guggenberger, G., Marschner, B., et al. (2006). Stabilization of organic matter in temperate soils: mechanisms and their relevance under different soil conditions – a review. Eur. J. Soil Sci. 57, 426–445. doi: 10.1111/j.1365-2389.2006.00809.x
Ma, B., Wang, H., Dsouza, M., Lou, J., He, Y., Dai, Z., et al. (2016). Geographic patterns of co-occurrence network topological features for soil microbiota at continental scale in eastern China. ISME J. 10, 1891–1901. doi: 10.1038/ismej.2015.261
Ma, G., Kang, J., Wang, J., Chen, Y., Lu, H., Wang, L., et al. (2020). Bacterial community structure and predicted function in wheat soil from the North China plain are closely linked with soil and plant characteristics after seven years of irrigation and nitrogen application. Front. Microbiol. 11:506. doi: 10.3389/fmicb.2020.00506
Magoc, T., and Salzberg, S. L. (2011). FLASH: fast length adjustment of short reads to improve genome assemblies. Bioinformatics 27, 2957–2963. doi: 10.1093/bioinformatics/btr507
Martens, D. A., Reedy, T. E., and Lewis, D. T. (2004). Soil organic carbon content and composition of 130-year crop, pasture and forest land-use managements. Glob. Change Biol. 10, 65–78. doi: 10.1046/j.1529-8817.2003.00722.x
Martin, M. (2011). Cutadapt removes adapter sequences from high-throughput sequencing reads. EMBnet J. 17, 10–12. doi: 10.14806/ej.17.1.200
Marx, M. C., Kandeler, E., Wood, M., Wermbter, N., and Jarvis, S. C. (2005). Exploring the enzymatic landscape: distribution and kinetics of hydrolytic enzymes in soil particle-size fractions. Soil Biol. Biochem. 37, 35–48. doi: 10.1016/j.soilbio.2004.05.024
May, L. A., Smiley, B., and Schmidt, M. G. (2001). Comparative denaturing gradient gel electrophoresis analysis of fungal communities associated with whole plant corn silage. Can. J. Microbiol. 47, 829–841. doi: 10.1139/w01-086
Nannipieri, P., Ascher, J., Ceccherini, M. T., Landi, L., Pietramellara, G., and Renella, G. (2003). Microbial diversity and soil functions. Eur. J. Soil Sci. 54, 655–670. doi: 10.1046/j.1351-0754.2003.0556.x
Navarro-Garcia, F., Casermeiro, M. A., and Schimel, J. P. (2012). When structure means conservation: effect of aggregate structure in controlling microbial responses to rewetting events. Soil Biol. Biochem. 44, 1–8. doi: 10.1016/j.soilbio.2011.09.019
Negassa, W. C., Guber, A. K., Kravchenko, A. N., Marsh, T. L., Hildebrandt, B., and Rivers, M. L. (2015). Properties of soil pore space regulate pathways of plant residue decomposition and community structure of associated bacteria. PLoS One 10:e0123999. doi: 10.1371/journal.pone.0123999
Newman, M. E. (2006). Modularity and community structure in networks. Proc. Natl. Acad. Sci. U.S.A. 103, 8577–8582. doi: 10.1073/pnas.0601602103
Newman, M. E. J. (2003). The structure and function of complex networks. Siam Rev. 45, 167–256. doi: 10.1137/s003614450342480
Nguyen, N. H., Song, Z. W., Bates, S. T., Branco, S., Tedersoo, L., Menke, J., et al. (2016). FUNGuild: an open annotation tool for parsing fungal community datasets by ecological guild. Fungal Ecol. 20, 241–248. doi: 10.1016/j.funeco.2015.06.006
Nie, M., Pendall, E., Bell, C., and Wallenstein, M. D. (2014). Soil aggregate size distribution mediates microbial climate change feedbacks. Soil Biol. Biochem. 68, 357–365. doi: 10.1016/j.soilbio.2013.10.012
Oksanen, J., Kindt, R., Legendre, P., O’Hara, B., Stevens, M. H. H., Oksanen, M. J., et al. (2007). The vegan package. Community Ecol. Package 10, 631–637.
Pan, G., Li, L., Zheng, J., Cheng, K., Zhang, X., Zheng, J., et al. (2014). “Benefits of SOM in agroecosystems: the case of China,” in Soil Carbon: Science, Management and Policy for Multiple Benefits, eds S. A. Banwart, E. Noellemeyer, and E. Milne (London: CABI), 314–327. doi: 10.1079/9781780645322.0314
Pan, G. X., Li, L. Q., Wu, L. S., and Zhang, X. H. (2004). Storage and sequestration potential of topsoil organic carbon in China’s paddy soils. Glob. Change Biol. 10, 79–92. doi: 10.1111/j.1365-2486.2003.00717.x
Pan, G. X., Lu, H. F., Li, L. Q., Zheng, J. F., Zhang, X. H., Cheng, K., et al. (2015). Soil carbon sequestration with bioactivity: a new emerging frontier for sustainable soil management. Adv. Earth Sci. 30, 940–951. doi: 10.11867/j.issn.1001-8166.2015.08.0940
Pan, G. X., Zhou, P., Li, Z. P., Smith, P., Li, L. Q., Qiu, D. S., et al. (2009). Combined inorganic/organic fertilization enhances N efficiency and increases rice productivity through organic carbon accumulation in a rice paddy from the Tai Lake region, China. Agric. Ecosyst. Environ. 131, 274–280. doi: 10.1016/j.agee.2009.01.020
Parks, D. H., Tyson, G. W., Hugenholtz, P., and Beiko, R. G. (2014). STAMP: statistical analysis of taxonomic and functional profiles. Bioinformatics 30, 3123–3124. doi: 10.1093/bioinformatics/btu494
Pituello, C., Dal Ferro, N., Simonetti, G., Berti, A., and Morari, F. (2016). Nano to macro pore structure changes induced by long-term residue management in three different soils. Agric. Ecosyst. Environ. 217, 49–58. doi: 10.1016/j.agee.2015.10.029
Powlson, D. S., Gregory, P. J., Whalley, W. R., Quinton, J. N., Hopkins, D. W., Whitmore, A. P., et al. (2011). Soil management in relation to sustainable agriculture and ecosystem services. Food Policy 36, S72–S87. doi: 10.1016/j.foodpol.2010.11.025
Prosser, J. I., Bohannan, B. J., Curtis, T. P., Ellis, R. J., Firestone, M. K., Freckleton, R. P., et al. (2007). The role of ecological theory in microbial ecology. Nat. Rev. Microbiol. 5, 384–392. doi: 10.1038/nrmicro1643
Ranjard, L., Poly, F., Combrisson, J., Richaume, A., Gourbiere, F., Thioulouse, J., et al. (2000). Heterogeneous cell density and genetic structure of bacterial pools associated with various soil microenvironments as determined by enumeration and DNA fingerprinting approach (RISA). Microb. Ecol. 39, 263–272. doi: 10.1007/s002480000032
Raynaud, X., and Nunan, N. (2014). Spatial ecology of bacteria at the microscale in soil. PLoS One 9:e87217. doi: 10.1371/journal.pone.0087217
Rillig, M. C., Muller, L. A., and Lehmann, A. (2017). Soil aggregates as massively concurrent evolutionary incubators. ISME J. 11, 1943–1948. doi: 10.1038/ismej.2017.56
Roller, B. R., and Schmidt, T. M. (2015). The physiology and ecological implications of efficient growth. ISME J. 9, 1481–1487. doi: 10.1038/ismej.2014.235
Schlatter, D. C., Kahl, K., Carlson, B., Huggins, D. R., and Paulitz, T. (2018). Fungal community composition and diversity vary with soil depth and landscape position in a no-till wheat-based cropping system. FEMS Microbiol. Ecol. 94:fiy098. doi: 10.1093/femsec/fiy098
Schmid, C. A. O., Schroder, P., Armbruster, M., and Schloter, M. (2018). Organic amendments in a long-term field trial—consequences for the bulk soil bacterial community as revealed by network analysis. Microb. Ecol. 76, 226–239. doi: 10.1007/s00248-017-1110-z
Schmidt, M. W., Torn, M. S., Abiven, S., Dittmar, T., Guggenberger, G., Janssens, I. A., et al. (2011). Persistence of soil organic matter as an ecosystem property. Nature 478, 49–56. doi: 10.1038/nature10386
Sessitsch, A., Weilharter, A., Gerzabek, M. H., Kirchmann, H., and Kandeler, E. (2001). Microbial population structures in soil particle size fractions of a long-term fertilizer field experiment. Appl. Environ. Microbiol. 67, 4215–4224. doi: 10.1128/aem.67.9.4215-4224.2001
Sinsabaugh, R. L., Lauber, C. L., Weintraub, M. N., Ahmed, B., Allison, S. D., Crenshaw, C., et al. (2008). Stoichiometry of soil enzyme activity at global scale. Ecol. Lett. 11, 1252–1264. doi: 10.1111/j.1461-0248.2008.01245.x
Six, J., Conant, R. T., Paul, E. A., and Paustian, K. (2002). Stabilization mechanisms of soil organic matter: implications for C-saturation of soils. Plant Soil 241, 155–176. doi: 10.1023/A:1016125726789
Six, J., Elliott, E. T., and Paustian, K. (2000). Soil macroaggregate turnover and microaggregate formation: a mechanism for C sequestration under no-tillage agriculture. Soil Biol. Biochem. 32, 2099–2103. doi: 10.1016/S0038-0717(00)00179-6
Six, J., and Paustian, K. (2014). Aggregate-associated soil organic matter as an ecosystem property and a measurement tool. Soil Biol. Biochem. 68, A4–A9. doi: 10.1016/j.soilbio.2013.06.014
Soil Survey Staff (2014). Keys to Soil Taxonomy. Washington, DC: USDA-Natural Resources Conservation Service.
Song, H. K., Song, W., Kim, M., Tripathi, B. M., Kim, H., Jablonski, P., et al. (2017). Bacterial strategies along nutrient and time gradients, revealed by metagenomic analysis of laboratory microcosms. FEMS Microbiol. Ecol. 93:fix114. doi: 10.1093/femsec/fix114
Stemmer, M., Gerzabek, M. H., and Kandeler, E. (1998). Organic matter and enzyme activity in particle-size fractions of soils obtained after low-energy sonication. Soil Biol. Biochem. 30, 9–17. doi: 10.1016/S0038-0717(97)00093-X
Sun, D. S., Li, K. J., Bi, Q. F., Zhu, J., Zhang, Q. C., Jin, C. W., et al. (2017). Effects of organic amendment on soil aggregation and microbial community composition during drying-rewetting alternation. Sci. Total Environ. 574, 735–743. doi: 10.1016/j.scitotenv.2016.09.112
Tatusov, R. L., Galperin, M. Y., Natale, D. A., and Koonin, E. V. (2000). The COG database: a tool for genome-scale analysis of protein functions and evolution. Nucleic Acids Res. 28, 33–36. doi: 10.1093/nar/28.1.33
Tecon, R., Ebrahimi, A., Kleyer, H., Erev Levi, S., and Or, D. (2018). Cell-to-cell bacterial interactions promoted by drier conditions on soil surfaces. Proc. Natl. Acad. Sci. U.S.A. 115, 9791–9796. doi: 10.1073/pnas.1808274115
Tecon, R., and Or, D. (2017). Biophysical processes supporting the diversity of microbial life in soil. FEMS Microbiol. Rev. 41, 599–623. doi: 10.1093/femsre/fux039
Trivedi, P., Delgado-Baquerizo, M., Jeffries, T. C., Trivedi, C., Anderson, I. C., Lai, K., et al. (2017). Soil aggregation and associated microbial communities modify the impact of agricultural management on carbon content. Environ. Microbiol. 19, 3070–3086. doi: 10.1111/1462-2920.13779
Upton, R. N., Bach, E. M., and Hofmockel, K. S. (2019). Spatio-temporal microbial community dynamics within soil aggregates. Soil Biol. Biochem. 132, 58–68. doi: 10.1016/j.soilbio.2019.01.016
Vincent, M. E., Liu, W., Haney, E. B., and Ismagilov, R. F. (2010). Microfluidic stochastic confinement enhances analysis of rare cells by isolating cells and creating high density environments for control of diffusible signals. Chem. Soc. Rev. 39, 974–984. doi: 10.1039/b917851a
Vogel, C., Mueller, C. W., Hoschen, C., Buegger, F., Heister, K., Schulz, S., et al. (2014). Submicron structures provide preferential spots for carbon and nitrogen sequestration in soils. Nat. Commun. 5:2947. doi: 10.1038/ncomms3947
Vos, M., Wolf, A. B., Jennings, S. J., and Kowalchuk, G. A. (2013). Micro-scale determinants of bacterial diversity in soil. FEMS Microbiol. Rev. 37, 936–954. doi: 10.1111/1574-6976.12023
Wallenius, K., Rita, H., Mikkonen, A., Lappi, K., Lindstrom, K., Hartikainen, H., et al. (2011). Effects of land use on the level, variation and spatial structure of soil enzyme activities and bacterial communities. Soil Biol. Biochem. 43, 1464–1473. doi: 10.1016/j.soilbio.2011.03.018
Wander, M. (2004). “Soil organic matter fractions and their relevance to soil function,” in Soil Organic Matter in Sustainable Agriculture, eds F. Magdoff and R. Weil (Boca Raton, FL: CRC Press.).
Wang, P., Liu, Y., Li, L., Cheng, K., Zheng, J., Zhang, X., et al. (2015). Long-term rice cultivation stabilizes soil organic carbon and promotes soil microbial activity in a salt marsh derived soil chronosequence. Sci. Rep. 5:15704. doi: 10.1038/srep15704
Wang, W., Luo, X., Chen, Y., Ye, X., Wang, H., Cao, Z., et al. (2019). Succession of composition and function of soil bacterial communities during key rice growth stages. Front. Microbiol. 10:421. doi: 10.3389/fmicb.2019.00421
Wang, Y. D., Hu, N., Ge, T. D., Kuzyakov, Y., Wang, Z. L., Li, Z. F., et al. (2017). Soil aggregation regulates distributions of carbon, microbial community and enzyme activities after 23-year manure amendment. Appl. Soil Ecol. 111, 65–72. doi: 10.1016/j.apsoil.2016.11.015
Wang, Y. D., Wang, Z. L., Zhang, Q. Z., Hu, N., Li, Z. F., Lou, Y. L., et al. (2018). Long-term effects of nitrogen fertilization on aggregation and localization of carbon, nitrogen and microbial activities in soil. Sci. Total Environ. 624, 1131–1139. doi: 10.1016/j.scitotenv.2017.12.113
Whalen, J. K., and Chang, C. (2002). Macroaggregate characteristics in cultivated soils after 25 annual manure applications. Soil Sci. Soc. Am. J. 66, 1637–1647. doi: 10.2136/sssaj2002.1637
Wiesmeier, M., Urbanski, L., Hobley, E., Lang, B., von Lutzow, M., Marin-Spiotta, E., et al. (2019). Soil organic carbon storage as a key function of soils – a review of drivers and indicators at various scales. Geoderma 333, 149–162. doi: 10.1016/j.geoderma.2018.07.026
Wilpiszeski, R. L., Aufrecht, J. A., Retterer, S. T., Sullivan, M. B., Graham, D. E., Pierce, E. M., et al. (2019). Soil aggregate microbial communities: towards understanding microbiome interactions at biologically relevant scales. Appl. Environ. Microbiol. 85:e0324-19. doi: 10.1128/AEM.00324-19
Wu, H. B., Guo, Z. T., and Peng, C. H. (2003). Land use induced changes of organic carbon storage in soils of China. Glob. Change Biol. 9, 305–315. doi: 10.1046/j.1365-2486.2003.00590.x
Yan, H., Ouyang, M., Zhang, X. H., Ying, D., Zhao, X. J., Zhang, Y. J., et al. (2018). Effects of different gradients of heavy metal contamination on soil fungal community structure in paddy soils. Soils 50, 513–521. doi: 10.13758/j.cnki.tr.2018.03.011
Zhang, H., Ding, W., He, X., Yu, H., Fan, J., and Liu, D. (2014). Influence of 20-year organic and inorganic fertilization on organic carbon accumulation and microbial community structure of aggregates in an intensively cultivated sandy loam soil. PLoS One 9:e92733. doi: 10.1371/journal.pone.0092733
Zhang, P., Zheng, J., Pan, G., Zhang, X., Li, L., and Tippkotter, R. (2007). Changes in microbial community structure and function within particle size fractions of a paddy soil under different long-term fertilization treatments from the Tai Lake region, China. Colloids Surf. B Biointerfaces 58, 264–270. doi: 10.1016/j.colsurfb.2007.03.018
Zhang, Q., Zhou, W., Liang, G. Q., Sun, J. W., Wang, X. B., and He, P. (2015). Distribution of soil nutrients, extracellular enzyme activities and microbial communities across particle-size fractions in a long-term fertilizer experiment. Appl. Soil Ecol. 94, 59–71. doi: 10.1016/j.apsoil.2015.05.005
Zhang, S. L., Wang, R. J., Yang, X. Y., Sun, B. H., and Li, Q. H. (2016). Soil aggregation and aggregating agents as affected by long term contrasting management of an Anthrosol. Sci. Rep. 6:39107. doi: 10.1038/srep39107
Keywords: rice paddy, aggregate-size fraction, microbial community, soil organic carbon, co-occurrence network, functional profile
Citation: Rui Z, Lu X, Li Z, Lin Z, Lu H, Zhang D, Shen S, Liu X, Zheng J, Drosos M, Cheng K, Bian R, Zhang X, Li L and Pan G (2022) Macroaggregates Serve as Micro-Hotspots Enriched With Functional and Networked Microbial Communities and Enhanced Under Organic/Inorganic Fertilization in a Paddy Topsoil From Southeastern China. Front. Microbiol. 13:831746. doi: 10.3389/fmicb.2022.831746
Received: 08 December 2021; Accepted: 28 February 2022;
Published: 11 April 2022.
Edited by:
Annamaria Bevivino, Italian National Agency for New Technologies, Energy and Sustainable Economic Development (ENEA), ItalyReviewed by:
Shuo Jiao, Northwest A&F University, ChinaKe-Qing Xiao, University of Leeds, United Kingdom
Copyright © 2022 Rui, Lu, Li, Lin, Lu, Zhang, Shen, Liu, Zheng, Drosos, Cheng, Bian, Zhang, Li and Pan. This is an open-access article distributed under the terms of the Creative Commons Attribution License (CC BY). The use, distribution or reproduction in other forums is permitted, provided the original author(s) and the copyright owner(s) are credited and that the original publication in this journal is cited, in accordance with accepted academic practice. No use, distribution or reproduction is permitted which does not comply with these terms.
*Correspondence: Genxing Pan, gxpan@njau.edu.cn