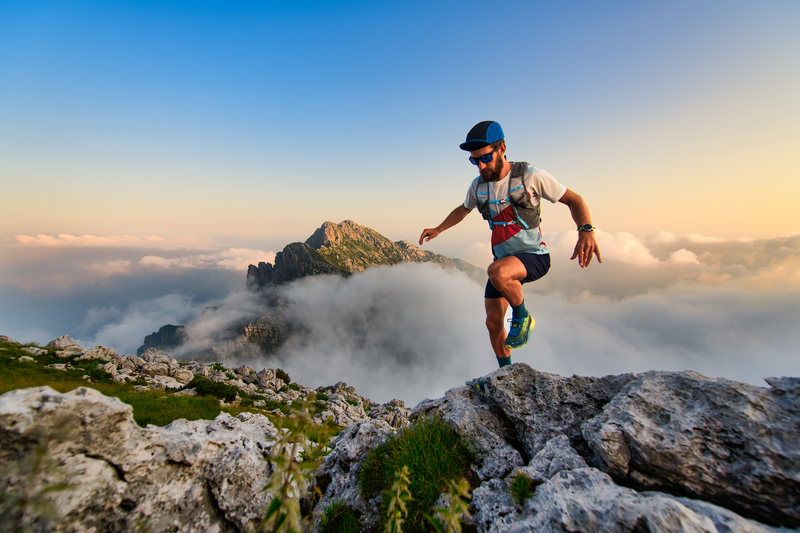
95% of researchers rate our articles as excellent or good
Learn more about the work of our research integrity team to safeguard the quality of each article we publish.
Find out more
ORIGINAL RESEARCH article
Front. Microbiol. , 18 April 2022
Sec. Systems Microbiology
Volume 13 - 2022 | https://doi.org/10.3389/fmicb.2022.826881
The physiological state of the host affects the gut microbes. The estrus cycle is critical to the reproductive cycle of sows. However, the association between gut microbes and animal estrus is poorly understood. Here, high-throughput 16S rRNA sequencing and liquid chromatography-mass spectrometry (LC-MS) non-targeted metabolome technology were used to study the estrous cycles in Diannan small ear pigs. Significantly different gut microbiota and metabolites of sows at estrous and diestrus were screened out and the correlation was analyzed. We found that the intestinal microbial composition and microbial metabolism of Diannan small ear sows were significantly different at diestrus and metestrus. The abundances of Spirochaetes, Spirochaetia, Spirochaetales, Spirochaetaceae, Deltaproteobacteria, unidentified_Alphaproteobacteria, Ruminococcus_sp_YE281, and Treponema_berlinense in intestinal microorganisms of Diannan small ear sows at metestrus are significantly higher than that at diestrus. Propionic acid, benzyl butyrate, sucrose, piperidine, and 5-aminoimidazole-4-carboxamide ribonucleotide (AICAR) were significantly enriched at metestrus compared with diestrus, which were involved in the energy metabolism-related pathways and activated protein kinase (AMPK) signaling pathway. At diestrus and metestrus, differential microbiota of unidentified_Alphaproteobacteria, Intestinimonas, Peptococcus, Terrisporobacter, and differential metabolites of piperidine, propionic acid, and benzyl butyrate, sucrose, 4-methyl catechol, and AICAR exist a certain degree of correlation. Therefore, unidentified_Alphaproteobacteria, Ruminococcus_sp_YE281, and Treponema_berlinense may have a potential role at metestrus of the Diannan small ear sows. AICAR may be apotential marker of estrus Diannan small ear sows feces, but further studies about the specific mechanism are needed. These findings provide a new perspective for sows production management and improving sows reproductive performance.
The reproductive performance of sows directly affects the benefit of pig farms (Van Wettere et al., 2017). Estrus plays a vital role in the reproductive cycle of sows because effective estrus can promote reproduction performance (Andersson et al., 2016). A previous study established that Chinese indigenous pigs, such as Meishan, Erhualian, and Mi pigs, outperformed European pigs in terms of reproductive performance and estrous expression features, including larger litter sizes and a more distinct presentation of estrous behaviors (Bazer et al., 1988; Fan et al., 2002). Gilts of these Chinese pig breeds mature earlier. For instance, the mean age at puberty onset in Meishan gilts was 115 days, compared with 235 days in large white gilts (Hunter et al., 1993). They exhibit behavioral estrous for a little more extended time and have slightly shorter estrous cycles than landrace and large white (Bazer et al., 1988). Diannan small ear pig is a typical native pig species in China, with the above characteristics.
The gastrointestinal tract of mammals is composed of about 1014 microorganisms (Zhao et al., 2015; Yang et al., 2016). Intestinal microorganisms have a vast range of metabolic activities and produce abundant microbial metabolites, are often referred to as a “metabolic organ” of the host (LeBlanc et al., 2013). Intestinal microorganisms and their metabolites affect multiple physiological functions of the host, which can promote the absorption of nutrients and energy metabolism, promote healthy intestinal development, etc. (Backhed et al., 2004; Dai et al., 2010). The composition and abundance of intestinal microorganisms are directly related to the host animal’s health status and production performance. When the external environment, diet, and physiological state change, the composition and abundance of intestinal microorganisms will be impacted and then affect the host (Hang and Zhu, 2012).
Sex hormones affect the composition of the microbial community (Hooper et al., 2001; Velagapudi et al., 2010; Nicholson et al., 2012). On the contrary, the diversity of the microbial community also affects the metabolism of sex hormones. That is, the intestinal microorganisms and the host sex hormone secretion and metabolism have a two-way effect (Melvin, 2016). This study results suggest that there may be a specific correlation between intestinal microorganisms and animal estrus, and estrus, as an essential period in animal production, may also be closely related to intestinal microorganisms. However, there are still discrepancies between related studies conducted on various animal species. The results of a related study on Murrah buffalo and dholes suggest a reversible effect and a possible correlation between the gut microbiome and animal reproductive physiology (Wu et al., 2020; Sharma et al., 2021). On the contrary, relevant studies in female mice established that the estrous cycle does not result in any significant shift in the intestinal microbial community in the reproductively mature, regularly cycling female mouse (Guo et al., 2016; Wallace et al., 2018). As far as we are aware, no report exists on the intestinal microorganisms of estrus sows.
Therefore, this study aims to study the differences and correlations between intestinal microorganisms and metabolites in sows at diestrus and metestrus and provide a new perspective for sows production management and improvement of sows reproductive performance.
All experiment procedures were performed according to the Guide for Animal Care and Use of Laboratory Animals in the Institutional Animal Care and Use Committee of Yunnan Agricultural University. The Department Animal Ethics Committee approved the experimental protocol of the Yunnan Agricultural University. A total of six third-party Diannan small ear sows were chosen as the study object raised in the pig-breeding institute of the Yunnan Academy of Animal Husbandry and Veterinary Sciences. They were raised under a standardized feeding regimen with free access to water.
The feces of six Diannan small ear sows were collected and assigned to the DA group on the tenth day following the end of the sows’ previous estrus cycle, i.e., while the sows were in diestrus. Estrous detection was carefully performed twice ∼ three times daily at proestrus. The vulva’s reddening and swelling, as well as testing by a boar, were defined as standing reflexes of estrous expression and were scored visually using a standard scoring system (Rydhmer et al., 1994). The feces of six Diannan small ear sows were collected and assigned as the DC group when metestrus was detected in the sows.
Sterilized sampling tubes were quickly placed in a liquid nitrogen tank to collect the samples. Following their return to the laboratory, the feces samples were stored in a refrigerator set to −80°C.
The fecal DNA extraction kit was used to extract the total DNA of the samples (Beijing Tiangen Biochemical Technology Corporation Ltd.). The purity and concentration of DNA were determined by agarose gel electrophoresis and an appropriate amount of sample DNA was placed in a centrifuge tube. The sample was diluted to 1 ng/μl with sterile water. The diluted genomic DNA was used as the template for PCR expansion and the amplified region was the v3–v4 region of the 16S rRNA gene. The specific primers with barcode were used and the extended primers were 338F (5′-ACTCCTACGGGAGGCAGCA-3′) and 806R (5′-GGACTACHVGGGTWTCTAAT-3′). PCR products were detected by electrophoresis using 2% agarose gel. The PCR product was mixed with the same number of samples according to the concentration of the PCR product. After the samples were thoroughly mixed, the PCR product was purified by 1X TAE gel electrophoresis with a concentration of 2% and the target band was cut and recovered (Thermo Fisher Scientific Company GeneJET Adhesive Recovery Kit). Ion Plus Fragment Library Kit 48 RXNS from Thermo Fisher Scientific was used for library construction. After the library was qualified by Qubit quantitative and library testing, Ion S5™XL of Thermo Fisher Scientific was used for computer sequencing.
Cutadapt (V1.9.1)1 was used to cut low-quality parts of reading and then compared with the species annotation database to detect chimera sequences (Aßhauer et al., 2015). Chimera sequences were removed to obtain the final effective data (Clean Reads). All Clean Reads of all samples were clustered using Uparse software (Uparse v7.0.1001)2 (Haas et al., 2011), and by default, the sequence was clustered as operational taxonomic units (OTUs) with 97% identity. Species annotation of OTUs sequence was performed by Mothur method and SSUrRNA database of SILVA132 (the threshold was set as 0.8–1; Edgar, 2013). Fast multisequence alignment using the MUSCLE software (Version 3.8.31) to obtain system occurrence relationships for all the OTUs sequences (Quast and Pruesse, 2013). The samples were analyzed for alpha diversity and beta diversity by the QIIME software (Version 1.9.1). Unifrac distance was calculated by OTUs relation in samples (Lozupone and Knight, 2005; Lozupone et al., 2011), and Unweighted Pairgroup Method with Arithmetic Mean (UPGMA) were constructed. The LEfSe software was used for intergroup differential species analysis, and the screening value of linear discrimination criterion (LDA Score) was 2 (Segata et al., 2011). Use the Tax4Fun software to predict the function of all samples (Qin et al., 2012).
Take the liquid sample to be detected 100 μl (0.1 mg tissue liquid nitrogen grinding) placed in EP tube, add in 400 including 80% of methanol aqueous solution (four times the volume of methanol), vortex oscillation, in −20°C stand for 60 min, 14,000 g, 4°C centrifugal 20 min, take a certain amount of supernatant put in 1.5 ml centrifuge tube, vacuum freeze-drying, the residue with 100 μl complex solvents to dissolve, vortex oscillation, 14,000 g, 4°C centrifugal 15 min, take that into the supernatant fluid sample liquid chromatography-mass spectrometry (LC-MS) analysis.
Chromatographic conditions: positive model: mobile phase A: 0.1% formic acid, 95% acetonitrile, 10 mM ammonium acetate; mobile phase B: 0.1% formic acid, 50% acetonitrile, 10 mM ammonium acetate. Negative mode: mobile phase A: 95% acetonitrile, 10 mM ammonium acetate, pH 9.0; mobile phase B: 50% acetonitrile, 10 mM ammonium acetate, pH 9.0. Column temperature: 40°C; and flow rate: 0.3 ml/min. Mass spectrometry conditions: m/z 100–1,500 was selected for the scanning range. The settings of ESI are as follows: spray voltage: 3.2 kv; sheath gas flow rate: 35 arb; aux gas flow rate: 10 arb; and temperature: 320°C. Polarity:positive; Negative; MS/MS level 2 scans are data-dependent scans.
Import the output data (.raw) file into compound discoverer (CD) search software for simple filtering of retention time, mass charge ratio, and other parameters. Then, different samples were aligned according to the retention time deviation of 0.2 min and mass deviation of 5 PPM to make the identification more accurate. According to the set mass deviation of 5 PPM, signal strength deviation of 30%, the signal-to-noise ratio of 3, the minimum signal strength of 100,000, and other information, the peak was extracted. At the same time, the peak area was quantified, the target ions were integrated, the molecular formula was predicted and compared with the mz Cloud database, the background ions were removed with blank samples, the quantitative results were normalized with quality control (QC) samples, and finally, the identification and quantitative results of the data were obtained.
Pearson correlation analysis was conducted with SPSS version 22.0 and a visual relational network was constructed in Cytoscape software (v3.5.1) according to the correlation coefficient (r) of the different microbiota and metabolites.
Through the comparative analysis of OTUs of the two groups of samples, it was found that the total OTUs of the DA and DC groups were 1,111, while the OTUs of the DA and DC groups were 168 and 208, respectively (Figure 1).
At the phylum level, a total of 24 phyla were noted, including Firmicutes, Bacteroidetes, Actinobacteria, Proteobacteria, Spirochaetes, etc., among which Firmicutes and Bacteroidetess were the major phyla of bacteria in both the DA and DC groups, accounting for more than 90% of total microbiota in both the groups (Figure 2A). At the genus level, a total of 218 genera were noted, including Terrisporobacter, Bacteroides, unidentified_Clostridiales, Lactobacillus, unidentified_Ruminococcaceae, etc. (Figure 2B).
Figure 2. The histogram of relative abundance for intestinal microbiota in Diannan small ear sows (select the top 10 microbes at each level). (A) Phylum level, (B) genus level.
After 97% identity clustering, the DA group got 801 OTUs on average and the DC group got 807 OTUs on average. OTUs are then further annotated to calculate and compare the alpha diversity among the samples (Table 1). However, there is no significant difference of Chao1, ACE, Shannon, Simpson, and PD whole-tree indexes between the DA and DC groups. The unweighted pair group method with weighted mean (UPGMA) showed that samples of the DA and DC groups are clustered in different branches, indicating that the microbiota structure of these two groups is different (Figure 3).
The LEfSe multistage discriminant analysis showed that there were 13 biomarkers (LDA score = 2) in the intestinal microbial samples of pigs in the DA and DC groups (Figure 4). The relative abundance of Spirochaetes (phylum level), Spirochaetia and Deltaproteobacteria (class level), Spirochaetales (order level), Spirochaetaceae (family level), unidentified_Alphaproteobacteria (genus level), Ruminococcus_sp_YE281, and Treponema_berlinense (species level) in the DC group was significantly higher than that in the DA group (P < 0.05).
Tax4Fun software was used for functional prediction analysis. The results showed that at the Kyoto Encyclopedia of Genes and Genomes (KEGG) level 2, DA and DC samples were mainly involved in carbohydrate metabolism, transmembrane transport, replication and repair, translation, etc. At the KEGG level 3, DA and DC samples are mainly involved in transport proteins, DNA repair and recombinant proteins, two-component system, tRNA biosynthesis, etc. (Figure 5).
In the positive and negative ion modes, the correlation of QC samples (Figure 6) and R2 values are close to 1 (Figure 7). A total of 2,505 and 1,848 metabolites were detected in the fecal samples. It can be seen that oleic acid amide and pentadecanoic acid are the two metabolites with the highest relative expression under positive and negative ion mode, followed by roxatidine, myricoic acid, (+) -cp 55,940, oleic acid, etc.
Figure 7. The partial least square-discriminant analysis (PLS-DA) chart. (A) Positive ion mode and (B) negative ion mode.
The threshold was set as VIP > 1.0, fold change FC > 2.0 or FC < 0.5 and P < 0.05, and the differentially expressed metabolites were screened out (Figure 8). In the positive ion mode, there were 34 metabolites with significant differences between the DC and DA groups, among which 18 metabolites were significantly upregulated and 16 metabolites were significantly downregulated. The differential metabolites were mainly dibenzothiophene, heophylline B, etc. There were 52 significantly different metabolites in the anion mode, of which 45 metabolites were significantly upregulated and 7 metabolites were significantly downregulated. The differential metabolites were mainly 6-hydroxy-1,2-adipate dioctyl ester, morpholinone, masalossiol, 2-ammonia-4-oxygen butyrate, etc.
Figure 8. The volcano map of differential metabolites in estrus Diannan small ear sows. (A) Positive ion mode and (B) negative ion mode.
The cluster analysis diagram showed that the differential metabolites in the DA and DC groups are clustered, respectively, indicating that the expression patterns of metabolites in the sows at diestrus and metestrus are different. The samples in the same group may have similar functions or participate in the same biological process (Figure 9). Two benzothiophene and L-(+)-valine, ethyl mercury ions were extremely significant positive correlation (P < 0.01), aminomethyl phosphonic acid and ethyl mercury ions were significantly negative correlation (P < 0.05), sucrose and 6-hydroxy-1,2-adipic acid dioctyl ester, misha ossification alcohol, 2-ammonia-4-oxygen generation butyrate were extremely significant positive correlation (P < 0.01), and (3R, 4S, 9R, 11R)-27-(4-hydroxy phenyl)-4-methyl-3,9,11-seven alkoxy three alcohol has significant negative correlation (P < 0.05; Tables 2–4).
Figure 9. Clustering diagram of differential metabolites. (A) Positive ion mode and (B) negative ion mode.
Table 3. The correlation of significant different metabolites of the top 10 in positive ion mode (top 10).
Table 4. The correlation of significant different metabolites of the top 10 in negative ion mode (top 10).
The KEGG analysis (Figure 10) showed that in the positive ion mode, the significant differences were in the biosynthesis pathways of tolane, piperidine, and pyridine alkaloids involved by piperidine (P < 0.05) and protein digestion and absorption pathways (P < 0.05). In the negative ion mode, propionic acid, benzyl butyrate, and sucrose are involved in carbohydrate digestion and absorption of pathways (P < 0.01). Sucrose is involved in galactose metabolism pathways (P < 0.05), and starch and sucrose metabolism pathways (P < 0.05). 4-methylcatechol participated in dimethyl benzene degradation pathways (P < 0.05) and 5-aminoimidazole-4-formamide nucleotide (AICAR) was involved in the AMPK signaling pathway (P < 0.05; Table 5).
Table 5. The significant difference of the Kyoto Encyclopedia of Genes and Genomes (KEGG) enrichment table of the positive and negative ion modes.
There was a significant positive correlation between unidentified_Alphaproteobacteria and sucrose (P < 0.05). However, Intestinimonas with benzyl butyrate exhibited a significantly negative correlation (P < 0.05). There was a significant negative correlation between Peptococcus and propionic acid, benzyl butyrate, AICAR, and 4-methyl catechol (P < 0.05; Figures 11, 12).
Figure 11. The correlation heat map of differential intestinal microbiota and metabolites. Note: Com_148_neg: propionic acid; Com_4607_neg: benzyl butyrate; Com_5521_neg: sucrose; Com_1794_neg: AICAR; Com_735_neg: 4-methyl catechol; Com_706_pos: piperidine.
Figure 12. The correlation network map of differential intestinal microbiota and metabolites. Red/green indicates that the shape represents metabolite/bacteria, red/blue lines represent positive/negative correlation, and numbers represent correlation coefficients.
Animal intestines are home to many complex and diverse microbiota, and there is a mutually beneficial symbiosis between the microbiota and the host (Thompson and Trexler, 1971; Hayakawa et al., 2016). Recently, it has been demonstrated that the gut microbiota is a critical component of host physiology (Sekirov et al., 2010). Considering the important, dynamic, and reflective role of gut microbiota in host physiology and metabolism, conducting a study on estrus’s effect on gut microbes is optimizing sow reproductive performance and production management (Hooper et al., 2002; Pluznick, 2014). However, little information has been conducted on the gut microbiota in Diannan small ear sows. Therefore, we explored the possible association between estrus and gut microbiota and microbial metabolites using high-throughput 16S rRNA sequencing and LC-MS non-targeted metabolome technology at diestrus and metestrus.
Our findings revealed that Firmicutes and Bacteroidetes are the most abundant phylum in sow’s intestines, accounting for more than 90% of the total sequence, which is consistent with an earlier study (Kim et al., 2012; Poole et al., 2013). Firmicutes and Bacteroidetes play a critical role in maintaining the normal physiological function of the intestinal tract and microbial balance, which is of great significance to the growth and health of animals (Armougom and Raoult, 2008; Xu et al., 2019).
This study showed significant differences in the microbial structure were identified in sows at diestrus and metestrus, and the abundance of Ruminococcus_sp_YE281, Treponema_berlinense, and unidentified_Alphaproteobacteria in the feces of Diannan small ear sows at metestrus were significantly higher than those at diestrus. Ruminococcus_sp_YE281 is a species of bacteria under the Ruminococcus. Ruminococcus can degrade cellulose in plant cell walls (Fernando et al., 2010; Guan et al., 2018). Treponema_berlinense is a genus of bacteria under the Treponema. Treponema can degrade oligosaccharides, polysaccharides, lignocellulose, and indigestible substances (Guo, 2008). unidentified_Alphaproteobacteria is a genus of bacteria under the Proteobacteria. Shin et al.’s (2015) study have shown that Proteobacteria contains a variety of pathogenic bacteria and are a marker of instability in the intestinal microbiota. However, some studies have also shown that Proteobacteria can assist in the degradation of lignin (Fang et al., 2012). Whether the high abundance of unidentified_Alphaproteobacteria is beneficial or harmful to sows at metestrus needs further verification. It is worth noting that Spirobacteriaceae was found in the feces of sows in metestrus. Spirobacteriaceae and some species have been identified in humans as the leading cause of syphilis, Lyme disease, recurrent fever, and other diseases (Karami et al., 2014). There may be due to that sows transition from restlessness to arching and standing still, dull eyes, anorexia in metestrus (Tang et al., 2018).
Function analysis indicated that more bacterial genes are involved in carbohydrate metabolism, amino acid metabolism, energy metabolism; that is correlated with the enrichment of Bacteroidetes in the gut of Diannan small ear sows. Bacteroidetes are good at degrading non-fibrous substances and are the primary polysaccharide degradation and utilization bacteria, which can improve the utilization rate of carbohydrates, proteins, and other substances by the host and enhance the immunity of the host (Becker et al., 2014).
The metabolomics results of this study found that the propionic acid, benzyl butyrate, and sucrose content at metestrus Diannan small ear sows’ feces was significantly higher than at diestrus, these metabolites are involved in carbohydrate digestion and absorption pathway, which is consistent with the previous study results (Sankar and Archunan, 2008; Flores et al., 2012). We also found an extremely significant increase in piperidine concentration at metestrus sows feces than at diestrus, which involved the protein digestion and absorption pathways. At metestrus, sows showed anorexia, and the continuous excitement and agitation led to the reduction of blood glucose levels (Jezková and Smrcková, 1990). Therefore, to balance the blood glucose level, and maintain the nutritional needs of the body Diannan small ear sow needs to digest and absorb more carbohydrates and protein.
During estrus, the sows secrete a large number of sex hormones such as luteinizing hormone and follicle-stimulating hormone, while the concentration of progesterone gradually decreases (Toyama et al., 2018). Our study indicated that the concentration of AICAR in estrus sow’s feces was significantly higher in metestrus than in diestrus. AICAR is an activator of AMPK, which is involved in the AMPK signaling pathway. Tosca et al. (2005) found that in vitro culture of mouse granulosa cells, activation of AMPK by AICAR significantly reduced progesterone secretion by affecting gonadotropin secretion. Kimura’s earlier studies found that animal feces contained compounds associated with estrus, especially in females (Kimura, 2001). Sankar and Archunan confirmed that changes in compounds in feces could be an effective method to detect estrus in animals (Sankar and Archunan, 2008). Feces are one of the main agents for the elimination of metabolites in animals, so it may potentially convey a great deal of information about also animals internal physiology and provide a source of chemical signals. Therefore, this study suggested that AICAR may be a potential marker of estrus sows’ feces.
The correlation between intestinal microbiota and metabolites suggests that intestinal microbiota plays an essential role in maintaining body health (Chow et al., 2010). Our study found that the unidentified_Alphaproteobacteria was significantly positively correlated with sucrose, indicating that unidentified_Alphaproteobacteria can improve the carbohydrate digestion and absorption pathway of Diannan small ear sows at metestrus. It proves that unidentified_Alphaproteobacteria is a beneficial bacteria for Diannan smaller sows at metestrus. Thingholm et al. (2019) found that Intestinimona was significantly associated with obesity, and the abundance of Intestinimonas was significantly reduced when people were obese. In this study, Intestinimonas and benzyl butyrate, significantly have a negative correlation. These results show that when the Diannan smaller sows are at metestrus, the host’s carbohydrate digestion and absorption pathway can be improved by reducing the abundance of intestinimonas to meet the nutritional needs of Diannan smaller sows at estrus and maintain the weight of Diannan smaller sows at estrus. In addition, we also discovered that Terrisporobacter and 4-methyl catechol, piperidine, Peptococcus and, propionic acid, benzyl butyrate, AICAR, 4-methyl catechol significantly have a negative correlation, but the specific interaction mechanism still needs to be explored.
The data presented in the study are deposited in the GenBank repository, accession numbers ON026865-ON028634.
The animal study was reviewed and approved by Institutional Animal Care and Use Committee of Yunnan Agricultural University. Written informed consent was obtained from the owners for the participation of their animals in this study.
SMZ and XCG were responsible for the conception and design of the experiment and participated in drafting the manuscript. JHZ, HCS, XQZ, MHY, YH, HBP, and YGZ participated in the acquisition of the data and analyses of the results and agreed to be accountable for the accuracy and integrity of the data. All authors read and approved the final manuscript.
This study was supported by the Major Science and Technology Project of Yunnan Province (No. 202102AA310054), National Natural Science Foundation of China (Nos. 31760645, 31260592, and 31060331), Technological Innovation Talent Program (No. 2020FA011), and State School Cooperation (No. 2020ZXND02).
The authors declare that the research was conducted in the absence of any commercial or financial relationships that could be construed as a potential conflict of interest.
All claims expressed in this article are solely those of the authors and do not necessarily represent those of their affiliated organizations, or those of the publisher, the editors and the reviewers. Any product that may be evaluated in this article, or claim that may be made by its manufacturer, is not guaranteed or endorsed by the publisher.
Andersson, L. M., Okada, H., Miura, R., Okada, H., Yoshioka, K., Aso, H., et al. (2016). Wearable wireless estrus detection sensor for cows. Comput. Electron. Agric. 127, 101–108. doi: 10.1016/j.anireprosci.2017.03.002
Armougom, F., and Raoult, D. (2008). Use of pyrosequencing and DNA barcodes to monitor variations in firmicutes and bacteroidetes communities in the gut microbiota of obese humans. BMC Genom. 9:576. doi: 10.1186/1471-2164-9-576
Aßhauer, K. P., Wemheuer, B., Daniel, R., and Meinicke, P. (2015). Tax4Fun: predicting functional profiles from metagenomic 16S rRNA data. Bioinformatics 31, 2882–2884. doi: 10.1093/bioinformatics/btv287
Backhed, F., Ding, H., Wang, T., Hooper, L. V., Koh, G. Y., and Nagy, A. (2004). The gut microbiota as an environmental factor that regulates fat storage. Proc. Natl. Acad. Sci. U.S.A. 101, 15718–15723. doi: 10.1073/pnas.0407076101
Bazer, F. W., Thatcher, W. W., Martinat-Botte, F., and Terqui, M. J. (1988). Sexual maturation and morphological development of the reproductive tract in large white and prolific Chinese Meishan pigs. Reprod. Fertil. 83:723e8. doi: 10.1530/jrf.0.0830723
Becker, A. A., Hesta, M., Hollants, J., Janssens, G. P., and Huys, G. (2014). Phylogenetic analysis of faecal microbiota from captive cheetahs reveals underrepresentation of Bacteroidetes and Bifidobacteriaceae. BMC Microbiol. 14:43. doi: 10.1186/1471-2180-14-43
Chow, J., Lee, S. M., Shen, Y., Khosravi, A., and Mazmanian, S. K. (2010). Host-bacterial symbiosis in health and disease. Adv. Immunol. 107, 243–274. doi: 10.1016/B978-0-12-381300-8.00008-3
Dai, Z. L., Jing, Z., and Wu, G. (2010). Utilization of amino acids by bacteria from the pig small intestine. Amino Acids 39, 1201–1215. doi: 10.1007/s00726-010-0556-9
Edgar, R. C. (2013). UPARSE: highly accurate OTU sequences from microbial amplicon reads. Nat. Methods. 10, 996–998. doi: 10.1038/nmeth.2604
Fan, B., Wang, Z. G., Li, Y. J., Zhao, X. L., Liu, B., Zhao, S. H., et al. (2002). Genetic variation analysis within and among Chinese indigenous swine populations using microsatellite markers. Anim. Genet. 33:422e7. doi: 10.1046/j.1365-2052.2002.00898.x
Fang, W., Fang, Z., Zhou, P., Chang, F., Hong, Y., Zhang, X., et al. (2012). Evidence for lignin oxidation by the giant panda fecal microbiome. PLoS One 7:e50312. doi: 10.1371/journal.pone.0050312
Fernando, S. C., Purvis, H. T. II, Najar, F. Z., Sukharnikov, L. O., Krehbiel, C. R., Nagaraja, T. G., et al. (2010). Rumen microbial population dynamics during adaptation to a high-grain diet. Appl. Environ. Microbiol. 76, 7482–7490. doi: 10.1128/AEM.00388-10
Flores, R., Shi, J., and Fuhrman, B. (2012). Fecal microbial determinants of fecal and systemic estrogens and estrogen metabolites: a cross-sectional study. J. Transl. Med. 10:253. doi: 10.1186/1479-5876-10-253
Guan, Y., Yang, H., Han, S., Feng, L., Wang, T., and Ge, J. (2018). Correction to: comparison of the gut microbiota composition between wild and captive sika deer (Cervus nippon hortulorum) from feces by high-throughput sequencing. AMB Express 8:15.
Guo, X. L. (2008). Detection of Firmicutes and Bacteroidetes In The Pig Gut And The Correlation Betw Een Their Abundance And Fat Deposit. Ph.D Thesis, Yaan: Sichuan Agricultural University(in Chinese).
Guo, Y., Qi, Y., Yang, X., Zhao, L., Wen, S., Liu, Y., et al. (2016). Association between polycystic ovary syndrome and gut microbiota. PLoS One 11:e0153196. doi: 10.1371/journal.pone.0153196
Haas, B. J., Gevers, D., and Earl, A. M. (2011). Chimeric 16S rRNA sequence formation and detection in Sanger and 454-pyrosequenced PCR amplicons. Genome Res. 21, 494–504. doi: 10.1101/gr.112730.110
Hang, S., and Zhu, W. (2012). Gut bacterial and Lactobacilli communities of weaning piglets in response to mannan oligosaccharide and sugar beet pulp in vitro fermentation. J. Integr. Agric. 11, 122–133. doi: 10.1016/s1671-2927(12)60790-1
Hayakawa, T., Masuda, T., Kurosawa, D., and Tsukahara, T. (2016). Dietary administration of probiotics to sows and/or their neonates improves the reproductive performance, incidence of post-weaning diarrhea and histopathological parameters in the intestine of weaned piglets. Anim. Sci. J. 87, 1501–1510. doi: 10.1111/asj.12565
Hooper, L. V., Midtvedt, T., and Gordon, J. I. (2002). How host-microbial interactions shape the nutrient environment of the mammalian intestine. Annu. Rev. Nutr. 22, 283–307. doi: 10.1146/annurev.nutr.22.011602.092259
Hooper, L. V., Wong, M. H., Thelin, A., Hansson, L., Falk, P. G., and Gordon, J. I. (2001). Molecular analysis of commensal host-microbial relationships in the intestine. Science 291, 881–884. doi: 10.1126/science.291.5505.881
Hunter, M. G., Biggs, C., Foxcroft, G. R., McNeilly, A. S., and Tilton, J. E. (1993). Comparisons of endocrinology and behavioural events during the periovulatory period in Meishan and Large-White hybrid gilts. J. Reprod. Fertil. 97:475e80. doi: 10.1530/jrf.0.0970475
Jezková, D., and Smrcková, M. (1990). Změny glukozémie a laktacidémie u brezích prasnic, fétů a u prasnic a selat do desátého dne porodu [Changes in blood levels of glucose and lactic acid in pregnant sows and fetuses and in sows and piglets 10 days after parturition]. Vet. Med. (Praha). 35, 613–620.
Karami, A., Sarshar, M., Ranjbar, R., and Rahim, S. (2014). The Phylum Spirochaetaceae. The Prokaryotes. Heidelberg: Springer Berlin Heidelberg, 915–929.
Kim, H. B., Borewicz, K., and White, B. A. (2012). Microbial shifts in the swine distal gut in response to the treatment with antimicrobial growth promoter, tylosin. Proc. Natl. Acad. Sci. U.S.A. 109, 15485–15490. doi: 10.1073/pnas.1205147109
Kimura, R. (2001). Volatile substances in feces, urine and urine-marked feces of feral horses. Can. J. Anim. Sci. 81, 411–420. doi: 10.4141/a00-068
LeBlanc, J. G., Milani, C., and de Giori, G. S. (2013). Bacteria as vitamin suppliers to their host: a gut microbiota perspective. Curr. Opin. Biotechnol. 24, 160–168. doi: 10.1016/j.copbio.2012.08.005
Lozupone, C., and Knight, R. (2005). UniFrac: a new phylogenetic method for comparing microbial communities. Appl. Environ. Microbiol. 12, 8228–8235. doi: 10.1128/AEM.71.12.8228-8235.2005
Lozupone, C., Lladser, M. E., and Knights, D. (2011). Unifrac: an effective distance metric for microbial community comparison. ISME J. 5, 169–172. doi: 10.1038/ismej.2010.133
Melvin, R. (2016). The Effects Of Exercise And Estrogen On Gut Microbiota In Female Mice. New Jersey, NJ: M.S. Rutgers, The State University of New Jersey.
Nicholson, J. K., Holmes, E., Kinross, J., Burcelin, R., Gibson, G., Jia, W., et al. (2012). Host-gut microbiota metabolic interactions. Science 336, 1262–1267. doi: 10.1126/science.1223813
Pluznick, J. L. (2014). Gut microbes and host physiology: what happens when you host billions of guests? Front. Endocrinol. 5:91. doi: 10.3389/fendo.2014.00091
Poole, T. L., Suchodolski, J. S., and Callaway, T. R. (2013). The effect of chlortetracycline on faecal microbial populations in growing swine. J. Glob. Antimicrob. Resist. 1, 171–174. doi: 10.1016/j.jgar.2013.04.004
Qin, J., Li, Y., and Cai, Z. (2012). A metagenome-wide association study of gut microbiota in type 2 diabetes. Nature 490, 55–60. doi: 10.1038/nature11450
Quast, C., and Pruesse, E. (2013). The SILVA ribosomal RNA gene database project: improved data processing and web-based tools. Nucl. Acids Res. 41, D590–D596. doi: 10.1093/nar/gks1219
Rydhmer, L., Eliasson-Selling, L., Johansson, K., Stern, S., and Andersson, K. (1994). A genetic study of estrous symptoms at puberty and their relationship to growth and leanness in gilts. J. Anim. Sci. 72:1964e70. doi: 10.2527/1994.7281964x
Sankar, R., and Archunan, G. (2008). Identification of putative pheromones in bovine (Bos taurus) faeces in relation to estrus detection. Anim. Reprod. Sci. 103, 149–153. doi: 10.1016/j.anireprosci.2007.04.014
Segata, N., Izard, J., and Waldron, L. (2011). Metagenomic biomarker discovery and explanation. Genome Biol. 12:R60. doi: 10.1186/gb-2011-12-6-r60
Sekirov, I., Russell, S. L., and Antunes, L. C. M. (2010). Gut microbiota in health and disease. Physiol. Rev. 90, 859–904.
Sharma, R., Kumar Singh, P., Onteru, S. K., and Singh, D. (2021). Faecal microbiome analysis reveals Clostridiales and Bacteroidales as signature gut microbes during estrus of buffalo. Reprod. Biol. 21:100509. doi: 10.1016/j.repbio.2021.100509
Shin, N. R., Whon, T. W., and Bae, J. W. (2015). Proteobacteria: microbial signature of dysbiosis in gut microbiota. Trends Biotechnol. 33, 496–503. doi: 10.1016/j.tibtech.2015.06.011
Tang, L. T., Ran, X. Q., Mao, N., Zhang, F. P., Niu, X., Ruan, Y. Q., et al. (2018). Analysis of alternative splicing events by RNA sequencing in the ovaries of Xiang pig at estrous and diestrous. Theriogenology 119, 60–68. doi: 10.1016/j.theriogenology.2018.06.022
Thingholm, L. B., Rühlemann, M. C., Koch, M., Fuqua, B., Laucke, G., Boehm, R., et al. (2019). Obese individuals with and without Type 2 diabetes show different gut microbial functional capacity and composition. Cell Host Microbe 26, 252.e–264.e. doi: 10.1016/j.chom.2019.07.004
Thompson, G. R., and Trexler, P. C. (1971). Gastrointestinal structure and function in germ-free or gnotobiotic animals. Gut 12, 230–235. doi: 10.1136/gut.12.3.230
Tosca, L., Froment, P., Solnais, P., Ferré, P., Foufelle, F., and Dupont, J. (2005). Adenosine 5′-monophosphate- protein kinase regulates progesterone secretion in rat granulosa cells. Endocrinology 146, 4500–4513. doi: 10.1210/en.2005-0301
Toyama, H., Murase, H., Sato, F., Kawada, Y., Inoue, R., Watanabe, G., et al. (2018). Change of microbiota composition and sex hormones during estrus cycles in mare. J. Reprod. Dev. 1.
Van Wettere, W. H. E. J., Weaver, A. C., Greenwood, E. C., Terry, R., Hughes, P. E., and Kind, K. L. (2017). Controlling lactation oestrus: the final frontier for breeding herd management. Mol. Reprod. Dev. 84, 883–896. doi: 10.1002/mrd.22838
Velagapudi, V. R., Hezaveh, R., Reigstad, C. S., Gopalacharyulu, P., Yetukuri, L., Islam, S., et al. (2010). The gut microbiota modulates host energy and lipid metabolism in mice. J. Lipid Res. 51, 1101–1112. doi: 10.1194/jlr.M002774
Wallace, J. G., Potts, R. H., Szamosi, J. C., Surette, M. G., and Sloboda, D. M. (2018). The murine female intestinal microbiota does not shift throughout the estrous cycle. PLoS One 13:e0200729. doi: 10.1371/journal.pone.0200729
Wu, X., Shang, Y., Wei, Q., Chen, J., Zhang, H., Chen, Y., et al. (2020). Gut microbiota in dholes during estrus. Front. Microbiol. 11:575731. doi: 10.3389/fmicb.2020.575731
Xu, S., Zhang, P., and Cao, M. (2019). Microbial mechanistic insights into the role of sweet potato vine on improving health in chinese meishan gilt model. Animals (Basel). 9:632. doi: 10.3390/ani9090632
Yang, H., Huang, X., and Fang, S. (2016). Uncovering the composition of microbial community structure and metagenomics among three gut locations in pigs with distinct fatness. Sci. Rep. 6:27427. doi: 10.1038/srep27427
Keywords: Diannan small ear pig, sows, diestrus, metestrus, intestinal microbiota, 16S rRNA, LC-MS
Citation: Guan X, Zhu J, Sun H, Zhao X, Yang M, Huang Y, Pan H, Zhao Y and Zhao S (2022) Analysis of Gut Microbiota and Metabolites in Diannan Small Ear Sows at Diestrus and Metestrus. Front. Microbiol. 13:826881. doi: 10.3389/fmicb.2022.826881
Received: 01 December 2021; Accepted: 04 March 2022;
Published: 18 April 2022.
Edited by:
Junjun Wang, China Agricultural University, ChinaReviewed by:
Shiyu Tao, Huazhong Agricultural University, ChinaCopyright © 2022 Guan, Zhu, Sun, Zhao, Yang, Huang, Pan, Zhao and Zhao. This is an open-access article distributed under the terms of the Creative Commons Attribution License (CC BY). The use, distribution or reproduction in other forums is permitted, provided the original author(s) and the copyright owner(s) are credited and that the original publication in this journal is cited, in accordance with accepted academic practice. No use, distribution or reproduction is permitted which does not comply with these terms.
*Correspondence: Sumei Zhao, emhhb3NtMjAwOUAxMjYuY29t
†These authors have contributed equally to this work
Disclaimer: All claims expressed in this article are solely those of the authors and do not necessarily represent those of their affiliated organizations, or those of the publisher, the editors and the reviewers. Any product that may be evaluated in this article or claim that may be made by its manufacturer is not guaranteed or endorsed by the publisher.
Research integrity at Frontiers
Learn more about the work of our research integrity team to safeguard the quality of each article we publish.