- 1Jilin Provincial Key Laboratory of Animal Resource Conservation and Utilization, Northeast Normal University, Changchun, China
- 2College of Animal Science and Technology, Jilin Agricultural University, Changchun, China
- 3Key Laboratory of Vegetation Ecology, Ministry of Education, Changchun, China
- 4College of Life Sciences, Henan Normal University, Xinxiang, China
Host-associated skin bacteria are essential for resisting pathogen infections and maintaining health. However, we have little understanding of how chiropteran skin microbiota are distributed among bat species and their habitats, or of their putative roles in defending against Pseudogymnoascus destructans in China. In this study, we characterized the skin microbiomes of four bat species at five localities using 16S rRNA gene amplicon sequencing to understand their skin microbial composition, structure, and putative relationship with disease. The alpha- and beta-diversities of skin microbiota differed significantly among the bat species, and the differences were affected by environmental temperature, sampling sites, and host body condition. The chiropteran skin microbial communities were enriched in bacterial taxa that had low relative abundances in the environment. Most of the potential functions of skin microbiota in bat species were associated with metabolism. Focusing on their functions of defense against pathogens, we found that skin microbiota could metabolize a variety of active substances that could be potentially used to fight P. destructans. The skin microbial communities of bats in China are related to the environment and the bat host, and may be involved in the host’s defense against pathogens.
Introduction
Animal skin is the first line of defense against pathogen invasion and carries diverse symbiotic microbiota that affect host development, reproduction, and health (Braendle et al., 2003; Koropatnick et al., 2004; Jani and Briggs, 2018). Meanwhile, the host, environment, and pathogens also affect the development of host-associated skin microbiomes (Bates et al., 2018; Jiménez et al., 2019). Thus, uncovering patterns in the distribution of skin bacterial communities across host species and their environments could help increase our understanding of how host-associated bacteria are distributed, and their potential roles in defending against pathogens.
The host species is generally a valuable predictor of skin microbiome structure (Jiménez et al., 2019; Kruger, 2020). In 38 mammalian species, host order and species had the most significant influences on skin microbial communities (Ross et al., 2018). For closely related species, such as congeners, the microbial communities also differed, but with relatively smaller variation (Muletz-Wolz et al., 2018; Jiménez et al., 2019). Different sympatric host species exhibited different bacterial community composition, even when they shared the same environment (Kueneman et al., 2014; Muletz-Wolz et al., 2018). These studies suggest that the evolutionary history, as well as the biological and chemical traits of hosts, might be predominant influences on the composition of skin-associated bacteria.
Environment is another important factor influencing skin microbial community structure due to continuous skin contact with the external environment (Loudon et al., 2014; Avena et al., 2016). Massive numbers of bacteria exist in the environments, and they provide a reservoir for host skin microbiota and the possibility of bacterial transmission from the environment to the hosts (Muletz et al., 2012; Ross et al., 2019). Hosts can also select some specific bacteria to colonize their skin (Walke et al., 2014; Rebollar et al., 2016). Additionally, environmental conditions, such as temperature and salinity, might affect the community structure of host-associated microorganisms (Lokmer and Mathias Wegner, 2015; Hughey et al., 2017). Therefore, investigations along environmental gradients could clarify how environmental characteristics affect host-associated microbial communities.
An invading pathogen may damage the host skin microbiome (Stecher et al., 2007; Barman et al., 2008). For instance, the deadly fungus Batrachochytrium dendrobatidis disturbed the amphibian skin bacterial community composition and structure in a natural epidemic and in experimental infections (Jani and Briggs, 2014). Previous studies revealed some specific bacterial strains in the skin that inhibited the growth of this pathogen (Woodhams et al., 2015; O’Sullivan et al., 2019). Skin-associated bacteria may produce a variety of active secondary metabolites, including terpenoids, polyketides, alkaloids, and peptides, all of which can protect the host against pathogens (Arasu et al., 2013; Cantrell et al., 2019). However, our understanding of the function of the skin microbial community is still limited, though it is important in host defense against pathogens.
Previous studies have focused on the skin microbiome in animals such as corals, sponges, amphibians, and humans (Rosenberg et al., 2007; Giles et al., 2013; Byrd et al., 2018; Jani and Briggs, 2018). There has been an emphasis on the factors shaping host-associated microbiomes and host defense against pathogens (Krediet et al., 2013; Lemieux-Labonté et al., 2017). However, studies on the skin microbiota of bats are limited but increasing, especially considering the rich diversity and global distribution of chiropterans (Lemieux-Labonté et al., 2016; Winter et al., 2017; Fountain et al., 2019; Grisnik et al., 2020). As we know, over the past decades, a psychrophilic fungus Pseudogymnoascus destructans (Gargas et al., 2009) has invaded chiropteran hair follicles, sebaceous glands, and apocrine glands (Meteyer et al., 2009). It causes white-nose syndrome (WNS) and has killed millions of bats across North America (Lorch et al., 2016). Usually, the P. destructans load and infection prevalence reach maximum levels during late hibernation (Langwig et al., 2015). It has been proposed that some symbiotic skin bacteria can inhibit the growth of P. destructans (Hoyt et al., 2015; Li et al., 2022). Yet, little research on the skin microbiome associated with WNS has been conducted (Avena et al., 2016; Lemieux-Labonté et al., 2017; Ange-Stark et al., 2019; Li et al., 2021; Vanderwolf et al., 2021). In contrast to bats in North America, bats in China have relatively low P. destructans loads and infection prevalence, and do not have WNS disease symptoms and mortality (Hoyt et al., 2016a,b). This suggests that bats are resistant to P. destructans in China. It is possible that bats have developed intrinsic and adaptive immune responses to P. destructans through their long-term coevolution in China. Alternatively, considering that skin microbiota have an important function in defense against pathogens, host-associated bacteria are involved in defending against fungal invasion. One recent study determined the temporal and spatial dynamics of microbe populations and their potential functions on greater horseshoe bats (Rhinolophus ferrumequinum) in China (Li et al., 2021). However, the distribution of host-associated bacteria across different host species and how the presence of pathogens influenced it is still not well-understood.
We investigated the skin microbiome of four widely distributed bat species across five localities in China during late hibernation, when bats typically have the greatest fungal load and prevalence (Hoyt et al., 2020). We attempted to clarify variation in bat skin and environmental microbial community composition and structure, and the putative defense mechanism of skin microbiota against pathogens. We had three objectives: (1) elucidate the effects of environment and host on the skin microbiome; (2) clarify geographic variation in the microbiome using Murina leucogaster as an example; and (3) predict and compare microbiome putative functions, with an emphasis on those related to pathogen defense.
Materials and Methods
Field Sampling
We collected epidermal swabs from four bat species: Mu. leucogaster (n = 46), R. ferrumequinum (n = 59), Myotis petax (n = 17), and Rhinolophus pusillus (n = 9). We also collected corresponding environment samples (n = 35) from five localities: New cave (Jilin Province), Gezi cave (Jilin Province), Di cave (Jilin Province), Temple cave (Liaoning Province), and Water channel (Henan Province). Data was collected at the end of March and the beginning of April in 2018 (Table 1 and Figure 2D). The R. ferrumequinum samples used in this research were previously studied by Li et al. (2021). In order to examine the geographic variation in skin microbiomes, we selected Mu. leucogaster as an example and collected samples from four sites: Gezi cave, Temple cave, Di cave, and New cave.
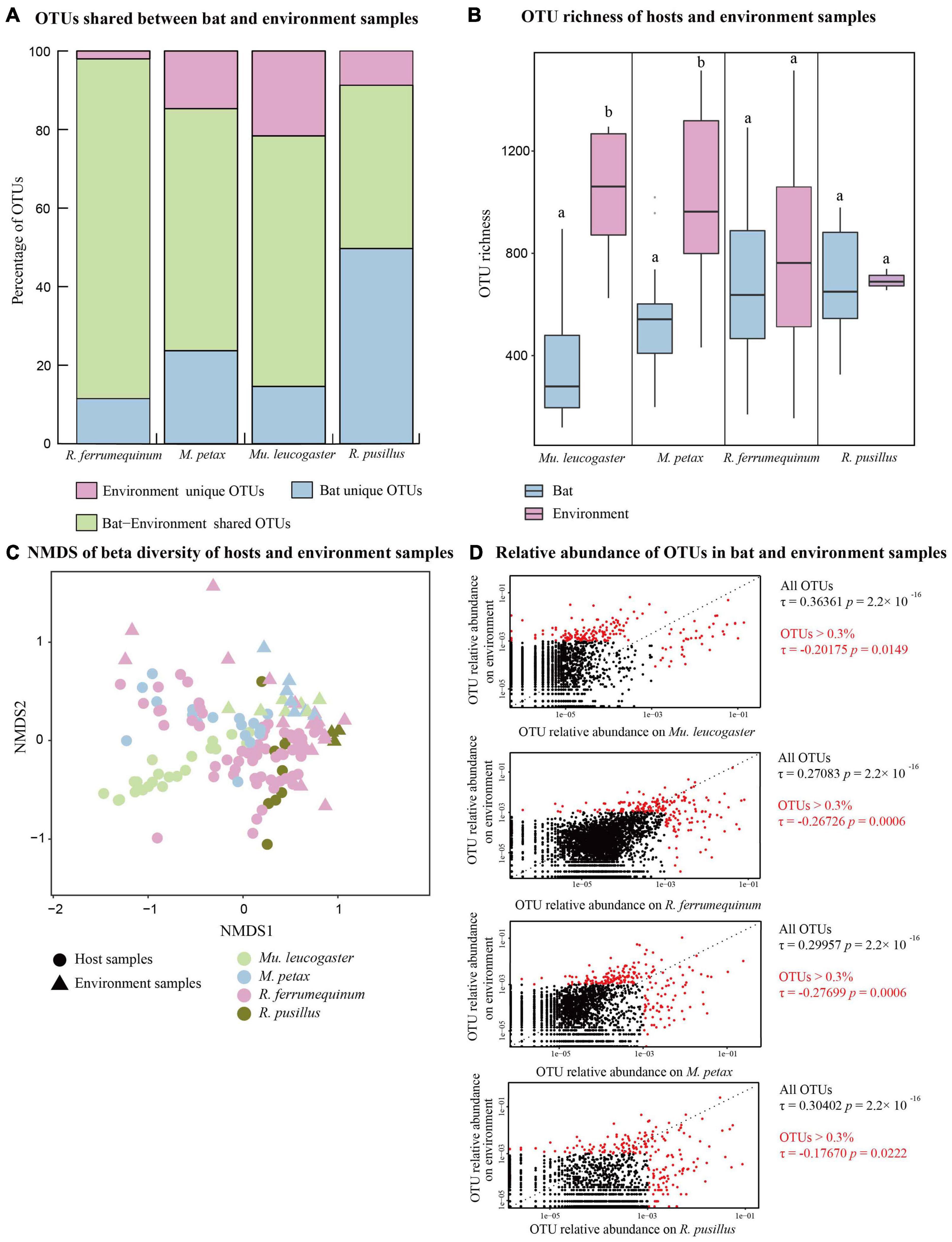
Figure 1. Bat skin and environment bacterial communities of four bat species across five sites. (A) Percentage of shared and unique Operational Taxonomic Units (OTUs) of each bat species and environment samples. (B) OTU richness of hosts and environment samples. Letters represent significant differences among groups. (C) Beta diversities of bat and environment samples. Non-metric multidimensional scaling analysis of Bray Curtis distances. (D) Relative abundances of OTUs on each bat species and its corresponding environment samples. Red dots show OTUs with a total relative abundance >0.3%. Kendall’s ranked correlations, RTau, and P-values are shown to the right of each plot.
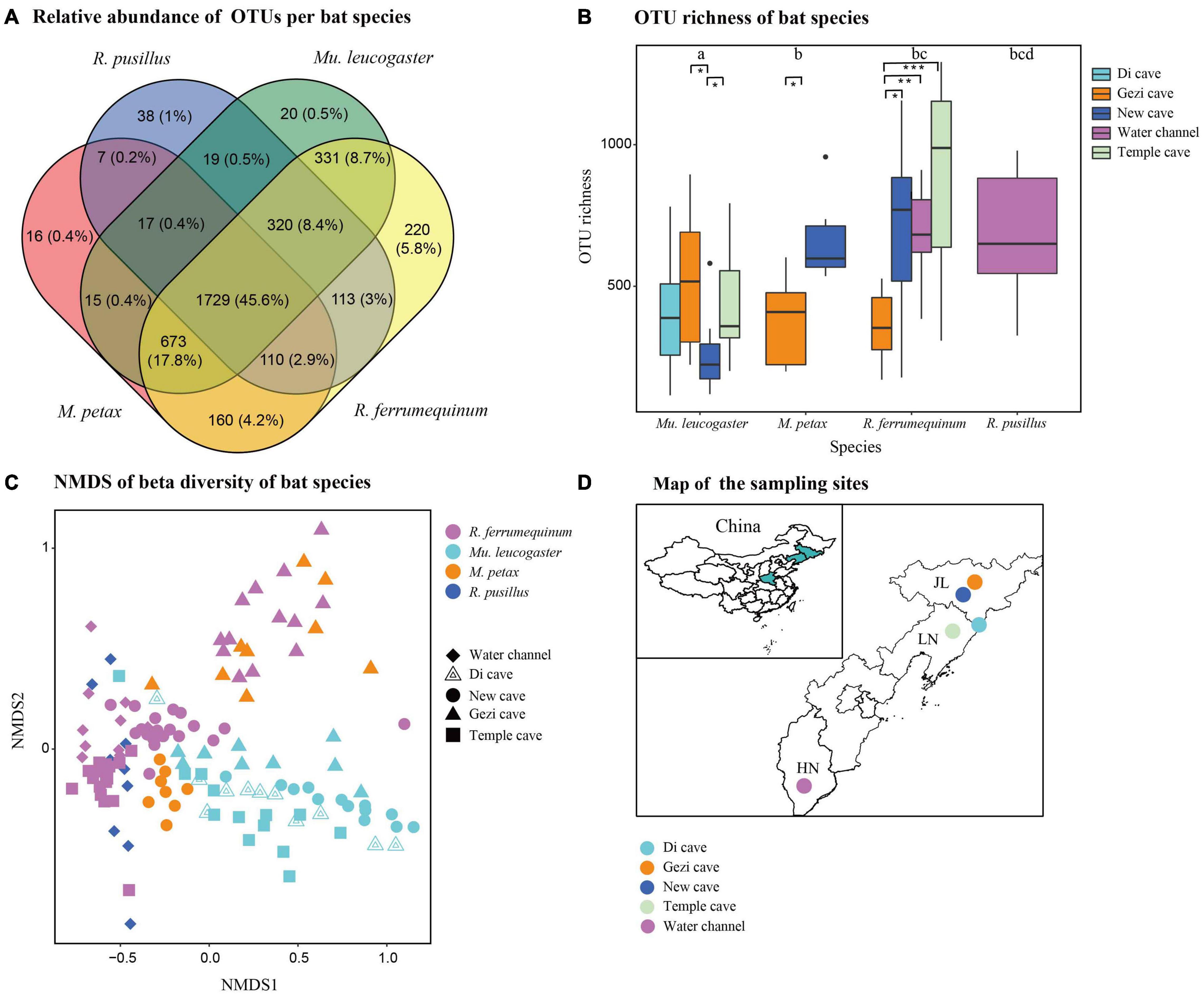
Figure 2. Skin bacterial community structure of four bat species from five sites. (A) Relative abundance of shared and unique Operational Taxonomic Units (OTUs) on each bat species. (B) OTU richness of four bat species. Letters and * represent significant differences among groups. *P < 0.05, **P < 0.01, ***P < 0.001. (C) Beta diversity of four bat species. Non-metric multidimensional scaling analysis of Bray Curtis distances. (D) Map of the sampling sites in China.
We dipped sterile polyester swabs in sterile water and wiped five times along the bats’ forearms and muzzles, as previously described (Langwig et al., 2015), to test for P. destructans. Bat skin bacterial samples were collected with a similar method, wiping the microbes in the bats’ entire dorsal surface of the wing membrane. Environment samples were also obtained by swabbing the cave walls under the bats (∼5 cm) five times using linear strokes. These samples were stored in 500 μl RNAlater (TIANGEN, Beijing, China) tubes at −20°C within 24 h of sampling until returning to the laboratory, where samples were stored in −80°C refrigerator for DNA extraction. We measured bat roosting temperatures using an infrared thermometer (Fluke, 62 MAX IR, Everett, WA, United States). After swabbing, we measured bat forearm length and weight and calculated the body mass index (BMI = weight/forearm length) and identified the sex and species. Bats were released immediately after sampling. Sampling was performed by the same person to limit individual differences in swabbing techniques. All studies were approved by the Laboratory Animal Welfare and Ethics Committee of Jilin Agricultural University.
Pseudogymnoascus destructans Test
Fungal DNA was extracted from swabs using modified DNeasy Blood and Tissue kits (Qiagen, Hilden, Germany). The modified protocol included the use of lyticase during the lysis step (Hoyt et al., 2016a). Each DNA extraction plate contained seven negative controls (blanks) and one positive control derived from a P. destructans ATCC MYA-4855 isolate (Ingala et al., 2017). We used real-time quantitative PCR (qPCR) to examine P. destructans following the method of Muller et al. (2013). All samples were run in duplicate. Serial dilutions of the positive control, from 2 ng/μl to 2 fg/μl, were prepared and analyzed with qPCR to calculate fungal loads (P. destructans load = 10^[(Ct −22.04942)/−3.34789] (Langwig et al., 2015).
Bacterial DNA Extraction, Processing, and Sequencing
Genomic DNA was extracted from swabs using the E.Z.N.A™ Mag-Bind Soil DNA Kit (OMEGA Bio-Tek, Norcross, GA, United States) according to manufacturer protocol. PCR amplification was used on the first pair of primers (universal 341F/805R) to target the V3–V4 region of the 16S rDNA and contained 17 bp barcodes (Lu et al., 2015). The PCR reaction system and conditions have been described (Li et al., 2021). The amplification products were purified with the Agencourt AMPure XP beads (Beckman Coulter, Brea, CA, United States). A Qubit 2.0 DNA Assay Kit (Life Technologies, Carlsbad, CA, United States) was used to check the DNA concentration of each sample. PCR amplicons of all samples were sequenced with paired-end 2 × 300 bp on the Illumina MiSeq platform at Sangon Biotech Co., Ltd. (Shanghai, China).
We amplified 9,355,062 raw sequences from the 131 swabs of bat species and 35 corresponding environment samples. The number of reads in the bat samples was 7,311,640 [average 55,814 reads/sample (range of 36,784–89,591)], and the number of reads in the environment samples was 2,043,422 [average 58,383 reads/sample (range of 35,835–91,204)]. We used Cutadpat to remove adapter sequences from the high-throughput sequencing reads (Martin, 2011). Quantitative Insights Into Microbial Ecology (QIIME v 1.9.1) was used to process the forward and reverse reads based on the 10 bp barcode (Caporaso et al., 2010). High-quality reads met the following parameters: they contained no N character sequences, no errors in barcode sequence, and a minimum of five consecutive base pairs (Q ≥ 20). After quality processing, a total of 6,705,497 (average 51,187 reads/sample) and 1,940,539 reads (average 55,443 reads/sample) were obtained from bat and environment samples, respectively. Sequences were processed using VSEARCH v. 7.0.1234 (Edgar, 2010) and then clustered at the ≥97% similarity threshold to generate an Operational Taxonomic Unit (OTU) database, with singletons and chimeric sequences excluded during this process. Taxonomy was assigned using the RDP classifier and Greengenes database (DeSantis et al., 2006; Cole et al., 2007). The OTU table was filtered using a minimum cluster size of 0.001% of the total reads to improve accuracy (Bokulich et al., 2013). The OTUs classified as mitochondrial and chloroplastic were removed. Finally, the OTU table was rarefied according to the sample with the lowest number of reads. The final rarefied OTU table, including bat and environment samples, had 3,818 OTUs.
Data Analysis
We clarified the relationship between host skin microbiota and corresponding environment microbiota using the content of shared and unique OTUs. The alpha diversity (OTU richness and Shannon diversity) of skin microbial diversity of host and environment samples was calculated. T-tests and Wilcoxon tests were used to compare alpha diversities between host species and their environment samples. Beta diversity was calculated with Bray Curtis distance matrices and plotted using non-metric multidimensional scaling (NMDS) in k = 2 dimensions using the ordinate() and plot_ordination() functions in the R package phyloseq (McMurdie and Holmes, 2013). Permutational multivariate analysis of variance (PERMANOVA) was used to test the significance of beta diversity between bat and environment samples using the adonis() function in the vegan package (Oksanen et al., 2015). Kendal’s tau ranked correlations of OTU relative abundance were calculated between host species and their environment samples (Fitzpatrick and Allison, 2014). We used all OTUs and OTUs with a relative abundance greater than 0.3% to calculate the correlations of host and environment samples.
We determined whether the skin microbiota differed among the individual host species from multiple sites. We used a Venn diagram to represent the relative abundance of shared and unique OTUs among the host species. For alpha diversity, OTU richness and Shannon diversity were calculated, and Kruskal-Wallis tests were used to determine differences across host species. We used generalized linear models (GLM) with a quasi-Poisson distribution to examine variation depending on factors such as sites, BMI, temperature, sex, P. destructans infection status, and P. destructans load in OTU richness and Shannon diversity. The calculation and visualization of beta diversity among host species and sites was as described above. To assess the influence of each explanatory variable (BMI, temperature, sex, P. destructans infection status and P. destructans load) on host species microbiota composition, we used partial distance-based redundancy analysis (db-RDA) while controlling for the host and site variables. Adjusted R-squared (R2) values were calculated to clarify the explanatory variables (Starcher, 1942). Significance of partial db-RDA was tested via 9,999 permutations with the anova.cca() function of the vegan package. The unweighted pair group method with arithmetic mean (UPGMA) was used on Bray Curtis distances of mean >0.1% for OTU relative abundances at the genus level to determine clustering patterns across host species. We performed generalized linear mixed models (GLMMs) to assess OTUs that were differentially abundant between species in the glmmTMB package using a beta distribution and a logit link (Brooks et al., 2017). In this model, the OTU and species were set as fixed effects, and site was included as a random effect.
Functional prediction was conducted using Phylogenetic Investigation of Communities by Reconstruction of Unobserved States2 (PICRUSt2; Douglas et al., 2020) from the 16S rRNA gene sequences (Langille et al., 2013; Beaumont et al., 2021). The predicted functional gene abundance was based on the OTU table. The obtained prediction results can be used to classify gene families by KEGG Orthology (Wei et al., 2020). The linear discriminant analysis (LDA) size effect (LEfSe) was carried out to determine the significant differences in KEGG pathways across bat species (Segata et al., 2011), with an LDA score >2.0 considered as a cut-off (Clemente et al., 2015). We explored gene relative abundance differences among species from two functional classes associated with bacterial defense mechanisms: metabolism of terpenoids and polyketides (MTP) and biosynthesis of secondary metabolites (BSM) (Rebollar et al., 2018). Kruskal-Wallis tests and ANOVA were used to analyze the significant differences of gene relative abundance among the bat species.
To evaluate the geographic variation in bacterial community structure in Mu. leucogaster, we compared alpha- (OTU richness and Shannon diversity) and beta-diversities across four sites. We used indicator Value tests (IndVal) (Dufrêne and Legendre, 1997) to identify the most representative OTUs at genus levels with a relative abundance >1% on Mu. leucogaster across sites. The IndVal values were computed in the package indicspecies accessed by the multipatt() function (De Cáceres and Legendre, 2009), and significance was assessed with 9,999 permutations. OTUs with IndVal values ≥0.4 were considered to be indicators (Lemieux-Labonté et al., 2017). Kendal’s tau ranked correlations of OTU relative abundance >0.1% were calculated on Mu. leucogaster across sites. We used LEfSe analysis to evaluate the significant differences in KEGG pathways on Mu. leucogaster across sites. Kruskal-Wallis tests and ANOVA were used to detect gene relative abundances of BSM and MTP in Mu. leucogaster. All statistical analyses were performed in R version 4.0.5 (R Core Team, 2019).
Results
Pseudogymnoascus destructans Infection
The fungal loads were significantly different among the four bat species (Kruskal-Wallis test, Chi-squared = 30.245, P < 0.001). Myotis petax had the highest P. destructans infection intensity (average log-transformed P. destructans loads: −2.62), followed by R. ferrumequinum (−4.20), Mu. leucogaster (−4.24), and R. pusillus (−6.11) (Supplementary Figure 1A). The highest P. destructans prevalence was on M. petax (Supplementary Figure 1B). Among different localities for Mu. leucogaster, P. destructans loads were the lowest in Temple cave (−5.28) (ANOVA, F = 12.6, P < 0.001), and the highest P. destructans prevalence was in Gezi cave (−3.69) (Supplementary Figure 1B).
Microbial Communities Between Host and Environment
The majority of the OTUs on bat skin were also present in the environment, with the exception of R. pusillus (Figure 1A). The OTU richness was significantly lower than the environment samples in M. petax and Mu. leucogaster (M. petax: t = −2.99, P = 0.018; Mu. leucogaster: w = 5, P = 0.003), but similar in R. ferrumequinum (t = −0.84, P = 0.406) and R. pusillus (t = −0.14, P = 0.887) (Figure 1B). The Shannon diversity was also significantly lower than the environment samples in M. petax and Mu. leucogaster (M. petax: t = −3.11, P = 0.014; Mu. leucogaster: w = 0, P < 0.001), but similar in R. ferrumequinum (w = 432, P = 0.236) and R. pusillus (w = 22, P = 0.145) (Supplementary Figure 2A). All hosts combined, or each host separately, had significantly different microbial structures in comparison with corresponding environment samples (all hosts: NMDS with stress = 0.18; PERMANOVA, Pseudo-F1,141 = 13.19, P = 0.001, R2 = 0.173; R. ferrumequinum: PERMANOVA, Pseudo-F1,76 = 7.07, P = 0.001, R2 = 0.086; M. petax: PERMANOVA, Pseudo-F1,23 = 2.33, P = 0.007, R2 = 0.096; Mu. leucogaster: PERMANOVA, Pseudo-F1,28 = 11.11, P = 0.001, R2 = 0.292; R. pusillus: PERMANOVA, Pseudo-F1,11 = 3.36, P = 0.008, R2 = 0.251, Figure 1C).
Each bat species was positively correlated with the relative abundances of OTUs in its corresponding environment samples (Figure 1D), with Mu. leucogaster having the highest correlation (Mu. leucogaster: Rτ = 0.364, P < 0.001; R. ferrumequinum: Rτ = 0.271, P < 0.001; M. petax: Rτ = 0.300, P < 0.001; R. pusillus: Rτ = 0.304, P < 0.001). When we calculated ranked correlations with the most relatively abundant OTUs (OTUs > 0.3%), all of the correlations between bat and environment samples became significantly negative (Figure 1D; Mu. leucogaster: Rτ = −0.202, P = 0.014; R. ferrumequinum: Rτ = −0.267, P = 0.001; M. petax: Rτ = −0.277, P = 0.001; R. pusillus: Rτ = −0.177, P = 0.022) (Figure 1D).
Structure and Function of Skin Bacterial Communities Across Hosts
Host species shared the majority of the relative abundances of OTUs on skin bacterial communities in all four species (45.6%). Few OTUs unique to individual host species were found (ranging from 0.4% for M. petax to 5.8% for R. ferrumequinum) (Figure 2A). OTU richness was significantly different from host species at all sites (Kruskal-Wallis: Chi-squared = 29.54, P < 0.01; Figure 2B). In the GLM analysis, we found that sites (Chi-squared = 17.52, P = 0.001) and BMI (Chi-squared = 5.59, P = 0.018) were the significant factors associated with changes in OTU richness. Temperature, sex, P. destructans infection status, and fungal load did not significantly affect alpha diversity measures (all P > 0.05). Shannon diversity was also significantly different from host species across all sites (Kruskal-Wallis: Chi-squared = 30.14, P < 0.01; Supplementary Figure 2B). In the GLM analysis, we found that sites (Chi-squared = 22.6, P = 0.001) and BMI (Chi-squared = 5.37, P = 0.02) were the significant factors associated with changes in Shannon diversity.
Beta diversity analysis indicated that skin bacterial communities significantly differed among the four bat species (NMDS with stress = 0.17; Bray-Curtis, PERMANOVA, Pseudo-F3,130 = 3.41, P = 0.001, R2 = 0.074; Figure 2C). Site was also a significant predictor of skin bacterial communities on bat species (Bray-Curtis, PERMANOVA, Pseudo-F3,130 = 4.11, P = 0.001, R2 = 0.115). The partial db-RDA analysis showed that bat roosting temperature was significantly correlated with microbial composition changes (Bray-Curtis, PERMANOVA, Pseudo-F3,62 = 2.41, P = 0.002, R2 = 0.011) when we controlled for the host species and sites. Likewise, P. destructans infection status, fungal load, BMI, and sex did not significantly affect the beta diversity measures (all P > 0.05). All 21 OTUs were differentially abundant among the bat species (GLMMs, P < 0.05, Table 2). M. petax had communities dominated by the genera Citrobacter and families Micrococcaceae and Pasteurellaceae. R. ferrumequinum was dominated by OTUs from the genera Brackiella, Corynebacterium, and Staphylococcus. However, R. pusillus had relatively fewer dominant OTUs from the genera Mycobacterium and Kaistobacter. Mu. leucogaster had a rich complement of dominant OTUs, such as Pseudomonas, Sphingobacterium, and Flavobacterium (Table 2).
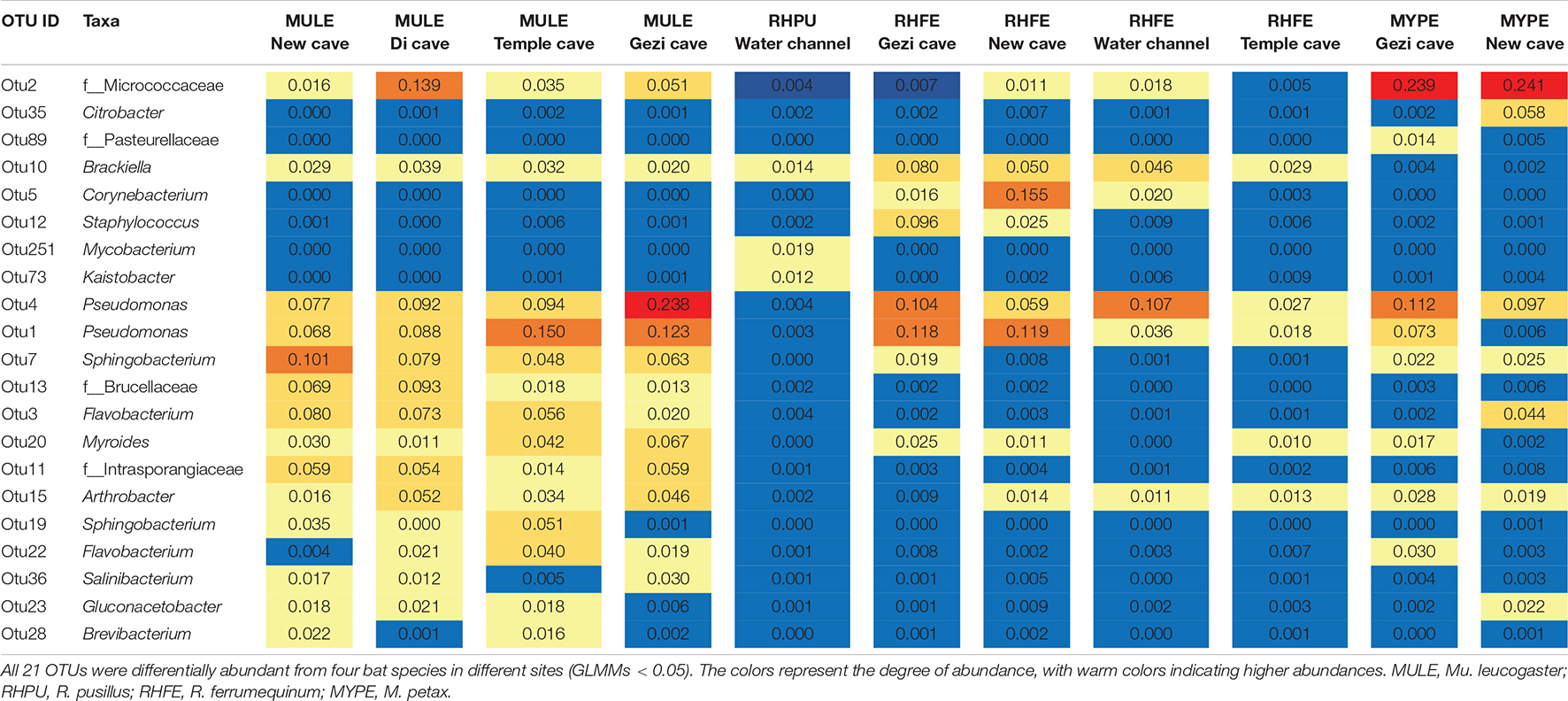
Table 2. Heatmap of the average relative abundance (>1%) of bacterial Operational Taxonomic Units (OTUs) that were differentially abundant among bat species sampled from different localities.
We detected significant differences (LEfSe analysis: LDA score > 2, P < 0.05) in gene relative abundances from KEGG Orthology groups (KOs) on chiropteran skin microbiomes using PICRUSt2 (Figure 3A). Most of the KOs were related to metabolism, followed by genetic information processing, organic systems, and environmental information processing (Supplementary Table 1). For two bacterial defense mechanisms, metabolism of terpenoids and polyketides (MTP) and biosynthesis of secondary metabolites (BSM), 19 out of 21 pathways and 18 out of the 30 pathways described in KEGG were present in bat skin microbiomes, respectively. For those pathways with gene relative abundances >0.1%, different bat species enriched similar pathways but most of them had significant variation across hosts (Figures 3B,C). We analyzed phenazine biosynthesis because of the presence of anti-P. destructans active substances in this pathway (Li et al., 2022); however, no significant variation in gene abundance was found among the bat species (Supplementary Table 2).
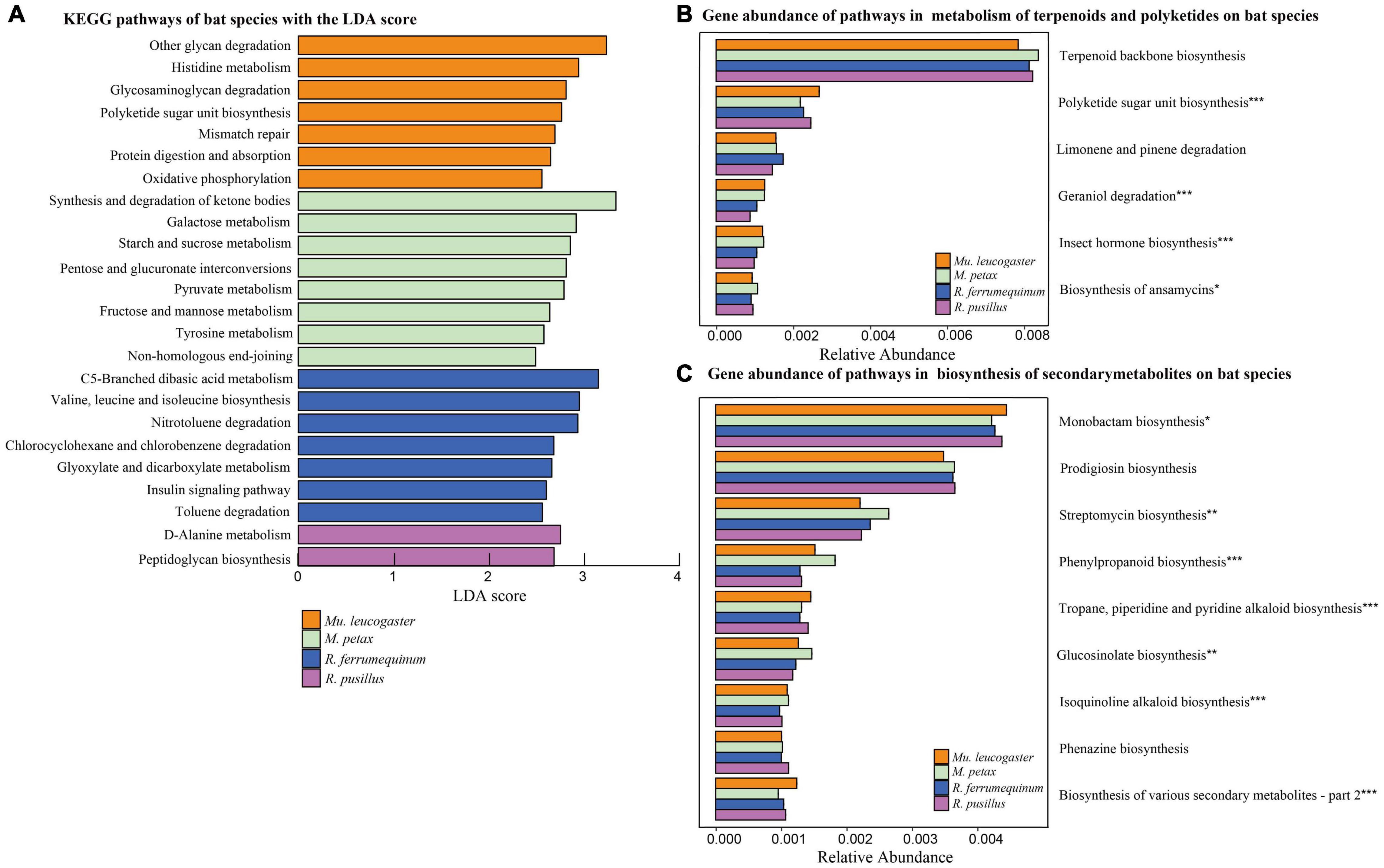
Figure 3. Functional gene predictions. (A) KEGG pathways of bat species based on LEfSe analysis. KEGG pathways with the highest linear discriminant analysis (LDA) scores. Gene abundance of pathways within the metabolism of terpenoids and polyketides (B) and biosynthesis of secondary metabolites (C) on bat species. The gene relative abundances of pathways >0.1%. Asterisks indicate significant difference among groups. *P < 0.05, **P < 0.01, ***P < 0.001.
Skin Bacterial Community and Function Differences of Murina leucogaster
Operational Taxonomic Unit richness of Mu. leucogaster was significantly different across sites (Kruskal-Wallis: Chi-squared = 8.17, P = 0.01; Figure 2B), while no single factor (temperature, BMI, sex, P. destructans infection status or load) was found to significantly affect it (GLM: P > 0.05). Shannon diversity of Mu. leucogaster was not significantly different across sites (Kruskal-Wallis: Chi-squared = 1.04, P = 0.79; Supplementary Figure 2B). Beta diversity of Mu. leucogaster skin communities were also consistently different across sites (NMDS with stress = 0.15; Bray-Curtis, PERMANOVA, Pseudo-F3,45 = 5.50; P = 0.001, R2 = 0.282; Figure 2C), but no factor significantly influenced the bacterial community structure (partial db-RDA analysis, all P > 0.05). The mean relative abundance of bacterial taxa on Mu. leucogaster differed across sites (Figure 4A). Pseudomonas was the dominant genus in Gezi cave (36.1%), Temple cave (26.9%), and Di cave (19.4%). However, the dominant genus in New cave was Myroides (19.3%). Multiple indicator taxa were detected at different locations, such as Salinibacterium, Rhodococcus, and Pseudomonas at Gezi cave (Figure 4B). The P. destructans loads and relative abundance (>0.1%) of many genera on Mu. leucogaster across sites showed a negative correlation, but the results were not statistically significant (data not shown).
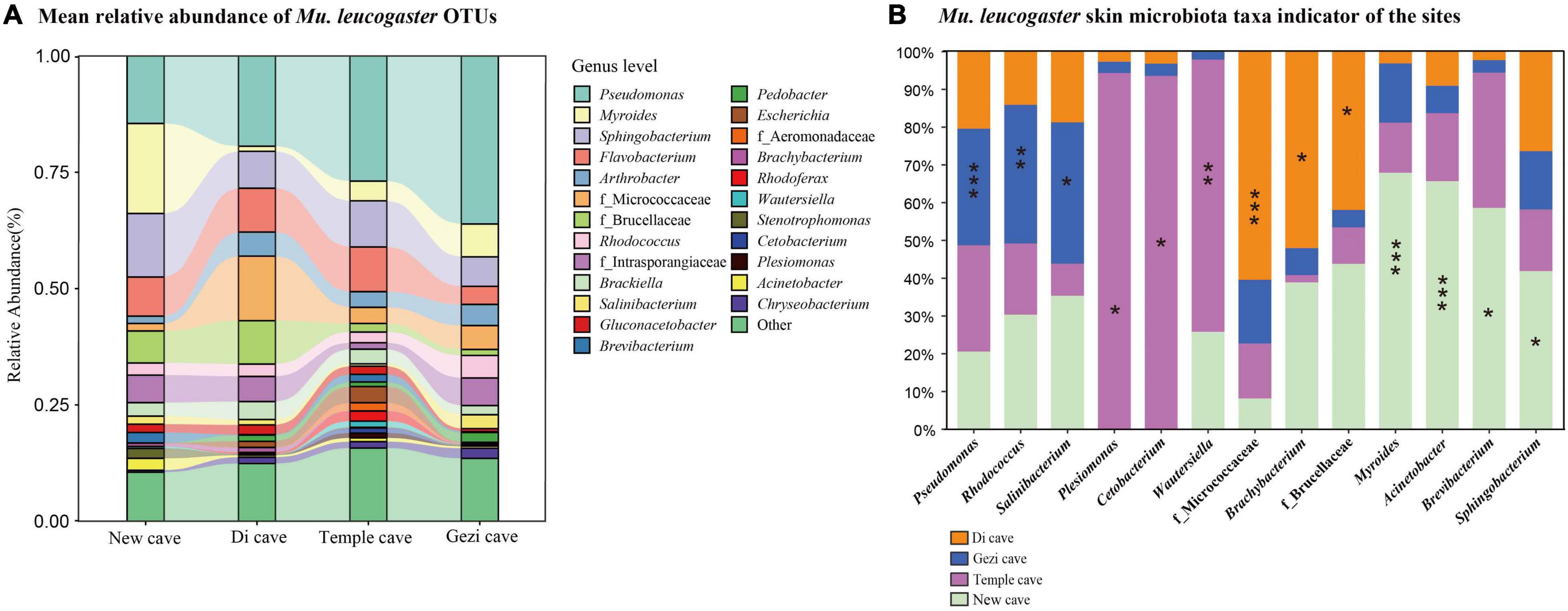
Figure 4. Skin bacterial community composition of Mu. leucogaster. (A) Alluvial diagram of mean relative abundances of bacterial taxa (genus level) of Mu. leucogaster across sites. The relative abundances of bacterial genera > 1%. (B) Mu. leucogaster skin microbiota taxa indicator of the sites. Significant indicators were found among the 13 most abundant taxa representing more than 1% of total abundance with IndVal analysis. Stars indicate significant representative taxa. *P < 0.05, **P < 0.01, ***P < 0.001, IndVal ≥ 0.4.
LEfSe analysis revealed significant differences in the gene relative abundances from KOs across sites in Mu. leucogaster (LDA score > 2, P < 0.05), and most KOs were associated with metabolism (Supplementary Figure 3A). In MTP and BSM, except for isoquinoline alkaloid biosynthesis, the gene abundances (>0.1%) of all other pathways were significantly different on Mu. leucogaster (Supplementary Figure 4A).
Discussion
In this study, P. destructans loads differed among bat species, and M. petax had the highest fungal loads. Typically, Myotis were the most commonly infected species with P.
destructans in Europe (Puechmaille et al., 2011) and North America (Turner et al., 2011). Myotis petax typically roosted in cave entrances with their bodies in close contact with the cave wall, similar to the roosting style of M. lucifugus, increasing the chance of P. destructans infection (Turner et al., 2011). The high fungal loads of M. petax were also possibly associated with the immune capacity of the bats. Furthermore, significant differences in fungal loads were detected in Mu. leucogaster across different sites, presumably due to the different caves, providing different microclimate for P. destructans survival.
The environment affects the community structure and composition of skin microbiota (Manus et al., 2017; Muletz-Wolz et al., 2018). We found that the skin bacterial communities of bat species differed from the corresponding environments in alpha- and beta-diversities (Figures 1B,C), even if they shared the majority of OTUs (Figure 1A). These results are consistent with other studies on bats (Avena et al., 2016; Grisnik et al., 2020), indicating that different bat species share the most bacterial taxa with their corresponding environmental samples at the class level (Avena et al., 2016). In particular, the high abundance of OTUs in bat skin had a negative correlation with corresponding environmental OTUs (Figure 1D), which was also found in Panamanian frogs and R. ferrumuequinum (Rebollar et al., 2016; Li et al., 2021). This result suggested that the presence of unique ecological niche-bacterial taxa in skin favored specific growth. However, the cave wall under the bat could not fully reflect the real environmental conditions, so additional environmental samples such as soil and rock should be considered in future studies. In fact, the skin microbial assemblages do not fully reflect the microbiota of the environment, which can be significantly influenced by multiple factors (Ross et al., 2019). For instance, the host may change its skin microbiota for defense against pathogen invasion, even though it is constantly exposed to the external environment (Rosenthal et al., 2011).
Host species is also one of the important factors shaping the skin microbiome (Muletz-Wolz et al., 2018). In this study, skin microbiota varied across bat species, but the different bat species shared the majority of OTUs. This suggested a similar classification of their skin microbiota, which was consistent with results on neotropical bats (Lemieux-Labonté et al., 2016), but the community diversity was different among the bat species (Avena et al., 2016; Lemieux-Labonté et al., 2016). Significant microbial differences in alpha diversity (Figure 2B and Supplementary Figure 2B) and community structure (Figure 2C) were found among the bat species, driven by BMI and temperature. Host body condition makes a significant contribution to skin alpha-diversity in Eleutherodactylus coqui (Longo and Zamudio, 2017), and the relative abundance of Corynebacterium in human skin microbiota is significantly correlated with BMI (Brandwein et al., 2019). Temperature may indirectly affect microbial community structure by causing changes in host physiology (such as immune function) (Hooper et al., 2012). For humpback whales, variations in the average relative abundance of four core genera of skin microbiome were closely associated with decreasing water temperature (Bierlich et al., 2018). Meanwhile, microorganisms also need suitable temperatures to grow and reproduce. Therefore, temperature becomes a filter of the environment, allowing bacteria with specific characteristics to grow within a certain temperature range, while also potentially increasing the growth of pathogens (Langwig et al., 2015, 2016).
In addition to the variation in skin microbial communities across species, Mu. leucogaster had significant geographic differences in OTU richness and community structure (Figures 2B,C). However, the alpha- and beta-diversities were not affected by BMI, temperature, sex, P. destructans infection status, and fungal load, implying that sampling position had an important influence on the skin microbiome (Avena et al., 2016). Those differences could be associated with the geomorphology of the caves, such as different space, population size, humidity and temperature. In studies on M. lucifugus, local environment was an important factor explaining the pattern of skin microbial communities (Lemieux-Labonté et al., 2017). During hibernation, bats roost on the cave walls for long periods of time, which provides a change for host skin to exchange bacteria with the environment and may select for bacteria with low abundance in the environment (Li et al., 2021). Host genetic factors and other host-intrinsic characteristics also could directly influence the composition of human skin microbiota (Si et al., 2015). Thus, it is also possible that genetic differentiation among bat populations may explain differences in bacterial communities. Further calculation of genetic diversity is needed to elucidate the specific differences.
To better assess bat skin microbiota-pathogen interactions, we analyzed putative functions of the chiropteran microbiome. Many genes were related to metabolic pathways in chiropteran skin microbiota (Figure 3A). Microbial metabolism is a fundamental process of life activity. The metabolism of amino acids and carbohydrates plays an important role in the maintenance of physiological homeostasis and disease defense in hosts (Fuhrer et al., 2010; Thomas et al., 2012). We focused on analyses of the metabolic pathways associated with defense mechanisms, including MTP and BSM (Figures 3B,C). Similar functional classifications of the skin microbiota were found across bat species and sites, suggesting the existence of consistent defense mechanisms.
The MTP class includes degradation pathways such as limonene, pinene, geraniol, and citronellol. Pseudomonas and Rhodococcus strains have become model organisms for these pathways (Marmulla and Harder, 2014). These two genera inhibit the growth of several conservation relevant fungal diseases, including snake fungal disease, chytridiomycosis, and WNS (Hoyt et al., 2015; Woodhams et al., 2015; Cornelison et al., 2016; Lemieux-Labonté et al., 2017). After Eptesicus fuscus is inoculated with P. destructans, Pseudomonas, and Rhodococcus are still the dominant genera in the skin microbiota (Lemieux-Labonté et al., 2020). In this study, Pseudomonas had a high abundance in all species except R. pusillus (Table 2). Similarly, the skin microbiota of Mu. leucogaster in Gezi cave were enriched with a large number of Pseudomonas, Rhodococcus, and Salinibacterium as indicator taxa (Figures 4A,B). The P. destructans load and prevalence of Mu. leucogaster in Gezi cave were the highest (Supplementary Figures 1A,B), and the OTU richness was also the highest, suggesting the production of more antifungal metabolites (degradation pathways) or more effective competition with the pathogen for resources (Longo et al., 2015). The Pseudomonas isolated from R. ferrumequinum and M. petax in Gezi cave in vitro efficiently inhibited the growth of P. destructans (Li et al., 2022). The BSM class pathways may be involved in bacterial competition within the community and in protecting the host against pathogens (Rebollar et al., 2016). The highest gene relative abundance of pathways in BSM, across bat species and sites, was involved in monobactam biosynthesis, followed by prodigiosin biosynthesis (Figure 3C and Supplementary Figure 4A). Many novel monobactam derivatives have been evaluated for their antibacterial activities against Gram-negative pathogenic strains, including Aztreonam and BAL30072 compounds (Fu et al., 2016). Serratia produces prodigiosin that significantly inhibits the growth of B. dendrobatidis and B. salamandrivorans (Woodhams et al., 2018). The phenazine-1-carboxylic acid produced by P. yamanorum GZD14026 isolated from M. petax in Gezi cave effectively inhibited P. destructans (Li et al., 2022). These metabolic pathways could be involved in protecting the host against P. destructans, leading to asymptomatic bats with a low infection burden.
Our study results demonstrated strong variation of skin bacterial community structure and composition across bat species and sampling sites. The BMI of bats and sampling sites could drive variation in microbial diversity, while environmental temperature and location significantly influenced community structure. However, host skin microbial communities significantly differed from those of the surrounding environments. Among Mu. leucogaster populations, strong geographic variation in community structure was found. The putative functions of the bat skin microbiome suggested that secondary metabolite pathways—the MTP and BSM classes—provide potential defense against pathogens and could play a role in protecting bats from the invasion of P. destructans. However, the functions of chiropteran skin symbiotic bacteria need further study to fully understand the interactions between microbes, hosts, and pathogens.
Data Availability Statement
The datasets presented in this study can be found in online repositories. The names of the repository/repositories and accession number(s) can be found in the article/Supplementary Material.
Ethics Statement
The animal study was reviewed and approved by the Laboratory Animal Welfare and Ethics Committee of Jilin Agricultural University.
Author Contributions
ZL performed data analysis and drafted the manuscript. ZL, AL, WD, HL, SL, and LJ collected the samples. KS and JF developed the study concept, design, and supervised the project. AL and KS reviewed and revised the manuscript. All authors contributed to the article and approved the submitted version.
Funding
This work was supported by the National Natural Science Foundation of China (Grant Nos. 32171525, 31961123001, and 31870354).
Conflict of Interest
The authors declare that the research was conducted in the absence of any commercial or financial relationships that could be construed as a potential conflict of interest.
Publisher’s Note
All claims expressed in this article are solely those of the authors and do not necessarily represent those of their affiliated organizations, or those of the publisher, the editors and the reviewers. Any product that may be evaluated in this article, or claim that may be made by its manufacturer, is not guaranteed or endorsed by the publisher.
Acknowledgments
We are grateful to Joseph R. Hoyt for his help in sampling in the field.
Supplementary Material
The Supplementary Material for this article can be found online at: https://www.frontiersin.org/articles/10.3389/fmicb.2022.808788/full#supplementary-material
References
Ange-Stark, M., Cheng, T. L., Hoyt, J. R., Langwig, K. E., Parise, K. L., Frick, W. F., et al. (2019). White-nose syndrome restructures bat skin microbiomes. bioRxiv doi: 10.1101/614842
Arasu, M. V., Al-Dhabi, N. A., Saritha, V., Duraipandiyan, V., Muthukumar, C., and Kim, S. J. (2013). Antifeedant, larvicidal and growth inhibitory bioactivities of novel polyketide metabolite isolated from Streptomyces sp. AP-123 against Helicoverpa armigera and Spodoptera litura. BMC Microbiol. 13:105. doi: 10.1186/1471-2180-13-105
Avena, C. V., Parfrey, L. W., Leff, J. W., Archer, H. M., Frick, W. F., Langwig, K. E., et al. (2016). Deconstructing the bat skin microbiome: influences of the host and the environment. Front. Microbiol. 7:1753. doi: 10.3389/fmicb.2016.01753
Barman, M., Unold, D., Shifley, K., Amir, E., Hung, K., Bos, N., et al. (2008). Enteric salmonellosis disrupts the microbial ecology of the murine gastrointestinal tract. Infect. Immun. 76, 907–915. doi: 10.1128/IAI.01432-07
Bates, K. A., Clare, F. C., O’Hanlon, S., Bosch, J., Brookes, L., Hopkins, K., et al. (2018). Amphibian chytridiomycosis outbreak dynamics are linked with host skin bacterial community structure. Nat. Commun. 9:693. doi: 10.1038/s41467-018-02967-w
Beaumont, M., Cauquil, L., Bertide, A., Ahn, I., Barilly, C., Gil, L., et al. (2021). Gut microbiota-derived metabolite signature in suckling and weaned piglets. J. Proteome. Res. 20, 982–994. doi: 10.1021/acs.jproteome.0c00745
Bierlich, K. C., Miller, C., DeForce, E., Friedlaender, A. S., Johnston, D. W., and Apprill, A. (2018). Temporal and regional variability in the skin microbiome of humpback whales along the Western Antarctic Peninsula. Appl. Environ. Microbiol. 84, e2574–e2517. doi: 10.1128/AEM.02574-17
Bokulich, N. A., Subramanian, S., Faith, J. J., Gevers, D., Gordon, J. I., Knight, R., et al. (2013). Quality-filtering vastly improves diversity estimates from Illumina amplicon sequencing. Nat. Methods. 10, 57–59. doi: 10.1038/nmeth.2276
Braendle, C., Miura, T., Bickel, R., Shingleton, A. W., Kambhampati, S., and Stern, D. L. (2003). Developmental origin and evolution of bacteriocytes in the aphid-Buchnera symbiosis. PLoS Biol. 1:E21. doi: 10.1371/journal.pbio.0000021
Brandwein, M., Katz, I., Katz, A., and Kohen, R. (2019). Beyond the gut: skin microbiome compositional changes are associated with BMI. Hum. Microbiome. J. 13, 100063. doi: 10.1016/j.humic.2019.100063
Brooks, M. E., Kristensen, K., van Benthem, K. J., Magnusson, A., Berg, C. W., Nielsen, A., et al. (2017). Modeling zero-inflated count data with glmmTMB. bioRxiv 2017:132753. doi: 10.1101/132753
Byrd, A. L., Belkaid, Y., and Segre, J. A. (2018). The human skin microbiome. Nat. Rev. Microbiol. 16, 143–155. doi: 10.1038/nrmicro.2017.15
Cantrell, T. P., Freeman, C. J., Paul, V. J., Agarwal, V., and Garg, N. (2019). Mass spectrometry-based integration and expansion of the chemical diversity harbored within a marine sponge. J. Am. Soc. Mass. Spectrom. 30, 1373–1384. doi: 10.1007/s13361-019-02207-5
Caporaso, J. G., Kuczynski, J., Stombaugh, J., Bittinger, K., Bushman, F. D., Costello, E. K., et al. (2010). QIIME allows analysis of high-throughput community sequencing data. Nat. Methods. 7, 335–336. doi: 10.1038/nmeth.f.303
Clemente, J. C., Pehrsson, E. C., Blaser, M. J., Sandhu, K., Gao, Z., Wang, B., et al. (2015). The microbiome of uncontacted Amerindians. Sci. Adv. 1:e1500183. doi: 10.1126/sciadv.1500183
Cole, J. R., Chai, B., Farris, R. J., Wang, Q., Kulam-Syed-Mohideen, A. S., McGarrell, D. M., et al. (2007). The ribosomal database project (RDP-II): introducing myRDP space and quality controlled public data. Nucleic Acids Res. 35, D169–D172. doi: 10.1093/nar/gkl889
Cornelison, C. T., Cherney, B., Gabriel, K. T., Barlament, C. K., and Crow, S. A. Jr. (2016). Contact-independent antagonism of Ophidiomyces ophiodiicola, the causative agent of snake fungal disease by Rhodococcus rhodochrous DAP 96253 and select volatile organic compounds. J. Vet. Sci. Technol. 7:6. doi: 10.4172/2157-7579.1000397
De Cáceres, M., and Legendre, P. (2009). Associations between species and groups of sites: indices and statistical inference. Ecology 90, 3566–3574. doi: 10.1890/08-1823.1
DeSantis, T. Z., Hugenholtz, P., Larsen, N., Rojas, M., Brodie, E. L., Keller, K., et al. (2006). Greengenes, a chimera-checked 16S rRNA gene database and workbench compatible with ARB. Appl. Environ. Microbiol. 72, 5069–5072. doi: 10.1128/AEM.03006-05
Douglas, G. M., Maffei, V. J., Zaneveld, J., Yurgel, S. N., Brown, J. R., Taylor, C. M., et al. (2020). PICRUSt2: An improved and extensible approach for metagenome inference. bioRxiv doi: 10.1101/672295
Dufrêne, M., and Legendre, P. (1997). Species assemblages and indicator species: the need for a flexible asymmetrical approach. Ecol. Monogr. 67, 345–366. doi: 10.1890/0012-96151997067[0345:SAAIST]2.0.CO;2
Edgar, R. C. (2010). Search and clustering orders of magnitude faster than BLAST. Bioinformatics 26, 2460–2461. doi: 10.1093/bioinformatics/btq461
Fitzpatrick, B. M., and Allison, A. L. (2014). Similarity and differentiation between bacteria associated with skin of salamanders (Plethodon jordani) and free-living assemblages. FEMS Microbiol. Ecol. 88, 482–494. doi: 10.1111/1574-6941.12314
Fountain, K., Roberts, L., Young, V., Barbon, A., Frosini, S. M., Lloyd, D. H., et al. (2019). Diversity of staphylococcal species cultured from captive livingstone’s fruit bats (pteropus livingstonii) and their environment. J. Zoo. Wildlife. Med. 50, 266–269. doi: 10.1638/2018-0121
Fu, H. G., Hu, X. X., Li, C. R., Li, Y. H., Wang, Y. X., Jiang, J. D., et al. (2016). Design, synthesis and biological evaluation of monobactams as antibacterial agents against gram-negative bacteria. Eur. J. Med. Chem. 110, 151–163. doi: 10.1016/j.ejmech.2016.01.024
Fuhrer, A., Sprenger, N., Kurakevich, E., Borsig, L., Chassard, C., and Hennet, T. (2010). Milk sialyllactose influences colitis in mice through selective intestinal bacterial colonization. J. Exp. Med. 207, 2843–2854. doi: 10.1084/jem.20101098
Gargas, A., Trest, M. T., Christensen, M., Volk, T. J., and Blehert, D. S. (2009). Geomyces destructans sp. nov. associated with bat white-nose syndrome. Mycotaxon 108, 147–154. doi: 10.5248/108.147
Giles, E. C., Kamke, J., Moitinho-Silva, L., Taylor, M. W., Hentschel, U., Ravasi, T., et al. (2013). Bacterial community profiles in low microbial abundance sponges. FEMS Microbiol. Ecol. 83, 232–241. doi: 10.1111/j.1574-6941.2012.01467.x
Grisnik, M., Bowers, O., Moore, A. J., Jones, B. F., Campbell, J. R., and Walker, D. M. (2020). The cutaneous microbiota of bats has in vitro antifungal activity against the white nose pathogen. FEMS Microbiol. Ecol. 96, fiz193. doi: 10.1093/femsec/fiz193
Hooper, L. V., Dan, R. L., and Macpherson, A. J. (2012). Interactions between the microbiota and the immune system. Science 336, 1268–1273. doi: 10.1126/science.1223490
Hoyt, J. R., Cheng, T. L., Langwig, K. E., Hee, M. M., Frick, W. F., and Kilpatrick, A. M. (2015). Bacteria isolated from bats inhibit the growth of Pseudogymnoascus destructans, the causative agent of white-nose syndrome. PLoS One 10:e0121329. doi: 10.1371/journal.pone.0121329
Hoyt, J. R., Langwig, K. E., Sun, K., Parise, K. L., Li, A., Wang, Y., et al. (2020). Environmental reservoir dynamics predict global infection patterns and population impacts for the fungal disease white-nose syndrome. Proc. Natl. Acad. Sci. U.S.A. 117, 7255–7262. doi: 10.1073/pnas.1914794117
Hoyt, J. R., Sun, K., Parise, K. L., Lu, G., Langwig, K. E., Jiang, T., et al. (2016b). Widespread bat white-nose syndrome fungus, northeastern China. Emerg. Infect. Dis. 22, 140–142. doi: 10.3201/eid2201.151314
Hoyt, J. R., Langwig, K. E., Sun, K., Lu, G., Parise, K. L., Jiang, T., et al. (2016a). Host persistence or extinction from emerging infectious disease: insights from white-nose syndrome in endemic and invading regions. Proc. Biol. Sci. 283:20152861. doi: 10.1098/rspb.2015.2861
Hughey, M. C., Pena, J. A., Reyes, R., Medina, D., Belden, L. K., and Burrowes, P. A. (2017). Skin bacterial microbiome of a generalist Puerto Rican frog varies along elevation and land use gradients. PeerJ 5:e3688. doi: 10.7717/peerj.3688
Ingala, M. R., Ravenelle, R. E., Monro, J. J., and Frank, C. L. (2017). The effects of epidermal fatty acid profiles, 1-oleoglycerol, and triacylglycerols on the susceptibility of hibernating bats to Pseudogymnoascus destructans. PLoS One 12:e0187195. doi: 10.1371/journal.pone.0187195
Jani, A. J., and Briggs, C. J. (2014). The pathogen Batrachochytrium dendrobatidis disturbs the frog skin microbiome during a natural epidemic and experimental infection. Proc. Natl. Acad. Sci. U.S.A. 111, E5049–E5058. doi: 10.1073/pnas.1412752111
Jani, A. J., and Briggs, C. J. (2018). Host and aquatic environment shape the amphibian skin microbiome but effects on downstream resistance to the pathogen Batrachochytrium dendrobatidis are variable. Front. Microbiol. 9:487. doi: 10.3389/fmicb.2018.00487
Jiménez, R. R., Alvarado, G., Estrella, J., and Sommer, S. (2019). Moving beyond the host: unraveling the skin microbiome of endangered Costa Rican amphibians. Front. Microbiol. 10:2060. doi: 10.3389/fmicb.2019.02060
Koropatnick, T. A., Engle, J. T., Apicella, M. A., Stabb, E. V., Goldman, W. E., and McFall-Ngai, M. J. (2004). Microbial factor-mediated development in a host-bacterial mutualism. Science 306, 1186–1188. doi: 10.1126/science.1102218
Krediet, C. J., Ritchie, K. B., Paul, V. J., and Teplitski, M. (2013). Coral-associated micro-organisms and their roles in promoting coral health and thwarting diseases. Proc. Biol. Sci. 280:20122328. doi: 10.1098/rspb.2012.2328
Kruger, A. (2020). Frog skin microbiota vary with host species and environment but not chytrid infection. Front. Microbiol. 11:1330. doi: 10.3389/fmicb.2020.01330
Kueneman, J. G., Parfrey, L. W., Woodhams, D. C., Archer, H. M., Knight, R., and McKenzie, V. J. (2014). The amphibian skin-associated microbiome across species, space and life history stages. Mol. Ecol. 23, 1238–1250. doi: 10.1111/mec.12510
Langille, M. G., Zaneveld, J., Caporaso, J. G., McDonald, D., Knights, D., Reyes, J. A., et al. (2013). Predictive functional profiling of microbial communities using 16S rRNA marker gene sequences. Nat. Biotechnol. 31, 814–821. doi: 10.1038/nbt.2676
Langwig, K. E., Frick, W. F., Hoyt, J. R., Parise, K. L., Drees, K. P., Kunz, T. H., et al. (2016). Drivers of variation in species impacts for a multi-host fungal disease of bats. Philos. Trans. R. Soc. Lond. B. Biol. Sci. 371:20150456. doi: 10.1098/rstb.2015.0456
Langwig, K. E., Frick, W. F., Reynolds, R., Parise, K. L., Drees, K. P., Hoyt, J. R., et al. (2015). Host and pathogen ecology drive the seasonal dynamics of a fungal disease, white-nose syndrome. Proc. Biol. Sci. 282:20142335. doi: 10.1098/rspb.2014.2335
Lemieux-Labonté, V., Dorville, N. A. S., Willis, C. K. R., and Lapointe, F. J. (2020). Antifungal potential of the skin microbiota of hibernating big brown bats (Eptesicus fuscus) infected with the causal agent of white-nose syndrome. Front. Microbiol. 11:1776. doi: 10.3389/fmicb.2020.01776
Lemieux-Labonté, V., Simard, A., Willis, C. K. R., and Lapointe, F. J. (2017). Enrichment of beneficial bacteria in the skin microbiota of bats persisting with white-nose syndrome. Microbiome 5:115. doi: 10.1186/s40168-017-0334-y
Lemieux-Labonté, V., Tromas, N., Shapiro, B. J., and Lapointe, F. J. (2016). Environment and host species shape the skin microbiome of captive neotropical bats. PeerJ 4:e2430. doi: 10.7717/peerj.2430
Li, A., Li, Z., Dai, W., Parise, K. L., Leng, H., Jin, L., et al. (2021). Bacterial community dynamics on bats and the implications for pathogen resistance. Environ. Microbiol. 2021:15754. doi: 10.1111/1462-2920.15754
Li, Z., Li, A., Hoyt, J. R., Dai, W., Leng, H., Li, Y., et al. (2022). Activity of bacteria isolated from bats against Pseudogymnoascus destructans in China. Microb. Biotechnol. 15, 469–481. doi: 10.1111/1751-7915.13765
Lokmer, A., and Mathias Wegner, K. (2015). Hemolymph microbiome of Pacific oysters in response to temperature, temperature stress and infection. ISME J. 9, 670–682. doi: 10.1038/ismej.2014.160
Longo, A. V., and Zamudio, K. R. (2017). Temperature variation, bacterial diversity and fungal infection dynamics in the amphibian skin. Mol. Ecol. 26, 4787–4797. doi: 10.1111/mec.14220
Longo, A. V., Savage, A. E., Hewson, I., and Zamudio, K. R. (2015). Seasonal and ontogenetic variation of skin microbial communities and relationships to natural disease dynamics in declining amphibians. R. Soc. Open. Sci. 2:140377. doi: 10.1098/rsos.140377
Lorch, J. M., Palmer, J. M., Lindner, D. L., Ballmann, A. E., George, K. G., Griffin, K., et al. (2016). First detection of bat white-nose syndrome in western North America. mSphere 1, e148–e116. doi: 10.1128/mSphere.00148-16
Loudon, A. H., Woodhams, D. C., Parfrey, L. W., Archer, H., Knight, R., McKenzie, V., et al. (2014). Microbial community dynamics and effect of environmental microbial reservoirs on red-backed salamanders (Plethodon cinereus). ISME J. 8, 830–840. doi: 10.1038/ismej.2013.200
Lu, Y. Z., Ding, Z. W., Ding, J., Fu, L., and Zeng, R. J. (2015). Design and evaluation of universal 16S rRNA gene primers for high-throughput sequencing to simultaneously detect DAMO microbes and anammox bacteria. Water Res. 87, 385–394. doi: 10.1016/j.watres.2015.09.042
Manus, M. B., Yu, J. J., Park, L. P., Mueller, O., Windsor, S. C., Horvath, J. E., et al. (2017). Environmental influences on the skin microbiome of humans and cattle in rural Madagascar. Evol. Med. Public. Health. 2017, 144–153. doi: 10.1093/emph/eox013
Marmulla, R., and Harder, J. (2014). Microbial monoterpene transformations-a review. Front. Microbiol. 5:346. doi: 10.3389/fmicb.2014.00346
Martin, M. (2011). Cutadapt removes adapter sequences from high-throughput sequencing reads. Embnet J. 17, 10–12. doi: 10.14806/ej.17.1.200
McMurdie, P. J., and Holmes, S. (2013). phyloseq: an R package for reproducible interactive analysis and graphics of microbiome census data. PLoS One 8:e61217. doi: 10.1371/journal.pone.0061217
Meteyer, C. U., Buckles, E. L., Blehert, D. S., Hicks, A. C., Green, D. E., Shearn-Bochsler, V., et al. (2009). Histopathologic criteria to confirm white-nose syndrome in bats. J. Vet. Diagn. Invest. 21, 411–414. doi: 10.1177/104063870902100401
Muletz, C. R., Myers, J. M., Domangue, R. J., Herrick, J. B., and Harris, R. N. (2012). Soil bioaugmentation with amphibian cutaneous bacteria protects amphibian hosts from infection by Batrachochytrium dendrobatidis. Biol. Conserv. 152, 119–126. doi: 10.1016/j.biocon.2012.03.022
Muletz-Wolz, C. R., Yarwood, S. A., Campbell Grant, E. H., Fleischer, R. C., and Lips, K. R. (2018). Effects of host species and environment on the skin microbiome of Plethodontid salamanders. J. Anim. Ecol. 87, 341–353. doi: 10.1111/1365-2656.12726
Muller, L. K., Lorch, J. M., Lindner, D. L., O’Connor, M., Gargas, A., and Blehert, D. S. (2013). Bat white-nose syndrome: a real-time TaqMan polymerase chain reaction test targeting the intergenic spacer region of Geomyces destructans. Mycologia 105, 253–259. doi: 10.3852/12-242
Oksanen, J., Kindt, R., Legendre, P., O’Hara, B., and Suggests, M. (2015). Vegan community ecology package: ordination methods, diversity analysis and other functions for community and vegetation ecologists. Available online at: https://CRAN.R-project.org/package=vegan.
O’Sullivan, J. N., Rea, M. C., O’Connor, P. M., Hill, C., and Ross, R. P. (2019). Human skin microbiota is a rich source of bacteriocin-producing staphylococci that kill human pathogens. FEMS Microbiol. Ecol. 95:fiy241. doi: 10.1093/femsec/fiy241
Puechmaille, S. J., Wibbelt, G., Korn, V., Fuller, H., Forget, F., Mühldorfer, K., et al. (2011). Pan-European distribution of white-nose syndrome fungus (Geomyces destructans) not associated with mass mortality. PLoS One 6:e19167. doi: 10.1371/journal.pone.0019167
Rebollar, E. A., Gutiérrez-Preciado, A., Noecker, C., Eng, A., Hughey, M. C., Medina, D., et al. (2018). The skin microbiome of the neotropical frog Craugastor fitzingeri: Inferring potential bacterial-host-pathogen interactions from metagenomic data. Front. Microbiol. 9:466. doi: 10.3389/fmicb.2018.00466
Rebollar, E. A., Hughey, M. C., Medina, D., Harris, R. N., Ibáñez, R., and Belden, L. K. (2016). Skin bacterial diversity of Panamanian frogs is associated with host susceptibility and presence of Batrachochytrium dendrobatidis. ISME J. 10, 1682–1695. doi: 10.1038/ismej.2015.234
Rosenberg, E., Koren, O., Reshef, L., Efrony, R., and Zilber-Rosenberg, I. (2007). The role of microorganisms in coral health, disease and evolution. Nat. Rev. Microbiol. 5, 355–362. doi: 10.1038/nrmicro1635
Rosenthal, M., Goldberg, D., Aiello, A., Larson, E., and Foxman, B. (2011). Skin microbiota: microbial community structure and its potential association with health and disease. Infect. Genet. Evol. 11, 839–848. doi: 10.1016/j.meegid.2011.03.022
Ross, A. A., Müller, K. M., Weese, J. S., and Neufeld, J. D. (2018). Comprehensive skin microbiome analysis reveals the uniqueness of human skin and evidence for phylosymbiosis within the class Mammalia. Proc. Natl. Acad. Sci. U.S.A. 115, E5786–E5795. doi: 10.1073/pnas.1801302115
Ross, A. A., Rodrigues Hoffmann, A., and Neufeld, J. D. (2019). The skin microbiome of vertebrates. Microbiome 7:79. doi: 10.1186/s40168-019-0694-6
Segata, N., Izard, J., Waldron, L., Gevers, D., Miropolsky, L., Garrett, W. S., et al. (2011). Metagenomic biomarker discovery and explanation. Genome. Biol. 12:R60. doi: 10.1186/gb-2011-12-6-r60
Si, J., Lee, S., Park, J. M., Sung, J., and Ko, G. (2015). Genetic associations and shared environmental effects on the skin microbiome of Korean twins. BMC Genom. 16:992. doi: 10.1186/s12864-015-2131-y
Starcher, G. W. (1942). Methods of correlation analysis. J. Appl. Sport. Psychol. 26, 112–113. doi: 10.1037/h0053436
Stecher, B., Robbiani, R., Walker, A. W., Westendorf, A. M., Barthel, M., Kremer, M., et al. (2007). Salmonella enterica serovar typhimurium exploits inflammation to compete with the intestinal microbiota. PLoS Biol. 5:2177–2189. doi: 10.1371/journal.pbio.0050244
Thomas, C. M., Hong, T., van Pijkeren, J. P., Hemarajata, P., Trinh, D. V., Hu, W., et al. (2012). Histamine derived from probiotic Lactobacillus reuteri suppresses TNF via modulation of PKA and ERK signaling. PLoS One 7:e31951. doi: 10.1371/journal.pone.0031951
Turner, G. G., Reeder, D. M., and Coleman, J. T. H. (2011). A five-year assessment of mortality and geographic spread of white-nose syndrome in North American bats, with a look to the future. Bat. Res. News. 52, 13–27.
Vanderwolf, K. J., Campbell, L. J., Goldberg, T. L., Blehert, D. S., and Lorch, J. M. (2021). Skin fungal assemblages of bats vary based on susceptibility to white-nose syndrome. ISME J. 15, 909–920. doi: 10.1038/s41396-020-00821-w
Walke, J. B., Becker, M. H., Loftus, S. C., House, L. L., Cormier, G., Jensen, R. V., et al. (2014). Amphibian skin may select for rare environmental microbes. ISME J. 8, 2207–2217. doi: 10.1038/ismej.2014.77
Wei, L., Zeng, B., Zhang, S., Li, F., Kong, F., Ran, H., et al. (2020). Inbreeding alters the gut microbiota of the banna minipig. Animals 10:2125. doi: 10.3390/ani10112125
Winter, A. S., Hathaway, J. J. M., Kimble, J. C., Buecher, D. C., Valdez, E. W., Porras-Alfaro, A., et al. (2017). Skin and fur bacterial diversity and community structure on American southwestern bats: effects of habitat, geography and bat traits. PeerJ 5:e3944. doi: 10.7717/peerj.3944
Woodhams, D. C., Alford, R. A., Antwis, R. E., Archer, H., Becker, M. H., Belden, L. K., et al. (2015). Antifungal isolates database of amphibian skin-associated bacteria and function against emerging fungal pathogens. Ecology 96:595. doi: 10.1890/14-1837.1
Woodhams, D. C., LaBumbard, B. C., Barnhart, K. L., Becker, M. H., Bletz, M. C., Escobar, L. A., et al. (2018). Prodigiosin, violacein, and volatile organic compounds produced by widespread cutaneous bacteria of amphibians can inhibit two Batrachochytrium fungal pathogens. Microb. Ecol. 75, 1049–1062. doi: 10.1007/s00248-017-1095-7
Keywords: bat, Pseudogymnoascus destructans, pathogen defense, skin microbiota, white-nose syndrome
Citation: Li Z, Li A, Dai W, Leng H, Liu S, Jin L, Sun K and Feng J (2022) Skin Microbiota Variation Among Bat Species in China and Their Potential Defense Against Pathogens. Front. Microbiol. 13:808788. doi: 10.3389/fmicb.2022.808788
Received: 04 November 2021; Accepted: 18 February 2022;
Published: 31 March 2022.
Edited by:
John J. Maurer, Virginia Tech, United StatesReviewed by:
Jianping Xu, McMaster University, CanadaDaniel Bruce Raudabaugh, University of Illinois at Urbana-Champaign, United States
Karen Vanderwolf, Trent University, Canada
Copyright © 2022 Li, Li, Dai, Leng, Liu, Jin, Sun and Feng. This is an open-access article distributed under the terms of the Creative Commons Attribution License (CC BY). The use, distribution or reproduction in other forums is permitted, provided the original author(s) and the copyright owner(s) are credited and that the original publication in this journal is cited, in accordance with accepted academic practice. No use, distribution or reproduction is permitted which does not comply with these terms.
*Correspondence: Keping Sun, c3Vua3AxMjlAbmVudS5lZHUuY24=; Jiang Feng, ZmVuZ2pAbmVudS5lZHUuY24=