Corrigendum: Comparative respiratory tract microbiome between carbapenem-resistant Acinetobacter baumannii colonization and ventilator associated pneumonia
- 1State Key Laboratory for Diagnosis and Treatment of Infectious Diseases, National Clinical Research Center for Infectious Diseases, National Medical Center for Infectious Diseases, Collaborative Innovation Center for Diagnosis and Treatment of Infectious Diseases, The First Affiliated Hospital, Zhejiang University School of Medicine, Hangzhou, China
- 2State Key Laboratory for Turbulence and Complex Systems, Department of Biomedical Engineering, College of Future Technology and Center for Quantitative Biology, Peking University, Beijing, China
- 3Department of Intensive Care Unit, The First Affiliated Hospital, Zhejiang University School of Medicine, Hangzhou, China
- 4Shenzhen Institute of Respiratory Diseases, The First Affiliated Hospital (Shenzhen People’s Hospital), Southern University of Science and Technology, Shenzhen, China
Background: Carbapenem-resistant Acinetobacter baumannii (CRAB) is a common cause of ventilator-associated pneumonia (VAP) in intensive care unit (ICU) patients, but its infection and colonization state are difficult to distinguish. If the judgment is wrong, it may aggravate the abuse of antibiotics and further accelerate the evolution of drug resistance. We sought to provide new clues for the diagnosis, pathogenesis and treatment of CRAB VAP based on lower respiratory tract (LRT) microbiota.
Methods: A prospective study was conducted on patients with mechanical ventilation from July 2018 to December 2019 in a tertiary hospital. Multi-genomics studies (16S rRNA amplicon, metagenomics, and whole-genome sequencing [WGS]) of endotracheal deep aspirate (ETA) were performed.
Results: Fifty-two ICU patients were enrolled, including 24 with CRAB VAP (CRAB-I), 22 with CRAB colonization (CRAB-C), and six CRAB-negative patients (infection-free) (CRAB-N). Diversity of pulmonary microbiota was significantly lower in CRAB-I than in CRAB-C or CRAB-N (mean Shannon index, 1.79 vs. 2.73 vs. 4.81, P < 0.05). Abundances of 11 key genera differed between the groups. Acinetobacter was most abundant in CRAB-I (76.19%), moderately abundant in CRAB-C (59.14%), and least abundant in CRAB-N (11.25%), but its interactions with other genera increased in turn. Metagenomics and WGS analysis showed that virulence genes were more abundant in CRAB-I than in CRAB-C. Multi-locus sequence typing (MLST) of 46 CRAB isolates revealed that the main types were ST208 (30.43%) and ST938 (15.22%), with no difference between CRAB-I and CRAB-C.
Conclusion: Lower respiratory tract microbiota dysbiosis including elevated relative abundance of Acinetobacter and reduced bacterial interactions, and virulence enrichment may lead to CRAB VAP.
Introduction
Acinetobacter baumannii (AB) is a ubiquitous microorganism that can contaminate the surface of hospital equipment and colonize skin, wounds, and other parts of patients (Shamsizadeh et al., 2017). Ventilator associated pneumonia (VAP) is a frequent complication in ICUs and is associated with prolonged mechanical ventilation, longer intensive care unit (ICU) stay, and poorer outcomes (Papazian et al., 2020). Acinetobacter baumannii is frequently isolated from the respiratory tract in patients with tracheal intubation, which is considered to be a high-risk factor for VAP (Consales et al., 2011; Huang et al., 2018). Yin et al. (2021) found that the prevalence of AB was high (31.7%) among VAP pathogens in 15 teaching hospitals in China from 2007 to 2016. Due to the heavy use of broad-spectrum antibacterial agents in ICU patients, most AB strains were multi-drug resistant or even pan-drug resistant. A survey of hospital-acquired pneumonia (HAP) and VAP conducted from 2007 to 2013 revealed that multidrug-resistant AB (MDRAB) increased yearly and ranked first in some ICU bacterial lists (Hu et al., 2016).
The carbapenem-resistant AB (CRAB) genome encodes various drug-resistance genes and virulence factors (VFs), including efflux pumps, iron acquisition systems, secretion systems, phospholipases, and capsular polysaccharides, which help the bacterium survive antibiotic treatment and colonize in the environment (Harding et al., 2018; Uppalapati et al., 2020). Without effective therapy, the mortality of patients with nosocomial CRAB infection remains high (Xie et al., 2018; Yin et al., 2021). Accordingly, the World Health Organization (WHO) has designated CRAB as a pathogen that poses a major threat to human health and that should be urgently targeted by new antibiotics (WHO, 2017).
A key step in containing CRAB is the rational use of antibiotics based on accurate diagnosis of infection. However, the challenge of differentiating CRAB colonization and infection could lead clinicians to prescribe excessive broad-spectrum antibiotics, potentially promoting the occurrence of drug-resistant bacteria and their spread in hospitals. Therefore, clinical infection control requires reliable methods for distinguishing CRAB colonization from CRAB infection. Reduction in lower respiratory tract (LRT) microbiota diversity or elevated abundance of certain strains may lead to infections (Dickson et al., 2014; Kelly et al., 2016). For example, LRT microbiota diversity was lower in 13 infected patients than in healthy control patients, and pathogenic bacteria in four subjects was consistent with the dominant microbiota identified by 16S rRNA analysis (Kelly et al., 2016). Through 16S rRNA analysis of 263 samples, Emonet et al. (2019) found that the low relative abundance of species in oropharyngeal secretions during intubation was strongly associated with subsequent VAP.
However, many aspects of the relationship between respiratory microecology and infection remain unknown, and current research is focused mainly on chronic lung diseases (Faner et al., 2017; Budden et al., 2019). Budden et al. (2019) reviewed recent advances in understanding the composition of the lung microbiome and found that bacteria, viruses, and fungi from the respiratory tract produce structural ligands and metabolites that interact with the host and alter the development and progression of chronic respiratory diseases. Hakansson et al. (2018) suggested that a complex interplay between the host, environment, and properties of the colonizing microorganisms determines disease development and severity. Moreover, Roquilly et al. (2019) proposed that the diversity of the microbiome and mucosal immunity are associated with hospital acquired pneumonia (HAP). Metagenomic analysis of microbiome structure and function will aid in understanding the pathogenesis and regulatory networks of AB during infection (Faner et al., 2017).
This is the first study to analyse and compare the characteristics of LRT microbiota of CRAB-negative, CRAB-colonized, and CRAB-infected patients using 16S rRNA, metagenomics, and whole-genome sequencing (WGS). We investigated whether VAP patients are associated with unique LRT microbiota to explore the pathogenesis of VAP at the level of the microbiota and provide an improved basis for clinical decision-making.
Materials and Methods
Study Design and Patient Enrolment
This prospective study was conducted from July 2018 to December 2019 in the adult ICUs of the First Affiliated Hospital, College of Medicine of Zhejiang University, China. The patient inclusion criteria were as follows: (1) patient was mechanically ventilated and hospitalized in the ICU; (2) Acute Physiology and Chronic Health Evaluation (APACHE) II score was greater than 12; and (3) collection of endotracheal deep aspirate (ETA) specimens was possible. Exclusion criteria were as follows: (1) age < 18 years; (2) comorbidity of chronic lung disease or lung cancer; (3) hospital stay < 24 h; and (4) co-infection with other bacteria. VAP was defined by the criteria of the Centers for Disease Control and Prevention (CDC) of the United States based on clinical, laboratory, radiological, and microbiological data (Horan et al., 2008). Patients meeting VAP criteria with positive culture for CRAB ETA were assigned to the CRAB VAP group (CRAB-I). Respiratory tract CRAB colonization (CRAB-C) was defined as CRAB-positive culture from ETA without VAP. Control patients (CRAB-N) were patients with neither CRAB VAP nor CRAB colonization. Approval was obtained from the ethical board of the hospital (reference number: 2016-458-1).
Patient Sampling and Bacterial Isolation
The aspirate samples were transported to the microbiology laboratory within 2 h. ETAs were inoculated with a calibrated loop (0.001 ml). A gram-stained smear was prepared for all specimens and examined microscopically. If the number of squamous epithelial cells is less than 10/ each low power field, it is judged as qualified. MacConkey agar (Oxoid, United Kingdom) and incubated aerobically at 37°C overnight. Acinetobacter baumannii was identified by using biochemical methods and matrix-assisted laser desorption-time of flight mass spectrometry (MALDI-TOF MS) (Bruker, Bremen, Germany). The confirmed A. baumannii from clinical and sputum samples were stored at −80°C for further study. The resistance of A. baumannii to antibiotics, including Piperacillin/tazobactam (TZP), Cefoperazone/sulbactam (CSL), Ceftazidime (CAZ), Ceftriaxone (CRO), Cefepime (FEP), Ciprofloxacin (CIP), Levofloxacin (LVX), Imipenem (IPM), Meropenem (MEM), Trimethoprim/sulfamethoxazole (TMX/SXT), Amikacin (AMK), and Gentamicin (GEN), was analyzed according to the recommendation of Clinical and Laboratory Standards (CLSI, 2018). Resistance to Tigecycline (TGC) and Polymyxin (POL) was examined by the broth microdilution method and evaluated according to the European Committee on Antimicrobial Susceptibility testing (2016). Escherichia coli ATCC25922 was used as a quality control strain. CRAB was defined as AB strains that were non-susceptible to imipenem or meropenem. For CRAB-C group, we selected samples and strains cultured for the first time for metagenomics and whole-genome sequencing. If the patients were in CRAB-I group, we selected the samples of the latest infection state and the cultured strains for sequencing.
Clinical Data
Clinical data including demographic variables, length of ICU stay, length of hospital stay, comorbidities, previous invasive procedures (central line insertion, intubation, continuous renal replacement therapy, and surgery under general an aesthesia), as well as lengths and types of antibiotic treatments and severity of illness [Acute Physiology and Chronic Health Evaluation (APACHE II)], were recorded (LeGall et al., 1986). Data on the levels of serum markers for liver and renal function [e.g., bilirubin, alanine transaminase (ALT), aspartate transaminase (AST), gamma glutamyl transpeptidase (γ-GT), urea, and creatinine] as well as those of blood biomarkers of infection other than core temperature [e.g., white blood cell (WBC) and CRP] were also collected.
16S rRNA Amplicon and Data Analysis
Total genomic DNA was extracted from samples using the cetyl trimethylammonium bromide (CTAB) method. We performed PCR amplification and pooled purified amplicons and then carried out the paired-end sequencing on an Illumina NovaSeq PE250 platform. After demultiplexing and trimming of the barcode and primer sequence using FLASH (V1.2.7) (Magoč and Salzberg, 2011), the paired-end raw read data of each sample was acquired. Subsequently, quality control was carried out using Qiime (Caporaso et al., 2010; Bokulich et al., 2013) and effective tags were obtained for analysis. OTUs, clustered with a 97% similarity cut-off using Usearch (Version 7.0) (Edgar et al., 2011), were taxonomically annotated using Mothur and SILVA database (Quast et al., 2013). MUSCLE (Version 3.8.31) software was used for rapid multiple sequence alignment and the phylogenetic analysis for all OTU representative sequences (Edgar, 2004). We then constructed the abundance matrices at the levels of phylum, class, order, family, and genus were constructed for each sample. Alpha diversity (Shannon and Simpson index within a sample) and Beta diversity (Bray–Curtis dissimilarity matrix across samples) were calculated using the phyloseq R package (version 1.32.0). PCoA and NMDS analysis based on Bray–Curtis dissimilarity was performed using the Vegan R package (version 2.5.6) and visualized using the ggplot2 R package (version 3.3.2). Analysis of similarity (ANOSIM) was used to test whether there is significant. We also calculated the co-occurrence relationships between bacteria using Python-based SparCC tool with SparCC correlation method and visualized using Cytoscape (Version 3.8.0) (Csardi and Nepusz, 2006). Sample names and the corresponding NCBI accession numbers are listed in Supplementary Table 1.
Metagenomics Sequencing and Analysis
Following fragmentation of microbial DNA, metagenomic sequencing was performed on an Illumina NovaSeq 6000. After paired-end Illumina sequencing, we employed a previously reported bioinformatics pipeline to detect and profile the airway microbiome (Dickson et al., 2014; Kelly et al., 2016; Faner et al., 2017; Emonet et al., 2019). The low-quality sequences were filtered out or trimmed using PRINSEQ-lite (Version 0.19.3). And then de novo assemblies were generated using SPAdes genome assembler (Version 3.11.1) (Nurk et al., 2017) and coding sequences were predicted using MetaGeneMark (Version 3.38). Taxonomy assignments of both the clean reads and coding sequences were performed by Kaiju classifier (Version 1.7.2) (Menzel et al., 2016) with the National Center for Biotechnology Information Refseq database. We also utilized tool StrainPhlAn (Truong et al., 2017) to detected the multiple CRAB strains in ETA metagenomics samples. The functions of coding sequences were obtained using DIAMOND software (Version 0.9.30) with Kyoto Encyclopedia of Gene and Genomes (KEGG) database (Buchfink et al., 2015; Kanehisa et al., 2017). Furthermore, virulence genes in metagenomics sequences were identified by comparing the coding sequences against the Virulence Factor Database using DIAMOND software (Chen et al., 2016). The correlation coefficient between bacteria and virulence genes was generated using Python-based SparCC tool with SparCC correlation method. We performed multivariate linear regressions with feature selection, using the lasso penalized maximum likelihood technique in the “glmnet” R package (Version 4.0.2). Sample names and the corresponding NCBI accession numbers are listed in Supplementary Table 1.
Whole-Genome Sequencing and Analysis
The genomic DNA of 46 isolates was extracted using a Qiagen DNA purification kit (Qiagen, Hilden, Germany) and sequenced on an Illumina HiSeq 4000-PE150. For each isolate, de novo assembly of reads was performed using SPAdes (version 3.11) genome assembler. CheckM was used to check genome completeness and possible contamination into the genome (Parks et al., 2015). We annotated the assemblies using Prokka (version 1.14.6) (Tatusova et al., 2016). GFF format files produced by Prokka were subjected to Roary (version 3.11.2) (Page et al., 2015) to construct core genome. Meanwhile, we analyzed whether there were multiple CRAB ST clones in one ETA sample using the raw reads through MetaMLST (Zolfo et al., 2017). A maximum-likelihood phylogenetic tree was constructed using RaxML software (version 8.2.12) with 1,000 bootstraps replicates (Price et al., 2010). Average nucleotide identity (ANI) was calculated by using a pyani (version 0.2.10). ANI values above 95% between genomes of these isolates denote the same source (Jain et al., 2018). We then conducted MLST analysis according to the Institute Pasteur scheme (MLST-IP) and Oxford Database (MLST-OD) (Gaiarsa et al., 2019). Clonal complexes (CCs) were assigned by eBURST and were defined as single locus (Hall, 2013). CCs were named according to the number of the predicted founder ST. Furthermore, we detected Antimicrobial resistance genes (ARG) and AB virulence genes by comparing genome assemblies against the ResFinder antibiotic resistance gene database using the Abricate software (version 0.8) and against the Virulence Factor Database using the DIAMOND software (version 0.9.30), respectively (Seemann, 2017). We also determined the capsular polysaccharide (KL) and lipooligosaccharide outer core (OCL) synthesis of the A. baumannii using Kaptive software (version 0.5.1) (Wyres et al., 2020). Strain names and the corresponding NCBI accession numbers are listed in Supplementary Table 1.
Statistical Analysis
The Linear Discriminant Analysis Effect Size (LEfSe) program was used to identify bacterial taxa and virulence genes that were differently abundant between sample types (Segata et al., 2011). Normally distributed continuous variables were represented as means ± standard deviation (SD) and compared using Student’s t-test, whereas non-normally distributed continuous variables were represented as median and interquartile range (IQR) and compared using Mann–Whitney U-tests. Categorical variables were compared by the χ2 test or two-tailed Fisher’s exact test, as appropriate.
Accession Numbers
All sequencing data during the current study are available in the Sequence Read Archive (SRA). Metagenomics data and the 16S rRNA gene data are under BioProject PRJNA 681291, and the WGS data under BioProject PRJNA 679997.
Results
Characteristics of the Study Population
A total of 101 patients were screened. According to the inclusion criteria, 64 patients were enrolled, and 52 patients completed the entire study protocol (Figure 1): 24 with CRAB-I, 22 with CRAB-C, and six with CRAB-N. The CRAB-I and CRAB-C patients did not differ in terms of age, sex, or severity indices (APACHE II scores), but C-reactive protein (CRP) and 30 day mortality were higher in CRAB-I (Supplementary Table 2). There was no significant difference in the prior antibiotic therapy between CRAB-C and CRAB-I. All CRAB isolates were highly resistant to all antibiotics except amikacin, polymyxin, and tetracycline (Supplementary Figure 1).
Comparison of Microbiota of Endotracheal Deep Aspirate
Overview
16S rRNA sequencing from a total of 52 ETA specimens revealed that the average Operation taxonomic units (OTU) numbers for CRAB-N, CRAB-C, and CRAB-I were 1,427, 2,422, and 2,248, respectively. Rarefaction curves of numbers of observed OTUs per sample and group indicated that almost all OTUs present in each group were detected (Supplementary Figure 2). Three samples failed to sequence. The LRT microbiome was examined in the remaining 49 ETA specimens by shotgun metagenomic sequencing (Supplementary Table 1). The 16S rRNA sequencing data identified 597 genera, of which 249 (41.2%) were also identified in the metagenomic data (Supplementary Figures 3, 4). All of the alpha diversity indices in the metagenomic analysis were higher than those in the 16S amplicon analysis (Supplementary Figure 5). Interestingly, only single ST was found in each metagenomics sample, which was the same ST as that was identified in the corresponding WGS data.
Carbapenem-Resistant Acinetobacter baumannii-Positive Patients Had Reduced Microbiota Diversity
Relative to CRAB-N, the diversity of pulmonary microbiota in the CRAB-C group was significantly reduced, and a further reduction was observed in the CRAB-I group (Shannon index in CRAB-N, -C, and -I: 4.80 ± 1.47, 2.73 ± 1.24, and 1.79 ± 0.95; Simpson index: 0.90 ± 0.08, 0.60 ± 0.23, and 0.40 ± 0.20, respectively; P < 0.05 for all alfa-diversity index; Figures 2A,B). Moreover, principal co-ordinate analysis (PCoA) and non-metric multidimensional scaling (NMDS) analysis of the Bray–Curtis dissimilarity metric revealed that the composition of the microbiota between the three groups were quite different (P = 0.001 and Stress = 0.113; respectively; Figures 2C,D). Analysis of similarity (ANOSIM) comparative analysis revealed that the differences between the three groups were higher than those within each group, indicating that the microbial community structure of the LRT microbiota in the three groups was significantly distinct (Supplementary Table 3).
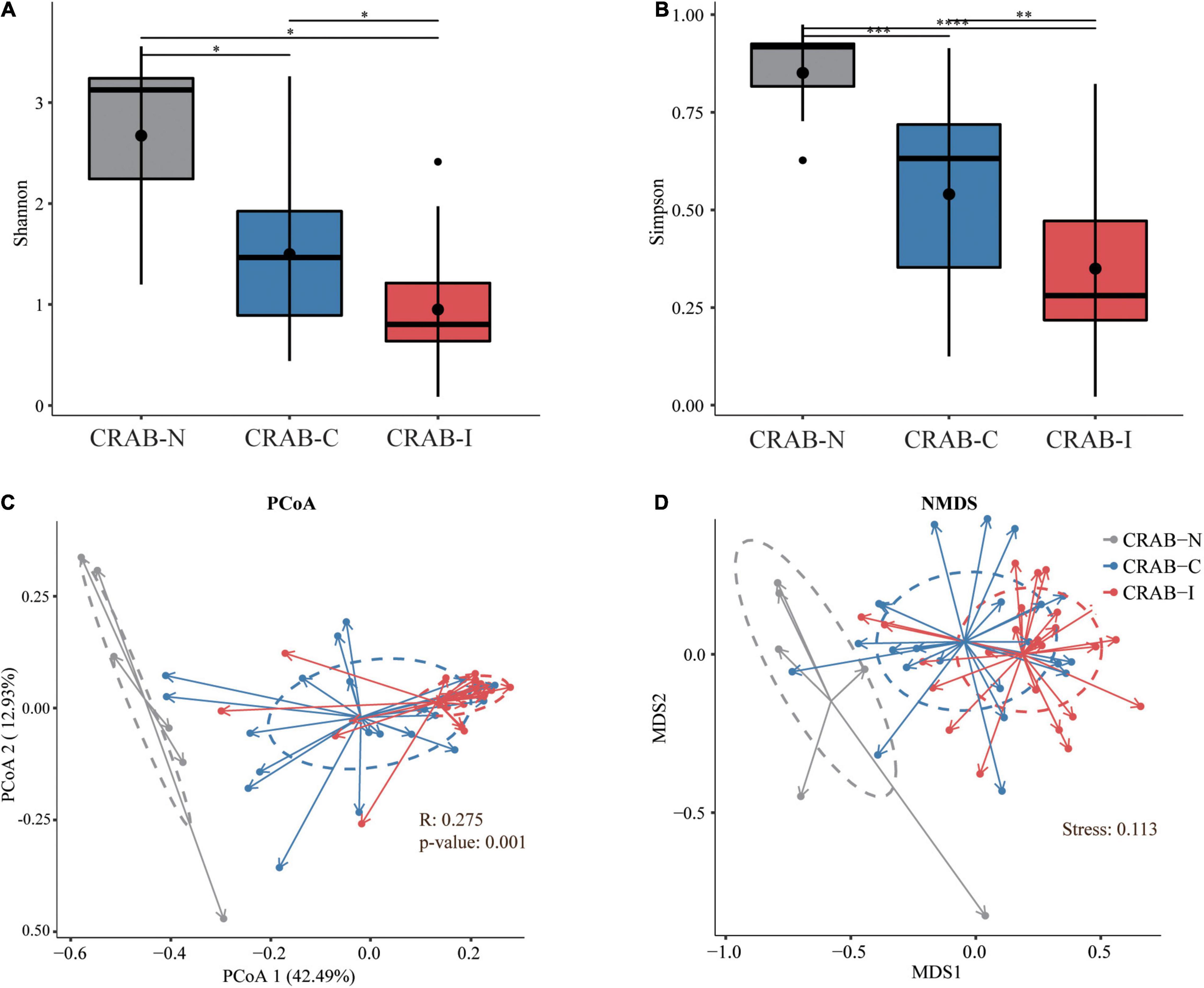
Figure 2. Phylogenetic diversity comparison of lower respiratory tract (LRT) microbiota in LRT microbiota of patients with neither VAP nor CRAB LRT colonization (CRAB-N), LRT microbiota of patients with CRAB colonization but without VAP (CRAB-C), and LRT microbiota of patients who developed CRAB VAP (CRAB-I) patients. (A) Box plots depict microbiota diversity differences according to the Shannon index among three group. (B) Box plots depict microbiota diversity differences according to the Gini Simpson index among three group. The upper and lower ranges of the box represent the 75 and 25% quartiles, respectively. (C) The X-axis shows PCo 1 and the Y-axis PCo 2 form Bray-Curtis distances for β-diversity. The changes per patient are visualized in as dashed lines dark red for CRAB-I, in blue for CRAB-C and in gray for CRAB-N. Logistic regression analysis showed that the change of PCo1 was significantly lower in patients who developed pneumonia (P = 0.001). (D) The X-axis shows MDS 1 and the Y-axis MDS 2 form Bray-Curtis distances for β-diversity (Stress = 0.113). The changes per patient are visualized in as dashed lines dark red for CRAB-I, in blue for CRAB-C and in gray for CRAB-N. Significant differences are indicated by *P < 0.05, **P < 0.01, ***P < 0.001, and ****P < 0.0001.
Bacterial Taxonomic Characters in the Three Groups
To obtain a global view of LRT microbiota in the study subjects, we compared the taxa at the phylum and genus levels between the three groups by 16S rRNA amplicon analysis. Overall, Proteobacteria (43.57%), Firmicutes (2.58%), and Bacteroidetes (2.42%) were the dominant phyla in the 52 ETA specimens (Figure 3A). The relative abundance of Proteobacteria was significantly higher in the CRAB-I and CRAB-C groups than in the CRAB-N group (91.77 vs. 84.50 vs. 49.25%, P < 0.05), and Firmicutes exhibited the opposite pattern (2.23 vs. 4.38 vs. 15.67%, P < 0.05; Figure 3B). At the genus level, the bacterial composition of the CRAB-positive groups differed from that of the negative group. Acinetobacter was the predominant genus in CRAB-I and CRAB-C patients (76.19 vs. 59.14%), followed by Klebsiella (5.80 vs. 8.01%) and Pseudomonas (2.84 vs. 6.88%). By contrast, in the CRAB-N group, the top three genera were Acinetobacter (11.24%), Haemophilus (8.67%), and Pseudomonas (9.68%) (Figures 3C,D).
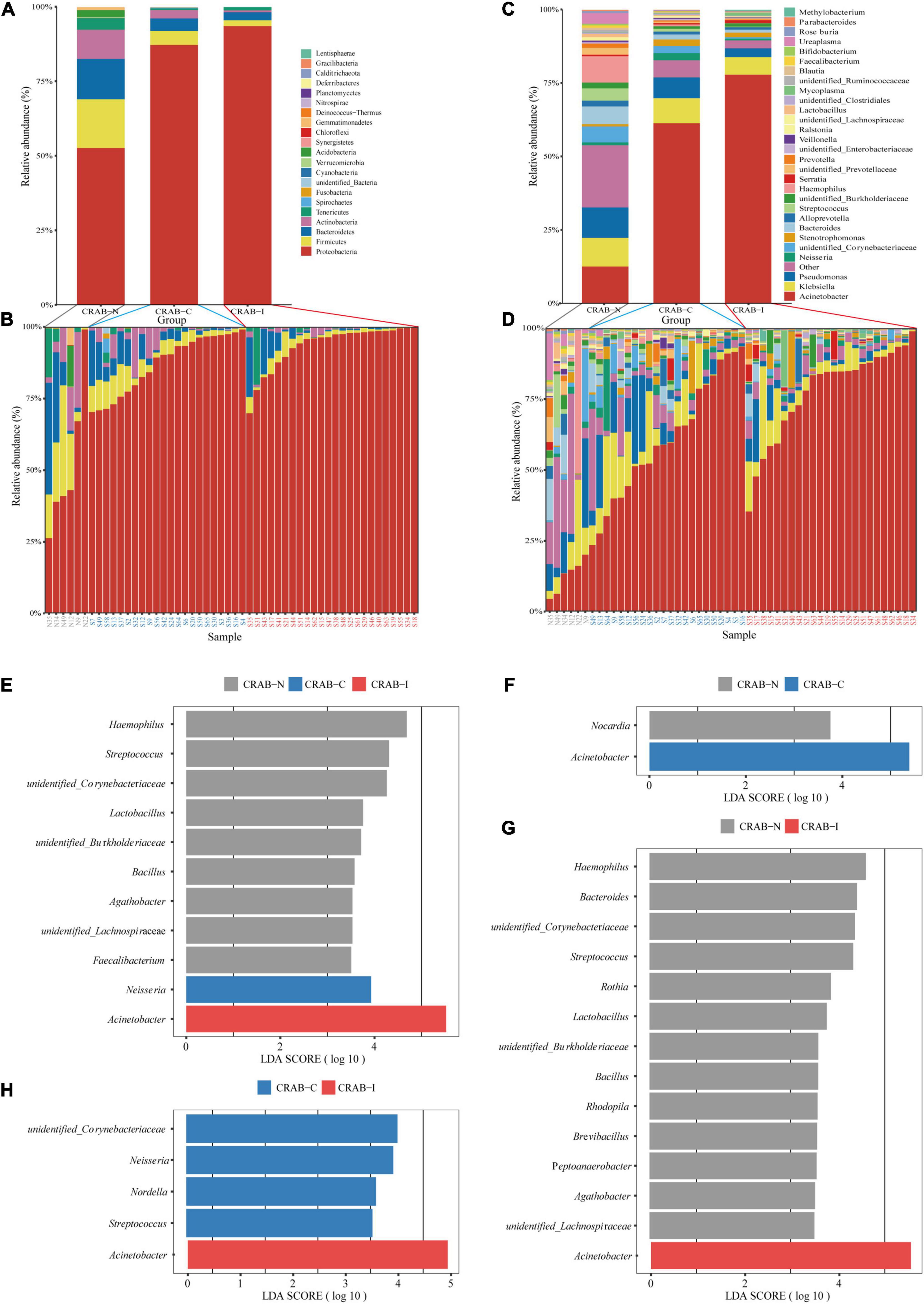
Figure 3. Comparison of phylum and genus of the LRT microbiota in the three patient groups and linear discriminant analysis effect size (LEfSe) analysis of the microbiota at genus level. (A) Comparison of the average abundance of each bacterial phylum in each group, respectively. (B) Comparison of the average abundance of bacterial phylum in each patient, respectively. (C) Comparison of the average abundance of each bacterial genus in each group, respectively. (D) Comparison of the average abundance of bacterial genus in each patient, respectively. The changes per patient are visualized in as dashed lines dark red for CRAB-I, in blue for CRAB-C and in gray for CRAB-N. (E) LDA scores indicated significant differences in the microbiota among the CRAB-N (gray), CRAB-C (blue), and CRAB-I patients (red). (F) LDA scores indicated significant differences in the microbiota between the CRAB-N (gray) and CRAB-C patients (blue). (G) LDA scores indicate significant differences in the microbiota between the CRAB-I patients (red) and CRAB-N controls (gray). (H) LDA scores indicate significant differences in the microbiota between the CRAB-I (red) and CRAB-C patients (blue). LDA scores > 3.5. The subject group is indicated by the color key at the top right corner.
The Linear Discriminant Analysis Effect Size (LEfSe) comparison identified 11 significant biomarker genera (Figure 3E) with discrimination value in the three groups. Further pairwise comparison revealed two significant biomarker genera (Acinetobacter and Nocardia) between the CRAB-C and CRAB-I groups (Figure 3F), and the CRAB-I group had 14 significant biomarker genera with the CRAB-N group: Acinetobacter was enriched in the CRAB-I group and 13 other genera, including Haemophilus, Bacteroides, and Streptococcus, were enriched in the CRAB-N group (Figure 3G). In addition, five key genera (Acinetobacter, unidentified_Corynebacteriacea, Nesseria, Nordella, and Streptococcus) differentiated between the CRAB-C and CRAB-I groups (Figure 3H).
Identifying Potential Microbial Interactions by Correlation Network Analysis
Overall, the microbial co-occurrence network constructed from CRAB-I had a lower complexity index than those of CRAB-C and CRAB- N at the genus level (4,239.37 vs. 4,257.25 vs. 7,459.00) (Figure 4). The total number of negative microbial interactions, as indicated by the number of edges between the nodes, was highest in CRAB-N, moderate in CRAB-C, and lowest in CRAB-I (CRAB-N: n = 2,356, 2,189 positive and 167 negative; CRAB-C: n = 1,436, 1,411 positive and 25 negative; CRAB-I: n = 1,533, 1,516 positive and 17 negative). Notably, in the CRAB-I group, Acinetobacter was abundant and significantly negatively correlated with four genera (Klebsiella, Pseudomonas, unidentified_Erysipelotrichaceae, and Oscillibacter), whereas in the CRAB-C group, it was negatively correlated with five other members of the microbiota (Limnobacter, Brevundimonas, Dialister, Barnesiella, and Bilophila), and in the CRAB-N group, it had six negative connections. Thus, the number of negative interactions decreased much more between CRAB-N and CRAB-I than the number of positive interactions.
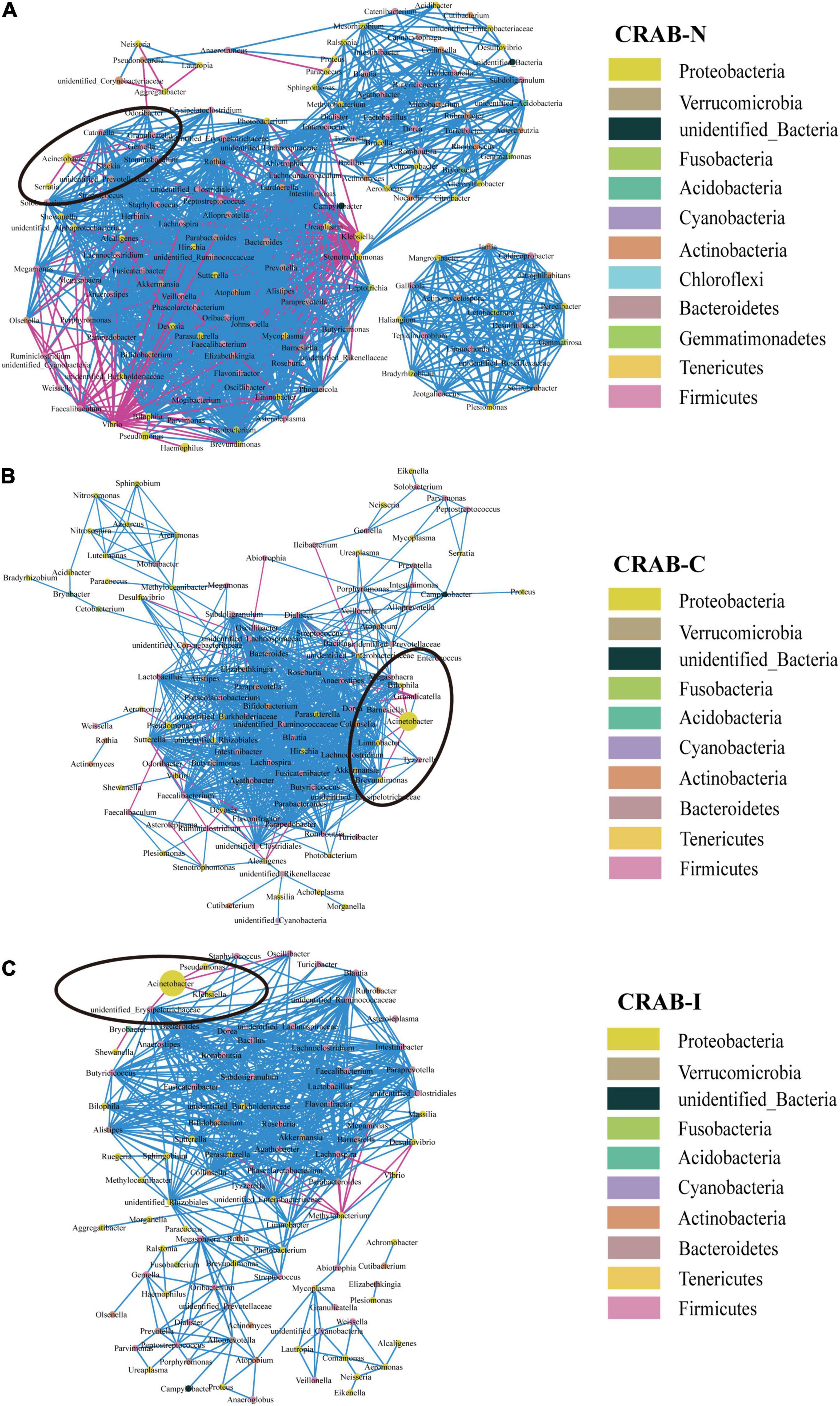
Figure 4. Co-occurring network of microbial communities in LRT samples from CRAB-N (A), CRAB-C (B), and CRAB-I patients (C). A co-occurring network containing strong (ρ > 0.6) and significant (FDR-adjusted P < 0.05) correlations was represented. Each node represents a genusa and the nodes are colored by phylum. The size of each node is proportional to the number of connections. The thickness of each edge is proportional to the ρ. Light blue lines represent negative correlations, and red lines represent positive correlations.
Comparison of Functional Profiles of Microbiota
The Linear Discriminant Analysis Effect Size analysis using a logarithmic LDA score cut-off of 2.5 identified 46 and 55 different pathways in CRAB-C and CRAB-I, respectively, relative to CRAB-N, of which 40 and 45, respectively, increased. These pathways included fatty acid degradation, oxidative phosphorylation, nicotinate and nicotinamide metabolism, transport, porphyrin and chlorophyll metabolism, benzoate degradation, and biofilm formation in Vibrio cholerae (Figures 5A,B). Moreover, signaling proteins (KEGG pathway ko99995, Figure 5C) were more active in CRAB-C than in CRAB-I.
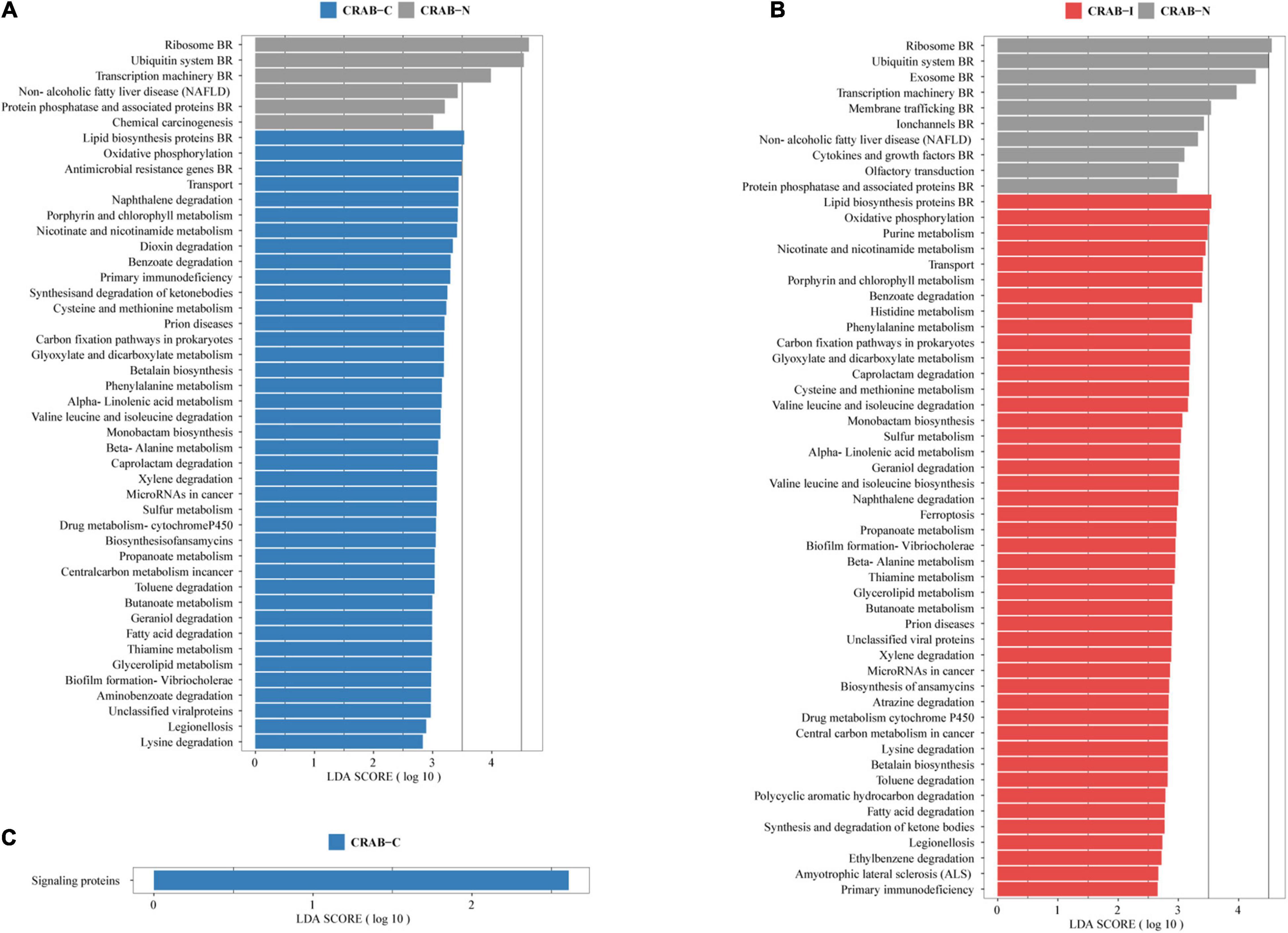
Figure 5. Functionally distinct and LEfSe analysis based on KEGG pathway. (A) Linear discriminant analysis (LDA) scores indicate significant differences in the microbiota between the CRAB-C patients (blue) and CRAB-N controls (gray); (B) LDA scores indicate significant differences in the microbiota between the CRAB-I patients (red) and CRAB-N controls (gray); (C) LDA scores indicate significant differences in the microbiota between the CRAB-C patients (blue) and CRAB-I patients (red). LDA scores > 2.5. CRAB, carbapenem-resistant Acinetobacter baumannii; LRT, lower respiratory tract; VAP, ventilator associated pneumonia; CRAB-N, LRT microbiota of patients with neither VAP nor CRAB LRT colonization; CRAB-C, LRT microbiota of patients with CRAB colonization but without VAP; CRAB-I, LRT microbiota of patients who developed CRAB VAP.
Comparison of Virulence Factors in the Microbiome
In the three groups of patients, we detected a total of 1,628 virulence genes, divided into 69 functional groups. The top 10 most abundant functional virulence groups in each group accounted for 26.5% of all factors, and the composition of the “toxicity” functional group differed between the three groups of patients (Figure 6A). These factors tended to be involved in iron uptake, siderophore biosynthesis, immune evasion, and biofilm formation. Ninety-five virulence genes (relative abundance > 0.1% for each) differed in abundance between CRAB-N and CRAB-C, and 105 differed between CRAB-N and CRAB-I (Supplementary Table 4). Eleven genes, including AB57_ 0984, AB57_ 0990, AB57_ 0992, and mymA, were more abundant in CRAB-I than in CRAB-C (Figure 6B and Supplementary Table 4). Virulence gene networks constructed from CRAB-I had a higher complexity index than those from CRAB-C and CRAB-N patients (56,206.99 vs. 44,722.75 vs. 11,052.12) (Figure 6C). More virulence genes associated with Acinetobacter were detected in CRAB-I than in CRAB-C and CRAB-N, with functions including immune evasion, iron uptake, and VFDB-unclassified (Figure 6D). Consistent with this association, the levels of VFs and the relative abundances of genera (Acinetobacter and Methylorubrum) or species exhibited a strong and significant positive correlation (R2 = 0.529, P = 1.1e-06; R2 = 0.755, P = 7.6e-12, Pearson’s correlation; Figures 7A,B), indicating that differences in the abundance of VFs were driven by differences in the species present in each group of patients.
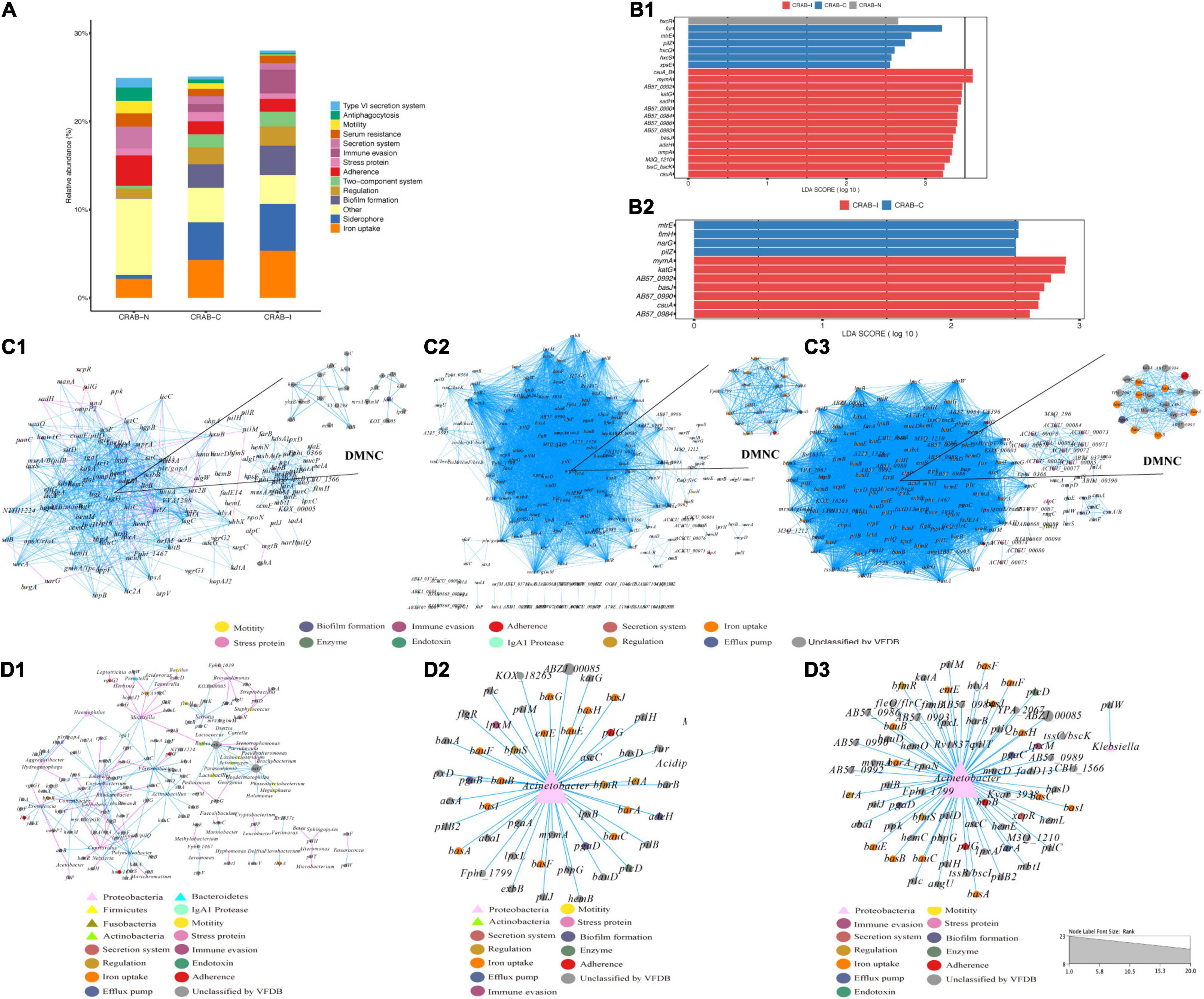
Figure 6. Comparison of virulence factors and Network analysis. (A) Summary of the top 10 relative abundances of function group of virulence genomes in each group; (B) LEfSe and LDA scores indicated significant differences in the virulence genes among groups. (B1) Represented differences among the CRAB-N, CRAB-C, and CRAB-I patients; (B2) Represented differences between the CRAB-C and CRAB-I patients. (C) Co-occurring network of virulence genomes communities in LRT samples from three patient groups (C1: CRAB-N, C2: CRAB-C, C3: CRAB-I). The nodes are colored by the function of virulence genomes, the upper right corner shows DMNC; (D) Co-occurring network of virulence genomes and microbiota communities in LRT samples from three patient groups based on correlation analysis (D1: CRAB-N, D2: CRAB-C, D3: CRAB-I). The nodes are shaped by virulence genomes and bacteria, sized by the relative abundances and colored by the function of virulence genomes or phylum. The connections in the network represent a strong (r > 0.8) and significant (q < 0.05) correlations. The size of each node is proportional to the number of connections. Light blue lines represent positive correlations, and red lines represent negative correlations.
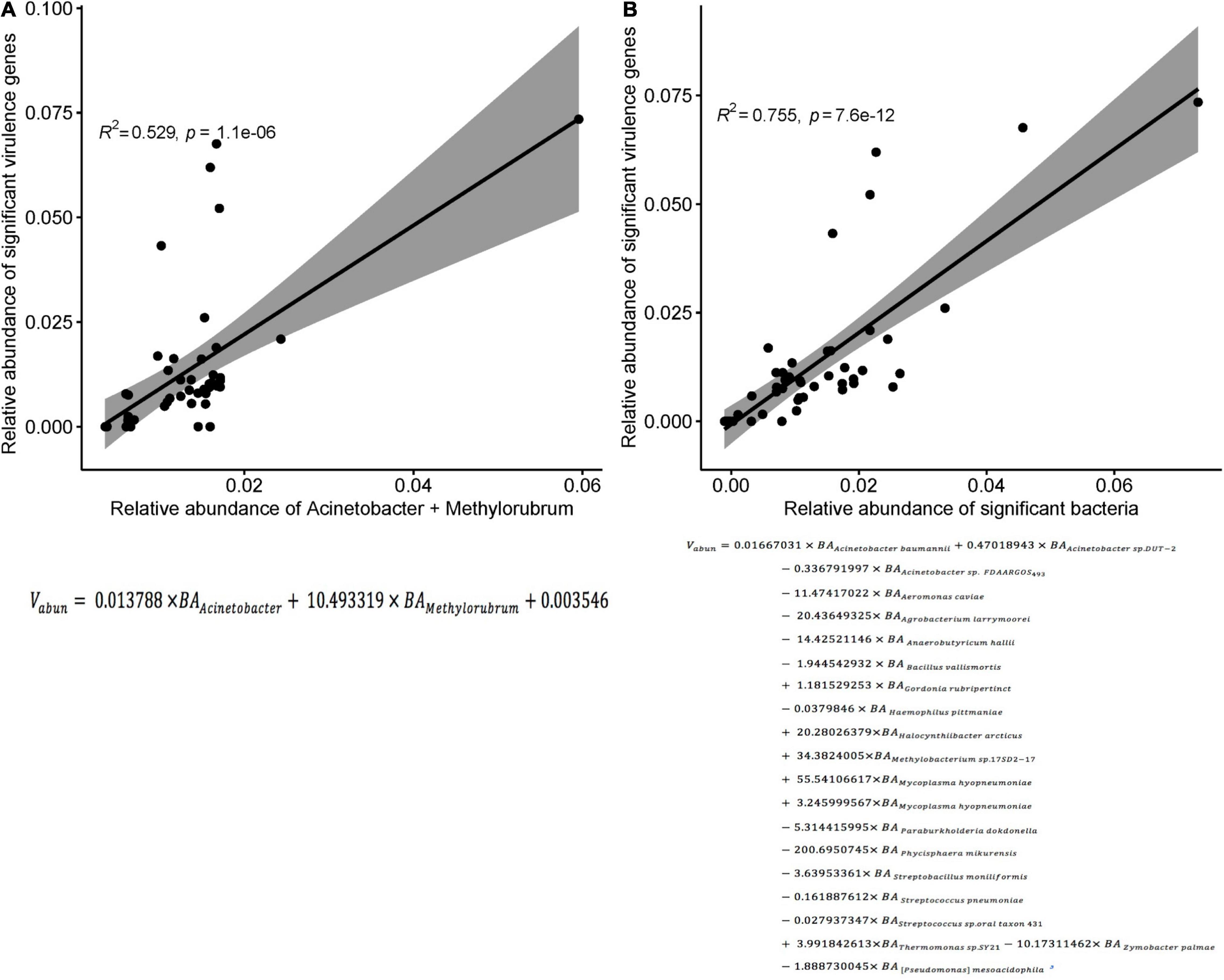
Figure 7. Correlation between the sum of the relative abundances of genus or species and the significant virulence genes density. (A) Correlation between the sum of the relative abundances of Acinetobacter and Methylorubrum and the significant virulence genes density in each sample (R2 = 0.529, p < 0.0001; Pearson’s correlation). (B) Correlation between the sum of the relative abundances of species and the significant virulence genes density in each sample (R2 = 0.755, p < 0.0001; Pearson’s correlation). Vabun, Sum of abundance of significant virulence genes; BAi: abundance of bacteria i.
Whole-Genome Analysis of Carbapenem-Resistant Acinetobacter baumannii
We calculated quality of microbial genomes and found the completeness was 99.85% ± 0.27% and the contamination was 0.96 ± 0.43. The average nucleotide identity (ANI) of the 46 CRAB strains was >95%, indicating that they belonged to the same species (Supplementary Table 1 and Supplementary Figure 6A). MLST of 46 CRAB was dominated by ST208 (30.43%), followed by ST938 (15.22%). eBURST analysis revealed that seven ST types (87.5%) clustered in the same clonal complexes (CCs) (CC92) (Supplementary Figure 6B). The KL types were mainly KL9, KL2, KL93, and KL7, and all strains belonged to the OCL1 type (Figure 8). We observed no statistically significant difference in ST or KL type between the CRAB-I and CRAB-C groups (P = 0.478 and 0.444; respectively).
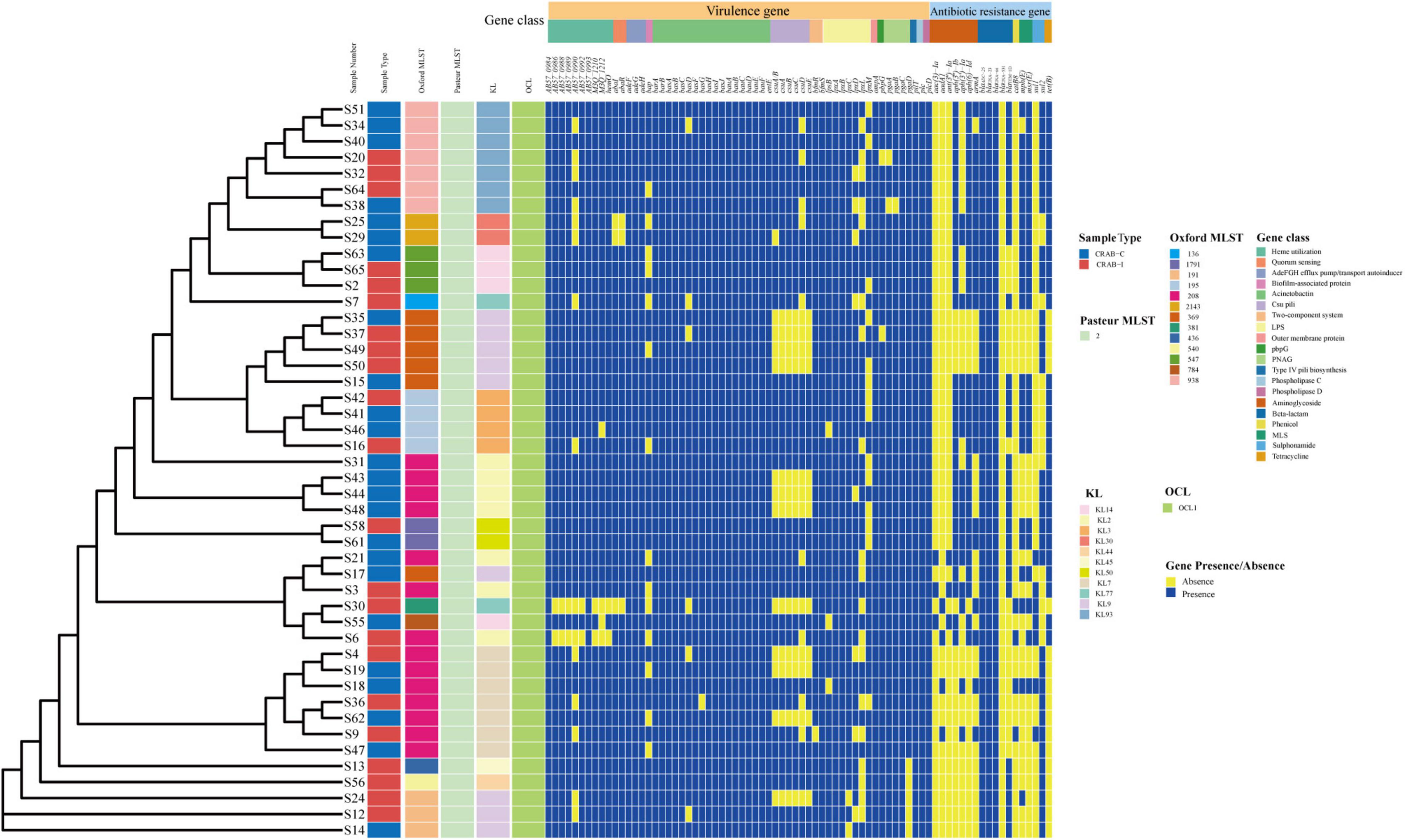
Figure 8. Molecular type, resistant and virulence genes analysis of Acinetobacter baumannii isolates.
All CRAB isolates harbored blaADC–25, blaOXA–23, and blaOXA–66. All isolates harbored more than one oxacillinase gene, and 28 (60.8%) harbored the class A β-lactamase gene blaTEM. The number of resistance genes did not differ significantly between the two groups (Figure 8). Annotation and analysis of virulence genes showed that strains from CRAB-I had more virulence genes than those from CRAB-C (Figure 8), and chi-square tests (Table 1) revealed that AB57_0990, Lpxl, and ABZJ_00085 were more abundant in CRAB-I, consistent with the metagenomics analysis (Figure 6B).

Table 1. The Virulence genes with significant difference between infection and colonization groups of carbapenem-resistant Acinetobacter baumannii.
Discussion
Carbapenem-resistant Acinetobacter baumannii has a high clinical prevalence and is a common pathogen in VAP, but a positive ETA culture alone cannot effectively distinguish between bacterial colonization and infection, representing a longstanding clinical challenge in the management of severely ill patients. Therefore, we investigated the difference in LRT microecology between infected and colonized patients using multi-genomics methods, with the goal of clarifying the clinical management of CRAB infection.
In recent years, several studies have shown that changes in the LRT microbiome are related to the occurrence of lung diseases, but few studies have examined the relationship between respiratory microbiota and infection, and most of those focused on pulmonary tuberculosis and pulmonary fibrosis (Krishna et al., 2016; Emonet et al., 2019). In this study, the 16S rRNA analysis of 52 patients revealed that the α and β diversity of the LRT microbiome was significantly lower in CRAB-I patients than in CRAB-C and CRAB-N patients (Figure 2). The ETA microbiota in the CRAB-N group consisted mainly of Proteobacteria and Haemophilus, consistent with a previous report (Dickson et al., 2017), and was more diverse than in the CRAB-C and CRAB-I groups. The microbiota of the latter two groups were mainly Proteobacteria and Acinetobacter, and the abundance of Acinetobacter in CRAB-I was as high as 76.19% (Figure 3). Further LEfSe analysis (Figure 3H) revealed that, in comparison with CRAB-C patients (who were enriched in unidentified_Corynebacteriacea, Nesseria, Nordella, and Streptococcus), CRAB-I patients had a higher abundance of Acinetobacter. The relative abundance of Acinetobacter increased in the order CRAB-N, CRAB-C, and CRAB-I; this trend was confirmed by Woo et al. (2020). Together, these results indicated that a dynamic evolution of pulmonary microbiota, including a decline in diversity and enrichment of Acinetobacter, occurs prior to the onset of CRAB VAP (Dickson et al., 2016; Zakharkina et al., 2017).
Network analysis revealed that the connections between bacteria were most abundant in CRAB-N, less abundant in CRAB-C, and least abundant in CRAB-I; in parallel, the number of genera negatively associated with Acinetobacter also decreased (6, 5, and 4 negative connections in CRAB-N, CRAB-C, and CRAB-I, respectively). In CRAB-I, only four genera (Klebsiella, Pseudomonas, unidentified_Erysipelotrichaceae, and Oscillibacter) were negatively correlated with Acinetobacter (Figure 4). Zakharkina et al. (2017) found that Acinetobacter, Pseudomonas, Staphylococcus, and Burkholderia were negatively correlated with the development of VAP; Wouter et al. (de Steenhuijsen Piters et al., 2016) found that an increase in the abundance of Lactobacillus and Rothia strains was negatively correlated with the specific microbial infection of VAP patients. These findings suggest that disturbance of the respiratory microbiota relieves negative inhibition of CRAB and is therefore likely to promote infection of the host. However, this idea requires further validation.
Functional metagenomic studies of the respiratory tract microbiome are also valuable for detecting bacterial pathogenesis. Mice infected with Streptococcus pneumoniae and Haemophilus influenzae could cause pulmonary inflammatory responses by activating the MAPK signal pathway (Weiser et al., 2018). In this study, KEGG functional analysis revealed that genes involved in 40 and 45 metabolic pathways (including oxidative phosphorylation, phenylalanine metabolism, fatty acid degradation) were more abundant in the CRAB-C and CRAB-I groups, respectively, than in the CRAB-N group; moreover, signaling protein pathways were more active in CRAB-C patients than in CRAB-I patients (Figure 5). A previous review described how assessment of microbial function using metagenomics, metatranscriptomics, and metabolomics has identified metabolites produced by respiratory microbiota (especially fatty acids, sugars, and amino acids) that can influence host immunity (Budden et al., 2019). This also indicated that the change in bacterial pathogenicity from CRAB-N to CRAB-I may be associated with more active metabolism; this possibility is worthy of further study.
During progression from colonization to infection, bacterial invasiveness and toxicity play a key role. Metagenomics analysis revealed that four major virulence gene clusters (iron uptake, siderophore, immune evasion, and biofilm formation) increased in abundance from the CRAB-N to the CRAB-I group (Figure 6A). The number of virulence genes annotated and networks constructed was significantly higher in the CRAB-I group in the CRAB-C and CRAB-N groups (Figure 6C). Wilcoxon tests showed that the abundance of virulence genes related to heme utilization, such as AB57_ 0984, AB57_ 0990, AB57_ 0992, and mymA, was higher in the CRAB-I group than in the CRAB-C group (Supplementary Table 4 and Figure 6B). AB57_ 0984, a LysR family transcription regulator, is linked to elevated invasiveness (Gebhardt et al., 2020). AB57_ 0990 (a member of the TonB family) and the TonB system play key roles in the pathogenicity of AB (Runci et al., 2019). Network diagram and fitted curve analysis confirmed that these virulence genes were associated with Acinetobacter (Figures 6D, 7). In addition, WGS of strains from CRAB-C and CRAB-I patients revealed that more virulence genes such as Lpxl, ABZJ_00085 and AB57_0990 were present in the infection group (Table 1 and Figure 8). The enrichment in virulence of CRAB indicated that enhancement of bacterial pathogenicity could be another key factor that promotes infection after perturbation of the microbiota.
In terms of patient clinical characteristics, we observed no significant differences in gender, age, or severity of disease at the time of enrolment between the three groups. The number of days of mechanical ventilation before collection was smaller in CRAB-N patients than in CRAB-positive patients, and mortality was higher in CRAB-I patients (Supplementary Table 2). ST208 was the main type (30.43%), followed by ST938 and ST195, and bacterial MLST distribution did not differ significantly between the colonization and infection group (Figure 8). All isolates were highly drug-resistant, and blaoxa–23 was the major determinant of resistance (Fu et al., 2010).
Our results indicate that to draw conclusions about the importance of microbiota evolution, it will be necessary to perform consecutive observations of individual patients, spanning the period from colonization to infection. In future studies, transcriptome and proteome analysis could be used to explore germ–host interactions and pathogenesis.
Conclusion
By combining 16S rRNA amplicon analysis, metagenomics sequencing, and WGS, we characterized the respiratory tract microbiome of patients with CRAB VAP and explored the differences in microbiota between bacterial colonization and infection. Our results revealed that LRT microbiome dysbiosis, including declining diversity, the rise of Acinetobacter to dominance, weakening of the negative regulation of Acinetobacter, and significant enhancement of virulence, could promote the occurrence of infection. Thus, multi-genomics investigational methods could be used to develop new diagnostic measures for CRAB VAP.
Data Availability Statement
The datasets presented in this study can be found in online repositories. The names of the repository/repositories and accession number(s) can be found below: https://www.ncbi.nlm.nih.gov/, PRJNA681291 https://www.ncbi.nlm.nih.gov/, PRJNA679997.
Ethics Statement
The studies involving human participants were reviewed and approved by the ethical board of the Research Ethics Committee of the First Affiliated Hospital of Medicine, Zhejiang University in China (No. 2016-458-1). Written informed consent for participation was not required for this study in accordance with the national legislation and the institutional requirements. Written informed consent was not obtained from the individual(s) for the publication of any potentially identifiable images or data included in this article.
Author Contributions
YX, HZ, and TX designed the study. QG, TX, ML, LG, XY, YL, and CW analyzed and interpreted the data. YZ, YW, SZ, XC, and XK collected the samples. PS, KZ, BZ, QL, and YC oversaw the field projects. QF provided clinical support. TX, QG, and YZ wrote the manuscript. All authors critically reviewed the manuscript and approved the submitted version.
Funding
This study was partially supported by grants from the National Key Research and Development Program of China (2021YFC2300300, 2017YFC1200203, and 2017YFC1200205), National Natural Science Foundation of China (81971984 and 31671366), Key research and development program of Zhejiang province (No. 2021C03068), and Research Project of Jinan Microecological Biomedicine Shandong Laboratory (JNL-2022006B).
Conflict of Interest
The authors declare that the research was conducted in the absence of any commercial or financial relationships that could be construed as a potential conflict of interest.
Publisher’s Note
All claims expressed in this article are solely those of the authors and do not necessarily represent those of their affiliated organizations, or those of the publisher, the editors and the reviewers. Any product that may be evaluated in this article, or claim that may be made by its manufacturer, is not guaranteed or endorsed by the publisher.
Acknowledgments
We thank Jinru Ji, Chaoqun Ying, and Zhiying Liu for providing assistance with bacterial identification, and Juan Hu for providing support for sample collection.
Supplementary Material
The Supplementary Material for this article can be found online at: https://www.frontiersin.org/articles/10.3389/fmicb.2022.782210/full#supplementary-material
Supplementary Material 1 | Comparative respiratory tract microbiome between Carbapenem-resistant Acinetobacter baumannii colonization and ventilator associated pneumonia.
Abbreviations
CRAB, carbapenem-resistant Acinetobacter baumannii; LRT, lower respiratory tract; VAP, ventilator associated pneumonia; CRAB-N, LRT microbiota of patients with neither VAP nor CRAB LRT colonization; CRAB-C, LRT microbiota of patients with CRAB colonization but without VAP; CRAB-I, LRT microbiota of patients who developed CRAB VAP; LefSe, linear discriminant analysis effect size; LDA, linear discriminant analysis; DMNC, density of maximum neighborhood component; VFs, virulence factors.
References
Bokulich, N. A., Subramanian, S., Faith, J. J., Gevers, D., Gordon, J. I., Knight, R., et al. (2013). Quality-filtering vastly improves diversity estimates from Illumina amplicon sequencing. Nat. Methods 10, 57–59. doi: 10.1038/nmeth.2276
Buchfink, B., Xie, C., and Huson, D. H. (2015). Fast and sensitive protein alignment using DIAMOND. Nat. Methods 12, 59–60. doi: 10.1038/nmeth.3176
Budden, K. F., Shukla, S. D., Rehman, S. F., Bowerman, K. L., Keely, S., Hugenholtz, P., et al. (2019). Functional effects of the microbiota in chronic respiratory disease. Lancet Respir. Med. 7, 907–920. doi: 10.1016/S2213-2600(18)30510-1
Caporaso, J. G., Kuczynski, J., Stombaugh, J., Bittinger, K., Bushman, F. D., and Costello, E. K. (2010). QIIME allows analysis of high-throughput community sequencing data. Nat. Methods 7, 335–336. doi: 10.1038/nmeth.f.303
Chen, L. H., Zheng, D., Liu, B., Yang, J., and Jin, Q. (2016). VFDB 2016: hierarchical and refined dataset for big data analysis–10 years on. Nucleic Acids Res. 44, D694–D697. doi: 10.1093/nar/gkv1239
CLSI (2018). Performance Standards for Antimicrobial Susceptibility Testing. CLSI Approved Standard M100-S15. Wayne, IL: Clinical and Laboratory Standards Institute.
Consales, G., Gramigni, E., Zamidei, L., Bettocchi, D., and De Gaudio, A. R. (2011). A multidrug-resistant Acinetobacter baumannii outbreak in intensive care unit: antimicrobial and organizational strategies. J. Crit. Care 26, 453–459. doi: 10.1016/j.jcrc.2010.12.016
Csardi, G., and Nepusz, T. (2006). The Igraph software package for complex network research. Inter. J. Complex Syst. 1695, 1–9. doi: 10.1186/1471-2105-12-455
de Steenhuijsen Piters, W. A., Huijskens, E. G., Wyllie, A. L., Biesbroek, G., van den Bergh, M. R., Veenhoven, R. H., et al. (2016). Dysbiosis of upper respiratory tract microbiota in elderly pneumonia patients. ISME J. 10, 97–108. doi: 10.1038/ismej.2015.99
Dickson, R. P., Erb-Downward, J. R., Freeman, C. M., McCloskey, L., Falkowski, N. R., Huffnagle, G. B., et al. (2017). Bacterial topography of the healthy human lower respiratory tract. mBio. 8:14. doi: 10.1128/mBio.02287-16
Dickson, R. P., Erb-Downward, J. R., and Huffnagle, G. B. (2014). Towards an ecology of the lung: new conceptual models of pulmonary microbiology and pneumonia pathogenesis. Lancet Respir. Med. 2, 238–246. doi: 10.1016/S2213-2600(14)70028-1
Dickson, R. P., Singer, B. H., Newstead, M. W., Falkowski, N. R., Erb-Downward, J. R., Standiford, T. J., et al. (2016). Enrichment of the lung microbiome with gut bacteria in sepsis and the acute respiratory distress syndrome. Nat. Microbiol. 1:16113. doi: 10.1038/nmicrobiol.2016.113
Edgar, R. C. (2004). MUSCLE: a multiple sequence alignment method with reduced time and space complexity. BMC Bioinformatics 5:113. doi: 10.1186/1471-2105-5-113
Edgar, R. C., Haas, B. J., Clemente, J. C., Quince, C., and Knight, R. (2011). UCHIME improves sensitivity and speed of chimera detection. Bioinformatics 27, 2194–2200. doi: 10.1093/bioinformatics/btr381
Emonet, S., Lazarevic, V., Leemann Refondini, C., Gaïa, N., Leo, S., Girard, M., et al. (2019). Identification of respiratory microbiota markers in ventilator-associated pneumonia. Intensive Care Med. 45, 1082–1092. doi: 10.1007/s00134-019-05660-8
European Committee on Antimicrobial Susceptibility testing (2016). Recommendations for MIC Determination of Colistin (polymyxin E) as Recommended by the Joint CLSI-EUCAST Polymyxin Breakpoints Working Group. Växjö: European Committee on Antimicrobial Susceptibility testing.
Faner, R., Sibila, O., Agustí, A., Bernasconi, E., Chalmers, J. D., Huffnagle, G. B., et al. (2017). The microbiome in respiratory medicine: current challenges and future perspectives. Eur. Respir. J. 49:1602086. doi: 10.1183/13993003.02086-2016
Fu, Y., Zhou, J., Zhou, H., Yang, Q., Wei, Z., Yu, Y., et al. (2010). Wide dissemination of OXA-23-producing carbapenem-resistant Acinetobacter baumannii clonal complex 22 in multiple cities of China. J. Antimicrob. Chemother. 65, 644–650. doi: 10.1093/jac/dkq027
Gaiarsa, S., Batisti Biffignandi, G., Esposito, E. P., Castelli, M., Jolley, K. A., Brisse, S., et al. (2019). Comparative Analysis of the Two Acinetobacter baumannii Multilocus Sequence Typing (MLST) Schemes. Front. Microbiol. 10:930. doi: 10.3389/fmicb.2019.00930
Gebhardt, M. J., Czyz, D. M., Singh, S., Zurawski, D. V., Becker, L., and Shuman, H. A. (2020). GigC, a LysR family transcription regulator, is required for cysteine metabolism and virulence in Acinetobacter baumannii. Infect. Immun. 89, e00180–20. doi: 10.1128/IAI.00180-20
Hakansson, A. P., Orihuela, C. J., and Bogaert, D. (2018). Bacterial-host interactions: physiology and pathophysiology of respiratory infection. Physiol. Rev. 98, 781–811. doi: 10.1152/physrev.00040.2016
Hall, B. G. (2013). Building phylogenetic trees from molecular data with MEGA. Mol. Biol. Evol. 30, 1229–1235. doi: 10.1093/molbev/mst012
Harding, C. M., Hennon, S. W., and Feldman, M. F. (2018). Uncovering the mechanisms of Acinetobacter baumannii virulence. Nat. Rev. Microbiol. 16, 91–102.
Horan, T. C., Andrus, M., and Dudeck, M. A. (2008). CDC/NHSN surveillance definition of health care-associated infection and criteria for specific types of infections in the acute care setting. Am. J. Infect. Control. 36, 309–332. doi: 10.1016/j.ajic.2008.03.002
Hu, F. P., Guo, Y., Zhu, D. M., Wang, F., Jiang, X. F., Xu, Y. C., et al. (2016). Resistance trends among clinical isolates in China reported from CHINET surveillance of bacterial resistance, 2005-2014. Clin. Microbiol. Infect. 22(Suppl. 1) S9–S14. doi: 10.1016/j.cmi.2016.01.001
Huang, Y., Jiao, Y., Zhang, J., Xu, J., Cheng, Q., Li, Y., et al. (2018). Infection assembly of Shanghai Respiratory Society. Microbial etiology and prognostic factors of ventilator-associated pneumonia: a multicenter retrospective study in Shanghai. Clin. Infect. Dis. 67(Suppl. 2) S146–S152. doi: 10.1093/cid/ciy686
Jain, C., Rodriguez, R. L. M., Phillippy, A. M., Konstantinidis, K. T., and Aluru, S. (2018). High throughput ANI analysis of 90K prokaryotic genomes reveals clear species boundaries. Nat. Commun. 9:5114.
Kanehisa, M., Furumichi, M., Tanabe, M., Sato, Y., and Morishima, K. (2017). KEGG: new perspectives on genomes, pathways, diseases and drugs. Nucleic Acids Res. 45, D353–D361. doi: 10.1093/nar/gkw1092
Kelly, B. J., Imai, I., Bittinger, K., Laughlin, A., Fuchs, B. D., Bushman, F. D., et al. (2016). Composition and dynamics of the respiratory tract microbiome in intubated patients. Microbiome 4:7. doi: 10.1186/s40168-016-0151-8
Krishna, P., Jain, A., and Bisen, P. S. (2016). Microbiome diversity in the sputum of patients with pulmonary tuberculosis. Eur. J. Clin. Microbiol. Infect. Dis. 35, 1205–1210. doi: 10.1007/s10096-016-2654-4
LeGall, J. R., Loirat, P., and Alpérovitch, A. (1986). APACHE II–a severity of disease classification system. Crit. Care Med. 14, 754–755. doi: 10.1097/00003246-198608000-00027
Magoč, T., and Salzberg, S. L. (2011). FLASH: fast length adjustment of short reads to improve genome assemblies. Bioinformatics 27, 2957–2963. doi: 10.1093/bioinformatics/btr507
Menzel, P., Ng, K. L., and Krogh, A. (2016). Fast and sensitive taxonomic classification for metagenomics with Kaiju. Nat. Commun. 7:11257. doi: 10.1038/ncomms11257
Nurk, S., Meleshko, D., Korobeynikov, A., and Pevzner, P. A. (2017). metaSPAdes: a new versatile metagenomic assembler. Genome Res. 27, 824–834. doi: 10.1101/gr.213959.116
Page, A. J., Cummins, C. A., Hunt, M., Wong, V. K., Reuter, S., Holden, M. T., et al. (2015). Roary: rapid large-scale prokaryote pan genome analysis. Bioinformatics 31, 3691–3693. doi: 10.1093/bioinformatics/btv421
Papazian, L., Klompas, M., and Luyt, C. E. (2020). Ventilator-associated pneumonia in adults: a narrative review. Intensive Care Med. 46, 888–906. doi: 10.1007/s00134-020-05980-0
Parks, D. H., Imelfort, M., Skennerton, C. T., Hugenholtz, P., and Tyson, G. W. (2015). CheckM: assessing the quality of microbial genomes recovered from isolates, single cells, and metagenomes. Genome Res. 25, 1043–1055. doi: 10.1101/gr.186072.114
Price, M. N., Dehal, P. S., and Arkin, A. P. (2010). FastTree 2–approximately maximum-likelihood trees for large alignments. PLoS One 5:e9490. doi: 10.1371/journal.pone.0009490
Quast, C., Pruesse, E., Yilmaz, P., Gerken, J., Schweer, T., Yarza, P., et al. (2013). The SILVA ribosomal RNA gene database project: improved data processing and web-based tools. Nucleic Acids Res. 41, D590–D596. doi: 10.1093/nar/gks1219
Roquilly, A., Torres, A., Villadangos, J. A., Netea, M. G., Dickson, R., Becher, B., et al. (2019). Pathophysiological role of respiratory dysbiosis in hospital-acquired pneumonia. Lancet Respir. Med. 7, 710–720. doi: 10.1016/S2213-2600(19)30140-7
Runci, F., Gentile, V., Frangipani, E., Rampioni, G., Leoni, L., Lucidi, M., et al. (2019). Contribution of active iron uptake to Acinetobacter baumannii pathogenicity. Infect. Immun. 87, e00755–18. doi: 10.1128/IAI.00755-18
Seemann, T. (2017). Abricate. Available online at: https://github.com/tseemann/abricate. (accessed February 11, 2018).
Segata, N., Izard, J., Waldron, L., Gevers, D., Miropolsky, L., Garrett, W. S., et al. (2011). Metagenomic biomarker discovery and explanation. Genome Biol. 12:R60. doi: 10.1186/gb-2011-12-6-r60
Shamsizadeh, Z., Nikaeen, M., Nasr, E. B., Mirhoseini, S. H., Hatamzadeh, M., and Hassanzadeh, A. (2017). Detection of antibiotic resistant Acinetobacter baumannii in various hospital environments: potential sources for transmission of Acinetobacter infections. Environ. Health Prev. Med. 22:44. doi: 10.1186/s12199-017-0653-4
Tatusova, T., DiCuccio, M., Badretdin, A., Chetvernin, V., Nawrocki, E. P., Zaslavsky, L., et al. (2016). NCBI prokaryotic genome annotation pipeline. Nucleic Acids Res. 44, 6614–6624.
Truong, D. T., Tett, A., Pasolli, E., Huttenhower, C., and Segata, N. (2017). Microbial strain-level population structure and genetic diversity from metagenomes. Genome Res. 27, 626–638. doi: 10.1101/gr.216242.116
Uppalapati, S. R., Sett, A., and Pathania, R. (2020). The outer membrane proteins OmpA, CarO, and OprD of Acinetobacter baumannii confer a two-pronged defense in facilitating its success as a potent human pathogen. Front. Microbiol. 11:589234. doi: 10.3389/fmicb.2020.589234
Weiser, J. N., Ferreira, D. M., and Paton, J. C. (2018). Streptococcus pneumoniae: transmission, colonization and invasion. Nat. Rev. Microbiol. 16, 355–367. doi: 10.1038/s41579-018-0001-8
WHO (2017). Publishes List of Bacteria for Which new Antibiotics are Urgently Needed. Available online: https://www.who.int/news-room/detail/27-02-2017-who-publishes-list-of-bacteria-for-which-new-antibiotics-are-urgently-needed (accessed August 01, 2020).
Woo, S., Park, S. Y., Kim, Y., Jeon, J. P., Lee, J. J., and Hong, J. Y. (2020). The dynamics of respiratory microbiota during mechanical ventilation in patients with pneumonia. J. Clin. Med. 9:638. doi: 10.3390/jcm9030638
Wyres, K. L., Cahill, S. M., Holt, K. E., Hall, R. M., and Kenyon, J. J. (2020). Identification of Acinetobacter baumannii loci for capsular polysaccharide (KL) and lipooligosaccharide outer core (OCL) synthesis in genome assemblies using curated reference databases compatible with Kaptive. Microb. Genom. 6:e000339. doi: 10.1099/mgen.0.000339
Xie, J., Yang, Y., Huang, Y., Kang, Y., Xu, Y., Ma, X., et al. (2018). The current epidemiological landscape of ventilator-associated pneumonia in the intensive care unit: a multicenter prospective observational study in China. Clin Infect. Dis. 67(Suppl. 2) S153–S161. doi: 10.1093/cid/ciy692
Yin, Y., Zhao, C., Li, H., Jin, L., Wang, Q., Wang, R., et al. (2021). Clinical and microbiological characteristics of adults with hospital-acquired pneumonia: a 10-year prospective observational study in China. Eur. J. Clin. Microbiol. Infect. Dis. 40, 683–690. doi: 10.1007/s10096-020-04046-9
Zakharkina, T., Martin-Loeches, I., Matamoros, S., Povoa, P., Torres, A., Kastelijn, J. B., et al. (2017). The dynamics of the pulmonary microbiome during mechanical ventilation in the intensive care unit and the association with occurrence of pneumonia. Thorax 72, 803–810. doi: 10.1136/thoraxjnl-2016-209158
Keywords: carbapenem-resistant Acinetobacter baumannii (CRAB), ventilator-associated pneumonia (VAP), multi-genomics analysis, microbiome, virulence gene
Citation: Xiao T, Guo Q, Zhou Y, Shen P, Wang Y, Fang Q, Li M, Zhang S, Guo L, Yu X, Liao Y, Wang C, Chi X, Kong X, Zhou K, Zheng B, Luo Q, Chen Y, Zhu H and Xiao Y (2022) Comparative Respiratory Tract Microbiome Between Carbapenem-Resistant Acinetobacter baumannii Colonization and Ventilator Associated Pneumonia. Front. Microbiol. 13:782210. doi: 10.3389/fmicb.2022.782210
Received: 24 September 2021; Accepted: 28 January 2022;
Published: 04 March 2022.
Edited by:
Iraj Rasooli, Shahed University, IranReviewed by:
Prashant P. Patil, National Institute of Allergy and Infectious Diseases, National Institutes of Health (NIH), United StatesHuiluo Cao, The University of Hong Kong, Hong Kong SAR, China
Andreza Martins, Federal University of Rio Grande do Sul, Brazil
Copyright © 2022 Xiao, Guo, Zhou, Shen, Wang, Fang, Li, Zhang, Guo, Yu, Liao, Wang, Chi, Kong, Zhou, Zheng, Luo, Chen, Zhu and Xiao. This is an open-access article distributed under the terms of the Creative Commons Attribution License (CC BY). The use, distribution or reproduction in other forums is permitted, provided the original author(s) and the copyright owner(s) are credited and that the original publication in this journal is cited, in accordance with accepted academic practice. No use, distribution or reproduction is permitted which does not comply with these terms.
*Correspondence: Huaiqiu Zhu, aHF6aHVAcGt1LmVkdS5jbg==; Yonghong Xiao, eGlhb3lvbmdob25nQHpqdS5lZHUuY24=
†These authors have contributed equally to this work