- State Key Laboratory of Animal Nutrition, Institute of Animal Sciences, Chinese Academy of Agricultural Sciences, Beijing, China
High nitrogen utilization efficiency (NUE) is important for increasing milk protein production and decreasing the feed nitrogen cost and nitrogen emission to the environment. Currently, there is a limited whole picture of the relationship between ruminal bacteriome and the NUE of dairy cows, even though some information has been revealed about the bacteriome and milk or milk protein production of dairy cows. The purpose of this study was to compare the rumen bacterial community in dairy cows with different nitrogen utilization efficiency under the same diet. The natural abundance of 15N between the animal proteins and diet (Δ15N) was used as a simple, non-invasive, and accurate biomarker for NUE in ruminants to mark the individual variation. Dairy cows with high NUE (HE_HP, n = 7), medium NUE (ME_MP, n = 7), and low NUE (LE_LP, n = 7) were selected from 284 Holstein dairy cows with the same diet. Measurement of the rumen fermentation indices showed that the proportion of propionate was higher in HE_HP cows and ME_MP cows than in LE_LP cows (P < 0.05). The diversity of rumen bacterial community was higher in LE_LP cows than in ME_MP cows and HE_HP cows by 16S rRNA sequencing analysis (P < 0.05). Moreover, at the genus level, the relative abundances of Succinivibrionaceae_UCG_001, uncultured_Selenomonadaceae, and Acidaminococcus were higher in HE_HP cows than in LE_LP cows (P < 0.05). Interestingly, we found that these bacteria were positively correlated with milk protein yield and negatively correlated with Δ15N (P < 0.05). However, Clostridia_UCG_014, Saccharofermentans, Bacilli_RF39, and Desulfovibrio were lower in HE_HP cows and ME_MP cows than in LE_LP cows (P < 0.05), which were negatively correlated with milk protein yield and positively correlated with Δ15N (P < 0.05). In conclusion, the study showed that the diversity and relative abundances of rumen bacteria differed among different NUE cows, indicating that rumen bacteriome contributes to nitrogen metabolism in dairy cows.
Introduction
With the development of animal husbandry, most of the nitrogen intake by dairy cows is excreted by feces and urine. Nitrogen excretion leads to emission of greenhouse gases by livestock, followed by nitrogen loss in soil (N2O), volatilization of NH3, and leaching of NO3–, to environment pollution, aquatic and terrestrial eutrophication, and acidification (Angelidis et al., 2019). Oenema and Tamminga (2005) showed that the global amount of nitrogen annually excreted by livestock ranged from 8 × 107 T to 1.3 × 108 T, and the production of cattle contributed 60% of the total emissions. Therefore, it is necessary to improve the nitrogen utilization efficiency (NUE) of dairy cows by dietary regulation. NUE can be affected by heredity, nutrition, and management (Wang et al., 2007; Broderick et al., 2008; Cantalapiedra-Hijar et al., 2014)(Broderick et al., 2008; Wang et al., 2007; Cantalapiedra-Hijar et al., 2014). It was reported that the NUE of dairy cows was still at a low level (only ∼25%) (Huhtanen and Hristov, 2009), and more nitrogen was emitted to the environment (Calsamiglia et al., 2010).
Stable isotope fractionation, a result of the change in the ratio of heavy to light isotope (15N/14N), can be used to characterize the nutrition status of animals (Lavery and Ferris, 2021). δ15N is the ratio of 15N/14N in the animal minus 15N/14N ratio in the standard. N partitioning is different in plasma, feces, and milk compared to diet. It was reported that N isotope fractionation can predict NUE (Cheng et al., 2013). Plasma nitrogen isotopic fractionation (Δ15N = δ15Nplasma - δ15Ndiet) can be especially used as a predictor to effectively assess NUE (Cheng et al., 2016). Cantalapiedra-Hijar et al. (2018) suggested that Δ15N could be used to predict NUE variation across diets and individuals. Δ15N was demonstrated to be negatively correlated with NUE and the synthesis of microbe protein under different conditions (Cheng et al., 2016).
Rumen microbiota can convert fiber into digestible substances such as volatile fatty acid (VFAs) and microbial proteins. Ruminal VFAs produced by rumen microbial fermentation can provide 70% of the energy requirements in dairy cows (Matthews et al., 2019). Moreover, the bacteria in the rumen accounts for more than 95% of the microbial community, which is the main contributor to the performance of dairy cows (Castillo-Gonzalez et al., 2014). Li et al. (2019) found that the microbial composition and function between high residual feed intake steers and low residual feed intake steers were different, suggesting that microbiome interactions are the major contributor to the variations of feed efficiency. Xue et al. (2018) revealed that pan and core bacteriome were associated with milking traits in lactating dairy cows which were fed the same diet under the same environment, and they also found that the abundance of Sharpea was higher in high-milk-protein-yield (MPY) cows than in low-MPY cows. Succinivibrio and Clostridium were enriched in low-MPY cows compared to high-MPY cows. Although specific bacteria were related to milk composition (Jami et al., 2014), there are few studies on uncovering the function of rumen bacteria to NUE. The interaction of rumen bacteria and NUE is not clear.
In this study, Δ15N and MPY, as NUE indicators, were used to discuss NUE’s relationship with the rumen bacteriome. The objective of the study was to reveal the potential effects of rumen bacteriome on NUE in dairy cows.
Materials and Methods
Animals and Experimental Design
The experiment procedures were approved by the animal care committee (approval number: ISA2020-82). A total of 284 healthy lactating Holstein dairy cows (parity = 2; days in milk = 48 ± 1, mean ± SEM) were selected from 4,086 dairy cows in a commercial dairy farm. The dairy cows were fed an identical diet (Supplementary Table 1) and had free access to water. Milk yield was recorded for a month, and milk samples were collected on the last day at a volume ratio of 4:3:3 homologized to the morning (0500h), afternoon (1400h), and evening (1700h). Milk protein was tested by infrared analysis using near-infrared spectroscopy. Milk protein yield was calculated using milk protein content multiplied by the milk yield. Milk urea nitrogen was measured with the Urea Assay Kit (Nanjing Jiancheng Bioengineering Institute, Nanjing). Blood was collected using a 10-ml heparin anticoagulant tube from the caudal vein within 2 h after morning feeding. The blood samples were centrifuged at 4,000 × g for 5 min to obtain plasma and stored at −20°C. The plasma 15N and diet 15N were determined by Elemental Analysis-Isotope Ratio Mass (Isoprime100, England). Δ15N was calculated using δ15N plasma - δ15N diet (Oenema and Tamminga, 2005). Based on Δ15N and MPY, 7 high NUE [HE_HP: 1.9‰ < Δ15N < 2.2‰; 1.7 kg/day < MPY < 2.0 kg/day, days in milk (DIM) = 59 ± 9] dairy cows, 7 medium NUE (ME_MP: 2.2‰ < Δ15N < 2.4‰; 1.4 kg/day < MPY < 1.7 kg/day, DIM = 54 ± 15) dairy cows, and 7 low NUE (LE_LP: 2.4 ‰ < Δ15N < 2.7 ‰; 1.0 kg/day < MPY < 1.4 kg/day, DIM = 63 ± 9) dairy cows were selected (Figure 1). The rumen liquid was collected within 2 h after the morning feeding using oral stomach and tube and stored in liquid nitrogen immediately.
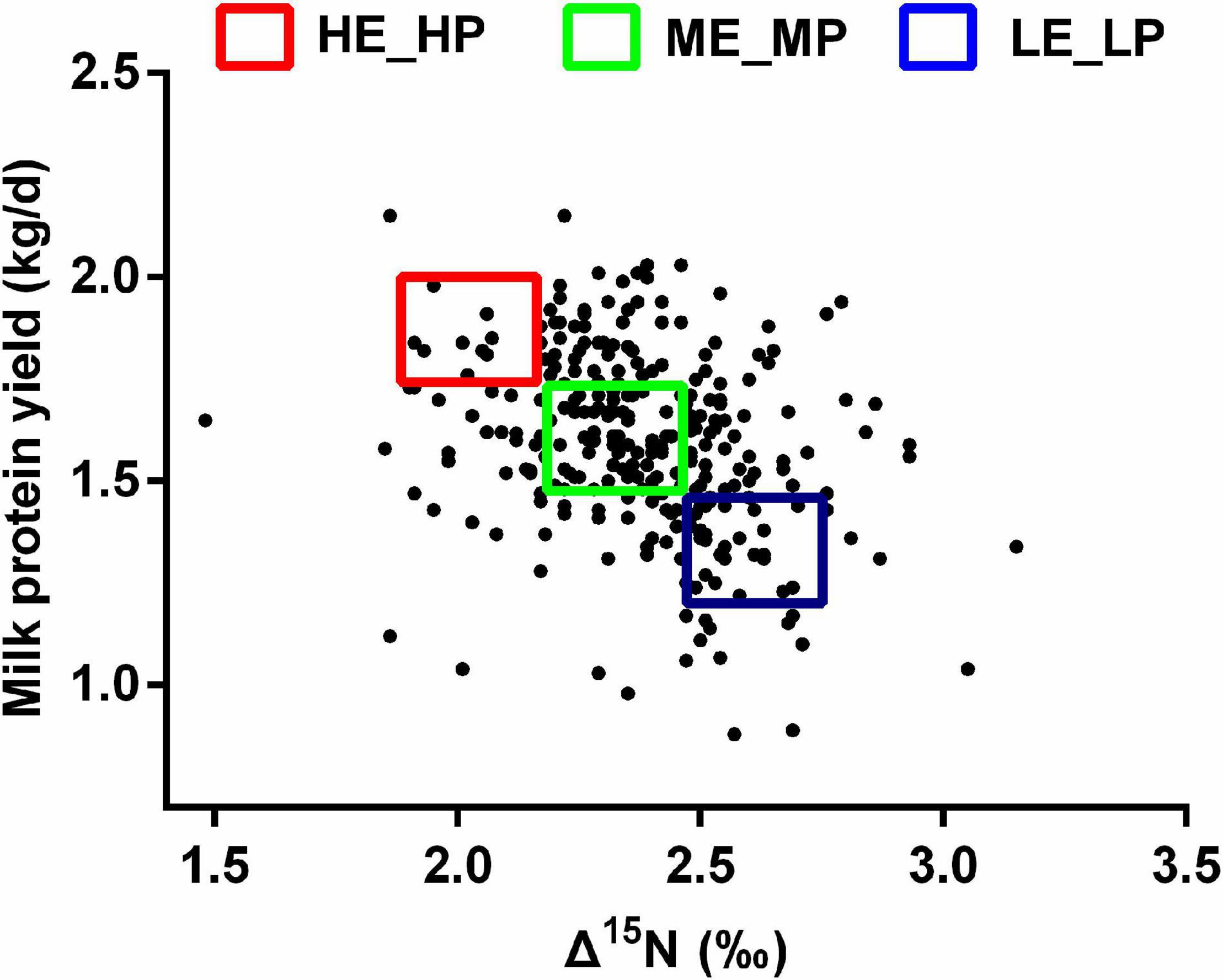
Figure 1. Scatter plot of Δ15N and milk protein yield among the HE_HP cows, ME_MP cows, and LE_LP cows.
Rumen Fermentation Parameter Detection
The rumen liquid was centrifuged at 13,000 × g at 4°C for 5 min to obtain the supernatant. Then, 600 μl of the supernatant was mixed with 50 μl of 25% metaphosphoric acid and was centrifuged at 12,000 × g for 20 min to obtain the supernatant for the analysis of ammonia nitrogen and VFAs. The ammonia nitrogen concentrations were determined using phenol-sodium hypochlorite colorimetric analysis (Broderick and Clayton, 1997). The proportion of VFAs was determined using GC (Agilent, 7890A) (Liang et al., 2017).
DNA Extraction and 16S rRNA Gene Sequencing
Total DNA of rumen liquid was extracted from the rumen liquid of 21 dairy cows using cetyl trimethyl ammonium bromide (Coolaber, Beijing) plus bead-beating method (Minas et al., 2011). The qualities and quantities of the DNA samples were measured using NanoDrop (Thermo, Beijing). The DNA was amplified using primers 341F (5′-CCTAYGGGRBGCASCAG-3′) and 806R (5′-GGACTACNNGGGTATCTAAT-3′), which target the V3–V4 region of the bacterial 16S rRNA gene. The reactions were performed in a 50-μl reaction mixture containing 2 μl DNA, 3.0 μl of each primer, 1.0 μl of dNTP, 0.25 of DNA polymerase (Takara, Dalian), 5 μl of 10 × PCR buffer, and 38.75 of ddH2O. The PCR cycling procedures were as follows: 95°C for 5 min, 30 cycles of 94°C for 30 s, 50°C for 30 s, 72°C for 30 s, and followed by 72°C for 15 min. The amplicon sequencing library was constructed using TruSeq Nano DNA LT Library Prep Kit (Illumina, America). After the library quality control was finished, the library was sequenced on an Illumina HiSeq platform (Illumina, America).
Bioinformatic Analysis
Reads were removed primers, demultiplexed, and quality-filtered using Vsearch (Rognes et al., 2016). The reads were truncated at any site of >3 sequential bases receiving a quality score of <Q20, and the reads with <75% (of total read length) consecutive high-quality base calls were removed. Operational taxonomic units (OTU) were analyzed using QIIME 2.0 and clustered at 97% similarity using Vsearch (Haas et al., 2011). OTUs were filtered based on the number of samples in an out <10 and aligned using MAFFT. The phylogenetic tree was constructed using FASTTREE. OTUs were further conducted for species annotation using SKLEARN based on SILVA-138 database. Data rarefying was performed using the minimum size of reads in a sample. The statistical bioinformatics analysis of this study was performed using MicrobiomeAnalyst (Chong et al., 2020). The OTU-level alpha diversity of rumen bacteria was obtained using Chao1 index, ACE index, Shannon index, and Simpson index. The OTU-level beta diversity was performed based on weighted UniFrac and visualized by principal coordinate analysis (PCoA) using the phyloseq package in R (McMurdie and Holmes, 2013). Linear discriminant analysis effect size (LEfSe) was performed on non-parametric factorial Kruskal–Wallis sum-rank test to identify bacteria with significantly different abundances in the three groups (Segata et al., 2011), followed by linear discriminant analysis (LDA) to calculate the effect size of each differently abundant bacteria. The heat map of bacteria and MPY, Δ15N was generated using RevolverMaps.1
Statistical Analysis
Lactation performance (DIM, milk yield, milk protein, milk urea nitrogen, and milk protein yield), Δ15N, rumen fermentation parameter concentrations (VFA, NH3–N), and alpha diversity indices of HE_HP cows, ME_MP cows, and LE_LP cows were analyzed using one-way ANOVA. The relative abundances of bacteria and alpha diversity indices among the three groups were compared using Kruskal–Wallis test. Dunn’s multiple-comparisons test was used for multiple comparisons of means among the three groups. Statistical analysis was performed using GraphPad Prism 7.0, with P < 0.05 considered as statistical significance. LEfSe was used to compare the relative abundances of rumen bacteria in HE_HP cows, ME_MP cows, and LE_LP cows, and significant differences were considered by a LDA score >2 and P < 0.05. Correlation analysis between the specific bacteria and NUE was performed using Spearman’s correlation.
Results
Production and Rumen Volatile Fatty Acid Related to Nitrogen Utilization Efficiency
As to the selected 21 dairy cows, the milk yield was 44.37 ± 1.75 kg/day (mean ± SEM), the MPY of 21 dairy cows was 1.50 ± 0.06 kg/day, Δ15N was 2.30 ± 0.05 ‰, the composition of acetate was 60.22 ± 0.60%, propionate was 27.15 ± 0.57%, isobutyrate was 0.47 ± 0.02%, butyrate was 9.79 ± 0.22%, isovalerate was 0.84 ± 0.04%, valerate was 1.52 ± 0.08%, and NH3–N was 8.85 ± 0.67 mg/dl. Milk yield and MPY in HE_HP were the highest among the three groups (P < 0.01) and in LE_LP cows were the lowest. In contrast, Δ15N in HE_HP cows was the lowest and in LE_LP cows was the highest (P < 0.01; Table 1). The DIM, milk protein, and milk urea nitrogen showed no significance among the HE_HP cows, ME_MP cows, and LE_LP cows (P > 0.05). Furthermore, the ruminal levels of NH3–N and the proportion of butyrate and valerate were not different among the three groups (P > 0.05). However, the HE_HP cows exhibited a significantly lower concentration of acetate (58.89%) and a higher concentration of propionate (28.87%) compared to LE_LP cows (P < 0.05).
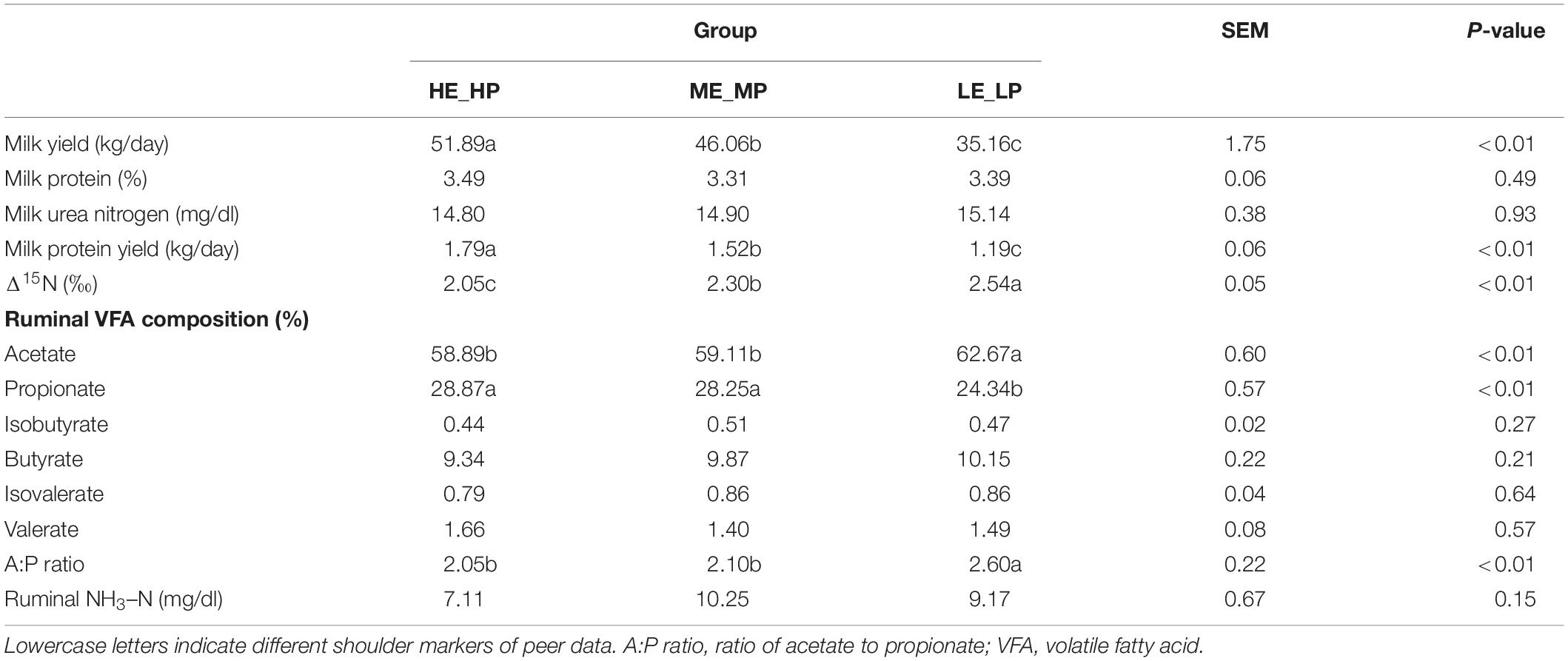
Table 1. The production, nitrogen utilization efficiency indexes, and rumen fermentation parameters of dairy cows.
Rumen Bacterial Diversity Related to Nitrogen Utilization Efficiency
Amplicon sequencing of the 16S rRNA generated a total of 1,647,027 high-quality sequences and 8,987 OTUs across 21 samples (Supplementary Figure 1). The rarefaction curves revealed that the sequencing depth was sufficient to cover most species of rumen bacteria (Supplementary Figure 2). The Chao1 index and ACE index (P > 0.05) were not significantly different in HE_HP cows, ME_MP cows, and LE_LP cows (Figures 2A,B). The Shannon index and Simpson index (P < 0.01) were significantly higher in LE_LP cows compared to HE_HP cows and ME_MP cows (Figures 2C,D). The PCoA based on weighted UniFrac distances showed that the bacterial composition between the HE_HP and LE_LP groups was different (PERMANOVA, P < 0.01), while the ME_MP group had no significant difference compared to the HE_HP group (Figure 3), and the ME_MP group had the most homogeneous community distribution compared to the other two groups.
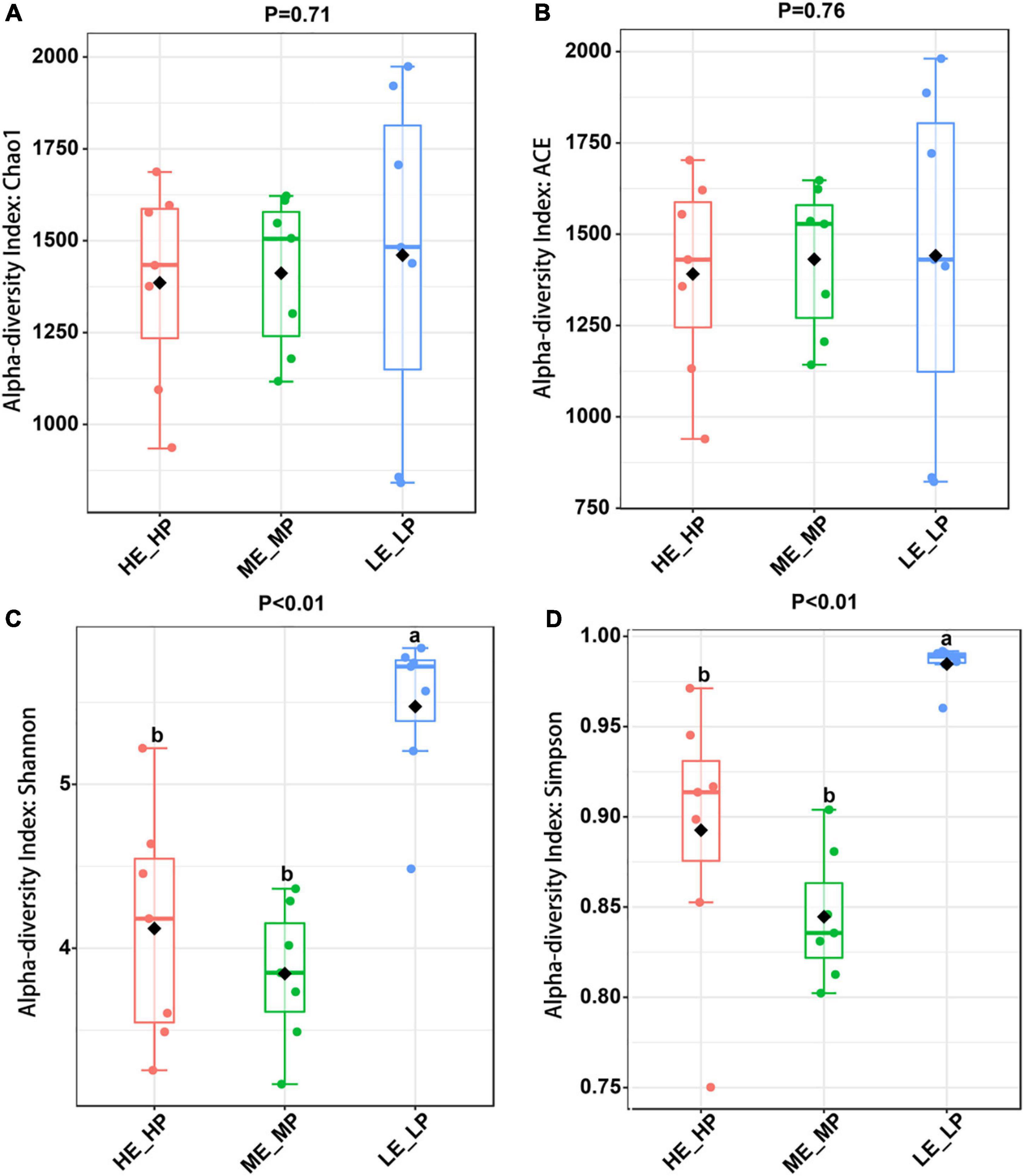
Figure 2. Alpha diversity analysis of rumen microbiota in different nitrogen utilization efficiency groups. (A) Chao 1 index among the HE_HP cows, ME_MP cows, and LE_LP cows. (B) ACE index among the HE_HP cows, ME_MP cows, and LE_LP cows. (C) Shannon index among the HE_HP cows, ME_MP cows, and LE_LP cows. (D) Simpson index among the HE_HP cows, ME_MP cows, and LE_LP cows.
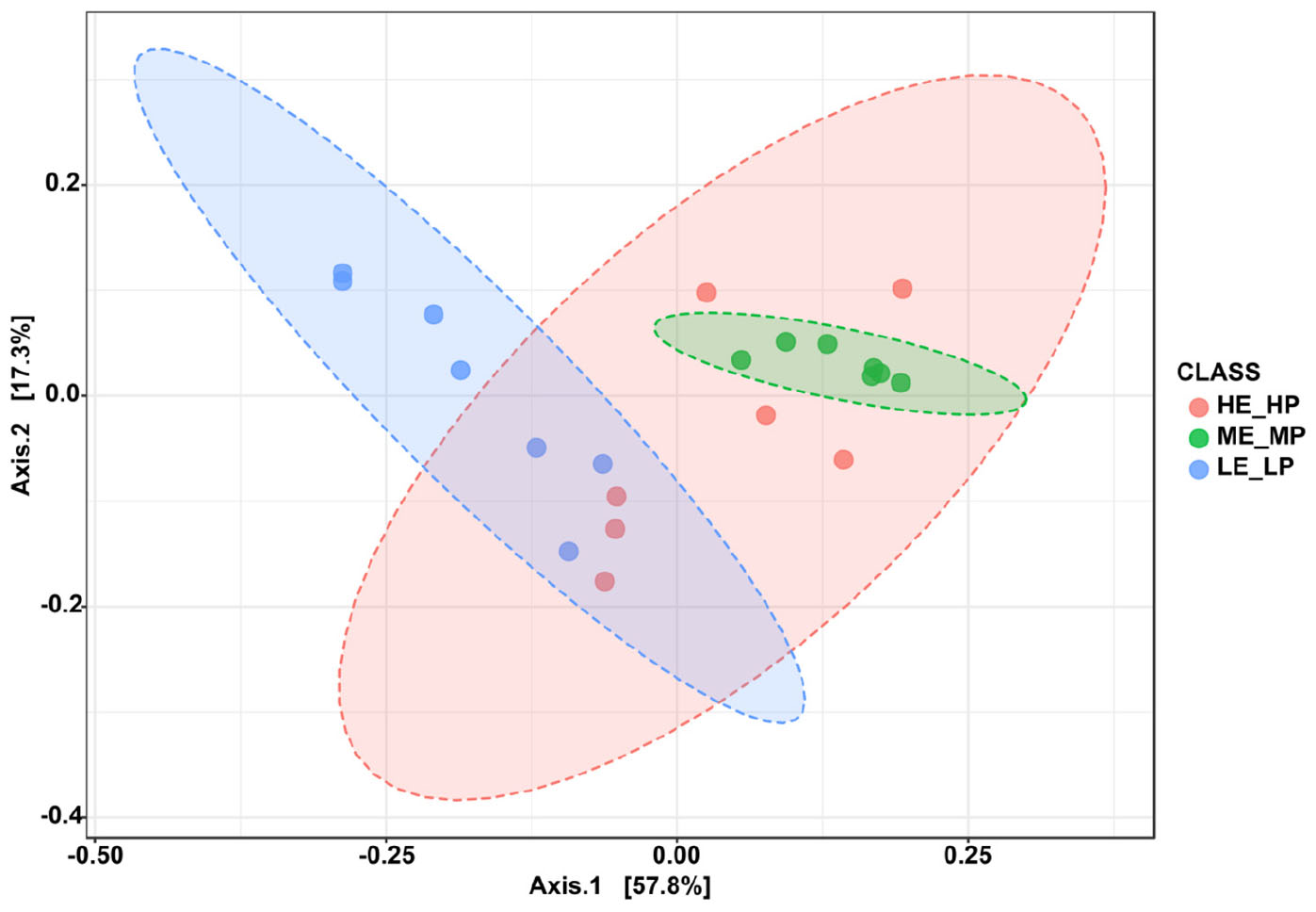
Figure 3. Principal coordinate analysis of bacteria beta diversity at species level among the HE_HP cows, ME_MP cows, and LE_LP cows.
Different Rumen Bacterial Taxa in Dairy Cows With Different Nitrogen Utilization Efficiency
A total of 24 bacterial phyla were identified; the predominant bacterial phyla were Bacteroidota (41.24 ± 8.02%), Firmicutes (30.25 ± 11.96%), and then Proteobacteria (25.78 ± 14.97%) (Supplementary Figure 3). At the family level, the predominant bacterial families were Prevotellaceae (32.76 ± 9.4%), Succinivibrionaceae (23.48 ± 16.25%), and Lachnospiraceae (12.84 ± 4.79%) (Supplementary Figure 4). At the genus level, a total of 326 genera were identified; Prevotella (29.85 ± 9.53%), Succinivibrionaceae_UCG_001 (23.42 ± 16.38%), Muribaculaceae (4.61 ± 3.95%), and Succiniclasticum (3.35 ± 2.91%) were the most abundant genera (Figure 4).
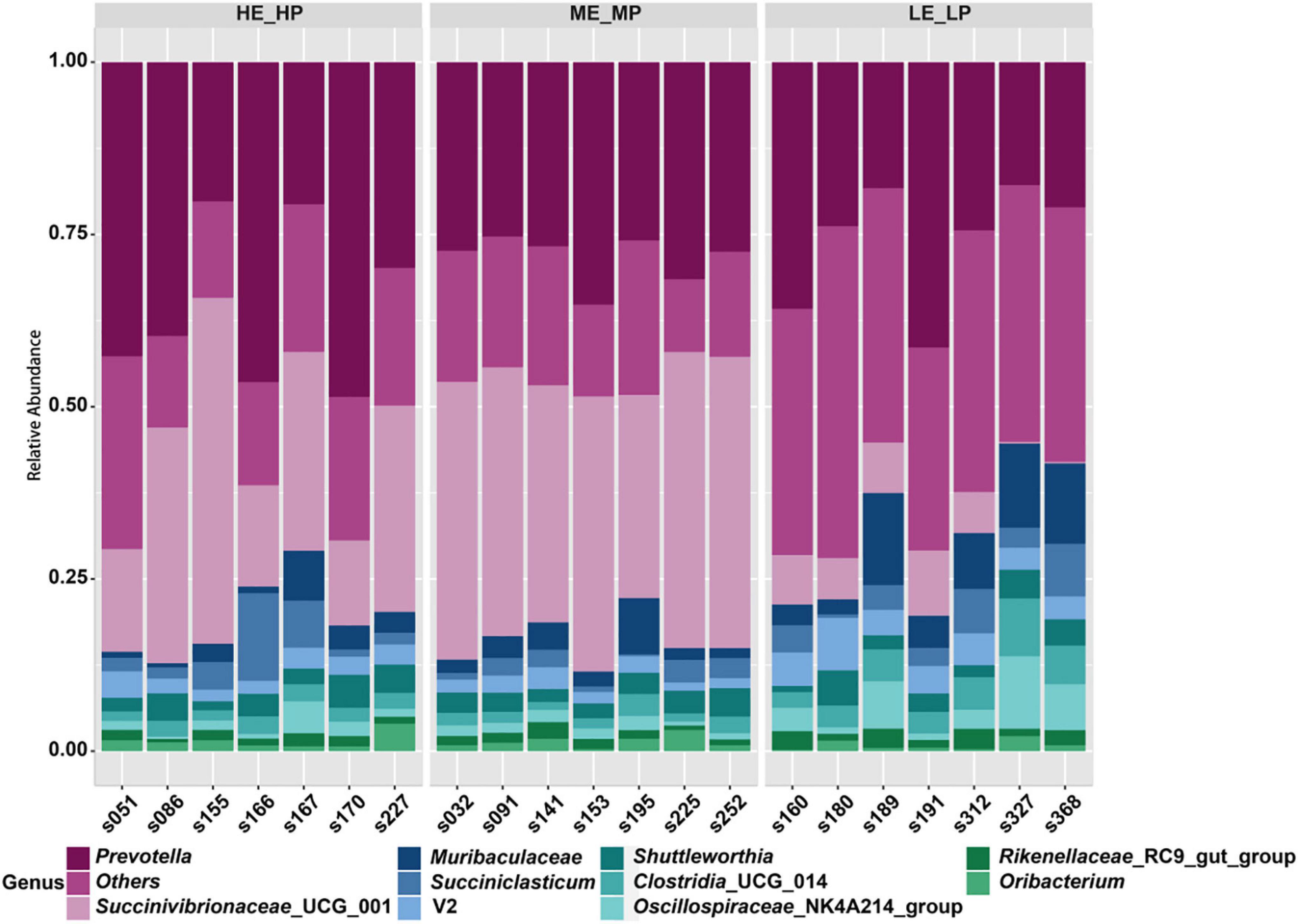
Figure 4. Relative abundances of rumen microbiota at the genus level among the HE_HP cows, ME_MP cows, and LE_LP cows.
The different abundant bacteria at the genus level among the HE_HP cows, ME_MP cows, and LE_LP cows were revealed by LEfSe (Figure 5). The relative abundance of Succinivibrionaceae_UCG_001, uncultured_Selenomonadaceae, and Acidaminococcus was higher in HE_HP cows (P < 0.05). Moreover, Clostridia_UCG_014, Saccharofermentans, Bacilli_ RF39, and Desulfovibrio were higher in LE_LP cows (P < 0.01). There were differences in the abundances of bacterial taxa among the three groups (Table 2). Meanwhile, LEfSe was used to screen the different OTUs (Supplementary Table 2). The genera corresponding to OTUs with significant differences included Succinivibrionaceae_UCG_001, Shuttleworthia, Clostridia_UCG_014, uncultured_Selenomonadaceae, Lachnos piraceae_NK3A20_group, Christensenellaceae_R-7_group, Desu lfovibrio, Saccharofermentans, Allisonella, and Eubact erium_eligens_group (P < 0.05), and there was 20% less genus Succinivibrionaceae_UCG_001 in LE_LP cows than in the other two groups. Moreover, the most differently abundant OTUs belonged to the phylum of Firmicutes.
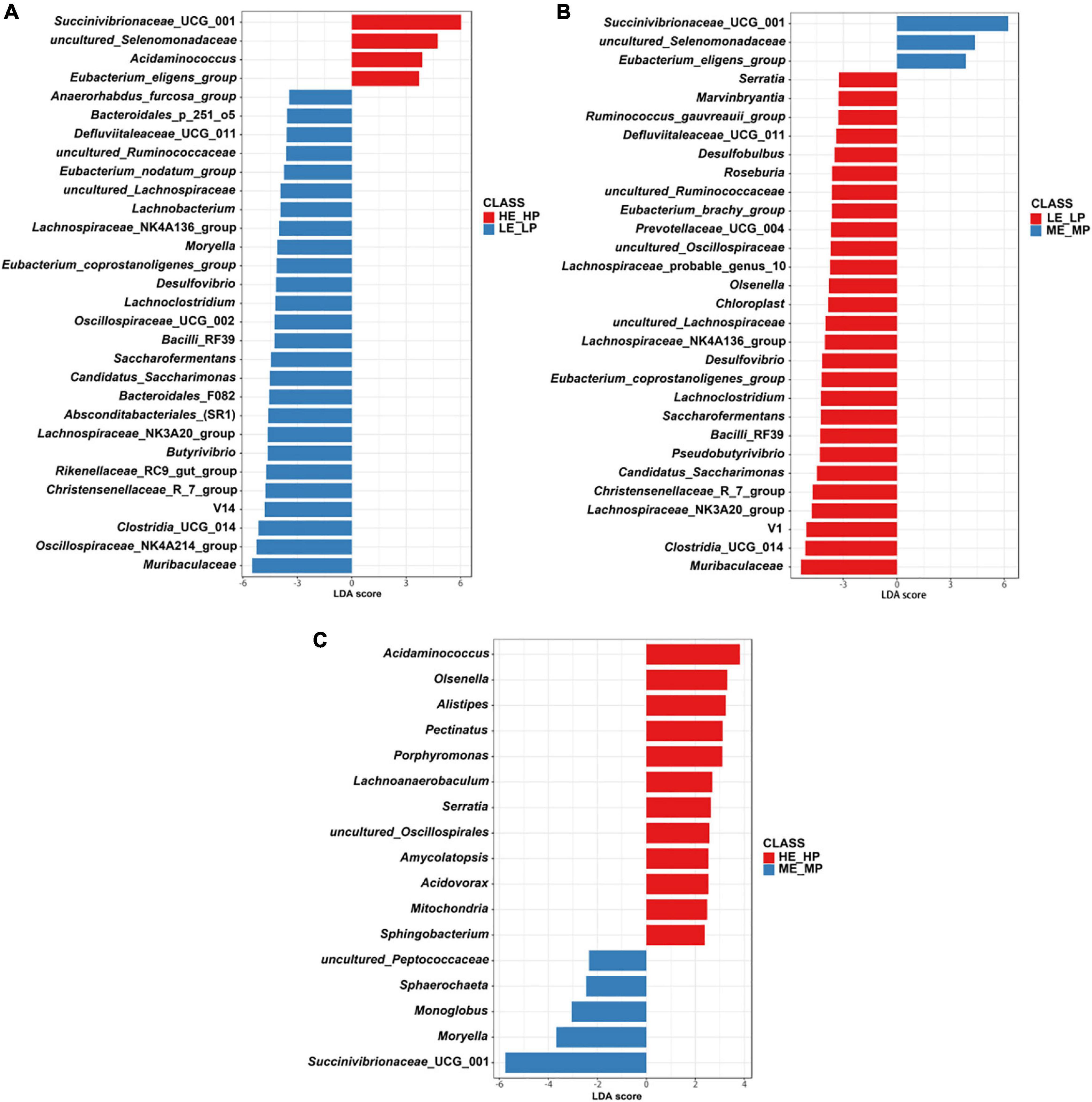
Figure 5. Comparison of the relative abundances of rumen bacteria among the HE_HP cows, ME_MP cows, and LE_LP cows by linear discriminant analysis effect size (P < 0.05; linear discriminant analysis, >2). (A) Different rumen bacteria between HE_HP cows and LE_LP cows. (B) Different rumen bacteria between LE_LP cows and ME_MP cows. (C) Different rumen bacteria between HE_HP cows and ME_MP cows.
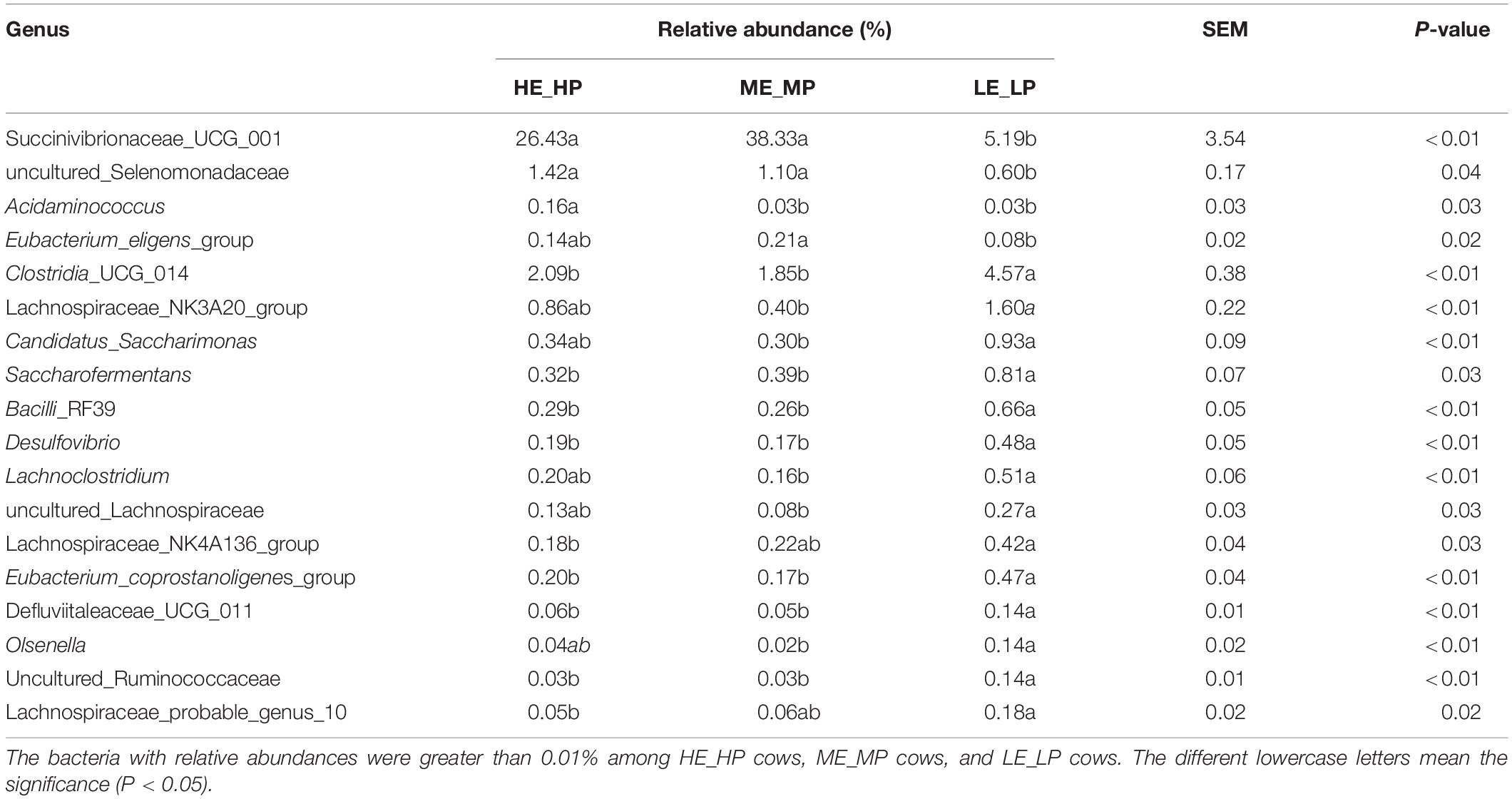
Table 2. Relative abundances of specific bacteria in dairy cows with different nitrogen utilization efficiency.
Relationships of Different Rumen Bacteria With Δ15N and Milk-Protein-Yield
In the study, in all of the 326 encountered genera, the relative abundance of 127 bacterial taxa was >0.01%. We analyzed the 127 bacterial taxa and Δ15N and MPY using Spearman’s correlation. The correlation analysis revealed that 32 bacterial taxa were significantly correlated with Δ15N and MPY (Figure 6). The results showed that Δ15N and MPY possessed remarkable relationships with bacterial taxa, including five positive relationships and 30 negative relationships (P < 0.05). Among them, Succinivibrionaceae_UCG_001, uncultured_Selenomonadaceae, Acidaminococcus, Eubacterium_xylanophilum_group, and Lachnospiraceae_NC2004_group were positively correlated with MPY and negatively correlated with Δ15N. The scatter plots of the specific bacteria and Δ15N and MPY are shown in Figure 7, which shows that the same genus of dairy cows with different NUE had different abundance levels, but Clostridia_UCG_014, Saccharofermentans, Bacilli_RF39, and Desulfovibrio were negatively correlated with MPY and positively correlated with Δ15N (P < 0.05).
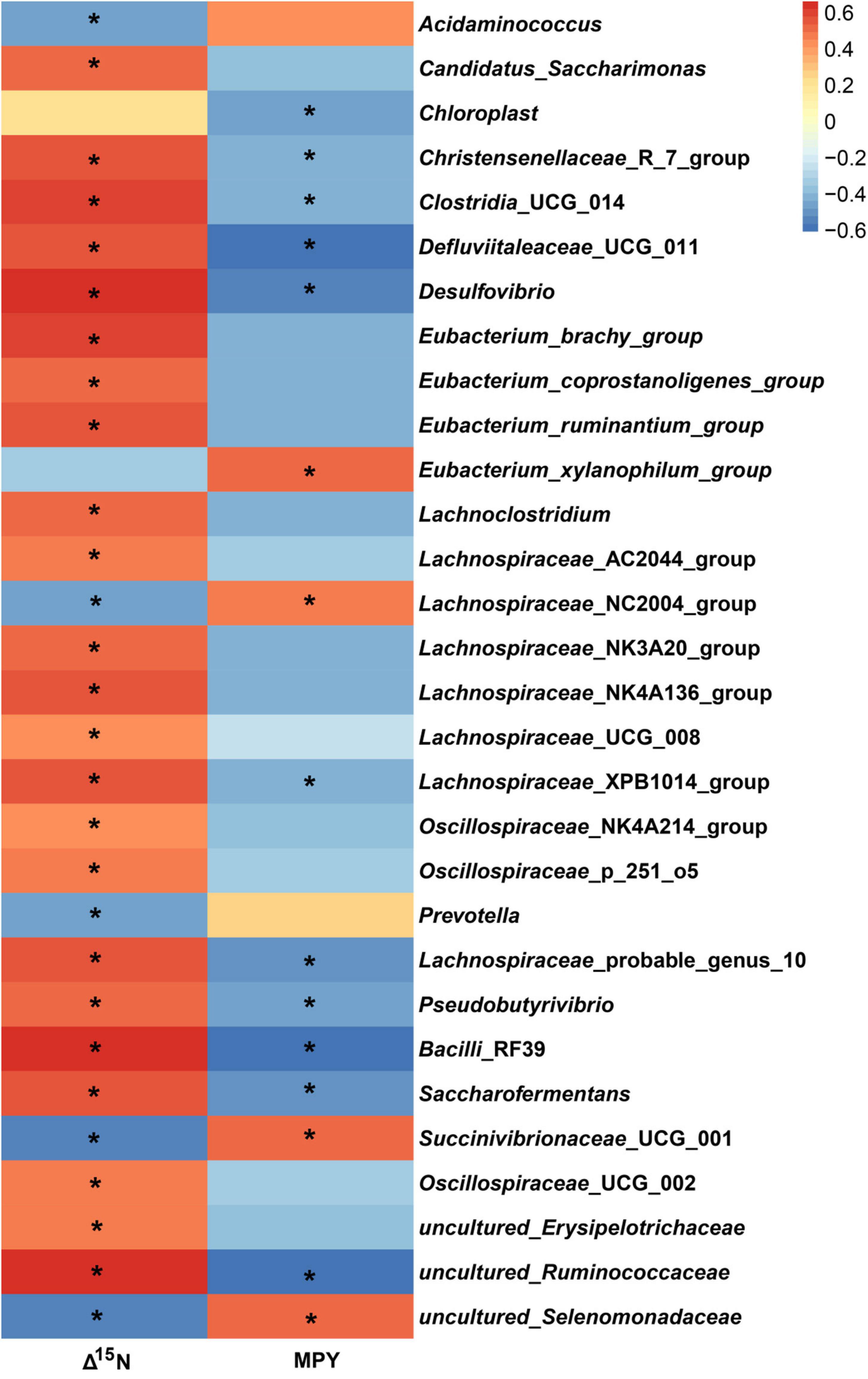
Figure 6. Spearman correlations between specific bacterial genera, Δ15N, and MPY. The asterisk means significant difference (P < 0.05).
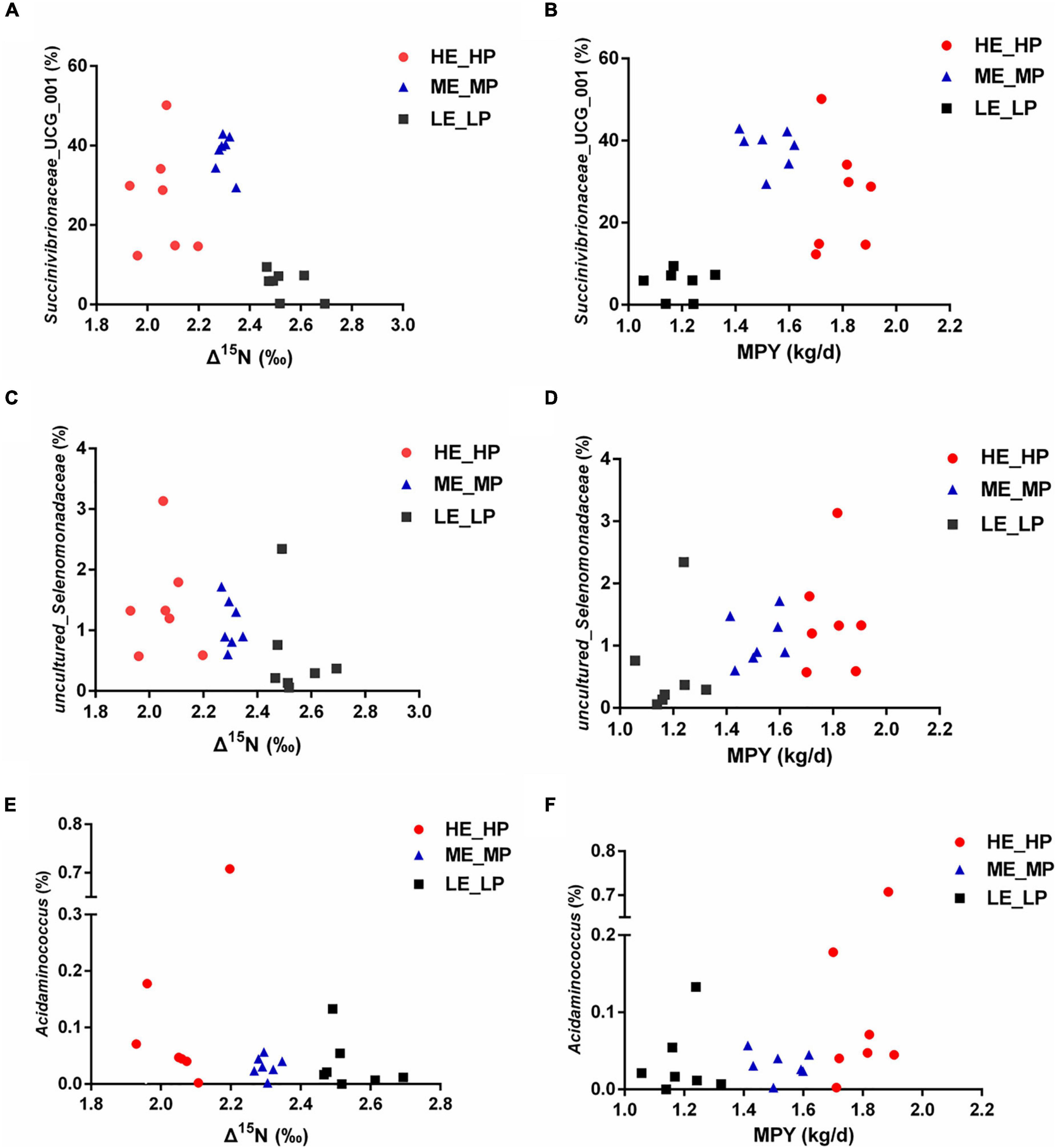
Figure 7. Relative abundances of rumen bacteria and Δ15N and milk protein yield (MPY). (A) Scatter plot of Succinivibrionaceae_UCG_001 and Δ15N. (B) Scatter plot of Succinivibrionaceae_UCG_001 and MPY. (C) Scatter plot of uncultured_Selenomonadaceae and Δ15N. (D) Scatter plot of uncultured_Selenomonadaceae and MPY. (E) Scatter plot of Acidaminococcus and Δ15N. (F) Scatter plot of Acidaminococcus and MPY.
Discussion
At present, the most effective way to improve the NUE of dairy cows is to match the dietary nitrogen content and energy supply during rumen fermentation by changing the diet composition (Reid et al., 2015). In addition, maximum NUE is usually achieved at the cost of some loss-of-production performance, but it can be achieved by understanding the key mechanisms that regulate nitrogen metabolism. The indexes commonly used to evaluate the NUE of dairy cows include blood urea nitrogen, milk urea nitrogen, milk protein yield, and Δ15N (Kohn et al., 2005; Burgos et al., 2007; Warinner and Tuross, 2010). The Δ15N, compared to other indicators, can overcome the differences at an individual level and is better for large groups of cows (Cantalapiedra-Hijar et al., 2016). In recent years, several studies have proved Δ15N as a biomarker to assess NUE, and many scholars have pointed out that Δ15N was negatively correlated with NUE and rumen microbial protein synthesis efficiency (Cheng et al., 2011, 2014; Cantalapiedra-Hijar et al., 2015). MPY is an important index to measure the lactating performance of dairy cows, which is a new biomarker for high milk protein and high yield (Wu et al., 2018; Xue et al., 2019). However, the MPY is not necessarily high when NUE is high, which is not in line with our aims of efficiency and production. Thus, the cows were divided into HE_HP cows, ME_MP cows, and LE_LP cows according to Δ15N and MPY, and the rumen fermentation indexes were compared. The proportion of propionate and the ratio of acetate to propionate, which is mainly involved in carbohydrate metabolism, were higher in HE_HP cows and ME_MP cows than in LE_LP cows, suggesting that propionate had a closed correlation with the rumen nitrogen metabolism. The propionate produced in the rumen was absorbed and transferred to glucose which provided energy for milk protein synthesis. Similarly, Xue et al. (2019) also found that high-milk-production and high-protein-content cows had significantly more ruminal propionate compared to those with low milk production and protein content. However, ruminal NH3–N showed no significant changes among the three groups, which may be due to its low variation and levels (<11 mg/dl).
The rumen bacteria play an important role in dietary nutrient digestion and absorption. Furthermore, we detected that the diversity of rumen bacteria and specific rumen bacterial taxa contributed to NUE among the HE_HP cows, ME_MP cows, and LE_LP cows. The alpha diversity indices of Shannon and Simpson showed a lower bacterial diversity in HE_HP cows. Xue et al. (2020) compared the rumen microbial structure of high-MPY cows and low-MPY cows and found that the ruminal microbial richness in high-MPY cows was lower. It has been reported that the higher feed efficiency of dairy cows possessed a lower diversity, indicating that HE_HP cows can have higher feed efficiency than ME_MP cows and LE_LP cows and also suggesting that HE_HP cows have higher ruminal fermentation efficiency (Li and Guan, 2017). A lower diversity of the rumen microbiota has been identified in cows with a higher milk yield (Shabat et al., 2016), which is consistent with our results.
We revealed the difference in the relative abundances of rumen bacteria that may be associated with NUE. The family Succinivibrionaceae was higher in HE_HP cows and ME_MP cows than in LE_LP cows. Succinivibrionaceae, including 6 genera (Anaerobiospirillum, Succinivibrionaceae_UCG_001, Ruminobacter, Succinivibrionaceae_UCG_002, Succinimonas, and Succinivibrio), was the most active rumen bacterial family in beef (Li and Guan, 2017). Succinivibrionaceae is one of the contributors to producing succinate, and succinate can be used by other microorganisms to synthesize propionate and valerate, and the abundance of Succinivibrionaceae has been reported to be related to energy metabolism and methane emission (Wallace et al., 2015). Xue et al. (2020) pointed out that the methanogens in rumen had a lower relative abundance in high-MPY dairy cows, suggesting that these cows can produce less methane and sufficiently satisfy the higher performance of milk protein and milk yield. Succinivibrionaceae_UCG_001 composed the core rumen microbiota in young cattle and is the most abundant genus of the phylum Proteobacteria (Wang et al., 2019). In the study, we found that Succinivibrionaceae_UCG_001 was higher in HE_HP cows and ME_MP cows than in LE_LP cows, indicating that HE_HP cows and ME_MP cows have higher levels of propionate, a major substrate for gluconeogenesis, and it is enough to supply glucose for milk production to influence NUE. Furthermore, there are 6 OTUs belonging to Succinivibrionaceae_UCG_001 in the top 50 specific OTUs. It has been reported that Succinivibrionaceae_UCG_001 was negatively correlated with the ratio of acetate to propionate (Cao et al., 2020). The Spearman correlation analysis showed that Succinivibrionaceae_UCG_001 was positively related with MPY and negatively with Δ15N, which supported the important contribution of Succinivibrionaceae to nitrogen metabolism in dairy cows. Our results revealed that the production of propionate and acetate might affect the glucose supply, which could influence the NUE of dairy cows. The uncultured_Selenomonadaceae also had more abundance in HE_HP cows and ME_MP cows, and it was positively correlated with MPY and negatively correlated with Δ15N. It indicated that the following research on the uncultured_Selenomonadaceae was needed to reveal its contribution to NUE. Moreover, Acidaminococcus was higher in HE_HP cows than in ME_MP cows and LE_LP cows, and it was negatively related with Δ15N. Acidaminococcus has been demonstrated to utilize amino acids as the sole energy source to produce acetic and butyric acids, which were negatively associated with metabolic diseases such as obesity (Rogosa, 1969; Li et al., 2021). The effect of Acidaminococcus on ruminant NUE was still unclear, and more culturing studies are needed to find out its role in nitrogen metabolism.
However, the results also revealed that the relative abundances of Clostridia_UCG_014, Saccharofermentans, Bacilli_RF39, and Desulfovibrio were higher in LE_LP cows than in HE_HP cows and ME_MP cows (relative abundance, >0.1%). Thus, it might demonstrate that these four bacteria were negatively associated with NUE. Clostridia_UCG_014 is a kind of pro-inflammatory bacteria (Wang et al., 2021), and its high abundance potentially led to the production of more proinflammatory metabolites to interfere with nitrogen metabolism. Saccharofermentans isolated from sludge treating brewery wastewater can ferment cellulose and starch to produce acetate (Chen et al., 2010; Dai et al., 2016). A recent study has indicated the higher abundance of Saccharofermentans in the rumen of cows with laminitis (Opdahl et al., 2018). Bacilli_RF39 was a novel Tenericutes lineage and reported in goats and the human gut (Zhang et al., 2015; Nayfach et al., 2019). Due to the loss of ability to biosynthesize fatty acids, it is difficult to degrade the cellular membranes of plants for dairy cows. Desulfovibrio can reduce sulfate to sulfide compounds, which can block the oxidation of short-chain fatty acids in the rumen and compete with Firmicutes for energy substance and carbon source (Jia et al., 2012). In our study, Saccharofermentans, Bacilli_RF39, and Desulfovibrio were negatively correlated with MPY and were positively correlated with Δ15N, suggesting that those bacteria had a negative function in improving the rumen nitrogen metabolism. Although Δ15N is a good biomarker for NUE, it is not the actual NUE. Here we just found out the presence of related bacterial species to Δ15N. The activity and function of these bacteria contributing to the NUE which are more direct and important need to be revealed in the future. More productive data, e.g., individual feed intake, are beneficial to reveal the closely related rumen microbes to NUE, which needs to be addressed in future studies. In addition, microbes such as archaea, protozoa, and anaerobic fungi also had roles in ruminal nitrogen metabolism, but we were solely focused on the bacteria community in this study. Therefore, the contribution of archaea, protozoa, and anaerobic fungi on nitrogen utilization efficiency has to be revealed in the following studies.
Conclusion
The relative abundances and diversity of rumen bacteria in dairy cows were clearly different among high- and low-NUE cows. The relative abundances of Succinivibrionaceae, Selenomonadaceae, and Acidaminococcus were higher in the rumen of high-NUE cows, which positively related with MPY and negatively with Δ15N. The study indicated that ruminal bacteria was a potential regulation target to enhance the nitrogen utilization of dairy cows. However, the activity and function of these bacteria contributing to the NUE need to be studied in the future.
Data Availability Statement
The original contributions presented in the study are included in the article/Supplementary Material, further inquiries can be directed to the corresponding authors.
Ethics Statement
The animal study was reviewed and approved by Animal Care and Use Committee for Livestock of the Institute of Animal Sciences, Chinese Academy of Agricultural Sciences (approval number: ISA2020-82).
Author Contributions
MnL contributed to conceptualization, software, data curation, and writing—original draft. HZ performed data curation. MgL took charge of the software. NZ was in charge of supervision. SZ contributed to writing—review and editing. JW was in charge of administration. All authors contributed to the article and approved the submitted version.
Funding
This research was funded by the State Key Laboratory of Animal Nutrition (2004DA125184G2108), Scientific Research Project for Major Achievements of Agricultural Science and Technology Innovation Program (CAAS-ZDXT2019004), Agricultural Science and Technology Innovation Program (ASTIP-IAS12), and China Agriculture Research System of MOF and MARA.
Conflict of Interest
The authors declare that the research was conducted in the absence of any commercial or financial relationships that could be construed as a potential conflict of interest.
Publisher’s Note
All claims expressed in this article are solely those of the authors and do not necessarily represent those of their affiliated organizations, or those of the publisher, the editors and the reviewers. Any product that may be evaluated in this article, or claim that may be made by its manufacturer, is not guaranteed or endorsed by the publisher.
Supplementary Material
The Supplementary Material for this article can be found online at: https://www.frontiersin.org/articles/10.3389/fmicb.2022.815225/full#supplementary-material
Footnotes
References
Angelidis, A., Crompton, L., Misselbrook, T., Yan, T., Reynolds, C. K., and Stergiadis, S. (2019). Evaluation and prediction of nitrogen use efficiency and outputs in faeces and urine in beef cattle. Agr. Ecosyst. Environ. 280, 1–15.
Broderick, G. A., and Clayton, M. K. (1997). A statistical evaluation of animal and nutritional factors influencing concentrations of milk urea nitrogen. J. Dairy Sci. 80, 2964–2971. doi: 10.3168/jds.S0022-0302(97)76262-3
Broderick, G. A., Stevenson, M. J., Patton, R. A., Lobos, N. E., and Olmos Colmenero, J. J. (2008). Effect of supplementing rumen-protected methionine on production and nitrogen excretion in lactating dairy cows. J. Dairy Sci. 91, 1092–1102. doi: 10.3168/jds.2007-0769
Burgos, S. A., Fadel, J. G., and Depeters, E. J. (2007). Prediction of ammonia emission from dairy cattle manure based on milk urea nitrogen: relation of milk urea nitrogen to urine urea nitrogen excretion. J. Dairy Sci. 90, 5499–5508. doi: 10.3168/jds.2007-0299
Calsamiglia, S., Ferret, A., Reynolds, C. K., Kristensen, N. B., and van Vuuren, A. M. (2010). Strategies for optimizing nitrogen use by ruminants. Animal 4, 1184–1196. doi: 10.1017/S1751731110000911
Cantalapiedra-Hijar, G., Dewhurst, R. J., Cheng, L., Cabrita, A. R. J., Fonseca, A. J. M., Nozière, P., et al. (2018). Nitrogen isotopic fractionation as a biomarker for nitrogen use efficiency in ruminants: a meta-analysis. Animal 12, 1827–1837. doi: 10.1017/S1751731117003391
Cantalapiedra-Hijar, G., Fouillet, H., Huneau, J. F., Fanchone, A., Doreau, M., Nozière, P., et al. (2016). Relationship between efficiency of nitrogen utilization and isotopic nitrogen fractionation in dairy cows: contribution of digestion v. metabolism? Animal 10, 221–229. doi: 10.1017/S1751731115002025
Cantalapiedra-Hijar, G., Ortigues-Marty, I., Sepchat, B., Agabriel, J., Huneau, J. F., and Fouillet, H. (2015). Diet-animal fractionation of nitrogen stable isotopes reflects the efficiency of nitrogen assimilation in ruminants. Br. J. Nutr. 113, 1158–1169. doi: 10.1017/S0007114514004449
Cantalapiedra-Hijar, G., Peyraud, J. L., Lemosquet, S., Molina-Alcaide, E., Boudra, H., Nozière, P., et al. (2014). Dietary carbohydrate composition modifies the milk N efficiency in late lactation cows fed low crude protein diets. Animal 8, 275–285. doi: 10.1017/S1751731113002012
Cao, N., Wu, H., Zhang, X. Z., Meng, Q. X., and Zhou, Z. M. (2020). Calcium propionate supplementation alters the ruminal bacterial and archaeal communities in pre- and postweaning calves. J. Dairy Sci. 103, 3204–3218. doi: 10.3168/jds.2019-16964
Castillo-Gonzalez, A. R., Burrola-Barraza, M. E., Dominguez-Viveros, J., and Chavez-Martinez, A. (2014). Rumen microorganisms and fermentation microorganismos fermentación ruminal. Aerch. Med. Vet. 46, 349–361. doi: 10.4067/S0301-732X2014000300003
Chen, S., Niu, L., and Zhang, Y. (2010). Saccharofermentans acetigenes gen. nov., sp. nov., an anaerobic bacterium isolated from sludge treating brewery wastewater. Int. J. Syst. Evol. Microbiol. 60, 2735–2738. doi: 10.1099/ijs.0.017590-0
Cheng, L., Edwards, G. R., Dewhurst, R. J., Nicol, A. M., and Pacheco, D. (2016). The effect of dietary water soluble carbohydrate to nitrogen ratio on nitrogen partitioning and isotopic fractionation of lactating goats offered a high-nitrogen diet. Animal 10, 779–785. doi: 10.1017/S1751731115002335
Cheng, L., Kim, E. J., Merry, R. J., and Dewhurst, R. J. (2011). Nitrogen partitioning and isotopic fractionation in dairy cows consuming diets based on a range of contrasting forages. J. Dairy Sci. 94, 2031–2041. doi: 10.3168/jds.2010-3763
Cheng, L., Sheahan, A. J., Gibbs, S. J., Rius, A. G., Kay, J. K., Meier, S., et al. (2013). Technical note: nitrogen isotopic fractionation can be used to predict nitrogen-use efficiency in dairy cows fed temperate pasture. J. Anim. Sci. 91, 5785–5788. doi: 10.2527/jas.2012-5378
Cheng, L., Woodward, S. L., Dewhurst, R. J., Zhou, H., and Edwards, G. R. (2014). Nitrogen partitioning, energy use efficiency and isotopic fractionation measurements from cows differing in genetic merit fed low-quality pasture in late lactation. Anim. Prod. Sci. 54, 1651–1656. doi: 10.1071/AN14171
Chong, J., Liu, P., Zhou, G., and Xia, J. (2020). Using microbiome analyst for comprehensive statistical, functional, and meta-analysis of microbiome data. Nat. Protoc. 15, 799–821. doi: 10.1038/s41596-019-0264-1
Dai, Y., Yan, Z., Jia, L., Zhang, S., Gao, L., Wei, X., et al. (2016). The composition, localization and function of low-temperature-adapted microbial communities involved in methanogenic degradations of cellulose and chitin from Qinghai-Tibetan Plateau wetland soils. J. Appl. Microbiol. 121, 163–176. doi: 10.1111/jam.13164
Haas, B. J., Gevers, D., Earl, A. M., Feldgarden, M., Ward, D. V., Giannoukos, G., et al. (2011). Human microbiome consortium, Petrosino, J.F., Knight, R., Birren, B.W. Chimeric 16S rRNA sequence formation and detection in Sanger and 454-pyrosequenced PCR amplicons. Genome Res. 21, 494–504. doi: 10.1101/gr.112730.110
Huhtanen, P., and Hristov, A. N. (2009). A meta-analysis of the effects of dietary protein concentration and degradability on milk protein yield and milk N efficiency in dairy cows. J. Dairy Sci. 92, 3222–3232. doi: 10.3168/jds.2008-1352
Jami, E., White, B. A., and Mizrahi, I. (2014). Potential role of the bovine rumen microbiome in modulating milk composition and feed efficiency. PLoS One 9:e85423. doi: 10.1371/journal.pone.0085423
Jia, W., Whitehead, R. N., Griffiths, L., Dawson, C., Bai, H., Waring, R. H., et al. (2012). Diversity and distribution of sulphate-reducing bacteria in human faeces from healthy subjects and patients with inflammatory bowel disease. FEMS Immunol. Med. Microbiol. 65, 55–68. doi: 10.1111/j.1574-695X
Kohn, R. A., Dinneen, M. M., and Russek-Cohen, E. (2005). Using blood urea nitrogen to predict nitrogen excretion and efficiency of nitrogen utilization in cattle, sheep, goats, horses, pigs, and rats. Anim. Sci. 83, 879–889. doi: 10.2527/2005.834879x
Lavery, A., and Ferris, C. P. (2021). Proxy measures and novel strategies for estimating nitrogen utilisation efficiency in dairy cattle. Animals 11:343. doi: 10.3390/ani11020343
Li, F., and Guan, L. L. (2017). Metatranscriptomic profiling reveals linkages between the active rumen microbiome and feed efficiency in beef cattle. Appl. Environ. Microbiol. 83, e00061–17. doi: 10.1128/AEM.00061-17
Li, F., Hitch, T. C. A., Chen, Y., Creevey, C. J., and Guan, L. L. (2019). Comparative metagenomic and metatranscriptomic analyses reveal the breed effect on the rumen microbiome and its associations with feed efficiency in beef cattle. Microbiome 7:6. doi: 10.1186/s40168-019-0618-5
Li, J., Dong, L., Liu, Y., and Gao, J. (2021). Stimulation of Codonopsis pilosula polysaccharide on bifidobacterium of human gut bacteria in vitro. Evid. Based Complement Alternat. Med. 2021:9524913. doi: 10.1155/2021/9524913
Liang, Y. S., Li, G. Z., Li, X. Y., Lü, J. Y., Li, F. D., Tang, D. F., et al. (2017). Growth performance, rumen fermentation, bacteria composition, and gene expressions involved in intracellular pH regulation of rumen epithelium in finishing Hu lambs differing in residual feed intake phenotype. J. Anim. Sci. 95, 1727–1738. doi: 10.2527/jas.2016.1134
Matthews, C., Crispie, F., Lewis, E., Reid, M., O’Toole, P. W., and Cotter, P. D. (2019). The rumen microbiome: a crucial consideration when optimising milk and meat production and nitrogen utilisation efficiency. Gut Microbes 10, 115–132. doi: 10.1080/19490976.2018.1505176
McMurdie, P. J., and Holmes, S. (2013). phyloseq: an R package for reproducible interactive analysis and graphics of microbiome census data. PLoS One 8:e61217. doi: 10.1371/journal.pone.0061217
Minas, K., McEwan, N. R., Newbold, C. J., and Scott, K. P. (2011). Optimization of a high-throughput CTAB-based protocol for the extraction of qPCR-grade DNA from rumen fluid, plant and bacterial pure cultures. FEMS Microbiol. Lett. 325, 162–169. doi: 10.1111/j.1574-6968.2011.02424.x
Nayfach, S., Shi, Z. J., Seshadri, R., Pollard, K. S., and Kyrpides, N. C. (2019). New insights from uncultivated genomes of the global human gut microbiome. Nature 568, 505–510. doi: 10.1038/s41586-019-1058-x
Oenema, O., and Tamminga, S. (2005). Nitrogen in global animal production and management options for improving nitrogen use efficiency. Sci. China C Life Sci. 2, 871–887. doi: 10.1007/BF03187126
Opdahl, L. J., Gonda, M. G., and St-Pierre, B. (2018). Identification of uncultured bacterial species from firmicutes, bacteroidetes and CANDIDATUS saccharibacteria as candidate cellulose utilizers from the rumen of beef cows. Microorganisms 6:17. doi: 10.3390/microorganisms6010017
Reid, M., O’Donovan, M., Elliott, C. T., Bailey, J. S., Watson, C. J., Lalor, S. T., et al. (2015). The effect of dietary crude protein and phosphorus on grass-fed dairy cow production, nutrient status, and milk heat stability. J. Dairy Sci. 8, 517–531. doi: 10.3168/jds.2014-8437
Rognes, T., Flouri, T., Nichols, B., Quince, C., and Mahé, F. (2016). VSEARCH: a versatile open source tool for metagenomics. Peer J. 4:e2584. doi: 10.7717/peerj.2584
Rogosa, M. (1969). Acidaminococcus gen. n., Acidaminococcus fermentans sp. n., anaerobic gram-negative diplococci using amino acids as the sole energy source for growth. J. Bacteriol. 98, 56–66. doi: 10.1128/jb.98.2.756-766.1969
Segata, N., Izard, J., Waldron, L., Gevers, D., Miropolsky, L., Garrett, W. S., et al. (2011). Metagenomic biomarker discovery and explanation. Genome Biol. 12:R60. doi: 10.1186/gb-2011-12-6-r60
Shabat, S. K., Sasson, G., Doron-Faigenboim, A., Durman, T., Yaacoby, S., and Berg Miller, M. E. (2016). Specific microbiome-dependent mechanisms underlie the energy harvest efficiency of ruminants. ISME J. 10, 2958–2972. doi: 10.1038/ismej.2016.62
Wallace, R. J., Rooke, J. A., McKain, N., Duthie, C. A., Hyslop, J. J., Ross, D. W., et al. (2015). The rumen microbial metagenome associated with high methane production in cattle. BMC Genomics 16:839. doi: 10.1186/s12864-015-2032-0
Wang, B., Ma, M. P., Diao, Q. Y., and Tu, Y. (2019). Saponin-induced shifts in the rumen microbiome and metabolome of young cattle. Front. Microbiol. 10:356. doi: 10.3389/fmicb.2019.00356
Wang, C., Liu, J. X., Yuan, Z. P., Wu, Y. M., Zhai, S. W., and Ye, H. W. (2007). Effect of level of metabolizable protein on milk production and nitrogen utilization in lactating dairy cows. J. Dairy Sci. 90, 2960–2965. doi: 10.3168/jds.2006-129
Wang, Y., Nan, X., Zhao, Y., Jiang, L., Wang, H., Zhang, F., et al. (2021). Dietary supplementation of inulin ameliorates subclinical mastitis via regulation of rumen microbial community and metabolites in dairy cows. Microbiol. Spectr. 9:e0010521. doi: 10.1128/Spectrum.00105-21
Warinner, C., and Tuross, N. (2010). Brief communication: tissue isotopic enrichment associated with growth depression in a pig: implications for archaeology and ecology. Am. J. Phys. Anthropol. 141, 486–493. doi: 10.1002/ajpa.21222
Wu, X. H., Sun, H. Z., Xue, M. Y., Wang, D. M., Guan, L. L., and Liu, J. X. (2018). Serum metabolome profiling revealed potential biomarkers for milk protein yield in dairy cows. J. Proteomics 184, 54–61. doi: 10.1016/j.jprot.2018.06.005
Xue, M. Y., Sun, H. Z., Guan, L. L., and Liu, J. X. (2018). Assessment of rumen microbiota from a large dairy cattle cohort reveals the pan and core bacteriomes contributing to varied phenotypes. Appl. Environ. Microbiol. 84, e00970–18. doi: 10.1128/AEM.00970-18
Xue, M. Y., Sun, H. Z., Wu, X. H., Guan, L. L., and Liu, J. X. (2019). Assessment of rumen bacteria in dairy cows with varied milk protein yield. J. Dairy Sci. 102, 5031–5041. doi: 10.3168/jds.2018-15974
Xue, M. Y., Sun, H. Z., Wu, X. H., Liu, J. X., and Guan, L. L. (2020). Multi-omics reveals that the rumen microbiome and its metabolome together with the host metabolome contribute to individualized dairy cow performance. Microbiome 8:64. doi: 10.1186/s40168-020-00819-8
Keywords: rumen, nitrogen utilization efficiency, bacteriome, dairy cows, milk
Citation: Li M, Zhong H, Li M, Zheng N, Wang J and Zhao S (2022) Contribution of Ruminal Bacteriome to the Individual Variation of Nitrogen Utilization Efficiency of Dairy Cows. Front. Microbiol. 13:815225. doi: 10.3389/fmicb.2022.815225
Received: 15 November 2021; Accepted: 07 February 2022;
Published: 18 March 2022.
Edited by:
Anil Kumar Puniya, National Dairy Research Institute (ICAR), IndiaReviewed by:
Sabine Marie Podmirseg, University of Innsbruck, AustriaFabio S. Lima, University of California, Davis, United States
Copyright © 2022 Li, Zhong, Li, Zheng, Wang and Zhao. This is an open-access article distributed under the terms of the Creative Commons Attribution License (CC BY). The use, distribution or reproduction in other forums is permitted, provided the original author(s) and the copyright owner(s) are credited and that the original publication in this journal is cited, in accordance with accepted academic practice. No use, distribution or reproduction is permitted which does not comply with these terms.
*Correspondence: Jiaqi Wang, jiaqiwang@vip.163.com; Shengguo Zhao, zhaoshengguo1984@163.com