- 1Research Center for Eco-Environmental Sciences, Chinese Academy of Sciences, Beijing, China
- 2College of Resource and Environment, University of Chinese Academy of Sciences, Beijing, China
In synthetic biology, engineering principles are applied to system design. The development of synthetic microbial consortia represents the intersection of synthetic biology and microbiology. Synthetic community systems are constructed by co-cultivating two or more microorganisms under certain environmental conditions, with broad applications in many fields including ecological restoration and ecological theory. Synthetic microbial consortia tend to have high biological processing efficiencies, because the division of labor reduces the metabolic burden of individual members. In this review, we focus on the environmental applications of synthetic microbial consortia. Although there are many strategies for the construction of synthetic microbial consortia, we mainly introduce the most widely used construction principles based on cross-feeding. Additionally, we propose methods for constructing synthetic microbial consortia based on traits and spatial structure from the perspective of ecology to provide a basis for future work.
Introduction
The concept of synthetic biology was developed in the last century. Synthetic biology is an applied discipline in which engineering principles are applied to system design (Kitney and Freemont, 2012). The goal of synthetic biology is to design and manipulate bio-based parts, devices, and systems to create new functions. It can also be used to redesign existing natural biological systems. Synthetic biology has extensive applications in the fields of energy, medicine, and environmental science. An important emerging area of research in synthetic biology is the development of synthetic microbial consortia, which refers to artificial consortia systems constructed by co-cultivating two or more microorganisms under certain environmental conditions (De Roy et al., 2014; Grosskopf and Soyer, 2014). Synthetic microbial consortia have wide applications and represent the intersection of synthetic biology and microbiology.
Synthetic microbial consortia, like synthetic biology broadly, are based on a closed-loop research method of design-build-test-learn (DBTL) (Lawson et al., 2019) and have the characteristics of engineering. Two classic engineering strategies, “top-down” and “bottom-up,” are used to obtain synthetic microbial consortia (Figure 1). In addition to these two classic strategies, there is also a construction strategy that combines the two strategies.
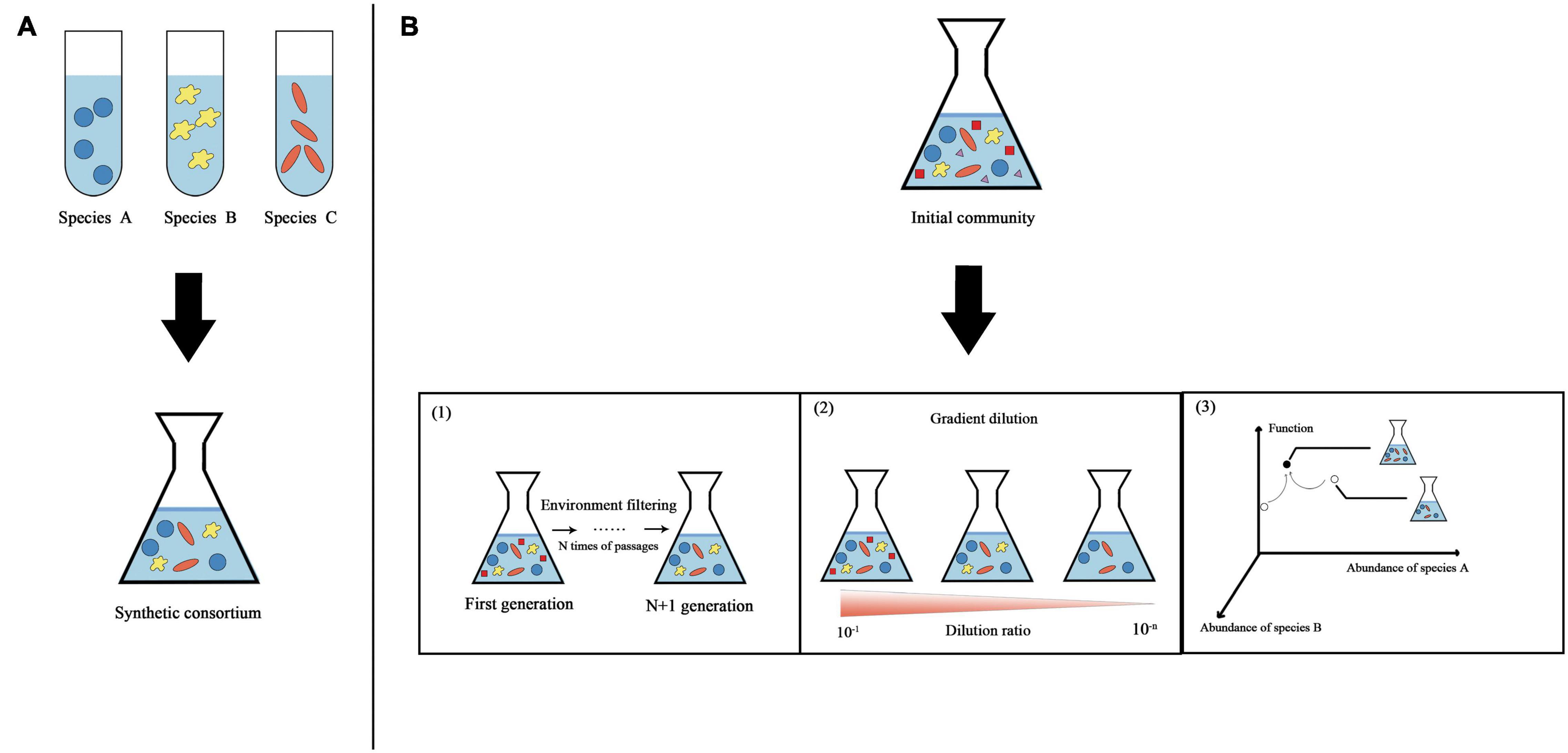
Figure 1. Overview of two classic strategies used to obtain synthetic microbial consortia. (A) “Bottom-up” strategy. Different species are assembled according to rules to obtain synthetic consortia with specific functions. (B) “Top-down” strategy. Using natural microbial communities, physical and chemical parameters are optimized in a bioreactor to maximize the community function. Three specific methods for obtaining synthetic consortia are shown here based on this strategy. (1) Continued enrichment. A stable synthetic consortium is obtained through ongoing environmental filtering of the original community. (2) Gradient dilution. Gradient dilution of the original community to extinction is performed to obtain a synthetic consortium with relatively few species but functional stability. (3) Directed evolution. Microbial community structure-function graphs are constructed from multiple stable communities with different functions. The community state in the graph is determined by the abundance of different species in the stabilized community (this graph is simplified to show only two dimensions). We first select stable communities with relatively strong functions from the community library and then apply ecological perturbations to generate neighborhood variation; thereby, the communities gradually approach stable states with maximal functions.
Top-Down
Assembly is the main concept in the “top-down” strategy. This strategy refers to the establishment of a stable co-cultivation system for multiple bacterial groups in accordance with certain principles to perform desired functions. Synthetic microbial consortia formed by this strategy have been shown to be more effective than single strains in terms of the synthesis or degradation of organic matter (Zuroff and Curtis, 2012). However, the identification and culture of microorganisms is an important prerequisite for this strategy. Although various techniques, such as in situ culture, high-throughput culture, resuscitation stimulation, and cell sorting, have promoted research on uncultured microorganisms, most microorganisms remain unculturable (Xie et al., 2021). Furthermore, the long-term regulation of community structure is a challenge to this strategy. In long-term cultivation, different microbial taxa compete for the same resources, and one species tends to be dominant.
Bottom-Up
A selection process is the basis for this strategy. Using natural microbial communities, physical and chemical parameters are optimized in a bioreactor to maximize the community function and finally obtain the minimal active microbial consortia (MAMC) (Puentes-Tellez and Falcao Salles, 2018). They are critical players in a complex ecosystem. MAMC have the same division of labor as “top-down” consortia, yet retain functional redundancy. They are co-evolved from a group of microorganisms under certain culture conditions, and may have better temporal stability than that of “top-down” consortia (Gilmore et al., 2019). However, the acquisition of the MAMC is random, and it is difficult to determine the direction of evolution.
This strategy involves two main methods: continuous enrichment (Gilmore et al., 2019) and serial dilution (Diaz-Garcia et al., 2021). However, these two methods are random and cannot guarantee the ecological balance of the synthetic consortium. Some studies combine directed evolution to propose a method to find stable synthetic consortia based on a top-down strategy. Chang et al. (2021) constructed a multidimensional coordinate system of microbial community structure-function, containing multiple stable communities with different functions. Community status is determined by the abundance of different species in stable communities. A community with strong function is selected from the community library, and communities showing variation are generated by ecological disturbance, gradually approaching a stable community with the maximum function. Types of ecological disturbance include species invasion, species elimination, random small displacement of nutrients, etc. The directed evolution of communities can enable the efficient selection of ecologically stable functional synthetic consortia.
Multi-Strategy
To address the shortcomings of the “top-down” and “bottom-up” strategies, researchers have recently tried to combine these strategies to design synthetic microbial consortia. We can assemble multiple members into consortia according to the principle of metabolic networks and then achieve enhanced functions by changing environmental variables to regulate the consortia. Controlling the inoculation rate is the most common method for the assembly of multiple consortium members; however, synthetic microbial consortia constructed by this method often deviate rapidly from the initial conditions. A method based on temperature cycling has been proposed (Krieger et al., 2021) to effectively control a synthetic microbial consortia assembled by Escherichia coli and Pseudomonas putida. Many environmental variables can be altered to adjust the composition of the synthetic microbial consortia, like temperature, and thereby to adjust the metabolic function of the consortia. These methods are still essentially based on ecological mechanisms.
Different strategies have been applied to the construction of synthetic microbial consortia. Table 1 gives examples of the construction of synthetic microbial consortia by different strategies.
Application of Synthetic Microbial Consortia in Environmental Science
Synthetic microbial consortia have important significance in many fields, such as bioprocessing (Shong et al., 2012) and fermentation (Wang et al., 2016). But we pay more attention to its application in the environmental field. The value of the synthetic consortium in the environmental field is mainly in two areas: ecological restoration and ecological theory.
Ecological Restoration
Restoration ecology using synthetic microbial consortia mainly involves two major aims, the degradation of pollutants and the restoration of biodiversity. Pure bacterial systems may show good performance in the laboratory but often face challenges when applied to actual contaminated sites (Liang et al., 2020). Synthetic microbial consortia may show better adaptability and even higher degradation efficiencies than those of pure bacterial systems. For example, Acinetobacter sp. XM-02 and Pseudomonas sp. have been used to degrade alkane (diesel and crude oil) pollution (Chen et al., 2014). Acinetobacter sp. XM-02 can degrade alkane alone. Although Pseudomonas sp. cannot degrade alkanes, it can produce surfactants that increase the contact of microorganisms with the oil surface by reducing the surface tension of the medium. The degradation rate of alkane by the co-culture system was 8.06% higher than that of a single strain. Community or species inoculation is one approach to restore ecological diversity. It is mainly used in the restoration of saline-alkaline soil or soils with reduced soil fertility. In a previous study (Anees et al., 2020), the efficacy of phytoremediation of saline-alkaline soil was improved by combining halotolerant and chitinolytic bacteria. Halotolerant bacteria have an antagonistic effect on plant pathogens, and chitinolytic bacteria may help plants escape salt stress.
Ecological Theory
The assembly of microbial consortia can provide insight into the mechanism underlying microbial community formation and the link between the structure of microbial communities and ecological functions. The phylogenetic limiting similarity hypothesis (PLSH), a classic hypothesis in ecology, suggests that the struggle for existence is stronger between more closely related species. Violle et al. (2011) used bacterivorous ciliated protist species as samples and established a number of synthetic microbial consortia with pairwise interactions in different microcosms to evaluate the relationship between phylogenetic correlation and competitive interactions in ecological communities. The complexity of natural microbial communities may make it difficult to control artificially imposed factors and interpret patterns (Emery and Gross, 2007; De Roy et al., 2014). Synthetic microbial consortia have interspecific interactions similar to natural microbial communities for analyses of associated community changes. Therefore, synthetic microbial consortia have become a powerful tool for ecological theory.
Construction of Synthetic Microbial Consortia Based on Cross-Feeding
Definition and Application of Cross-Feeding
Cross-feeding is a common principle for constructing synthetic microbial consortia. It is a common process in natural communities (Zelezniak et al., 2015) and is considered to contribute to the maintenance of the diversity and stability of natural microbial communities (Klitgord and Segre, 2011; Tsoi et al., 2018). When a metabolite is fully present in the environment, microorganisms may lose some functions due to mutations and form auxotrophs (D’Souza and Kost, 2016). However, neighboring microorganisms that produce the metabolite can become a new source, and they build an interaction network for cross-feeding (Pande et al., 2014). A broad definition of cross-feeding has been proposed (Fritts et al., 2021), in which four requirements must be met. (1) The compounds must be transferred from the producer to the receiver. (2) The transferred compounds must be taken up by the receiver or participate in energy conversion. (3) The adaptability of the producer and the receiver will change due to the acquired compounds. (4) Cross-feeding must involve different species or groups of different genotypes. Cross-feeding compounds vary; the most common are metabolites, followed by extracellular enzymes, siderophores, and so on. In addition, cross-feeding can be unidirectional, bidirectional, and multidirectional (Figure 2), and species or groups vary from two to many. This is a simple method for the classification of cross-feeding, and more specialized classifications have been developed (Smith et al., 2019). The most common example of cross-feeding in nature is the degradation of lignocellulose (Lee et al., 2021). In this process, each member of the microbial community completes a step in the degradation pathway, releasing an intermediate product to be used by the next member, and some members degrade toxic intermediates. There is also cross-feeding of product exchange through microbe-microbe surface interactions, such as the use of electrically conductive pili (epili) for electron transport by community members during methanogenesis (Rotaru et al., 2014). When microorganisms exchange metabolites by cross-feeding and all members can benefit from this exchange, this is referred to as functional specialization or division of labor (Giri et al., 2019). We can build synthetic microbial consortia based on cross-feeding by reasonable microbial division of labor. The community not only retains some of the complexity of natural communities, such as the ability to strengthen ecosystem functions and resist environmental disturbances (Sieuwerts et al., 2008; Spus et al., 2015), but also uses interactions to increase productivity or resource utilization (Bernstein et al., 2012; Jones et al., 2017). Table 2 shows examples of the application of cross-feeding principles to construct synthetic microbial consortia.
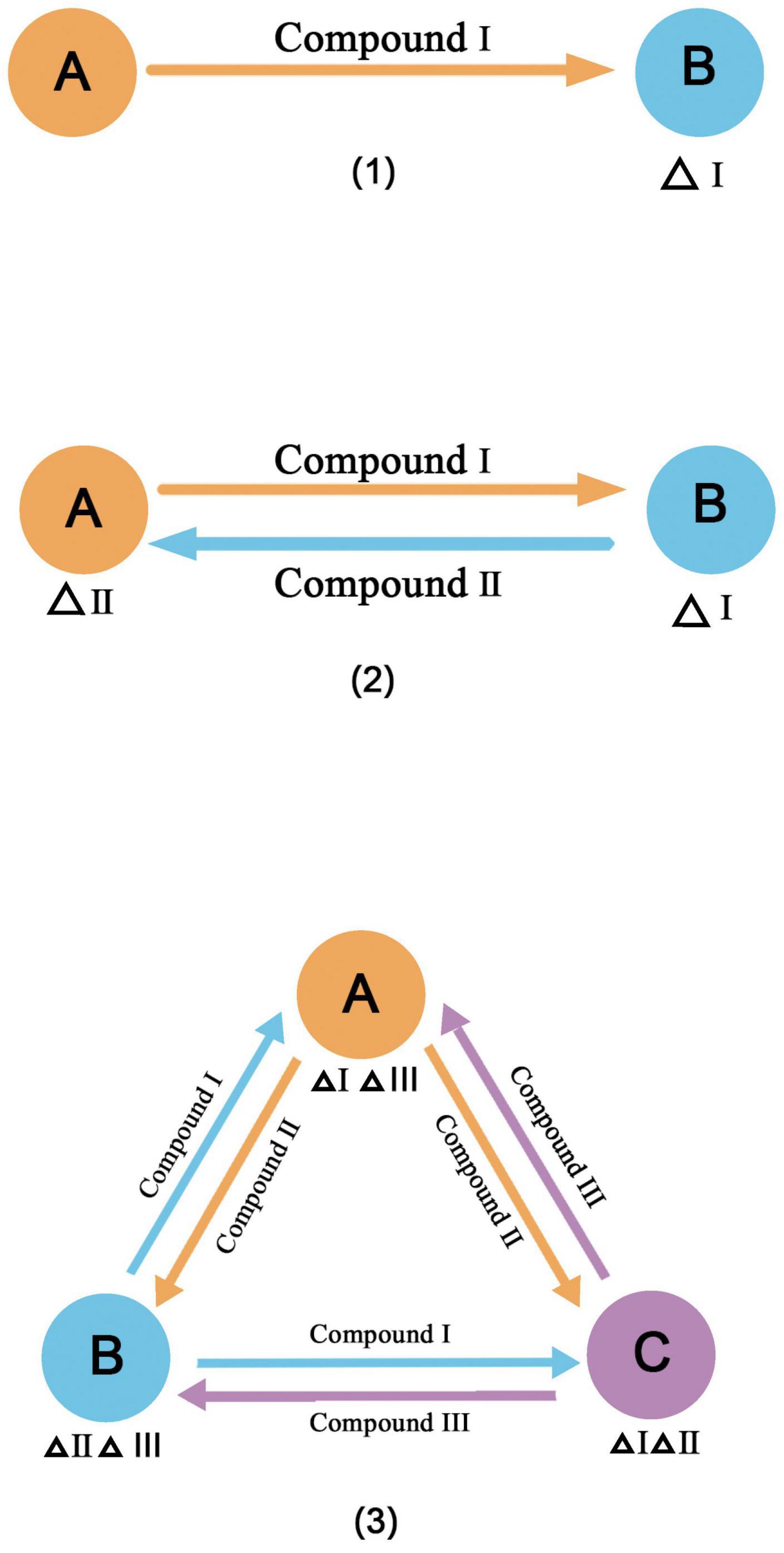
Figure 2. Three forms of cross feeding (1) unidirectional (2) bidirectional (3) multidirectional. Δindicates the lack of materials.
A combination of mathematical modeling and biology has been used to design and construct synthetic microbial consortia. Ecological models describing microbial interactions have been developed, such as the generalized Lotka–Volterra (gLV) and consumer-resource (C-R) models (Coyte et al., 2015; Niehaus et al., 2019). However, the parameters are often highly complex and substantial data are required for model fitting. Therefore, a series of optimizations based on these models have been developed to enable the description of more complex communities. Liao et al. (2020) established a coarse-grained metabolic model to simulate unidirectional, bidirectional, and multidirectional cross-feeding and accurately predicting the response to interference. Zelezniak et al. (2015) described a genome-scale modeling method to study the complex cross-feeding of multiple species and substances in the natural environment. In addition to ecological models, metabolic network models are used for the construction and subsequent regulation of synthetic microbial consortia. Flux balance analysis (FBA) is a typical constraint-based metabolic network model (Biggs et al., 2015; O’Brien et al., 2015; Biggs and Papin, 2017). It uses linear programming to determine the metabolic flux distribution of the reaction network based on gene annotations and biochemical reactions in the literature. FBA can optimize the metabolic network and predict gene knockouts expected to produce the best metabolic response (Burgard et al., 2003), providing guidance for the construction of synthetic microbial consortia based on cross-feeding.
Quorum sensing (QS) can also tune some intermediate metabolites for cross-feeding. Quorum sensing is a density-dependent microbial mechanism. When the concentration of a signaling substance reaches a threshold level, some microbial activities are activated and the phenotype changes. QS can regulate the secretion of extracellular digestive enzymes (Allen et al., 2016) to coordinate the cooperation of Pseudomonas aeruginosa. Guo et al. (2021) also found that some species can provide expensive metabolites to DSF-secreting species after the addition of exogenous diffusible signal factor (DSF) to the anammox microbial co-culture system.
Cross-feeding increases target yields and improves metabolic activity compared to those for single species. Cross-feeding combined with metabolic engineering can reconstitute heterologous metabolic pathways in different species and use a variety of tools and strategies to maximize metabolic flux of target products (Park et al., 2019). Metabolic engineering can optimize the operating efficiency of the synthetic consortium, and the combination of rapid product detection technology, metabolomics (Zhalnina et al., 2018a), isotope tracing (Gebreselassie and Antoniewicz, 2015) and other testing technologies can shorten the “design-build-test-learn (DBTL)” cycle time. Combining computational techniques with metabolic engineering can also shorten the period of the DBTL cycle. Nakazawa et al. (2021) developed a specific vocabulary model. The model searches relevant literature in the fields of synthetic biology and metabolic engineering to suggest next steps for genetic modification based on the relevance of the genetic modification history. This model can also be extended to the optimization of synthetic consortium DBTL loops.
Additionally, metabolic engineering can exploit the strengths and interactions of different species to improve productivity. Zhou et al. (2015) used E. coli and Saccharomyces cerevisiae to produce paclitaxel precursors, using the rapid growth of E. coli and the advanced protein expression mechanism of S. cerevisiae to improve the production efficiency of paclitaxel. Cross-feeding and co-cultivation can also improve the metabolic activity. Many bacteria enter a viable but not culturable (VBNC) state under adverse environmental conditions. In this state, the metabolic activity of microorganisms will be reduced and the function of the community will be affected (Wang et al., 2020). But co-cultivation and cross-feeding can convert some VBNC bacteria into a culturable state for them to function (Senoh et al., 2010).
Disadvantages of Synthetic Microbial Consortium Construction Based on Cross-Feeding
Although cross-feeding is commonly used for the construction of synthetic microbial consortia, this strategy has some disadvantages. These disadvantages are mainly related to the maintenance of the stability of the consortia.
First, although it is generally believed that cross-feeding is a cooperative interspecific relationship, there is still competition between species. Studies have pointed out that there are almost no examples of real interspecific cooperative relationships (De Mazancourt et al., 2005; Oliveira et al., 2014). As a typical example, Prochlorococcus provides a carbon source for the marine bacterium SAR11, but the species compete for sulfur resources (Becker et al., 2018).
Second, although cross-feeding is theorized to show multiple stable states (Vet et al., 2018), ecological stability does not indicate evolutionary-genetic stability (Miller and Klausmeier, 2017). Co-cultivation of species with cross-feeding relationships may lead to functional enhancement. Hillesland et al. (2014) cultured Desulfovibrio vulgaris with Methanococcus maripaludis and found that over the first 300 generations, the co-culture evolved higher compound yields and faster growth rates. However, co-cultivation can also lead to the breakdown of the cross-feeding system. The consortia based on amino acid cross-feeding (Wintermute and Silver, 2010) may be stable in the short term. However, since the output of amino acids is not fixed, when this output is insufficient during environmental selection (Kerner et al., 2012), the steady state will be destroyed. Mutations can also disrupt the stability of synthetic microbial consortia. Cheaters can easily arise by genetic mutations that produce functional gene deletions (Pande et al., 2014). Cheaters benefit from mutually beneficial and symbiotic interactions but cannot contribute to the system, ultimately leading to the collapse of the cross-feeding system. When cheaters have an advantage in the consumption of intermediate metabolites in cross-feeding, they may lead to the extinction of some species, including cross-feeding species (Sun et al., 2019).
The details of intermediate metabolites affect the function of the consortia. The production of intermediate metabolites may determine whether the metabolites are beneficial. LaSarre et al. (2017) used E. coli and photoheterotrophic Arabidopsis to construct a synthetic system based on cross-feeding. In this system, Arabidopsis produces NH4+ as an ammonia source and E. coli releases organic acids to provide carbon sources for Arabidopsis. However, when Arabidopsis thaliana secretes high levels of NH4+, it will stimulate E. coli to produce more toxic organic acids. This phenomenon is called dose-dependent toxicity. This phenomenon also exists in the microbial co-culture system. LaSarre et al. (2017) constructed an anaerobic co-culture system of fermentative E. coli and Rhodopseudomonas palustris, and the exchanged metabolites were carbon (organic acids) and nitrogen (ammonium). When R. palustris increased NH4+ production, E. coli organic acid production also increased. This results in acidification of the culture system, leading to an imbalance in species ratios and reduced carbon conversion efficiency. In addition, the feed the faster grower hypothesis (FEG) (Hammarlund et al., 2019) also suggests that when intermediate metabolites are added to a bidirectional cross-feeding system, the coexistence of consortium members depends on relative growth rates. If the faster-growing species loses its dependence on another species by utilizing alternative sources, the slow-growing species will die. In addition, intermediate metabolites may become toxic substances due to environmental factors, such as pH (Daims et al., 2016). In addition to intermediate metabolites, some inhibitors of the fermentation process also inhibit the efficiency of the synthetic consortium. The production of lactic acid from lignocellulose is a common function of synthetic consortia (Shahab et al., 2018). However, the process is challenging due to inhibitory by-products. They include phenolic compounds from lignin degradation, furan from sugar degradation, fatty acids, inorganic ions, and bioalcohols (Zhang et al., 2016). These compounds inhibit fermentation substantially by affecting cell growth and enzymatic activity, hindering lignocellulose utilization and efficient fermentation.
External interference also has an impact on synthetic microbial consortia based on cross-feeding. For example, due to the short-board effect, in a multidirectional cross-feeding system, antibiotic resistance of the consortia often depends on the most intolerant member (Adamowicz et al., 2018). It is also possible that when a key member of the consortium is disturbed and dies due to external interference, a mutually beneficial symbiosis will turn into a competitive relationship, leading to the collapse of the system (Hammarlund et al., 2021).
The concept of cross-feeding spans multiple disciplines, such as ecology and metabolomics. It is not easy to construct and maintain synthetic microbial consortia based on cross-feeding, and this approach requires insights from various perspectives.
Synthetic Microbial Consortia Construction—Inspiration From Community Ecology
A community is a complex of animals, plants, and microorganisms that inhabit the same area. Many ecological processes are often not completed by a species but by communities of multiple species acting together. Therefore, community ecology research is of great significance.
In studies of animal and plant communities, many theoretical models have been developed to explain ecological phenomena (Dt, 1982; Jetschke, 2002). However, due to complex interspecific relationships, high mutation rates, high rates of horizontal gene transfer (HGT), and other factors, microbial communities often show distinct characteristics from those of animal and plant communities (Head and Editor, 2007), making the application of theoretical models difficult. In particular, several key issues are as follows. (1) Many microorganisms cannot be cultivated in the laboratory, limiting our ability to test theoretical models. (2) Environmental conditions are important determinants of microbial communities (Wilpiszeski et al., 2020; Chen et al., 2022). (3) In animal and plant communities, population dynamics modeling is used to study relationships within the community matrix; however, when there are a large number of populations in the community, some model parameters are difficult to determine (Novack-Gottshall, 2000). This issue was also mentioned in our discussion of the construction of synthetic microbial consortia based on cross-feeding. Advances in molecular biology technologies have provided an opportunity to apply theories and methods developed for animal and plant communities to microbial communities, especially in microbial biogeography (Martiny et al., 2006). However, there are still limitations, including complex data processing (Raes and Bork, 2008), complex genotype–phenotype relationships (Temperton and Giovannoni, 2012), and complex interactions.
Treating the synthetic microbial consortia as a “community,” the phenotype of a component species may be different from that of the species cultivated separately. Additionally, unlike a single species, synthetic microbial consortia retain some of the complexity of natural communities and therefore can perform more functions than is possible by a species (Spus et al., 2015; Jones et al., 2017). The cross-feeding strategy is still the most common approach for constructing synthetic microbial consortia. As mentioned above, this strategy still has many disadvantages, mainly the difficulty in maintaining the stability of the synthetic consortium.
The synthetic consortium is similar to the natural community, and its long-term functional maintenance depends on its stability, i.e., the ability of the community to maintain or return to its original state after disturbance (Pimm, 1984). Stability itself is a multi-dimensional concept. Common indicators for stability include resistance stability and resilience stability. Resistance stability is the ability of a community to resist external disturbances and maintain its original structure and function. Resilience stability is the ability of a community to return to its original state after being damaged by external disturbance factors. Community stability is associated with species diversity, which also presents challenges for synthetic consortia because synthetic consortia are often the result of the simplification of species in natural communities. The reduction of species diversity leads to the loss of functional redundancy, which is the key to community functional stability (Shade et al., 2012). Additionally, different from natural communities, the functional stability of synthetic consortia is affected by several other factors. (1) Synthetic consortia tend to be cultured in larger numbers and for longer periods of time in industrial applications, leading to the loss of specific phenotypes (Rugbjerg et al., 2018). (2) Changes in environmental conditions during the cultivation process can reduce stability, particularly the accumulation of toxic products and changes in environmental pH. (3) Spatiotemporal heterogeneity of the availability in resources (such as nutrients and oxygen) can affect overall metabolism (Muller et al., 2010).
Because the construction method based on cross-feeding cannot well consider the stability of synthetic consortia, we refer to the emergence of many new theories and methods in community ecology in recent years, which provides new directions for the construction of synthetic microbial consortia.
Construction of Synthetic Microbial Consortia Based on Traits
Concept of Traits
Traits refer to the (phenotypic) characteristics that can be observed in individuals, including biochemical characteristics, cell morphology or dynamic processes, anatomical structures, organ functions, or behavioral characteristics (Violle et al., 2007). Traits can be classified according to their complexity. For example, some traits are controlled by a single gene and can be regarded as simple traits; other traits are controlled by multiple genes and may also be affected by the environment and these are referred to as complex traits (Frankham, 1996). Traits can also be classified into continuous traits, such as salt tolerance at different temperatures, and discrete traits, such as the ability of microorganisms to fix nitrogen or carbon dioxide. Traits can also be divided into response traits and effect traits (Webb et al., 2010). Response traits are related to the response of organisms to environmental changes (such as drought tolerance); effect traits refer to the impact of organisms on ecosystem processes (such as photosynthetic capacity). Additionally, functional traits are any morphological and physiological characteristics of microorganisms in a specific environment, such as size, reproduction time, or response to a certain antibiotic (McGill et al., 2006).
Trait-based methods are increasingly used in ecology to study ecological processes and community assemblies. They have been applied to studies of animals (Frimpong and Angermeier, 2010), higher plants (Van Bodegom et al., 2012), and plankton (Litchman and Klausmeier, 2008). Taking plants as an example, a trait-based method has been proposed (Van Bodegom et al., 2012) to describe global vegetation functions able to predict the continuous response of plant communities to multiple environmental variables. This method resolves the shortcomings of using plant function types (PFTs) to describe ecosystem functions. Compared with individual-based models commonly used in ecology, the trait-based model can better capture correlations between community functions and environmental changes (Zakharova et al., 2019). Kruk et al. (2021) used trait-based modeling to analyze how trait-related differences in species fitness affect phytoplankton species invasion.
Microbial ecologists have recently used trait-based models for plants or plankton for reference in microbial ecology research, providing a new framework for studying the geographic distribution, function, and trends of microbial communities (Wallenstein and Hall, 2011). Fungi were an initial research object. Chaudhary et al. (2020) collected morphological and functional traits of arbuscular mycorrhizal fungal spores to study the influence of random processes (diffusion) on fungal community structure. Studies have found that arbuscular mycorrhizal (AM) fungal spores in the air exhibit traits that are more conducive to airborne transmission (such as a smaller average spore size). Guittar et al. (2019) used a trait-based model to study the succession of the infant intestinal flora and found that the community composition was basically stable after one year. Predicted values for most traits become more accurate over time, indicating that succession is at least partially functionally deterministic. In studies of environmental microorganisms, trait-based methods have also been developed to predict primary productivity (Follows et al., 2007), metabolism (Hall et al., 2008), and litter decomposition (Allison, 2012). For example, enzyme kinetics and physiological traits have been used to represent ammonia-oxidizing bacteria (AOB), ammonia-oxidizing archaea (AOA), and nitrite-oxidizing bacteria (NOB). Trait-based modeling has been used to successfully predict various functional changes along environmental gradients (Bouskill et al., 2012).
Trait-based methods are of great significance in microbial ecology. This approach makes up for the shortcomings of analyses based on taxonomy, functional genes, or protein sequences, and more effectively associates microbial communities with ecosystem functions. However, microbial ecology based on traits is still in its infancy (Krause et al., 2014) for two primary reasons. First, it is difficult to define which traits are important. For many microorganisms, the key traits that determine environmental adaptability are still unclear. Second, it is difficult to quantify and characterize microbial traits. The metabolic diversity of bacteria, genomic plasticity, and trade-offs between traits make the simple division of environmental microorganisms into functional groups impossible. Furthermore, to study traits at the community level, the definition of community-weighted trait means (Violle et al., 2007) alone is far from sufficient because this may conceal local functional diversity.
New technologies have facilitated the measurement and quantification of microbial traits. For example, microbial culture technologies have been developed. Microfluidic technology (Sanati Nezhad, 2014) expands the range of microorganisms that can be cultivated, allowing us to obtain more information about the size, metabolic rate, and nutrient utilization of microorganisms. Isotope tracing methods include stable isotope tracing (Casey et al., 2007) and nano-SIMS (Behrens et al., 2012) and can be used to study the utilization of substrates by microorganisms at different scales. Raman spectroscopy (Huang et al., 2007) can reveal the chemical composition of cells. The development of sequencing technologies and omics methods has also been useful for trait-based research. Metabolomics (Li et al., 2021) can characterize the ability of microorganisms to utilize different substrates. Metagenomics (Wrighton et al., 2012) has improved our understanding of uncultivated microorganisms. Single-cell sequencing (Martinez-Garcia et al., 2012) can be used to study uncultured microorganisms and to accurately distinguish the genotype of each cell. However, it is important to account for the influence of the environment on phenotypes when using omics and sequencing technologies. Increasing studies have evaluated associations between microbial genotypes and phenotypes (Green et al., 2008; Martiny et al., 2015). In the near future, it may be possible to determine traits based on genotype data. A future goal is to establish a standardized trait database, like that available for plants, for easy access to information.
Application of Trait-Based Methods to Synthetic Microbial Consortia
The construction of synthetic microbial consortia involves many principles of microbial ecology. Regardless of whether we adopt a “top-down” or “bottom-up” strategy, it is necessary to determine the number of species that should be retained/assembled to maximize the function of the synthetic microbial consortia. Krause et al. (2014) concluded that diversity and functionality mainly involve three major modes of action: complementary effects, selection effects, and promotion effects (inhibitory effects). These mechanisms provide a basis for manipulating diversity, thereby altering community functionality. We also need to consider community stability. Functional redundancy may be the key to community stability (Louca et al., 2018). The construction of synthetic groups based on cross-feeding is typically only considered from a functional perspective and does not consider functional redundancy and stability. Triado-Margarit et al. (2019) used a trait-based model to determine an environmental threshold, above which community traits tend to be stable, there is indicating that there is functional redundancy. This case uses the definition of traits to better understand the end point (steady state) of community assembly.
Research in microbial ecology is traditionally based on taxonomy, with the classification of groups based on the current species definition (Schleifer, 2009). With the development of molecular biotechnology, methods based on functional genes or protein sequences have been applied to microbial ecological research. However, such methods are often susceptible to the plasticity of traits, leading to differences in genotype and phenotype (Coutinho et al., 2016). Salles et al. (2009) found that the complementary effects of traits relative to taxa or gene or protein sequences can better predict denitrification performance. This indicates that trait-based methods may better describe or predict the functions of the community than traditional taxonomy-based methods.
Combining the above two points, our strategy for the construction of synthetic microbial consortia based on traits is to select an appropriate combination of features according to the final functional requirements and manipulate diversity to complete the assembly of the consortia. There is an example of this approach in plants (Wanapaisan et al., 2018), in which a model was built based on three traits (i.e., thick bark, dense wood, and a moderate leaf nitrogen concentration) and post-disaster restoration of mountain forests in western North America was carried out by controlling the number of species and introducing specific new species. It is even possible to establish a species library and select taxa with appropriate traits from this library according to the function of the synthetic microbial consortia. Figure 3 summarizes the process of constructing synthetic microbial consortia based on trait models.
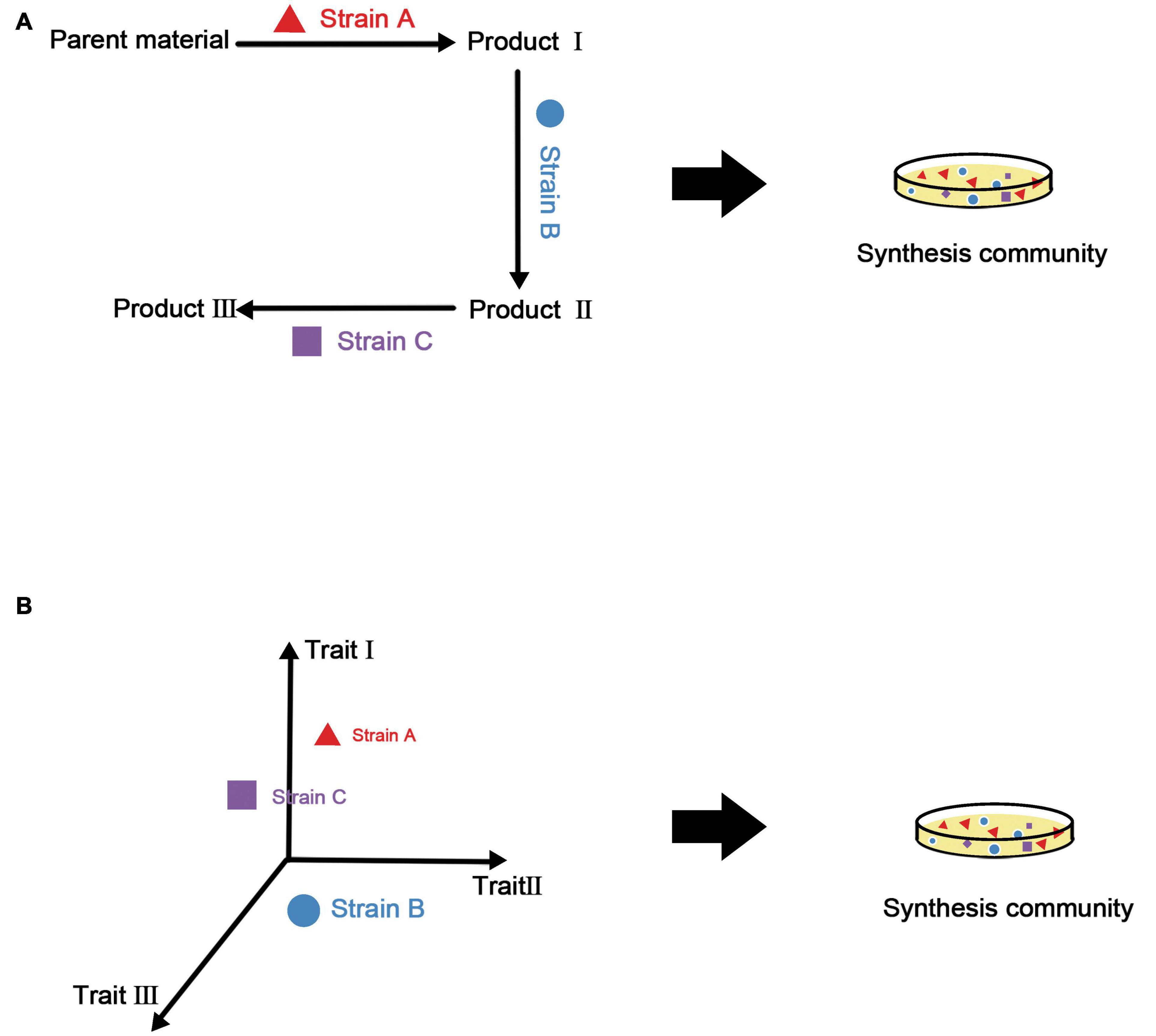
Figure 3. Construction methods for synthetic microbial consortia based on metabolic associations and traits. (A) Using metabolic associations, metabolites of various strains are utilized to build a complete metabolic network. Although the functionality of the synthetic microbial consortia may be improved under this method, it is difficult to evaluate the stability of the consortia. (B) The trait-based method uses a multi-dimensional model from the perspective of ecological niches to obtain synthetic microbial consortia with good stability and functionality.
Not only for the design and construction phase of synthetic microbial consortia but also for the regulation of synthetic microbial consortia, trait-based models are a good tool. Trait-based methods can use the functional traits of a community in a specific environment as a basis to predict the community’s response to specific environmental changes. Le Roux et al. (2016) used a trait-based model to classify soil NOB into several groups determined by physiological traits, and predicted that NOB will affect three climatic factors (CO2 increase, precipitation, and nitrogen addition). Using trait-based models, we can rationally regulate synthetic microbial consortia by adjusting environmental factors. Although trait-based models cover multiple dimensions, there are some current techniques improve the construction of a trait-based synthetic consortium, such as machine learning (Mallick et al., 2019), metabolomics (Zhalnina et al., 2018a) and laboratory ecosystems (Zhalnina et al., 2018b). Mallick et al. (2019) used machine learning to predict metabolites that might not be observed in microbial communities. Metabolomic characteristics of microbial communities are inferred from metagenomes based on the MelonnPan computational framework, successfully predicting more than 50% of metabolites at the community level. Based on computational models, we can better combine a set of optimal microbial traits and infer the metabolites of synthetic consortia.
Constructing Synthetic Microbial Consortia Based on Spatial Structure
Species composition and structure are two basic characteristics of a community. When understanding microbial communities, we often focus on the community composition, such as species diversity and interactions. In recent years, synthetic microbial consortia have been constructed based on interactions between species (Yurtsev et al., 2016; Li et al., 2017; Ren et al., 2018). However, increasing studies have shown that spatial structure is equally important for the function of microbial communities (Kim et al., 2008).
Concept of Spatial Structure
The spatial structure of the community refers to the distribution of the population in the community and the spatial arrangement of its attributes (Ledo et al., 2014). It reflects the interactions among individuals in horizontal space and is a universal characteristic of the community. The spatial structure is the result of the combined effects of population biological characteristics, intra-specific and inter-specific interactions, and environmental conditions (Druckenbrod et al., 2005). There are some key strategies for constructing community spatial structure: the self-assembly of microorganisms, i.e., the “differential adhesion hypothesis” (Steinberg, 1975, 2007) and construction strategies based on cell movement, cell morphological changes, etc. (Figure 4).
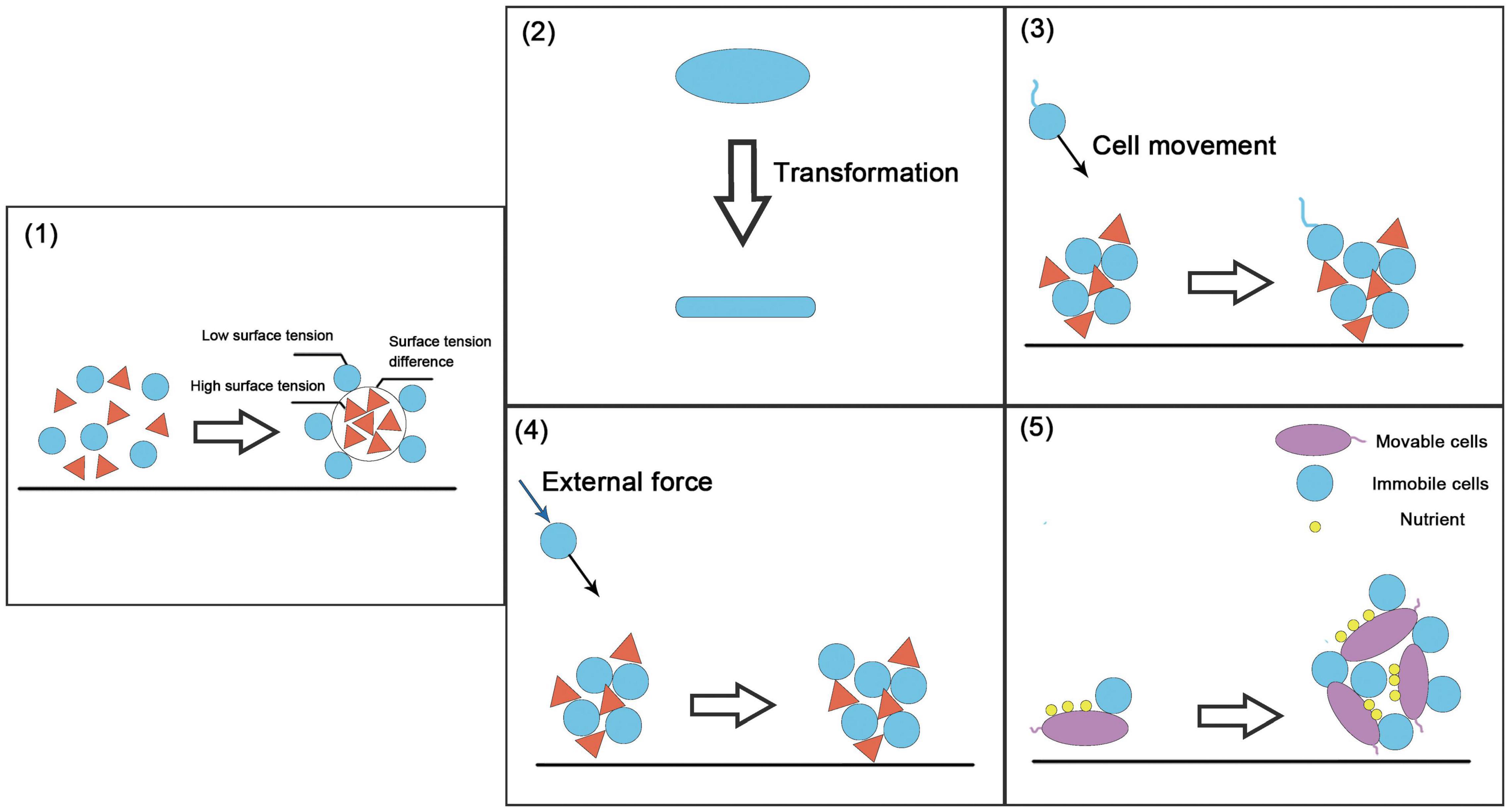
Figure 4. Examples of spatial structure-based construction strategies. (1) Self-organized construction strategy (i.e., the differential adhesion hypothesis), in which the difference in surface tension and interfacial tension between moving cells produces selective adhesion. (2) Changes in cell shape. (3) Movable cells produce specific movements under the action of environmental information. (4) Immovable cells move or diffuse with the help of external environmental forces such as liquid flow. (5) Immovable cells move via movable cells.
Spatial stratification mainly includes two categories in natural communities: resistance to stress and changes in interactions. The spatial structure constructed by multiple species is often stronger than that of a species in these two aspects. For example, compared with the species, the seawater biofilm community is more resistant to antibiotics (Burmolle et al., 2006). In the soil environment, the spatial structure affects the interaction between microorganisms, which in turn affects many phenomena, such as nitrification (Ebrahimi and Or, 2016). It should be noted that the spatial structure can also change metabolite exchange into cross-feeding (Liu et al., 2017), allowing us to simultaneously incorporate cross-feeding approaches to better construct synthetic microbial consortia. The spatial structure of the community helps us understand the structure and function of the community and dynamic population processes and reveals the mechanism underlying the maintenance of species diversity in the natural community. In higher plant and animal ecology, spatial structure is widely used in ecosystem restoration and maintenance.
In the laboratory, microbial systems that can be cultivated are very limited. Many microorganisms cannot produce stable communities when mixed well. This can be explained by unbalanced competition between species for nutrients (Kim et al., 2008). This further demonstrates that the functionality of the microbial community is not only related to diversity but also to the spatial structure of the community (Liu et al., 2016; Pandin et al., 2017).
Compared with higher plant communities, microbial communities are more complex and difficult to observe, with no obvious vertical and horizontal structure. Another key issue in spatial ecology is the scale of analysis. The scale is either too small to characterize the complete community structure or too large to find local characteristics. Technological advances have provided additional means to study the micro-scale spatial structure of microorganisms in the laboratory. Microfluidic technology is an emerging method to study the micro-scale spatial structure of microorganisms. Microfluidic technology can accurately control environmental parameters within a small range and, combined with microscopic imaging technology, can be used to observe biological processes in real time (Sanati Nezhad, 2014). Massalha et al. (2017) used microfluidic-based technology to study the chemotactic behavior of bacteria relative to plant roots. Combined labeling and spectral imaging fluorescence in situ hybridization (CIASI-FISH) can simultaneously identify and locate different microorganisms and further determine the distribution of a certain species. This method extends the observation scale to the community (Wilbert et al., 2020).
Application of Spatial Structure in Synthetic Microbial Consortia
The micro-scale spatial structure in the natural environment is considered very important for microbial ecology (Treves et al., 2003; Leibold et al., 2004). In the natural environment, bacteria are affected by a series of physical, chemical, and biological factors on the microscale. They ultimately affect some traits on the community scale by affecting the diffusion of bacteria and nutrients (Konig et al., 2020). Although artificial environments created in the laboratory are not as complex as the natural environment, spatial structure is still an important factor. Synthetic microbial consortia constructed by a “bottom-up” strategy can be assembled with different metabolic divisions of bacteria to build a complete metabolic network with certain functions. However, in the actual process of constructing synthetic microbial consortia, the consortia may not perform the ideal function. Because the consortia are affected by species competition, the uniformity of nutrients, and the transmission of signaling molecules and other factors, they cannot grow in a well-mixed condition. Vallespir Lowery and Ursell (2019) used an improved Lotka–Volterra model in a two-dimensional environment to prove that although two microorganisms cannot coexist under homogenized conditions, they can coexist in a structured environment. Kim et al. (2008) constructed synthetic microbial consortia composed of three kinds of bacteria with nutritional interactions; they found that stability is low when the mixture is good but observed stable coexistence after separation and cultivation using a microfluidic device. This indicates that the micro-scale spatial structure is necessary for the coexistence of species in synthetic microbial consortia. This study also divides the microbial consortia into three levels. The first-level consortium is unstable when it loses its metabolic coupling, that is, it is most stable under uniformly mixed conditions. The second-level consortium is unstable when well mixed, but when the species separate spatially, the consortium gradually becomes stable until the maximum separation distance is reached. The third-level consortium is unstable, irrespective of spatial distance. To build stable synthetic microbial consortium, it may be necessary to determine the spatial distance threshold to maintains the stable coexistence of consortium members.
There are many ways to control the spatial structure of natural communities, and these methods can provide a reference for the control of the spatial structure of synthetic microbial consortia. First, the spatial structure is often generated based on some kind of information in the environment. The most common is the concentrations of metabolites. Guo et al. (2018) found that cells maintain a desired spatial structure based on the local concentration of metabolites. Exopolysaccharide (EPS) promotes aggregation in the biofilm, and changing the EPS concentration can directly trigger a change in the biofilm spatial structure (Ziemba et al., 2016). In addition to directly altering community spatial structure by changing EPS concentrations, chemotaxis is equally important for spatial structure. Chemotaxis is a motor behavioral response of bacteria driven by changes in the concentration of chemical substances in the environment (Berg, 1975). Chemotaxis plays an important role in both rhizosphere microbial colonization and pollutant degradation. Moisture is a common chemotaxis-based regulator. Azospirillum brasilense and Pseudomonas fluorescens migrate to roots at a faster rate with increasing soil water contents by chemotaxis (Bashan, 1986). The O2 concentration is also a common regulatory factor. Koza et al. (2011) found that wild Pseudomonas fluorescens SBW25 can construct an O2 gradient and generate spatial stratification according to different O2 concentrations. For some multi-species populations based on cross-feeding, the spatial organization is driven by metabolite exchange. Therefore, changing the concentration of intermediate metabolites may change the original community structure. Using an ecological approach to change specific species in a community can also change the spatial structure. In a typical example, spraying Bacillus subtilis can change the spatial organization of the Aspergillus flavus biofilm on citrus leaves (Huang et al., 2012). In a four-component population of Stenotrophomonas rhizophila, Xanthomonas retroflexus, Microbacterium oxydans, and Paenibacillus amylolyticus, the least abundant M. oxydans is the key species influencing the spatial structure of the community (Liu et al., 2017). QS is also associated with various processes, such as EPS production and biofilm formation, and can also be used to modulate the spatial structure of microorganisms (Zhang et al., 2012; Ma et al., 2018). We can promote the aggregation of specific microorganisms by rationally regulating QS signaling substances. N-Butyryl-L-homoserine lactone (C4-HSL) has been detected in syntrophic Geobacter communities, which can promote aggregate formation among different species (Wei et al., 2017). Bordeleau et al. (2015) found that microbial type IV pili gene transcription is enhanced by the signaling molecule cyclic diguanosine monophosphate (c-di-GMP), which promotes Clostridium difficile cell aggregation.
Some physical methods can be used to directly construct the ideal spatial structure of a biological community. Microfluidics is commonly used to control the spatial structure of microorganisms. Alnahhas et al. used a microfluidic device to control the temporal dynamics of the spatial distribution of two different E. coli strains. This study used two cell capture areas of different sizes to control the dynamic ratio of the two strains (Alnahhas et al., 2019). Optical tweezers can capture cells at the single-cell level for precise three-dimensional control (Dholakia and Čižmár, 2011). Miccio et al. controlled the orientation of E. coli by light-induced dielectrophoresis (Miccio et al., 2016). 3D printing technology provides the possibility of artificially constructing the spatial structure of a community, which is often not possible by traditional bioreactors (Rupal et al., 2019). This technology has been applied to mammalian cells. Grix et al. (2018) used 3D printing technology to construct a sinusoidal liver lobule model. The model shows higher protein expression than that in traditional monolayer culture. In environmental sciences, 3D printing technology can be used to build more efficient bioreactors. Elliott et al. (2017) designed a moving bed biofilm reactor (MBBR) with a high specific surface area using 3D printing technology combined with mathematical modeling. However, research on 3D printing technology in environmental sciences has focused on improving the physical and chemical properties of the reactor and has not considered factors such as metabolic coupling. When constructing synthetic microbial consortia, a feasible approach may be modeling and designing the spatial structure of a two-bacteria or even a multi-bacterial combination system and using 3D printing to make a suitable carrier to obtain synthetic microbial consortia or bioreactor with a three-dimensional structure (Figure 5).
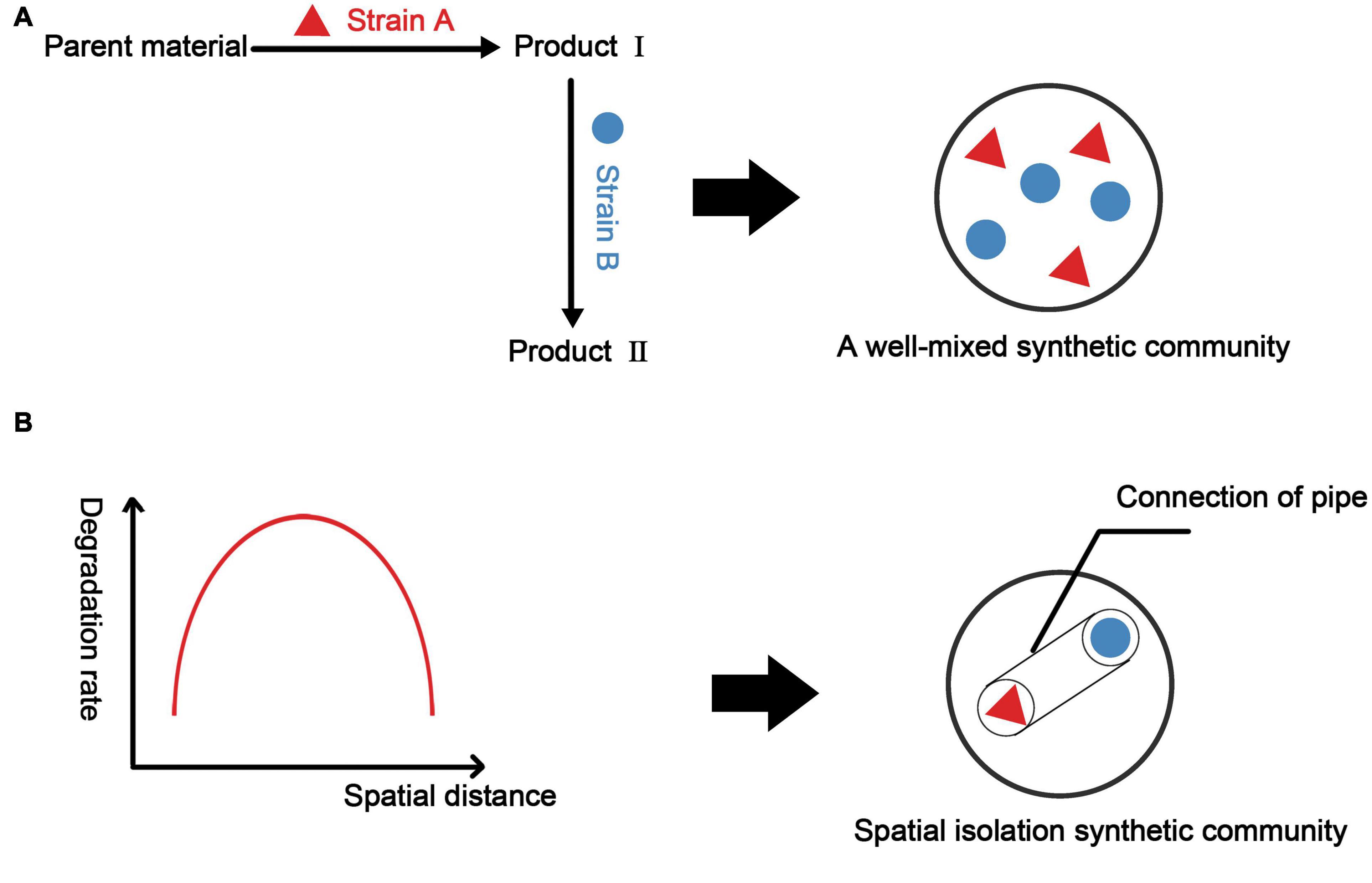
Figure 5. Construction methods for synthetic microbial consortia based on metabolic associations and spatial structure. (A) Considering only the metabolic network to construct synthetic microbial consortia, we often obtain well-mixed synthetic microbial consortia. Here, we take two strains as examples. (B) Considering the spatial structure, it is assumed that there is a function relating consortium performance to the spatial distance between two strains (in fact, it may be a more complicated spatial model). Based on this function (model), we select the spatial distance with the best consortia performance and construct a vector that can ensure the metabolic communication between the strains by controlling the spatial distance between the two strains.
Conclusion and Future Perspectives
The concept of synthetic biology has expanded. It is no longer limited to assembling DNA or organelles. Strains can be used as components to assemble and ultimately build functional consortia. The effective synthetic microbial consortia may show improved survival rates and functions in the natural environment than those of a species. However, the assembly process and underlying principle are more complicated because synthetic microbial consortia are constructed based on laws governing natural communities. It is necessary to consider many factors, including metabolic networks, spatial structure, and stability. In addition to engineering principles, we may also draw inspiration from ecology and apply these insights to the construction of synthetic microbial consortia.
Author Contributions
YL prepared the draft of manuscript. AM and GZ edited the manuscript. All authors contributed to the article and approved the submitted version.
Funding
This work was supported by the National Key Research and Development Program of China (2018YFA0901200), the Second Tibetan Plateau Scientific Expedition and Research Program (2019QZKK0402 and 2019QZKK0307), and Science and Technology Service Network Initiative (KFJ-EW-STS-140).
Conflict of Interest
The authors declare that the research was conducted in the absence of any commercial or financial relationships that could be construed as a potential conflict of interest.
Publisher’s Note
All claims expressed in this article are solely those of the authors and do not necessarily represent those of their affiliated organizations, or those of the publisher, the editors and the reviewers. Any product that may be evaluated in this article, or claim that may be made by its manufacturer, is not guaranteed or endorsed by the publisher.
References
Adamowicz, E. M., Flynn, J., Hunter, R. C., and Harcombe, W. R. (2018). Cross-feeding modulates antibiotic tolerance in bacterial communities. ISME J. 12, 2723–2735. doi: 10.1038/s41396-018-0212-z
Ahmad, F., Anwar, S., Firdous, S., Da-Chuan, Y., and Iqbal, S. (2018). Biodegradation of bispyribac sodium by a novel bacterial consortium BDAM: optimization of degradation conditions using response surface methodology. J. Hazard Mater. 349, 272–281. doi: 10.1016/j.jhazmat.2017.12.065
Allen, R. C., McNally, L., Popat, R., and Brown, S. P. (2016). Quorum sensing protects bacterial co-operation from exploitation by cheats. ISME J. 10, 1706–1716. doi: 10.1038/ismej.2015.232
Allison, S. D. (2012). A trait-based approach for modelling microbial litter decomposition. Ecol. Lett. 15, 1058–1070. doi: 10.1111/j.1461-0248.2012.01807.x
Alnahhas, R. N., Winkle, J. J., Hirning, A. J., Karamched, B., Ott, W., Josic, K., et al. (2019). Spatiotemporal dynamics of synthetic microbial consortia in microfluidic devices. ACS Synth. Biol. 8, 2051–2058. doi: 10.1021/acssynbio.9b00146
Anees, M., Qayyum, A., Jamil, M., Rehman, F. U., Abid, M., Malik, M. S., et al. (2020). Role of halotolerant and chitinolytic bacteria in phytoremediation of saline soil using spinach plant. Int. J. Phytoremed. 22, 653–661. doi: 10.1080/15226514.2019.1707160
Bashan, Y. (1986). Migration of the rhizosphere bacteria Azospirillum brusilense and Pseudomonas fluorescens towards wheat roots in the soil. Microbiology 132, 3407–3414. doi: 10.1099/00221287-132-12-3407
Becker, J. W., Hogle, S. L., Rosendo, K., and Chisholm, S. W. (2018). Co-culture and biogeography of Prochlorococcus and SAR11. ISME J. 13, 1506–1519. doi: 10.1101/460428
Behrens, S., Kappler, A., and Obst, M. (2012). Linking environmental processes to the in situ functioning of microorganisms by high-resolution secondary ion mass spectrometry (NanoSIMS) and scanning transmission X-ray microscopy (STXM). Environ. Microbiol. 14, 2851–2869. doi: 10.1111/j.1462-2920.2012.02724.x
Berg, H. C. (1975). Chemotaxis in bacteria. Annu. Rev. Biophys. Bioeng. 4, 119–136. doi: 10.1146/annurev.bb.04.060175.001003
Bernstein, H. C., Paulson, S. D., and Carlson, R. P. (2012). Synthetic Escherichia coli consortia engineered for syntrophy demonstrate enhanced biomass productivity. J. Biotechnol. 157, 159–166. doi: 10.1016/j.jbiotec.2011.10.001
Biggs, M. B., and Papin, J. A. (2017). Managing uncertainty in metabolic network structure and improving predictions using EnsembleFBA. PLoS Comput. Biol. 13:e1005413. doi: 10.1371/journal.pcbi.1005413
Biggs, M. B., Medlock, G. L., Kolling, G. L., and Papin, J. A. (2015). Metabolic network modeling of microbial communities. Wiley Interdiscip. Rev. Syst. Biol. Med. 7, 317–334. doi: 10.1002/wsbm.1308
Bordeleau, E., Purcell, E. B., Lafontaine, D. A., Fortier, L. C., Tamayo, R., and Burrus, V. (2015). Cyclic di-GMP riboswitch-regulated type IV pili contribute to aggregation of Clostridium difficile. J. Bacteriol. 197, 819–832. doi: 10.1128/JB.02340-14
Bouskill, N. J., Tang, J., Riley, W. J., and Brodie, E. L. (2012). Trait-based representation of biological nitrification: model development, testing, and predicted community composition. Front. Microbiol. 3:364. doi: 10.3389/fmicb.2012.00364
Burgard, A. P., Pharkya, P., and Maranas, C. D. (2003). Optknock: a bilevel programming framework for identifying gene knockout strategies for microbial strain optimization. Biotechnol. Bioeng. 84, 647–657. doi: 10.1002/bit.10803
Burmolle, M., Webb, J. S., Rao, D., Hansen, L. H., Sorensen, S. J., and Kjelleberg, S. (2006). Enhanced biofilm formation and increased resistance to antimicrobial agents and bacterial invasion are caused by synergistic interactions in multispecies biofilms. Appl. Environ. Microbiol. 72, 3916–3923. doi: 10.1128/AEM.03022-05
Casey, J. R., Lomas, M. W., Mandecki, J., and Walker, D. E. (2007). Prochlorococcus contributes to new production in the Sargasso Sea deep chlorophyll maximum. Geophys. Res. Lett. 34:L10604. doi: 10.1029/2006gl028725
Chang, C. Y., Vila, J. C. C., Bender, M., Li, R., Mankowski, M. C., Bassette, M., et al. (2021). Engineering complex communities by directed evolution. Nat. Ecol. Evol. 5, 1011–1023. doi: 10.1038/s41559-021-01457-5
Chaudhary, V. B., Nolimal, S., Sosa-Hernandez, M. A., Egan, C., and Kastens, J. (2020). Trait-based aerial dispersal of arbuscular mycorrhizal fungi. New Phytol. 228, 238–252. doi: 10.1111/nph.16667
Chen, J., Liu, H., Bai, Y., Qi, J., Qi, W., Liu, H., et al. (2022). Mixing regime shapes the community assembly process, microbial interaction and proliferation of cyanobacterial species Planktothrix in a stratified lake. J. Environ. Sci. (China) 115, 103–113. doi: 10.1016/j.jes.2021.07.001
Chen, Y., Li, C., Zhou, Z., Wen, J., You, X., Mao, Y., et al. (2014). Enhanced biodegradation of alkane hydrocarbons and crude oil by mixed strains and bacterial community analysis. Appl. Biochem. Biotechnol. 172, 3433–3447. doi: 10.1007/s12010-014-0777-6
Coutinho, R. M., Klauschies, T., and Gaedke, U. (2016). Bimodal trait distributions with large variances question the reliability of trait-based aggregate models. Theor. Ecol. 9, 389–408. doi: 10.1007/s12080-016-0297-9
Coyte, K. Z., Schluter, J., and Foster, K. R. (2015). The ecology of the microbiome: networks, competition, and stability. Science 350, 663–666. doi: 10.1126/science.aad2602
Daims, H., Lucker, S., and Wagner, M. (2016). A new perspective on microbes formerly known as nitrite-oxidizing bacteria. Trends Microbiol. 24, 699–712. doi: 10.1016/j.tim.2016.05.004
De Mazancourt, C., Loreau, M., and Dieckmann, U. L. F. (2005). Understanding mutualism when there is adaptation to the partner. J. Ecol. 93, 305–314. doi: 10.1111/j.0022-0477.2004.00952.x
De Roy, K., Marzorati, M., Van den Abbeele, P., Van de Wiele, T., and Boon, N. (2014). Synthetic microbial ecosystems: an exciting tool to understand and apply microbial communities. Environ. Microbiol. 16, 1472–1481. doi: 10.1111/1462-2920.12343
Dejonghe, W., Berteloot, E., Goris, J., Boon, N., Crul, K., Maertens, S., et al. (2003). Synergistic degradation of linuron by a bacterial consortium and isolation of a single linuron-degrading variovorax strain. Appl. Environ. Microbiol. 69, 1532–1541. doi: 10.1128/AEM.69.3.1532-1541.2003
Dholakia, K., and Čižmár, T. (2011). Shaping the future of manipulation. Nat. Photonics 5, 335–342. doi: 10.1038/nphoton.2011.80
Diaz-Garcia, L., Huang, S., Sproer, C., Sierra-Ramirez, R., Bunk, B., Overmann, J., et al. (2021). Dilution-to-stimulation/extinction method: a combination enrichment strategy to develop a minimal and versatile lignocellulolytic bacterial consortium. Appl. Environ. Microbiol. 87:e02427–20. doi: 10.1128/AEM.02427-20
Druckenbrod, D. L., Shugart, H. H., and Davies, I. (2005). Spatial pattern and process in forest stands within the Virginia piedmont. J. Veg. Sci. 16, 37–48. doi: 10.1111/j.1654-1103.2005.tb02336.x
D’Souza, G., and Kost, C. (2016). Experimental evolution of metabolic dependency in bacteria. PLoS Genet. 12:e1006364. doi: 10.1371/journal.pgen.1006364
Dvorak, P., Bidmanova, S., Damborsky, J., and Prokop, Z. (2014). Immobilized synthetic pathway for biodegradation of toxic recalcitrant pollutant 1,2,3-trichloropropane. Environ. Sci. Technol. 48, 6859–6866. doi: 10.1021/es500396r
Ebrahimi, A., and Or, D. (2016). Microbial community dynamics in soil aggregates shape biogeochemical gas fluxes from soil profiles – upscaling an aggregate biophysical model. Glob. Chang Biol. 22, 3141–3156. doi: 10.1111/gcb.13345
Elliott, O., Gray, S., McClay, M., Nassief, B., Nunnelley, A., Vogt, E., et al. (2017). Design and manufacturing of high surface area 3D-printed media for moving bed bioreactors for wastewater treatment. J. Contemp. Water Res. Educ. 160, 144–156. doi: 10.1111/j.1936-704X.2017.03246.x
Emery, S. M., and Gross, K. L. (2007). Dominant species identity, not community evenness, regulates invasion in experimental grassland plant communities. Ecology 88, 954–964. doi: 10.1890/06-0568
Follows, M. J., Dutkiewicz, S., Grant, S., and Chisholm, S. W. (2007). Emergent biogeography of microbial communities in a model ocean. Science 315, 1843–1846. doi: 10.1126/science.1138544
Frankham, R. (1996). Introduction to quantitative genetics (4th edn). Trends Genet. 12:280. doi: 10.1016/0168-9525(96)81458-2
Frimpong, E., and Angermeier, P. (2010). Trait-Based Approaches in the Analysis of Stream Fish Communities. Bethesda, MD: American Fisheries Society, 109–136.
Fritts, R. K., McCully, A. L., and McKinlay, J. B. (2021). Extracellular metabolism sets the table for microbial cross-feeding. Microbiol. Mol. Biol. Rev. 85:e00135–20. doi: 10.1128/MMBR.00135-20
Gebreselassie, N. A., and Antoniewicz, M. R. (2015). (13)C-metabolic flux analysis of co-cultures: a novel approach. Metab. Eng. 31, 132–139. doi: 10.1016/j.ymben.2015.07.005
Gilmore, S. P., Lankiewicz, T. S., Wilken, S. E., Brown, J. L., Sexton, J. A., Henske, J. K., et al. (2019). Top-down enrichment guides in formation of synthetic microbial consortia for biomass degradation. ACS Synth. Biol. 8, 2174–2185. doi: 10.1021/acssynbio.9b00271
Giri, S., Waschina, S., Kaleta, C., and Kost, C. (2019). Defining division of labor in microbial communities. J. Mol. Biol. 431, 4712–4731. doi: 10.1016/j.jmb.2019.06.023
Green, J. L., Bohannan, B. J., and Whitaker, R. J. (2008). Microbial biogeography: from taxonomy to traits. Science 320, 1039–1043. doi: 10.1126/science.1153475
Grix, T., Ruppelt, A., Thomas, A., Amler, A. K., Noichl, B. P., Lauster, R., et al. (2018). Bioprinting perfusion-enabled liver equivalents for advanced organ-on-a-chip applications. Genes (Basel) 9:176. doi: 10.3390/genes9040176
Grosskopf, T., and Soyer, O. S. (2014). Synthetic microbial communities. Curr. Opin. Microbiol. 18, 72–77. doi: 10.1016/j.mib.2014.02.002
Guittar, J., Shade, A., and Litchman, E. (2019). Trait-based community assembly and succession of the infant gut microbiome. Nat. Commun. 10:512. doi: 10.1038/s41467-019-08377-w
Guo, Y., Tikhonov, M., and Brenner, M. P. (2018). Local growth rules can maintain metabolically efficient spatial structure throughout growth. Proc. Natl. Acad. Sci. U.S.A. 115, 3593–3598. doi: 10.1073/pnas.1801853115
Guo, Y., Zhao, Y., Tang, X., Na, T., Pan, J., Zhao, H., et al. (2021). Deciphering bacterial social traits via diffusible signal factor (DSF) -mediated public goods in an anammox community. Water Res. 191:116802. doi: 10.1016/j.watres.2020.116802
Hall, E. K., Neuhauser, C., and Cotner, J. B. (2008). Toward a mechanistic understanding of how natural bacterial communities respond to changes in temperature in aquatic ecosystems. ISME J. 2, 471–481. doi: 10.1038/ismej.2008.9
Hammarlund, S. P., Chacon, J. M., and Harcombe, W. R. (2019). A shared limiting resource leads to competitive exclusion in a cross-feeding system. Environ. Microbiol. 21, 759–771. doi: 10.1111/1462-2920.14493
Hammarlund, S. P., Gedeon, T., Carlson, R. P., and Harcombe, W. R. (2021). Limitation by a shared mutualist promotes coexistence of multiple competing partners. Nat. Commun. 12:619. doi: 10.1038/s41467-021-20922-0
Head, I., and Editor, J. (2007). Microorganisms, macroorganisms and ecology. Proceedings of a joint symposium of the society for general microbiology, environmental microbiology group, and the british ecological society September 13, 2006 York, united kingdom. FEMS Microbiol. Ecol. 62, 133–232. doi: 10.1111/j.1574-6941.2007.00395.x
Hillesland, K. L., Lim, S., Flowers, J. J., Turkarslan, S., Pinel, N., Zane, G. M., et al. (2014). Erosion of functional independence early in the evolution of a microbial mutualism. Proc. Natl. Acad. Sci. U.S.A. 111, 14822–14827. doi: 10.1073/pnas.1407986111
Huang, T. P., Tzeng, D. D., Wong, A. C., Chen, C. H., Lu, K. M., Lee, Y. H., et al. (2012). DNA polymorphisms and biocontrol of Bacillus antagonistic to citrus bacterial canker with indication of the interference of phyllosphere biofilms. PLoS One 7:e42124. doi: 10.1371/journal.pone.0042124
Huang, W. E., Stoecker, K., Griffiths, R., Newbold, L., Daims, H., Whiteley, A. S., et al. (2007). Raman-FISH: combining stable-isotope Raman spectroscopy and fluorescence in situ hybridization for the single cell analysis of identity and function. Environ. Microbiol. 9, 1878–1889. doi: 10.1111/j.1462-2920.2007.01352.x
Jetschke, G. (2002). The unified neutral theory of biodiversity and biogeography. Ecology 83, 1771–1772.
Jones, J. A., Vernacchio, V. R., Collins, S. M., Shirke, A. N., Xiu, Y., Englaender, J. A., et al. (2017). Complete biosynthesis of anthocyanins using E. coli polycultures. mBio 8:e00621–17. doi: 10.1128/mBio.00621-17
Kerner, A., Park, J., Williams, A., and Lin, X. N. (2012). A programmable Escherichia coli consortium via tunable symbiosis. PLoS One 7:e34032. doi: 10.1371/journal.pone.0034032
Kim, H. J., Boedicker, J. Q., Choi, J. W., and Ismagilov, R. F. (2008). Defined spatial structure stabilizes a synthetic multispecies bacterial community. Proc. Natl. Acad. Sci. U.S.A. 105, 18188–18193. doi: 10.1073/pnas.0807935105
Kitney, R., and Freemont, P. (2012). Synthetic biology – the state of play. FEBS Lett. 586, 2029–2036. doi: 10.1016/j.febslet.2012.06.002
Klitgord, N., and Segre, D. (2011). Ecosystems biology of microbial metabolism. Curr. Opin. Biotechnol. 22, 541–546. doi: 10.1016/j.copbio.2011.04.018
Konig, S., Vogel, H. J., Harms, H., and Worrich, A. (2020). Physical, chemical and biological effects on soil bacterial dynamics in microscale models. Front. Ecol. Evol. 8:53. doi: 10.3389/fevo.2020.00053
Koza, A., Moshynets, O., Otten, W., and Spiers, A. J. (2011). Environmental modification and niche construction: developing O2 gradients drive the evolution of the Wrinkly Spreader. ISME J. 5, 665–673. doi: 10.1038/ismej.2010.156
Krause, S., Le Roux, X., Niklaus, P. A., Van Bodegom, P. M., Lennon, J. T., Bertilsson, S., et al. (2014). Trait-based approaches for understanding microbial biodiversity and ecosystem functioning. Front. Microbiol. 5:251. doi: 10.3389/fmicb.2014.00251
Krieger, A. G., Zhang, J., and Lin, X. N. (2021). Temperature regulation as a tool to program synthetic microbial community composition. Biotechnol. Bioeng. 118, 1381–1392. doi: 10.1002/bit.27662
Kruk, C., Piccini, C., Devercelli, M., Nogueira, L., Accattatis, V., Sampognaro, L., et al. (2021). A trait-based approach predicting community assembly and dominance of microbial invasive species. Oikos 130, 571–586. doi: 10.1111/oik.07694
LaSarre, B., Deutschbauer, A. M., Love, C. E., and McKinlay, J. B. (2020). Covert cross-feeding revealed by genome-wide analysis of fitness determinants in a synthetic bacterial mutualism. Appl. Environ. Microbiol. 86:e00543–20. doi: 10.1128/AEM.00543-20
LaSarre, B., McCully, A. L., Lennon, J. T., and McKinlay, J. B. (2017). Microbial mutualism dynamics governed by dose-dependent toxicity of cross-fed nutrients. ISME J. 11, 337–348. doi: 10.1038/ismej.2016.141
Lawson, C. E., Harcombe, W. R., Hatzenpichler, R., Lindemann, S. R., Loffler, F. E., O’Malley, M. A., et al. (2019). Common principles and best practices for engineering microbiomes. Nat. Rev. Microbiol. 17, 725–741. doi: 10.1038/s41579-019-0255-9
Le Roux, X., Bouskill, N. J., Niboyet, A., Barthes, L., Dijkstra, P., Field, C. B., et al. (2016). Predicting the responses of soil nitrite-oxidizers to multi-factorial global change: a trait-based approach. Front. Microbiol. 7:628. doi: 10.3389/fmicb.2016.00628
Ledo, A., Canellas, I., Barbeito, I., Gordo, F. J., Calama, R. A., and Gea-Izquierdo, G. (2014). Species coexistence in a mixed Mediterranean pine forest: spatio-temporal variability in trade-offs between facilitation and competition. For. Ecol. Manag. 322, 89–97. doi: 10.1016/j.foreco.2014.02.038
Lee, J. A., Baugh, A. C., Shevalier, N. J., Strand, B., Stolyar, S., and Marx, C. J. (2021). Cross-feeding of a toxic metabolite in a synthetic lignocellulose-degrading microbial community. Microorganisms 9:321. doi: 10.3390/microorganisms9020321
Leibold, M. A., Holyoak, M., Mouquet, N., Amarasekare, P., Chase, J. M., Hoopes, M. F., et al. (2004). The metacommunity concept: a framework for multi-scale community ecology. Ecol. Lett. 7, 601–613. doi: 10.1111/j.1461-0248.2004.00608.x
Lewis, M., Kim, M. H., Wang, N., and Chu, K. H. (2016). Engineering artificial communities for enhanced FTOH degradation. Sci. Total Environ. 572, 935–942. doi: 10.1016/j.scitotenv.2016.07.223
Li, Q., Xing, Y., Fu, X., Ji, L., Li, T., Wang, J., et al. (2021). Biochemical mechanisms of rhizospheric Bacillus subtilis-facilitated phytoextraction by alfalfa under cadmium stress – microbial diversity and metabolomics analyses. Ecotoxicol. Environ. Saf. 212:112016. doi: 10.1016/j.ecoenv.2021.112016
Li, T., Li, C. T., Butler, K., Hays, S. G., Guarnieri, M. T., Oyler, G. A., et al. (2017). Mimicking lichens: incorporation of yeast strains together with sucrose-secreting cyanobacteria improves survival, growth, ROS removal, and lipid production in a stable mutualistic co-culture production platform. Biotechnol. Biofuels 10:55. doi: 10.1186/s13068-017-0736-x
Liang, J., Fang, X., Lin, Y., and Wang, D. (2018). A new screened microbial consortium OEM2 for lignocellulosic biomass deconstruction and chlorophenols detoxification. J. Hazard Mater. 347, 341–348. doi: 10.1016/j.jhazmat.2018.01.023
Liang, Y., Ma, A., Song, M., Xie, F., Zhuang, X., and Zhuang, G. (2020). Advances in biodegradation of perfluorooctane sulfonate (PFOS). Microbiol. China 47, 2536–2539. doi: 10.13344/j.microbiol.china.190981
Liao, C., Wang, T., Maslov, S., and Xavier, J. B. (2020). Modeling microbial cross-feeding at intermediate scale portrays community dynamics and species coexistence. PLoS Comput. Biol. 16:e1008135. doi: 10.1371/journal.pcbi.1008135
Litchman, E., and Klausmeier, C. A. (2008). Trait-Based Community Ecology of Phytoplankton. Annu. Rev. Ecol. Evol. Syst. 39, 615–639. doi: 10.1146/annurev.ecolsys.39.110707.173549
Liu, W., Roder, H. L., Madsen, J. S., Bjarnsholt, T., Sorensen, S. J., and Burmolle, M. (2016). Interspecific bacterial interactions are reflected in multispecies biofilm spatial organization. Front. Microbiol. 7:1366. doi: 10.3389/fmicb.2016.01366
Liu, W., Russel, J., Roder, H. L., Madsen, J. S., Burmolle, M., and Sorensen, S. J. (2017). Low-abundant species facilitates specific spatial organization that promotes multispecies biofilm formation. Environ. Microbiol. 19, 2893–2905. doi: 10.1111/1462-2920.13816
Louca, S., Polz, M. F., Mazel, F., Albright, M. B. N., Huber, J. A., O’Connor, M. I., et al. (2018). Function and functional redundancy in microbial systems. Nat. Ecol. Evol. 2, 936–943. doi: 10.1038/s41559-018-0519-1
Ma, H., Wang, X., Zhang, Y., Hu, H., Ren, H., Geng, J., et al. (2018). The diversity, distribution and function of N-acyl-homoserine lactone (AHL) in industrial anaerobic granular sludge. Bioresour. Technol. 247, 116–124. doi: 10.1016/j.biortech.2017.09.043
Mallick, H., Franzosa, E. A., McLver, L. J., Banerjee, S., Sirota-Madi, A., Kostic, A. D., et al. (2019). Predictive metabolomic profiling of microbial communities using amplicon or metagenomic sequences. Nat. Commun. 10:3136. doi: 10.1038/s41467-019-10927-1
Martinez-Garcia, M., Brazel, D., Poulton, N. J., Swan, B. K., Gomez, M. L., Masland, D., et al. (2012). Unveiling in situ interactions between marine protists and bacteria through single cell sequencing. ISME J. 6, 703–707. doi: 10.1038/ismej.2011.126
Martiny, J. B., Bohannan, B. J., Brown, J. H., Colwell, R. K., Fuhrman, J. A., Green, J. L., et al. (2006). Microbial biogeography: putting microorganisms on the map. Nat. Rev. Microbiol. 4, 102–112. doi: 10.1038/nrmicro1341
Martiny, J. B., Jones, S. E., Lennon, J. T., and Martiny, A. C. (2015). Microbiomes in light of traits: a phylogenetic perspective. Science 350:aac9323. doi: 10.1126/science.aac9323
Massalha, H., Korenblum, E., Malitsky, S., Shapiro, O. H., and Aharoni, A. (2017). Live imaging of root-bacteria interactions in a microfluidics setup. Proc. Natl. Acad. Sci. U.S.A. 114, 4549–4554. doi: 10.1073/pnas.1618584114
McGill, B. J., Enquist, B. J., Weiher, E., and Westoby, M. (2006). Rebuilding community ecology from functional traits. Trends Ecol. Evol. 21, 178–185. doi: 10.1016/j.tree.2006.02.002
Miccio, L., Marchesano, V., Mugnano, M., Grilli, S., and Ferraro, P. (2016). Light induced DEP for immobilizing and orienting Escherichia coli bacteria. Optics Lasers Eng. 76, 34–39. doi: 10.1016/j.optlaseng.2015.03.025
Miller, E. T., and Klausmeier, C. A. (2017). Evolutionary stability of coexistence due to the storage effect in a two-season model. Theor. Ecol. 10, 91–103. doi: 10.1007/s12080-016-0314-z
Muller, S., Harms, H., and Bley, T. (2010). Origin and analysis of microbial population heterogeneity in bioprocesses. Curr. Opin. Biotechnol. 21, 100–113. doi: 10.1016/j.copbio.2010.01.002
Nakazawa, S., Imaichi, O., Kogure, T., Kubota, T., Toyoda, K., Suda, M., et al. (2021). History-driven genetic modification design technique using a domain-specific lexical model for the acceleration of DBTL cycles for microbial cell factories. ACS Synth. Biol. 10, 2308–2317. doi: 10.1021/acssynbio.1c00234
Niehaus, L., Boland, I., Liu, M., Chen, K., Fu, D., Henckel, C., et al. (2019). Microbial coexistence through chemical-mediated interactions. Nat. Commun. 10:2052. doi: 10.1038/s41467-019-10062-x
Novack-Gottshall, P. M. (2000). Book and software reviews-large-scale perspectives in community ecology. Complexity 6, 58–59.
O’Brien, E. J., Monk, J. M., and Palsson, B. O. (2015). using genome-scale models to predict biological capabilities. Cell 161, 971–987. doi: 10.1016/j.cell.2015.05.019
Oliveira, N. M., Niehus, R., and Foster, K. R. (2014). Evolutionary limits to cooperation in microbial communities. Proc. Natl. Acad. Sci. U.S.A. 111, 17941–17946. doi: 10.1073/pnas.1412673111
Pande, S., Merker, H., Bohl, K., Reichelt, M., Schuster, S., de Figueiredo, L. F., et al. (2014). Fitness and stability of obligate cross-feeding interactions that emerge upon gene loss in bacteria. ISME J. 8, 953–962. doi: 10.1038/ismej.2013.211
Pandin, C., Le Coq, D., Canette, A., Aymerich, S., and Briandet, R. (2017). Should the biofilm mode of life be taken into consideration for microbial biocontrol agents? Microb. Biotechnol. 10, 719–734. doi: 10.1111/1751-7915.12693
Park, S. Y., Yang, D., Ha, S. H., and Lee, S. Y. (2019). Metabolic engineering of microorganisms for the production of natural compounds. Adv. Biosyst. 2:1700190. doi: 10.1002/adbi.201700190
Pimm, S. L. (1984). The complexity and stability of ecosystems. Nature 307, 321–326. doi: 10.1038/307321a0
Puentes-Tellez, P. E., and Falcao Salles, J. (2018). Construction of effective minimal active microbial consortia for lignocellulose degradation. Microb. Ecol. 76, 419–429. doi: 10.1007/s00248-017-1141-5
Raes, J., and Bork, P. (2008). Molecular eco-systems biology: towards an understanding of community function. Nat. Rev. Microbiol. 6, 693–699. doi: 10.1038/nrmicro1935
Ren, G., Ma, A., Zhang, Y., Deng, Y., Zheng, G., Zhuang, X., et al. (2018). Electron acceptors for anaerobic oxidation of methane drive microbial community structure and diversity in mud volcanoes. Environ. Microbiol. 20, 2370–2385. doi: 10.1111/1462-2920.14128
Rotaru, A. E., Shrestha, P. M., Liu, F., Markovaite, B., Chen, S., Nevin, K. P., et al. (2014). Direct interspecies electron transfer between Geobacter metallireducens and Methanosarcina barkeri. Appl. Environ. Microbiol. 80, 4599–4605. doi: 10.1128/AEM.00895-14
Rugbjerg, P., Myling-Petersen, N., Porse, A., Sarup-Lytzen, K., and Sommer, M. O. A. (2018). Diverse genetic error modes constrain large-scale bio-based production. Nat. Commun. 9:787. doi: 10.1038/s41467-018-03232-w
Rupal, B. S., Garcia, E. A., Ayranci, C., and Qureshi, A. J. (2019). 3D printed 3D-microfluidics: recent developments and design challenges. J. Integrated Design Process Sci. 22, 5–20. doi: 10.3233/jid-2018-0001
Salles, J. F., Poly, F., Schmid, B., and Le Roux, X. (2009). Community niche predicts the functioning of denitrifying bacterial assemblages. Ecology 90, 3324–3332. doi: 10.1890/09-0188.1
Sanati Nezhad, A. (2014). Microfluidic platforms for plant cells studies. Lab. Chip 14, 3262–3274. doi: 10.1039/c4lc00495g
Schleifer, K. H. (2009). Classification of Bacteria and Archaea: past, present and future. Syst. Appl. Microbiol. 32, 533–542. doi: 10.1016/j.syapm.2009.09.002
Senoh, M., Ghosh-Banerjee, J., Ramamurthy, T., Hamabata, T., Kurakawa, T., Takeda, M., et al. (2010). Conversion of viable but nonculturable Vibrio cholerae to the culturable state by co-culture with eukaryotic cells. Microbiol. Immunol. 54, 502–507. doi: 10.1111/j.1348-0421.2010.00245.x
Shade, A., Peter, H., Allison, S. D., Baho, D. L., Berga, M., Burgmann, H., et al. (2012). Fundamentals of microbial community resistance and resilience. Front. Microbiol. 3:417. doi: 10.3389/fmicb.2012.00417
Shahab, R. L., Luterbacher, J. S., Brethauer, S., and Studer, M. H. (2018). Consolidated bioprocessing of lignocellulosic biomass to lactic acid by a synthetic fungal-bacterial consortium. Biotechnol. Bioeng. 115, 1207–1215. doi: 10.1002/bit.26541
Shong, J., Jimenez Diaz, M. R., and Collins, C. H. (2012). Towards synthetic microbial consortia for bioprocessing. Curr. Opin. Biotechnol. 23, 798–802. doi: 10.1016/j.copbio.2012.02.001
Sieuwerts, S., de Bok, F. A., Hugenholtz, J., and van Hylckama Vlieg, J. E. (2008). Unraveling microbial interactions in food fermentations: from classical to genomics approaches. Appl. Environ. Microbiol. 74, 4997–5007. doi: 10.1128/AEM.00113-08
Smith, N. W., Shorten, P. R., Altermann, E., Roy, N. C., and McNabb, W. C. (2019). The classification and evolution of bacterial cross-feeding. Front. Ecol. Evol. 7:153.
Spus, M., Li, M., Alexeeva, S., Wolkers-Rooijackers, J. C., Zwietering, M. H., Abee, T., et al. (2015). Strain diversity and phage resistance in complex dairy starter cultures. J. Dairy Sci. 98, 5173–5182. doi: 10.3168/jds.2015-9535
Steinberg, M. S. (1975). “Reconstruction of tissues by dissociated cells,” in Models for Cell Rearrangement, ed. G. D. Mostow (New Haven, CT: Yale University Press), 82–99. doi: 10.1126/science.141.3579.401
Steinberg, M. S. (2007). Differential adhesion in morphogenesis: a modern view. Curr. Opin. Genet. Dev. 17, 281–286. doi: 10.1016/j.gde.2007.05.002
Sun, Z., Koffel, T., Stump, S. M., Grimaud, G. M., and Klausmeier, C. A. (2019). Microbial cross-feeding promotes multiple stable states and species coexistence, but also susceptibility to cheaters. J. Theor. Biol. 465, 63–77. doi: 10.1016/j.jtbi.2019.01.009
Temperton, B., and Giovannoni, S. J. (2012). Metagenomics: microbial diversity through a scratched lens. Curr. Opin. Microbiol. 15, 605–612. doi: 10.1016/j.mib.2012.07.001
Treves, D. S., Xia, B., Zhou, J., and Tiedje, J. M. (2003). A two-species test of the hypothesis that spatial isolation influences microbial diversity in soil. Microb. Ecol. 45, 20–28. doi: 10.1007/s00248-002-1044-x
Triado-Margarit, X., Capitan, J. A., Menendez-Serra, M., Ortiz-Alvarez, R., Ontiveros, V. J., Casamayor, E. O., et al. (2019). A Randomized trait community clustering approach to unveil consistent environmental thresholds in community assembly. ISME J. 13, 2681–2689. doi: 10.1038/s41396-019-0454-4
Tsoi, R., Wu, F., Zhang, C., Bewick, S., Karig, D., and You, L. (2018). Metabolic division of labor in microbial systems. Proc. Natl. Acad. Sci. U.S.A. 115, 2526–2531. doi: 10.1073/pnas.1716888115
Vallespir Lowery, N., and Ursell, T. (2019). Structured environments fundamentally alter dynamics and stability of ecological communities. Proc. Natl. Acad. Sci. U.S.A. 116, 379–388. doi: 10.1073/pnas.1811887116
Van Bodegom, P. M., Douma, J. C., Witte, J. P. M., Ordoñez, J. C., Bartholomeus, R. P., and Aerts, R. (2012). Going beyond limitations of plant functional types when predicting global ecosystem-atmosphere fluxes: exploring the merits of traits-based approaches. Glob. Ecol. Biogeogr. 21, 625–636. doi: 10.1111/j.1466-8238.2011.00717.x
Vet, S., de Buyl, S., Faust, K., Danckaert, J., Gonze, D., and Gelens, L. (2018). Bistability in a system of two species interacting through mutualism as well as competition: Chemostat vs. Lotka-Volterra equations. PLoS One 13:e0197462. doi: 10.1371/journal.pone.0197462
Violle, C., Navas, M. L., Vile, D., Kazakou, E., Fortunel, C., Hummel, I., et al. (2007). Let the concept of trait be functional! Oikos 116, 882–892. doi: 10.1111/j.2007.0030-1299.15559.x
Violle, C., Nemergut, D. R., Pu, Z., and Jiang, L. (2011). Phylogenetic limiting similarity and competitive exclusion. Ecol. Lett. 14, 782–787. doi: 10.1111/j.1461-0248.2011.01644.x
Wallenstein, M. D., and Hall, E. K. (2011). A trait-based framework for predicting when and where microbial adaptation to climate change will affect ecosystem functioning. Biogeochemistry 109, 35–47. doi: 10.1007/s10533-011-9641-8
Wanapaisan, P., Laothamteep, N., Vejarano, F., Chakraborty, J., Shintani, M., Muangchinda, C., et al. (2018). Synergistic degradation of pyrene by five culturable bacteria in a mangrove sediment-derived bacterial consortium. J. Hazard Mater. 342, 561–570. doi: 10.1016/j.jhazmat.2017.08.062
Wang, E. X., Ding, M. Z., Ma, Q., Dong, X. T., and Yuan, Y. J. (2016). Reorganization of a synthetic microbial consortium for one-step vitamin C fermentation. Microb. Cell Fact 15:21. doi: 10.1186/s12934-016-0418-6
Wang, Y., Wang, H., Wang, X., Xiao, Y., Zhou, Y., Su, X., et al. (2020). Resuscitation, isolation and immobilization of bacterial species for efficient textile wastewater treatment: a critical review and update. Sci. Total Environ. 730:139034. doi: 10.1016/j.scitotenv.2020.139034
Webb, C. T., Hoeting, J. A., Ames, G. M., Pyne, M. I., and LeRoy Poff, N. (2010). A structured and dynamic framework to advance traits-based theory and prediction in ecology. Ecol. Lett. 13, 267–283. doi: 10.1111/j.1461-0248.2010.01444.x
Wei, W., Zhang, Y., Komorek, R., Plymale, A., Yu, R., Wang, B., et al. (2017). Characterization of syntrophic geobacter communities using ToF-SIMS. Biointerphases 12:05G601. doi: 10.1116/1.4986832
Wilbert, S. A., Mark Welch, J. L., and Borisy, G. G. (2020). Spatial ecology of the human tongue dorsum microbiome. Cell Rep. 30, 4003–4015.e3. doi: 10.1016/j.celrep.2020.02.097
Wilpiszeski, R. L., Gionfriddo, C. M., Wymore, A. M., Moon, J. W., Lowe, K. A., Podar, M., et al. (2020). In-field bioreactors demonstrate dynamic shifts in microbial communities in response to geochemical perturbations. PLoS One 15:e0232437. doi: 10.1371/journal.pone.0232437
Wintermute, E. H., and Silver, P. A. (2010). Dynamics in the mixed microbial concourse. Genes Dev. 24, 2603–2614. doi: 10.1101/gad.1985210
Wrighton, K. C., Thomas, B. C., Sharon, I., Miller, C. S., Castelle, C. J., VerBerkmoes, N. C., et al. (2012). Fermentation, hydrogen, and sulfur metabolism in multiple uncultivated bacterial phyla. Science 337, 1661–1665. doi: 10.1126/science.1224041
Xie, B. B., Li, M., Anantharaman, K., and Ravin, N. V. (2021). Editorial: the uncultured microorganisms: novel technologies and applications. Front. Microbiol. 12:756287. doi: 10.3389/fmicb.2021.756287
Yurtsev, E. A., Conwill, A., and Gore, J. (2016). Oscillatory dynamics in a bacterial cross-protection mutualism. Proc. Natl. Acad. Sci. U.S.A. 113, 6236–6241. doi: 10.1073/pnas.1523317113
Zakharova, L., Meyer, K. M., and Seifan, M. (2019). Trait-based modelling in ecology: a review of two decades of research. Ecol. Modelling 407:108703. doi: 10.1111/brv.12504
Zelezniak, A., Andrejev, S., Ponomarova, O., Mende, D. R., Bork, P., and Patil, K. R. (2015). Metabolic dependencies drive species co-occurrence in diverse microbial communities. Proc. Natl. Acad. Sci. U.S.A. 112, 6449–6454. doi: 10.1073/pnas.1421834112
Zhalnina, K., Louie, K. B., Hao, Z., Mansoori, N., da Rocha, U. N., Shi, S., et al. (2018a). Dynamic root exudate chemistry and microbial substrate preferences drive patterns in rhizosphere microbial community assembly. Nat. Microbiol. 3, 470–480. doi: 10.1038/s41564-018-0129-3
Zhalnina, K., Zengler, K., Newman, D., and Northen, T. R. (2018b). Need for laboratory ecosystems to unravel the structures and functions of soil microbial communities mediated by chemistry. mBio 9:e01175–18. doi: 10.1128/mBio.01175-18
Zhang, G., Zhang, F., Ding, G., Li, J., Guo, X., Zhu, J., et al. (2012). Acyl homoserine lactone-based quorum sensing in a methanogenic archaeon. ISME J. 6, 1336–1344. doi: 10.1038/ismej.2011.203
Zhang, L., Li, X., Yong, Q., Yang, S. T., Ouyang, J., and Yu, S. (2016). Impacts of lignocellulose-derived inhibitors on L-lactic acid fermentation by Rhizopus oryzae. Bioresour. Technol. 203, 173–180. doi: 10.1016/j.biortech.2015.12.014
Zhou, K., Qiao, K., Edgar, S., and Stephanopoulos, G. (2015). Distributing a metabolic pathway among a microbial consortium enhances production of natural products. Nat. Biotechnol. 33, 377–383. doi: 10.1038/nbt.3095
Zhuang, W. Q., Yi, S., Bill, M., Brisson, V. L., Feng, X., Men, Y., et al. (2014). Incomplete Wood-Ljungdahl pathway facilitates one-carbon metabolism in organohalide-respiring Dehalococcoides mccartyi. Proc. Natl. Acad. Sci. U.S.A. 111, 6419–6424. doi: 10.1073/pnas.1321542111
Ziemba, C., Shabtai, Y., Piatkovsky, M., and Herzberg, M. (2016). Cellulose effects on morphology and elasticity of Vibrio fischeri biofilms. NPJ Biofilms Microbiomes 2:1. doi: 10.1038/s41522-016-0001-2
Keywords: synthetic biology, cross-feeding, traits, spatial structure, microbial consortia
Citation: Liang Y, Ma A and Zhuang G (2022) Construction of Environmental Synthetic Microbial Consortia: Based on Engineering and Ecological Principles. Front. Microbiol. 13:829717. doi: 10.3389/fmicb.2022.829717
Received: 06 December 2021; Accepted: 31 January 2022;
Published: 23 February 2022.
Edited by:
Kunal R. Jain, Sardar Patel University, IndiaReviewed by:
Aindrila Mukhopadhyay, Lawrence Berkeley National Laboratory, United StatesChirayu Desai, Charotar University of Science and Technology, India
Jagat Rathod, National Cheng Kung University, Taiwan
Copyright © 2022 Liang, Ma and Zhuang. This is an open-access article distributed under the terms of the Creative Commons Attribution License (CC BY). The use, distribution or reproduction in other forums is permitted, provided the original author(s) and the copyright owner(s) are credited and that the original publication in this journal is cited, in accordance with accepted academic practice. No use, distribution or reproduction is permitted which does not comply with these terms.
*Correspondence: Anzhou Ma, YXptYUByY2Vlcy5hYy5jbg==